Ketosis risk derived from mid-infrared predicted traits and its relationship with herd milk yield, health and fertility
- 1ZuchtData EDV-Dienstleistungen GmbH, Vienna, Austria
- 2Regional State Association for Performance and Quality Inspection in Animal Breeding of Baden Württemberg, Stuttgart, Germany
- 3LKV-Austria, Vienna, Austria
Milk analysis using mid-infrared spectroscopy (MIR) is a fast and inexpensive way of examining milk samples on a large scale for fat, protein, lactose, urea and many other novel traits. A new indicator trait for ketosis, KetoMIR, which is based on clinical ketosis diagnoses and MIR-predicted traits, was developed by the Regional State Association for Performance and Quality Inspection in Animal Breeding of Baden Württemberg in 2015. The KetoMIR result is available for each cow at milk recording during the first 120 days in milk and presented to farmers in three classes: 1 = low ketosis risk, 2 = moderate ketosis risk and 3 = high ketosis risk. The aim of the current study was to analyze the phenotypic relationships between KetoMIR and milk yield, fertility and health at the herd level. Annual herd reports from 12,909 herds with an average herd size of 27 cows were available for the analyses. Overall, the mean incidence of ketosis (KetoMIR risk class 2 or 3) at the herd level was 14.0%. Farms with the lowest ketosis risk (≤10% of cows in the herd with a moderate or high ketosis risk) differed in all variables from the farms with the highest ketosis risk (>50% of cows in the herd with a moderate or high ketosis risk). The increased ketosis risk based on KetoMIR was associated with lower average herd milk yield (-1,975 kg milk). Mean herd somatic cell count in first and higher lactations was increased by 60,500 and 134,400 cells/ml, respectively. The interval from calving to first service was prolonged by +36.5 days, as was the calving interval with +58.2 days. The newly developed KetoMIR trait may be used in ketosis prevention programs.
Introduction
Ketosis is one of the most common metabolic diseases of dairy cows and occurs at the beginning of lactation. The incidence of clinical ketosis is low and is only 1.3% in Austria (Fürst-Waltl and Schwarzenbacher, 2021). Subclinical ketosis occurs much more frequently but is not routinely recorded. Egger-Danner et al. (2016) and Liebminger (2021) reported that 10 to 20% of cows in Austria are affected with subclinical ketosis. The range of ketosis incidence differs for each country, as for example in the Netherlands, much higher values were reported by Vanholder et al. (2015) in 23 dairy farms, 11.6% of cows had clinical ketosis and 47.2% had subclinical ketosis. Lean et al. (2023) conducted a retrospective meta-analysis based on individual cow data from Australia, Canada and the United States. They reported the incidence of clinical ketosis was 3.3%, whereas the incidence of subclinical ketosis was 26.8%.
Ketosis can be diagnosed by measuring ketone bodies present in blood, urine, or milk. Various handheld blood ketone meters are available to farmers to detect cows with subclinical ketosis (e.g. Sailer et al., 2018; Khol et al., 2019). However, due to practical limitations associated with individual blood sampling and testing, routine screening of all at-risk animals with blood tests is not feasible. Analysis of individual milk samples by mid-infrared (MIR) spectrometry remains the most promising method for detecting ketosis because it allows complete screening of the health status of the animal and the herd (Benedet et al., 2019). In recent years, many novel MIR-predicted traits related to metabolic health have been derived (de Roos et al., 2007; Grelet et al., 2016; Franceschini et al., 2022). A new ketosis risk indicator trait, KetoMIR, based on clinical ketosis diagnoses and MIR-predicted traits, was developed at the Regional State Association for Performance and Quality Inspection in Animal Breeding of Baden Württemberg as part of the OptiMIR project (Hamann et al., 2017; Drössler et al., 2018). The new ketosis risk indicator is intended as a herd management tool that allows farmers to monitor the metabolic situation of their herd during the first 120 days of lactation. In Baden-Württemberg, KetoMIR has been routinely used since 2015, and in Austria since 2017. The aim of the present study was to investigate the relationship of ketosis risk based on KetoMIR with milk yield, health and fertility at herd level in Austrian dairy farms. For this purpose, the phenotypic associations between these traits were analyzed.
