Showcasing a digital twin for higher educational buildings: developing the concept toward human centricity
- Department of Energy Technology, KTH Royal Institute of Technology, Stockholm, Sweden
Digital twin technology is an emerging technology within the built environment. Yet, there are many unexplored opportunities to utilize digital twins for facilitating the transformation toward a climate-neutral building stock while also meeting the expectations from the building occupants. This article presents a case study of a digital twin, developed for an existing commercial building stock of campus areas in Sweden. The overarching purpose of the digital twin is to support both building occupants and building operators. This two-fold human-centric approach represents a novel approach for building digital twins. The digital twin is based on 3D scanning, and together with geospatial data, a real-like navigational indoor environment is created. Three innovative features are presented: the building analysis module, the digital twin mobile application, and the building operations module. The results show that the digital twin improves the building occupant’s experience by supporting navigation and providing access to the room booking system via this dedicated interface. Building management is also benefited by the digital twin through easier access to building data aggregated into one platform and a state-of-the-art analysis tool for optimizing the use of indoor space. The digital twin holds future potential to achieve operational excellence by incorporating feedback mechanisms and utilizing artificial intelligence to enable intelligent fault detection and prevention.
Introduction
The era of Industry 4.0 revolutionizes technology development at a pace that has never been seen before. Digital transformation is brought forward by the fourth industrial revolution and its concepts, such as Internet of Things (IoT), machine learning (ML), and artificial intelligence (AI), which are expected to contribute to the social, economic, environmental, and sustainable welfare (Morteza, 2020; Arsiwala et al, 2023).
Digital twin technology is one of these emerging technologies that has successfully been adopted by, for example, the manufacturing and aerospace industry where this technology has improved the efficiency of processes by real-time monitoring, production control, and production planning (Liu et al., 2021). Digital twins are developed to help understand what may happen when a system is projected to change, as well as presenting the real-time current status of its physical counterpart.
It was not until recently that digital twins started to evolve within the built environment, and the literature is indicating that the building industry is running behind in digital twin development (Lu et al., 2019; Fuller et al., 2020; Kořínek et al, 2021; Hosamo et al., 2022a). Digital innovations for improving the way buildings are operated and utilized are yet to be validated in real building contexts as innovations for buildings are broadly represented by pilot studies (Hosamo Haidar et al., 2022b). Therefore, despite the successful use of the digital twin technology in other industries, it still remains to be proven what digital twins could offer to the built environment (Jiang et al., 2021). As the building industry faces critical challenges, such as meeting climate goals, lowering energy use, improving resource efficiency, and ensuring the indoor environmental quality (IEQ) the opportunities brought forward by digital twins for buildings are essential for sustainable development.
Innovative technologies are needed to assess the relationship between buildings and their occupants (Hosamo et al., 2022a). Europeans spend approximately 90 percent of their time indoors, being at home, in school, at work, or in other commercial buildings, such as sports and recreation facilities (European Commission, 2003). Potentially, this means that people are extensively exposed to indoor pollutions (European Commission, 2003). It is, therefore, crucial to ensure a good IEQ as this affects the health, performance, and wellbeing of the building occupants. According to the IEA EBC Annex 79 (O Brien et al., 2020), research agrees that buildings fail to meet such demands from the building occupants.
Despite the fact that most buildings are constructed for people and their activities, human–building relationships are little understood (O’Brien et al., 2020; Hosamo et al., 2022b). This is especially relevant for the energy performance gap, representing the gap between the predicted and the actual building energy use. Earlier research has proven that this gap can differ significantly, and this is a major challenge to achieve sustainable buildings (Delzendeh et al., 2017). When combining large datasets with a virtual model, digital twins show potential to utilize their innovative features to increase this understanding (Arsiwala et al., 2023), and it is, therefore, necessary to incorporate a human-centric approach when designing digital twins for buildings. In addition, the human perspective should also gain an increase in focus toward building management and the building operators assigned to ensure day-to-day operational excellence and IEQ.
This study presents a digital twin for an existing commercial building stock of higher educational buildings in Sweden, thus targeting the lack of literature on digital twins dedicated for university campuses (Arsiwala et al, 2023). The method for establishing the digital twin, including three human-centric digital twin features, is presented. These three features resemble each of the novel capabilities that have been validated in the case study context. The digital twin is developed with typical digital twin features, such as enhanced monitoring, improved control, and new prediction capabilities. However, more uniquely, it is also developed to optimize the use of the building space. The occupant-centric approach has been extended toward a human-centric approach, including both the building occupants and the building operators. Thus, the digital twin concept is developed to enhance the experience of the end-users and for facilitating the building operator’s daily work.
It should be noted that the digital twin is designed for the operational phase. Hence, considerations regarding the design phase, construction phase, and demolition phase are excluded. In addition, the digital twin is developed for higher educational buildings; therefore, its replicability for other branches within commercial buildings and residential buildings cannot be validated.
The remainder of this article is organized as follows: Section 2 provides a background and literature review of digital twins for buildings, focusing on the identified challenges, research gap, and digital twin features. Section 3 presents the digital twin design process and the three digital twin features. Section 4 presents the results, key findings, and targeted challenges in relation to what have been stated in the previous literature. Section 5 concludes the key learnings from the digital twin case study, and future research areas are suggested.
Background
Digital twins and their definitions
Digital twin technology originated in the 1960s when NASA implemented this technology to simulate spaceflight processes (Allen, 2021). In 2003, the term digital twin was coined by Michael Grieves in a course on Product Lifecycle Management at the University of Michigan (Grieves, 2005). Michael Grieves developed the concept toward the manufacturing industry, introducing the novel perspective of connecting a physical product with its virtual counterpart. The manufacturing industry adopted this concept, and it was proven to enhance productivity by the simulation of processes and prediction of faults. Often blamed to be a late adopter of new technologies, the building industry has recently started to investigate the benefits of the digital twin concept in the built environment (PropTech, 2023). This is reflected in the scarce amount of scientific articles and the fact that the number of publications did not start to evolve until 2018 (Opoku et al., 2021).
There are numerous definitions of a digital twin, and depending on the purpose and industry, definitions might vary accordingly (Liu et al., 2021). A succinct definition for digital twin technology for buildings has been provided by Bäcklund et al. (2022), who suggested the following definition of a digital twin for buildings:
“A digital model of a real system that coexists1 and exchanges operational data and other useful information on a near real-time scale so that the operation of the real system can be influenced.”
According to the IEA EBC Annex 79, the term occupant-centric is defined as follows: “an occupant-centric building design means to keep the occupant’s needs (comfort and health) as a priority and to use explicit occupant modeling to design for and recognize the bi-directional interaction between occupants and buildings” (O’Brien et al., 2020). As mentioned in the introduction, this study develops the occupant-centric term toward human-centric. There was no definition found for a human-centric digital twin in the literature; therefore, the following definition is proposed:
“A human-centric digital twin also incorporates compliant building occupant data, along with real-time building data visualized in a user-friendly interface for both building occupants and building management.”
