Cognitive Trajectories and Dementia Risk: A Comparison of Two Cognitive Reserve Measures
- 1Aging Research Center, Department of Neurobiology, Care Sciences and Society, Karolinska Institutet and Stockholm University, Stockholm, Sweden
- 2Centre for Cognition and Decision Making, Institute for Cognitive Neuroscience, National Research University Higher School of Economics, Moscow, Russia
- 3Centre for Neurolinguistics and Psycholinguistics, Vita-Salute San Raffaele University, Milan, Italy
- 4Stockholm Gerontology Research Center, Stockholm, Sweden
- 5The Swedish School of Sport and Health Sciences, GIH, Stockholm, Sweden
- 6Department of Medicine and Wisconsin Alzheimer’s Disease Research Center, University of Wisconsin School of Medicine and Public Health, Madison, WI, United States
- 7Division of Clinical Geriatrics, Department of Neurobiology, Care Sciences and Society, Karolinska Institutet, Stockholm, Sweden
Background and Objectives: Cognitive reserve (CR) is meant to account for the mismatch between brain damage and cognitive decline or dementia. Generally, CR has been operationalized using proxy variables indicating exposure to enriching activities (activity-based CR). An alternative approach defines CR as residual variance in cognition, not explained by the brain status (residual-based CR). The aim of this study is to compare activity-based and residual-based CR measures in their association with cognitive trajectories and dementia. Furthermore, we seek to examine if the two measures modify the impact of brain integrity on cognitive trajectories and if they predict dementia incidence independent of brain status.
Methods: We used data on 430 older adults aged 60+ from the Swedish National Study on Aging and Care in Kungsholmen, followed for 12 years. Residual-based reserve was computed from a regression predicting episodic memory with a brain-integrity index incorporating six structural neuroimaging markers (white-matter hyperintensities volume, whole-brain gray matter volume, hippocampal volume, lateral ventricular volume, lacunes, and perivascular spaces), age, and sex. Activity-based reserve incorporated education, work complexity, social network, and leisure activities. Cognition was assessed with a composite of perceptual speed, semantic memory, letter-, and category fluency. Dementia was clinically diagnosed in accordance with DSM-IV criteria. Linear mixed models were used for cognitive change analyses. Interactions tested if reserve measures modified the association between brain-integrity and cognitive change. Cox proportional hazard models, adjusted for brain-integrity index, assessed dementia risk.
Results: Both reserve measures were associated with cognitive trajectories [β × time (top tertile, ref.: bottom tertile) = 0.013; 95% CI: –0.126, –0.004 (residual-based) and 0.011; 95% CI: –0.001, 0.024, (activity-based)]. Residual-based, but not activity-based reserve mitigated the impact of brain integrity on cognitive decline [β (top tertile × time × brain integrity) = –0.021; 95% CI: –0.043, 0.001] and predicted 12-year dementia incidence, after accounting for the brain-integrity status [HR (top tertile) = 0.23; 95% CI: 0.09, 0.58].
Interpretation: The operationalization of reserve based on residual cognitive performance may represent a more direct measure of CR than an activity-based approach. Ultimately, the two models of CR serve largely different aims. Accounting for brain integrity is essential in any model of reserve.
Introduction
Aging is associated with gray- and white-matter lesions, atrophy, and functional disruptions that affect most areas of the brain (Walhovd et al., 2011). These changes have been linked to decline in several cognitive functions, as well as an increased risk of dementia (Frisoni et al., 2010; Kalpouzos and Nyberg, 2012; Lindenberger, 2014). However, a remarkable degree of inter-individual variability has been observed in the trajectories of cognitive decline and in the timing of dementia onset that cannot be accounted for by the brain parameters alone (Cosentino and Stern, 2019). Indeed, structural shrinkage, synaptic loss, and white matter degradation, are thought to be counteracted by the mechanisms that involve preservation, repair, or replenishment of neural resources (Cabeza et al., 2018).
A widely adopted model of resilience in cognitive aging and dementia (Nyberg et al., 2012) is that of cognitive reserve (Barulli and Stern, 2013; Stern et al., 2020) (CR). It assumes that some individuals are capable of coping with cognitive demands better than others in the face of brain-integrity loss (Stern, 2009). CR is presumed to act through two mechanisms: neural reserve, the efficiency or capacity of pre-existing functional brain networks; and neural compensation, the ability to use alternative cognitive strategies or neural pathways to circumvent deterioration (Steffener et al., 2011). Although the premise of CR has been generally accepted, operationalizing the construct has proven to be a challenge (Pettigrew and Soldan, 2019). The most commonly adopted approach to date has been to use prior stimulating experiences (most often education) as a proxy of reserve (Janse, 2012). Recently, this approach has been advanced in a life-course framework whereby, in addition to education, occupational complexity, social network, and engagement in leisure activities have been combined into a life-long indicator of CR (Wang et al., 2017; Dekhtyar et al., 2019). In line with this work, a scale for assessing cognitive reserve that incorporates many suspected contributors from different life stages has been developed (Nucci et al., 2012), validated (León et al., 2014), and adapted to several contexts (Maiovis et al., 2016; Altieri et al., 2018).
However, relying on recollected historical accounts of prior stimulating activities, which are at best an indirect proxy of reserve, may also introduce the risk of reverse causation and recall bias. Therefore, a more direct measure of CR that is also not dependent on self-reported information is highly warranted. An alternative approach has been suggested in which reserve is defined as the discrepancy between expected cognitive performance, given the level of brain integrity, and actual performance (Reed et al., 2010). Characterized as residual variance in cognitive performance, not explained by individual neuropathology and demographics, this operationalization has been suggested by some to offer a more precise measurement of reserve (Bocancea et al., 2021). The residual-based measure of CR, initially developed in a clinical sample of AD patients, has rarely been utilized in population-based aging cohorts. Importantly, it remains to be compared with a conventional operationalization of CR based on lifelong experiences, in its capacity to predict cognitive trajectories, as well as incident dementia.
In this study we aim to (1) compare residual-based and activity-based measures of CR in their association with cognitive trajectories, (2) assess the two measures of reserve in their ability to modulate the association between brain integrity and cognitive change trajectories, and (3) investigate if residual-based and activity-based CR are associated with dementia incidence after accounting for the levels of brain integrity.
Materials and Methods
Participants
Participants in this population-based cohort study were from the Swedish National Study on Aging and Care in Kungsholmen (SNAC-K), a community-based, longitudinal cohort study of adults aged 60+ years, living at home or in an institution in the Kungsholmen district of Stockholm (Lagergren et al., 2004). SNAC-K participants were randomly selected from 11 age cohorts (60, 66, 72, 78, 81, 84, 87, 90, 93, 96, and 99+ years). The younger age cohorts (60–72 years) were re-examined every 6 years, whereas the older cohorts (78+ years) were followed up every 3 years. At baseline (March 2001–August 2004), 3,363 of the 4,590 eligible individuals (73.3%) underwent examination. The SNAC-K magnetic resonance imaging (MRI) subsample (n = 555) included participants who were non-institutionalized and free from dementia and disability, recruited between September 2001 and October 2003 (Ferencz et al., 2013). Of the 555 participants, 125 were excluded due to incompleteness or suboptimal quality of MRI data (n = 43), presence of neurological or psychiatric diseases (n = 64), questionable dementia (n = 5), or missing cognition data (n = 13) at baseline, resulting in 430 subjects eligible for inclusion (see Supplementary Figure 1 for flowchart). In this study, we used follow-up data on cognition and dementia from four waves after the baseline assessment, resulting in a mean follow-up of 12 years. SNAC-K was approved by the Regional Ethical Review Board in Stockholm and written informed consent was obtained from participants or their next of kin.
Cognitive Assessment
At baseline and at each follow-up wave, participants were administered a cognitive test battery according to a standardized procedure (Laukka et al., 2020). From the cognitive battery, five domains were available: perceptual speed [digit cancellation (Zazzo, 1974) and pattern comparison (Salthouse and Babcock, 1991)], episodic memory [word recall and word recognition (Laukka et al., 2013)], semantic memory (Dureman, 1960; Nilsson et al., 1997), letter fluency (A and F), and category fluency (animals and professions). For a more detailed description of the cognitive battery, see Laukka et al. (2013). In the analysis of cognitive trajectories, we used a composite index of cognitive performance computed as the average of z-scores for the domains of perceptual speed, semantic memory, letter fluency, and category fluency. A composite score of episodic memory was used in the operationalization of residual-based CR (see below).
In addition to a cognitive test battery, we also extracted information on Mini-Mental State Examination (MMSE), which was available at baseline and across all follow-up examinations. MMSE is a widely used, easy-to-administer, 30-item screening questionnaire, assessing various aspects of cognitive functioning, including temporal and spatial awareness, memory, language, and arithmetic (Folstein et al., 1975).