Materials and methods
Description of KetoMIR
The trait KetoMIR was developed at the Regional State Association for Performance and Quality Inspection in Animal Breeding of Baden Württemberg as part of the OptiMIR project (Hamann et al., 2017; Drössler et al., 2018). The new trait was derived based on clinical ketosis diagnoses and MIR-predicted traits. The model used is a logistic regression and includes nine MIR-predicted traits to predict ketosis risk, including standard milk components, ketone bodies, fatty acids and minerals. A more detailed description of the MIR-predicted traits included in the model cannot be given for reasons of confidentiality. Lactation number, lactation week, breed and sampling time (mixed, morning, evening) are included as fixed effects in the model. The result from the logistic regression model is a continuous value between 0 and 1. The KetoMIR result is available for each cow at milk recording during the first 120 days in milk and is presented to farmers in three classes. Class 1 indicates low ketosis risk (0-0.5), class 2 indicates moderate ketosis risk (>0.5-0.75), and class 3 indicates high ketosis risk (>0.75). A more detailed description of the model can be found in Hamann et al. (2017) and Drössler et al. (2018).
Data
Annual herd reports from 2017 were provided by ZuchtData (Vienna, Austria), which included herd reports from all Austrian dairy herds under milk recording (in total 21,342 herds). Herds with less than 10 cows and missing data were deleted, which resulted in 12,909 herds. For analyses, herd reports from 12,909 herds with an average herd size of 27 cows were available.
The annual herd reports provide the basis for evaluating the general performance, health and fertility of the herds. The following herd variables were available in the reports: herd means for milk yield, somatic cell count, interval from calving to first insemination and calving interval (see a more detailed description in Table 1). Additionally, the proportion of test-day records with a moderate or high ketosis risk (KetoMIR risk class 2 or 3) within the first 120 days in milk was available for each herd for the year 2017. A summary statistic of the analyzed traits is given in Table 1.
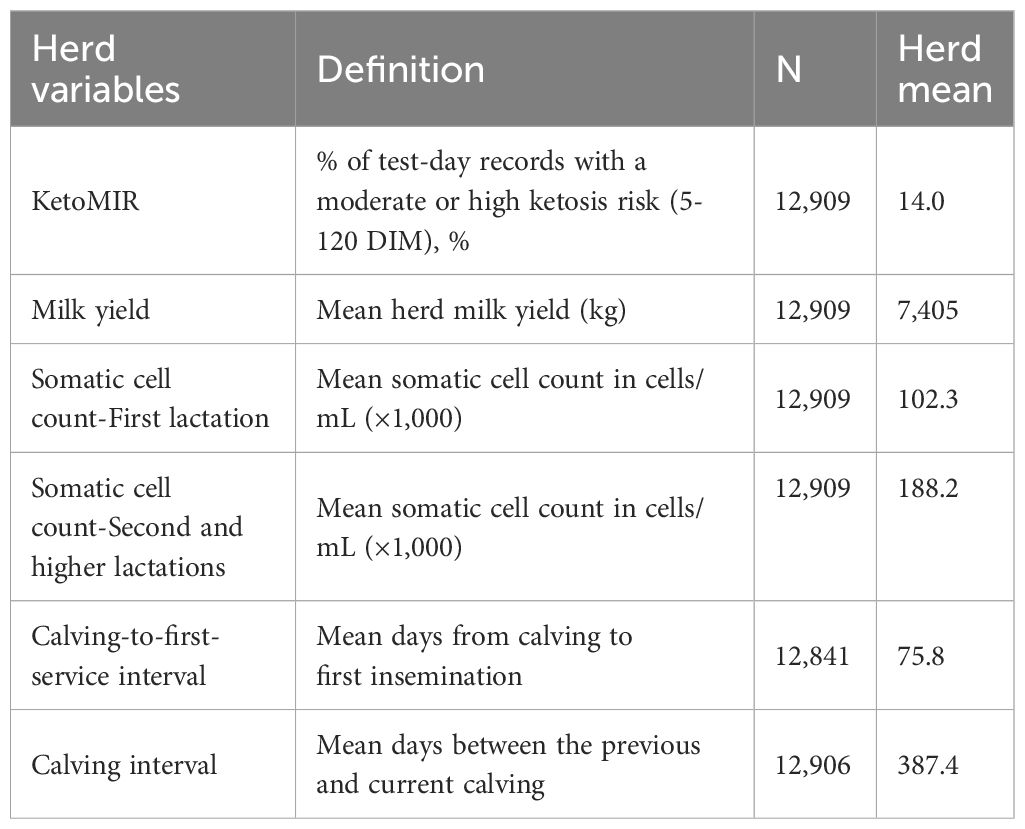
Table 1 Definition of herd variables in Austrian annual herd reports, number of herds and herd mean values for KetoMIR (% of cows in the herd with a moderate or high ketosis risk), milk yield, somatic cell count, calving-to-first-service-interval and calving interval.
