MicroRNAs Associated With Colon Cancer: New Potential Prognostic Markers and Targets for Therapy
- 1Department of Clinical Laboratory, Affiliated Hospital of Guilin Medical University, Guilin, China
- 2Office of Drug Clinical Trials, Affiliated Hospital of Guilin Medical University, Guilin, China
- 3Department of Clinical Laboratory, General Hospital of Northern Theater Command, Shenyang, China
- 4School of Automation and Electrical Engineering, Linyi University, Linyi, China
- 5College of Computer Science, Shenyang Aerospace University, Shenyang, China
MicroRNAs (miRNAs) are a kind of non-coding RNA (ncRNA) that regulate the expression of target genes and play a role in the occurrence and development of cancers. Colon cancer (COAD) is the second most common cause of cancer-related mortality. However, the prognostic value of miRNAs in COAD is still confusing. In this study, we obtain miRNAs and messenger RNAs (mRNAs) expression profiles of COAD from the Cancer Genome Atlas (TCGA) database. After preliminary data screening and preprocessing, we acquire the expression data of 894 miRNAs and 17,019 mRNAs. Then, compared with the normal samples, 39 upregulated miRNAs and 54 downregulated miRNAs are identified by differential expression analysis. Furthermore, we obtain 1,487 upregulated mRNAs and 2,847 downregulated mRNAs. We confirm nine key miRNAs related to the survival rate of COAD patients. Moreover, by using bioinformatics methods, we get 461 common genes from both the target genes of these nine key miRNAs and differentially expressed mRNAs. Through analyzing the protein-protein interaction (PPI) network of these 461 common genes and survival analysis, we confirm five hub genes as promising biomarkers for COAD prognosis. It is worth mentioning that no previous reports have found that PGR and KCNB1 are related to COAD. We expect these key miRNAs and hub genes will provide a new way for the study of COAD.
Introduction
Non-coding RNAs (ncRNAs) are a kind of RNA which cannot be translated into protein. NcRNAs were once considered junk RNA. During the last few decades, with the development of high-throughput sequencing technology, people have realized the critical function of ncRNAs (Bussotti et al., 2013). NcRNAs contain functional types of RNA such as transfer RNAs, ribosomal RNAs, microRNAs (miRNAs), long non-coding RNAs (lncRNAs), and so on. Although most of the biological functions are performed by proteins in organisms, ncRNAs also play important roles in various biological processes (Chen et al., 2017, 2019a; Zhou et al., 2017, 2018b; Ferreira and Esteller, 2018). MiRNAs are endogenous, short ncRNAs which can regulate the expression of more than 30% of human genes (Lewis et al., 2005). Abnormal expression of miRNAs is related to various biological modifications, such as apoptosis, cell differentiation, and carcinogenesis (Su et al., 2017; Truong et al., 2017). More and more studies show that miRNAs play a necessary role in the occurrence and development of a variety of cancers, including lung cancer, breast cancer, and colon cancer (Seo et al., 2019).
Colon cancer (COAD) is a common malignant tumor that happens at the junction of rectum and sigmoid colon. In 2018, COAD ranked second for mortality and fourth for incidence (Bray et al., 2018). The 5-year survival rate of colon cancer patients is ~65% (Siegel et al., 2017). Until now, the histological feature is the only prognostic indicator for COAD. According to the histological feature, it is hard to decide to receive adjuvant chemotherapy after surgery or not. Therefore, it is imperative to excavate biomarkers for prognosis of COAD. In 2016, Caritg et al. confirmed three miRNAs (miR-103a-3p, miR-143-5p, and miR-215) as prognostic markers of COAD in patients (Caritg et al., 2016). Then Bobowicz et al. reported that five miRNAs (miR-1296, miR-135b, miR-539, miR-572, and miR-185) have prognostic values for colon cancer in patients (Bobowicz et al., 2016). Later, Maierthaler et al. showed that the miR-122 and miR-200 families have prognostic value in COAD (Maierthaler et al., 2017). However, experimentally determining the prognostic value of miRNAs is not only time-consuming but also costly. So far, many computational methods have been proposed in the field of bioinformatics, for example, circRNA-disease association (Ge et al., 2019; Zhao et al., 2019), drug-side effect (Ding et al., 2019), lncRNA-miRNA interaction (Liu et al., 2020), and lncRNA-protein interaction predictions (Hu et al., 2018; Zhao et al., 2018a,b; Bao et al., 2019; Shen et al., 2019). Therefore, there is an urgent need for developing effective bioinformatics analysis to identify molecular mechanisms related to COAD from the accumulated clinical and experimental data.
TCGA is a large database which contains epigenomic and standardized clinical data from massive samples of each kind of cancer. The data of TCGA includes exon expression data and miRNAs expression data, copy number segments, DNA methylation, phenotype, and so on. Gene chips laid on a high-throughput test can detect thousands of gene expressions in one experiment. Therefore, TCGA database can help us to obtain a large amount of genetic information about cancer in a short time and provide new targets for diagnosis and treatment of cancer (Rajendran et al., 2012, 2018; Rajendran, 2016). Several lines of evidence suggest that some modifications in miRNAs, such as ectopia, mutation, and overexpression, can cause severe pathological alterations (Xiao and Rajewsky, 2009). These effects are attributable to the translation of messenger RNA (mRNA) into protein regulated by miRNA (Bartel, 2018). Besides, different kinds of miRNA present particular expression levels in specific sorts of cancers (Hou et al., 2017). Recently, Xu et al. demonstrated that four miRNAs were significantly associated with the overall survival of COAD patients (Xu et al., 2016). Nevertheless, this study only analyzed the relationship between the expression of miRNAs and COAD patient survival. It did not explore the role of the target genes of these miRNAs in COAD. Hence, the mRNA and miRNA data of COAD from TCGA should be considered together to detect new biomarkers.
In this study, we download miRNA and mRNA expression data of COAD from TCGA database. We do a series of analyses of miRNA and mRNA, such as expression difference analysis, Gene Ontology (GO) analysis, Kyoto Encyclopedia of Genes and Genomes (KEGG) analysis, survival analysis, and PPI network analysis. Then nine key miRNAs (miR-217, miR-144, miR-129, miR-125a, miR-125b, miR-375, miR-328, miR-486, and miR-194) and five hub genes (PPARGC1A, COL1A1, SYT1, PGR, and KCNB1) are confirmed to have prognostic value in COAD. The novel promising prognostic miRNA and mRNA identified in our study will provide a new approach for clinical and experimental research in COAD.