Dementia Diagnosis
Dementia was clinically diagnosed according to DSM-IV criteria. A three-step procedure was employed, where two physicians working independently made a preliminary diagnosis and a third opinion was sought from the senior neurologist in the event of discordant assessments (Fratiglioni et al., 1997). For participants who died prior to follow-up assessment and did not receive a clinical diagnosis, dementia was ascertained through hospital records, hospital discharge registers, and death certificates.
Neuroimaging Measures
Images were acquired with a Philips Intera 1.5T MRI scanner. The MRI protocol included an axial 3D T1-weighted fast-field-echo sequence (time of repetition (TR) 15 ms, time to echo (TE) 7 ms, flip angle (FA) 15°, field of view (FOV) 20, matrix 256 × 256), a fluid-attenuated inversion recovery (FLAIR) sequence (TR 6,000 ms, TE 100 ms, inversion time 1,900 ms, FA 90°, echo train length 21, FOV 184 × 230, matrix 204 × 256), and a proton density/T2-weighted fast-spin-echo sequence (TR 4,000 ms, TE 18/90 ms, FA 90°, echo train length 6, FOV 187.5 × 250, matrix 192 × 256, 5 mm slices, without the use of gap and angulation). Global white matter hyperintensities (WMH) volumes were manually drawn on FLAIR images and further interpolated on the corresponding T1-weighted images, to compensate for between-slices gap in FLAIR (intra-rater reliability assessed with Dice coefficient: 0.76, see Köhncke et al. (2016) for details). T1-weighted images were segmented into gray matter, white matter, and cerebrospinal fluid (CSF) using SPM12 in MATLAB R2012b (Statistical Parametric Mapping1), and subsequently visually inspected to check the quality of segmented images. Hippocampal volume (HCV) was extracted via an automated segmentation of the T1-weighted images (Fischl et al., 2002; Marseglia et al., 2019) using the Freesurfer 5.1 image analysis suite,2 and the lateral ventricular volume (LVV) was estimated via an automated segmentation performed with the ALVIN toolbox (Kempton et al., 2011). Number of lacunes, defined as round or ovoid fluid-filled cavities, 3–15 mm in diameter, consistent with a previous acute small deep brain infarct or hemorrhage in the territory of one perforating arteriole (Wardlaw et al., 2013), was assessed visually. The number and size of perivascular spaces (PVS) were also evaluated with a visual rating scale, and combined to derive a PVS score, as reported elsewhere (Laveskog et al., 2018). An index of brain integrity was computed using a structural equation model (SEM) by combining WMH volume, whole-brain gray matter volume (GMV), HCV, LVV, number of lacunes, and the PVS score (more estimation details in the secion “Statistical Analysis”).
Cognitive Reserve Measures
Residual-Based Cognitive Reserve
Residual-based CR was defined as the discrepancy between observed and predicted levels of cognitive functioning, given the extent of observed brain integrity, and further accounting for age and sex. Consistent with previous work on residual-based CR (Reed et al., 2010; Zahodne et al., 2013), we used episodic memory as the index domain from which to derive reserve, as it is markedly impaired in aging. We fitted a linear regression model in which episodic memory performance was the dependent variable, whereas the observed level of brain integrity, based on the latent index derived from SEM (see section “Statistical Analysis”), was the independent variable; age and sex were included as covariates. From this linear model, we computed the residuals, the difference between observed and predicted levels of cognitive performance for each individual, which constituted our measure of residual-based CR.
Activity-Based Lifelong Cognitive Reserve
Activity-based CR incorporated four life experiences, hypothesized to contribute to the development of CR: Early-life education, midlife substantive work complexity, late-life leisure activities, and late-life social network. Information on life experiences was obtained from a nurse interview and accompanying questionnaires at the SNAC-K baseline assessment (Dekhtyar et al., 2019).
Statistical Analysis
Deriving Cognitive Reserve Measures
We first used SEM to compute a latent brain-integrity index from six neuroimaging measures: WMH volume, whole-brain GMV, HCV, LVV, PVS score, and number of lacunes; age and sex were included as covariates. All volumetric measurements were corrected by the total intracranial volume (ICV) (Jack et al., 1989). Maximum likelihood with missing values (MLMV) estimation was used to estimate the model. Model fit was assessed using conventional criteria. Omitted paths were explored using modification indices and predicted values of the latent brain-integrity index were extracted. Next, we fitted a linear regression model with episodic memory score as the dependent variable and the latent brain-integrity index, sex, and age as independent variables. From this model, we calculated the residuals, which constituted the individual measure of residual-based CR. In the analyses, we used residual-based CR (mean: 0, range: –2.65, 2.23) both as continuous and categorical variable (tertile operationalization: low, moderate, and high reserve).
Activity-based CR measure was obtained using SEM that extracted a common latent factor from four stimulating life experiences: early life education, midlife substantive work complexity, late life leisure activities, and late life social network. A value of the latent variable was predicted for each individual, and the resulting continuous variable, activity-based CR, was approximately normally distributed with a mean of 0 (range: –3.28, 2.91). For the analyses, activity-based CR was considered continuously and categorically as tertiles.
Predicting Longitudinal Trajectories of Cognition
Next, we tested whether the two CR measures were associated with cognitive change over 12 years of follow-up. Cognitive trajectories were assessed using separate linear mixed-effects models with maximum-likelihood estimation, including the following factors as fixed effects: age, sex, follow-up time, brain-integrity index, CR indicator (residual-based or activity-based), alongside an interaction term for CR and time. Random effects for individual intercepts and slopes over time were also included. Predicted margins of cognitive trajectories were computed from the model using a tertile operationalization of CR.
Assessing CR as a Modulator of the Impact of Brain Integrity on Cognition
We investigated the role of the CR measures in modulating the relationship between the brain-integrity index and cognitive trajectories over time, by fitting two linear mixed-effects regression models (one for residual-based CR, the other for activity-based CR) with the composite cognitive score as the dependent variable. Independent variables included main effects of age, sex, follow-up time, brain-integrity index, and corresponding CR indicator, as well as the three-way interaction among brain-integrity index, the corresponding CR measure, and time.
Investigating Dementia Incidence in Relation to Two CR Measures
Cox proportional hazard models were used to assess the relative risk of dementia over 12 years in relation to the two CR measures. Separate models were estimated for activity-based and residual-based CR, and both included controls for age, sex, and brain-integrity index. Proportionality assumption was tested using Schoenfeld residuals. Follow-up time was computed as time since baseline until dementia diagnosis, death, or the last examination.
Sensitivity Analyses
In addition to cognitive test score trajectories, we also examined MMSE change over time. The reason for this analysis was to mitigate potential circularity bias, whereby residual-based CR was derived from a cognitive domain (episodic memory) and was subsequently related to a set of cognitive domains, which themselves may be correlated with the one used in the derivation of CR. Notably, the correlation between episodic memory at baseline and the composite score of four cognitive domains was only 0.35 when averaged over the follow-up (correlation between baseline episodic memory and mean MMSE over the follow-up was 0.31). Using MMSE also increased statistical power, as it had better coverage over the follow-up than cognitive assessment. In another sensitivity analysis, we derived residual CR using performance in all five cognitive domains, rather than just episodic memory, and related this index with MMSE change and dementia incidence over time. This ensured that a full spectrum of cognitive performance was considered both in the derivation of CR and in the outcome analysis, while circularity was mitigated by using different families of tests and outcomes.
Data Availability
Data are from the Swedish National Study on Aging and Care in Kungsholmen (SNAC-K3). Applications for data use can be submitted at https://www.snac-k.se/application/registration.php. For more information, contact Maria Wahlberg (Maria.Wahlberg@ki.se) at the Aging Research Center, Karolinska Institute.
Results
Baseline characteristics of the study population are presented in Table 1. Individuals with higher scores on residual-based CR were on average more educated, had higher work complexity, larger social network, and more intact cognitive functioning (measured with MMSE).
Deriving Cognitive Reserve Measures
To derive residual-based CR, we first constructed a latent index of brain integrity. The best-fitting SEM for the brain-integrity index is presented in Figure 1. The model fit the data well (CFI = 0.964; TLI = 0.939; RMSEA = 0.07). The factor loadings were highest for GMV and HCV, followed by WMH and LVV, and lowest for lacunes and PVS score. A value of the latent brain-integrity index was predicted for each individual. The resulting continuous variable was used as independent variable in the regression in which episodic memory was the dependent variable, and age and sex were included as covariates. The brain-integrity index was positively related to episodic memory performance (p < 0.01) and the residuals extracted from this model constituted the measure of residual-based CR. The correlation between the residual-based CR and the activity-based CR indicators was positive and statistically significant (r = 0.14; p = 0.004; see Supplementary Figure 2 for the SEM model used to derive activity-based CR).