Statistical analyses
All analyses are based on the herd reports from 2017, and are, therefore, taken from herd mean information and not individual cow data. The analyses were carried out across breeds. The main dairy cattle breed in Austria is Fleckvieh (74.7%), followed by Holstein (7.4%) and Brown Swiss (5.6%) (RZA Annual Report, 2022). A separate analysis for each breed was not possible as many herds have more than one breed or crossbred cows and the herd means for milk yield, somatic cell count, interval from calving to first insemination and calving interval are routinely calculated for each herd across all animals (without taking the breed into account).
All statistics were calculated using SAS software (SAS Institute Inc.). The farms were assigned to one of the following 6 groups according to the proportion of cows in the herd with a moderate or high risk of ketosis (KetoMIR risk class 2 or 3): ≤10, >10-≤20, >20-≤30, >30-≤40, >40-≤50, >50%. The Kruskal-Wallis nonparametric test was used to determine whether there were significant differences between the groups (Kruskal and Wallis, 1952). For multiple comparison, the Dwass, Steel, Critchlow-Fligner multiple comparison procedure was applied if the p-value was lower than 0.05 for the global null hypothesis (Critchlow and Fligner, 1991).
Results
In our study, the mean incidence of cows with a moderate or high ketosis risk based on KetoMIR was 14% at the herd level (Table 1). Highly elevated incidences of >30% were found in 8.6% of farms (Figure 1).
