Pre-operative immune cell numbers and ratios are associated with peri-operative adverse outcomes in transfused patients
- 1Department of Anaesthesia, Royal Brisbane and Women’s Hospital, The University of Queensland, Brisbane, QLD, Australia
- 2Faculty of Medicine, The University of Queensland, Brisbane, QLD, Australia
- 3Faculty of Medicine, Princess Alexandra Hospital and Southern Clinical School, The University of Queensland, Brisbane, QLD, Australia
- 4Statistics Unit, Queensland Institute of Medical Research (QIMR) Berghofer, Brisbane, QLD, Australia
- 5Research and Development, Australian Red Cross Lifeblood, Brisbane, QLD, Australia
- 6Biomedical Science, School of Health, University of the Sunshine Coast, Brisbane, QLD, Australia
Background and objectives: Transfusion-related immune modulation (TRIM) and associated adverse outcomes during major surgery are increasingly important to patients and health services internationally. A panel of pre-operative blood tests is an essential part of the pre-operative anaesthetic assessment. This panel of blood tests commonly considers numbers of immune cells (i.e., lymphocytes, monocytes, and neutrophils and cell ratios) that may be used as biomarkers to evaluate and potentially predict post-operative adverse outcomes.
Design: This retrospective data collection from eight hospital databases, within the Royal Brisbane and Women's Hospital, considered only patients who received blood transfusion during surgery (2016–2018) (n = 2,121). The association between pre-operative immune cell numbers and ratios and adverse outcomes were assessed. Adverse outcomes were coded using the International Classification of Diseases-10 (ICD-10) coding which specifically considered transfusion-related immune modulation. Results were adjusted for confounding factors.
Results: After adjustment, decreased pre-operative lymphocyte numbers and increased neutrophil/lymphocyte ratio (NLR) were associated with increased odds of developing infection; decreased NLR with decreased odds of developing adverse renal outcomes; and decreased lymphocyte numbers with decreased odds of developing adverse cardiovascular outcomes. Monocyte numbers, neutrophil numbers, and the lymphocyte/monocyte ratio (LMR) were not associated with increased adverse outcomes after adjustment.
Conclusion: Pre-operative lymphocyte numbers and NLR are associated with adverse outcomes during peri-operative transfusion. Future assessment of peri-operative immune modulation should include the assessment of immune cell function and numbers.
1 Introduction
Peri-operative adverse outcomes hold serious implications for clinical patient care and the cost of healthcare services internationally (1). An estimated 310 million major surgeries occur in the world each year: 1%–4% of patients pass away, 15% experience serious adverse outcomes, and 5%–15% are readmitted within 30 days. A large 5-year study by Dencker et al. in the United States of America (2021, n = 5,880,829) identified a growing incidence of peri-operative adverse outcomes (2). Various outcomes were considered, including surgical site infection (superficial, deep and of internal organs), urinary tract infection, pneumonia, thrombophlebitis, deep venous thrombosis (DVT), myocardial infarction, cardiac arrest, pulmonary embolism, stroke, and sepsis. Surgical infection risk, potentially associated with immune modulation, has major implications worldwide (3, 4). Severe infection can lead to multi-organ failure, increased length of stay in hospital and the intensive care unit (ICU), and mortality. When the International Surgical Outcomes Study (ISOS) Group analysed surgical outcomes, they found 4,032 infections occurred in 2,927 patients (2,927/44,814, 6.5%), including superficial surgical-site infection (n = 1,320, 32.7%), pneumonia (n = 708, 17.6%), and urinary tract infection (n = 681, 16.9%) (5). We agree with Wan et al. that “Recognition of modifiable risk factors will help inform appropriate prevention strategies” and we therefore decided to conduct this study. Even though immune competency may currently not be modifiable it is essential to consider this additional important factor within future research. The ability to identify which patients could experience worse outcomes is becoming increasingly valued. An assessment of peri-operative immune competence may, therefore, provide valuable information.
Traditional research considered TRIM in the context of adverse outcomes related to infection and cancer recurrence (6). ABT is a risk factor for ventilator-associated pneumonia and infection in trauma patients (7, 8), and blood-stream and acquired nosocomial infection in critically ill patients (9, 10). ABT was a confounding factor during major cardiac surgery, where 1.5% of patients developed pneumonia and the mortality rate was 21% (11). When considering critically ill patients Taylor et al. reported a nosocomial infection rate of 14.3% in transfused and 5.8% in non-transfused patients (e.g., pneumonia, peritonitis, cystitis, pyelonephritis, urinary tract infection, bacteremia, etc.) (12). In addition to infection-related outcomes, TRIM is now used more broadly to include other adverse outcomes related to pro-inflammatory and immune modulatory pathways following ABT, including myocardial infarction, stroke, renal impairment, and respiratory complications (12–14). Furthermore, it was demonstrated that by using intraoperative cell salvage (ICS) as an alternative to ABT, many (previously mentioned) adverse outcomes are reduced or potentially avoided (15, 16).
There are important knowledge gaps within current literature. Firstly, we should consider the factors that may play a role in a patient's pre-operative immune capacity that may lead to post-operative adverse outcomes. A healthy immune response (cellular and humoral) is essential to ensure adequate protection against peri-operative infection (17). Peri-operative changes to the immune response are however complex and multi-factorial. The extent of tissue damage, type of surgery (17) surgical inflammation, hypotension, hypothermia (18), transfusion-related immune modulation (TRIM) (14, 19), anaesthetic technique (20, 21) and anaesthetic drugs may alter immune responses (22). Further confounding factors include pre-existing patient comorbidities (e.g., Diabetes mellitus), and immunosuppressive drugs (23). Other medications provided during anaesthesia, including analgesia and anti-emetics, may result in immune suppression. Corcoran et al. (in a landmark anaesthetic study) recently confirmed that dexamethasone (used for its anti-emetic properties) did not increase the incidence of surgical site infection (24). Other outcomes related to peri-operative immune modulation were not considered by Corcoran et al. Although exact mechanisms often remain unclear, many factors impair the normal immune response and increase the risk of developing surgical infection. Furthermore, in addition to infection-related consequences, altered immune responses hold further organ specific implications (e.g., cardiovascular, renal, liver, etc.) (25). A deeper understanding of the confounding factors and other outcomes potentially associated with peri-operative immune suppression is essential.
The mechanisms associated with altered immune responses (i.e., immune modulation) peri-operatively are also important considerations. The potential causes of TRIM have been studied extensively (14) and appear to be multifactorial. The precise mechanism(s) of TRIM remains largely undefined. This study is not designed to elicit the mechanisms mediating TRIM. Instead, we aim to find biomarkers associated with immune modulation. Such biomarkers can then be used during large clinical studies, sufficiently powered to evaluate factors and techniques that could improve outcomes in surgical patients. For example, even though adverse outcomes are potentially reduced following ICS (15, 16), due to the complex nature of ICS research, uncertainty related to the mechanism of TRIM and many confounding factors, large sample sizes are required to ensure robust statistical analysis. Biomarkers that are differentially altered, potentially associated with TRIM and feasible to measure in large sample sizes, would be invaluable during future research to identify the best transfusion modalities when considering clinical outcomes in patients.
The next requirement would be to identify methods that would enable the measurement of immune competency during peri-operative research. Various biomarkers have been studied to evaluate and describe the pathophysiology associated with immune suppression during surgery and anaesthesia (17). We do not propose that these cell numbers, used as biomarkers in our study, cause adverse outcomes as such, but they are instead considered to reflect changes in the immune response at a local and systemic level during peri-operative transfusion. The prognostic value of biomarkers were demonstrated, for example for lymphocyte/monocyte ratio (LMR) (26), platelet/lymphocyte ratio (PLR) and neutrophil/lymphocyte ratio (NLR) (27) during gastrointestinal cancer surgery; NLR, monocyte/lymphocyte (MLR) (28) and monocyte numbers (29) during lung cancer surgery; NLR and LMR (30) during pancreatic cancer surgery and human leukocyte antigen—DR isotype (HLA-DR) expression on monocytes during surgery for ruptured abdominal aortic aneurysm (31). A knowledge gap exists when considering peri-operative lymphocyte sub-populations, also considering NLR and LMR, and their association with the risk of developing infection following peri-operative transfusion. Using immune cell numbers and ratios as biomarkers to assess and potentially predict clinical outcomes associated with peri-operative transfusion may be valuable and relevant for many surgical procedures.