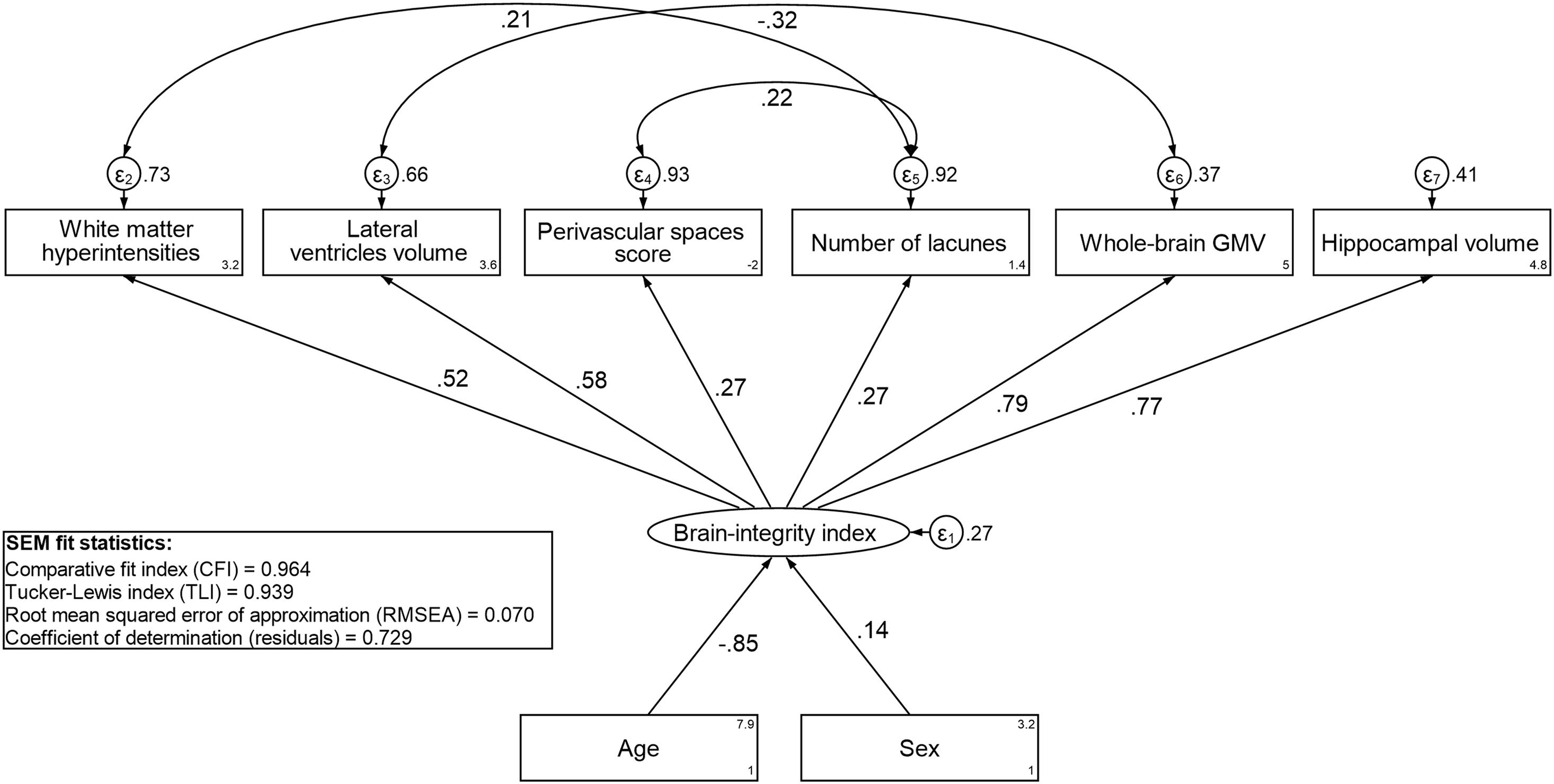
Figure 1. Standardized estimates from the best-fitting structural equation model (SEM) for the brain-integrity index.
Predicting Longitudinal Trajectories of Cognition
A continuous operationalization of residual-based CR did not yield an association with cognitive change trajectories (Table 2). Using a categorical operationalization of residual-based CR (tertile split) revealed that, relative to individuals in the lowest tertile of residual-based CR, those in the highest tertile exhibited slower cognitive decline over time (β for interaction with time: 0.013; p < 0.05). Predicted margins for cognitive trajectories according to tertiles of residual-based CR are presented in Figure 2A.
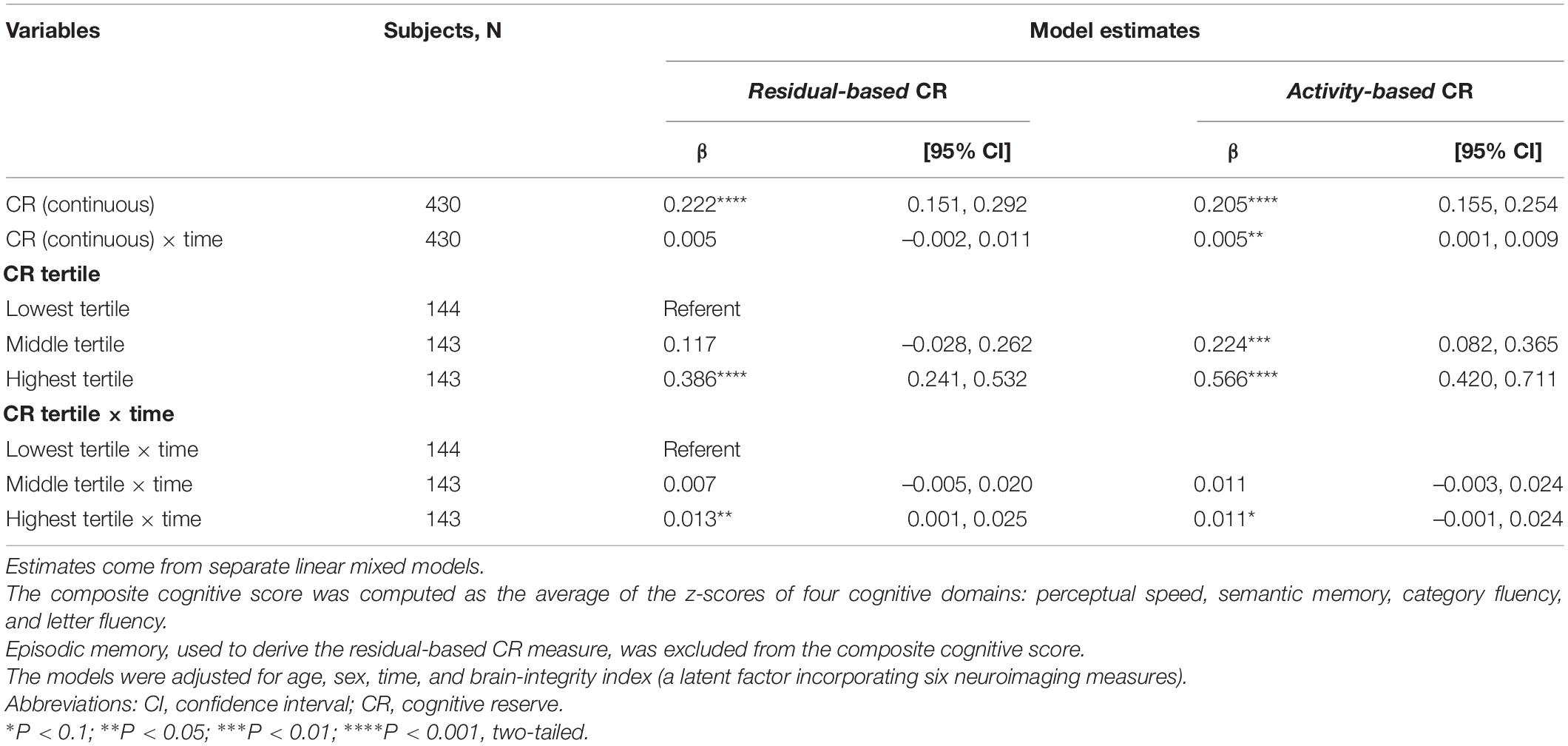
Table 2. Estimates from the linear mixed models predicting composite cognitive performance over time based on residual- and activity-based CR (continuous and in tertiles).
