The Hitchhiker's Guide to Integration of Social and Ethical Awareness in Precision Livestock Farming Research
- 1Department of Biosystems and Technology, Swedish University of Agricultural Sciences, Alnarp, Sweden
- 2Department of Animal Science, Michigan State University, East Lansing, MI, United States
- 3Department of People and Society, Swedish University of Agricultural Sciences, Alnarp, Sweden
While fully automated livestock production may be considered the ultimate goal for optimising productivity at the farm level, the benefits and costs of such a development at the scale at which it needs to be implemented must also be considered from social and ethical perspectives. Automation resulting from Precision Livestock Farming (PLF) could alter fundamental views of human-animal interactions on farm and, even further, potentially compromise human and animal welfare and health if PLF development does not include a flexible, holistic strategy for integration. To investigate topic segregation, inclusion of socio-ethical aspects, and consideration of human-animal interactions within the PLF research field, the abstracts from 644 peer-reviewed publications were analysed using the recent advances in the Natural Language Processing (NLP). Two Latent Dirichlet Allocation (LDA) probabilistic models with varying number of topics (13 and 3 for Model 1 and Model 2, respectively) were implemented to create a generalised research topic overview. The visual representation of topics produced by LDA Model 1 and Model 2 revealed prominent similarities in the terms contributing to each topic, with only weight for each term being different. The majority of terms for both models were process-oriented, obscuring the inclusion of social and ethical angles in PLF publications. A subset of articles (5%, n = 32) was randomly selected for manual examination of the full text to evaluate whether abstract text and focus reflected that of the article as a whole. Few of these articles (12.5%, n = 4) focused specifically on broader ethical or societal considerations of PLF or (9.4%, n = 3) discussed PLF with respect to human-animal interactions. While there was consideration of the impact of PLF on animal welfare and farmers in nearly half of the full texts examined (46.9%, n = 15), this was often limited to a few statements in passing. Further, these statements were typically general rather than specific and presented PLF as beneficial to human users and animal recipients. To develop PLF that is in keeping with the ethical values and societal concerns of the public and consumers, projects, and publications that deliberately combine social context with technological processes and results are needed.
Introduction
Precision Livestock Farming (PLF) technologies are being proposed as solutions that allow farmers to balance producing animal products to meet growing human demands while creating conditions for good animal welfare, health, and environmental sustainability (Guarino et al., 2017; Tullo et al., 2019). The use of PLF is intended to help farmers better understand their animals as individuals, allowing them to monitor and manage animals in real time based on data from the animals themselves (Smith et al., 2015; Guarino et al., 2017). The use of PLF may also lead to fewer humans working on the farm, which may be beneficial in terms of risks related to physical, chemical, and biological injury and exposure. Limiting exposure to zoonotic diseases that may have pandemic implications could be another relevant reason to reduce numbers of human workers on farms (Dawood et al., 2011).
Historically, jobs in the livestock sector were physically demanding, repetitive, and conducted in harsh and adverse environments (Kolstrup and Jakob, 2016; Kumaraveloo and Lunner Kolstrup, 2018). Though there has been increased use of machinery on farms, particularly since the 1950s, manual labour is still extensively used in livestock production, providing jobs for workers across different generations, countries, and socio-economic conditions (Kolstrup, 2008, 2012; Lunner-Kolstrup and Ssali, 2016; Martin, 2016). However, farmers are increasingly using different sensors and digital decision-supporting tools to improve their daily workflow and maximise the economic output of their production systems while optimising labour-intensive tasks and management related to them (Karttunen et al., 2016; Hartung et al., 2017; Lunner-Kolstrup et al., 2018; Klerkx et al., 2019). A shift to relying on automation for monitoring animals as well as for performing physically demanding and repetitive work related to caregiving could lead to a radical paradigm shift in livestock sector priorities, drifting away human-animal interactions as a dominant feature at the core of farming. Using fully-automated technology to complete more husbandry tasks on livestock and poultry farms will change the nature of work on farms and could have impacts on how and how often farmers interact with their animals (Hartung et al., 2017; Kling-Eveillard et al., 2020). Research will be needed to more completely understand the questions that will arise in response to such changes in human-animal interactions, including:
1. Does reliance on technology change farmer satisfaction with their jobs?
2. Will the need for technology force some farmers out of work due to the monetary cost or need for re-learning how to farm with the technology?
3. Will automation lead to an improved ability to monitor and manage animals as individuals on farms of increasing size?
4. Will animals on fully-automated farms live lives worth living? Particularly if increased technology drives further intensification and increases in farm size?
5. Will automation lead to ethical conundrums related to farming if animals are cared for solely by technology and not humans?
For PLF to really address these questions, there must be explicit consideration of the ethical issues surrounding the use of technology with sentient beings, as mammals, birds, and even fish are widely considered to be capable of feeling by the public and demonstrated to be so by science (Duncan, 2006; Proctor et al., 2013; Russell et al., 2015; Rossi and Mattei, 2019; Rotz et al., 2019). To maintain farmers' and consumers' trust and ensure that processes that potentially could become automated and adapted for “minimised human involvement” remain ethically and socially acceptable, research addressing such consequences and challenges of digitalisation and AI in livestock production is needed before such technologies are fully integrated in animal agriculture (Stilgoe et al., 2013; Torresen, 2018).
Compared to the plant agriculture section, the animal agriculture sector has been relatively slow to adopt smart farming technologies (Kamphuis et al., 2015). Possible reasons include lack of clear financial benefit relative to existing practises, outputs that do not provide clear management advice, privacy concerns, and reluctance to use technology perceived as complex or not long lived (Daberkow and McBride, 2003; Kamphuis et al., 2015; Shepherd et al., 2018; Eastwood and Renwick, 2020). Increased automation of specific tasks in livestock production is postulated to contribute to more reliable and efficient outcomes in terms of consistently meeting standards of performance and product quality (Kamphuis et al., 2015; Bekara et al., 2017). However, the extensive use of automated solutions will significantly affect the daily work routine for farmers (Hansen, 2015; Marinoudi et al., 2019; Kling-Eveillard et al., 2020) and, potentially, shift the focus away from the animals (Rowe et al., 2019). Therefore, while there is a significant opportunity for improved logistics and automation as a result of using PLF solutions and AI, there are also barriers from a human adoption perspective that must be considered (Busse et al., 2015; Hartung et al., 2017).
The complexity of the cultural and legal frameworks around modern livestock production means that incorporating PLF, AI, and digitalisation into animal agriculture will also affect multiple aspects of the food sector and society at large (Millar and Mepham, 2001), such as:
1. Impact on the job market resulting from a shift from manual to automated labour;
2. The image of automated livestock production and its effects on individual animal welfare and health in the eyes of consumers;
3. Changes in the required competencies for working in the livestock sector;
4. Acceptance of PLF, AI and digitalisation by farmers and other end users;
5. Integration of PLF, AI, and digitalisation in different ethical, legal, and regulatory frameworks surrounding livestock production.
At present, PLF has been most well developed in the dairy industry, particularly the monitoring technologies that are linked to detecting oestrus and to robotic milking systems and their accompanying feeders (Mottram, 2016). However, for other species such as pigs and poultry, most of the solutions in PLF field are still in the research and development stage (Bailey et al., 2018; Benjamin and Yik, 2019; Li et al., 2020). At present, ethical frameworks, and consideration of societal consequences of automation in PLF do not appear to have developed in ways that constrain unintended or undesirable uses of such systems. To address this gap, we need to better integrate socio-ethical aspects into the assessment of innovative PLF solutions (Brey, 2012; Klerkx and Rose, 2020). However, such an assessment requires a deep understanding of the PLF research field's topics in a standardised, consistent way where initial experience-based bias is minimised.