Filling these knowledge gaps would be the next step. Over decades many investigators used similar study methods to evaluate outcomes associated with TRIM. Even though these methods informed knowledge gaps, we still do not have a clear understanding of all the factors that may be associated with adverse outcomes during peri-operative transfusion. Our study team therefore evaluated different techniques and considered different biomarkers (that were previously not considered) for use in future research. Many laboratory techniques were previously used to assess altered immune responses and associated peri-operative adverse outcomes including whole blood assays (32), mass cytometry (20), flow cytometry (20, 33), gene arrays (34). These study techniques are often complex, time-consuming, and expensive and therefore not feasible within large sample sizes required to consider all the confounding factors relevant to TRIM research. The standard anaesthetic assessment before major surgery routinely includes a 5-part differential full blood count (FBC), where numbers of neutrophils (40%–60%), lymphocytes (B cells and T cells, 20%–40%), monocytes (2%–8%), eosinophils (1%–4%) and basophils (0.5%–1%) are measured (35). The routine nature of this 5-part differential cell count makes it a convenient monitor to use when studying peri-operative immune competence, immune responses and related clinical outcomes (20). We therefore aimed to evaluate the value of these commonly measured immune cells to monitor and potentially predict peri-operative adverse outcomes. The main objective of this study was to assess adverse outcomes considered to be associated with immune modulation (infection and other organ system-related outcomes) through analysis of International Classification of Diseases-10 (ICD-10) coded information.
2 Materials and methods
2.1 Study design
During this retrospective cohort study, at the Royal Brisbane and Women's Hospital (RBWH, a quaternary referral teaching hospital in Queensland, Australia), 2,121 patients received peri-operative transfusion (male n = 984, female n = 1,137, 2016–2018). An estimated 33,000 surgical procedures occur at the RBWH each year (only patients >12 years of age). Retrospective administrative data collected from eight electronic hospital databases (within the RBWH) were used, with ethics exemption from the RBWH Human Research Ethics Committee (HREC/16/QRBW/437).
A number of steps were considered to enable data collection: data collection plan, request approvals, request data from relevant data custodians, data retrieval, data combined, data connected (i.e., “linked”), correction (i.e., data cleaning), preparation for the next set of data, solutions to improve the efficiency of data collection (overcome some obstacles experienced), and plan again. Data associated with surgical procedures, transfusion and transfusion-related adverse outcomes are not held within one database at the RBWH. Each database is managed within the relevant department with different stakeholders, data ownership and related business rules. Communication between these databases is non-existent. Automated connection of data points is not enabled within the electronic patient record system. These data points (e.g., the timing of the specific procedure and the timing of the transfused blood products relevant to this procedure) were not connected and had to be linked within a software solution specifically designed for this project.
Data exported from eight hospital databases were combined within a novel Structured Query Language (SQL) database, developed for this project. This SQL database ensured each adverse outcome (ICD-10 code) analysed was related to the specific surgical procedure and relevant transfusion episode (i.e., date and time) (Table 1). One hundred and seventy-three individual ICD-10 coded outcomes were categorised within organ-specific groups (to enable statistical analysis), patient-specific comorbidities were added, and the best fit statistical model was identified. Sophisticated information technology support was essential at various stages to ensure data management and robust analysis of this large volume of data, with double checks for outliers, and multiple planned checks from within departments and at each stage of the data collection process.
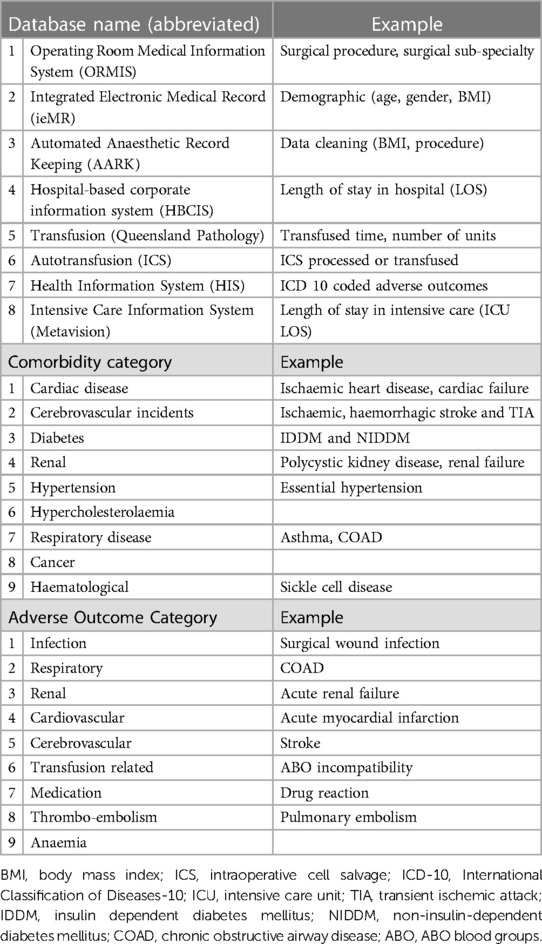
Table 1. Sources and examples of data collection: databases, comorbidity categories, and adverse outcome categories (adapted from the COSTOMICS study).
Exported data included the numbers of lymphocytes, monocytes, and neutrophils (Supplementary Table 1). Two ratios were created from the exported data: LMR and NLR. These cell numbers and ratios were used as biomarkers within this study. Pre-operative values (nearest to and mostly within 5 h before surgical start time, not older than 1 month) were available with FBC measurements performed as a standard part of clinical care.
2.2 Demographic
Socio-demographic and clinical data were collected to enable adjustment for confounding factors within the statistical model. Body Mass Index (BMI, kg/m2) was categorised as underweight (less than 18.5), normal weight (18.5–24.9), overweight (25–29.9), obese (30 or more) (36), and missing BMI data (comprising 17%).
2.3 Study outcomes assessed
Peri-operative adverse outcomes, using ICD-10 coded data (formally coded by professional coding staff), were extracted from the Health Information Systems (HIS) database (Table 1). Specific adverse outcomes that were previously identified when considering peri-operative transfusion were exported [i.e., ICD-10 codes for 173 different adverse outcomes categorised according to organ systems (Supplementary Table 2)], grouped within organ-specific categories and the number of patients experiencing an adverse outcome. Patients could experience more than one adverse outcome and adverse outcomes in more than one category. Furthermore, our analysis considered patients who received one of three modes of transfusion: ICS only (ICS), allogeneic packed red blood cells (pRBC) only (RBC) or allogeneic pRBC and ICS (RBC&ICS).
2.4 Statistical methods
Statistical analyses were performed using R version 4.1.1 (R Core Team, 2018, Vienna, Austria). Categorical variables were described using frequencies and percentages and analyzed using a chi-square test; Fisher's exact test was used when expected counts were small. Binary logistic regression was used to understand the association between adverse outcomes and biomarkers (lymphocyte-, monocyte-, neutrophil numbers, LMR and NLR), including adjustment for demographic variables and patient comorbidities.
Results were adjusted for patient comorbidities that were categorised according to relevant organ systems (i.e., cardiovascular, cerebrovascular, renal, respiratory, cancer and haematological conditions etc.) (Table 1). The number of comorbidities per patient was counted and patients were categorised as those with none, one, two or three or more comorbidities.
First univariate logistic models were performed for all variables. Multivariable models were created using a hybrid backward selection approach, where demographic variables were forced into the model. Biomarkers with P-values less than 0.2 were added to an initial multivariate model. Biomarkers were removed one at a time until only significant biomarkers remained. The final step was to further adjust the model in a separate block to assess the effect of the transfusion modality (i.e., ICS, RBC or RBC&ICS). Multicollinearity was assessed for the independent variables using a variance inflation factor. Due to non-linearity and outliers in the logistic models, the biomarkers were log base two transformed. A small constant of 0.01 was added to enable calculation of biomarkers when zeros were present. Descriptive statistics were reported as geometric (back-transformed) means and 95% confidence intervals. Pearson's correlation was used to explore the relationships between biomarkers.
3 Results
Between January 2016 and December 2018, 2,121 patients (male n = 984, female n = 1,137), receiving peri-operative blood transfusion at the RBWH were included in the study. The average age was 55 years, the mean BMI was 23.4 kg/m2, 1,936 patients received only allogeneic blood (RBC), 115 received only ICS (ICS) and 70 received both pRBCs and ICS (RBC&ICS). Pre-operative data for the biomarkers of interest were available for 2,087 of the 2,121 transfused study patients (Table 2). To improve clarity, we include a summary figure with significant results (Figure 1).