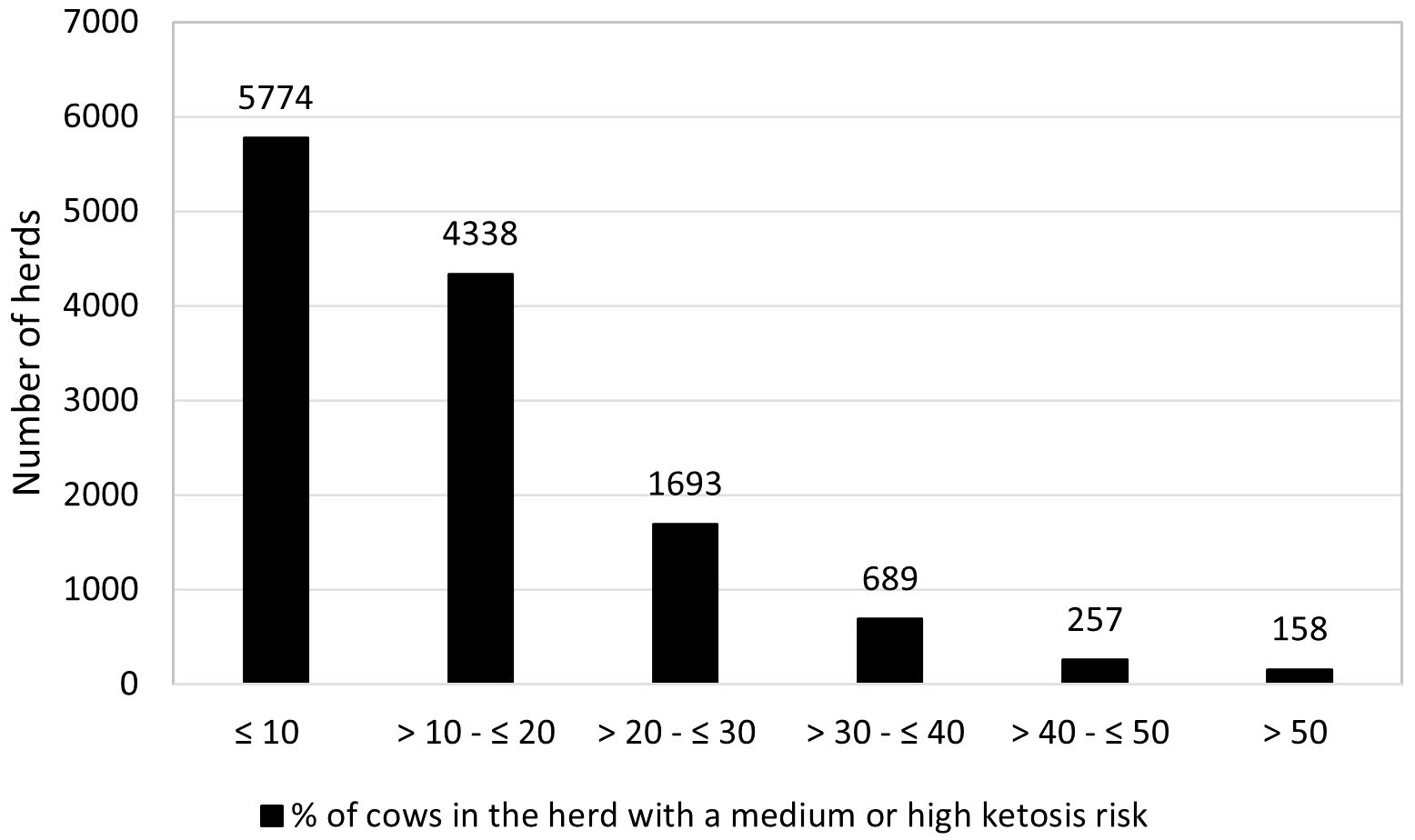
Figure 1 Number of herds in each ketosis risk class (% of cows in the herd with a moderate or high ketosis risk).
The Kruskal-Wallis test determined that there are significant differences in all investigated variables between farms at different risk of ketosis. The subsequently-applied Dwass, Steel, Critchlow-Fligner multiple comparison procedure showed that the farms with the lowest ketosis risk (≤10% of cows in the herd with a moderate or high ketosis risk) differ in all variables from the farms with the highest ketosis risk (>50% of cows in the herd with a moderate or high ketosis risk) (Table 2). The increased ketosis risk based on KetoMIR was associated with lower average herd milk yield (-1,975 kg milk). Mean herd somatic cell count in first and higher lactations was increased by 60,500 and 134,400 cells/ml, respectively. The interval from calving to first service was prolonged by +36.5 days, as was the calving interval with +58.2 days.
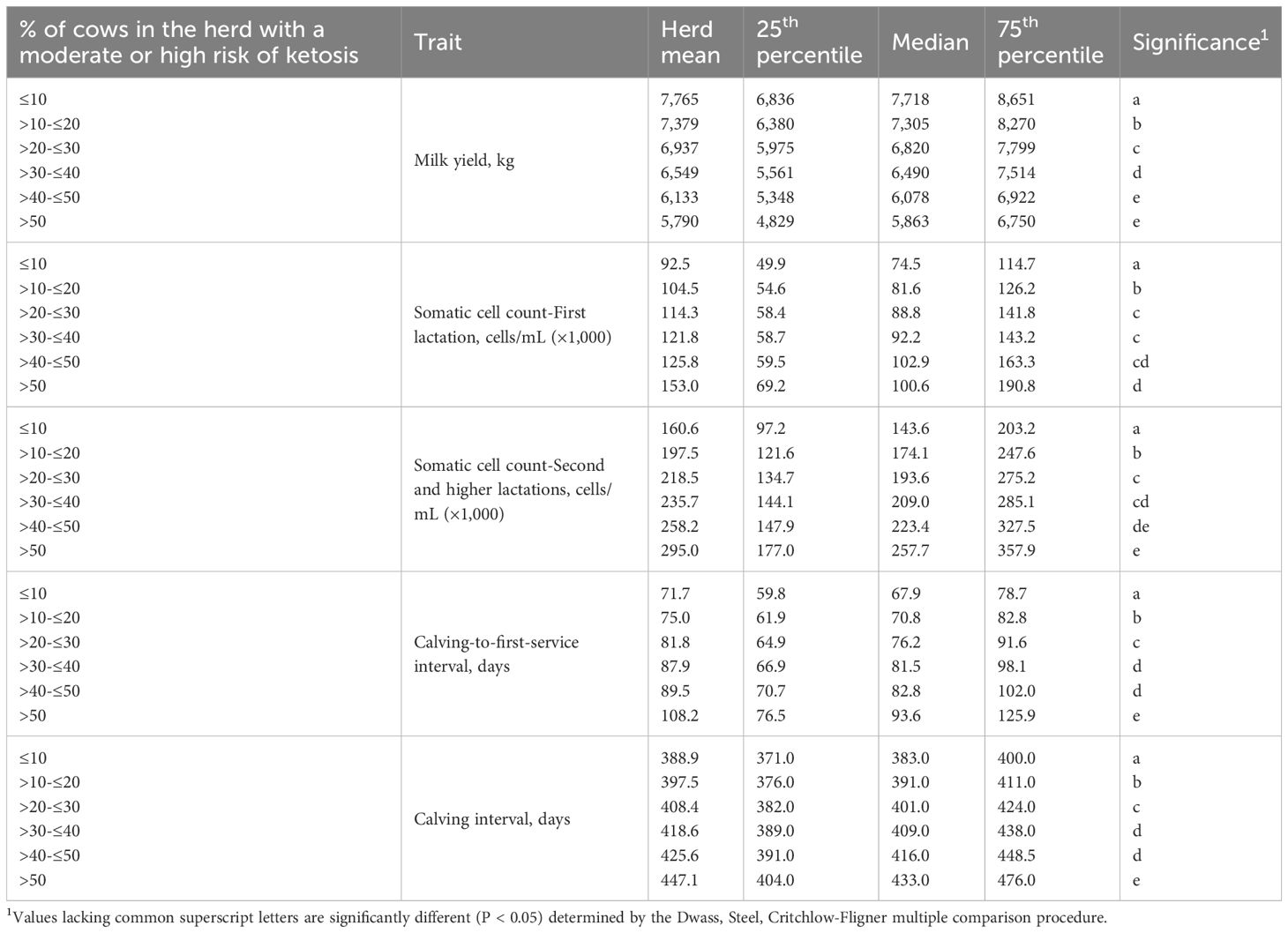
Table 2 Herd mean values, median, 25th percentile and 75th percentile for milk yield, somatic cell count, calving-to-first-service-interval and calving interval with respect to ketosis risk (% of cows in the herd with a moderate or high ketosis risk according to KetoMIR result).
Discussion