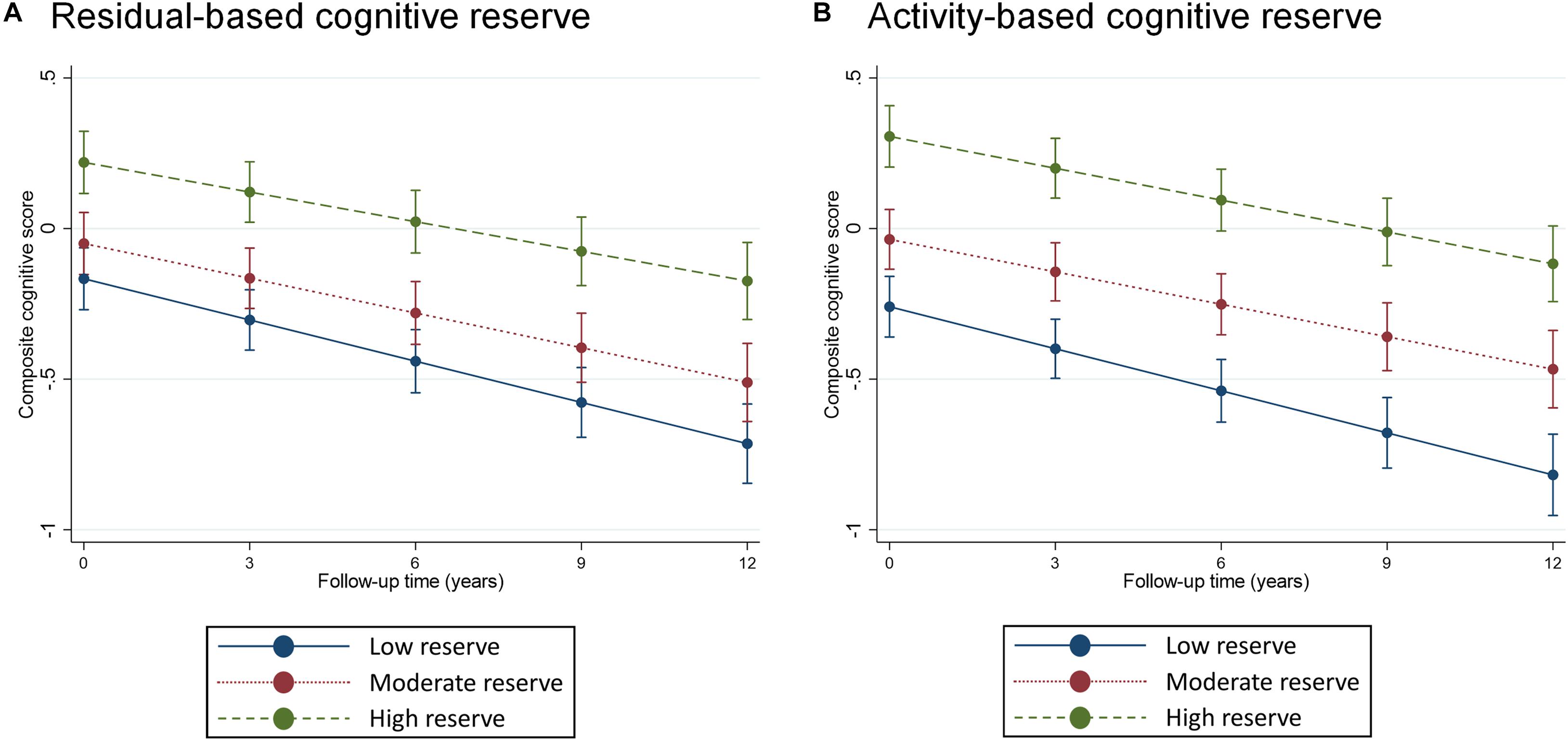
Figure 2. Predicted margins of cognitive change, measured using composite cognitive score, estimated separately for tertiles of residual-based (panel A) and activity-based (panel B) reserve. Predicted margins estimated from fully adjusted models presented in Table 2.
Activity-based CR exhibited a largely similar pattern of association with composite cognitive trajectories as did residual-based reserve. One difference was that, for activity-based CR, we also found a statistically significant association between a continuous operationalization and cognitive change (although the point estimate was identical to that of residual-based CR: β for interaction with time: 0.005, Table 2). Furthermore, relative to the bottom tertile, the top tertile of activity-based CR was only marginally associated with rate of cognitive decline (p = 0.08), although the point estimate (0.11) was quite similar to that of residual-based CR (0.13). Predicted margins for cognitive trajectories according to tertiles of activity-based CR are presented in Figure 2B.
Examining CR as Modulator in the Brain Integrity-Cognitive Change Association
Finally, we tested three-way interactions: CR (separately for activity- and residual-based) × brain-integrity index × time, to assess if CR modulated the impact of brain parameters on cognitive trajectories (Table 3). We found a statistically significant interaction among continuous residual-based CR and brain-integrity index over time (β = –0.011; p < 0.05), as well as a marginally statistically significant interaction among the top tertile of residual-based CR, brain-integrity index, and time (β = –0.021; p = 0.059; reference: low reserve × brain integrity × time). The margins plot of interaction (Figure 3) revealed that cognitive decline in response to impaired brain integrity was less pronounced in those with high residual-based CR than in those with low residual-based CR. In contrast, neither continuous nor categorical operationalizations of activity-based CR modulated the impact of brain integrity on composite cognitive trajectories.
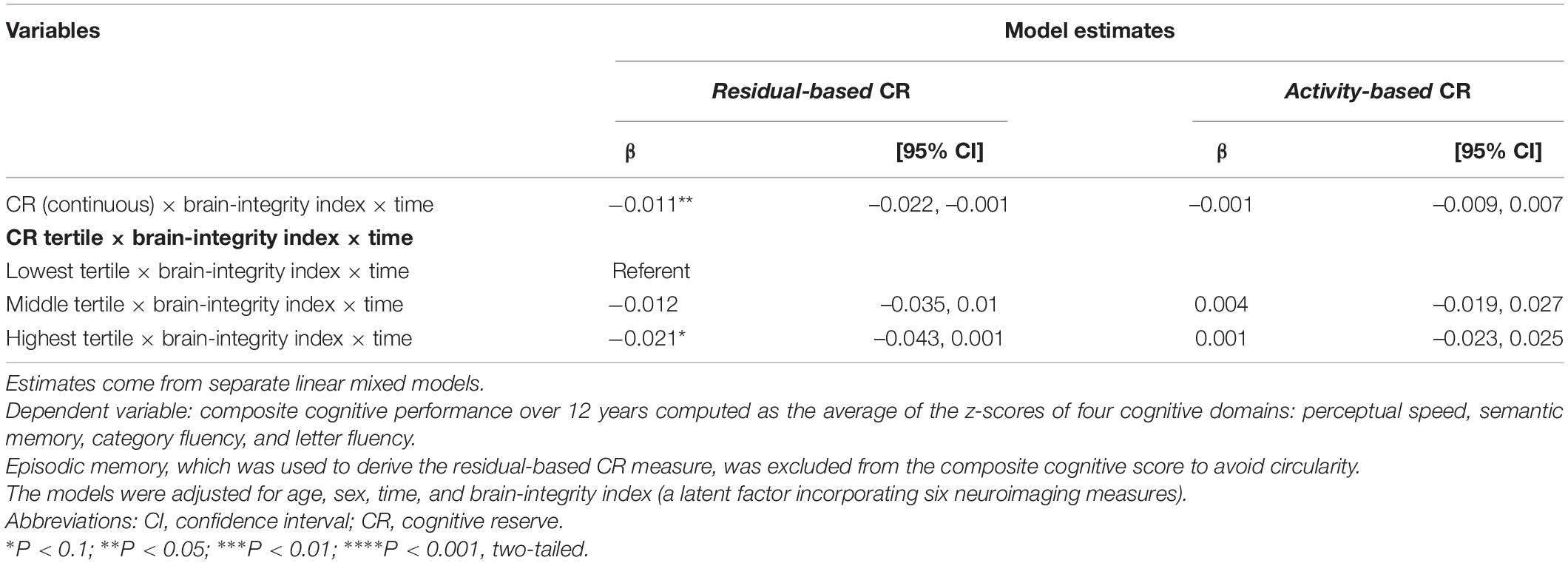
Table 3. Estimates from linear mixed models investigating three-way interactions among CR [estimated separately for residual- and activity-based CR (continuous and in tertiles)], brain-integrity index, and time.