Materials and Methods
Data Set, Differential Expression Analysis, and Survival Analysis
The “miRNA mature strand expression RNAseq by IlluminaHiseq,” “gene expression RNAseq,” and “phenotype” dataset of COAD are downloaded from TCGA. The “miRNA mature strand expression RNAseq by IlluminaHiseq” dataset contains the miRNA expression data from 261 samples, including 8 normal samples and 253 COAD samples. Then the “gene expression RNAseq” dataset consists of mRNA expression data from 329 samples, including 43 normal samples and 286 COAD samples. Furthermore, the “phenotype” dataset contains the clinical-pathological data of 545 samples, including 85 normal samples and 460 COAD samples.
We analyze the downloaded dataset as follows: above all, we separate COAD tissue and adjacent non-tumor colon tissue according to the sample number. Then we remove the data of miRNAs and mRNAs with reported expression data for <50% of the patients. Next, a CancerSubtypes package is employed to analyze the expression data of mRNA from the “gene expression RNAseq” dataset. The differentially expressed mRNAs are identified by using the thresholds which are |log2fold-change (FC)| > 1.0 and adjusted p < 0.05 for COAD samples compared with the normal samples. Then we identify the differentially expressed miRNA by analyzing the expression data of miRNAs from the “miRNA mature strand expression RNAseq by IlluminaHiseq” dataset in the similar way but using a different threshold which are |log2FC| > 3.0 and adjusted p < 0.05. In addition, a volcano map is drawn by ggplot2 package.
We use the Cox regression analysis to investigate the relationship between each miRNA/mRNA expression level and the overall survival rate of COAD patients in the “phenotype” dataset. Log-rank P < 0.05 is considered statistically significant for survival differences. Moreover, Kaplan–Meier curves of nine key miRNAs and five hub genes are drawn by the survminer package.
Prediction of Target Genes of miRNAs and Functional Enrichment Analysis
The target genes of nine key miRNAs are predicted by three kinds of online analysis software including miRDB (http://www.mirdb.org/miRDB/), TargetScanHuman (version 7.2, http://www.targetscan.org/), and mirDIP (http://ophid.utoronto.ca/mirDIP/). Then the Venn diagram is applied to confirm the common genes both in the target genes of miRNA and differentially expressed mRNA. To further understand the biological functions of the common genes, we perform GO and KEGG pathway enrichment analyses by using KOBAS (version 3.0; https://kobas.cbi.pku.edu.cn/anno_iden.php) online tool. P < 0.05 is regarded as statistically significant.
PPI Network Analysis
The STRING (version 11.0, http://string-db.org) is used for searching PPI of the common genes. At the start, a Venn diagram is used to identify the common genes both in the target genes of the nine key miRNAs and the differential expression mRNAs. After importing the official gene symbols of the common genes into STRING, we get the PPI network of the common genes. Then, Cytoscape (version 3.7.1) is applied for the visualization of PPI networks. The confidence score 0.4 is used as the cut-off criterion.
Confirmation of Hub Genes
CytoHubba, an app of Cytoscape, is applied to confirm hub genes. We employ a Venn diagram to extract the overlapping genes of the top 50 genes by six different algorithms, including MCC, Degree, Closeness, Radiality, Betweenness, and Stress. These overlapping genes are confirmed as the hub genes. Subsequently, we utilize Cox regression analysis to determine the prognostic role of the hub genes.
Result
Identification of Differentially Expressed miRNAs and mRNAs in COAD
Based on the analysis of the CancerSubtypes package, 93 differentially expressed miRNAs are acquired, including 39 upregulated miRNAs and 54 downregulated miRNAs (Figure 1A). Then in a similar way, 4,334 differentially expressed mRNAs containing 1,487 upregulated miRNAs and 2,847 downregulated mRNAs are extracted (Figure 1B).
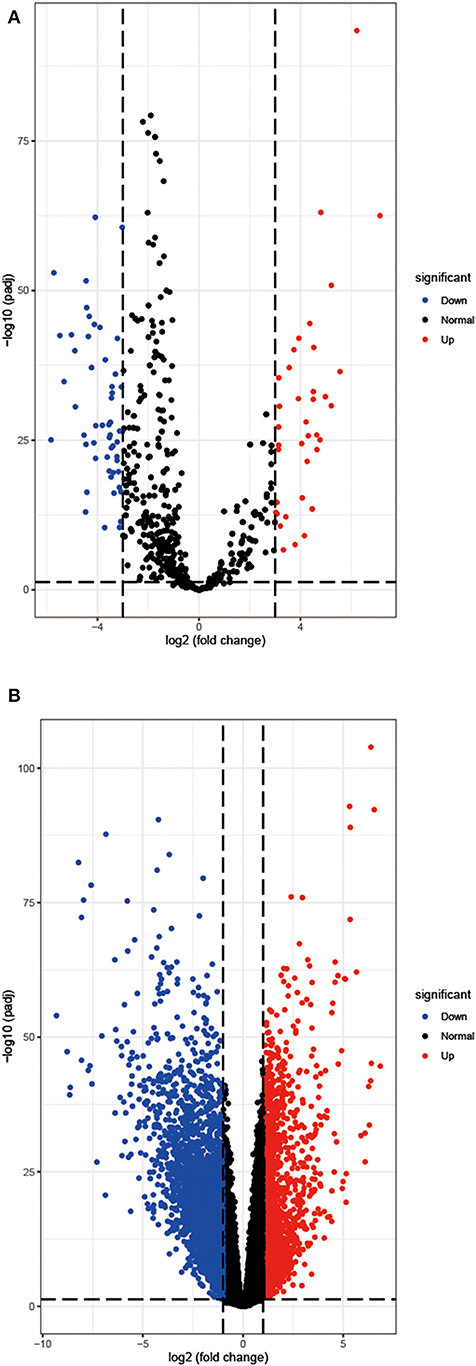
Figure 1. The differentially expressed miRNAs (A) and mRNAs (B) of COAD. Red, up-regulation; blue, and down-regulation.