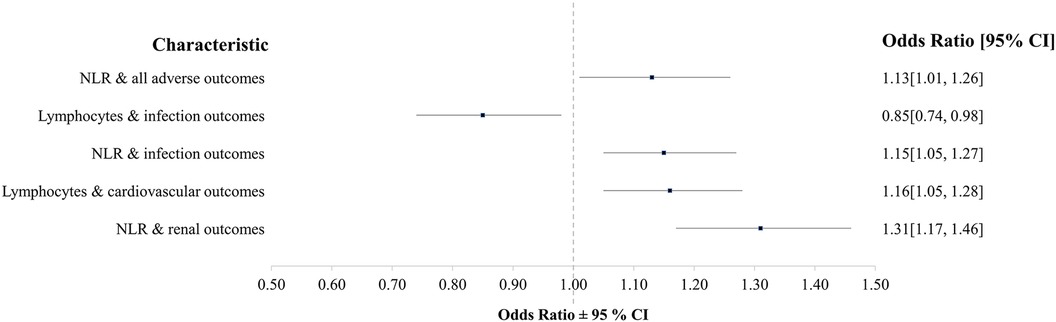
Figure 1. Summary of significant findings when considering the associations between organ-specific adverse outcomes and biomarkers in this study (NLR, neutrophil-lymphocyte-ratio).
3.1 Considering all adverse outcomes
We demonstrated that of the 2,087 patients who received transfusion and for whom we had biomarkers available, 1,882 patients (90.2%) experienced coded adverse outcomes; most commonly cardiovascular (n = 1,007, 48.3%), followed by post-operative infection (n = 502, 24.1%) (including other infections: wound infection, pneumonia, etc.), respiratory (n = 428, 20.5%) and adverse renal outcomes (n = 216, 10.3%) (Table 3). The incidence of cerebrovascular events (n = 21, 1%) and thromboembolic adverse outcomes (n = 47, 2.3%) were low.
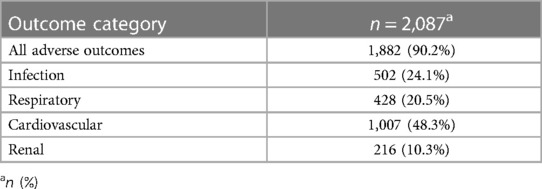
Table 3. Prevalence for adverse outcomes (numbers of patients experiencing adverse outcomes); considering organ-specific categories.
On average, patients experiencing adverse outcomes were older (mean 55.7 years; 95% CI: 54.8, 56.6) than patients who did not (mean 51.3 years; 95% CI: 48.5, 54.1; P = 0.002) (Supplementary Table 3). Adverse outcomes were more common in patients with lower lymphocyte numbers (mean 1.5; 95% CI: 1.4, 1.5; P = 0.045) and higher NLR (mean 4.6; CI: 4.3, 4.7; P = 0.061), compared to patients with higher lymphocyte numbers (mean 1.7; 95% CI: 1.5, 1.7) and lower NLR (mean 4.0; 95% CI: 3.5, 4.4). After adjustment for demographic variables in the multivariable model, NLR was significant (odds 1.13; 95% CI 1.01, 1.26; P = 0.035) with a 13% increase in odds with every doubling of NLR (Table 4). In the backward selection process, lymphocytes, age and comorbidities were no longer significant. In the final step, the model was adjusted by transfusion modality. This indicated greater odds of having any adverse outcome following RBC 5.71 (95% CI 3.63, 8.90; P < 0.001) and RBC&ICS 6.05 (95% CI 2.52, 17.0; P < 0.001) than ICS. After adjustment for transfusion modality, the NLR OR of 1.07 (95% CI 0.95, 1.20; P = 0.265) was no longer significant.
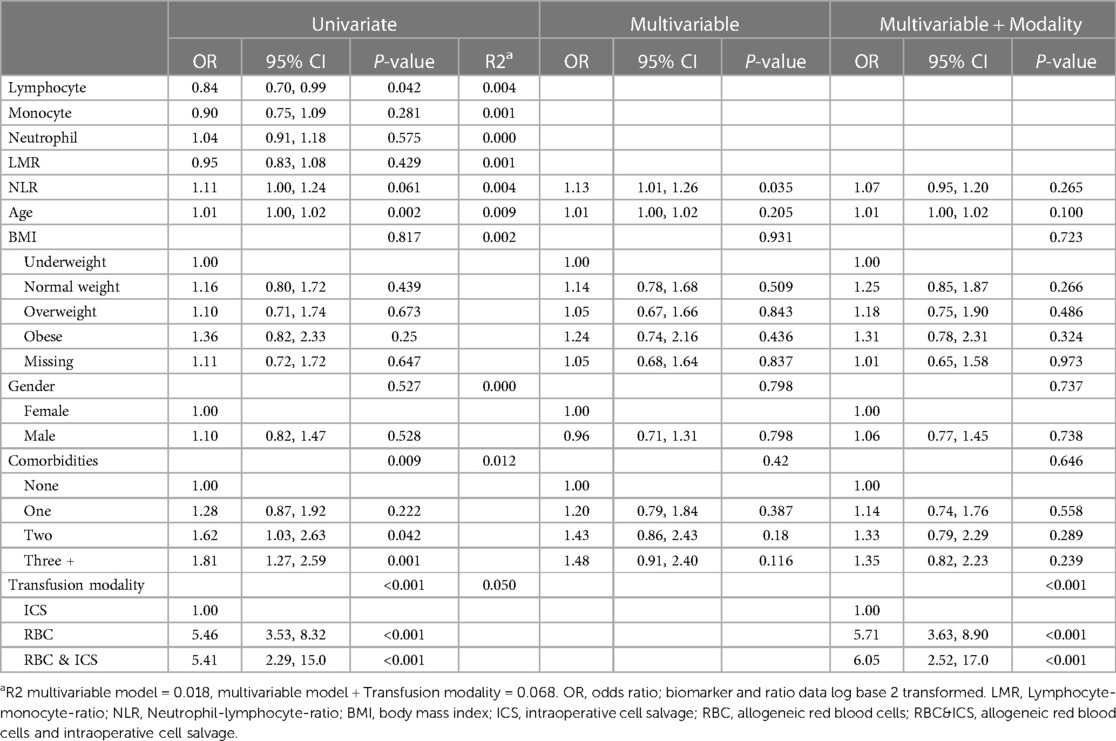
Table 4. The odds of adverse outcomes (i.e., within univariate, multivariable and multivariable and transfusion modality logistic regression models).
3.2 Adverse infection-related outcomes
Patients who developed post-operative infection were older (mean 59.7 years; CI 58.2, 61.2) than patients who did not (mean 53.8 years; CI 52.9–54.8) (P < 0.001); with lower lymphocyte numbers [mean 1.4, (CI 1.2, 1.3), P < 0.001], lower LMR [mean 1.9 (CI: 1.7, 1.9) P < 0.001] and higher NLR [mean 5.3 (CI: 4.8, 5.8), P < 0.001], compared to patients with no infection (mean lymphocytes 1.6 [CI: 1.5, 1.6], mean LMR 2.3 [CI: 2.1, 2.3]), mean NLR 4.3 [CI: 4.0, 4.4]) (Supplementary Table 4). After adjustment for demographic variables in the multivariable model and backward selection, NLR odds: 1.15 (95% CI 1.05, 1.27; P = 0.002) and lymphocyte odds: 0.85 (95% CI 0.74, 0.98; P = 0.028) remained significant (Table 5). As lymphocyte numbers doubled the odds of having an infection decreased by 15%. Transfusion modality was not found to be either a predictor of infection (P = 0.381) or to confound the effect of biomarkers.
