What Is the Role of Thalamostriatal Circuits in Learning Vocal Sequences?
- Department of Neuroscience, UT Southwestern Medical Center, Dallas, TX, United States
Basal ganglia (BG) circuits integrate sensory and motor-related information from the cortex, thalamus, and midbrain to guide learning and production of motor sequences. Birdsong, like speech, is comprised of precisely sequenced vocal elements. Learning song sequences during development relies on Area X, a vocalization related region in the medial striatum of the songbird BG. Area X receives inputs from cortical-like pallial song circuits and midbrain dopaminergic circuits and sends projections to the thalamus. It has recently been shown that thalamic circuits also send substantial projections back to Area X. Here, we outline a gated-reinforcement learning model for how Area X may use signals conveyed by thalamostriatal inputs to direct song learning. Integrating conceptual advances from recent mammalian and songbird literature, we hypothesize that thalamostriatal pathways convey signals linked to song syllable onsets and offsets and influence striatal circuit plasticity via regulation of cholinergic interneurons (ChIs). We suggest that syllable sequence associated vocal-motor information from the thalamus drive precisely timed pauses in ChIs activity in Area X. When integrated with concurrent corticostriatal and dopaminergic input, this circuit helps regulate plasticity on medium spiny neurons (MSNs) and the learning of syllable sequences. We discuss new approaches that can be applied to test core ideas of this model and how associated insights may provide a framework for understanding the function of BG circuits in learning motor sequences.
Introduction
The ability to adeptly sequence motor actions is central to animal survival, coordinated movement, and communication. Basal ganglia-thalamocortical loops have been demonstrated to be essential for the learning, coordination, and execution of sequenced motor actions (Jin et al., 2014; Tecuapetla et al., 2016; Park et al., 2020). The precise movements and sequencing of actions involved in producing learned vocalizations are one of the clearest and most readily addressable natural behaviors that can be used to examine how motor sequences are learned and controlled by the brain (Brainard and Doupe, 2002; Fee et al., 2004). Two major glutamatergic inputs to the striatum, the largest principal component of the basal ganglia, have been proposed to drive striatal activity that regulates vocal sequences: the corticostriatal and the thalamostriatal inputs (Kemp et al., 1971; Gerfen and Wilson, 1996; Smith et al., 2004; Figure 1A).
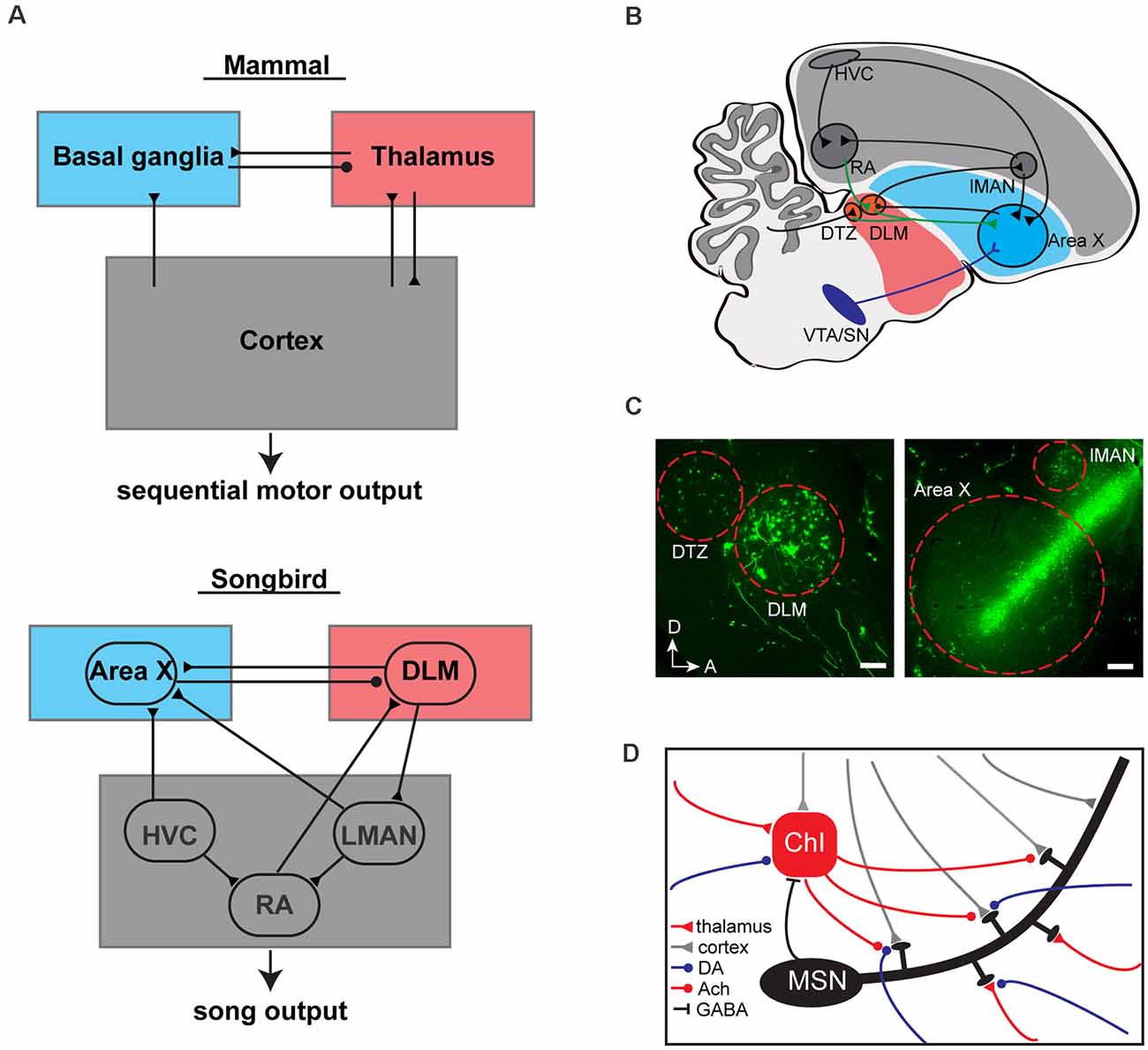
Figure 1. Basal ganglia (BG)-thalamocortical loops in mammals and songbirds. (A) BG-thalamocortical loops are evolutionarily conserved in songbirds and mammals to drive sequential motor (or song) output. Circles and triangles represent GABAergic and glutamatergic inputs, respectively. (B) Schematic of the BG-thalamocortical loops in songbirds. Area X receives multiple inputs from song circuits: glutamatergic inputs from cortical like song circuits HVC and LMAN; glutamatergic inputs from thalamic circuits DLM and DTZ; dopaminergic inputs from midbrain circuits VTA and SN. Circles, triangles, and Y-shaped projections represent GABAergic, glutamatergic, and dopaminergic inputs, respectively. (C) Representative sagittal sections through the thalamus (left panel) and Area X (right panel) when fluorescent tracers were injected into Area X (dextran, Alexa Fluor 488) of adult zebra finch. Left panel, parasagittal section through retrogradely labeled thalamic circuits DLM and DTZ, scale bar, 100 μm. Right panel, parasagittal section through retrogradely labeled cortical circuit LMAN, scale bar, 200 μm. D, dorsal; R, rostral. (D) Schematic of striatal microcircuits in mammals. The main glutamatergic inputs to the striatum are from the cortex (gray triangles) and thalamus (red triangles). Both inputs target overlapping populations of MSNs as well as ChIs and other interneurons (not shown). Modulatory inputs from dopaminergic (DA, blue circles) and cholinergic inputs from ChIs (Ach, red circles) are involved in modulating the synapses onto MSNs. MSNs provide GABAergic inputs to ChIs (black line) and other interneurons (not shown). ChI, cholinergic interneuron. MSNs, medium spiny neurons.
In contrast to the well-recognized functions of corticostriatal input in sequential behaviors (Hikosaka et al., 1999; Rothwell et al., 2015; Kupferschmidt et al., 2017), it is less clear whether and how the thalamostriatal input is involved in the motor learning and performance, especially when the sequences of motor output are fast and complex (Parker et al., 2016; Díaz-Hernández et al., 2018). An increasing body of literature suggests that cortical circuits are necessary for initial learning of motor sequence and, once learned, subcortical circuits may be sufficient for their expression (Kawai et al., 2015; Kupferschmidt et al., 2017; Wolff et al., 2019). Yet, how signals conveyed by distinct cortical and/or subcortical inputs to the striatum are integrated during learning and potentially decoupled once expert performance is achieved is still unclear. For example, how is time-sensitive information incorporated in the striatum from cortical and subcortical inputs? How do these pathways regulate synaptic plasticity during learning and once motor sequences are learned? Do thalamostriatal and corticostriatal circuits convey redundant, complementary, or distinct information to the striatum?