The reported incidence of ketosis based on KetoMIR in Austria is at the lower end of the range published in the literature for dairy cattle. A study in 10 European countries indicated that the overall prevalence of ketosis, defined as serum β-hydroxybutyrate concentration ≥ 1,200 to 1,400 µmol/L in cows within 2 to 15 days in milk was 21.8%, ranging from 11.2 to 36.6% (Suthar et al., 2013). Berge and Vertenten (2014) reported that 39% of the cows in western European dairy herds were classified as having ketosis, defined as a milk based keto test result ≥ 100 μmol/L. The herd average of ketosis was 43% in Germany, 53% in France, 31% in Italy, 46% in the Netherlands, and 31% in the United Kingdom. Of the 131 investigated farms, 112 (85%) had 25% or more of their fresh cows resulting as positive for ketosis. Brunner et al. (2019) reported prevalence of subclinical ketosis (β-hydroxybutyrate concentration ≥1.2 mmol/L) in dairy cows in Central and South America, Africa, Asia, Australia, New Zealand, and Eastern Europe. Across all investigated countries, the prevalence of subclinical ketosis was 24.1%, ranging from 8.3% up to 40.1%.
In our study, a higher incidence of cows at moderate or high risk of ketosis on the farm was associated with lower milk yield, higher somatic cell count, longer time from calving to first service and prolonged calving interval, which is in agreement with previous studies. A negative association between the herd prevalence of ketosis and milk yield was also recently reported by Couto Serrenho et al. (2023). As mentioned by Daros et al. (2022) it is likely that herds that produce more milk also have better general management and thus may have implemented more effective ketosis prevention protocols.