This research aims to use recent advances in the Natural Language Processing (NLP) sub-branch of AI to examine abstracts from peer-reviewed publications to create and visualise a generalised topic overview of literature in the PLF research field and investigate the extent to which broader socio-ethical aspects are included in this work. This was followed by a manual examination of the full text of a randomly selected subset of articles to evaluate whether the topical representation from abstracts aligned with complete article content.
Materials and Methods
Method Description
There are several commonly used methods for text data analysis and visualisation of meaningful information patterns. Natural Language Processing (NLP) is one of the sub-branches of AI that deals with effective interpretation between computer systems and humans using natural language.
One of the popular techniques in NLP is Topic Modelling (Hoffman et al., 2010; Sievert and Shirley, 2014)—an unsupervised technique used for clustering the information found in text data and performing dimensionality reduction for better representation of semantic structures (trends) within the text. Simply put, topic models look for hidden patterns within the text such as specific word occurrence in terms of context and similarities between words as well as their overall contribution to the text structure. Topic modelling helps reduce vast amounts of textual information to their core attributes for more straightforward interpretation, further analysis, and visualisation.
There are several approaches that could be used for topic modelling, one of which is Latent Dirichlet Allocation (LDA), which was used in the present study. The LDA is a generative probabilistic model that assumes that each and every topic is a mixture (or a “bag”) of words with their own “weights” contributing to the overall meaning/importance of the topic and that each text is a mixture of different topics tied together with their distribution probabilities.
At its core, the LDA is a Bayesian network, a statistical model representing the set of variables (topics) and their conditional dependencies (weights) via a directed acyclic graph. The plate notation of LDA model can be visualised as shown in Figure 1.
There are a number of topics (K), which consist of “bags” of words described by the Dirichlet distribution ϕ (phi) and controlled by the hyperparameter β (beta). Additionally, there are a number of documents (M), each containing words (N), contributing to the overall text complexity. The W is an example of a word whose weight contributes to topic Z's importance, with θ (theta) being the Dirichlet distribution of all topics across all documents, and this distribution is controlled by another hyperparameter α (alpha).
These two hyperparameters, alpha, and beta, affect the output of the LDA model and could be adjusted depending on text complexity and the number of documents in need of analysis:
• alpha is responsible for document-topic density (and the higher this parameter is, the broader the description of the document becomes);
• beta is responsible for topic-word density (and the higher this parameter is, the more words will be assumed to contribute to the overall topic importance);
While alpha and beta hyperparameters are mostly used for fine-tuning the LDA model, there is also a third hyperparameter potentially adding the bias to the LDA model's output. This parameter is the number of topics (n) that the model will produce when provided with a certain number of documents as input. Potentially, the larger the number of topics (n) is, the more nuanced the representation of text can be achieved. However, it could also lead to an obscured picture due to the LDA model producing topics that are nearly identical. Thus, the n number is often set manually since the model cannot decide upon this variable itself. The usual approach is to initially use default model values and then adjust the n value after the first model run to achieve a broader or denser output as desired.
However, since the aim of this research was to perform an overview of words and trends present in abstracts with minimal human bias potentially affecting model output, a decision to automate the selection and evaluation of the optimal value for the n parameter was made. The iterative script written in the Python programming language was used to estimate LDA model coherence scores based on a different number of topics being applied to the target dataset. The iterative script operated with the following parameters: num_passes (number of times the model trains on all the words present in the target dataset) set to 50 and 250 to investigate the tendency for the overfitting of the model and n (number of topics potentially produced by LDA model) ranged from 1 to 25. The results of the evaluation can be seen in Figures 2, 3 (LDA Model 1) and (LDA Model 2), and the n-values leading to the highest coherence score (here, 13 and 3 for LDA Model 1 and 2, respectively) were used in the implementation of two final models. The decision to keep two different models with 13 and 3 topics, respectively was also motivated by the explorative nature of this study as an additional hypothesis was to evaluate how the interpretability of the PLF research field changes with a different number of topics produced by the LDA model and how those topics blend.
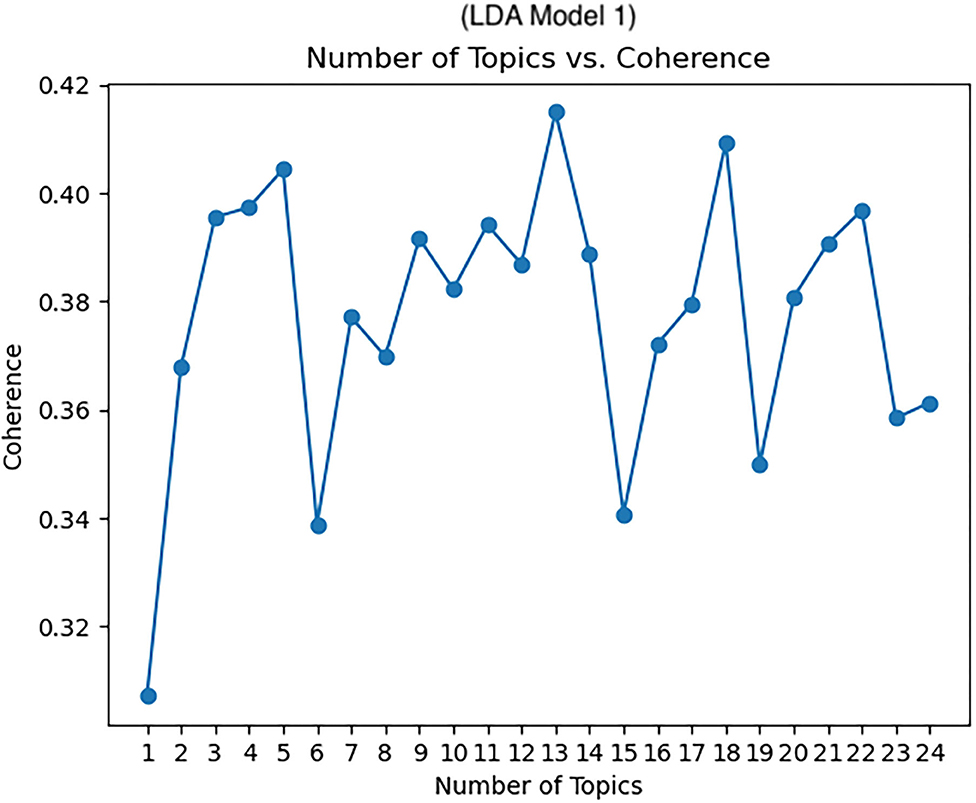
Figure 2. The evaluation results showing the optimal number of topics for highest coherence score for the LDA Model after 50 passes.