Literature review on digital twins for buildings
A systematic literature review methodology was used to frame the human-centric digital twin concept for buildings and to identify the research gaps and challenges from the current state-of-the-art research. The literature review was performed according to the PRISMA framework, illustrated in Figure 1. The Web of Science database was selected for the literature review since it is acknowledged as the world’s oldest and leading scientific database (Li et al, 2018). The search keywords were combined as follows: “digital twin” AND (“human-centric” OR “buildings”). The search process was refined by selecting the “Topic” field, and no time filter was applied. This search resulted in 342 articles published between 2019 and 2023, where 70 articles were relevant for buildings.
The original idea behind creating a digital twin for buildings was to improve monitoring and control to enhance building performance (Boje et al., 2020). Since most buildings are designed and constructed for people, an occupant-centric approach becomes inevitable. Recently, researchers have developed the digital twin concept toward an occupant-centric approach.
In this study, a human-centric aspect involves both the building operators and the building occupants as key stakeholders for building digital twins. A total of 19 of the articles were found eligible for this reasoning. Asad et al. presented a literature review of human-centric digital twins (Asad et al., 2023). They found that this concept is new to digital twins and is under development. By the means of real-time data and analyses, a digital twin for buildings could be utilized for improving building occupant thermal comfort (Hodavand et al, 2023). A few studies also incorporated feedback from the building occupants. Gnecco et al. claimed a human-centric digital twin approach by the means of wearable sensors and subjective survey data about indoor comfort (Gnecco et al, 2023). Donkers et al. (2022b) presented a proof-of-concept study where a method for assessing building occupants’ experiences was evaluated. They collected data and feedback via a smartwatch application. The occupant heart rate, outdoor visibility, and the time of the day were also measured. The occupants provided feedback on whether they were uncomfortable or comfortable, and their preferences were integrated using linked data. The researchers argued that their method contributed to digital twin technology development since their results captured the building occupants’ preferences and thereby enabled an increased understanding of the building occupants’ experiences. It was also argued that this method allowed for an improved understanding about how to operate a building with the consideration of the occupants’ experiences.
A total of 19 articles were literature reviews aiming at investigating the digital twin concept within the architecture, engineering, construction, and facility management (AEC-FM) industry. According to the literature, digital twins for buildings are suggested to serve as enablers for enhanced operation and maintenance and to achieve intelligent control and fault prediction and detection, which could lead to maintenance savings (Hosamo et al., 2022a; Zhao et al., 2022; Hosamo et al., 2023a). Hosamo et al. presented a digital twin applied as a pilot system for two buildings, where one key result was that the digital twin could detect and diagnose 17 more faults than the traditional building management system. In addition, the digital twin also predicted faults that would occur within 2 months (Hosamo et al., 2023a).
A total of 12 articles presented conceptual digital twins for buildings, where the prototypes lack real-world validation. A total of 32 articles presented real-world digital twins demonstrated in a physical building. Digital twins have traditionally been used to optimize one single asset (Jafari et al., 2020). Accordingly, few studies deal with digital twin technology for the entire operation and maintenance of a building (Zhao et al., 2022). Several studies presented digital twins that specifically targeted one building feature, such as light (Seo and Yun, 2022) or the indoor air quality (Arsiwala et al, 2023).
Arguably, the novelty of the digital twin concept for buildings results in a misinterpreted concept that is used without consistency. For example, digital twins were found to be classified as BIM models (Kaewunruen et al, 2019). In addition, none of the proposed digital twins is a real digital twin as per the definition, which would require a bi-directional link that can provide automated control and the optimization of the physical building based on the digital twin simulations. Hence, current building digital twins are merely digital building visualization and monitoring tools. As an example, Nurumova et al (2022) created a digital twin based on a BIM model for monitoring the indoor environment in a university building.
The common identified research gaps and challenges are summarized in Table 1. Given that digital twin technology is new to building design, construction, and operation, there are many challenges and research gaps yet to be discovered and resolved. What seems to be one of the major challenges is data integration (Donkers et al, 2022b; Hosamo et al., 2022b; Hosamo et al., 2023a; Arsiwala et al, 2023; Gnecco et al, 2023). This is especially relevant for commercial buildings that typically host several different types of building systems with different data formats, delivered by multiple system providers (Hosamo et al., 2022a). These systems are not compatible as data are stored in aggregated silos, which makes it difficult to merge them into one platform. There is also a lack of research on the integration of building data and building occupant data into one platform (Tang et al., 2019; Boje et al., 2020; Fuller et al., 2020; Kořínek et al, 2021; Nurumova et al, 2022). Furthermore, open data access is identified as a key concern for developing digital twins for the built environment. Locked-in business models and data ownership aspects work as bottlenecks for enabling open data access. The dominance of proprietary building systems delivered by the competing key players might be one explanation for the building sector to be running behind in streamlining business concepts together with technology development. Finally, there is a research gap on how to operate buildings, according to the expectations of the building occupants (Boje et al., 2020; Fuller et al., 2020; Nurumova et al, 2022; Arsiwala et al, 2023). Although there is plenty of research dealing with sensor technology and building data integrations, incorporating building occupants’ feedback and preferences is less studied and requires attention from the research society (Donkers et al, 2022a).
Building digital twin features
Table 2 presents an overview of the digital twin features commonly presented in the literature. These are divided into conceptual, operational, and human-centric features. Some features are considered more critical than others. According to Arsiwala et al (2023), a digital twin for buildings should consist of a wireless sensor network (WSN), data analytics, and a 3D BIM model. These three attributes are considered throughout the literature to be critical for establishing a digital twin, enabling real-time data visualization and advanced decision-making. IoT sensors are also considered essential for digital twins, measuring properties related to IEQ, such as temperature, relative humidity, the total volume of indoor volatile organic compound (TVOC) concentrations, indoor air quality, eCO2 (estimated concentration of carbon dioxide), and illuminance. A few studies also measured the physical properties of the building occupants, such as heart rate. These data were collected via a smartwatch application to understand the building occupants’ perception of their indoor comfort (Donkers et al, 2022b; Gnecco et al, 2023). Gnecco et al. (2023) also collected the skin temperature via the smartwatch application, and it was especially stated that human-centric data are necessary for a digital twin (Gnecco et al, 2023). Furthermore, Donkers et al. (2022a) collected meteorological data (temperature, weather type, wind speed and direction, humidity, and air pressure) via the following website: www.timeanddate.com/weather. An optional feature is ML, serving as a complement as it contributes to a higher level of functionality while providing advanced decision-making (Arsiwala et al, 2023).