Identification of miRNA With Prognostic Value in COAD
Through survival analysis, we find nine miRNAs that are significantly associated with the overall survival of COAD patients (Figure 2). The name, Log2FC, p-value and adjusted p-value of these key miRNAs are displayed in Table 1. In these miRNAs, miR-217 and miR-144 are upregulated, miR-129, miR-125a, miR-125b, miR-375, miR-328, miR-486, and miR-194 are downregulated. In COAD, miR-217 specifically inhibits DKK1, which is an important antagonist of the Wnt signaling pathway to promote apoptosis of colon cells (Jia et al., 2019). By controlling the expression of SMAD4, miR-144 inhibits invasion and migration of colon cancer cells (Sheng et al., 2019). High mobility group box protein 1 (HMGB1) plays a part in immune escape in COAD cells (Zheng and Zhu, 2018). MiR-129, which targets the 3′UTR of HMGB1, is able to repress the development of COAD (Wu et al., 2018). By inhibiting cell proliferation and inducing cell apoptosis, miR-125a acts as a suppressor of COAD (Tong et al., 2015). The level of miR-375 is lower in colorectal cancer tissues than normal human colon tissues. In addition, miR-375 exerts an inhibitory effect on the proliferation of colorectal cancer by targeting the 3′UTR of KLF4 (Mao et al., 2016). Recently, a new study reported that miR-375 has a prognostic value in COAD (Huang and Pan, 2019). By regulating SLC2A1/GLUT1, miR-328 participates in the Warburg effect in COAD (Santasusagna et al., 2018). MiR-486 is related to the molecular mechanisms of several cancers, including cervical cancer (Li et al., 2018a), breast cancer (Li et al., 2019), lung cancer (Tian et al., 2019), esophageal cancer (Lang and Zhao, 2018), ovarian cancer (Ma et al., 2016), and pancreatic cancer (Xia et al., 2019). Kelley et al. nearly demonstrated that the expression of miR-486 associates with early-stage of COAD (Kelley et al., 2018). Moreover, Ren et al. reported that miR-486 plays a prognostic role in COAD (Ren et al., 2016). MiR-194, whose expression is upregulated by p53, inhibits THBS1 expression to promote angiogenesis and facilitate tissue repair in COAD (Sundaram et al., 2011). In a previous study, miR-125b is confirmed as a prognostic biomarker of colorectal cancer (Zhou et al., 2018c). Up to now, the prognostic value of four miRNAs (miR-217, miR-125, miR-129, and miR-194) in COAD has not been previously reported. These miRNAs will become new potential prognostic biomarkers of COAD.
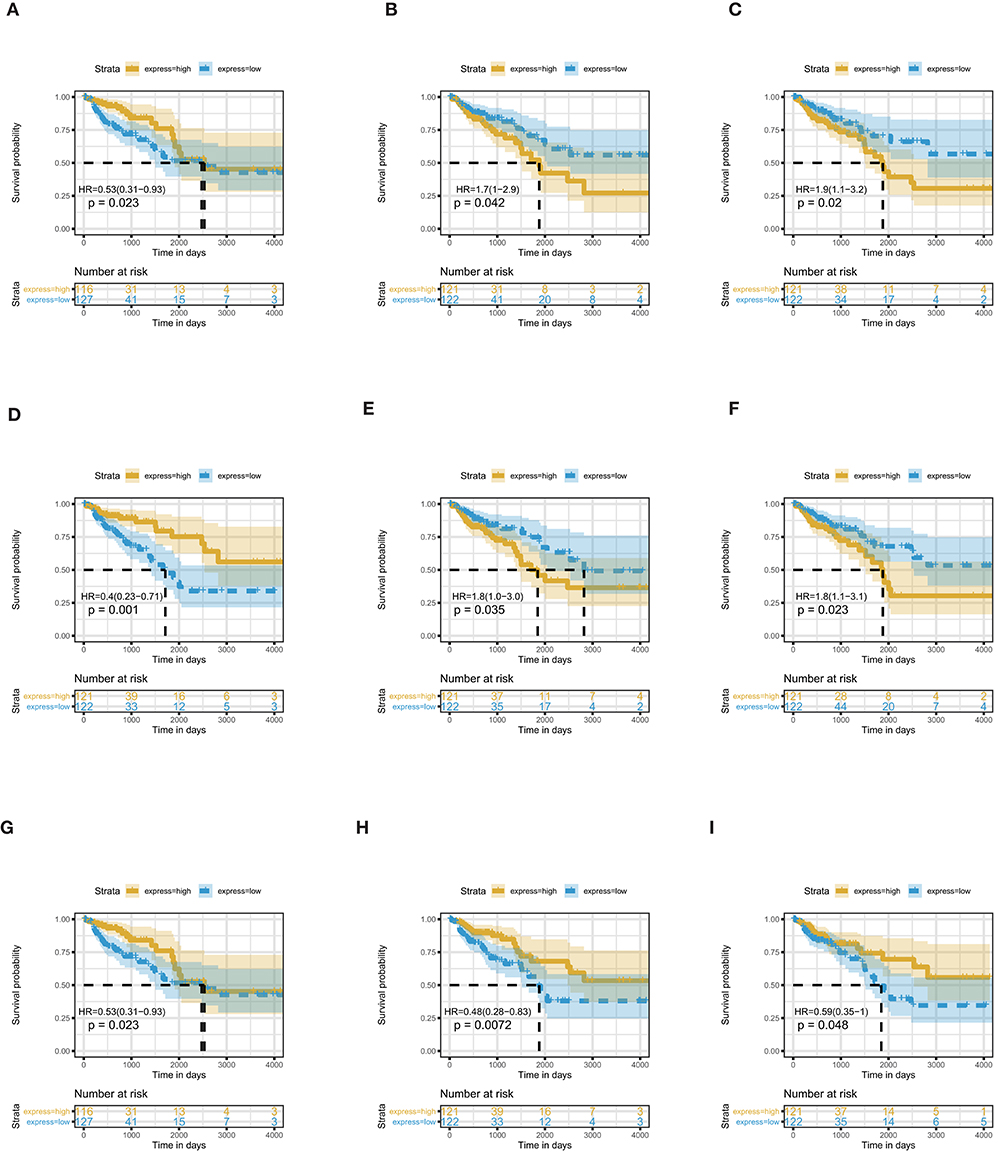
Figure 2. Nine differentially expressed miRNAs are associated with overall survival in COAD patients by using Kaplan–Meier curve, Log-rank test. (A) miR-129; (B) miR-217; (C) miR-125a; (D) miR-375; (E) miR-328; (F) miR-125b; (G) miR-144; (H) miR-194; (I) miR-486.