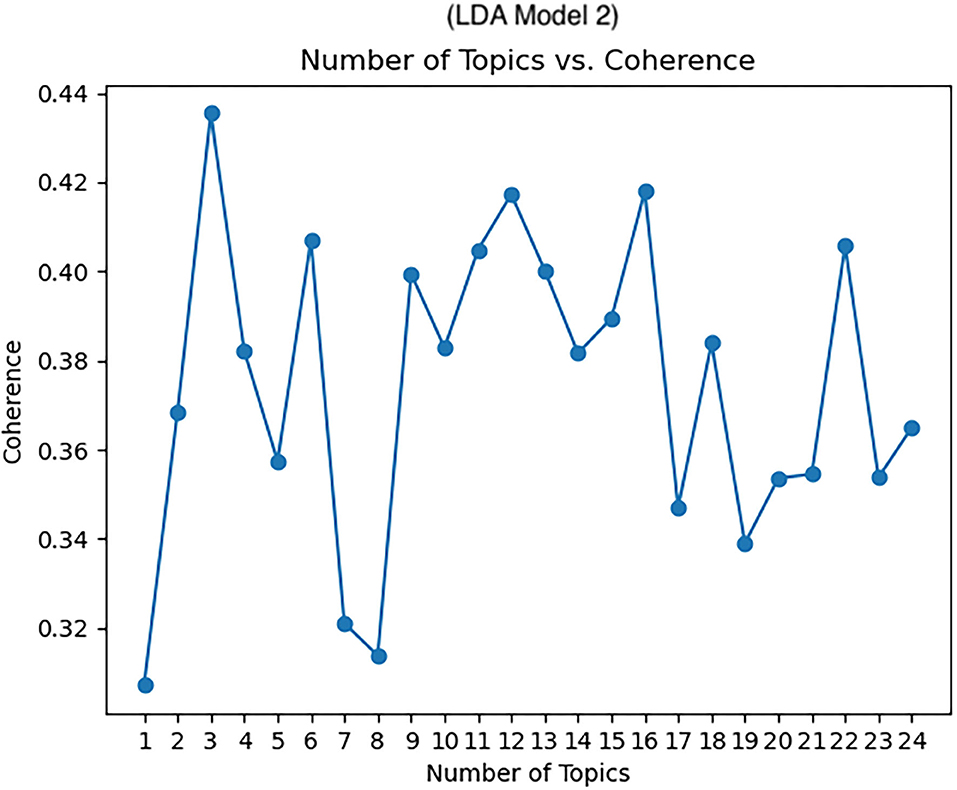
Figure 3. The evaluation results showing the optimal number of topics for highest coherence score for the LDA Model after 250 passes.
Dataset Preparation
One way to obtain correctly formatted text files of sufficient lengths is to use an official application programming interface (API) from scientific search engines (e.g., Web of Knowledge, ScienceDirect, Scopus). For the present study, Scopus was chosen as the primary engine for dataset compilation as Scopus allows output to several standard file formats (text, CSV, XML), making it easy to import data into the Python libraries used for pre-processing. Scopus also provides a specific structure to the output file, containing not only relevant text data but also links to full articles as well as indexed and author-defined keywords.
The following keywords were used (with and without word derivatives produced by the * operator) for building the search query in Scopus to identify articles for further analysis with LDA: precision livestock farming, PLF, ethic, social, value, impact, human-animal, relationship, technology, monitor, automation, welfare, farm, animal, and sensor. The decision of which search terms to initially use to identify articles for further analysis is the point at which the most human bias could be introduced. Terms were deliberately selected with the goal of discovering research articles focused on the development of technologies used within animal, not plant-based, agriculture for the automated monitoring and management of animals. Specific terms related to human-animal interactions as well as social and ethical aspects were also included to ensure that such research was identified rather than obscured by the plethora of technical studies that have been done.
To ensure that all the potentially relevant articles were found, several separate search queries with precision livestock farming/PLF keywords being the main ones and other keywords being added one at a time, and in different combinations, were conducted through the Scopus API. The search resulted in a joint Microsoft Excel file with 774 entries with separate columns containing each of the following variables: publication title, publication year, authors, abstract text, author keywords, indexed keywords, and publisher. These 774 entries were manually examined to remove articles not related (e.g., strictly animal/veterinary science with no mentioning of the PLF or technology, or with focus on plant production) to the search or with corrupted text data resulting in 644 articles falling within the defined search query. The abstract texts from each of the remaining 644 articles were then used for the NLP analysis to represent prominent trends within the PLF research domain.
The abstract text data were turned into a pandas (library for the Python programming language) dataframe to allow for higher computational efficiency during pre-processing and analysis stages.
Model Implementation
The LDA model was implemented in the Python programming language using the Gensim version of LDA. Gensim is a robust, resource-efficient library used for unsupervised semantic modelling from plain text.
To minimise subjectivity in the initial selection and interpretation of the terms present in the dataset, the following procedure was followed during pre-processing of data:
• all abstracts forming the target dataset were tokenised (split into smaller input units like words/terms for further processing via decapitalisation, removal of punctuation or other special characters);
• tokenised words/terms were lemmatised (reduction of the inflectional forms, e.g., prefix, suffix or infix, and sometimes derivationally related forms of a word to a common base form), using spacy and NLTK Python programming language packages for NLP;
• stopwords (common words/terms that do not contribute to the coherence of the text) were removed using NLTK package;
After selecting and evaluating the optimal model parameters, and the desired number of topics for output, the final LDA models yielded a coherence scores of 0.41 (LDA Model 1) and 0.43 (LDA Model 2). The coherence score (c_v) measures the relative distance between words within a topic. It is rare to see a coherence of 1 or 0.9 unless the words being measured are either identical words or bi-trigrams. The overall coherence score of a topic is the average of the semantic distances between words and could be used as a relative measure of “text interpretability.” Each topic produced by the LDA model is a vector that contains the words and their weights contributing to the final topic weight within the processed text/document.
The visual output of LDA models, with pyLDAvis library as a backend (Sievert and Shirley, 2014), is an interactive plot in HTML (webpage) format. For each model, the interactive plot consists of two panels. The left panel depicts circles representing a topic (cluster) of information, grouped based on Jensen-Shannon divergence, and the right panel presents horizontal bars visualising terms appearing within the selected topic. Each circle in the left panel represents one topic/cluster of information from the dataset, with the size of the circle being directly related to topic significance within the dataset. The distance between the centre points of different circles indicates topic similarity/difference based on the occurrence of words forming the particular topic. The bar chart in the right panel displays the 30 most relevant terms for the selected topic, where the uniqueness of a displayed term within a topic can be adjusted using a slider at the top of the panel, given a relevance parameter, λ. The length of the red bars represents the frequency of a term within the selected topic, and the length of the blue bars represents a term's frequency across the entire dataset. Setting the relevance (λ-value) closer to 0 highlights potentially rare, exclusive terms within the topic, while larger λ values highlight more common terms in the selected topic. Due to the non-supervised nature of the LDA algorithm, low relevance or λ-values and high term specificity do not contribute to the explorative nature of the study. By having the relevance metric set to 0.6, a general overview of each topic is made possible (Sievert and Shirley, 2014).
Manual Analysis of Full Text of Subset of Articles
To more deeply examine the selected articles with respect to coverage of ethical and social issues, we performed a manual content analysis of the full text of a subset of the articles. We used a random number generator to select 5% of the 644 articles subjected to the LDA (n = 32). To determine whether these articles did explicitly address ethical, societal, and human-animal implications of PLF technology, the following keywords and their derivatives were used to search the full text, including figures and tables: ethics, social, society, human, relationships, interactions, farmer, producer, manager, stockperson/stockpeople/stockman/stockmen, person, public, consumer, customer, welfare, well being (also well-being, wellbeing), concern, moral, value, impact, risk, challenge, care/caring. The following rules were applied to exclude use of search terms when used in a mathematical or analytical sense (e.g., human observer/decoder), technical sense (e.g., challenge) in an economic sense (e.g., value), study approval sense (e.g., ethics), or in a descriptive sense [e.g., concern(ing)], which were not relevant to the analysis.
Results
When comparing the initial terms produced by the two LDA models that were run with different parameters (13 vs. 3 topics and 50 vs. 250 passes for Model 1 and Model 2, respectively), the similarities of terms contributing to each model output are prominent, with only term order being different (Table 1).
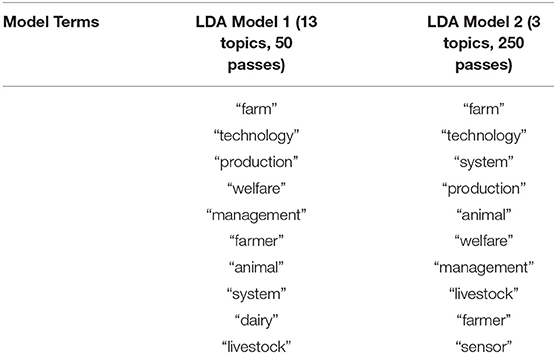
Table 1. Terms from the initial output produced by LDA models generated with the different number of passes.