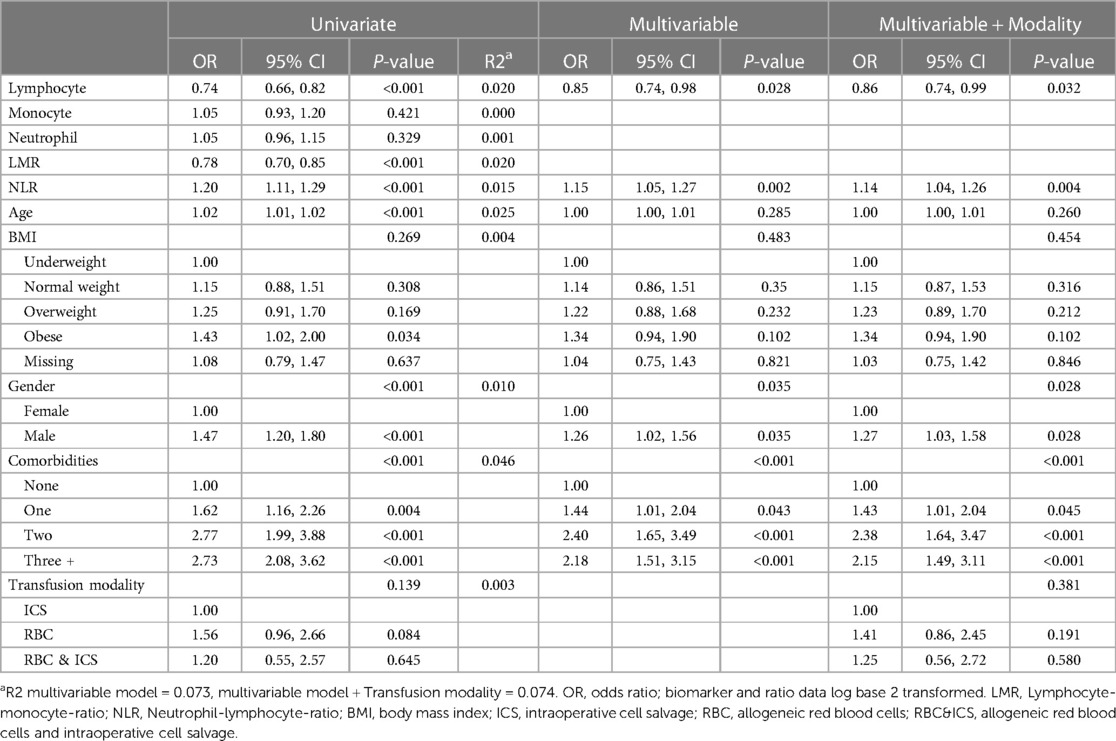
Table 5. The odds of infection related adverse outcomes (i.e., within univariate, multivariable and multivariable and transfusion modality logistic regression models).
3.3 Adverse respiratory outcomes
On average, patients who experienced adverse respiratory outcomes were older (mean 59.5 years; CI: 57.9, 61.2) compared to those who did not (mean 54.1 years; CI: 53.2, 55.1, P < 0.001) (Supplementary Table 5). Within the multivariable model, patients with higher BMI (overweight and obese), male gender, and two or more comorbidities were significantly associated with more adverse respiratory outcomes (Table 6).
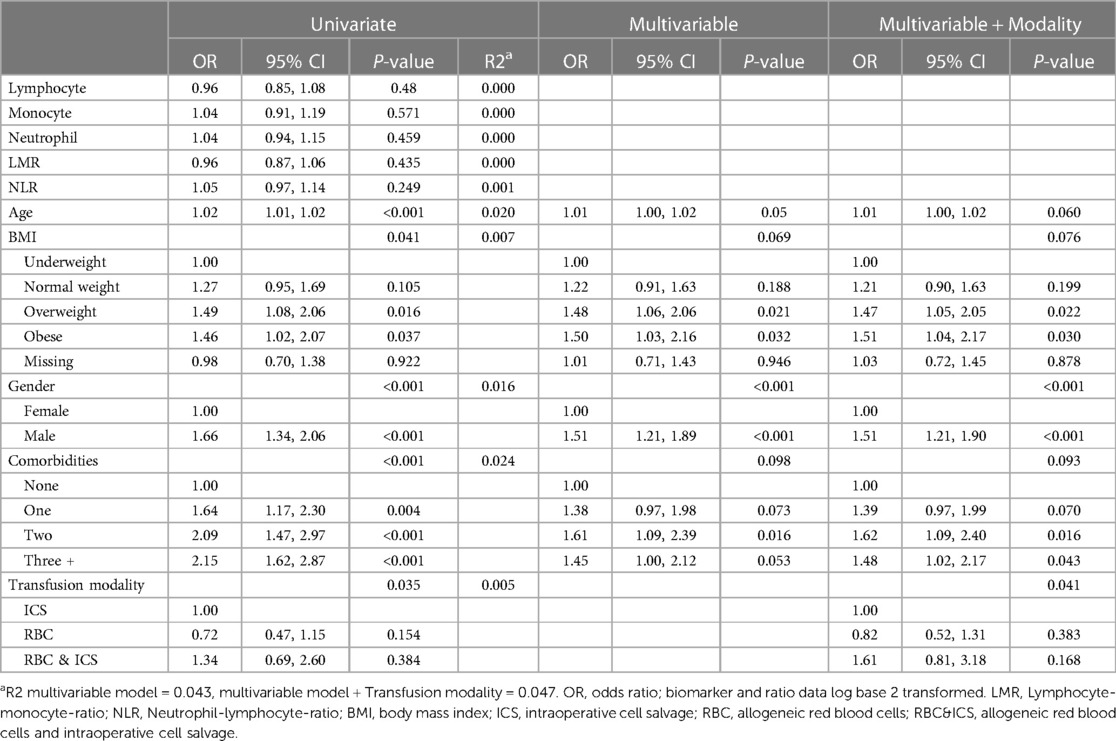
Table 6. The odds of respiratory adverse outcomes (i.e., within univariate, multivariable and multivariable and transfusion modality logistic regression models).
3.4 Adverse cardiovascular outcomes
When considering descriptive statistics and adverse cardiovascular outcomes, age differences were not significant (Supplementary Table 6). Patients with adverse cardiovascular outcomes had higher lymphocyte (mean 1.6; CI: 1.5, 1.6, P = 0.002) and neutrophil numbers (mean 6.9; CI: 6.5, 7.1; P = 0.056), compared to those with no adverse cardiovascular outcomes (lymphocytes mean 1.5; CI: 1.3, 1.4, neutrophils mean 6.4; CI: 6.0, 6.6). After adjustment for demographic variables in the multivariable model and backward selection, for every doubling of lymphocyte numbers, there was a 16% increase in the odds of adverse cardiovascular outcomes [odds 1.16, (CI 1.05, 1.28); P = 0.004] (Table 7). Finally, when adjusting the model by transfusion modality; RBC (odds 1.43, 95% CI 0.96, 2.14; P = 0.078) and RBC&ICS (odds 2.82, 95% CI 1.52, 5.34; P = 0.001) had greater odds of an adverse cardiovascular outcome than patients receiving only ICS. There was no confounding effect of transfusion modality on lymphocyte numbers.
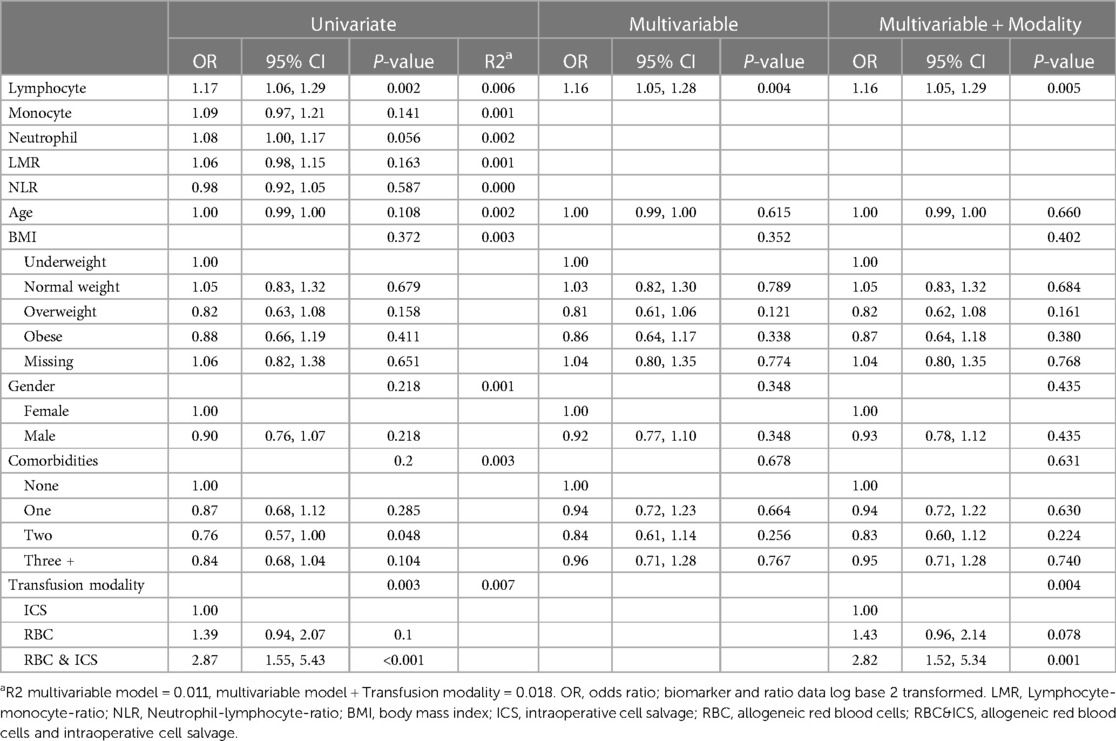
Table 7. The odds of cardiovascular adverse outcomes (i.e., within univariate, multivariable and multivariable and transfusion modality logistic regression models).