Many experimental paradigms have been developed in rodents to tackle these and related questions (Kawai et al., 2015; Rueda-Orozco and Robbe, 2015; Díaz-Hernández et al., 2018; Hidalgo-Balbuena et al., 2019). However, a coherent hypothesis with testable predictions for the role of thalamostriatal circuits in learning and performance of sequential behaviors has been slow to develop. The recent identification of thalamostriatal circuits in the songbird presents an opportunity to use song learning to generate hypotheses and predictions for thalamostriatal circuit function (Nicholson et al., 2018; Pidoux et al., 2018). Songbirds learn a sequence of vocal elements (song) from a vocal model (tutor) as juveniles and use this song in adulthood to attract mates and defend territory (Zann, 1996; Searcy and Beecher, 2009; Bradbury and Vehrencamp, 2011; Ikeda et al., 2020). The song is learned naturally during development. Therefore, the learning process is free from external reinforcers typically used in laboratory settings, such as food or water, which are known to impact dopaminergic reward circuits (Arbuthnott and Wickens, 2007; Moss and Bolam, 2008; Rice and Cragg, 2008). In addition, the song is highly quantifiable and trackable. Male zebra finches, for example, produce thousands of highly stereotyped song renditions daily which, when combined with neural recordings and/or circuit manipulations, permit detailed study of how brain circuits control behavioral learning and performance. Lastly, the neural circuits underlying song learning and production are well characterized and cellular homologies between song circuits and mammalian circuits are starting to be revealed (Farries, 2004; Pfenning et al., 2014; Gadagkar et al., 2016; Vallentin et al., 2016; Hisey et al., 2018; Xiao et al., 2018; Colquitt et al., 2021; Xiao et al., 2021).
From this perspective, we discuss the potential roles of the thalamostriatal pathway in vocal learning, propose a testable hypothesis invoking recent breakthroughs in rodents and songbirds, and discuss new methodologies that can be applied to test core ideas in our model. Rather than a detailed review of the state of the field, we attempt to provide a new avenue for understanding the function of BG circuitry in directing sequential behaviors.
Role of Inputs to Area X in Vocal Learning and Production
Area X is a specialized song nucleus within the striatum that receives inputs from cortical-like pallial song circuits [HVC (used as a proper name, formerly known as high vocal center) and LMAN (lateral part of the magnocellular nucleus of anterior neostriatum)] and dopaminergic input from midbrain circuits [VTA (ventral tegmental area) and SN (substantia nigra)]. Area X sends projections to the thalamic nucleus DLM (dorsolateral nucleus of the anterior thalamus) via pallidal-like cells and DLM, in turn, sends projections to the pallial song nucleus LMAN. Area X, DLM, and LMAN constitute the anterior forebrain pathway (AFP), a basal ganglia-thalamocortical loop associated with learning and sequencing of birdsong (Figure 1B). Both cortical input from HVC and midbrain input from VTA/SN in Area X are needed for song learning in juvenile birds but not needed for continued production of learned song in adulthood (Scharff et al., 2000; Miller et al., 2015; Hisey et al., 2018; Sánchez-Valpuesta et al., 2019). Ablation of either input in juvenile birds causes deficits in the imitation of tutor song, resulting in a less stereotyped acoustic features and sequencing of syllables. In contrast, individual lesions of either HVC neurons projecting to Area X or dopaminergic inputs in adult birds have little impact on the overall structure of the learned song. Nonetheless, lesions of dopaminergic inputs to Area X have been shown to disrupt the ability to modify song acoustic features in response to disruptive auditory feedback (Hoffmann et al., 2016; Saravanan et al., 2019). Overall, these observations align with findings in rodents indicating that corticostriatal pathways are necessary for initial learning but can be dispensable for performance once motor sequences are well learned (Tecuapetla et al., 2016; Kupferschmidt et al., 2017).
In contrast to a diminished role of the corticostriatal pathway in the performance of learned motor sequences, thalamostriatal inputs may be necessary for both learning and subsequent expert performance of motor sequences. Ablation of thalamic inputs to the dorsolateral striatum (DLS) prevents naïve rats from learning a new motor sequence and revert the performance of motor sequences in expert rats to levels similar to those observed in the early phases of learning (Hidalgo-Balbuena et al., 2019; Wolff et al., 2019). These results argue that thalamostriatal projections may be relevant in driving the dorsal striatum and necessary to perform a sequence of learned motor actions.
Are there thalamic projections to Area X? Tracer injections in Area X retrogradely label neurons in DLM and adjacent thalamic nuclei (Bottjer et al., 1989; Castelino et al., 2007; Person et al., 2008; Gale and Perkel, 2010; Pidoux et al., 2018; Figure 1C). However, retrograde labeling in the thalamus could result from tracer efflux into pallial regions immediately dorsal to Area X, including LMAN, which are known to receive inputs from the thalamus. Thus, the validity of thalamicalamic projections to Area X has until recently been controversial. Viral vector labeling of presynaptic axon terminals from DLM and DTZ (dorsal thalamic zone) has now been used to confirm that DLM and DTZ do indeed provide direct projections to Area X (Nicholson et al., 2018).
These two thalamostriatal projections, from DLM and DTZ, likely carry different types of information to Area X. In addition to reciprocal projections with Area X, DLM receives excitatory input from the motor cortical-like song circuit RA (robust nucleus of the arcopallium), which transmits premotor signals necessary for song learning (Wild, 1993; Vates et al., 1997; Luo and Perkel, 2002; Goldberg et al., 2012; Goldberg and Fee, 2012). DTZ, on the other hand, receives input from deep cerebellar nuclei (Person et al., 2008; Pidoux et al., 2018). Although much remains to be learned about the function of these two thalamostriatal pathways in song production and song learning, current evidence indicates that they may each play important roles in song learning and song motor control. Lesions of DLM in adult birds disrupt AFP-driven song initiation and lesions in juvenile birds disrupt early stages of vocal production (Goldberg and Fee, 2011; Chen et al., 2014). Much less is currently known about DTZ, but lesions of deep cerebellar nuclei have also been shown to disrupt song imitation in young birds (Pidoux et al., 2018). For the remainder of this perspective, we will focus on the potential roles of the DLM-Area X circuit in vocal learning.
What Signal is Encoded by Thalamostriatal Pathways in Songbirds?
Song syllables are the essential behavioral units of a song. For example, song truncation following a startling stimulus tends to occur at the end of individual syllables and not mid-syllable, suggesting syllable-level chunking of motor programs (Cynx, 1990). Songbirds learn to arrange syllables into sequences independently from learning the spectral content of each individual syllable, supporting the idea that syllables represent a meaningful behavioral unit (Tchernichovski et al., 2001; Liu et al., 2004; Ravbar et al., 2012; Lipkind et al., 2013, 2017). Moreover, Area X appears to be an important contributor to learning and controlling song syllable syntax. Lesions of Area X in juvenile birds result in birds with variable song syntax as adults (Sohrabji et al., 1990; Scharff and Nottebohm, 1991; Goldberg and Fee, 2011). Knockdown of the speech-linked gene FoxP2, overexpression of the mutant gene fragment that causes Huntington’s disease, and optogenetic excitation of dopaminergic inputs in Area X all cause progressive disruptions in song syllable sequencing, including repetition of song syllables and disruptions in song syntax in adult birds (Tanaka et al., 2016; Xiao et al., 2021).
Nonetheless, reinforcement-based models for song learning largely focus on learning features of song syllables rather than syllable sequences (Fee and Goldberg, 2011; Fee, 2012; Chen and Goldberg, 2020; Kornfeld et al., 2020). Therefore, from a circuit perspective, it is not clear how chunked motor programs associated with syllable level representations might be acquired during development. It has been proposed that temporal information associated with sequential events could emerge from the interaction of cortical and thalamic inputs to the BG (Mello et al., 2015; Paton and Lau, 2015). Yet, the neural signals used to encode information at different time scales are not known. In vitro electrophysiological recordings in mammals suggest that corticostriatal and thalamostriatal pathways encode information in temporally distinct ways (e.g., low vs. high release probability; synapses were facilitated vs. depressed by repetitive stimulation) and therefore constrain how they inform striatal circuits (Ding et al., 2008). More recently, in vivo work characterizing the sound-evoked responses of thalamostriatal and corticostriatal neurons further suggests that these pathways can convey different yet complementary auditory information to the striatum (Ponvert and Jaramillo, 2019). Although both pathways encode sound frequency information, corticostriatal inputs provide a more accurate representation of amplitude modulation rate and thalamostriatal inputs convey information about the precise timing of acoustic events. We propose that Area X receives three distinct streams of information about a song: a detailed timestamp for each moment in the song from HVC, a signal about the variability of spectral content at each moment from LMAN, and information about syllable onsets and offsets that permits syllable-level chunking of behavior from DLM. Together, these three inputs may provide essential substrates to support the learning of spectral features in syllables as well as syllable sequences.