In agreement with our results, van Straten et al. (2009) reported that cows with ketosis were 44% more likely to have an event with a somatic cell count >250,000 cells/ml than cows without ketosis. In addition, it was found that cows with extreme relative body weight loss in early lactation were more likely to have an increased somatic cell count throughout lactation.
An undesirable relationship of ketosis and fertility was reported by numerous authors (Reist et al., 2000; Walsh et al., 2007; Rutherford et al., 2016). Compton et al. (2015) found that concentration of β-hydroxybutyrate in blood ≥ 1.2 mmol/L at any stage within 5 weeks post-calving was associated with decreased 6-week pregnancy rate (78 vs. 85%). Recently, Alemu et al. (2023) reported a negative association between elevated milk β-hydroxybutyrate within 42 d and reproductive performance after the voluntary waiting period.
Conclusion
Milk is the most promising matrix to identify cows with ketosis, because it is easy to sample and allows a complete screening of the herd. Based on routinely available clinical ketosis diagnoses and MIR-predicted traits, a new ketosis risk indicator (KetoMIR) was developed to provide a new screening tool of the herd at milk recording. The ketosis risk was modeled for the first 120 days of lactation. Overall, an increased incidence of cows with a moderate or high ketosis risk on the farm indicates a problem and was associated with lower milk production, increased somatic cell count, longer time from calving to first service and prolonged calving interval. The use of milk MIR-predicted traits for herd-level ketosis screening could be an important factor in preventing ketosis to limit as much as possible the economic losses caused by this disease.
Data availability statement
The data used in the current study are not publicly available due to privacy restrictions of the data provider and owner (LKV Austria). Requests to access these datasets should be directed to F-JA Franz-Josef.Auer@lkv-austria.at.
Ethics statement
This study was an observational study of farms in their normal routines, and no interventions were conducted on any animals or humans for the purpose of this article. It thus did not require Institutional Animal Care and Use Committee or Institutional Review Board approval.
Author contributions
AK: Conceptualization, Formal analysis, Methodology, Visualization, Writing – original draft, Writing – review & editing. LD: Writing – original draft, Writing – review & editing. AW: Writing – original draft, Writing – review & editing. MM: Writing – original draft, Writing – review & editing, Data curation. F-JA: Writing – original draft, Writing – review & editing. CE-D: Conceptualization, Funding acquisition, Project administration, Supervision, Writing – original draft, Writing – review & editing.
Funding
The author(s) declare financial support was received for the research, authorship, and/or publication of this article. This work was conducted within the COMET-Project D4Dairy (Digitalization, Data integration, Detection and Decision support in Dairying, Project number: 872039). D4Dairy was supported by Federal Ministry for Climate Action, Environment, Energy, Mobility, Innovation and Technology (BMK), Federal Ministry for Digital and Economic Affairs (BMDW) and the provinces of Lower Austria and Vienna in the framework of COMET-Competence Centers of Excellent Technologies. The COMET program is handled by the Austrian Research Promotion Agency (FFG).
Acknowledgments
The authors would like to thank LKV Austria for providing access to various farm data.
Conflict of interest
Authors AK, MM, and CE-D were employed by the company ZuchtData EDV-Dienstleistungen GmbH, LD and AW by Regional State Association for Performance and Quality Inspection in Animal Breeding of Baden Württemberg and F-JA by LKV-Austria.
Publisher’s note
All claims expressed in this article are solely those of the authors and do not necessarily represent those of their affiliated organizations, or those of the publisher, the editors and the reviewers. Any product that may be evaluated in this article, or claim that may be made by its manufacturer, is not guaranteed or endorsed by the publisher.