3.5 Adverse renal outcomes
Patients with adverse renal outcomes were associated with older age (mean 63.4 years (CI: 61.4, 65.5) vs. mean 54.3 years (CI: 53.4, 55.2), P < 0.001) and lower lymphocyte numbers [mean 1.4 (CI: 1.2, 1.4), P = 0.005] and LMR [1.8 (CI: 1.5, 1.9) P < 0.001]. While those with no adverse renal outcomes had higher neutrophil numbers [mean 7.5 (CI: 6.7, 8.1), p = 0.013], and NLR [mean 5.9 (CI: 5.0, 6.6), P < 0.001] (Supplementary Table 7). The univariate logistic regression models indicated that lymphocytes, neutrophils, LMR and NLR were associated with renal adverse outcomes (P < 0.05) (Table 8). However after adjustment for demographic variables in the multivariable model, an association between higher NLR and increased odds of adverse renal outcomes was identified. When NLR doubled there was a 31% increase in odds of adverse renal outcomes [odds 1.31 (CI 1.17, 1.46), P < 0.001]. Adverse renal outcomes were also associated with BMI, gender, and comorbidities. Transfusion modality was not found to be associated (P = 0.710) or confound the effect of NLR. Furthermore, the incidence of adverse cerebrovascular events (n = 21, 1%) (Supplementary Table 8) and adverse thromboembolic (n = 47, 2.3%) (Supplementary Table 9) outcomes were low.
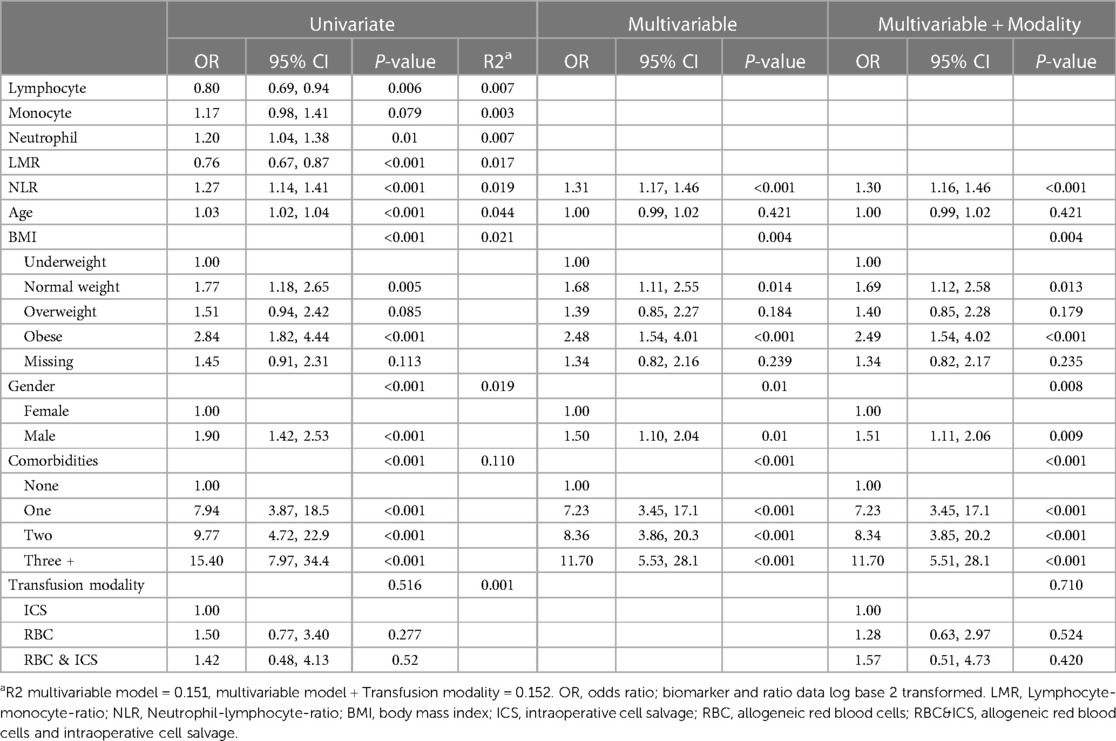
Table 8. The odds of renal adverse outcomes (i.e., within univariate, multivariable and multivariable and transfusion modality logistic regression models).
4 Discussion
Patients may experience adverse outcomes during major surgery that require blood transfusion. Pre-operative immune competence and the modulation of immune responses during surgery, potentially resulting in impaired immune responses, are important considerations (17). We therefore evaluated whether an association existed between pre-operative immune cell numbers (and ratios) and post-operative adverse outcomes in transfused patients. Patients who received blood transfusion within the study period were included and the association between immune cell numbers (and ratios) and ICD-10 coded adverse outcomes assessed. The association between ABT and altered immune responses (TRIM) within the peri-operative journey was previously confirmed (14, 37). By using ICD-10 coded adverse outcomes we reduced investigator bias and considered outcomes that were potentially related to TRIM and important to patients and hospitals internationally. Our study results demonstrate that pre-operative immune cell numbers (and ratios) are associated with adverse organ-specific peri-operative outcomes in patients who require transfusion during surgery.
Inflammatory biomarkers and immune cell ratios reflect changes in the immune response and are commonly used to predict cancer outcomes (26). The biomarkers in our study were considered in a novel way during peri-operative transfusion on the premise that they are valuable when considering immune consequences and outcomes after cancer surgery (never before considered for other sub-specialties or during peri-operative transfusion). Firstly, we considered the association between each biomarker in this study (i.e., lymphocytes, monocytes, neutrophils, LMR and NLR) and overall adverse outcomes. Previous studies confirmed lower lymphocyte numbers in those who received ABT (10). Furthermore, in previous studies, persistent leukocytosis and ongoing lymphopenia were associated with significantly higher mortality in trauma patients (38). Following adjustment within the multivariable model NLR remained significantly associated with adverse outcomes; with a 13% increase in the odds of developing an adverse outcome with every doubling of NLR. Before adjustment, adverse outcomes were more common in patients with lower lymphocyte and monocyte numbers and LMR, and higher neutrophil numbers and NLR. Chan et al. found LMR was a valuable predictor of survival in resectable bowel cancer; exceeding the value of previously established biomarkers (e.g., NLR) (26). Seeing LMR is available within standard pre-operative anaesthetic preparation, it would be a convenient biomarker to use in a large trial. We therefore considered the potential of LMR as a biomarker, but pre-operative LMR was not associated with adverse outcomes in transfused patients in this study.
We then considered the association between measured biomarkers and post-operative infection. ABT is independently associated with nosocomial infection in critically ill, and ventilator-associated pneumonia in trauma patients (7, 10). From a biological point of view, we know that a reduction in various immune cells, uptake of apoptotic cells, decreased HLA-DR, T cell “exhaustion” and increased suppressor cells further compromise immune defenses and a patient's ability to resist invading pathogens (3). Higher NLR was associated with poor health status and worse outcomes in patients with Coronavirus Disease 2019 (COVID-19) (39). Following adjustment within the multivariable model, the only significant remaining associations were the odds of developing an infection decreased by 15% if lymphocyte numbers increased (doubled) and the odds of developing an infection increased by 14% if NLR increased (i.e., doubled). These associations were significant before and after adjustment. Within the univariate model, lymphocytes, monocytes, neutrophils, LMR, NLR, age, gender and comorbidities were associated with changes in infection-related outcomes. Using an in vitro transfusion model, we previously demonstrated that exposure to ABT or ICS suppressed monocyte and dendritic cell immune responses, with improved immune competence following ICS (37). Furthermore, we recently demonstrated that monocyte numbers increased significantly at 48 h in patients who received pRBC and ICS during major orthopaedic surgery (40). In the current study, after adjustment, pre-operative monocyte numbers and LMR were not associated with infection-related adverse outcomes. Dendritic cell numbers were not considered here as they are not part of the standard differential FBC.