During singing, Area X neurons appear to encode information associated with the timing of specific moments in the song. Individual medium spiny neurons (MSNs) in Area X exhibit sparse activity (1–4 bursts/motif) that is precisely time-locked to particular points in song (Farries and Perkel, 2002; Goldberg et al., 2010; Goldberg and Fee, 2011; Woolley et al., 2014). The distribution of activity across the population of MSNs is thought to cover the entire sequence of song syllables in a bird’s song motif (Farries and Perkel, 2002; Goldberg et al., 2010; Fee and Goldberg, 2011; Woolley et al., 2014). This activity pattern may reflect, at least in part, excitatory input from HVC neurons projecting to Area X (HVCx neurons), which also exhibit sparse time-locked activity during song production (1–5 bursts/motif; Kozhevnikov and Fee, 2007; Goldberg et al., 2010; Fee and Goldberg, 2011; Woolley et al., 2014). In contrast to the precise temporal code of HVCx neurons, LMANx neurons exhibit more variable patterns of activity from song to song, despite a slight tendency to burst at particular points in song (Hessler and Doupe, 1999; Leonardo, 2004; Fee and Goldberg, 2011). While both HVCx and LMANx are thought to transmit efferent copies of premotor signals impinging on RA, HCVx is a likely source of the detailed timestamp for each moment in song in Area X, while LMANx is a likely source for information reflecting the variability of spectral features at each moment in song (Nixdorf-Bergweiler et al., 1995; Vates et al., 1997; Kozhevnikov and Fee, 2007; Prather et al., 2008; Fee and Goldberg, 2011).
Distinct from the temporal information transmitted from HVCx, we hypothesize that DLM conveys information about syllable onset/offset to Area X. DLM neurons appear to be strongly modulated at syllable onset and offset in young juvenile birds (<45 dph) when producing subsongs, with an average rate increase of 13.5 ± 1.9 Hz beginning 27.1 ± 6.2 ms prior to syllable onsets and average suppression of 8.2 ± 1.2 Hz beginning 40.0 ± 9.1 ms prior to syllable offset (Goldberg and Fee, 2012). This syllable-related activity persists after the subsong stage (> 45 dph) but may become less robust. Previously recorded DLM neurons are either identified LMAN-projecting neurons or suspected to be LMAN projecting. Future studies are needed to clarify whether DLM neurons shown to directly project to Area X carry syllable level representations. Of note, the origin of this putative syllable associated activity is thought to be driven by excitatory input from RA instead of inhibitory input from Area X (Goldberg and Fee, 2012), further strengthening the possibility that a thalamostriatal pathway might encode syllable-level representations that differ from corticostriatal inputs to Area X. Our suggestion that DLM neurons projecting to Area X carry syllable-level representations is consistent with the role of thalamostriatal pathways in initiation and terminating motor sequences. Lesions of DLM disrupt initiation of AFP-driven vocalizations (Chen et al., 2014). Further, the parafascicular (PFs) and the ventroposterior (VPs) neurons in the rat thalamus exhibit activity correlated with sequence initiation and execution, and corresponding thalamostriatal projections from these regions contribute to the smooth initiation and the appropriate execution of motor sequences (Díaz-Hernández et al., 2018).
How Might the Thalamostriatal Pathway Contribute to Synaptic Plasticity in Area X and Guide Vocal Learning?
Although the neuronal basis for how Area X MSNs integrate their various inputs remains largely unknown, local credit assignment models provide at least one basis for thinking about how reinforcement learning shapes synaptic plasticity and guides song learning in Area X (Fee and Goldberg, 2011; Fee, 2012; Chen and Goldberg, 2020; Kornfeld et al., 2020). These models posit that coincident signals from HVC, LMAN, and VTA/SN, in a manner following three-factor Hebbian learning rules (Kuśmierz et al., 2017), drive plastic changes at corticostriatal synapses. MSNs are proposed to integrate information about timing from HVC, vocal variability from LMAN, and performance evaluation from VTA/SN to drive iterative changes in song performance through changes of synaptic weights at HVC corticostriatal synapses (Kornfeld et al., 2020). Coincident activation of HVC and LMAN inputs sets the learning window (eligibility trace) and occurring in the presence of elevated dopaminergic input leads to the strengthening of HVC synapses onto MSNs. These models provide a straightforward proposal for how moment-by-moment differences in performance might be linked via reward signals to assign credit to relevant corticostriatal synapses. However, in this framework, it is less clear how syllable-level information can be temporally assigned and chunked to permit learning and rearrangement of syllable sequences during song development.
We propose a gated-reinforcement learning model, which takes thalamostriatal input as well as ChI pauses into consideration to help resolve credit assignment for song syllables in vocal learning (Figures 1D, 2D). In this model, two independent components constitute the third factor used to modulate Hebbian plasticity in Area X: a reward prediction error signal, similar to that implemented in the above model, and a top-down feedback signal, often referred to as an attentional signal (Roelfsema and Van Ooyen, 2005; Rombouts et al., 2015; Kuśmierz et al., 2017). The first component is encoded by dopaminergic input from VTA/SN to Area X, reflecting positive and negative reward signals. This signal is delivered throughout Area X. The second component is a thalamostratial signal encoded by an efferent copy of signals from the motor cortical-like song circuit RA (Figure 1B). RA is topographically organized and neurons in the dorsal third of RA innervate premotor respiratory regions in the medulla, as well as DLM (Roberts et al., 2008; Goldberg and Fee, 2012). Therefore, the information conveyed via this pathway likely reflects expiratory and inspiratory timing information, which is tightly locked to syllable onsets (expiration) and syllable offsets (inspiration; Goller and Cooper, 2004). We propose that this putative input to Area X limits the occurrence of plasticity to affect only the corticostriatal synapses relevant to the actions selected within individual syllables and can be harnessed to help learn syllable transitions.
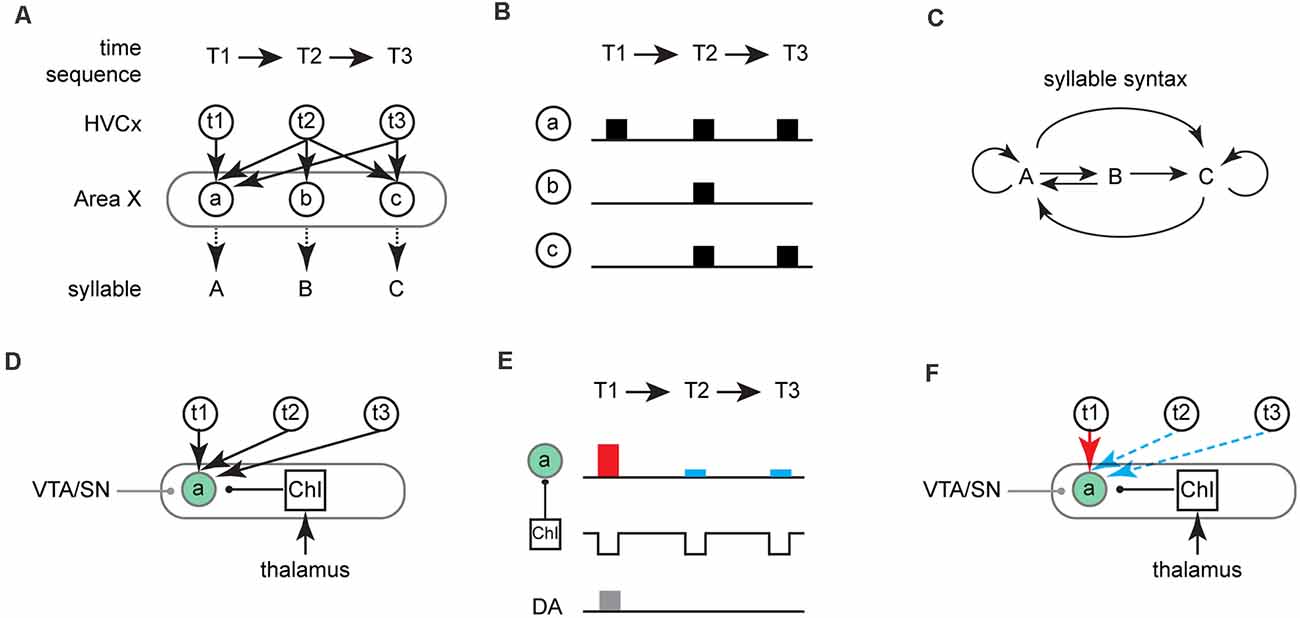
Figure 2. A gated-reinforcement learning model for sequence learning. (A) A schematic of the model for a three time-step (T1→T2→T3) song motif. Three ensembles of MSNs (a/b/c) in Area X (gray circle) are involved in learning the sequence of corresponding vocal elements (labeled as syllable A/B/C). MSN ensembles receive inputs from the ensemble of HVC neurons (HVCx, labeled as t1/t2/t3), each of which is active at the corresponding time-steps (T1–T3). Arrows between HVCx and an individual ensemble of MSNs indicate their synaptic connections and correspondent weights. In this hypothetical scenario, the probability of ensemble “a” of MSNs being activate is equal across all three time-steps for a given rendition of the song motif. This contrasts with ensemble “b” which can be activated exclusively at T2 or ensemble “c” which can be activated at either T2 or T3. (B) Theoretical neural activity in MSN ensembles (a/b/c) in Area X at different time steps given the corticostriatal connections shown in (A). (C) Syllable syntax map showing the potential syllable transitions that could result from activity patterns across MSN ensembles in Area X shown in (B). For instance, if ensemble “a” of MSNs is activate at all three time-step in one rendition, syntax “AAA” is produced. In another rendition, if ensemble “a” is activate at T1 and ensemble “c” is activated at T2 and T3, syntax “ACC” is produced. Arrows between different syllables indicate the corresponding transitions. Circle arrows besides “A” or “C” indicate repetition of the given syllable. (D) Schematic of the three inputs to the MSN ensemble “a”: VTA/SN, HVC, and ChIs. The square represents ChIs whose pauses are associated with syllable “A”. The green circle indicates that ensemble “a” is being depolarized at the same time that there are pauses in the activity of ChIs projecting onto ensemble “a”. Arrows indicate glutamatergic inputs from either HVC or DLM (thalamus). Lines with a circle at the end indicate modulatory inputs from either VTA/SN (gray, DA) or ChIs (black, Ach). (E) Schematic of gated-reinforcement learning. ChIs pauses are coincident with activation of ensemble “a” (green circle) while phasic dopamine signal (DA, gray rectangle) is active at T1. LTP (red rectangle) results at T1 when MSNs depolarization is coincident with ChIs pause and phasic increases in DA; LTD (blue rectangle) results at T2/T3 when MSNs depolarization is coincident with ChIs pause in the absence of phasic increases in DA. (F) Given the coincident activity of MSNs depolarization, cholinergic pauses and phasic increase in DA shown in (E), LTP (red arrow) results at “t1→a” synapses and LTD (blue dashed arrow) results at “t2→a” and “t3→a” synapses.