References
Alemu T. W., Santschi D. E., Cue R. I., Duggavathi R. (2023). Reproductive performance of lactating dairy cows with elevated milk β-hydroxybutyrate levels during first 6 weeks of lactation. J. Dairy Sci. 106, 5165–5181. doi: 10.3168/jds.2022-22406
Benedet A., Manuelian C. L., Zidi A., Penasa M., De Marchi M. (2019). Invited review: β-hydroxybutyrate concentration in blood and milk and its associations with cow performance. Animal 13, 1676–1689. doi: 10.1017/S175173111900034X
Berge A. C., Vertenten G. A. (2014). A field study to determine the prevalence, dairy herd management systems, and fresh cow clinical conditions associated with ketosis in western European dairy herds. J. Dairy Sci. 97, 2145–2154. doi: 10.3168/jds.2013-7163
Brunner N., Groeger S., Canelas Raposo J., Bruckmaier R. M., Gross J. J. (2019). Prevalence of subclinical ketosis and production diseases in dairy cows in Central and South America, Africa, Asia, Australia, New Zealand, and Eastern Europe. Transl. Anim. Sci. 3, 84–92. doi: 10.1093/tas/txy102
Compton C. W., Young L., McDougall S. (2015). Subclinical ketosis in post-partum dairy cows fed a predominantly pasture-based diet: defining cut-points for diagnosis using concentrations of beta-hydroxybutyrate in blood and determining prevalence. N Z Vet. J. 63, 241–248. doi: 10.1080/00480169.2014.999841
Couto Serrenho R., Church C., McGee D., Duffield T. F. (2023). Association of herd hyperketolactia prevalence with transition management practices and herd productivity on Canadian dairy farms-A retrospective cross-sectional study. J. Dairy Sci. 106, 2819–2829. doi: 10.3168/jds.2022-22377
Critchlow E., Fligner A. M. (1991). On distribution-free multiple comparisons in the one-way analysis of variance. Commun. Stat - Theory Methods 20, 127–139. doi: 10.1080/03610929108830487
Daros R. R., Weary D. M., von Keyserlingk M. A. G. (2022). Invited review: Risk factors for transition period disease in intensive grazing and housed dairy cattle. J. Dairy Sci. 105, 4734–4748. doi: 10.3168/jds.2021-20649
de Roos A. P. W., van den Bijgaart H. J. C. M., Hørlyk J., de Jong G. (2007). Screening for subclinical ketosis in dairy cattle by fourier transform infrared spectrometry. J. Dairy Sci. 90, 1761–1766. doi: 10.3168/jds.2006-203
Drössler K., Werner A., Dale L. (2018). “KetoMIR - ein neues Werkzeug für LKV-Mitgliedsbetriebe,” in Viehwirtschaftliche Fachtagung, vol. 45 . Ed. Bericht L. F. Z. (Raumberg-Gumpenstein, Austria), 57–62.
Egger-Danner C., Fuerst-Waltl B., Fuerst C., Gruber L., Hörtenhuber S., Koeck A., et al. (2016). Efficient Cow – Final report on the research project number 100861, BMLFUW-LE.1.3.2/0083-II/1/2012 (Vienna). Available at: https://dafne.at/projekte/efficient-cow (Accessed December 4, 2023).
Franceschini S., Grelet C., Leblois J., Gengler N., Soyeurt H., GplusE consortium (2022). Can unsupervised learning methods applied to milk recording big data provide new insights into dairy cow health? J. Dairy Sci. 105, 6760–6772. doi: 10.3168/jds.2022-21975
Fürst-Waltl B., Schwarzenbacher H. (2021). Züchterische Verbesserung der Stoffwechselstabilität. In: ZAR Seminar 2021: Strategien zur stoffwechselstabilen Milchkuh – frühzeitig erkennen und nachhaltig verbessern. Available at: https://www.rinderzucht.at/downloads/seminarunterlagen.html (Accessed December 4, 2023).
Grelet C., Bastin C., Gelé M., Davière J. B., Johan M., Werner A., et al. (2016). Development of Fourier transform mid-infrared calibrations to predict acetone, β-hydroxybutyrate, and citrate contents in bovine milk through a European dairy network. J. Dairy Sci. 99, 4816–4825. doi: 10.3168/jds.2015-10477
Hamann A., Werner A., Dale L., Herold P. (2017). Genetic analyses of ketosis and a newly developed risk indicator in Fleckvieh, Braunvieh and German Holstein. ICAR Tech. Ser. no. 22, 105–108.