Prediction of miRNA-mRNA Interaction and Functional Enrichment Analysis
The target gene predictive tools, including miRDB, mirDIP, and TargetScanHuman, are employed to find miRNAs-targeted genes. During this process, 7,592 genes are identified as the target genes of nine miRNAs with prognostic value in COAD. To improve the accuracy of subsequent analysis, a Venn diagram is used to find common genes both in the differentially expressed mRNAs and in the 7,592 target genes. At last, we get 461 common genes. To further understand the biological roles of these 461 common genes, we perform GO analysis and KEGG pathways enrichment analysis of them. The GO analysis shows that 418 genes are involved in cell and cell parts in the cellular component (CC) category. Regarding the biological process (BP) category, this result shows that most of the 461 common genes enrich several functions, including cellular process (392 genes), biological regulation (339 genes), regulation of biological process (321 genes), and regulation of cellular process (304 genes). Then for the molecular function (MF) group, a large proportion of the common genes are mainly enriched in binding and protein binding (Figure 3A). In addition, the KEGG Pathway analysis demonstrates that the 461 common genes enrich cancer-associated signaling pathways, containing pathways in cancer (17 genes, p-Value: 7.41E-06), cell adhesion molecules (CAMs) (16 genes, p-Value: 7.43E-11), cAMP signaling pathway (13 genes, p-Value: 1.22E-06), signaling pathways regulating the pluripotency of stem cells (12 genes, p-Value: 2.55E-06), MAPK signaling pathway (12 genes, p-Value: 6.88E-05), HIF-1 signaling pathway (10 genes, p-Value: 7.70E-07) and proteoglycans in cancer (10 genes, p-Value: 2.08E-04) (Figure 3B). Pathways in cancer include Wnt signaling pathway, P53 signaling pathway, and so on. These signaling pathways involve the development of COAD. A previous study showed that CAMs can regulate cancer cell invasion and tumor metastasis (Geletu et al., 2018). The cAMP signaling pathway takes part in regulating various biological processes including cell proliferation, secretion, metabolism and apoptosis. Targeted modulation of cAMP signaling pathway can induce proliferation and apoptosis of a variety of malignant lymphoma cells (Mehta and Patel, 2019).
Confirmation of Hub Genes
The 461 common genes are used to construct a PPI network which contains 351 nodes and 881 edges (Figure 4). We utilize the cytoHubba app of Cytoscape software to calculate the connectivity between the genes. Because the genes with high connectivity can play an important role in the cancers, we identify five hub genes. The gene symbol, Log2FC, p-value, and adjusted p-value of these hub genes are showed in Table 2. Furthermore, through survival analysis, we find the five hub genes that have prognostic value in COAD (Figure 5). Among them, when the level of PPARGC1A expression rises, the survival rate of COAD patients improves. Conversely, when the expression levels of COL1A1, SYT1, PGR, and KCNB1 decline, the survival rate of COAD patients improves. As an activator of p53, PPARGC1A can suppress cancer cell apoptosis (Sen et al., 2011). COL1A1 participates in the process of focal adhesion and may influence the metastatic ability of cells (Tian et al., 2015). COL1A1, which is upregulated in COAD, may be a biomarker for colon cancer progression (Yang et al., 2019). Because of down regulation in left-sided colon carcinoma compared with right-sided colon carcinoma, STY1 possibly plays a role in their genetic susceptibilities to neoplastic transformation (Zhu et al., 2013). As the receptor of hormones, PGR is very important for breast growth. Moreover, it is related to the development of breast cancer and endometrial cancer (Kurozumi et al., 2017; He et al., 2019). As a prognostic biomarker for gliomas, KCNB1 suppresses tumor growth by inducing autophagy (Wang et al., 2017). So far, there is no study showing the effect of PGR and KCNB1 in COAD. Therefore, PGR and KCNB1 may be new potential prognostic biomarkers in COAD.
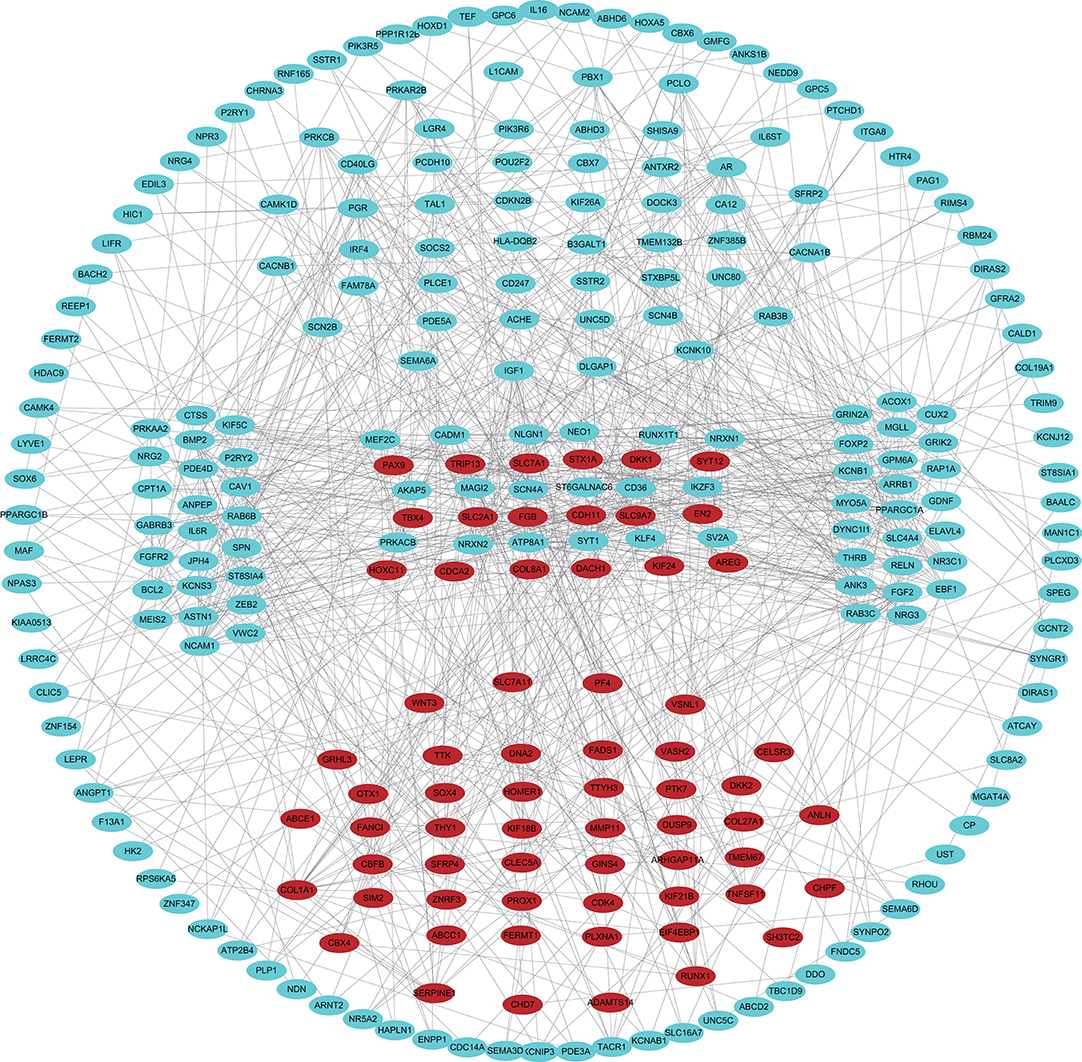
Figure 4. PPI network of common genes. The blue and red dots represent down-regulated and up-regulated genes, respectively.