The importance of peri-operative immune competence extends beyond infection risks (14). No significant associations between biomarkers and respiratory outcomes were found within the descriptive statistical analysis and therefore were not included in the multivariable analysis model. We considered adverse outcomes related to the cardiovascular system. Neutrophil numbers and NLR are potential biomarkers of inflammation and are used to stratify the risk of heart failure and mortality after myocardial infarction (41, 42). In our study, during the multivariable model and following adjustment, there was 16% increased odds of developing adverse cardiovascular outcomes if lymphocyte numbers increased (doubled). Even though, during univariate analysis, all the measured biomarkers were significantly associated with increased odds of developing adverse cardiovascular outcomes. Following adjustment within the multivariable model there was a 31% increase in adverse renal outcomes if NLR increased (doubled). More adverse renal outcomes were also associated with higher BMI, male gender, and comorbidities. Statistical modelling and adjustment for confounding factors are essential even though during the original descriptive analysis associations were identified for all measured biomarkers (except monocyte numbers) and during univariate analysis for lymphocytes, monocytes, neutrophils, LMR and NLR.
Confounding factors such as age, gender, type of surgery and comorbidities affect outcomes. Transfusion is however an additional important factor to consider in this complex picture. Adverse peri-operative outcomes were common in those who received blood transfusion; 90.2% of the patients in this study experienced at least one or more ICD-10 coded adverse outcome(s). Historically, the immune-related consequences of transfusion (i.e., impaired immune function, TRIM) were considered to relate only to post-operative infection and cancer recurrence (19). Direct adverse outcomes, for example febrile transfusion reactions, have been studied extensively (43). We believe that other adverse outcomes that occur later in the surgical journey (downstream) are increasingly important (i.e., the outcomes considered in this study), but are not studied, and are underestimated and underreported. Patients in this study experienced cerebrovascular events (1%) and cardiovascular (48.3%), infection (24.1%), respiratory (20.5%), renal (10.3%) and thromboembolic (2.3%) adverse outcomes following surgery and transfusion. Peri-operative immune consequences and adverse outcomes are complex. The study results were adjusted for potential confounding factors. Even though it is not possible to consider all potential confounding factors related to transfusion research here, we aimed to narrow down on important factors we believe should be included in future. Some of the biomarkers studied were no longer significantly associated with adverse outcomes during multivariable analysis. From these results we can define specific biomarkers of importance to include in future TRIM research.
The retrospective nature, single centre involvement and inherent heterogeneity in cases are limitations to this study. We did not aim to define the causes of TRIM or to consider the differences between patients who did and those who did not receive transfusion. Such a study would require a different statistical design. Instead, as preliminary work the intention was to gather information about relevant outcomes in transfused patients across many surgical sub-specialties and the potential association with pre-operative immune competency. The RBWH performs all surgical sub-specialties except cardiothoracic and pediatric surgery. For this preliminary work we considered all surgical procedures that required transfusion within the study period (Supplementary Table 10). This distinction did preclude smaller procedures from consideration and potentially reduced some confounding factors. Because there was no evidence available relevant to our research question, we started by considering all procedures at the RBWH where patients received blood transfusion (as a starting point to this conversation). Future research aimed to define the causes of TRIM should include a sub-analysis of surgical procedures, not considered here. The outcomes considered in this study were chosen because they were previously believed to be associated with TRIM and the clinical severity or consequences (i.e., myocardial infarction vs. skin rash) were not considered. It should be noted that due to the small effect size our results (even though statistically significant), may not be clinically relevant. Conservative ICD-10 coded outcomes and regression analysis demonstrated a statistically significant difference that should be explored in future research. We agree with Mukhtar et al. that the collection of hospital data from databases, using different formatting and software languages to assess adverse transfusion-related outcomes, is indeed very challenging (44). Current advances in artificial intelligence, database design and statistical ability will make this a growing field and allow for new insights into factors associated with TRIM.
Our study findings importantly direct future research. The value of NLR as an inflammatory marker, when considering associated diseases and immune competence is a growing field of study (45). NLR is a definitive biomarker and an interesting field for future TRIM research. Even though pre-operative monocyte numbers were not associated with adverse outcomes in this study, considering our earlier work, changes in monocyte numbers peri-operatively (i.e., at one- and three days post-operatively) may provide further insights during clinical outcome studies. Valuable biomarkers during future peri-operative transfusion research would include: lymphocyte numbers and NLR when considering infection-related outcomes, lymphocyte numbers when considering cardiovascular outcomes and NLR when considering renal outcomes.
In conclusion, we demonstrated that routinely measured pre-operative immune cell numbers are associated with post-operative adverse outcomes in transfused patients. The clinical association between cell numbers and ratios and adverse post-operative outcomes identified in our study will be valuable to consider in future research on immune modulation during peri-operative transfusion. Pre-operative immune cell numbers may be an important factor to consider when studying the immune modulation seen in patients who receive transfusion.
Data availability statement
Publicly available datasets were analyzed in this study. This data can be found here: https://doi.org/10.48610/34a0052, The University of Queensland Data collection.
Ethics statement
Ethics exemption from the RBWH Human Research Ethics Committee (HREC/16/QRBW/437): for the use of deidentified retrospective administrative data collected from eight electronic hospital databases. The studies were conducted in accordance with the local legislation and institutional requirements. Written informed consent for participation was not required from the participants or the participants’ legal guardians/next of kin because for the purposes of the research: only de-identified retrospectively collected hospital database data was used. For the purposes of the surgery: Written informed consent was provided for each surgical procedure and anaesthetic.
Author contributions
MR: Conceptualization, Data curation, Formal Analysis, Funding acquisition, Investigation, Methodology, Project administration, Resources, Supervision, Writing – original draft, Writing – review & editing. DS: Conceptualization, Formal Analysis, Investigation, Methodology, Supervision, Writing – original draft, Writing – review & editing. C-YC: Data curation, Investigation, Resources, Writing – review & editing. JP: Data curation, Investigation, Resources, Writing – review & editing. LJ: Data curation, Formal Analysis, Methodology, Resources, Software, Writing – original draft, Writing – review & editing. AZ: Conceptualization, Investigation, Methodology, Supervision, Writing – original draft, Writing – review & editing. MD: Conceptualization, Investigation, Methodology, Supervision, Writing – original draft, Writing – review & editing.
Funding
The author(s) declare financial support was received for the research, authorship, and/or publication of this article.
This work was supported by scholarship grant funding (towards PhD studies with the University Queensland, Brisbane, Australia) from the Royal Brisbane and Women’s Hospital (RBWH) and the RBWH Foundation Research Advisory Committee (2018 Scholarship, 2019 Scholarship, 2020 Scholarship), Brisbane, Queensland, Australia.
Acknowledgments
This work was supported by in-kind support from many departments and staff members at the Royal Brisbane and Women's Hospital (RBWH), Metro North Hospital and Health Service (MNHHS), University of the Sunshine Coast (USC), University of Queensland (UQ) and Queensland Institute of Medical Research Berghofer (QIMRB).
The ICS group (Anaesthetic department, Royal Brisbane and Women's Hospital, Brisbane, Queensland, Australia): Mr David Cullingham [Dip (AT)], Ms Trisha Bushell [Dip (AT)], Mr Warick Fawkes [Dip (AT)], Ms Russini Stapleton [Dip (AT)], Ms Yves Long [Dip (AT)], Mr Barry Elliott [Dip (AT)], Ms Cassie Hohnke [Dip (AT)], Ms Lee Elliott [Dip (AT)], Ms Kym Webster [Dip (AT)], Ms Vicki Swaine [Dip (AT), Director Anaesthetic Healthcare Practitioners] provided ICS and supported data collection. A special thank you to Peter Freeman [Dip (AT)], who tirelessly supported our ICS service for decades and did additional data cleaning for this project. Also at the Royal Brisbane and Women's Hospital (Brisbane, Queensland, Australia): Thank you to Ms Sue Williams [B App Sc (Med Tech) Supervising Scientist, Transfusion Haematology, Central Laboratory Pathology Qld.], Janelle T Toombes [BA, registered nurse, Cancer Care Services Nursing administration, Transfusion Clinical Nurse Consultant (CNC)], Natasha Keary (Cancer Care Services Nursing administration, Transfusion CNC) and Dr John Rowell (Director of Haematology at the time of data collection and Pathology Queensland). For information technology support, to collect and analyse data, we would like to thank Alan Westacott (data analysis, Pathology Queensland), Holli Cummins (BHlthSc. CHIA., Health Information Manager Lead, Health Information Systems), Charles Cheung [BbiomedSc (Hons). PG Dip. MSc, information technology] and for development of the Structured Query Language (SQL) database and data analysis to Edu van Zyl (MBA, B1 Developers Pty. Ltd., Toowong, Brisbane, Queensland, Australia).