The terms composing the initial output in both models are nearly identicaland require the broader context to support the initial hypothesis of the study: socio-ethical trends or those more focused on animal/farmer welfare are less dominant compared to more technical, process-oriented ones.
Figure 4 shows the visual output of LDA Model 1 (50 passes) with 13 topics identified and grouped based on their similarity and dominating trend.
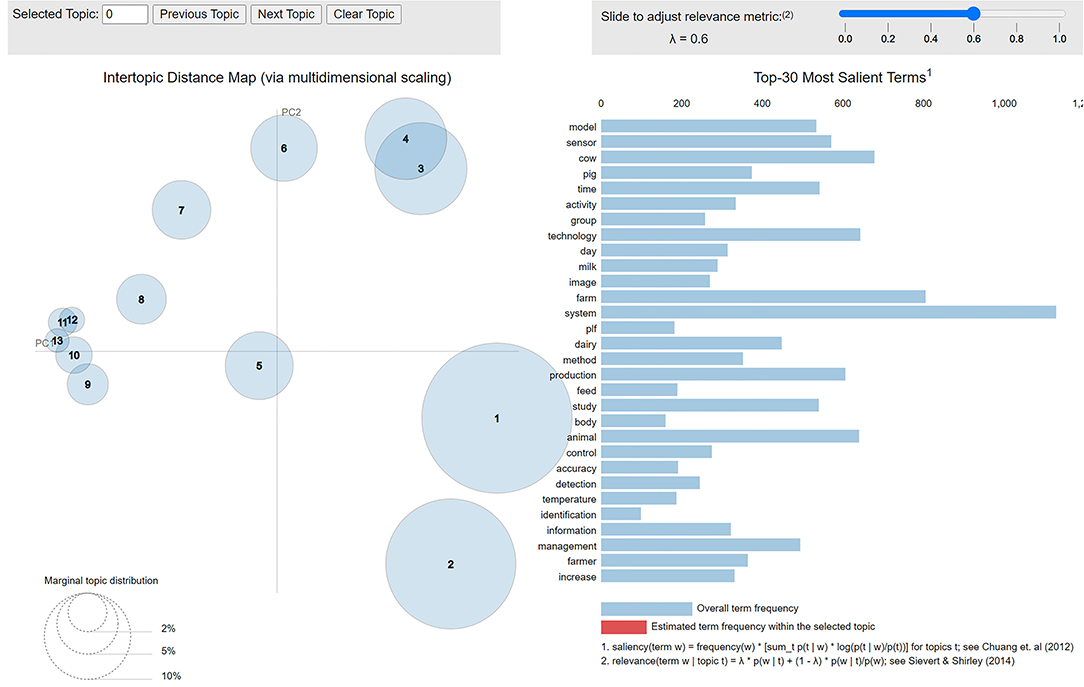
Figure 4. Overview of the results produced by LDA Model 1 (50 passes, 13 topics) with the relevance metric (λ) set to 0.6 and no specific topic cluster selected. Topics 1 and 2 depict broader generalistic PLF clusters, Topics 3 and 4 are oriented mainly toward dairy cattle, and the remaining topics are more focused and represent pig and poultry production as well as highly specific areas such as feeding, emission control, and so forth.
Figure 5 shows the visual output of LDA Model 2 (250 passes) with 3 topics identified and grouped based on their similarity and dominating trend.
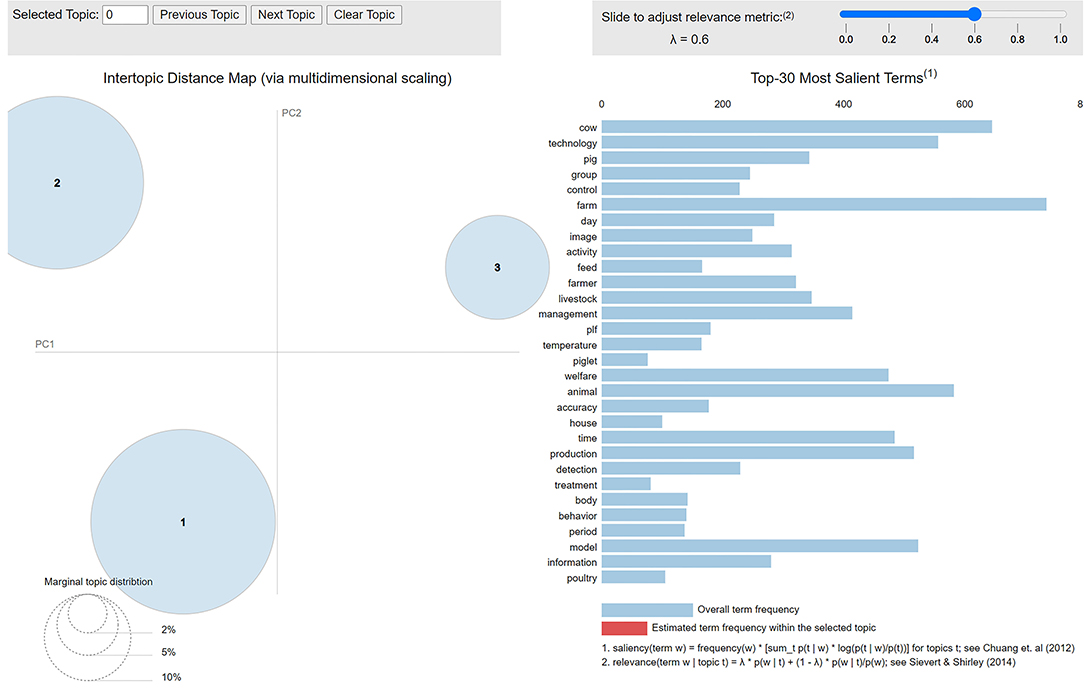
Figure 5. Overview of the results produced by LDA Model 2 (250 passes, 3 topics) with the relevance metric (λ) set to 0.6 and no specific topic cluster selected. Topics 1 and 2 depict broader generalistic PLF clusters with a predominant focus on dairy cattle. Topic 3 is more specifically oriented toward pig and poultry production and aspects such as feeding, housing, and management.
An overview of the terms from each topic produced by LDA Models 1 and 2 is presented in Table 2. For better segregation of the terms contributing to each topic, only the 5 highest ranked and 5 lowest ranked terms were selected from the 30 most relevant terms shown by pyLDAvis tool. In case of the term being a highly niched abbreviation, country name or PLF (or a direct derivative), the next term was chosen instead for better topic interpretability. The relevance metric (λ) was also set to 0.6 to provide a generalistic overview used and to align with the visual outputs of Figures 4 and 5.