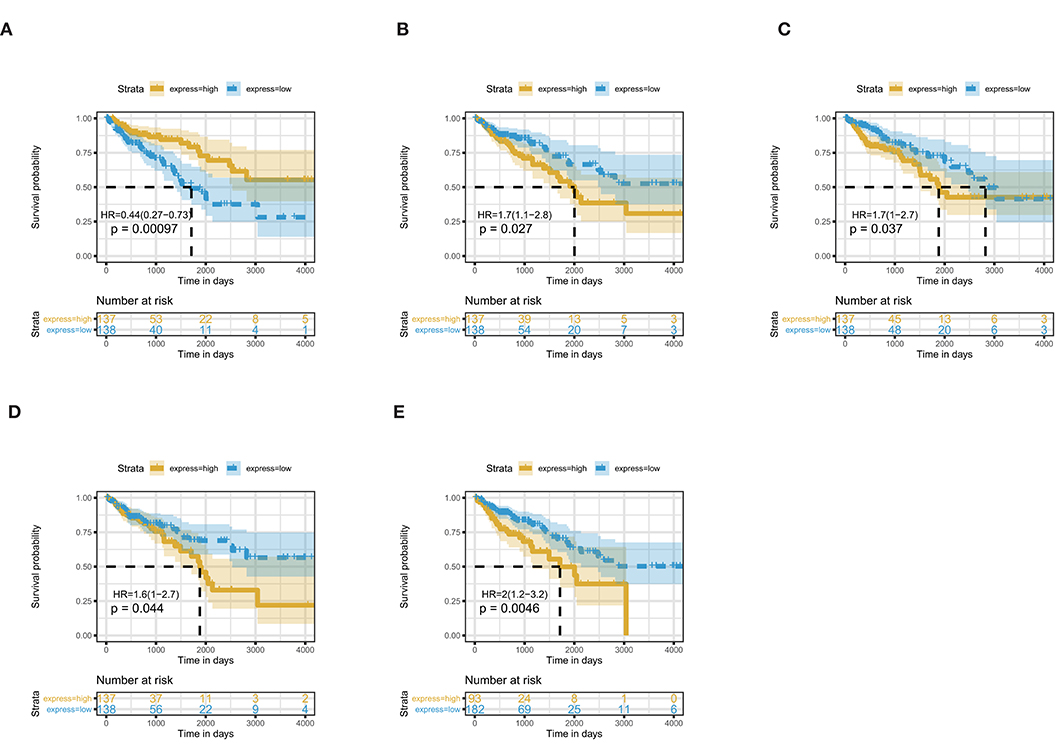
Figure 5. Five hub genes are associated with overall survival in COAD patients by using Kaplan–Meier curve, Log-rank test. (A) PPARGC1A; (B) COL1A1; (C) SYT1; (D) PGR; (E) KCNB1.
Discussion
COAD is a common gastrointestinal tumor. High mortality and morbidity are the main clinical features of this disease. At present, the diagnosis of COAD has many problems, such as poor specificity and low time efficiency (Benson et al., 2017; Sun et al., 2019). Previous studies show that some genetic changes are closely associated with the occurrence of COAD (Li et al., 2018b; Wei et al., 2018). For this reason, it is critical to find prognostic biomarkers for the treatment of COAD. It is well-known that the experimental research on the role of miRNAs needs many materials and costs huge time. Compared with traditional experimental methods, the bioinformatics method is cost-effective and timesaving when studying the role of miRNAs. Therefore, in this study we research the prognostic role of miRNAs in COAD using the bioinformatics methods. Furthermore, the role of target genes in COAD can further prove the accuracy of miRNAs predictive results. Hence, we assess not only four miRNAs but also two target genes of nine miRNAs with prognostic value as new potential prognostic markers for COAD. In addition, the target genes with prognostic value can also be therapeutic targets for COAD. These findings will provide a basis for further research on diagnoses of COAD, as well as a new target of monoclonal antibody drugs for treatment of COAD. In future, we plan to use several previous effective computational models to identify colon cancer-related miRNAs (Chen et al., 2018a,b, 2019b).
Despite some promising results, there are several limitations in the current study. First, all data analyzed in the study come from TCGA database, and there is no actual clinical patient data involved in the study. Next, we confirm several novel key miRNAs and hub genes that have never been reported to own prognostic value in COAD. But their molecular mechanisms should be further explored by the experimental way. In the end, more and more studies reported on the role of lncRNA in COAD (Wang et al., 2018; Zhou et al., 2018a). However, lncRNAs are not involved in this study. In the following study, we will collect enough clinical data for further verifying the results of the study. Then we will design a series of experiments to investigate the molecular mechanisms of novel key miRNAs and hub genes. Moreover, lncRNAs should be introduced into the prognostic prediction system for improving accuracy.
Data Availability Statement
Publicly available datasets were analyzed in this study. This data can be found here: https://www.cancer.gov/about-nci/organization/ccg/research/structural-genomics/tcga.
Author Contributions
QZ contributed to the design of the study protocol. JZ and YX performed statistical analysis and drawn the pictures. LQ and JS contributed to the writing of the study protocol. SL downloaded data. All authors approved the final version of the manuscript.
Funding
This work was supported by Doctoral Scientific Research Starting Foundation of Guilin Medical University, the Education Department of Liaoning Province under Grant No. LQN201711 and the National Natural Science Foundation of China under Grant No. 11805091.
Conflict of Interest
The authors declare that the research was conducted in the absence of any commercial or financial relationships that could be construed as a potential conflict of interest.
Abbreviations
miRNAs, microRNAs; COAD, colon cancer; TCGA, the Cancer Genome Atlas; PPI, protein-protein interaction; ncRNAs, Non-coding RNAs; HMGB1, High mobility group box protein 1; GO, Gene Ontology; KEGG, Kyoto Encyclopedia of Genes and Genomes; CAMs, cell adhesion molecules; CCL-1, cell adhesion molecule 1; CC, cellular component; BP, biological process; MF, molecular function; MAPK, Mitogen-activated protein kinase; HIF-1, hypoxia-inducible factor-1.