Conflict of interest
The authors declare that the research was conducted in the absence of any commercial or financial relationships that could be construed as a potential conflict of interest.
The author(s) declared that they were an editorial board member of Frontiers, at the time of submission. This had no impact on the peer review process and the final decision.
Publisher's note
All claims expressed in this article are solely those of the authors and do not necessarily represent those of their affiliated organizations, or those of the publisher, the editors and the reviewers. Any product that may be evaluated in this article, or claim that may be made by its manufacturer, is not guaranteed or endorsed by the publisher.
Supplementary material
The Supplementary Material for this article can be found online at: https://www.frontiersin.org/articles/10.3389/fanes.2024.1319588/full#supplementary-material
Supplemental Table 1
Correlation between immune cell sub-populations.
Supplemental Table 2
ICD-10 coded adverse outcomes considered in this study.
Supplemental Table 3
The association between biomarkers and any adverse outcomes (i.e., patients who experienced adverse outcomes vs. those who experienced no adverse outcomes), while considering patient characteristics and confounding factors.
Supplemental Table 4
The association between biomarkers and infection related adverse outcomes (i.e., patients who experienced adverse outcomes vs. those who experienced no adverse outcomes), while considering patient characteristics and confounding factors.
Supplemental Table 5
The association between biomarkers and respiratory adverse outcomes (i.e., patients who experienced adverse outcomes vs. those who experienced no adverse outcomes), while considering patient characteristics and confounding factors.
Supplemental Table 6
The association between biomarkers and cardiovascular adverse outcomes (i.e., patients who experienced adverse outcomes vs. those who experienced no adverse outcomes), while considering patient characteristics and confounding factors.
Supplemental Table 7
The association between biomarkers and renal adverse outcomes (i.e., patients who experienced adverse outcomes vs. those who experienced no adverse outcomes), while considering patient characteristics and confounding factors.
Supplemental Table 8
The association between biomarkers and cerebrovascular incidents (i.e., patients who experienced adverse outcomes vs. those who experienced no adverse outcomes), while considering patient characteristics and confounding factors.
Supplemental Table 9
The association between biomarkers and thromboembolic adverse outcomes (i.e., patients who experienced adverse outcomes vs. those who experienced no adverse outcomes), while considering patient characteristics and confounding factors.
Supplemental Table 10
Surgical procedures and sub-specialties included in this study.
References
1. Dobson GP. Trauma of Major Surgery: A Global Problem that is not Going Away. London: Elsevier (2020). p. 47–54.
2. Dencker EE, Bonde A, Troelsen A, Varadarajan KM, Sillesen M. Postoperative complications: an observational study of trends in the United States from 2012 to 2018. BMC Surg. (2021) 21(1):1–393. doi: 10.1186/s12893-021-01392-z
3. Skrupky LP, Kerby PW, Hotchkiss RS. Advances in the management of sepsis and the understanding of key immunologic defects. Anesthesiology. (2011) 115(6):1349–62. doi: 10.1097/ALN.0b013e31823422e8
4. Osborn MT, Tracy KJ, Dunne RJ, Pasquale MM, Napolitano ML. Epidemiology of sepsis in patients with traumatic injury. Crit Care Med. (2004) 32(11):2234–40. doi: 10.1097/01.CCM.0000145586.23276.0F
5. Wan Y, Patel A, Achary C, Hewson R, Phull M, Pearse R, et al. Postoperative infection and mortality following elective surgery in the international surgical outcomes study (ISOS). Br J Surg. (2021) 108(2):220–7. doi: 10.1093/bjs/znaa075
6. Blajchman MA. Immunomodulation and blood transfusion. Am J Ther. (2002) 9(5):389–95. doi: 10.1097/00045391-200209000-00005
7. Bochicchio GV, Napolitano L, Joshi M, Bochicchio K, Shih D, Meyer W, et al. Blood product transfusion and ventilator-associated pneumonia in trauma patients. Surg Infect (Larchmt). (2008) 9(4):415–22. doi: 10.1089/sur.2006.069
8. Sadjadi J, Cureton EL, Twomey P, Victorino GP. Transfusion, not just injury severity, leads to posttrauma infection: a matched cohort study. Am Surg. (2009) 75(4):307–12. doi: 10.1177/000313480907500408
9. Shorr AF, Jackson WL, Kelly KM, Fu M, Kollef MH. Transfusion practice and blood stream infections in critically ill patients. Chest. (2005) 127(5):1722–8. doi: 10.1378/chest.127.5.1722
10. White M, Barron J, Gornbein J, Lin JA. Are red blood cell transfusions associated with nosocomial infections in pediatric intensive care units? Pediatr Crit Care Med. (2010) 11(4):464–8. doi: 10.1097/PCC.0b013e3181ce708d
11. Arozullah AM, Khuri SF, Henderson WG, Daley J, PitNVASQI P. Development and validation of a multifactorial risk index for predicting postoperative pneumonia after major noncardiac surgery. Ann Intern Med. (2001) 135(10):847–57. doi: 10.7326/0003-4819-135-10-200111200-00005
12. Taylor RW, O'Brien J, Trottier SJ, Manganaro L, Cytron M, Lesko MF, et al. Red blood cell transfusions and nosocomial infections in critically ill patients. Crit Care Med. (2006 Sep) 34(9):2302–8. doi: 10.1097/01.Ccm.0000234034.51040.7f
13. Vamvakas EC, Blajchman MA. Transfusion-related immunomodulation (TRIM): an update. Blood Rev. (2007) 21(6):327–48. doi: 10.1016/j.blre.2007.07.003
14. Vamvakas EC. Transfusion-Related immunomodulation (TRIM): from renal allograft survival to postoperative mortality in cardiac surgery. In: Lee J, Donahoe M, editors. Hematologic Abnormalities and Acute Lung Syndromes. Cham: Springer International Publishing AG Switzerland (2017). p. 241–59.
15. Sirvinskas E, Veikutiene A, Benetis R, Grybauskas P, Andrejaitiene J, Veikutis V, et al. Influence of early re-infusion of autologous shed mediastinal blood on clinical outcome after cardiac surgery. Perfusion. (2007) 22(5):345–52. doi: 10.1177/0267659107088450
16. Carless PA, Henry DA, Moxey AJ, O'Connell D, Brown T, Fergusson DA. Cell salvage for minimising perioperative allogeneic blood transfusion. Cochrane Database Syst Rev. (2010) 2010(4):Cd001888. doi: 10.1002/14651858.CD001888.pub4
17. Dąbrowska AM, Słotwiński R. The immune response to surgery and infection. Cent-Eur J Immunol. (2014) 39(4):532–7. doi: 10.5114/ceji.2014.47741
18. Kurosawa S. Anesthesia in patients with cancer disorders. Curr Opin Anaesthesiol. (2012) 25(3):376–84. doi: 10.1097/ACO.0b013e328352b4a8
19. Hellings S, Blajchman MA. Transfusion-related immunosuppression. Anaesth Intensive Care. (2009) 10(5):231–4. doi: 10.1016/j.mpaic.2009.01.017
20. Torrance HD, Pearse RM, O’Dwyer MJ. Does major surgery induce immune suppression and increase the risk of postoperative infection? Curr Opin Anaesthesiol. (2016) 29(3):376–83. doi: 10.1097/ACO.0000000000000331
21. Nader ND. Immunomodulation mechanisms in disease and in the surgical patient. Taylor & Francis. (2017) 281(24):765–8. doi: 10.1080/08820139.2017.1373906
22. Sanders R III. Perioperative Immunity: is there an Anaesthetic Hangover? Oxford: Oxford University Press (2014). p. 210–2.