To walk through the model, imagine a simple song motif with three time-steps (T1-T3) and an ensemble of Area X projecting HVC neurons active during each of those time steps (HVCx, t1-t3). We hypothesize that Area X contains ensembles of MSNs which are responsible for the production of three distinct vocal elements that can ultimately be mapped onto the time-steps in the song motif during song learning (Figure 2A). For simplicity, we are only illustrating the corticostriatal input from HVC to Area X but envision an interaction between LMAN and HVC inputs onto MSNs as described in previous reinforcement learning models. Individual ensembles of MSNs receive inputs from the ensembles of HVC neurons and prior to sequence learning synaptic weights are equally distributed across all corticostriatal-MSN synapses (Figures 2A,B). Thus, a variety of syllable sequences can be produced across time-steps T1-T3 and which can lead to considerable variability in song syntax. For example, because all three ensembles of MSNs can be active at T2 and two ensembles of MSNs can be active at T3, six different potential syllable sequences can be generated in a motif (Figures 2B,C; T1-T3 = AAA, AAC, ABA, ABC, ACA, or ACC).
We hypothesize that DLM provides strong input to ChIs (Figure 2D). Recession or decreases in excitation from the thalamus are associated with individual syllables and cause pauses in ChIs activity. These pauses create temporal windows for synaptic plasticity at corticostriatal-MSN synapses. Within these windows, the coincidence of cortical excitatory input and phasic dopamine regulates plasticity at corticostriatal synapses (Figures 2D,E). Long-term potentiation (LTP) can be induced at relevant synapses (t1→a) when a ChIs pause is coincident with MSNs depolarization (e.g., driven by HVC input) and phasic dopamine), while long-term depression (LTD) can be induced at synapses (t2/3→a) when a ChIs pause is coincident with MSNs depolarization alone (Figures 2E,F). MSNs depolarization with phasic dopamine and without ChIs pauses (e.g., synapse t2→b in Figures 3A–C), will result in no change in synaptic plasticity (Zhang et al., 2019). As illustrated in Figures 2D,E, the syllable “A” is reinforced at T1 but not at T2 or T3, because MSNs depolarization, cholinergic pauses, and DA activation are only synchronized at T1.
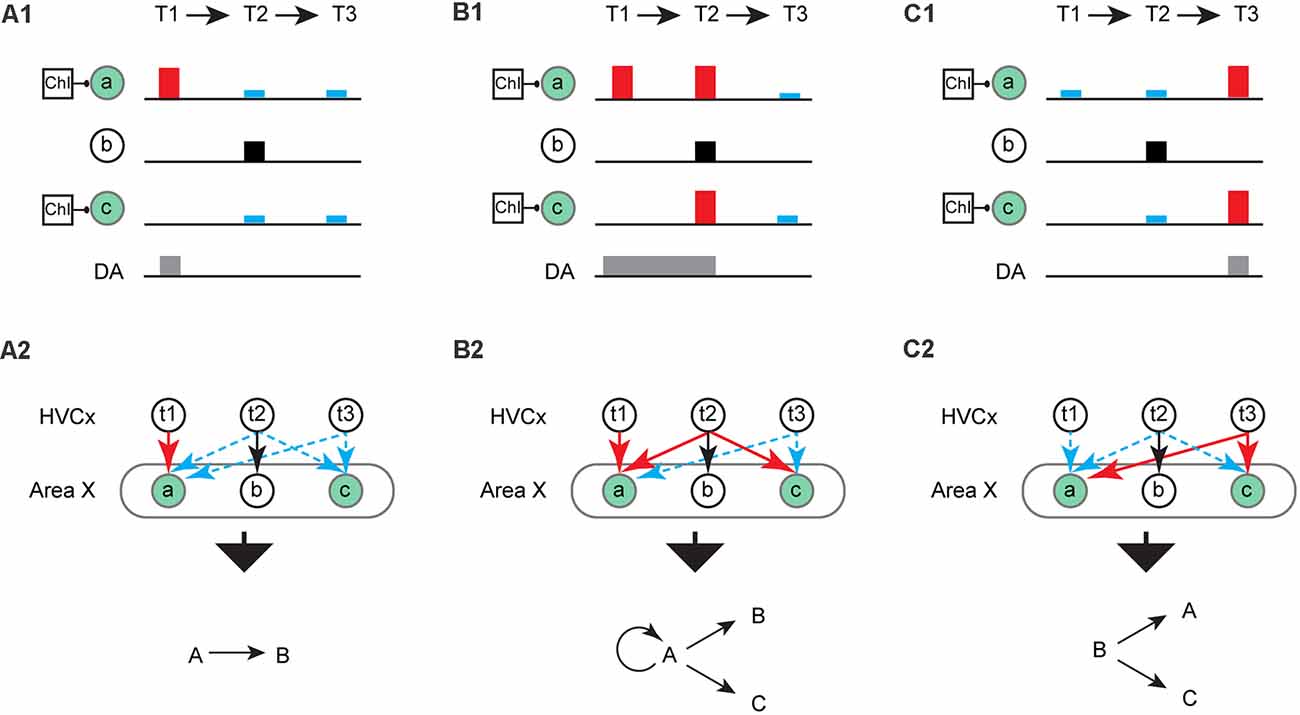
Figure 3. Hypothetical scenarios for learning syllable transitions. (A) A hypothetical scenario in which ChIs pauses are coincident with activation of ensembles “a” and “c” (green circle) and phasic increases in dopamine (DA, gray rectangle) occurs at T1. (A1) LTP (red rectangle) and LTD (blue rectangle) result at corticostriatal synapses on ensembles “a” and “c”, respectively. There is no change (black square) in the ensemble “b” when MSNs depolarization is not coincident with a pause in ChIs and/or phasic increases in DA. (A2) LTP (red arrow) results at “t1→a” synapses and LTD (blue dashed arrow) results at “t2/3→a” and “t2/3→c” synapses. Syllable “A” and “C” are omitted frequently at T2 and T3, resulting in reinforcement (learning) of the syllable sequence “A→B”. (B) Same as (A) but DA is active at T1 and T2 (B1). (B2) Syllable “A” and “C” are omitted frequently at T3 due to the LTD at “t3→a/c” synapses. Consequently, the production of syllable sequences “A→A”, “A→C” or “A→B” is reinforced. (C) Same as (A) but DA is active at T3 (C1). (C2) Syllable “A” is omitted frequently at T1 due to the LTD induced at “t1→a” synapses. Consequently, the production of syllable sequences “B→A” or “B→C” are reinforced.