Khol J. L., Freigassner K., Stanitznig A., Tichy A., Wittek T. (2019). Evaluation of a handheld device for the measurement of beta-hydroxybutyrate in capillary blood obtained by the puncture of the vulva as well as in venous whole blood in cattle. Pol. J. Vet. Sci. 22, 557–564. doi: 10.24425/pjvs.2019.129964
Kruskal W., Wallis W. (1952). Use of ranks in one-criterion variance analysis. J. Am. Stat. Assoc. 47, 583–621. doi: 10.1080/01621459.1952.10483441
Lean I. J., LeBlanc S. J., Sheedy D. B., Duffield T., Santos J. E. P., Golder H. M. (2023). Associations of parity with health disorders and blood metabolite concentrations in Holstein cows in different production systems. J. Dairy Sci. 106, 500–518. doi: 10.3168/jds.2021-21673
Liebminger M. (2021). Parameterschätzung für Stoffwechselmerkmale beim Fleckvieh. University of Natural Resources and Life Sciences, Vienna, Vienna, Austria. Diploma Thesis.
Reist M., Koller A., Busato A., Küpfer U., Blum J. W. (2000). First ovulation and ketone body status in the early postpartum period of dairy cows. Theriogenology 54, 685–701. doi: 10.1016/S0093-691X(00)00383-6
Rutherford A. J., Oikonomou G., Smith R. F. (2016). The effect of subclinical ketosis on activity at estrus and reproductive performance in dairy cattle. J. Dairy Sci. 99, 4808–4815. doi: 10.3168/jds.2015-10154
RZA Annual Report. (2022). Available at: https://www.rinderzucht.at/downloads/jahresberichte.html (Accessed December 4, 2023).
Sailer K. J., Pralle R. S., Oliveira R. C., Erb S. J., Oetzel G. R., White H. M. (2018). Technical note: Validation of the BHBCheck blood β-hydroxybutyrate meter as a diagnostic tool for hyperketonemia in dairy cows. J. Dairy Sci. 101, 1524–1529. doi: 10.3168/jds.2017-13583
Suthar V. S., Canelas-Raposo J., Deniz A., Heuwieser W. (2013). Prevalence of subclinical ketosis and relationships with postpartum diseases in European dairy cows. J. Dairy Sci. 96, 2925–2938. doi: 10.3168/jds.2012-6035
Vanholder T., Papen J., Bemers R., Vertenten G., Berge A. C. (2015). Risk factors for subclinical and clinical ketosis and association with production parameters in dairy cows in the Netherlands. J. Dairy Sci. 98, 880–888. doi: 10.3168/jds.2014-8362
van Straten M., Friger M., Shpigel N. Y. (2009). Events of elevated somatic cell counts in high-producing dairy cows are associated with daily body weight loss in early lactation. J. Dairy Sci. 92, 4386–4394. doi: 10.3168/jds.2009-2204
Keywords: ketosis, mid infrared spectra, milk components, herd management, dairy cattle
Citation: Köck A, Dale LM, Werner A, Mayerhofer M, Auer F-J and Egger-Danner C (2024) Ketosis risk derived from mid-infrared predicted traits and its relationship with herd milk yield, health and fertility. Front. Anim. Sci. 5:1367210. doi: 10.3389/fanim.2024.1367210
Received: 08 January 2024; Accepted: 12 March 2024;
Published: 19 April 2024.
Edited by:
Oleksiy Guzhva, Swedish University of Agricultural Sciences, SwedenReviewed by:
Vincenzo Lopreiato, University of Messina, ItalyFabio Abeni, Consiglio per la Ricerca in Agricoltura e l’Analisi dell’Economia Agraria (CREA), Italy
Copyright © 2024 Köck, Dale, Werner, Mayerhofer, Auer and Egger-Danner. This is an open-access article distributed under the terms of the Creative Commons Attribution License (CC BY). The use, distribution or reproduction in other forums is permitted, provided the original author(s) and the copyright owner(s) are credited and that the original publication in this journal is cited, in accordance with accepted academic practice. No use, distribution or reproduction is permitted which does not comply with these terms.
*Correspondence: Astrid Köck, koeck@zuchtdata.at