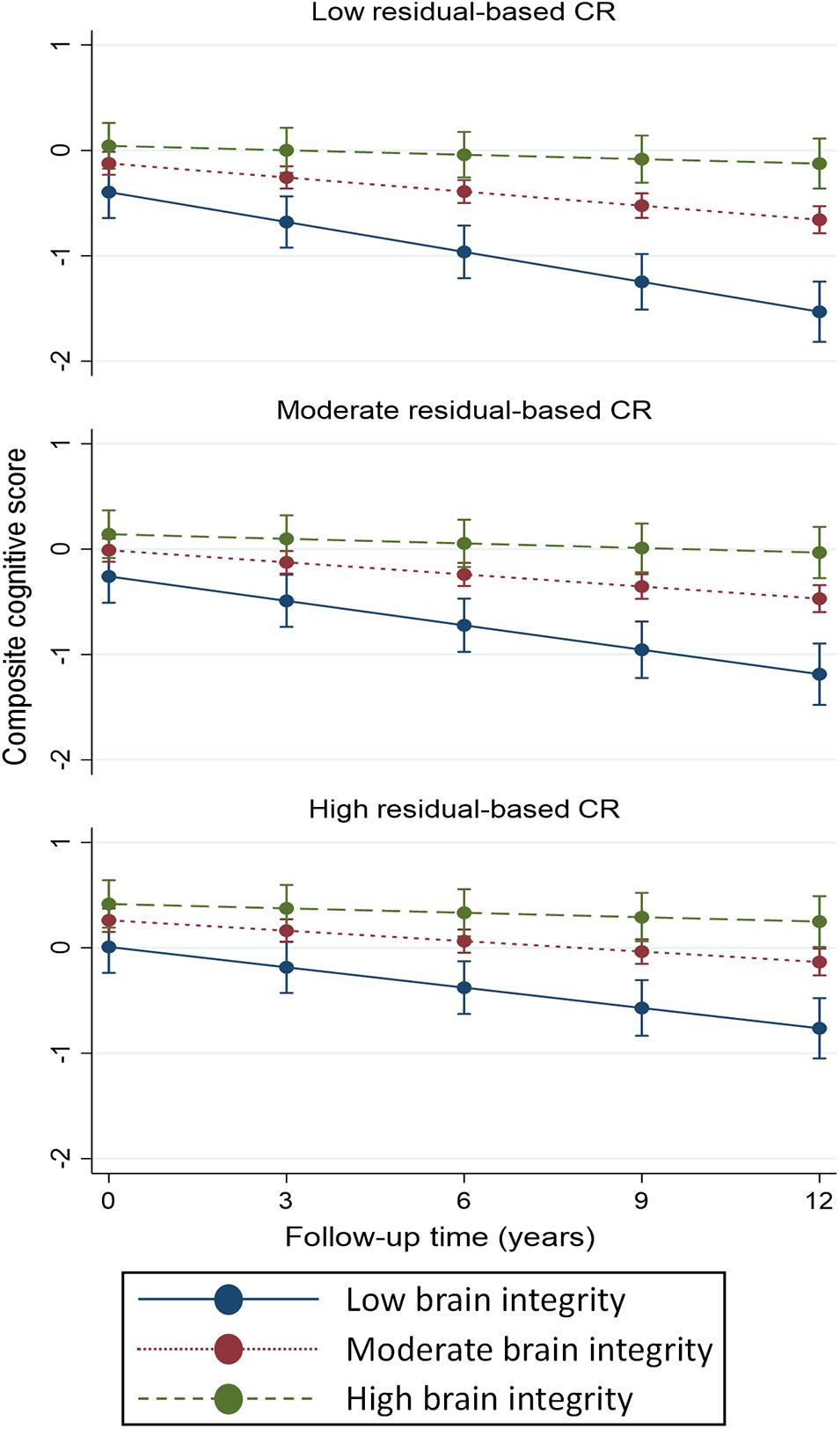
Figure 3. Predicted margins of cognitive change, based on composite cognitive score, in response to different levels of brain-integrity index, computed according to tertiles of residual-based CR. Predicted margins estimated from fully adjusted models presented in Table 3. Levels of brain-integrity index were defined as the 10th (low), 50th (moderate), and 90th (high) percentile.
Investigating Dementia Incidence in Relation to Two CR Measures
After a median of 11.5 years of follow-up (range: 0.76–15.7 years) of 419 participants (4218 person-years), 43 dementia cases were ascertained (incidence rate: 10.2 cases per 1000 person-years, 95% CI: 7.6–13.7). A continuous operationalization of residual-based CR was associated with a reduced hazard of dementia even after adjusting for the brain-integrity index (HR: 0.46; 95% CI: 0.33–0.66; Table 4). A model employing a tertile operationalization indicated that risk reduction was especially pronounced at the top third of residual-based CR (HR: 0.23; 95% CI: 0.09–0.58; reference: bottom tertile of residual-based CR, brain-integrity-adjusted model). The magnitude of risk reduction was less pronounced for activity-based CR and was no longer statistically significant upon further adjustment for the brain-integrity index. Proportionality assumption was not violated in any of the models.
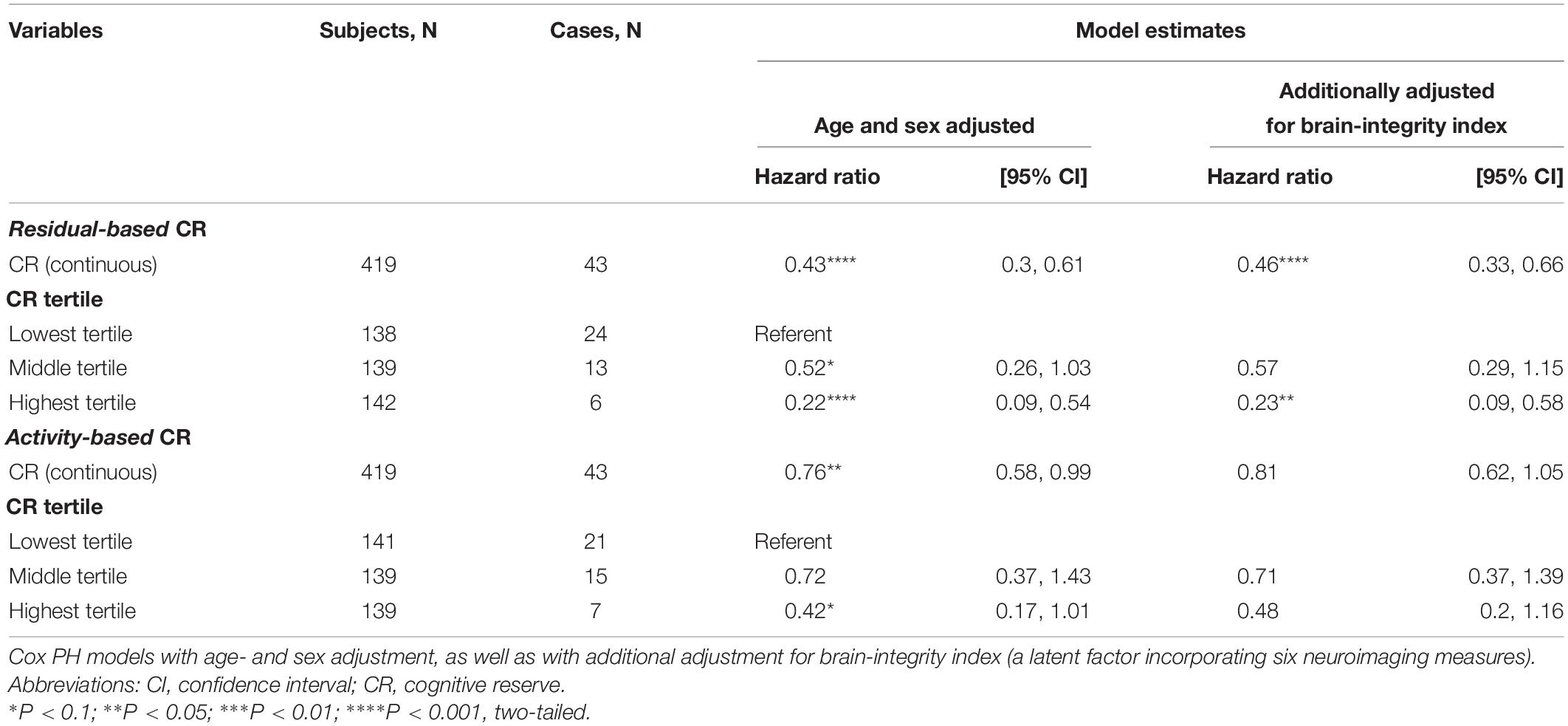
Table 4. Hazard ratios for dementia incidence over 12 years according to residual- and activity-based CR (continuous and in tertiles).
Sensitivity Analyses
Replacing composite cognitive performance with MMSE did not affect principal findings. Residual-based CR was associated with a slower decline in MMSE score over the follow-up (both continuously and categorically: top tertile vs. bottom). A similar pattern was observed for activity-based CR, although the middle tertile of activity-based CR also exhibited an association with MMSE change, relative to the lowest tertile (Supplementary Table 1 and Supplementary Figure 3).
In the modulation analysis using MMSE as outcome (Supplementary Table 2 and Supplementary Figure 4), a three-way interaction residual-based CR × brain-integrity-index × time was statistically significant using continuous operationalization (p < 0.05), and marginally significant using categorical operationalization (p = 0.06 for the middle tertile and p = 0.09 for the top tertile). Consistent with the original findings, no such modulation was observed when considering activity-based CR (either continuous or categorical).
Re-estimating MMSE trajectories and dementia incidence using residual performance in all cognitive domains, rather than just episodic memory, did not alter the main findings. This alternative composite-based residual CR exhibited a statistically significant association with MMSE change in both continuous and categorical operationalizations (Supplementary Table 3 and Supplementary Figure 5). Composite-based residual CR measure also modified the impact of brain integrity on MMSE trajectories: a three-way interaction CR × brain-integrity-index × time (p < 0.05) emerged for both continuous and categorical operationalizations (Supplementary Table 4 and Supplementary Figure 6). Finally, consistent with the original findings, dementia risk was reduced in those with higher scores on composite-based residual CR, even in models adjusted for the brain-integrity index (Supplementary Table 5).