The building digital twin toward human centricity
Higher educational buildings provide group study rooms, classrooms, computer rooms, laboratories, offices, auditoriums, and common areas, such as libraries and dining halls. In these environments, the people flow is dense. Visitors, staff, students, and researchers use these buildings extensively, often also outside normal office hours, including weekends and holidays. The other group of key stakeholders relevant for this building type is the building management; foremost, the building operators being responsible for fault management and interactions with building occupants. Thus, the building operators are tasked to ensure operational excellence, which essentially means to ensure an acceptable trade-off between IEQ and building energy use. As higher educational buildings have these key stakeholders with different objectives, the users’ purpose of a digital twin will also vary accordingly. The human-centric design is, therefore, identified as a key component that is essential to be incorporated in the digital twin concept to enhance the user experience for both parties, the building occupants and the building operators.
Figure 2 demonstrates the relationship between these two identified key stakeholders and the digital twin, resembled by the two loops. This is what we refer to as being human-centric.
The building occupants are represented on the left in Figure 2. The digital twin should ultimately work as a two-way communication tool where the building occupants should be able to communicate with the building operator through the digital twin interface to provide feedback about any perceived thermal comfort issue or other building-related faults. The building occupants should also be able to retain information about, for example, available rooms or relevant building status. The building operators are represented on the right in Figure 2. The digital twin should also be able to support the building operators in their daily work by gathering relevant building data in one interface. This is expected to enable improved analysis to ensure operational excellence. By definition1, a digital twin should be able to exchange data with its physical counterpart. Therefore, the two-way arrows represent a theoretical bi-directional data flow among the building occupant, the digital twin, and the building operator.
The digital twin from a system perspective
The system definition used in this study was adopted from C.W. Churchman, who argued that “a system is a set of parts organized to accomplish a set of goals” (Churchman, 1968). The system is defined as starting with its purpose. This leads to a discussion on the necessary components and their aims. Figure 3 presents the conceptual system model for a building digital twin, emphasizing internal and external relationships and the interdependence of selected data from within and outside the building boundaries. Thus, a digital twin representing a physical building should be defined within two system boundaries of a building’s local environment and a building’s global environment. The system’s local environment refers to the internal context where the building and the digital twin can exchange the same source of data, for example, the indoor temperature or the number of occupants, denoted as Datain. In addition, a digital twin should also be able to collect external data, Dataex, from a system global environment that refers to an external context outside the building boundaries, for example, weather forecasts or electricity prices. Such third-party data are important for an optimized operation or advanced analysis for the HVAC system performance or building energy use.
The digital twin presented in this study follows the definition of essential digital twin components, provided by Grieves (2005). Thus, as illustrated in Figure 3, there are three entities: the physical entity (the real building), the virtual entity (the digital twin), and the data connections (dataex and datain). It should be noted that the dotted arrow in Figure 3 represents third-party data that have a direct influence on the building. However, for an ideal digital twin system, the dotted arrow would not exist since all external data would first be processed via the digital twin and, thereafter, by the means of data analytics, suggest improvements for relevant building systems.
The ability to exchange data links the physical building with its virtual counterpart. This bidirectional data flow in this case study is defined as near real-time. Using the wording “near” emphasizes that sensor signals from the physical building to the digital twin occur with a defined interval of 10 min. This time interval was considered suitable; on account of this, no time-critical factors are displayed in the digital twin interface. Time critical events are handled through the building control system, thus separated from the digital twin.
The digital twin design process
The building stock captured in this digital twin possesses a great variation in constructions and designs. Due to the age of the existing building stock, mostly hard copy drawings were accessible, along with a few BIM models for recently constructed buildings. Although some buildings are recently constructed, other buildings were constructed in the 19th century and have undergone numerous renovations. These renovations have transformed design and the intended use of the buildings over time, and therefore, the available drawings were considered to be outdated.
Figure 4 describes the chosen design steps for the digital twin, involving the essential development phases: pre-study, sensor installation, and model generation using RealityCapture and the model population. These phases are briefly described under the following sub-headings. The Microsoft Azure IoT platform is used as integration toward the digital twin platform. The IoT platform is dedicated to collecting and processing IoT-sensor data; sensor data from smart building systems; technical documentation from word, excel, or the facility management system; and external third-party data. For more information about technical details, the authors of this article refer to a conference paper where these phases are described in detail (Bäcklund et al., 2022).
The pre-study phase
The first phase is the pre-study phase aiming to bring the building owner and tenant together to create a common vision about the digital twin. This is carried out through workshops where representatives from the building owner and the tenant participate. Representatives from the tenant were staff working at the university real estate and facility service and the IT office. These professionals have strategical knowledge about the facilities and room booking systems. From the building owner’s perspective, real estate developers participated together with business developers, IT managers, and project leaders. The workshops were facilitated by external project leaders according to a pre-defined process. The process aimed at delivering an effective workshop with a clear purpose and goal.
One important aspect of the pre-study phase is the inventory of which rooms to include in the digital twin as some buildings and rooms are not suitable to monitor, due to sensitive information. Eligible rooms and buildings are categorized depending on their size and function. Ensuring a consistent terminology of rooms in tenant systems and building owner systems is another important task.
It also needs to be defined which physical properties that are relevant to measure. The default properties are occupancy, temperature, and CO2 levels. Furthermore, noise measurement sensors are installed in rooms generally perceived as being noise sensitive, such as libraries. The type of sensor to be installed is also decided. In some buildings, traditional building systems have been replaced with smart building systems, such as smart HVAC and smart lighting systems, already equipped with sensors, measuring, for example, presence and CO2 level. The digital twin can collect these data from the building management system, and therefore, few or no additional sensors are required in such settings.
The sensor installation phase
The second phase is the sensor installation phase where the sensors are installed in strategic locations. Before the actual sensor installation can take place, an inventory is performed for eligible rooms and to decide what to measure in which room. Table 3 presents common room types and their physical properties to be measured. The rooms are carefully selected to produce compliant data to ensure privacy and anonymization of the building occupants, in line with the European General Data Protection Regulation, GDPR. The GDPR aims to ensure the right to everyone’s privacy, both at home and the workplace (GDPR, 2018). Personal data are defined as “any information that relates to an individual who can be directly or indirectly identified from it” (GDPR, 2018). The building occupants should not encounter any risk to be identified by the means of sensor data. The sensor installation phase also involves determining ownership and access to data, ensuring that data are managed and stored according to the current regulations.