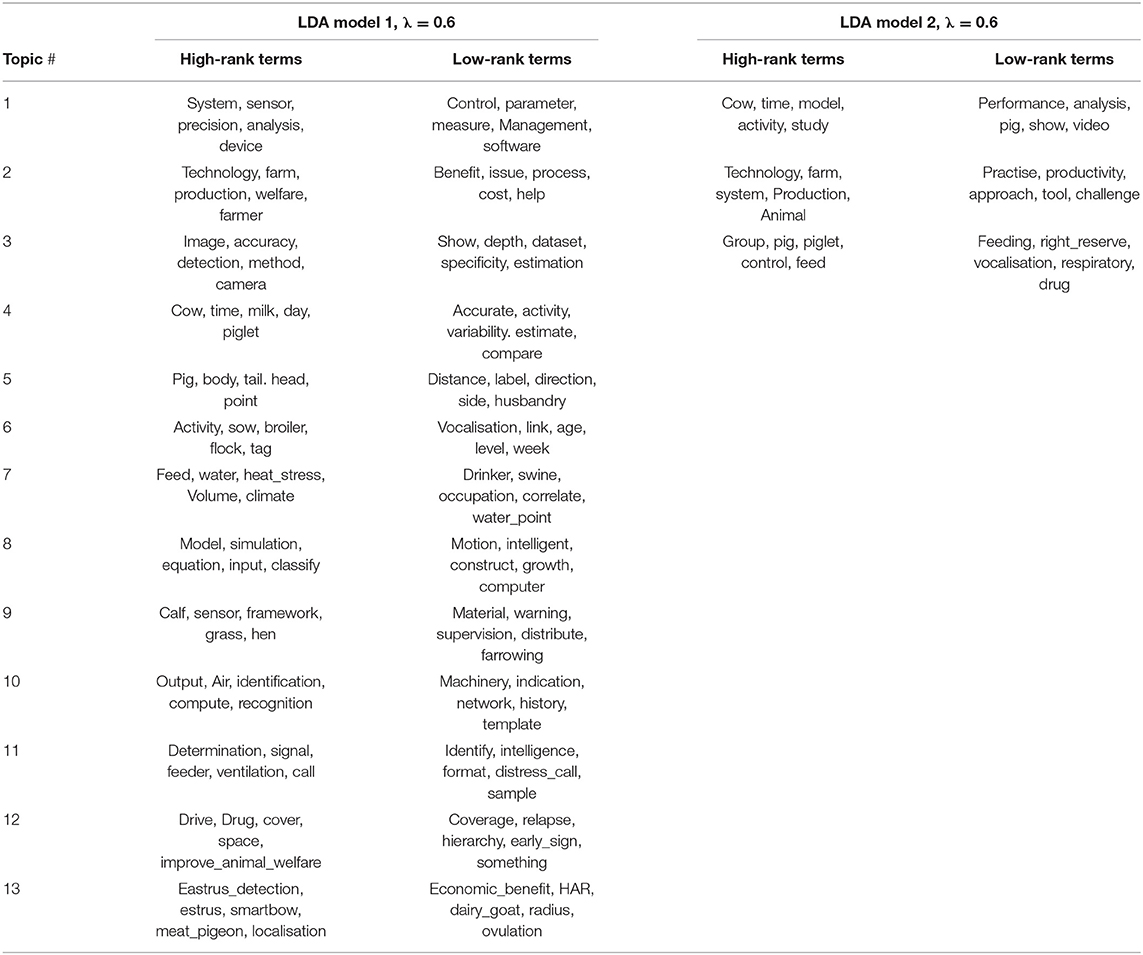
Table 2. Per-topic term overview of LDA Model 1 and LDA Model 2 with only 5 highest and 5 lowest ranked terms from each topic displayed.
Manual analysis of a subset of 32 articles (5% of the total dataset) identified three general types of articles: (1) those that made no mention of ethics, societal context, human-animal interactions, or human or animal welfare implications of PLF; (2) those that linked PLF to improved welfare of animals or to changed work (usually beneficial) of farmers; and (3) those that addressed ethical or social context surrounding PLF. In the first category, 15 of the 32 articles (46.9%) focused on development of PLF technology or data analysis and did not explicitly mention ethical or social issues related to using their PLF on farms, refer to how their PLF would impact farmers' work or interactions with animals, or consider implications of PLF on animal welfare. In the final category, four of the articles (12.5%) spent considerable (e.g., detailed discussion on a subject matter through the whole text) attention on ethical or societal implications of using PLF on farm. However, in one of these articles, PLF was only mentioned as a specific means of monitoring and assessing animal welfare to meet societal expectations rather than being the main focus of the paper (i.e., Hocquette and Chatellier, 2011).
Discussion
Due to the unsupervised nature of the method which potentially could remove the human bias from the review process, and capacity to process a large number of documents at a relatively low computational cost, the use of NLP and Topic Modelling is becoming more popular in academia for explorative literature studies (Valle et al., 2014; Liu et al., 2016; Asmussen and Møller, 2019; Muchene and Safari, 2021). The interpretation of the results produced by LDA models, however, might pose a challenge if the initial hypothesis is not supported by manual overview of the text material or if the number of topics produced by the model is not cross-fold validated against the initial dataset. There are several evaluation metrics (e.g., coherence, perplexity of the text material) that can be used for the assessment of the results produced by LDA models (Wallach et al., 2009; Mimno et al., 2011). However these metrics are highly contextual when applied for descriptive studies and not text classification, and also depend on the initial broadness of the analysed material. Higher coherence scores, achieved after the extensive hyperparameter tuning of the LDA model do not always guarantee human interpretability, thus producing diffuse word vectors that do not add to a comprehensible understanding of how the text data are clustered (Roberts et al., 2016).
The relatively low coherence scores produced during model evaluation and selection of the optimal number of topics (0.41 and 0.43 for LDA Model 1 and Model 2, respectively) for visual representation in pyLDAvis tool can potentially be explained by the broadness of PLF as a research field (Greene et al., 2014). Such broadness leads to interconnectability among the research trends and makes it difficult to locate and quantify specific areas of interest, in this case the ethical or societal aspects of innovative development within the livestock technology sector. The majority of terms produced by LDA Models 1 and 2, as seen in Table 2, are process-oriented with terms like “welfare,” “farmer,” “result” being used in exclusively descriptive context aiming at the direction of PLF development which was supported by the manual analysis of the full-text of selected articles.
As also indicated in Table 2, the ranking of terms contributing to each produced topic could lead to false assumptions of certain trends being over/underrepresented if the conclusion is based on the term ranking within the dataset corpus only. Since the LDA approach is unsupervised and operates based on term counts and approximation of distances to other terms contributing to the text structure, an additional qualitative approach to increase the interpretability of the results from the human perspective is highly advised when used for generating the research field overview (Asmussen and Møller, 2019).
In the present case, manual analysis of the full text of a subset of articles similarly revealed that the process of PLF development was the central focus of most articles. However, over half of the articles examined manually made at least passing mention of how the technology would affect farmers or animals in their care. Many of the articles that mentioned the impact of PLF on farmers described it as beneficial in terms of reduced physical or repetitive work and increased ability to monitor or make timely decisions (e.g., Kwong et al., 2009). A few articles did mention the need for farmers to gain new skills relative to using technology or managing data (e.g., Bánkuti et al., 2020) and one (i.e., Benaissa et al., 2020) mentioned frustration of farmers when technology does not work well or fails to integrate with other systems. Often the information to be gained by monitoring or aspect to be improved for the animal was related to animal welfare (e.g., Nóbrega et al., 2020), though in some cases increased production or reduced workload was the focus (e.g., Bekara et al., 2017; Abeni et al., 2019).
Improving animal welfare was often mentioned as a general reason for using PLF, as automated, continuous monitoring of animals was proposed as a way to improve focus on individual animals, particularly on farms of increasingly large size or with fewer stockpersons per animal (e.g., Morris et al., 2012; Norton and Berckmans, 2017). However, there were not often concrete examples as to how exactly a farmer could use the information to intervene effectively in a way that would address or prevent the welfare problem. Thus, most articles we examined were focused on developing the ability to detect a particular feature of interest, not on how the technology could be integrated usefully into management (Lunner-Kolstrup et al., 2018). Similarly, though the term ‘welfare’ was often used with respect to benefits of PLF to animals, the entirety of the concept was not typically captured (Van Erp-van der Kooij, 2020). Animal welfare is a complex, multidimensional concept that embodies more than simply good health or physical functioning, approaching a wholistic notion of quality of life from physical, emotional, and evolutionary perspectives (Fraser et al., 1997). Thus, most PLF only typically detects one attribute, either health or behaviour, that could influence welfare, not actually welfare itself (Buller et al., 2020).