Discussion
In this longitudinal population-based study of older adults, we found that a measure of cognitive reserve based on residual cognitive performance, unaccounted for brain integrity and sociodemographics, was associated with cognitive trajectories over a 12-year follow-up period. Furthermore, residual-based CR moderated the association between brain integrity and cognitive trajectories, such that in those with higher residual-based CR, the association between impaired brain integrity and cognitive decline was weakened compared to those with lower residual-based CR. Importantly, higher scores on residual-based CR were associated with 12-year hazard of dementia, even after accounting for brain-integrity levels. Conversely, although higher levels of activity-based CR were also associated with slower cognitive decline, this operationalization of CR neither modified the impact of brain integrity on the rate of cognitive change, nor was it associated with dementia occurrence net of the brain-integrity status.
Residual-based CR has been linked to cognitive trajectories in a handful of studies (Reed et al., 2010; Zahodne et al., 2013, 2015; Hohman et al., 2016; Habeck et al., 2017; Bettcher et al., 2019), and our findings are generally consistent with these earlier reports. The investigations with the most similar designs to the one reported here are Reed et al. (2010) and Zahodne et al. (2013). In a study of 305 adults varying in cognitive status, episodic-memory-based residual CR was linked to 3-year trajectories in executive function (Reed et al., 2010), whereas a study on 703 older adults, free from dementia at baseline, found differences in 3-year language ability trajectories according to residual-based CR (also derived using episodic memory) (Zahodne et al., 2013). Our study extends this literature in several important ways: (1) we examined cognitive trajectories over a prolonged follow-up period (12 years) in a population-based setting; (2) we were able to relate residual-based CR to a composite index of cognition based on measures of perceptual speed, verbal fluency, and semantic memory, as opposed to just executive function or language ability as used previously; (3) we extended the set of brain-integrity measures in deriving our residual-based measure of CR, incorporating WMH volume, whole-brain GMV, HCV, LVV, PVS, and lacunes; and (4) we examined 12-year dementia incidence in relation to residual-based CR. Incorporating MMSE trajectories and computing an alternative CR indicator based on residual performance in composite cognition, provided an important sensitivity test for the operationalization of residual-based CR adopted in this study.
A residual-based approach has been utilized in other studies, although they differ from ours in several important respects. One was based on cross-sectional data and derived residual-based CR from brain-integrity measures, without taking age into account (Habeck et al., 2017). Two other studies (Zahodne et al., 2015; Bettcher et al., 2019) examined changes in residual-based CR in relation to changes in cognition and brain atrophy. Finally, one study derived residual-based CR using cerebrospinal fluid markers of brain integrity (Hohman et al., 2016), although these findings were likely affected by circularity bias, as executive function and memory performance featured both in the derivation of CR measure and in the outcome analysis. Circularity might be a concern for our findings too, even though we excluded episodic memory from the outcome analysis. We aimed to further mitigate circularity through sensitivity analyses in which MMSE was used in place of cognition, as well as by analyzing dementia incidence. Notably, the correlation between episodic memory and composite cognitive performance over the follow-up was 0.35 (r = 0.31 for MMSE over time), suggesting that the bias due to circularity is unlikely to be considerable. Collectively, the present findings along with those from prior work discussed above highlight the utility of the residual-based approach in measuring cognitive reserve.
We made a further contribution by contrasting residual- and activity-based CR in the same study. Whereas both measures were associated with cognitive trajectories, only residual-based CR modified the brain integrity-cognitive change association and predicted dementia incidence after accounting for the brain-integrity levels. An apparent superior performance of residual-based CR is consistent with the findings of a recent meta-analysis that provided pooled estimates on MCI or dementia conversion across studies utilizing different reserve operationalizations (Nelson et al., 2021). The fact that activity-based CR did not yield modulatory or risk-reducing influences in our study could be due to the lack of precision in identifying reserve from diverse activities that may have differential contribution to the underlying construct. For instance, education or work complexity may affect cognitive outcomes through socioeconomic influences on health behaviors or access to material resources. These may in turn promote resistance to primary vascular pathologies, but ultimately play a lesser role in the resilience of cognitive function (Arenaza-Urquijo and Vemuri, 2018). Using a SEM-derived measure of activity-based reserve that focuses on common variance across all contributors while eliminating the variance that is unique to each one, has likely concealed their distinct influences. Specifically designed CR scales and questionnaires represent an alternative to SEM-based methods worthy of consideration in future studies (Nucci et al., 2012). We opted for a SEM-based measure here in order to (1) ensure comparability with our own and others’ prior work that also used SEM-based indexes of CR (Dekhtyar et al., 2019; Xu et al., 2019); (2) attenuate measurement error in observable factors contributing to CR, and (3) to integrate the impact of contributors not readily assessed in existing scales (e.g., social support).
Notably, the correlation between the two measures of reserve was weak (0.14), which compares to a correlation between residual-based CR and education of just 0.09 from a previous study (van Loenhoud et al., 2017). This suggests that the two CR measures likely incorporate distinct compensatory influences, and future studies ought to explore their unique neural bases. With respect to the seemingly limited relevance of activity-based reserve, the role of stimulating activities, particularly education, in age-related cognitive decline has been questioned in several studies (Berggren et al., 2018; Seblova et al., 2020). A recent comprehensive review concluded that cognitive stimulation likely relates to late-life cognition by affecting peak cognitive levels early in life, rather than the differential rates of decline during adulthood and aging (Lövdén et al., 2020). Although we documented an association between activity-based CR and cognitive trajectories and dementia in minimally adjusted models, the fact that it exhibited no mitigating effects against brain-integrity deterioration and lost its predictivity of dementia after brain integrity was accounted for, is in line with these recent appraisals of the role of stimulating activities.
Our findings provide input for the advancement of the CR theory, which continues to undergo considerable debate (Jones et al., 2011; Cabeza et al., 2018, 2019; Stern et al., 2019). On the one hand, we underscore the value of a residual-based approach which arguably offers a measure of reserve directly linked to its operational definition: the discrepancy between observed and expected cognitive performance, for a given level of brain integrity. However, residual-based CR can also be criticized for being dependent on the extent and quality of input parameters in the predictive model of cognition. In our study, it likely incorporated variance associated with unmeasured pathology that may affect episodic memory (notably: amyloid, tau, and TDP43), as well as the variance due to all other unobserved correlates of test performance. Thus, the association between this indicator and cognitive outcomes may not truly reflect reserve pathways, although it should be noted that a recent meta-analysis found residual-based CR to be associated with reduced progression to MCI or dementia, even in studies accounting for AD biomarkers (Nelson et al., 2021). Ultimately, residual- and activity-based operationalizations represent complementary approaches that can serve different aims. The former can be helpful for the prediction of future accelerated decline and dementia by identifying those with unexplained excessive cognitive deficits; information that can be especially useful for clinicians. The latter may give insight into how CR is formed in the first place, rather than providing its instantaneous measure. However, for either of these approaches to be consistent with the model of cognitive reserve, they must incorporate brain status in their operational definition; CR mechanisms should not be proposed when brain-integrity measures are not available at all, or are limited to a just a handful of markers that are insufficient to measure brain-integrity status (Habeck et al., 2017; Stern et al., 2020).
A strength of the present study is the longitudinal population-based design with long-term follow-up for cognition and dementia. The inclusion of a wide range of neuroimaging, cognitive, clinical, and life-experience measures is a further strength. Limitations include potential selectivity of healthier participants in the SNAC-K MRI subsample, which likely led to an underestimation of reported associations. Higher resolution MRI scans (as opposed to the 1.5T images used here) could have yielded a more detailed assessment of brain-integrity status, improving the specificity of our findings. By focusing on composite assessments, we strived for a holistic view of both brain integrity and cognition, which may have obscured important fine-grain detail, that ought to be explored further. For instance, the differential factor loadings of HCV (high), WMH (moderate), and PVS (low) to the brain-integrity index reported here, deserve attention in future studies looking to derive more specific measures of reserve. The absence of assessments of leisure participation and social network from before late life could also be a limitation. Finally, we used a more conventional regression-based technique to derive residual-based CR as opposed to SEM (Reed et al., 2010), and our residual estimate likely contain more measurement error. A linear regression approach, however, has been shown to be a reliable alternative to a latent-variable operationalization in a previous study (Zahodne et al., 2015).
Conclusion
In conclusion, we showed that residual-based CR derived in a population-based study of older adults was (1) associated with cognitive trajectories over 12 years of follow-up, (2) mitigated the impact of impaired brain integrity on cognitive decline, and (3) predicted dementia incidence even after accounting for brain-integrity status. In contrast, an activity-based measure derived from stimulating life experiences neither mitigated the brain integrity-cognitive change association, nor did it emerge as a predictor of dementia independent of brain integrity. Our findings provide insight into future applications of CR models of cognitive change and dementia. Both approaches possess unique advantages that can be tailored to address different aims. Ultimately, any model of CR needs to consider brain integrity, and the term reserve should be used when the measure in question modifies the link between brain integrity and cognitive outcomes or predicts dementia independent of brain integrity. In our study, only a residual-based measure of reserve fulfilled this requirement.