The model generation phase
The third phase involves the actual scanning of the building. If 2D drawings are available in any computer-aided design software, for example, AutoCAD, these could be imported to the BIM software application Autodesk Revit, to create a digital 3D geometry (Hadjidemetriou et al., 2023). For this case, where the geometry must be re-established, a scanning technology was utilized. Before scanning can take place, an inventory list of rooms selected for the digital twin is provided to the scanning personnel. Laser scanning enables reality capture and is performed via a backpack-based indoor mobile mapping system with a 360-degree camera. RealityCapture is the software’s name, and it gathers precise data about an already existing environment (Almukhtar et al., 2021). RealityCapture uses a photogrammetry software application developed for the purpose of creating 3D models of an existing indoor environment from camera photographs or laser scans and is, therefore, suitable for designing a digital twin from an already built environment. LiDAR (Light Detection and Ranging) is a laser technology that captures any given surface in 3D, and it enables precision in scanning with a point density of 5 mm. In addition, the scanning hardware is complemented with GNSS (Global Navigation Satellite System) hardware that provides georeferencing to give every point its exact location.
For higher educational buildings, ensuring GDPR compliant data becomes especially relevant when scanning office environments. Common areas were scanned when there were no people present. A comprehensive GDPR investigation was also performed, where the scanned pictures were validated via an algorithm trained to recognize people. If the algorithm identifies any people, it will blur their faces to ensure anonymization. In addition to the algorithm, information security professionals validated each picture manually. This manual process was time-consuming and required specialized resources, but it was necessary as a complement to the automatic process to ensure GDPR compliance.
The model population phase
The fourth phase is where data are processed and integrated into the digital twin platform. The georeferenced point cloud addressed in images using a 360-degree camera is integrated with other data sources, such as facility management systems, third-party systems, and sensor data. Data from word documents, BIM objects, and CAD drawings could also be imported. Any type of selected object could be defined as a point of interest (POI) and be equipped with relevant information, such as installation documents or technical specifications. Determining which POI to include is an interchangeable process, which means that objects could be added or removed depending on their purpose. Figure 5 illustrates an example of the digital twin interface of an open office area where sprinklers (SP) and the heating system (VS) are objectified as POIs. Other POIs are, for example, any electrical equipment, such as lighting, electrical outlets, and fuse boxes (EL), or general objects (G), for example, windows or doors. Clicking on each object provides more information, such as the geographical location and access to technical documents. Thereby, this digital twin represents a semantically rich digital twin where any type of relevant building feature can be mapped. The digital twin project team carefully evaluates which objects to insert as POIs. Furthermore, important properties, such as the navigation capability and building characteristics, are also integrated.
The digital twin collects and visualizes third-party data, defined as external information (Figure 3) via application programming interfaces (APIs). Its examples are data from room booking systems, noise maps from the municipality, flood risk maps, and fire risk maps from the Swedish Civil Contingencies Agency, local plans from municipalities, infrastructure maps from power grid companies, and market electricity prices from the Nordic power market, Nord Pool. As an example, Figure 6 illustrates the climate risk analysis where flood risk data are integrated with a campus map to simulate which buildings are at risk for flooding. It should be noted that such external data are not provided in near real-time.
The building analysis module
Figure 7 illustrates the building analysis module, developed with the intention to provide occupancy data to visualize behavioral patterns and trends about how the buildings are used. The upper graph in Figure 7 shows the aggregated use and booking in percentage, per week for a particular building (highlighted in the map to the right). The lower graph in Figure 7 shows the use capacity per hour during a workweek, Monday–Friday. The graphs in Figure 7 can also be selected with a finer granularity, to analyze specific floor plans and rooms. The legend under the graph represents the color scheme, where darker colors represent a higher use and lighter colors represent a lower use.
The module collects external data from room booking systems. These scheduling data are compared with the occupancy and presence sensor data. By combining these two types of data, the rate of the so-called “no-shows” will be revealed. No-shows are defined as rooms booked but not used. The goal is to improve the room vacancy and to promote sustainable behavior regarding the use of rooms. As a result, it is expected that buildings will be better utilized, resulting in more available space and, in the long-term, a decreased demand for new buildings.
The tenants receive monthly status reports about the use of their facilities. The data reports are constructed via Power BI2 and are based on the sensor data. The tenants use the digital twin interface as a visual complement to the Power BI reports. Analyzing the sensor data in this module will also help us understand which facilities are more popular per the given time unit, which creates an understanding that will help develop facilities according to user needs.
The digital twin mobile application
A mobile application was developed from the digital twin 3D interface, delivering a state-of-the-art application, allowing the users to access the digital twin on their mobile phones. The application is developed to target the needs of the building occupants by providing relevant building information in near real-time. The end-users, especially students, have been involved in the design process, providing input on which features the application should have to target their needs. Thus, presenting occupancy sensor data in near real-time enables building occupants to gain information about available rooms or less busy indoor areas. Displaying presence data makes it easy to locate unoccupied spaces and saves time. Room booking systems will also be integrated, which means that building occupants can use their mobile phones to manage their room bookings.
Figure 8 illustrates the design of the digital twin mobile interface. At the first sample interface [A], a country map is displayed where available campus areas are presented. At the second sample interface [B], a campus area was selected. At the third sample interface [C], a specific building and floor plan 2 were selected. At the fourth sample interface [D], the user searched for a “study room.” Based on occupancy sensor data and data from room booking systems, the application will suggest the available rooms.
Figure 9 illustrates the wayfinding feature, which is developed to serve as a digital assistant for building occupants to locate themselves within a campus area and its buildings. Navigating campus buildings is often difficult, especially for visitors or newcoming students and staff. The wayfinding function is expected to serve as a complement to physical signs and enable an improved orientation within larger building complexes.
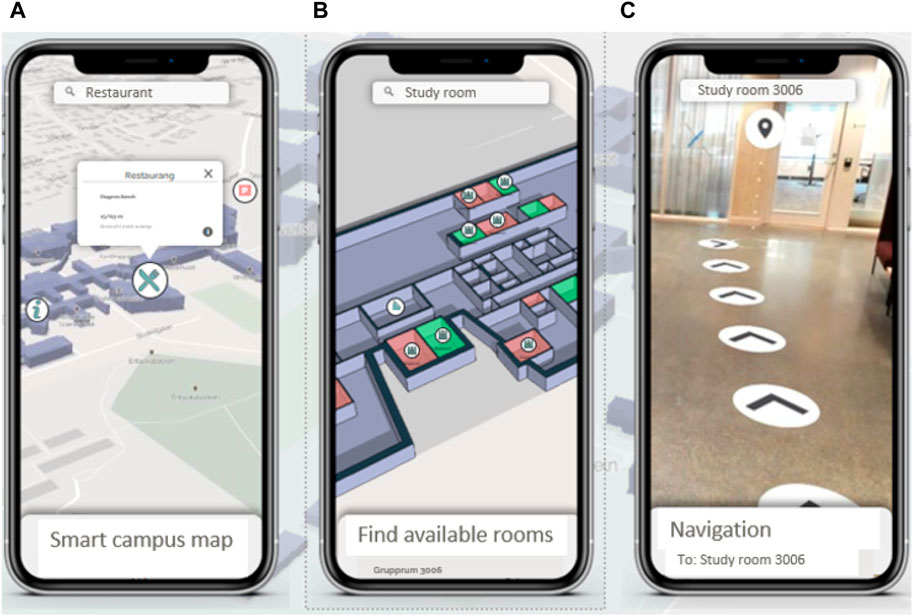
FIGURE 9. Sample interfaces (A–C) of the digital twin mobile application with the room locator and the wayfinding feature.