Three articles (9.4%) specifically mentioned human-animal interactions related to development or use of PLF. In one case the authors (i.e., Benaissa et al., 2020) stated that human-animal interactions were ignored when dairy cattle monitoring systems were being developed. In another article (i.e., Berckmans, 2014), PLF was proposed as a way of replacing farmers' eyes and ears, but rather than reducing human-animal interactions, this continuous monitoring by PLF was proposed to help compensate for the increasing disconnect between the modern farmer and their animals. The final article in this set (i.e., Mancini and Zamansky, 2014) focused on using technology of all types to improve animal welfare, including providing insight into human-animal interactions, and proposed developing a field of animal welfare informatics for this specific purpose. However, the psychological aspect of farmers losing daily and physical contact with their animals due to automation of tasks was not mentioned in the subset of articles that were manually analysed. Taking daily care of animals and knowing the animals as individuals have both been found to increase farmer job satisfaction and be strong motivating factors for choosing to work with animals, such as dairy cows, among farmers, employed workers, and students at agricultural schools (Kolstrup, 2012). Animal caretakers often have a strong sense of empathy for their animals, and may suffer when technology takes over the role of the caretaker and reduces their physical contact with the animals. In a study on possible associations between health of farm staff and dairy cows, it was found that farmers experienced more physical symptoms of health problems in dairy herds with lower cow disease incidence rates (Lunner K. C. Hultgren J., 2011). Conversely, the same study found that a high incidence rate of health problems in a herd was associated with more frequent or intense exposures to negative psychosocial environmental factors among the employed dairy workers. A possible explanation to this could be that keeping a dairy herd in good health requires a lot of physical manual work and enthusiasm, while when dairy cow health and well-being is poor it is mentally stressful. Thus, when introducing comprehensive AI into animal production, it is important to consider both impacts on animal welfare as well as on human health and welfare.
Some developers of PLF, as well as social scientists, economists and ethicists interested in agricultural technology or artificial intelligence have written specifically about the implications of PLF related to farmer satisfaction, job security, or privacy concerns; the welfare of animals living in systems where technology replaces humans; the value of PLF data in the food system; and to broader issues of agricultural sustainability (e.g., Adams-Progar et al., 2017; Wathes et al., 2008; Rojo-Gimeno et al., 2019; Tullo et al., 2019; Kling-Eveillard et al., 2020; Lovarelli et al., 2020; Werkheiser, 2020; Schillings et al., 2021). While there appear to be an increase in the number of these publications, as of yet, social and ethical questions do not appear to be fully integrated into the PLF research and development paradigm. This could be due to both the rapid speed at which PLF development is currently occurring, as well as prioritisation of solving technical problems over considering ethical ones.
However, though not every article presenting the use of computer vision or a body worn sensor can or should equally cover the ethical or social ramifications of such technology, more explicit consideration of consequences to both immediate and distant end users should be made (Werkheiser, 2020). Formation of stronger collaborative teams that include tech developers, scientist with animal knowledge, farmers with practical experience, and ethicists or social scientists would lead to more robust solutions. Such teams will become particularly important as we move beyond the steps of initially developing technology for detection types of tasks and move into automating this technology and develop commercial management applications. For example, ethical and legal boundaries that frame AI must be developed, and consequences to animals, farmers and rural communities fully considered before rather than after PLF is deployed (Stilgoe et al., 2013; Torresen, 2018). Data ownership issues and privacy concerns must be balanced with demands for traceability and transparency in the food system (Adams-Progar et al., 2017). Improvements from earlier detection of problems and targeted treatment of individual animals must be balanced with whether these animals will have lives worth living if PLF leads to further intensification and increases in farm size (Schillings et al., 2021).
Conclusions
Examination of peer-reviewed scientific literature related to PLF using an automated Natural-Language Processing approach indicates that most articles on PLF are process-oriented and do not address the social or ethical context in which this technological development occurs. Subsequent analysis of full text of a subset of the identified articles found that connexions between PLF technology and applications that could minimise human labour or improve animal welfare were the most common considerations of how PLF technology could impact humans and animals. Though articles devoted explicitly to ethical uses of PLF technology, economic implications of PLF or considerations of social consequences exist, and consideration of such societal topics does appear in some technology-oriented articles, the topics and terms associated with ethics and society were not well represented among the common topic themes or terms identified in this study. Research efforts and resulting articles that engage diverse perspectives to bridge the divide between technology developers and social scientists are needed to keep PLF development grounded by the needs, uses and consideration of those it will effect, both human and animal, on and off the farm.
Data Availability Statement
The datasets generated for this study can be found in online repositories. The names of the repository/repositories and accession number(s) can be found below: https://github.com/dr-moo/PLF_Topic_Modelling.
Author Contributions
OG, JS, and CK: conceptualisation and writing—original draft preparation. OG and JS: methodology and data analysis. OG: validation and data curation. All authors contributed to the article and approved the submitted version.
Conflict of Interest
The authors declare that the research was conducted in the absence of any commercial or financial relationships that could be construed as a potential conflict of interest.
Publisher's Note
All claims expressed in this article are solely those of the authors and do not necessarily represent those of their affiliated organizations, or those of the publisher, the editors and the reviewers. Any product that may be evaluated in this article, or claim that may be made by its manufacturer, is not guaranteed or endorsed by the publisher.