Building from this, we hypothesize that windows for plasticity regulated by ChIs might ultimately account for the ability to learn syllable sequences and the variety of sequence rearrangements typically observed during the song learning process. To illustrate this, consider when ChIs pauses are associated with ensembles “a” and “c” while a phasic increase in DA is restricted at T1. In this example, a syllable sequence “AB” can be reinforced as the dominant sequence, with syllable “C” or “A” largely omitted at T3 (Figure 3A). If a phasic increase in DA is extended to T2 while maintaining the same pattern of ChIs pauses, three different transitions may emerge (“AA” or “AC” and the less likely “AB”; Figure 3B). In another scenario, when phasic DA is restricted at T3, the syllable “A” is predicted to be omitted at T1 while syllable “C” or “A” may be produced at T3, resulting in two potential transitions (“BA” or “BC”; Figure 3C). Although increasingly speculative, a similar process might be used for inserting silent gaps or merging of song syllables through the removal of silent gaps. For example, we can imagine that vocal element “B” initially described in Figure 2A is a silent gap. This silent gap can be coincident with the activity of an ensemble of MSNs in Area X but will usually not be coincident with ChIs pauses (as depicted in the scenarios in Figure 3). If “B” is a silent gap, it is simple to see how a gap can develop before (Figure 3C2), or after a syllable (Figure 3A2). Similarly, two syllables “A” and “C”, for example, can be merged into a single syllable by removing the intervening gap between them.
Incorporating syllable-level ChI activity into existing basal ganglia reinforcement models addresses potential limitations of previous models and is supported by what is currently known about BG circuits in songbirds and mammals (Figure 1D). In mammals, ChIs may be faster to respond to changes in excitatory input than MSNs (Zhang et al., 2018). ChIs pause in response to receding excitatory input and resume tonic activity with subsequent excitatory input (Zhang et al., 2018). In vivo recording of putative ChIs in Area X of juvenile birds show that they exhibit activity peaks prior to syllable onsets and decreased activity during syllable production (Pidoux et al., 2015). The firing patterns of DLM neurons and ChIs, particularly the coincidence of rate changes relative to the syllable onset and offset (Goldberg and Fee, 2012; Pidoux et al., 2015), support our speculation that ChIs pauses are driven by transient decreases in excitatory input from DLM. In addition to providing a second factor controlling temporal windows for Hebbian plasticity, our model helps capture a little more of the known complexity of BG circuits, including cell types and known pathways. The pause response in striatal tonically active neurons, believed to represent ChIs, when coincident with phasic dopamine and depolarization of MSNs, could be sufficient for the induction of corticostriatal LTP in vivo, suggesting that ChIs pause might provide critical temporal constraints for the induction of plasticity in the BG (Zhang et al., 2019).
Several important questions remain regarding this proposed model. First, our model does not account for other known properties of thalamostriatal circuits. Aside from signaling through ChIs, thalamostriatal circuits also provide abundant direct inputs onto MSNs (Figure 1D; Lacey et al., 2007; Smith et al., 2009; Guo et al., 2015). Thus, thalamostriatal activity might have a significant direct influence on spike-timing-dependent plasticity (STDP) at corticostriatal synapses (Mendes et al., 2020). In addition, the distribution of cell types in Area X receiving direct input from DLM and/or DTZ remains to be examined. Second, our model undoubtedly oversimplifies how dopaminergic and cholinergic signaling can interact in the striatum. Although the interplay between dopaminergic and cholinergic neuromodulation in the striatum has been long established (Di Chiara et al., 1994; Threlfell et al., 2012; Straub et al., 2014; Zhang et al., 2018), the functional outcome of these interactions and their influence on MSNs at fast time scales needs further examination. Performing simultaneous manipulation and/or recording of both circuits in behaving birds will ultimately be needed to understand how these interactions relate to the proposed model. Lastly, we cannot exclude other possible mechanisms that can contribute to pauses in ChIs, such as midbrain dopamine input and/or GABAergic input from the midbrain or some other source (Lim et al., 2014; Zhang and Cragg, 2017; Ahmed et al., 2019).
Discussion: Outlook and Future Directions
Understanding the role of thalamostriatal pathways in learning and production of vocal motor sequences has been limited by the lack of tools to map the functional organization of these circuits and methods to selectively monitor or modulate pathway-specific neuronal populations intermingled within BG circuits. This situation has changed dramatically with the advent of optogenetic and genetic lesioning approaches as well as progress in optical methods which can be used to monitor or manipulate selected subsets of neuronal populations embedded in the thalamus and striatum.
To help test the role of RA-DLM-Area X circuits in vocal learning and production, Cre (recombinase) dependent genetic lesioning experiments can be performed to selectively ablate DLMX inputs in juvenile or adult birds. Similar approaches have been used to investigate the roles of intratelencephalic, corticostriatal, and dopaminergic pathways in vocal learning and production in zebra finches (Roberts et al., 2017; Hisey et al., 2018; Sánchez-Valpuesta et al., 2019) as well as the roles of the corticostriatal and thalamostriatal pathway during motor learning and execution in rats (Wolff et al., 2019). To test the role of ChIs in vocal learning and production, a Cre-dependent genetic lesioning strategy can also be used locally to eliminate ChIs in Area X. A novel vector has been developed to target transgene expression in ChIs in the monkey striatum (Martel et al., 2020). Either the vector can be used directly, or the choline acetyltransferase (ChAT) promoter can be assembled into an AAV construct to drive the expression of Cre in ChIs in Area X. Similar conditional expression of the transgene has been demonstrated to be efficient in Area X when the Cre-loxP system is delivered by two separate AAV constructs (Xiao et al., 2021). Alternatively, anti-ChAT conjugated saporin toxins, which are well established to specifically target and ablate ChIs in rodent striatum (Laplante et al., 2011; Aoki et al., 2015; Crevier-Sorbo et al., 2020), may provide a virus-free tool to eliminate ChIs in Area X.
To test the signals propagated in RA-DLM-Area X circuits, cell-type-specific calcium imaging approaches, which have previously been used to monitor the activity of HVC RA neurons during courtship song production (Daliparthi et al., 2019), can be used to monitor the activity of DLMX neurons during vocal learning in juvenile birds or vocal production in adult birds. Alternatively, axon-targeted GCaMP, which has been developed to enable in vivo imaging of thalamic boutons in deep cortical layers (Broussard et al., 2018), can be used to monitor the signal transmitted by DLMX input in Area X.
Simultaneous monitoring of MSNs and ChIs has been achieved during movement in mice (Gritton et al., 2019). To monitor the interaction between MSNs and ChIs during vocal learning, a similar strategy can be adopted while replacing the ChAT-Cre line with the viral vector expressing pChAT-Cre (Martel et al., 2020). Lastly, axon targeted optogenetic excitation and perhaps inhibition can be used to test whether DLM provides strong excitatory input in ChIs and whether this input is sufficient to control the timing of pauses in ChI activity in Area X. As a complementary approach to genetic lesioning experiments, closed-loop optogenetic manipulation in behaving birds can be used to examine the real time contribution of DLMX input in song learning and production with high temporal specificity. Similar approaches have been used to investigate the roles of the dopaminergic pathway in vocal learning and production in birds (Xiao et al., 2018, 2021).
Ultimately, detailed electrophysiological circuit dissection, employing optogenetic and cell-type-specific manipulations will be needed to gain a fuller perspective on circuit models for learning and controlling song syllables and song syllable sequences. With techniques currently in hand, these experiments are now feasible but will require a concerted effort across research groups to realize the full functional role of thalamostriatal circuits in vocal motor control and learning of motor syntax.
Data Availability Statement
The original contributions presented in the study are included in the article, further inquiries can be directed to the corresponding author.
Author Contributions
LX drafted and edited the manuscript. TR edited the manuscript. All authors contributed to the article and approved the submitted version.
Funding
The Roberts laboratory is currently supported by funding from the NIH (R01NS102488, R01NS108424, UF1 NS115821, R01DC014364) and the Simons foundation (SFARI Pilot Award 733903).
Conflict of Interest
The authors declare that the research was conducted in the absence of any commercial or financial relationships that could be construed as a potential conflict of interest.
Publisher’s Note
All claims expressed in this article are solely those of the authors and do not necessarily represent those of their affiliated organizations, or those of the publisher, the editors and the reviewers. Any product that may be evaluated in this article, or claim that may be made by its manufacturer, is not guaranteed or endorsed by the publisher.