At the first sample interface [A], the user searched for the term “Restaurant;” hence, the application will display restaurants located in the campus area. At the second sample interface [B], the user searched for the term “study room.” Based on presence sensor data, the application will display the available study rooms. At the third sample interface [C], the application uses the navigational feature to direct the user to “Study room 3006”.
It should be noted that Figures 8, 9 present the results of establishing this first mobile version of the digital twin.
The building operations module
The building operations module has been developed to gather data and relevant building information into one platform to support the building operators in their daily work. There are several benefits from gathering building data into one platform as it improves decision-making processes, and it also offers an improved understanding of the relationships between various stakeholders (Gnecco et al, 2023). Through its smart visualization interface, where the building operators can identify errors and abnormal variations, fault detection and prevention will be better managed (Nurumova et al, 2022). Available real-time data will also provide a basis for taking immediate actions toward improved indoor comfort (Nurumova et al., 2021). Furthermore, by the means of visualizing sensor data to monitor how the building systems operate, the building operators can track the state of each building continuously (Hosamo et al., 2023a).
This module was developed by the means of an interactive action-based research process involving the end-users. In-depth interviews and workshops were performed during a longer period of time. The human-centric aspect was a key concept, involving the end-users in the design process and allowing them to test the module and provide feedback. The module was developed by professionals being experienced in facility management to ensure building operators’ purpose-driven goals.
Figure 10 demonstrates a sample interface of the building operations module. Section 1 shows the campus area and buildings to review. In this sample, Campus Umeå University was selected. There is also a drop-down list at the building level. Section 2 shows an overview of the relevant building alarms related to indoor climate and energy use. Section 3 visualizes a to-do list of error reports, including a priority list. Some building errors are more critical than others, and therefore, it is important to prioritize amongst all the reported and identified events (Hosamo et al., 2023a). Section 4 visualizes the average temperature per building, as well as ventilation effectivity. The circle diagram in Section 5 visualizes the allocation of surveillance per ventilation, cooling, heating, elevators, and general construction parameters.
Results and discussion
The digital twin presented in this study extends the occupant-centric concept to a human-centric approach, thereby raising the importance of several key stakeholders within building boundaries. The digital twin interface allows the users to navigate in a real-like 3D environment, and the navigational 3D model is developed from a geographical model that provides millimeter precision and, thereby, represents a life-like copy of the physical environment. The digital twin is based on cloud computing and the Microsoft Azure IoT platform, specifically developed for the creation of digital twins. It is an adaptive tool that suits the built environment since it can be designed according to the local conditions with object models, and it can also be populated according to specific goals (Mateev, 2020).
The three developed digital twin features require the integration of technical data, together with building occupant data, into one platform. This has been identified by several authors as an important research gap (Hosamo et al., 2022a; Donkers et al, 2022a; Hosamo et al., 2023a; Arsiwala et al, 2023; Gnecco et al, 2023). This study also targets an identified research gap of building asset management (Arsiwala et al, 2023) by introducing the building operations module (Figure 10). Building management is complex and involves a broad variety of systems and requirements, and many systems are typically used in parallel (Hosamo et al., 2022a). Therefore, this module aims to collect and visualize all relevant building management data into one platform. By doing so, the building operators will have a quick access to data in one platform, which enables enhanced decision-making (Gnecco et al, 2023) and easier detection and prevention of faults (Hosamo et al., 2023a). It also aims to visualize complex building data in a coherent manner, considering that these data can be difficult to interpret (Hosamo et al., 2022b).
The building analysis module (Figure 7) was developed in close collaboration between the building owner and the tenants. This module aim to improve the use of building assets as many buildings and rooms are underutilized. The building analysis module enables analysis of the building use by the means of occupancy data together with data from room booking systems. This allows for an understanding of how, when, and where a certain indoor space is used. It also provides knowledge about which areas in a building are the most popular and during which hours these rooms are most frequently used. The preference of the room size and functionality will be revealed. This is important information for the building owner in order to ensure high-quality building facilities that fulfill the needs of the occupants. Furthermore, if existing buildings are better utilized, the demand for new buildings will decrease. Underutilized areas are costly and energy-inefficient, leading to less sustainable buildings.
The digital twin mobile application (Figures 8, 9) is a unique human-centric digital twin feature, offering the building occupants a life-like and navigational indoor environment. As higher educational buildings could be large and complex to navigate, the wayfinding feature will serve as an aid to help navigate in these areas. Moreover, the application provides users with near real-time information regarding occupancy statuses. This feature becomes particularly crucial during times of pandemic outbreaks, such as COVID-19, as it allows users to navigate toward unoccupied space. Additionally, integration with the room booking system ensures seamless access to available rooms. The application was developed together with the building occupants to ensure a user-friendly experience that serves the specific needs of the building occupants.
According to Figure 3, the digital twin presented in this case study has evolved from a novel system perspective with a strong focus on collecting such a variety of data to serve its goal of human centricity. This implies an emphasis on combining external and internal data to represent both a global and a local context of the system. It was anticipated that these two types of data would complement each other. In addition to building data, defined as internal data, integrating external data, such as electricity prices and weather forecasts, provides a basis for analyses and decision-making processes. This lead to smarter management and operation of the buildings, such as proactive planning for heavy rainfalls, securing buildings located in flood risk areas.
It should be acknowledged that there are still some developments that are lacking for this digital twin to fully meet the requirements of the digital twin definition. According to it, the digital twin should allow a two-way communication between the physical and virtual entity. Such a two-way connection should include the possibility for building systems to react or adapt to digital twin information. It is also crucial to assess human interactions and behaviors within the human–building relationship when developing a building digital twin. This is addressed by suggesting a feedback loop connecting the building occupants with the building operators through the digital twin interface. By doing so, the building management will gain direct information about how the building occupants experience IEQ parameters, such as thermal comfort. This feedback loop is conceptually illustrated in Figure 2 and is yet to be developed.
A challenge for building digital twins is that they must reflect their physical environment accurately. When there is a renovation or any other change that affects the building design, the digital twin needs to be updated (Bäcklund et al., 2022; Hosamo et al., 2023a). An outdated digital twin, based on faulty data, will affect its validity and credibility. How to efficiently update the digital twin in a timely and cost-effective manner without any discrepancies in the reliability of data remains a challenge. Updating the digital twin requires extensive resources and is expensive and time-consuming as the scanning process needs to be repeated for those areas that are modified. It also involves processes and routines on how to manage updates concerning external and internal data linked to the digital twin. A process for such updates is currently under development.