References
Abeni, F., Petrera, F., and Galli, A. (2019). A survey of Italian dairy farmers' propensity for precision livestock farming tools. Animals 9:202. doi: 10.3390/ani9050202
Adams-Progar, A., Fink, G., Walker, E., and Llewellyn, D. (2017). “Chapter 18: Security and privacy issues in the internet of cows,” in Security and Privacy in Cyber-Physical Systems: Foundations, Principles, and Applications, eds H. Song, G. A. Fink, and S. Jeschke (Hoboken, NJ: John Wiley & Sons, Inc.), 375–398. doi: 10.1002/9781119226079.ch18
Asmussen, C. B., and Møller, C. (2019). Smart literature review: a practical topic modelling approach to exploratory literature review. J. Big Data 6, 1–18. doi: 10.1186/s40537-019-0255-7
Bailey, D. W., Trotter, M. G., Knight, C. W., and Thomas, M. G. (2018). Use of GPS tracking collars and accelerometers for rangeland livestock production research. Trans. Anim. Sci. 2, 81–88. doi: 10.1093/tas/txx006
Bánkuti, F. I., Prizon, R. C., Damasceno, J. C., De Brito, M. M., Pozza, M. S. S., and Lima, P. G. L. (2020). Farmers' actions toward sustainability: a typology of dairy farms according to sustainability indicators. Animal 14, s417–s423. doi: 10.1017/S1751731120000750
Bekara, M. E. A., Bareille, N., Bidan, F., Allain, C., and Disenhaus, C. (2017). “An ex ante analysis of the economic profitability of automatic oestrus detection devices in different dairy farming systems in France,” in 8. European Conference on Precision Livestock Farming (ECPLF) (p. np). Available online at: https://hal.archives-ouvertes.fr/hal-01591150
Benaissa, S., Tuyttens, F. A. M., Plets, D., Trogh, J., Martens, L., Vandaele, L., et al. (2020). Calving and estrus detection in dairy cattle using a combination of indoor localization and accelerometer sensors. Comput. Electron. Agric. 168:105153. doi: 10.1016/j.compag.2019.105153
Benjamin, M., and Yik, S. (2019). Precision livestock farming in swine welfare: a review for swine practitioners. Animals 9:133. doi: 10.3390/ani9040133
Berckmans, D. (2014). Precision livestock farming technologies for welfare management in intensive livestock systems. Rev. Off. Int. Epizoot. 33, 189–196. doi: 10.20506/rst.33.1.2273
Brey, P. A. E. (2012). Anticipatory ethics for emerging technologies. Nanoethics 6, 1–13. doi: 10.1007/s11569-012-0141-7
Buller, H., Blokhuis, H., Lokhorst, K., Silberberg, M. and Veissier, I. (2020). Animal welfare management in a digital world. Animals 10:1779. doi: 10.3390/ani10101779
Busse, M., Schwerdtner, W., Siebert, R., Doernberg, A., Kuntosch, A., König, B., and Bokelmann, W. (2015). Analysis of animal monitoring technologies in Germany from an innovation system perspective. Agric. Syst. 138, 55–65. doi: 10.1016/j.agsy.2015.05.009
Daberkow, S. G., and McBride, W. D. (2003). Farm and operator characteristics affecting the awareness and adoption of precision agricultural technologies in the US. Precision Agric. 4, 163–177. doi: 10.1023/A:1024557205871
Dawood, F. S., Dong, L., Liu, F., Blau, D. M., Peebles, P. J., Lu, X., et al. (2011). A pre-pandemic outbreak of triple-reassortant swine influenza virus infection among university students, South Dakota, 2008. J. Infect. Dis. 204, 1165–1171. doi: 10.1093/infdis/jir502
Duncan, I. J. H. (2006). The changing concept of animal sentience. Appl. Anim. Behav. Sci. 100, 11–19. doi: 10.1016/j.applanim.2006.04.011
Eastwood, C., and Renwick, A. (2020). Innovation uncertainty impacts the adoption of smarter farming approaches. Front. Sustain. Food Syst. 4:24. doi: 10.3389/fsufs.2020.00024
Fraser, D., Weary, D. M., Pajor, E. A., and Milligan, B. N. (1997). A scientific conception of animal welfare that reflects ethical concerns. Anim. Welfare 6, 187–205. Available online at: https://www.wellbeingintlstudiesrepository.org/cgi/viewcontent.cgi?article=1000&context=ethawel
Greene, D., O'Callaghan, D., and Cunningham, P. (2014). “How many topics? stability analysis for topic models,” in Joint European Conference on Machine Learning and Knowledge Discovery in Databases (Berlin, Springer), 498–513. Available online at: https://arxiv.org/abs/1404.4606v3
Guarino, M., Norton, T., Berckmans, D., Vranken, E., and Berckmans, D. (2017). A blueprint for developing and applying precision livestock farming tools: a key output of the EU-PLF project. Anim. Front. 7, 12–17. doi: 10.2527/af.2017.0103
Hansen, B. G. (2015). Robotic milking-farmer experiences and adoption rate in Jæren, Norway. J. Rural Stud. 41, 109–117. doi: 10.1016/j.jrurstud.2015.08.004
Hartung, J., Banhazi, T., Vranken, E., and Guarino, M. (2017). European farmers' experiences with precision livestock farming systems. Anim. Front. 7, 38–44. doi: 10.2527/af.2017.0107
Hocquette, J. F., and Chatellier, V. (2011). Prospects for the European beef sector over the next 30 years. Anim. Front. 1, 20–28. doi: 10.2527/af.2011-0014
Hoffman, M., Bach, F. R., and Blei, D. M. (2010). “Online learning for latent dirichlet allocation,” in Advances in Neural Information Processing Systems (Vancouver, BC: Curran Associates, Inc., Hyatt Regency), 856–864.
Kamphuis, C., Steeneveld, W., and Hogeveen, H. (2015). “Economic modelling to evaluate the benefits of precision livestock farming technologies,” in Precision livestock farming applications: Making sense of sensors to support farm management (Wageningen: Wageningen Academic Publishers), 163–171.
Karttunen, J. P., Rautiainen, R. H., and Lunner-Kolstrup, C. (2016). Occupational health and safety of Finnish dairy farmers using automatic milking systems. Front. public Heal. 4:147. doi: 10.3389/fpubh.2016.00147
Klerkx, L., Jakku, E., and Labarthe, P. (2019). A review of social science on digital agriculture, smart farming and agriculture 4.0: New contributions and a future research agenda. NJAS-Wageningen J. Life Sci. 90:100315. doi: 10.1016/j.njas.2019.100315
Klerkx, L., and Rose, D. (2020). Dealing with the game-changing technologies of Agriculture 4.0: How do we manage diversity and responsibility in food system transition pathways? Glob. Food Sec. 24:100347. doi: 10.1016/j.gfs.2019.100347
Kling-Eveillard, F., Allain, C., Boivin, X., Courboulay, V., Creach, P., Philibert, A., et al. (2020). Farmers' representations of the effects of precision livestock farming on human-animal relationships. Livest. Sci. 104057. doi: 10.1016/j.livsci.2020.104057
Kolstrup, C. L. (2012). What factors attract and motivate dairy farm employees in their daily work? Work 41, 5311–5316. doi: 10.3233/WOR-2012-0049-5311
Kolstrup, C. L., and Jakob, M. (2016). Epidemiology of musculoskeletal symptoms among milkers and dairy farm characteristics in Sweden and Germany. J. Agromedicine 21, 43–55. doi: 10.1080/1059924X.2015.1106373
Kolstrup, C. (2008). Work Environment and Health among Swedish Livestock Workers. Alnarp: Acta Universitatis Agriculturae Sueciae, 1652–6880.
Kumaraveloo, K. S., and Lunner Kolstrup, C. (2018). Agriculture and musculoskeletal disorders in low-and middle-income countries. J. Agromedicine 23, 227–248. doi: 10.1080/1059924X.2018.1458671
Kwong, K. H., Wu, T. T., Goh, H. G., Stephen, B., Gilroy, M., Michie, C., et al. (2009). Wireless sensor networks in agriculture: Cattle monitoring for farming industries. Piers Online 5, 31–35. doi: 10.2529/PIERS081001110156
Li, N., Ren, Z., Li, D., and Zeng, L. (2020). Automated techniques for monitoring the behaviour and welfare of broilers and laying hens: toward the goal of precision livestock farming. Animal 14, 617–625. doi: 10.1017/S1751731119002155
Liu, L., Tang, L., Dong, W., et al. (2016). An overview of topic modeling and its current applications in bioinformatics. SpringerPlus 5:1608. doi: 10.1186/s40064-016-3252-8
Lovarelli, D., Bacenetti, J., and Guarino, M. (2020). A review on dairy cattle farming: is precision livestock farming the compromise for an environmental, economic and social sustainable production?. J. Clean. Prod. 262:121409. doi: 10.1016/j.jclepro.2020.121409
Lunner, K. C. and Hultgren J. (2011). Perceived physical and psychosocial exposure and health symptoms of dairy farm staff and possible associations with dairy cow health. J. Agric. Safety Health 17,111–125. doi: 10.13031/2013.36496
Lunner-Kolstrup, C., Hörndahl, T., and Karttunen, J. P. (2018). Farm operators' experiences of advanced technology and automation in Swedish agriculture: a pilot study. J. Agromedicine 23, 215–226. doi: 10.1080/1059924X.2018.1458670
Lunner-Kolstrup, C., and Ssali, T. K. (2016). Awareness and need for knowledge of health and safety among dairy farmers interviewed in Uganda. Front. Public heal. 4:137. doi: 10.3389/fpubh.2016.00137
Mancini, C., and Zamansky, A. (2014). “Charting unconquered territories: intelligent systems for animal welfare,” in 40th Annual Convention of the Society for the Study of Artificial Intelligence and the Simulation of Behaviour (pp. 181–182).