References
Ahmed, N. Y., Knowles, R., and Dehorter, N. (2019). New insights into cholinergic neuron diversity. Front. Mol. Neurosci. 12:204. doi: 10.3389/fnmol.2019.00204
Aoki, S., Liu, A. W., Zucca, A., Zucca, S., and Wickens, J. R. (2015). Role of striatal cholinergic interneurons in set-shifting in the rat. J. Neurosci. 35, 9424–9431. doi: 10.1523/JNEUROSCI.0490-15.2015
Arbuthnott, G. W., and Wickens, J. (2007). Space, time and dopamine. Trends Neurosci. 30, 62–69. doi: 10.1016/j.tins.2006.12.003
Bottjer, S. W., Halsema, K. A., Brown, S. A., and Miesner, E. A. (1989). Axonal connections of a forebrain nucleus involved with vocal learning in zebra finches. J. Comp. Neurol. 279, 312–326. doi: 10.1002/cne.902790211
Bradbury, J. W., and Vehrencamp, S. L. (2011). Principles of Animal Communication. Sunderland, MA: Sinauer Associates.
Brainard, M. S., and Doupe, A. J. (2002). What songbirds teach us about learning. Nature 417, 351–358. doi: 10.1038/417351a
Broussard, G. J., Liang, Y., Fridman, M., Unger, E. K., Meng, G., Xiao, X., et al. (2018). In vivo measurement of afferent activity with axon-specific calcium imaging. Nat. Neurosci. 21, 1272–1280. doi: 10.1038/s41593-018-0211-4
Castelino, C. B., Diekamp, B., and Ball, G. F. (2007). Noradrenergic projections to the song control nucleus Area X of the medial striatum in male zebra finches (Taeniopygia guttata). J. Comp. Neurol. 502, 544–562. doi: 10.1002/cne.21337
Chen, R., and Goldberg, J. H. (2020). Actor-critic reinforcement learning in the songbird. Curr. Opin. Neurobiol. 65, 1–9. doi: 10.1016/j.conb.2020.08.005
Chen, J. R., Stepanek, L., and Doupe, A. J. (2014). Differential contributions of basal ganglia and thalamus to song initiation, tempo, and structure. J. Neurophysiol. 111, 248–257. doi: 10.1152/jn.00584.2012
Colquitt, B. M., Merullo, D. P., Konopka, G., Roberts, T. F., and Brainard, M. S. (2021). Cellular transcriptomics reveals evolutionary identities of songbird vocal circuits. Science 371:eabd9704. doi: 10.1126/science.abd9704
Crevier-Sorbo, G., Rymar, V. V., Crevier-Sorbo, R., and Sadikot, A. F. (2020). Thalamostriatal degeneration contributes to dystonia and cholinergic interneuron dysfunction in a mouse model of Huntington’s disease. Acta Neuropathol. Commun. 8:14. doi: 10.1186/s40478-020-0878-0
Cynx, J. (1990). Experimental determination of a unit of song production in the zebra finch (Taeniopygia guttata). J. Comp. Psychol. 104, 3–10. doi: 10.1037/0735-7036.104.1.3
Daliparthi, V. K., Tachibana, R. O., Cooper, B. G., Hahnloser, R. H. R., Kojima, S., Sober, S. J., et al. (2019). Transitioning between preparatory and precisely sequenced neuronal activity in production of a skilled behavior. eLife 8:e43732. doi: 10.7554/eLife.43732
Di Chiara, G., Morelli, M., and Consolo, S. (1994). Modulatory functions of neurotransmitters in the striatum: ACh/dopamine/NMDA interactions. Trends Neurosci. 17, 228–233. doi: 10.1016/0166-2236(94)90005-1
Díaz-Hernández, E., Contreras-López, R., Sánchez-Fuentes, A., Rodríguez-Sibrían, L., Ramírez-Jarquín, J. O., and Tecuapetla, F. (2018). The thalamostriatal projections contribute to the initiation and execution of a sequence of movements. Neuron 100, 739–752.e5. doi: 10.1016/j.neuron.2018.09.052
Ding, J., Peterson, J. D., and Surmeier, D. J. (2008). Corticostriatal and thalamostriatal synapses have distinctive properties. J. Neurosci. 28, 6483–6492. doi: 10.1523/JNEUROSCI.0435-08.2008
Farries, M. A. (2004). The avian song system in comparative perspective. Ann. N Y Acad. Sci. 1016, 61–76. doi: 10.1196/annals.1298.007
Farries, M. A., and Perkel, D. J. (2002). A telencephalic nucleus essential for song learning contains neurons with physiological characteristics of both striatum and globus pallidus. J. Neurosci. 22, 3776–3787. doi: 10.1523/JNEUROSCI.22-09-03776.2002
Fee, M. S. (2012). Oculomotor learning revisited: a model of reinforcement learning in the basal ganglia incorporating an efference copy of motor actions. Front. Neural Circuits 6:38. doi: 10.3389/fncir.2012.00038
Fee, M. S., and Goldberg, J. H. (2011). A hypothesis for basal ganglia-dependent reinforcement learning in the songbird. Neuroscience 198, 152–170. doi: 10.1016/j.neuroscience.2011.09.069
Fee, M. S., Kozhevnikov, A. A., and Hahnloser, R. H. (2004). Neural mechanisms of vocal sequence generation in the songbird. Ann. N Y Acad. Sci. 1016, 153–170. doi: 10.1196/annals.1298.022
Gadagkar, V., Puzerey, P. A., Chen, R., Baird-Daniel, E., Farhang, A. R., and Goldberg, J. H. (2016). Dopamine neurons encode performance error in singing birds. Science 354, 1278–1282. doi: 10.1126/science.aah6837
Gale, S. D., and Perkel, D. J. (2010). Anatomy of a songbird basal ganglia circuit essential for vocal learning and plasticity. J. Chem. Neuroanat. 39, 124–131. doi: 10.1016/j.jchemneu.2009.07.003
Gerfen, C. R., and Wilson, C. J. (1996). “Chapter II The basal ganglia,” in Handbook of Chemical Neuroanatomy, eds L. W. Swanson, A. Björklund and T. Hökfelt (New York, NY: Elsevier), 371–468.
Goldberg, J. H., Adler, A., Bergman, H., and Fee, M. S. (2010). Singing-related neural activity distinguishes two putative pallidal cell types in the songbird basal ganglia: comparison to the primate internal and external pallidal segments. J. Neurosci. 30, 7088–7098. doi: 10.1523/JNEUROSCI.0168-10.2010
Goldberg, J. H., Farries, M. A., and Fee, M. S. (2012). Integration of cortical and pallidal inputs in the basal ganglia-recipient thalamus of singing birds. J. Neurophysiol. 108, 1403–1429. doi: 10.1152/jn.00056.2012
Goldberg, J. H., and Fee, M. S. (2011). Vocal babbling in songbirds requires the basal ganglia-recipient motor thalamus but not the basal ganglia. J. Neurophysiol. 105, 2729–2739. doi: 10.1152/jn.00823.2010
Goldberg, J. H., and Fee, M. S. (2012). A cortical motor nucleus drives the basal ganglia-recipient thalamus in singing birds. Nat. Neurosci. 15, 620–627. doi: 10.1038/nn.3047
Goller, F., and Cooper, B. G. (2004). Peripheral motor dynamics of song production in the zebra finch. Ann. N Y Acad. Sci. 1016, 130–152. doi: 10.1196/annals.1298.009
Gritton, H. J., Howe, W. M., Romano, M. F., Difeliceantonio, A. G., Kramer, M. A., Saligrama, V., et al. (2019). Unique contributions of parvalbumin and cholinergic interneurons in organizing striatal networks during movement. Nat. Neurosci. 22, 586–597. doi: 10.1038/s41593-019-0341-3
Guo, Q., Wang, D., He, X., Feng, Q., Lin, R., Xu, F., et al. (2015). Whole-brain mapping of inputs to projection neurons and cholinergic interneurons in the dorsal striatum. PLoS One 10:e0123381. doi: 10.1371/journal.pone.0123381
Hessler, N. A., and Doupe, A. J. (1999). Singing-related neural activity in a dorsal forebrain-basal ganglia circuit of adult zebra finches. J. Neurosci. 19, 10461–10481. doi: 10.1523/JNEUROSCI.19-23-10461.1999
Hidalgo-Balbuena, A. E., Luma, A. Y., Pimentel-Farfan, A. K., Peña-Rangel, T., and Rueda-Orozco, P. E. (2019). Sensory representations in the striatum provide a temporal reference for learning and executing motor habits. Nat. Commun. 10:4074. doi: 10.1038/s41467-019-12075-y
Hikosaka, O., Sakai, K., Lu, X., Nakahara, H., Rand, M. K., Nakamura, K., et al. (1999). Parallel neural networks for learning sequential procedures. Trends Neurosci. 22, 464–471. doi: 10.1016/s0166-2236(99)01439-3