The digital twin collects sensor data from the building every 10 min. This specific time resolution was chosen as a trade-off between usability and cost-effectiveness, also considered as sufficient for the current aim and purpose of the digital twin. In this first version, the digital twin will not be utilized for any time-critical purposes. In addition, transferring, storing, and analyzing building data from sensors, building systems, and third parties via the IoT platform to the digital twin are computationally heavy and require energy-intensive data storage. According to these given reasons, a time resolution of 10 min is acceptable. To highlight the fact that the data to the digital twin are not generated continuously, the preposition of “near” is applied to real time. Some previous literature studies within the field of digital twins, also developed their concepts using “near real-time” to describe their intended time resolution (Lee et al., 2021; Lee and Lee, 2021; Barkokebas et al, 2023; Bragatto et al., 2023). However, none of these studies provided any specific system characterization of “near real-time.” Therefore, this study acknowledged this term within the proposed system perspective of digital twins for buildings (Figure 3).
It should also be noted that future developments of the digital twin and its features can benefit from AI. As an example, by the means of ML, predictive capabilities could enable that future faults are “discovered” before they occur. When to take appropriate actions could then be visualized in the building operations module by a signal. ML could also improve the building energy use by identifying abnormalities at an early stage. However, ML is not yet the standard in building operations, leaving it open for future research to study its capabilities.
Conclusion and future research
This article presents a digital twin for the operational phase of higher educational buildings. The results presented in this study contribute, with new knowledge, to the field of digital twins for higher educational buildings, building occupants, and building management. The digital twin is designed with a human-centric approach, targeting the building occupants and the building operators. The aim of the digital twin is to improve the building occupants' experience and enhance the daily work life of the building operators. This represents a novel perspective on how digital twins can be designed and used within the built environment. The digital twin was also established to develop enhanced monitoring, control, and prediction capabilities to improve operational excellence. Furthermore, the digital twin aims to improve the general use and occupancy by enabling advanced analyses based on combining sensor data and data from room booking systems.
If the existing building stock is designed and operated according to the requirements of the building occupants, it is likely to be better utilized and the demand for new buildings would decrease. Furthermore, its uniqueness is also represented by the fact that this is the only digital twin case study for educational buildings that presents a theoretical bi-directional link with the aim to optimize a building asset based on digital twin intelligence.
The main contribution of this study is the development of the three digital twin features, where each of them represents novel characteristics of digital twins for buildings: first, we have the building analysis module, where the use of the buildings can be analyzed with the goal of achieving a sustainable building stock by optimizing the already built environment. Second, a state-of-the art digital twin mobile application is introduced. To the knowledge of the authors, this is the first human-centric digital twin mobile application. It is based on the digital twin platform and also designed together with the users to ensure their expectations and user needs. Third, the building operations module developed for the building operators as a digital aid, where relevant building data and information are displayed within one system.
A definition for human-centric digital twins and a new system perspective is also introduced in this study. Thus, the digital twin is designed with a system perspective of a local and global system environment, to highlight these interdependent relationships. It is also emphasized that for the purpose of this digital twin, data are updated every 10 min. This is what is referred to as near real-time data. These concepts are essential for the development of the digital twin as they frame user perspectives, technical requirements, and data collection intervals.
This study has some limitations that should be recognized. First, case studies are always subject to being context-specific (Eisenhardt and Graebner, 2007), meaning that the digital twin presented in this article is developed for higher educational buildings where most of the building occupants are students and staff. Furthermore, the building stock is located in Sweden, thus represented by, for example, the Swedish climate, weather, building types, and building codes. Second, the near-real-time data on the 10-min interval might resemble a limitation in some user cases that require quicker building status updates. Third, the lack of a cost-effective procedure that keeps the digital twin up-to-date is an important limitation and also a challenge for future research.
To conclude, digital twin technology for buildings is an emerging field with several resent publications. This implies new opportunities to be discovered, along with challenges and bottlenecks yet to be identified. This study highlights the challenges and research gaps identified by the previous literature, emphasizing a novel approach on how a human-centric building digital twin can support end-users and facility management.
The following aspects are recommended for future research endeavors:
• The industry silos need to be broken to make data sharing possible between the building system suppliers and the digital twin key stakeholders and to achieve cost-effectiveness. Future research should, therefore, target cross-sectoral cooperation and innovative business models for data sharing, adapted to smart building technology and IoT features.
• From the property owner’s perspective, it is relevant to realize the potential brought about by the digital twin technology for enabling an increased understanding on how commercial buildings are experienced by the building occupants. This will bring knowledge about how and why certain buildings or rooms are utilized to a greater extent. By the means of such data, the building owner would be able to achieve an optimal level of usage and more sustainable buildings.
• A well-functioning digital twin for buildings needs to be updated regularly in a data-compliant manner, by a systematic and cost-effective scanning procedure. This remains a central challenge for future research.
• Further detailed empirical studies are required to develop and enhance the human-centric design aspects of digital twins for buildings.
• A future opportunity for the presented digital twin mobile application is to implement a two-way communication feedback loop between the building occupants and the building operators via the digital twin mobile interface. This would allow the building occupants to provide information about thermal comfort, errors, or any other building-related issues. The building occupants could also gain information about the status of indoor climate, general information about smart building systems, or any other building-related issues. This would enhance the user perspective of a smart building and it would allow the building management to learn more about the building occupants, their behavior, and their perceived thermal comfort.
A showcasing video of the digital twin is available upon request.
Data availability statement
The original contributions presented in the study are included in the article/Supplementary Material; further inquiries can be directed to the corresponding author.
Author contributions
KB: conceptualization, data curation, formal analysis, funding acquisition, investigation, methodology, project administration, resources, software, validation, visualization, writing–original draft, and writing–review and editing. MM: conceptualization, funding acquisition, methodology, project administration, supervision, and writing–review and editing. PL: conceptualization, methodology, project administration, supervision, and writing–review and editing.
Funding
The author(s) declare that financial support was received for the research, authorship, and/or publication of this article. This study was supported by the Swedish Energy Agency and the IQ Samhällsbyggnad, under the E2B2 program, grant agreement 2018-016237, project number 47859-1 (Cost- and Energy-Efficient Control Systems for Buildings).
Conflict of interest
The authors declare that the research was conducted in the absence of any commercial or financial relationships that could be construed as a potential conflict of interest.
Publisher’s note
All claims expressed in this article are solely those of the authors and do not necessarily represent those of their affiliated organizations, or those of the publisher, the editors, and the reviewers. Any product that may be evaluated in this article, or claim that may be made by its manufacturer, is not guaranteed or endorsed by the publisher.
Footnotes
1Thus, only a digital model cannot make up a digital twin.
2Power BI is a data visualization tool provided by Microsoft.