Marinoudi, V., Sørensen, C. G., Pearson, S., and Bochtis, D. (2019). Robotics and labour in agriculture: a context consideration. Biosyst. Eng. 184, 111–121. doi: 10.1016/j.biosystemseng.2019.06.013
Martin, P. L. (2016). Migrant Workers in Commercial Agriculture. Available online at: http://www.ilo.org/wcmsp5/groups/public/ed_protect/protrav-migrant/documents/publication/wcms_538710.pdf.
Millar, K. M., and Mepham, T. B. (2001). Bioethical analysis of biotechnologies: lessons from automatic milking systems (AMS) and bovine somatotrophin (bST). BSAP Occasional Public. 28, 29–36. doi: 10.1017/S1463981500040954
Mimno, D., Wallach, H., Talley, E., Leenders, M., and McCallum, A. (2011). “Optimizing semantic coherence in topic models,” in Proceedings of the 2011 Conference on Empirical Methods in Natural Language Processing, 262–272.
Morris, J. E., Cronin, G. M., and Bush, R. D. (2012). Improving sheep production and welfare in extensive systems through precision sheep management. Anim. Produc. Sci. 52, 665–670. doi: 10.1071/AN11097
Mottram, T. (2016). Animal board invited review: precision livestock farming for dairy cows with a focus on oestrus detection. Animal 10, 1575–1584. doi: 10.1017/S1751731115002517
Muchene, L., and Safari, W. (2021). Two-stage topic modelling of scientific publications: a case study of University of Nairobi, Kenya. PLoS ONE 16:e0243208. doi: 10.1371/journal.pone.0243208
Nóbrega, L., Gonçalves, P., Antunes, M., and Corujo, D. (2020). Assessing sheep behavior through low-power microcontrollers in smart agriculture scenarios. Comput. Electron. Agric. 173:105444. doi: 10.1016/j.compag.2020.105444
Norton, T., and Berckmans, D. (2017). Developing precision livestock farming tools for precision dairy farming. Anim. Front. 7, 18–23. doi: 10.2527/af.2017.0104
Proctor, H. S., Carder, G., and Cornish, A. R. (2013). Searching for animal sentience: a systematic review of the scientific literature. Animals 3, 882–906. doi: 10.3390/ani3030882
Roberts, M. E., Stewart, B. M., and Tingley, D. (2016). Navigating the local modes of big data: the case of topic models. Comput. Soc. Sci. 4. doi: 10.1017/CBO9781316257340.004
Rojo-Gimeno, C., van der Voort, M., Niemi, J. K., Lauwers, L., Kristensen, A. R., and Wauters, E. (2019). Assessment of the value of information of precision livestock farming: A conceptual framework. NJAS-Wageningen J. Life Sci. 90:100311. doi: 10.1016/j.njas.2019.100311
Rossi, F., and Mattei, N. (2019). “Building ethically bounded AI,” in Proceedings of the AAAI Conference on Artificial Intelligence, 9785–9789. Available online at: http://dx.doi.org/10.1609/aaai.v33i01.33019785
Rotz, S., Gravely, E., Mosby, I., Duncan, E., Finnis, E., Horgan, M., et al. (2019). Automated pastures and the digital divide: How agricultural technologies are shaping labour and rural communities. J. Rural Stud. 68, 112–122. doi: 10.1016/j.jrurstud.2019.01.023
Rowe, E., Dawkins, M. S., and Gebhardt-Henrich, S. G. (2019). A systematic review of precision livestock farming in the poultry sector: Is technology focussed on improving bird welfare? Animals 9:614. doi: 10.3390/ani9090614
Russell, S., Dewey, D., and Tegmark, M. (2015). Research priorities for robust and beneficial artificial intelligence. Ai Mag. 36, 105–114. doi: 10.1609/aimag.v36i4.2577
Schillings, J., Bennett, R., and Rose, D. C. (2021). Exploring the potential of precision livestock farming technologies to help address farm animal welfare. Front. Anim. Sci. 2:639678. doi: 10.3389/fanim.2021.639678
Shepherd, M., Turner, J. A., Small, B., and Wheeler, D. (2018). Priorities for science to overcome hurdles thwarting the full promise of the ‘digital agriculture’revolution. J. Sci. Food Agric. 9346. doi: 10.1002/jsfa.9346
Sievert, C., and Shirley, K. (2014). “LDAvis: A method for visualising and interpreting topics,” in Proceedings of the Workshop on Interactive Language learning, Visualisation, and Interfaces, 63–70. Available online at: http://dx.doi.org/10.3115/v1/W14-3110
Smith, D., Lyle, S., Berry, A., Manning, N., Zaki, M., and Neely, A. (2015). Internet of Animal Health Things (IoAHT) Opportunities and Challenges. Cambridge: University of Cambridge.
Stilgoe, J., Owen, R., and Macnaghten, P. (2013). Developing a framework for responsible innovation. Res. Policy 42, 1568–1580. doi: 10.1016/j.respol.2013.05.008
Torresen, J. (2018). A review of future and ethical perspectives of robotics and AI. Front. Robot. AI 4, 75. doi: 10.3389/frobt.2017.00075
Tullo, E., Finzi, A., and Guarino, M. (2019). Environmental impact of livestock farming and Precision Livestock Farming as a mitigation strategy. Sci. Total Environ. 650, 2751–2760. doi: 10.1016/j.scitotenv.2018.10.018
Valle, D., Baiser, B., Woodall, C. W., and Chazdon, R. (2014). Decomposing biodiversity data using the Latent Dirichlet Allocation model, a probabilistic multivariate statistical method. Ecol. Lett. 17, 1591–1601. doi: 10.1111/ele.12380
Van Erp-van der Kooij, E. and Rutter, S.M. (2020). “Using precision farming to improve animal welfare,” in CAB Reviews: perspectives in Agriculture, Veterinary Science, Nutrition and Natural Resources, 15(051). Available online at: http://dx.doi.org/10.1079/PAVSNNR202015051
Wallach, H. M., Murray, I., Salakhutdinov, R., and Mimno, D. (2009). “Evaluation methods for topic models,” in Proceedings of the 26th annual international conference on machine learning, 1105–1112.
Wathes, C.M., Kristensen, H.H., Aerts, J.M. and Berckmans, D. (2008). Is precision livestock farming an engineer's daydream or nightmare, an animal's friend or foe, and a farmer's panacea or pitfall?. Comput. Electron Agric. 64, 2–10. doi: 10.1016/j.compag.2008.05.005
Keywords: human-animal interactions, natural language processing, ethics, social impact, responsible innovation
Citation: Guzhva O, Siegford JM and Lunner Kolstrup C (2021) The Hitchhiker's Guide to Integration of Social and Ethical Awareness in Precision Livestock Farming Research. Front. Anim. Sci. 2:725710. doi: 10.3389/fanim.2021.725710
Received: 15 June 2021; Accepted: 28 July 2021;
Published: 19 August 2021.
Edited by:
Fabyano Fonseca Silva, Universidade Federal de Viçosa, BrazilReviewed by:
Juliette Schillings, University of Reading, United KingdomPatrick Pageat, Institut de Recherche en Sémiochimie et Ethologie Appliquée (IRSEA), France
Copyright © 2021 Guzhva, Siegford and Lunner Kolstrup. This is an open-access article distributed under the terms of the Creative Commons Attribution License (CC BY). The use, distribution or reproduction in other forums is permitted, provided the original author(s) and the copyright owner(s) are credited and that the original publication in this journal is cited, in accordance with accepted academic practice. No use, distribution or reproduction is permitted which does not comply with these terms.
*Correspondence: Oleksiy Guzhva, oleksiy.guzhva@slu.se