Hisey, E., Kearney, M. G., and Mooney, R. (2018). A common neural circuit mechanism for internally guided and externally reinforced forms of motor learning. Nat. Neurosci. 21, 589–597. doi: 10.1038/s41593-018-0092-6
Hoffmann, L. A., Saravanan, V., Wood, A. N., He, L., and Sober, S. J. (2016). Dopaminergic contributions to vocal learning. J. Neurosci. 36, 2176–2189. doi: 10.1523/JNEUROSCI.3883-15.2016
Ikeda, M. Z., Trusel, M., and Roberts, T. F. (2020). Memory circuits for vocal imitation. Curr. Opin. Neurobiol. 60, 37–46. doi: 10.1016/j.conb.2019.11.002
Jin, X., Tecuapetla, F., and Costa, R. M. (2014). Basal ganglia subcircuits distinctively encode the parsing and concatenation of action sequences. Nat. Neurosci. 17, 423–430. doi: 10.1038/nn.3632
Kawai, R., Markman, T., Poddar, R., Ko, R., Fantana, A. L., Dhawale, A. K., et al. (2015). Motor cortex is required for learning but not for executing a motor skill. Neuron 86, 800–812. doi: 10.1016/j.neuron.2015.03.024
Kemp, J. M., Powell, T. P. S., and Harris, G. W. (1971). The termination of fibres from the cerebral cortex and thalamus upon dendritic spines in the caudate nucleus: a study with the Golgi method. Philos. Trans. R. Soc. Lond. B Biol. Sci. 262, 429–439. doi: 10.1098/rstb.1971.0105
Kornfeld, J., Januszewski, M., Schubert, P., Jain, V., Denk, W., and Fee, M. (2020). An anatomical substrate of credit assignment in reinforcement learning. bioRxiv [Preprint]. doi: 10.1101/2020.02.18.954354
Kozhevnikov, A. A., and Fee, M. S. (2007). Singing-related activity of identified HVC neurons in the zebra finch. J. Neurophysiol. 97, 4271–4283. doi: 10.1152/jn.00952.2006
Kupferschmidt, D. A., Juczewski, K., Cui, G., Johnson, K. A., and Lovinger, D. M. (2017). Parallel, but dissociable, processing in discrete corticostriatal inputs encodes skill learning. Neuron 96, 476–489.e5. doi: 10.1016/j.neuron.2017.09.040
Kuśmierz, L., Isomura, T., and Toyoizumi, T. (2017). Learning with three factors: modulating Hebbian plasticity with errors. Curr. Opin. Neurobiol. 46, 170–177. doi: 10.1016/j.conb.2017.08.020
Lacey, C. J., Bolam, J. P., and Magill, P. J. (2007). Novel and distinct operational principles of intralaminar thalamic neurons and their striatal projections. J. Neurosci. 27, 4374–4384. doi: 10.1523/JNEUROSCI.5519-06.2007
Laplante, F., Lappi, D. A., and Sullivan, R. M. (2011). Cholinergic depletion in the nucleus accumbens: effects on amphetamine response and sensorimotor gating. Prog. Neuropsychopharmacol. Biol. Psychiatry 35, 501–509. doi: 10.1016/j.pnpbp.2010.12.005
Leonardo, A. (2004). Experimental test of the birdsong error-correction model. Proc. Natl. Acad. Sci. U S A 101, 16935–16940. doi: 10.1073/pnas.0407870101
Lim, S. A., Kang, U. J., and Mcgehee, D. S. (2014). Striatal cholinergic interneuron regulation and circuit effects. Front. Synaptic Neurosci. 6:22. doi: 10.3389/fnsyn.2014.00022
Lipkind, D., Marcus, G. F., Bemis, D. K., Sasahara, K., Jacoby, N., Takahasi, M., et al. (2013). Stepwise acquisition of vocal combinatorial capacity in songbirds and human infants. Nature 498, 104–108. doi: 10.1038/nature12173
Lipkind, D., Zai, A. T., Hanuschkin, A., Marcus, G. F., Tchernichovski, O., and Hahnloser, R. H. R. (2017). Songbirds work around computational complexity by learning song vocabulary independently of sequence. Nat. Commun. 8:1247. doi: 10.1038/s41467-017-01436-0
Liu, W.-C., Gardner, T. J., and Nottebohm, F. (2004). Juvenile zebra finches can use multiple strategies to learn the same song. Proc. Natl. Acad. Sci. U S A 101, 18177–18182. doi: 10.1073/pnas.0408065101
Luo, M., and Perkel, D. J. (2002). Intrinsic and synaptic properties of neurons in an avian thalamic nucleus during song learning. J Neurophysiol 88, 1903–1914. doi: 10.1152/jn.2002.88.4.1903
Martel, A.-C., Elseedy, H., Lavigne, M., Scapula, J., Ghestem, A., Kremer, E. J., et al. (2020). Targeted transgene expression in cholinergic interneurons in the monkey striatum using canine adenovirus serotype 2 vectors. Front. Mol. Neurosci. 13:76. doi: 10.3389/fnmol.2020.00076
Mello, G. B., Soares, S., and Paton, J. J. (2015). A scalable population code for time in the striatum. Curr. Biol. 25, 1113–1122. doi: 10.1016/j.cub.2015.02.036
Mendes, A., Vignoud, G., Perez, S., Perrin, E., Touboul, J., and Venance, L. (2020). Concurrent thalamostriatal and corticostriatal spike-timing-dependent plasticity and heterosynaptic interactions shape striatal plasticity map. Cereb. Cortex 30, 4381–4401. doi: 10.1093/cercor/bhaa024
Miller, J. E., Hafzalla, G. W., Burkett, Z. D., Fox, C. M., and White, S. A. (2015). Reduced vocal variability in a zebra finch model of dopamine depletion: implications for Parkinson disease. Physiol. Rep. 3:e12599. doi: 10.14814/phy2.12599
Moss, J., and Bolam, J. P. (2008). A dopaminergic axon lattice in the striatum and its relationship with cortical and thalamic terminals. J. Neurosci. 28, 11221–11230. doi: 10.1523/JNEUROSCI.2780-08.2008
Nicholson, D. A., Roberts, T. F., and Sober, S. J. (2018). Thalamostriatal and cerebellothalamic pathways in a songbird, the Bengalese finch. J. Comp. Neurol. 526, 1550–1570. doi: 10.1002/cne.24428
Nixdorf-Bergweiler, B. E., Lips, M. B., and Heinemann, U. (1995). Electrophysiological and morphological evidence for a new projection of LMAN-neurones towards area X. Neuroreport 6, 1729–1732. doi: 10.1097/00001756-199509000-00006
Park, J., Coddington, L. T., and Dudman, J. T. (2020). Basal ganglia circuits for action specification. Annu. Rev. Neurosci. 43, 485–507. doi: 10.1146/annurev-neuro-070918-050452
Parker, P. R. L., Lalive, A. L., and Kreitzer, A. C. (2016). Pathway-specific remodeling of thalamostriatal synapses in Parkinsonian mice. Neuron 89, 734–740. doi: 10.1016/j.neuron.2015.12.038
Paton, J. J., and Lau, B. (2015). Tread softly and carry a clock’s tick. Nat. Neurosci. 18, 329–330. doi: 10.1038/nn.3959
Person, A. L., Gale, S. D., Farries, M. A., and Perkel, D. J. (2008). Organization of the songbird basal ganglia, including area X. J. Comp. Neurol. 508, 840–866. doi: 10.1002/cne.21699
Pfenning, A. R., Hara, E., Whitney, O., Rivas, M. V., Wang, R., Roulhac, P. L., et al. (2014). Convergent transcriptional specializations in the brains of humans and song-learning birds. Science 346:1256846. doi: 10.1126/science.1256846
Pidoux, L., Le Blanc, P., Levenes, C., and Leblois, A. (2018). A subcortical circuit linking the cerebellum to the basal ganglia engaged in vocal learning. eLife 7:e32167. doi: 10.7554/eLife.32167
Pidoux, M., Bollu, T., Riccelli, T., and Goldberg, J. H. (2015). Origins of basal ganglia output signals in singing juvenile birds. J. Neurophysiol. 113, 843–855. doi: 10.1152/jn.00635.2014
Ponvert, N. D., and Jaramillo, S. (2019). Auditory thalamostriatal and corticostriatal pathways convey complementary information about sound features. J. Neurosci. 39, 271–280. doi: 10.1523/JNEUROSCI.1188-18.2018
Prather, J. F., Peters, S., Nowicki, S., and Mooney, R. (2008). Precise auditory-vocal mirroring in neurons for learned vocal communication. Nature 451, 305–310. doi: 10.1038/nature06492
Ravbar, P., Lipkind, D., Parra, L. C., and Tchernichovski, O. (2012). Vocal exploration is locally regulated during song learning. J. Neurosci. 32, 3422–3432. doi: 10.1523/JNEUROSCI.3740-11.2012
Rice, M. E., and Cragg, S. J. (2008). Dopamine spillover after quantal release: rethinking dopamine transmission in the nigrostriatal pathway. Brain Res. Rev. 58, 303–313. doi: 10.1016/j.brainresrev.2008.02.004