References
Abdelrahman, M. M., Chong, A., and Miller., C. (2022). Personal thermal comfort models using digital twins: preference prediction with BIM-extracted spatial-temporal proximity data from Build2Vec. Build. Environ. 207, 108532. doi:10.1016/j.buildenv.2021.108532
Allen, B. D. (2021). Digital twins and living models at NASA. https://ntrs.nasa.gov/citations/20210023699.
Almukhtar, A., Saeed, Z. O., Henry, A., and Tah, J. H. M. (2021). Reality capture of buildings using 3D laser scanners. CivilEng 2 (1), 214–235. doi:10.3390/civileng2010012
Arsiwala, A., Elghaish, F., and Zoher, M. (2023). Digital twin with machine learning for predictive monitoring of CO2 equivalent from existing buildings. Energy Build. 284, 112851. doi:10.1016/j.enbuild.2023.112851
Asad, U., Khan, M., Khalid, A., and Waqas, A. L. (2023). Human-centric digital twins in industry: a comprehensive review of enabling technologies and implementation strategies. Sensors 23 (8), 3938. doi:10.3390/s23083938
Bäcklund, K., Molinari, M., Lundqvist, P., and Karlsson, P. (2022). Showcasing the first steps towards a digital twin for campus environments. E3S Web Conf. 362, 10003. doi:10.1051/e3sconf/202236210003
Barkokebas, B., Al-Hussein, M., and Hamzeh, F. (2023). Assessment of digital twins to reassign multiskilled workers in offsite construction based on lean thinking. J. Constr. Eng. Manag. 149 (1), 04022143. doi:10.1061/(ASCE)CO.1943-7862.0002420
Boje, C., Guerriero, A., Kubicki, S., and Rezgui, Y. (2020). Towards a semantic construction digital twin: directions for future research. Automation Constr. 114, 103179. doi:10.1016/j.autcon.2020.103179
Bragatto, T., Bucarelli, M. A., Carere, F., Cresta, M., Gatta, F. M., Geri, A., et al. (2023). Near real-time analysis of active distribution networks in a digital twin framework: a real case study. Sustain. Energy, Grids Netw. 35. 101128. doi:10.1016/j.segan.2023.101128
Delzendeh, E., Wu, S., Lee, A., and Zhou, Y. (2017). The impact of occupants’ behaviours on building energy analysis: a research review. Renew. Sustain. Energy Rev. 80 (December), 1061–1071. doi:10.1016/j.rser.2017.05.264
Donkers, A., de Vries, B., and Yang, D. (2022a). “Knowledge discovery approach to understand occupant experience in cross-domain semantic digital twins,” in LDAC 2022 Linked Data in Architecture and Construction: Proceedings of the 10th Linked Data in Architecture and Construction Workshop co-located with 19th European Semantic Web Conference (ESWC 2022), Hersonissos, Greece, January, 2022, 77–86.
Donkers, A., de Vries, B., and Yang, D. (2022b). “Knowledge discovery approach to understand occupant experience in cross-domain semantic digital twins,” in LDAC 2022 Linked Data in Architecture and Construction: Proceedings of the 10th Linked Data in Architecture and Construction Workshop Co-Located with 19th European Semantic Web Conference (ESWC 2022), Hersonissos, Greece, January, 2022, 77–86.
Eisenhardt and Graebner (2007). Eisenhardt and graebner (2007).Pdf. https://josephmahoney.web.illinois.edu/BADM504_Fall%202019/Eisenhardt%20and%20Graebner%20(2007).pdf.
European Commission (2003). Text. Indoor air pollution: new EU research reveals higher risks than previously thought. https://ec.europa.eu/commission/presscorner/detail/en/IP_03_1278.
Fuller, A., Fan, Z., Day, C., and Barlow, C. (2020). Digital twin: enabling technologies, challenges and open research. IEEE Access 8, 108952–108971. doi:10.1109/ACCESS.2020.2998358
GDPR (2018). What is GDPR, the EU’s new data protection law? https://gdpr.eu/what-is-gdpr/.
Gnecco, V. M., Vittori, F., and Anna, L. P. (2023). Digital twins for decoding human-building interaction in multi-domain test-rooms for environmental comfort and energy saving via graph representation. Energy Build. 279, 112652. doi:10.1016/j.enbuild.2022.112652
Gregor, M., and Tibaut, A. (2020). “Ontology based information creation approach for digital twins: early-stage findings,” in Service oriented, holonic and multi-agent manufacturing systems for industry of the future (Cham, Germany: Springer International Publishing). doi:10.1007/978-3-030-27477-1_31
Grieves, M. (2005). Product lifecycle management: the new paradigm for enterprises. Int. J. Prod. Dev. - Int J Prod Dev 2, 71. doi:10.1504/IJPD.2005.006669
Hadjidemetriou, L., Stylianidis, N., Englezos, D., Papadopoulos, P., Eliades, D., Timotheou, S., et al. (2023). A digital twin architecture for real-time and offline high granularity analysis in smart buildings. Sustain. Cities Soc. 98, 104795. doi:10.1016/j.scs.2023.104795
Hodavand, F., Ramaji, I. J., and Sadeghi, N. (2023). Digital twin for fault detection and diagnosis of building operations: a systematic review. Buildings 13 (6), 1426. doi:10.3390/buildings13061426
Hosamo, H., Imran, A., Juan, C.-C., Paul, S., Svidt, K., and Nielsen, H. (2022b). A review of the digital twin technology in the AEC-FM industry. Adv. Civ. Eng. 2022, 1–17. doi:10.1155/2022/2185170
Hosamo, H. H., Henrik, K. N., Ammar, N. A., Paul, R. S., and Svidt, K. (2022a). A review of the digital twin technology for fault detection in buildings. Front. Built Environ. 8. doi:10.3389/fbuil.2022.1013196
Hosamo, H. H., Henrik, K. N., Ammar, N. A., Paul, R. S., and Svidt, K. (2023a). Digital twin framework for automated fault source detection and prediction for comfort performance evaluation of existing non-residential Norwegian buildings. Energy Build. 281, 112732. doi:10.1016/j.enbuild.2022.112732
Hosamo, H. H., Henrik, K. N., Kraniotis, D., Paul, R. S., and Svidt, K. (2023b). Improving building occupant comfort through a digital twin approach: a bayesian Network model and predictive maintenance method. Energy Build. 288, 112992. doi:10.1016/j.enbuild.2023.112992
Huynh, D., and Nguyen-Ky, S. (2020). Engaging building automation data visualisation using building information modelling and progressive web application. Open Eng. 10 (1), 434–442. doi:10.1515/eng-2020-0054
Jafari, M. A., Zaidan, E., Ali, G., Mahani, K., and Farzan, F. (2020). “Improving building energy footprint and asset performance using digital twin technology,” in Ifac papersonline (Amsterdam: Elsevier). doi:10.1016/j.ifacol.2020.11.062
Jiang, F., Ma, L., Tim, B., and Chen, K. (2021). Digital twin and its implementations in the Civil engineering sector. Automation Constr. 130, 103838. doi:10.1016/j.autcon.2021.103838
Kaewunruen, S., Rungskunroch, P., and Welsh, J. (2019). A digital-twin evaluation of net zero energy building for existing buildings. Sustainability 11 (1), 159. doi:10.3390/su11010159
Kořínek, M., Tázlar, O., and Štekerová, K. (2021). Digital twin models: BIM meets NetLogo. https://digilib.uhk.cz/bitstream/handle/20.500.12603/518/KORINEK_Milan_Ondrej_TAZLAR_Kamila_STEKEROVA.pdf?sequence=1&isAllowed=y.