Data Availability Statement
The data analyzed in this study is subject to the following licenses/restrictions: Data are from the Swedish National Study on Aging and Care in Kungsholmen (SNAC-K; https://www.snac-k.se/). Data access is conditional on approval from the SNAC-K database committee. Applications for data use can be submitted at https://www.snac-k.se/application/registration.php. For more information, contact Maria Wahlberg (Maria.Wahlberg@ki.se) at the Aging Research Center, Karolinska Institute.
Ethics Statement
The studies involving human participants were reviewed and approved by Regional Ethical Review Board in Stockholm. The patients/participants provided their written informed consent to participate in this study.
Author Contributions
SD, FG, and LF: conception and design of the study. LF, GK, and EJL: acquisition of data. All authors: analysis or interpretation of data, drafting or revising the manuscript for intellectual content figures, contributed to the article, and approved the submitted version.
Funding
This work was supported by the funders of the Swedish National Study on Aging and Care: The Ministry of Health and Social Affairs, Sweden; the participating county councils and municipalities, the Swedish Research Council, and the Swedish Research Council for Health, Working Life, and Welfare are acknowledged. Specific grants are also acknowledged: The Basic Research Program of the National Research University Higher School of Economics (FG), and the Swedish Research Council for Health, Working Life, and Welfare (FORTE; Grant No. 2019-01076) (SD).
Conflict of Interest
The authors declare that the research was conducted in the absence of any commercial or financial relationships that could be construed as a potential conflict of interest.
Publisher’s Note
All claims expressed in this article are solely those of the authors and do not necessarily represent those of their affiliated organizations, or those of the publisher, the editors and the reviewers. Any product that may be evaluated in this article, or claim that may be made by its manufacturer, is not guaranteed or endorsed by the publisher.
Supplementary Material
The Supplementary Material for this article can be found online at: https://www.frontiersin.org/articles/10.3389/fnagi.2021.737736/full#supplementary-material
Footnotes
References
Altieri, M., Siciliano, M., Pappacena, S., Roldán-Tapia, M. D., Trojano, L., and Santangelo, G. (2018). Psychometric properties of the Italian version of the Cognitive Reserve Scale (I-CRS). Neurol. Sci. 39, 1383–1390. doi: 10.1007/s10072-018-3432-0
Arenaza-Urquijo, E. M., and Vemuri, P. (2018). Resistance vs resilience to Alzheimer disease: clarifying terminology for preclinical studies. Neurology 90, 695–703. doi: 10.1212/wnl.0000000000005303
Barulli, D., and Stern, Y. (2013). Efficiency, capacity, compensation, maintenance, plasticity: emerging concepts in cognitive reserve. Trends Cogn. Sci. 17, 502–509. doi: 10.1016/j.tics.2013.08.012
Berggren, R., Nilsson, J., and Lövdén, M. (2018). Education does not affect cognitive decline in aging: a bayesian assessment of the association between education and change in cognitive performance. Front. Psychol. 9:1138. doi: 10.3389/fpsyg.2018.01138
Bettcher, B. M., Gross, A. L., Gavett, B. E., Widaman, K. F., Fletcher, E., Dowling, N. M., et al. (2019). Dynamic change of cognitive reserve: associations with changes in brain, cognition, and diagnosis. Neurobiol. Aging 83, 95–104. doi: 10.1016/j.neurobiolaging.2019.08.016
Bocancea, D. I., Catharina van Loenhoud, A., Groot, C., Barkhof, F., van der Flier, W. M., and Ossenkoppele, R. (2021). Measuring resilience and resistance in aging and alzheimer disease using residual methods: a systematic review and meta-analysis. Neurology doi: 10.1212/WNL.0000000000012499
Cabeza, R., Albert, M., Belleville, S., Craik, F. I. M., Duarte, A., Grady, C. L., et al. (2019). Reply to ‘Mechanisms underlying resilience in ageing’. Nat. Rev. Neurosci. 20:247. doi: 10.1038/s41583-019-0139-z
Cabeza, R., Albert, M., Belleville, S., Craik, F. I. M., Duarte, A., Grady, C. L., et al. (2018). Maintenance, reserve and compensation: the cognitive neuroscience of healthy ageing. Nat. Rev. Neurosci. 19, 701–710. doi: 10.1038/s41583-018-0068-2
Cosentino, S., and Stern, Y. (2019). “Consideration of cognitive reserve,” in Handbook on the Neuropsychology of Aging and Dementia. Clinical Handbooks in Neuropsychology, eds L. Ravdin and H. Katzen (Cham: Springer), 11–23. doi: 10.1007/978-3-319-93497-6_2
Dekhtyar, S., Marseglia, A., Xu, W., Darin-Mattsson, A., Wang, H., and Fratiglioni, L. (2019). Genetic risk of dementia mitigated by cognitive reserve: a cohort study. Ann. Neurol. 86, ana.25501. doi: 10.1002/ana.25501
Ferencz, B., Laukka, E. J., Lövdén, M., Kalpouzos, G., Keller, L., Graff, C., et al. (2013). The influence of APOE and TOMM40 polymorphisms on hippocampal volume and episodic memory in old age. Front. Hum. Neurosci. 7:198. doi: 10.3389/fnhum.2013.00198
Fischl, B., Salat, D. H., Busa, E., Albert, M., Dieterich, M., Haselgrove, C., et al. (2002). Whole brain segmentation: automated labeling of neuroanatomical structures in the human brain. Neuron 33, 341–355. doi: 10.1016/S0896-6273(02)00569-X
Folstein, M. F., Folstein, S. E., and McHugh, P. R. (1975). “Mini-mental state”: a practical method for grading the cognitive state of patients for the clinician. J. Psychiatr. Res. 12, 189–198.
Fratiglioni, L., Viitanen, M., von Strauss, E., Tontodonati, V., Herlitz, A., and Winblad, B. (1997). Very old women at highest risk of dementia and Alzheimer’s disease: incidence data from the Kungsholmen Project, Stockholm. Neurology 48, 132–138. doi: 10.1212/wnl.48.1.132
Frisoni, G. B., Fox, N. C., Jack, C. R., Scheltens, P., and Thompson, P. M. (2010). The clinical use of structural MRI in Alzheimer disease. Nat. Rev. Neurol. 6, 67–77. doi: 10.1038/nrneurol.2009.215
Habeck, C., Razlighi, Q., Gazes, Y., Barulli, D., Steffener, J., and Stern, Y. (2017). Cognitive reserve and brain maintenance: orthogonal concepts in theory and practice. Cereb. Cortex 27, 3962–3969. doi: 10.1093/cercor/bhw208
Hohman, T. J., McLaren, D. G., Mormino, E. C., Gifford, K. A., Libon, D. J., and Jefferson, A. L. (2016). Asymptomatic Alzheimer disease. Neurology 87, 2443–2450. doi: 10.1212/WNL.0000000000003397
Jack, C. R., Twomey, C. K., Zinsmeister, A. R., Sharbrough, F. W., Petersen, R. C., and Cascino, G. D. (1989). Anterior temporal lobes and hippocampal formations: normative volumetric measurements from MR images in young adults. Radiology 172, 549–554. doi: 10.1148/radiology.172.2.2748838
Janse, E. (2012). A non-auditory measure of interference predicts distraction by competing speech in older adults. Aging Neuropsychol. Cogn. 19, 741–758. doi: 10.1080/13825585.2011.652590
Jones, R. N., Manly, J., Glymour, M. M., Rentz, D. M., Jefferson, A. L., and Stern, Y. (2011). Conceptual and measurement challenges in research on cognitive reserve. J. Int. Neuropsychol. Soc. 17, 593–601. doi: 10.1017/S1355617710001748
Kalpouzos, G., and Nyberg, L. (2012). “Multimodal neuroimaging in normal aging: structure–function interactions,” in Memory and Aging, eds M. Naveh-Benjamin and N. Ohta (London: Psychology Press), 285–316. doi: 10.4324/9780203156513-20
Kempton, M. J., Underwood, T. S. A., Brunton, S., Stylios, F., Schmechtig, A., Ettinger, U., et al. (2011). A comprehensive testing protocol for MRI neuroanatomical segmentation techniques: evaluation of a novel lateral ventricle segmentation method. Neuroimage 58, 1051–1059. doi: 10.1016/j.neuroimage.2011.06.080