Roberts, T. F., Hisey, E., Tanaka, M., Kearney, M. G., Chattree, G., Yang, C. F., et al. (2017). Identification of a motor-to-auditory pathway important for vocal learning. Nat. Neurosci. 20, 978–986. doi: 10.1038/nn.4563
Roberts, T. F., Klein, M. E., Kubke, M. F., Wild, J. M., and Mooney, R. (2008). Telencephalic neurons monosynaptically link brainstem and forebrain premotor networks necessary for song. J. Neurosci. 28, 3479–3489. doi: 10.1523/JNEUROSCI.0177-08.2008
Roelfsema, P. R., and Van Ooyen, A. (2005). Attention-gated reinforcement learning of internal representations for classification. Neural Comput. 17, 2176–2214. doi: 10.1162/0899766054615699
Rombouts, J. O., Bohte, S. M., Martinez-Trujillo, J., and Roelfsema, P. R. (2015). A learning rule that explains how rewards teach attention. Visual Cogn. 23, 179–205. doi: 10.1080/13506285.2015.1010462
Rothwell, P. E., Hayton, S. J., Sun, G. L., Fuccillo, M. V., Lim, B. K., and Malenka, R. C. (2015). Input- and output-specific regulation of serial order performance by corticostriatal circuits. Neuron 88, 345–356. doi: 10.1016/j.neuron.2015.09.035
Rueda-Orozco, P. E., and Robbe, D. (2015). The striatum multiplexes contextual and kinematic information to constrain motor habits execution. Nat. Neurosci. 18, 453–460. doi: 10.1038/nn.3924
Sánchez-Valpuesta, M., Suzuki, Y., Shibata, Y., Toji, N., Ji, Y., Afrin, N., et al. (2019). Corticobasal ganglia projecting neurons are required for juvenile vocal learning but not for adult vocal plasticity in songbirds. Proc. Natl. Acad. Sci. U S A 116, 22833–22843. doi: 10.1073/pnas.1913575116
Saravanan, V., Hoffmann, L. A., Jacob, A. L., Berman, G. J., and Sober, S. J. (2019). Dopamine depletion affects vocal acoustics and disrupts sensorimotor adaptation in songbirds. eNeuro 6:ENEURO.0190-19.2019. doi: 10.1523/ENEURO.0190-19.2019
Scharff, C., Kirn, J. R., Grossman, M., Macklis, J. D., and Nottebohm, F. (2000). Targeted neuronal death affects neuronal replacement and vocal behavior in adult songbirds. Neuron 25, 481–492. doi: 10.1016/s0896-6273(00)80910-1
Scharff, C., and Nottebohm, F. (1991). A comparative study of the behavioral deficits following lesions of various parts of the zebra finch song system: implications for vocal learning. J. Neurosci. 11, 2896–2913. doi: 10.1523/JNEUROSCI.11-09-02896.1991
Searcy, W. A., and Beecher, M. D. (2009). Song as an aggressive signal in songbirds. Anim. Behav. 78, 1281–1292. doi: 10.1016/j.anbehav.2009.08.011
Smith, Y., Raju, D., Nanda, B., Pare, J. F., Galvan, A., and Wichmann, T. (2009). The thalamostriatal systems: anatomical and functional organization in normal and parkinsonian states. Brain Res. Bull. 78, 60–68. doi: 10.1016/j.brainresbull.2008.08.015
Smith, Y., Raju, D. V., Pare, J.-F., and Sidibe, M. (2004). The thalamostriatal system: a highly specific network of the basal ganglia circuitry. Trends Neurosci. 27, 520–527. doi: 10.1016/j.tins.2004.07.004
Sohrabji, F., Nordeen, E. J., and Nordeen, K. W. (1990). Selective impairment of song learning following lesions of a forebrain nucleus in the juvenile zebra finch. Behav. Neural Biol. 53, 51–63. doi: 10.1016/0163-1047(90)90797-a
Straub, C., Tritsch, N. X., Hagan, N. A., Gu, C., and Sabatini, B. L. (2014). Multiphasic modulation of cholinergic interneurons by nigrostriatal afferents. J. Neurosci. 34, 8557–8569. doi: 10.1523/JNEUROSCI.0589-14.2014
Tanaka, M., Singh Alvarado, J., Murugan, M., and Mooney, R. (2016). Focal expression of mutant huntingtin in the songbird basal ganglia disrupts cortico-basal ganglia networks and vocal sequences. Proc. Natl. Acad. Sci. U S A 113, E1720–E1727. doi: 10.1073/pnas.1523754113
Tchernichovski, O., Mitra, P. P., Lints, T., and Nottebohm, F. (2001). Dynamics of the vocal imitation process: how a zebra finch learns its song. Science 291, 2564–2569. doi: 10.1126/science.1058522
Tecuapetla, F., Jin, X., Lima, S. Q., and Costa, R. M. (2016). Complementary contributions of striatal projection pathways to action initiation and execution. Cell 166, 703–715. doi: 10.1016/j.cell.2016.06.032
Threlfell, S., Lalic, T., Platt, N. J., Jennings, K. A., Deisseroth, K., and Cragg, S. J. (2012). Striatal dopamine release is triggered by synchronized activity in cholinergic interneurons. Neuron 75, 58–64. doi: 10.1016/j.neuron.2012.04.038
Vallentin, D., Kosche, G., Lipkind, D., and Long, M. A. (2016). Neural circuits. Inhibition protects acquired song segments during vocal learning in zebra finches. Science 351, 267–271. doi: 10.1126/science.aad3023
Vates, G. E., Vicario, D. S., and Nottebohm, F. (1997). Reafferent thalamo-“cortical” loops in the song system of oscine songbirds. J. Comp. Neurol. 380, 275–290. doi: 10.1002/(sici)1096-9861(19970407)380:2<275::aid-cne9>3.0.co;2-0
Wild, J. M. (1993). Descending projections of the songbird nucleus robustus archistriatalis. J. Comp. Neurol. 338, 225–241. doi: 10.1002/cne.903380207
Wolff, S. B. E., Ko, R., and Ölveczky, B. P. (2019). Distinct roles for motor cortical and thalamic inputs to striatum during motor learning and execution. bioRxiv [Preprint]. doi: 10.1101/825810
Woolley, S. C., Rajan, R., Joshua, M., and Doupe, A. J. (2014). Emergence of context-dependent variability across a basal ganglia network. Neuron 82, 208–223. doi: 10.1016/j.neuron.2014.01.039
Xiao, L., Chattree, G., Oscos, F. G., Cao, M., Wanat, M. J., and Roberts, T. F. (2018). A basal ganglia circuit sufficient to guide birdsong learning. Neuron 98, 208–221.e5. doi: 10.1016/j.neuron.2018.02.020
Xiao, L., Merullo, D. P., Koch, T. M. I., Cao, M., Co, M., Kulkarni, A., et al. (2021). Expression of FoxP2 in the basal ganglia regulates vocal motor sequences in the adult songbird. Nat. Commun. 12:2617. doi: 10.1038/s41467-021-22918-2
Zann, R. A. (1996). The Zebra Finch: A Synthesis of Field and Laboratory Studies. Oxford; New York: Oxford University Press.
Zhang, Y.-F., and Cragg, S. J. (2017). Pauses in striatal cholinergic interneurons: what is revealed by their common themes and variations? Front. Syst. Neurosci. 11:80. doi: 10.3389/fnsys.2017.00080
Zhang, Y.-F., Fisher, S. D., Oswald, M., Wickens, J. R., and Reynolds, J. N. J. (2019). Coincidence of cholinergic pauses, dopaminergic actvition and depolarization drives synaptic plasticity in the striatum. bioRxiv [Preprint]. doi: 10.1101/803536
Keywords: songbird, reinforcement learning, vocal learning, cholinergic interneurons, striatum
Citation: Xiao L and Roberts TF (2021) What Is the Role of Thalamostriatal Circuits in Learning Vocal Sequences? Front. Neural Circuits 15:724858. doi: 10.3389/fncir.2021.724858
Received: 14 June 2021; Accepted: 23 August 2021;
Published: 22 September 2021.
Edited by:
Jared Brent Smith, Regenxbio Inc., United StatesReviewed by:
C. Daniel Meliza, University of Virginia, United StatesMaxime Assous, Rutgers University, Newark, United States
Copyright © 2021 Xiao and Roberts. This is an open-access article distributed under the terms of the Creative Commons Attribution License (CC BY). The use, distribution or reproduction in other forums is permitted, provided the original author(s) and the copyright owner(s) are credited and that the original publication in this journal is cited, in accordance with accepted academic practice. No use, distribution or reproduction is permitted which does not comply with these terms.
*Correspondence: Todd F. Roberts, Todd.Roberts@utsouthwestern.edu