References
Bao, S., Zhao, H., Yuan, J., Fan, D., Zhang, Z., Su, J., et al. (2019). Computational identification of mutator-derived lncRNA signatures of genome instability for improving the clinical outcome of cancers: a case study in breast cancer. Brief. Bioinform. bbz118. doi: 10.1093/bib/bbz118
Benson, A. B., Venook, A. P., Cederquist, L., Chan, E., Chen, Y.-J., Cooper, H. S., et al. (2017). Colon cancer, version 1.2017, NCCN clinical practice guidelines in oncology. J. Natl. Compr. Cancer Netw. 15, 370–398. doi: 10.6004/jnccn.2017.0036
Bobowicz, M., Skrzypski, M., Czapiewski, P., Marczyk, M., Maciejewska, A., Jankowski, M., et al. (2016). Prognostic value of 5-microRNA based signature in T2-T3N0 colon cancer. Clin. Exp. Metas. 33, 765–773. doi: 10.1007/s10585-016-9810-1
Bray, F., Ferlay, J., Soerjomataram, I., Siegel, R. L., Torre, L. A., and Jemal, A. (2018). Global cancer statistics 2018: GLOBOCAN estimates of incidence and mortality worldwide for 36 cancers in 185 countries. CA Cancer J. Clin. 68, 394–424. doi: 10.3322/caac.21492
Bussotti, G., Notredame, C., and Enright, J. A. (2013). Detecting and comparing non-coding RNAs in the high-throughput era. Int. J. Mol. Sci. 14, 15423–15458. doi: 10.3390/ijms140815423
Caritg, O., Navarro, A., Moreno, I., Martínez-Rodenas, F., Cordeiro, A., Muñoz, C., et al. (2016). Identifying high-risk stage II colon cancer patients: a three-MicroRNA-based score as a prognostic biomarker. Clin. Colorect. Cancer 15, e175–e182. doi: 10.1016/j.clcc.2016.04.008
Chen, X., Wang, L., Qu, J., Guan, N.-N., and Li, J.-Q. (2018a). Predicting miRNA–disease association based on inductive matrix completion. Bioinformatics 34, 4256–4265. doi: 10.1093/bioinformatics/bty503
Chen, X., Xie, D., Zhao, Q., and You, Z.-H. (2019a). MicroRNAs and complex diseases: from experimental results to computational models. Brief. Bioinform. 20, 515–539. doi: 10.1093/bib/bbx130
Chen, X., Yan, C. C., Zhang, X., and You, Z.-H. (2017). Long non-coding RNAs and complex diseases: from experimental results to computational models. Brief. Bioinform. 18, 558–576. doi: 10.1093/bib/bbw060
Chen, X., Yin, J., Qu, J., and Huang, L. (2018b). MDHGI: matrix decomposition and heterogeneous graph inference for miRNA-disease association prediction. PLoS Comput. Biol. 14:e1006418. doi: 10.1371/journal.pcbi.1006418
Chen, X., Zhu, C.-C., and Yin, J. (2019b). Ensemble of decision tree reveals potential miRNA-disease associations. PLoS Comput. Biol. 15:e1007209. doi: 10.1371/journal.pcbi.1007209
Ding, Y., Tang, J., and Guo, F. (2019). Identification of drug-side effect association via multiple information integration with centered kernel alignment. Neurocomputing 325, 211–224. doi: 10.1016/j.neucom.2018.10.028
Ferreira, H. J., and Esteller, M. (2018). Non-coding RNAs, epigenetics, and cancer: tying it all together. Cancer Metas. Rev. 37, 55–73. doi: 10.1007/s10555-017-9715-8
Ge, E., Yang, Y., Gang, M., Fan, C., and Zhao, Q. (2019). Predicting human disease-associated circRNAs based on locality-constrained linear coding. Genomics 112, 1335–1342. doi: 10.1016/j.ygeno.2019.08.001
Geletu, M., Mohan, R., Arulanandam, R., Berger-Becvar, A., Nabi, I. R., Gunning, P. T., et al. (2018). Reciprocal regulation of the cadherin-11/Stat3 axis by caveolin-1 in mouse fibroblasts and lung carcinoma cells. Biochim. Biophys. Acta Mol. Cell Res. 1865, 794–802. doi: 10.1016/j.bbamcr.2018.02.004
He, D., Wang, X., Zhang, Y., Zhao, J., Han, R., and Dong, Y. (2019). DNMT3A/3B overexpression might be correlated with poor patient survival, hypermethylation and low expression of ESR1/PGR in endometrioid carcinoma: an analysis of the cancer genome atlas. Chin. Med. J. 132, 161–170. doi: 10.1097/CM9.0000000000000054
Hou, L.-K., Ma, Y.-S., Han, Y., Lu, G.-X., Luo, P., Chang, Z.-Y., et al. (2017). Association of microRNA-33a molecular signature with non-small cell lung cancer diagnosis and prognosis after chemotherapy. PLoS ONE 12:e0170431. doi: 10.1371/journal.pone.0170431
Hu, H., Zhang, L., Ai, H., Zhang, H., Fan, Y., Zhao, Q., et al. (2018). HLPI-ensemble: prediction of human lncRNA-protein interactions based on ensemble strategy. RNA Biol. 15, 797–806. doi: 10.1080/15476286.2018.1457935
Huang, Q. R., and Pan, X. B. (2019). Prognostic lncRNAs, miRNAs, and mRNAs form a competing endogenous RNA network in colon cancer. Front. Oncol. 9:712. doi: 10.3389/fonc.2019.00712
Jia, Y., Chen, L., Guo, S., and Li, Y. (2019). Baicalin induced colon cancer cells apoptosis through miR-217/DKK1-mediated inhibition of Wnt signaling pathway. Mol. Biol. Rep. 46, 1693–1700. doi: 10.1007/s11033-019-04618-9
Kelley, K. A., Wieghard, N., Chin, Y., Potter, A., Mori, M., Wong, M. H., et al. (2018). MiR-486-5p downregulation marks an early event in colorectal carcinogenesis. Dis. Colon. Rectum. 61, 1290–1296. doi: 10.1097/DCR.0000000000001192
Kurozumi, S., Matsumoto, H., Hayashi, Y., Tozuka, K., Inoue, K., Horiguchi, J., et al. (2017). Power of PgR expression as a prognostic factor for ER-positive/HER2-negative breast cancer patients at intermediate risk classified by the Ki67 labeling index. BMC Cancer 17:354. doi: 10.1186/s12885-017-3331-4
Lang, B., and Zhao, S. (2018). miR-486 functions as a tumor suppressor in esophageal cancer by targeting CDK4/BCAS2. Oncol. Rep. 39, 71–80. doi: 10.3892/or.2017.6064