Köhncke, Y., Laukka, E. J., Brehmer, Y., Kalpouzos, G., Li, T. Q., Fratiglioni, L., et al. (2016). Three-year changes in leisure activities are associated with concurrent changes in white matter microstructure and perceptual speed in individuals aged 80 years and older. Neurobiol. Aging 41, 173–186. doi: 10.1016/j.neurobiolaging.2016.02.013
Lagergren, M., Fratiglioni, L., Hallberg, I. R., Berglund, J., Elmståhl, S., Hagberg, B., et al. (2004). A longitudinal study integrating population, care and social services data. The Swedish National study on Aging and Care (SNAC). Aging Clin. Exp. Res. 16, 158–168. doi: 10.1007/BF03324546
Laukka, E. J., Köhncke, Y., Papenberg, G., Fratiglioni, L., and Bäckman, L. (2020). Combined genetic influences on episodic memory decline in older adults without dementia. Neuropsychology 34, 654–666. doi: 10.1037/neu0000637
Laukka, E. J., Lövdén, M., Herlitz, A., Karlsson, S., Ferencz, B., Pantzar, A., et al. (2013). Genetic effects on old-age cognitive functioning: a population-based study. Psychol. Aging 28, 262–274. doi: 10.1037/a0030829
Laveskog, A., Wang, R., Bronge, L., Wahlund, L. O., and Qiu, C. (2018). Perivascular spaces in old age: assessment, distribution, and correlation with white matter hyperintensities. Am. J. Neuroradiol. 39, 70–76. doi: 10.3174/ajnr.A5455
León, I., García-García, J., and Roldán-Tapia, L. (2014). Estimating cognitive reserve in healthy adults using the cognitive reserve scale. PLoS One 9:e102632. doi: 10.1371/journal.pone.0102632
Lindenberger, U. (2014). Human cognitive aging: corriger la fortune? Science 346, 572–578. doi: 10.1126/science.1254403
Lövdén, M., Fratiglioni, L., Glymour, M. M., Lindenberger, U., and Tucker-Drob, E. M. (2020). Education and cognitive functioning across the life span. Psychol. Sci. Public Interest 21, 6–41. doi: 10.1177/1529100620920576
Maiovis, P., Ioannidis, P., Nucci, M., Gotzamani-Psarrakou, A., and Karacostas, D. (2016). Adaptation of the Cognitive Reserve Index Questionnaire (CRIq) for the Greek population. Neurol. Sci. 37, 633–636. doi: 10.1007/s10072-015-2457-x
Marseglia, A., Fratiglioni, L., Kalpouzos, G., Wang, R., Bäckman, L., and Xu, W. (2019). Prediabetes and diabetes accelerate cognitive decline and predict microvascular lesions: a population-based cohort study. Alzheimers Dement. 15, 25–33. doi: 10.1016/j.jalz.2018.06.3060
Nelson, M. E., Jester, D. J., Petkus, A. J., and Andel, R. (2021). Cognitive reserve, alzheimer’s neuropathology, and risk of dementia: a systematic review and meta-analysis. Neuropsychol. Rev. 31, 233–250. doi: 10.1007/s11065-021-09478-4
Nilsson, L. G., Bäckman, L., Erngrund, K., Nyberg, L., Adolfsson, R., Bucht, G., et al. (1997). The betula prospective cohort study: memory, health, and aging. Aging Neuropsychol. Cogn. 4, 1–32. doi: 10.1080/13825589708256633
Nucci, M., Mapelli, D., and Mondini, S. (2012). Cognitive Reserve Index questionnaire (CRIq): a new instrument for measuring cognitive reserve. Aging Clin. Exp. Res. 24, 218–226. doi: 10.3275/7800
Nyberg, L., Lövdén, M., Riklund, K., Lindenberger, U., and Bäckman, L. (2012). Memory aging and brain maintenance. Trends Cogn. Sci. 16, 292–305.
Pettigrew, C., and Soldan, A. (2019). Defining cognitive reserve and implications for cognitive aging. Curr.Neurol. Neurosci. Rep. 19:1. doi: 10.1007/s11910-019-0917-z
Reed, B. R., Mungas, D., Farias, S. T., Harvey, D., Beckett, L., Widaman, K., et al. (2010). Measuring cognitive reserve based on the decomposition of episodic memory variance. Brain 133, 2196–2209. doi: 10.1093/brain/awq154
Salthouse, T. A., and Babcock, R. L. (1991). Decomposing adult age differences in working memory. Develop. Psychol. 27, 763–776. doi: 10.1037/0012-1649.27.5.763
Seblova, D., Berggren, R., and Lövdén, M. (2020). Education and age-related decline in cognitive performance: systematic review and meta-analysis of longitudinal cohort studies. Ageing Res. Rev. 58, 101005. doi: 10.1016/j.arr.2019.101005
Steffener, J., Reuben, A., Rakitin, B. C., and Stern, Y. (2011). Supporting performance in the face of age-related neural changes: testing mechanistic roles of cognitive reserve. Brain Imaging Behav. 5, 212–221. doi: 10.1007/s11682-011-9125-4
Stern, Y. (2009). Cognitive reserve. Neuropsychologia 47, 2015–2028. doi: 10.1016/j.neuropsychologia.2009.03.004
Stern, Y., Arenaza-Urquijo, E. M., Bartrés-Faz, D., Belleville, S., Cantilon, M., Chetelat, G., et al. (2020). Whitepaper: defining and investigating cognitive reserve, brain reserve, and brain maintenance. Alzheimers Dement. 16, 1305–1311. doi: 10.1016/j.jalz.2018.07.219
Stern, Y., Chételat, G., Habeck, C., Arenaza-Urquijo, E. M., Vemuri, P., Estanga, A., et al. (2019). Mechanisms underlying resilience in ageing. Nat. Rev. Neurosci. 20:246.
van Loenhoud, A. C., Wink, A. M., Groot, C., Verfaillie, S. C. J., Twisk, J., Barkhof, F., et al. (2017). A neuroimaging approach to capture cognitive reserve: application to Alzheimer’s disease. Hum. Brain Mapp. 38, 4703–4715. doi: 10.1002/hbm.23695
Walhovd, K. B., Westlye, L. T., Amlien, I., Espeseth, T., Reinvang, I., Raz, N., et al. (2011). Consistent neuroanatomical age-related volume differences across multiple samples. Neurobiol. Aging 32, 916–932.
Wang, H.-X., MacDonald, S. W. S., Dekhtyar, S., and Fratiglioni, L. (2017). Association of lifelong exposure to cognitive reserve-enhancing factors with dementia risk: a community-based cohort study. PLoS Med. 14:e1002251. doi: 10.1371/journal.pmed.1002251
Wardlaw, J. M., Smith, E. E., Biessels, G. J., Cordonnier, C., Fazekas, F., Frayne, R., et al. (2013). Neuroimaging standards for research into small vessel disease and its contribution to ageing and neurodegeneration. Lancet Neurol. 12, 822–838. doi: 10.1016/S1474-4422(13)70124-8
Xu, H., Yang, R., Qi, X., Dintica, C., Song, R., Bennett, D. A., et al. (2019). Association of lifespan cognitive reserve indicator with dementia risk in the presence of brain pathologies. JAMA Neurol. 76, 1184–1191. doi: 10.1001/jamaneurol.2019.2455
Zahodne, L. B., Manly, J. J., Brickman, A. M., Narkhede, A., Griffith, E. Y., Guzman, V. A., et al. (2015). Is residual memory variance a valid method for quantifying cognitive reserve? A longitudinal application. Neuropsychologia 77, 260–266. doi: 10.1016/j.neuropsychologia.2015.09.009
Zahodne, L. B., Manly, J. J., Brickman, A. M., Siedlecki, K. L., Decarli, C., and Stern, Y. (2013). Quantifying cognitive reserve in older adults by decomposing episodic memory variance: replication and extension. J. Int. Neuropsychol. Soc. 19, 854–862. doi: 10.1017/S1355617713000738
Keywords: cognitive reserve, dementia, cognitive change, life course, residual-based cognitive reserve, population-based cohort, structural MRI
Citation: Gallo F, Kalpouzos G, Laukka EJ, Wang R, Qiu C, Bäckman L, Marseglia A, Fratiglioni L and Dekhtyar S (2021) Cognitive Trajectories and Dementia Risk: A Comparison of Two Cognitive Reserve Measures. Front. Aging Neurosci. 13:737736. doi: 10.3389/fnagi.2021.737736
Received: 07 July 2021; Accepted: 26 July 2021;
Published: 25 August 2021.
Edited by:
Sara Palermo, Unità di Neuroradiologia, Carlo Besta Neurological Institute (IRCCS), ItalyReviewed by:
Manuela Altieri, University of Campania‘Luigi Vanvitelli, ItalyMargarida Sobral, Hospital Magalhães Lemos, Portugal
Copyright © 2021 Gallo, Kalpouzos, Laukka, Wang, Qiu, Bäckman, Marseglia, Fratiglioni and Dekhtyar. This is an open-access article distributed under the terms of the Creative Commons Attribution License (CC BY). The use, distribution or reproduction in other forums is permitted, provided the original author(s) and the copyright owner(s) are credited and that the original publication in this journal is cited, in accordance with accepted academic practice. No use, distribution or reproduction is permitted which does not comply with these terms.
*Correspondence: Serhiy Dekhtyar, serhiy.dekhtyar@ki.se