23. Glaser R, Rabin B, Chesney M, Cohen S, Natelson B. Stress-induced immunomodulation: implications for infectious diseases? JAMA. (1999) 281(24):2268–70. doi: 10.1001/jama.281.24.2268
24. Corcoran TB, Myles PS, Forbes AB, Cheng AC, Bach LA, O’Loughlin E, et al. Dexamethasone and surgical-site infection. N Engl J Med. (2021) 384(18):1731–41. doi: 10.1056/NEJMoa2028982
25. Sedghi S, Kutscher HL, Davidson BA, Knight PR. Volatile anesthetics and immunity. Immunol Investig. (2017) 46(8):793–804. doi: 10.1080/08820139.2017.1373905
26. Chan JC, Chan DL, Diakos CI, Engel A, Pavlakis N, Gill A, et al. The lymphocyte-to-monocyte ratio is a superior predictor of overall survival in comparison to established biomarkers of resectable colorectal cancer. Annals of Surgery (Ann Surg). (2017) 265(3):539–46. doi: 10.1097/SLA.0000000000001743
27. Kwon H-C, Kim SH, Oh SY, Lee S, Lee JH, Choi H-J, et al. Clinical significance of preoperative neutrophil-lymphocyte versus platelet-lymphocyte ratio in patients with operable colorectal cancer. Biomarkers. (2012) 17(3):216–22. doi: 10.3109/1354750X.2012.656705
28. Yuan C, Li N, Mao X, Liu Z, Ou W, Wang S-Y. Elevated pretreatment neutrophil/white blood cell ratio and monocyte/lymphocyte ratio predict poor survival in patients with curatively resected non-small cell lung cancer: results from a large cohort. Thorac Cancer. (2017) 8(4):350. doi: 10.1111/1759-7714.12454
29. Kumagai S, Marumo S, Shoji T, Sakuramoto M, Hirai T, Nishimura T, Arima T, Fukui M, Huang C-L. Prognostic impact of preoperative monocyte counts in patients with resected lung adenocarcinoma. Lung Cancer. 2014;85(3):457–64. doi: 10.1016/j.lungcan.2014.06.015
30. Sierzega M, Lenart M, Rutkowska M, Surman M, Mytar B, Matyja A, et al. Preoperative neutrophil-lymphocyte and lymphocyte-monocyte ratios reflect immune cell population rearrangement in resectable pancreatic cancer. Ann Surg Oncol. (2017) 24(3):808–15. doi: 10.1245/s10434-016-5634-0
31. Haveman JW, van den Berg AP, Verhoeven EL, Nijsten MW, van den Dungen JJ, Zwaveling JH. HLA-DR expression on monocytes and systemic inflammation in patients with ruptured abdominal aortic aneurysms. Critical Care. (2006) 10(4):1–7. doi: 10.1186/cc5017
32. Volk H-D. Immunodepression in the surgical patient and increased susceptibility to infection. Critical Care. (2002) 6(4):279–81. doi: 10.1186/cc1507
33. Monneret G, Venet F. Sepsis-induced immune alterations monitoring by flow cytometry as a promising tool for individualized therapy. Cytometry B Clin Cytom. (2016) 90(4):376–86. doi: 10.1002/cyto.b.21270
34. Xiao W, Mindrinos MN, Seok J, Cuschieri J, Cuenca AG, Gao H, et al. A genomic storm in critically injured humans. J Exp Med. (2011) 208(13):2581–90. doi: 10.1084/jem.20111354
35. Mount Sinai. Blood Differential Test. Mount Sinai: Icahn School of Medicine (2021). Available online at: Available at: https://www.mountsinai.org/health-library/tests/blood-differential-test
36. World Health Organization regional office for Europe. A healthy lifestyle-WHO recommendations. WHO (2023) 11/05/2023. Newsroom, fact sheets. Available online at: https://www.who.int/europe/news-room/fact-sheets/item/a-healthy-lifestyle—who-recommendations (accessed May 11, 2023).
37. Roets M, Sturgess DJ, Obeysekera MP, Tran TV, Wyssusek KH, Punnasseril JEJ, et al. Intraoperative cell salvage as an alternative to allogeneic (donated) blood transfusion: a prospective observational evaluation of the immune response profile. Cell Transplant. (2020) 29:0963689720966265. doi: 10.1177/0963689720966265
38. Heffernan DS, Monaghan SF, Thakkar RK, Machan JT, Cioffi WG, Ayala A. Failure to normalize lymphopenia following trauma is associated with increased mortality, independent of the leukocytosis pattern. Crit Care. (2012) 16(1):1–10. doi: 10.1186/cc11157
39. King AH, Mehkri O, Rajendram P, Wang X, Vachharajani V, Duggal A. A high neutrophil-lymphocyte ratio is associated with increased morbidity and mortality in patients with coronavirus disease 2019. Crit Care Explor. (2021) 3(5):e0444–e0444. doi: 10.1097/CCE.0000000000000444
40. Roets M, Sturgess D, Tran T, Obeysekera M, Perros A, Tung J-P, et al. Intraoperative cell salvage: the impact on immune cell numbers. PLoS One. (2023) 18(8):e0289177. doi: 10.1371/journal.pone.0289177
41. Arruda-Olson AM, Reeder GS, Bell MR, Weston SA, Roger VL. Neutrophilia predicts death and heart failure after myocardial infarction: a community-based study. Circ Cardiovasc Qual Outcomes. (2009) 2(6):656–62. doi: 10.1161/CIRCOUTCOMES.108.831024
42. Azab BMD, Zaher MMD, Weiserbs KFP, Torbey EMD, Lacossiere KMD, Gaddam SMD, et al. Usefulness of neutrophil to lymphocyte ratio in predicting short- and long-term mortality after non–ST-elevation myocardial infarction. Am J Cardiol. (2010) 106(4):470–6. doi: 10.1016/j.amjcard.2010.03.062
43. SHOT (SHOT Office, Manchester Blood Centre, Plymouth Grove, Manchester, M13 9LL.). Annual SHOT Report (2021). Report. (Serious Hazards of Transfusion (SHOT)). 28 May. MHRA. (2023) Available online at: https://www.shotuk.org/shot-reports/report-summary-and-supplement-2021/2021-annual-shot-report-individual-chapters/ (accessed February 02, 2024).
44. Mukhtar SA, Leahy MF, Trentino K, Koay A, Semmens JB, Tovey J, et al. Effectiveness of a patient blood management data system in monitoring blood use in western Australia. Anaesth Intensive Care. (2013) 41(2):207–15. doi: 10.1177/0310057X1304100210
Keywords: immune cells, immune modulation, adverse outcomes, transfusion, biomarkers
Citation: Roets M, Sturgess DJ, Chen C-Y, Punnasseril JEJ, Jones L, van Zundert AA and Dean MM (2024) Pre-operative immune cell numbers and ratios are associated with peri-operative adverse outcomes in transfused patients. Front. Anesthesiol. 3:1319588. doi: 10.3389/fanes.2024.1319588
Received: 12 November 2023; Accepted: 23 January 2024;
Published: 13 February 2024.
Edited by:
Hong Liu, UC Davis Health, United StatesReviewed by:
Aveek Jayant, Homi Bhabha Cancer Hospital and Research Centre, IndiaKatherine Forkin, University of Virginia, United States
© 2024 Roets, Sturgess, Chen, Punnasseril, Jones, van Zundert and Dean. This is an open-access article distributed under the terms of the Creative Commons Attribution License (CC BY). The use, distribution or reproduction in other forums is permitted, provided the original author(s) and the copyright owner(s) are credited and that the original publication in this journal is cited, in accordance with accepted academic practice. No use, distribution or reproduction is permitted which does not comply with these terms.
*Correspondence: Michelle Roets m.roets@uq.edu.au
Abbreviations ABO, ABO blood groups; ABT, allogeneic blood transfusion; pRBC, allogeneic packed red blood cells; RBC, allogeneic packed red blood cells only; AARK, Automated Anaesthetic Record Keeping; BMI, kg/m2, body mass index; CVS, cardiovascular; COAD, chronic obstructive airway disease; CD, cluster of differentiation; COVID-19, Coronavirus Disease 2019; DVT, deep venous thrombosis; FBC, full blood count; HIS, Health Information Systems; HBCIS, Hospital-based corporate information system; HLA-DR, Human Leukocyte Antigen – DR isotype; HREC, Human Research Ethics Committee; IDDM, insulin dependent diabetes mellitus; ieMR, Integrated Electronic Medical Record; ICU, intensive care unit; ICD-10, International Classification of Diseases-10; ISOS, International Surgical Outcomes Study; ICS, Intraoperative cell salvage; LOS, length of stay in hospital; LMR, lymphocyte/monocyte ratio; LNR, lymphocyte/neutrophil ratio; NK, Natural killer; NLR, neutrophil/lymphocyte ratio; NIDDM, non-insulin-dependent diabetes mellitus; ORMIS, Operating Room Medical Information System; PLR, platelet/lymphocyte ratio; RBWH, Royal Brisbane and Women's Hospital; SQL, Structured Query Language; TRIM, transfusion related immune modulation; TIA, transient ischemic attack; VIF, variance inflation factor.