Lewis, B. P., Burge, C. B., and Bartel, D. P. (2005). Conserved seed pairing, often flanked by adenosines, indicates that thousands of human genes are microRNA targets. Cell 120, 15–20. doi: 10.1016/j.cell.2004.12.035
Li, C., Zheng, X., Li, W., Bai, F., Lyu, J., and Meng, Q. H. (2018a). Serum miR-486-5p as a diagnostic marker in cervical cancer: with investigation of potential mechanisms. BMC Cancer 18:61. doi: 10.1186/s12885-017-3753-z
Li, F., Li, Q., and Wu, X. (2018b). Construction and analysis for differentially expressed long non-coding RNAs and MicroRNAs mediated competing endogenous RNA network in colon cancer. PLoS ONE 13:e0192494. doi: 10.1371/journal.pone.0192494
Li, H., Mou, Q., Li, P., Yang, Z., Wang, Z., Niu, J., et al. (2019). MiR-486-5p inhibits IL-22-induced epithelial-mesenchymal transition of breast cancer cell by repressing Dock1. J. Cancer 10, 4695–4706. doi: 10.7150/jca.30596
Liu, H., Ren, G., Chen, H., Liu, Q., Yang, Y., and Zhao, Q. (2020). Predicting lncRNA–miRNA interactions based on logistic matrix factorization with neighborhood regularized. Knowled. Based Syst. 191:105261. doi: 10.1016/j.knosys.2019.105261
Ma, H., Tian, T., Liang, S., Liu, X., Shen, H., Xia, M., et al. (2016). Estrogen receptor-mediated miR-486-5p regulation of OLFM4 expression in ovarian cancer. Oncotarget 7, 10594–10605. doi: 10.18632/oncotarget.7236
Maierthaler, M., Benner, A., Hoffmeister, M., Surowy, H., Jansen, L., Knebel, P., et al. (2017). Plasma miR-122 and miR-200 family are prognostic markers in colorectal cancer. Int. J. Cancer 140, 176–187. doi: 10.1002/ijc.30433
Mao, Q., Quan, T., Luo, B., Guo, X., Liu, L., and Zheng, Q. (2016). MiR-375 targets KLF4 and impacts the proliferation of colorectal carcinoma. Tumor Biol. 37, 463–471. doi: 10.1007/s13277-015-3809-0
Mehta, A., and Patel, B. M. (2019). Therapeutic opportunities in colon cancer: focus on phosphodiesterase inhibitors. Life Sci. 230, 150–161. doi: 10.1016/j.lfs.2019.05.043
Rajendran, V. (2016). Structural analysis of oncogenic mutation of isocitrate dehydrogenase 1. Mol. BioSyst. 12, 2276–2287. doi: 10.1039/C6MB00182C
Rajendran, V., Gopalakrishnan, C., and Sethumadhavan, R. (2018). Pathological role of a point mutation (T315I) in BCR-ABL1 protein—a computational insight. J. Cell. Biochem. 119, 918–925. doi: 10.1002/jcb.26257
Rajendran, V., Purohit, R., and Sethumadhavan, R. (2012). In silico investigation of molecular mechanism of laminopathy caused by a point mutation (R482W) in lamin A/C protein. Amino Acids 43, 603–615. doi: 10.1007/s00726-011-1108-7
Ren, C., Chen, H., Han, C., Fu, D., Zhou, L., Jin, G., et al. (2016). miR-486-5p expression pattern in esophageal squamous cell carcinoma, gastric cancer and its prognostic value. Oncotarget 7, 15840–15853. doi: 10.18632/oncotarget.7417
Santasusagna, S., Moreno, I., Navarro, A., Muñoz, C., Martinez, F., Hernández, R., et al. (2018). miR-328 mediates a metabolic shift in colon cancer cells by targeting SLC2A1/GLUT1. Clin. Transl. Oncol. 20, 1161–1167. doi: 10.1007/s12094-018-1836-1
Sen, N., Satija, Yatendra K., and Das, S. (2011). PGC-1α, a key modulator of p53, promotes cell survival upon metabolic stress. Mol. Cell 44, 621–634. doi: 10.1016/j.molcel.2011.08.044
Seo, A. H., Moeng, S., Sim, S., Kuh, J. H., Choi, Y. S., and Park, K. J. (2019). MicroRNA-based combinatorial cancer therapy: effects of MicroRNAs on the efficacy of anti-cancer therapies. Cells 9:E29. doi: 10.3390/cells9010029
Shen, C., Ding, Y., Tang, J., Jiang, L., and Guo, F. (2019). LPI-KTASLP: Prediction of lncRNA-protein interaction by semi-supervised link learning with multivariate information. IEEE Access 7, 13486–13496. doi: 10.1109/ACCESS.2019.2894225
Sheng, S., Xie, L., Wu, Y., Ding, M., Zhang, T., and Wang, X. (2019). MiR-144 inhibits growth and metastasis in colon cancer by down-regulating SMAD4. Biosci. Rep. 39:BSR20181895. doi: 10.1042/BSR20181895
Siegel, R. L., Miller, K. D., Fedewa, S. A., Ahnen, D. J., Meester, R. G. S., Barzi, A., et al. (2017). Colorectal cancer statistics, 2017. 67, 177–193. doi: 10.3322/caac.21395
Su, J., Lu, E., Lu, L., and Zhang, C. (2017). MiR-29a-3p suppresses cell proliferation in laryngocarcinoma by targeting prominin 1. FEBS Open Bio. 7, 645–651. doi: 10.1002/2211-5463.12199
Sun, J., Zhao, H., Lin, S., Bao, S., Zhang, Y., Su, J., et al. (2019). Integrative analysis from multi-centre studies identifies a function-derived personalized multi-gene signature of outcome in colorectal cancer. J. Cell. Mol. Med. 23, 5270–5281. doi: 10.1111/jcmm.14403
Sundaram, P., Hultine, S., Smith, L. M., Dews, M., Fox, J. L., Biyashev, D., et al. (2011). p53-responsive miR-194 inhibits thrombospondin-1 and promotes angiogenesis in colon cancers. 71, 7490–7501. doi: 10.1158/0008-5472.CAN-11-1124
Tian, F., Wang, J., Ouyang, T., Lu, N., Lu, J., Shen, Y., et al. (2019). MiR-486-5p serves as a good biomarker in nonsmall cell lung cancer and suppresses cell growth with the involvement of a target PIK3R1. Front. Genet. 10:688. doi: 10.3389/fgene.2019.00688
Tian, Z.-Q., Li, Z.-H., Wen, S.-W., Zhang, Y.-F., Li, Y., Cheng, J.-G., et al. (2015). Identification of commonly dysregulated genes in non-small-cell lung cancer by integrated analysis of microarray data and qRT-PCR validation. Lung 193, 583–592. doi: 10.1007/s00408-015-9726-6