Lee, D., Lee, S. H., Masoud, N., Krishnan, M. S., and Li, V. C. (2021). Integrated digital twin and blockchain framework to support accountable information sharing in construction projects. Automation Constr. 127, 103688. doi:10.1016/j.autcon.2021.103688
Lee, D., and Lee, S. H. (2021). Digital twin for supply chain coordination in modular construction. Appl. Sci. 11 (13), 5909. doi:10.3390/app11135909
Li, K., Rollins, J., and Yan, E. (2018). Web of science use in published research and review papers 1997–2017: a selective, dynamic, cross-domain, content-based analysis. Scientometrics 115 (1), 1–20. doi:10.1007/s11192-017-2622-5
Liu, M., Fang, S., Dong, H., and Xu, C. (2021). Review of digital twin about concepts, technologies, and industrial applications. J. Manuf. Syst. Digital Twin towards Smart Manuf. Industry 4, 346–361. doi:10.1016/j.jmsy.2020.06.017
Lu, Q., Kumar Parlikad, A., Woodall, P., Xie, X., Liang, Z., Konstantinou, E., et al. (2019). Developing a digital twin at building and city levels: case study of west cambridge campus. J. Manag. Eng. 36. doi:10.1061/(ASCE)ME.1943-5479.0000763
Morteza, G. (2020). Industry 4.0, digitization, and opportunities for sustainability. J. Clean. Prod. 252. 119869. doi:10.1016/j.jclepro.2019.119869
Nurumova, K., Ramaji, I., and Kermanshachi, S. (2022). “Leveraging digital twin for enhancing occupants’ comfort: a case study,”ASCE International Conference on Computing in Civil Engineering, Orlando, FL, USA, August, 2021, 417–424. doi:10.1061/9780784483893.052
Nurumova, K., Ramaji, I. J., and Kermanshachi, S. (2021). Leveraging digital twin for enhancing occupants comfort. A Case Study Proj., doi:10.1061/9780784483893.052
O Brien, W., Wagner, A., Schweiker, M., Mahdavi, A., Day, J., Mikkel, B. K., et al. (2020). Introducing IEA EBC Annex 79: key challenges and opportunities in the field of occupant-centric building design and operation. Build. Environ. 178, 106738. doi:10.1016/j.buildenv.2020.106738
Opoku, D.-G. J., Perera, S., Osei-Kyei, R., and Rashidi, M. (2021). Digital twin application in the construction industry: a literature review. J. Build. Eng. 40, 102726. doi:10.1016/j.jobe.2021.102726
Pan, Y., Braun, A., Brilakis, I., and Borrmann, A. (2022). Enriching geometric digital twins of buildings with small objects by fusing laser scanning and AI-based image recognition. Automation Constr. 140, 104375. doi:10.1016/j.autcon.2022.104375
PropTech (2023). PropTech3.0.Pdf. https://www.sbs.ox.ac.uk/sites/default/files/2018-07/PropTech3.0.pdf.
Researchgate (2015). Digital twin: manufacturing excellence through virtual factory replication. https://www.researchgate.net/publication/275211047_Digital_Twin_Manufacturing_Excellence_through_Virtual_Factory_Replication.
Seo, H., and Yun, W.-S. (2022). Digital twin-based assessment framework for energy savings in university classroom lighting. Buildings 12 (5), 544. doi:10.3390/buildings12050544
Tang, S., Shelden, D. R., Eastman, C. M., Pishdad-Bozorgi, P., and Gao, X. (2019). A review of building information modeling (BIM) and the Internet of Things (IoT) devices integration: present status and future trends. Automation Constr. 101, 127–139. doi:10.1016/j.autcon.2019.01.020
Visartsakul, B., and Damrianant, J. (2023). A review of building information modeling and simulation as virtual representations under the digital twin concept. Eng. Thail. 27 (1), 11–27. doi:10.4186/ej.2023.27.1.11
Wang, W., Hu, H., Zhang, J. C., and Hu, Z. (2020). “Digital twin-based framework for green building maintenance system,” in 2020 IEEE International Conference On Industrial Engineering And Engineering Management, Singapore, December, 2020 (IEEE). doi:10.1109/ieem45057.2020.9309951
Webofscience (2024). Seven metaphors to understand digital twins of built assets-web of science core collection. https://www-webofscience-com.focus.lib.kth.se/wos/woscc/full-record/WOS:000619313900001.
Wu, Y., Shang, J., and Fan, X. (2021). RegARD: symmetry-based coarse registration of smartphone’s colorful point clouds with CAD drawings for low-cost digital twin buildings. Remote Sens. 13 (10), 1882. doi:10.3390/rs13101882
Yalcinkaya, M., and Singh, V. (2019). VisualCOBie for facilities management: a BIM integrated, visual search and information management platform for COBie extension. Facilities 37 (7/8), 502–524. doi:10.1108/F-01-2018-0011
Keywords: digital twin, human-centric, higher educational buildings, building occupants, building operators, sustainable buildings
Citation: Bäcklund K, Lundqvist P and Molinari M (2024) Showcasing a digital twin for higher educational buildings: developing the concept toward human centricity. Front. Built Environ. 10:1347451. doi: 10.3389/fbuil.2024.1347451
Received: 04 December 2023; Accepted: 26 January 2024;
Published: 20 February 2024.
Edited by:
Chaoqun Zhuang, The Alan Turing Institute, United KingdomReviewed by:
Jiawei Huang, Environmental Systems Research Institute, United StatesJorge Luis Rojas Arce, National Autonomous University of Mexico, Mexico
Copyright © 2024 Bäcklund, Lundqvist and Molinari. This is an open-access article distributed under the terms of the Creative Commons Attribution License (CC BY). The use, distribution or reproduction in other forums is permitted, provided the original author(s) and the copyright owner(s) are credited and that the original publication in this journal is cited, in accordance with accepted academic practice. No use, distribution or reproduction is permitted which does not comply with these terms.
*Correspondence: Katarina Bäcklund, katbac@kth.se