Tong, Z., Liu, N., Lin, L., Guo, X., Yang, D., and Zhang, Q. (2015). miR-125a-5p inhibits cell proliferation and induces apoptosis in colon cancer via targeting BCL2, BCL2L12 and MCL1. Biomed. Pharmacother. 75, 129–136. doi: 10.1016/j.biopha.2015.07.036
Truong, A. D., Rengaraj, D., Hong, Y., Hoang, C. T., Hong, Y. H., and Lillehoj, H. S. (2017). Differentially expressed JAK-STAT signaling pathway genes and target microRNAs in the spleen of necrotic enteritis-afflicted chicken lines. Res. Vet. Sci. 115, 235–243. doi: 10.1016/j.rvsc.2017.05.018
Wang, H.-Y., Wang, W., Liu, Y.-W., Li, M.-Y., Liang, T.-Y., Li, J.-Y., et al. (2017). Role of KCNB1 in the prognosis of gliomas and autophagy modulation. Sci. Rep. 7:14. doi: 10.1038/s41598-017-00045-7
Wang, L., Wei, Z., Wu, K., Dai, W., Zhang, C., Peng, J., et al. (2018). Long noncoding RNA B3GALT5-AS1 suppresses colon cancer liver metastasis via repressing microRNA-203. Aging 10, 3662–3682. doi: 10.18632/aging.101628
Wei, H. T., Guo, E. N., Liao, X. W., Chen, L. S., Wang, J. L., Ni, M., et al. (2018). Genomescale analysis to identify potential prognostic microRNA biomarkers for predicting overall survival in patients with colon adenocarcinoma. Oncol. Rep. 40, 1947–1958. doi: 10.3892/or.2018.6607
Wu, Q., Meng, W.-Y., Jie, Y., and Zhao, H. (2018). LncRNA MALAT1 induces colon cancer development by regulating miR-129-5p/HMGB1 axis. J. Cell. Physiol. 233, 6750–6757. doi: 10.1002/jcp.26383
Xia, L., Song, M., Sun, M., Chen, W., and Yang, C. (2019). miR-486 promotes capan-2 pancreatic cancer cell proliferation by targeting phosphatase and tensin homolog deleted on chromosome 10 (PTEN). Front. Genet. 10:541. doi: 10.3389/fgene.2019.00541
Xiao, C., and Rajewsky, K. (2009). MicroRNA control in the immune system: basic principles. Cell 136, 26–36. doi: 10.1016/j.cell.2008.12.027
Xu, J., Zhao, J., and Zhang, R. (2016). Four microRNAs signature for survival prognosis in colon cancer using TCGA data. Sci. Rep. 6:38306. doi: 10.1038/srep38306
Yang, W., Ma, J., Zhou, W., Li, Z., Zhou, X., Cao, B., et al. (2019). Identification of hub genes and outcome in colon cancer based on bioinformatics analysis. Cancer Manag. Res. 11, 323–338. doi: 10.2147/CMAR.S173240
Zhao, Q., Yang, Y., Ren, G., Ge, E., and Fan, C. (2019). Integrating bipartite network projection and KATZ measure to identify novel CircRNA-disease associations. IEEE Trans. NanoBiosci. 18, 578–584. doi: 10.1109/TNB.2019.2922214
Zhao, Q., Yu, H., Ming, Z., Hu, H., Ren, G., and Liu, H. (2018a). The bipartite network projection-recommended algorithm for predicting long non-coding RNA-protein interactions. Mol. Ther. Nucleic Acids 13, 464–471. doi: 10.1016/j.omtn.2018.09.020
Zhao, Q., Zhang, Y., Hu, H., Ren, G., Zhang, W., and Liu, H. (2018b). IRWNRLPI: integrating random walk and neighborhood regularized logistic matrix factorization for lncRNA-protein interaction prediction. Front. Genet. 9:239. doi: 10.3389/fgene.2018.00239
Zheng, Y., and Zhu, G. (2018). HMGB1 suppresses colon carcinoma cell apoptosis triggered by coculture with dendritic cells via an ER stressassociated autophagy pathway. Mol. Med. Rep. 17, 3123–3132. doi: 10.3892/mmr.2017.8202
Zhou, M., Hu, L., Zhang, Z., Wu, N., Sun, J., and Su, J. (2018a). Recurrence-associated long non-coding RNA signature for determining the risk of recurrence in patients with colon cancer. Mol. Ther. Nucleic Acids 12, 518–529. doi: 10.1016/j.omtn.2018.06.007
Zhou, M., Zhang, Z., Zhao, H., Bao, S., Cheng, L., and Sun, J. (2018b). An immune-related six-lncRNA signature to improve prognosis prediction of glioblastoma multiforme. Mol. Neurobiol. 55, 3684–3697. doi: 10.1007/s12035-017-0572-9
Zhou, M., Zhao, H., Xu, W., Bao, S., Cheng, L., and Sun, J. (2017). Discovery and validation of immune-associated long non-coding RNA biomarkers associated with clinically molecular subtype and prognosis in diffuse large B cell lymphoma. Mol. Cancer 16:16. doi: 10.1186/s12943-017-0580-4
Zhou, X. G., Huang, X. L., Liang, S. Y., Tang, S. M., Wu, S. K., Huang, T. T., et al. (2018c). Identifying miRNA and gene modules of colon cancer associated with pathological stage by weighted gene co-expression network analysis. Onco. Targets Ther. 11, 2815–2830. doi: 10.2147/OTT.S163891
Keywords: miRNA, hub gene, colon cancer, prognostic marker, bioinformatics
Citation: Zhu J, Xu Y, Liu S, Qiao L, Sun J and Zhao Q (2020) MicroRNAs Associated With Colon Cancer: New Potential Prognostic Markers and Targets for Therapy. Front. Bioeng. Biotechnol. 8:176. doi: 10.3389/fbioe.2020.00176
Received: 31 December 2019; Accepted: 20 February 2020;
Published: 10 March 2020.
Edited by:
Meng Zhou, Wenzhou Medical University, ChinaReviewed by:
Yi Xiong, Shanghai Jiao Tong University, ChinaYuangen Yao, Huazhong Agricultural University, China
Copyright © 2020 Zhu, Xu, Liu, Qiao, Sun and Zhao. This is an open-access article distributed under the terms of the Creative Commons Attribution License (CC BY). The use, distribution or reproduction in other forums is permitted, provided the original author(s) and the copyright owner(s) are credited and that the original publication in this journal is cited, in accordance with accepted academic practice. No use, distribution or reproduction is permitted which does not comply with these terms.
*Correspondence: Qi Zhao, zhaoqi@lnu.edu.cn
†These authors have contributed equally to this work