Robust optimal damper placement based on robustness index simultaneously considering variation of elastoplastic design criteria and input level
- Department of Architecture and Architectural Engineering, Graduate School of Engineering, Kyoto University, Nishikyo, Japan
Dampers should be installed at appropriate quantities and locations to control building vibrations against excitations such as earthquakes and wind loads. One of the objectives of the structural optimization problem for damper placement is to minimize the initial cost of damper installation to satisfy various structural constraints under a set of input levels and target performance values. However, it is arbitrary what input levels should be used in the design, and it is also necessary to account for various uncertainties in the inputs and structural properties. This study presents a new method for assessing the robustness of building structures with design variables while simultaneously considering various phases of structural performance criteria and input amplitudes. The proposed robustness index is a multidimensional function that can take into account the influence of different input levels on the structural performance. In this paper, the proposed new robustness index is applied to the robust optimal design of the damper placement, where the damping coefficient of the linear oil damper added to the building is uncertain. The worst resonant seismic motion for the building is investigated based on the critical double impulse method and its equivalent one-cycle sine wave, which is used as the input seismic motion. By applying the equivalent one-cycle sine wave to the structural response analysis with variations in the input velocity amplitude, the proposed robustness index is effective in comprehensively assessing the relationships between the input velocity amplitude of the seismic motion and the upper response limit of the structure under the variation of the damping coefficient of the oil damper. The comprehensive and efficient evaluation of these relationships enables a more detailed assessment of the influence of uncertainties in design variables on structural performance. In the numerical examples, the optimal damper placement for a 12-story building model is discussed based on the robustness and structural performance of both acceleration and story ductility distribution.
1 Introduction
In the structural design of buildings, it is common practice to evaluate structural safety with respect to design criteria by calculating the maximum response of major structural components such as columns and beams and vibration control components such as oil dampers. These maximum responses are obtained by conducting time history response analysis to design seismic motion with the prescribed material properties and performance. In addition, from the viewpoint of rational design, many examples of structural optimization exist, with seismic response as one of the constraints (Fragiadakis and Papadrakakis, 2008; Kaveh et al., 2010; Gholizadeh, 2015; Papavasileiou and Charmpis, 2016). However, the components of a building may exhibit variations (i.e., uncertainties) in a given performance due to various factors such as construction errors, manufacturing variations, and aging. Since there is a concern that such variations in structural properties may cause the building response beyond the design criteria, it is necessary to understand and adequately account for the influence of the variations on structural performance during structural design.
To investigate the effect of uncertainties in structural properties on the seismic response of buildings, many studies have been conducted on uncertainty analysis methods, including those by Ben-Haim and Elishakoff (1990), Takewaki and Ben-Haim (2005), Henriques et al. (2008), Elishakoff and Ohsaki (2010), and Fujita and Takewaki (2011). One of the purposes of the uncertainty analysis is to find upper and lower bounds of response to parameters with uncertainties, and several efficient methods have been proposed. For example, the interval analysis method is one of the conventional uncertainty analysis methods, in which the uncertain parameters are defined as interval variables with lower and upper bounds. Various interval analysis methods have been proposed (Moens and Vandepitte, 2004; Chen et al., 2009; Moens and Hanss, 2011; Faes and Moens, 2020; Wang et al., 2022). In interval analysis, there is no need to use the probability quantities required for variation based on probability theory since it yields definite upper and lower bounds on the range of the variation of the parameters. Although the interval analysis approach is a classical method, it is easy to handle from a practical standpoint, and it will continue to be used as one of the effective methods to account for uncertainties such as design variables.
Robustness is defined as the resistance or stability of a building performance against various types of uncertainties. In the field of engineering, the keyword “robust design” has been focused on in order to actively consider the variations caused by various uncertainties during design. For example, it has been desired to establish a robust building structure system based on the assumption of variations due to various factors (Doltsinis and Kang, 2004; Lagaros and Papadrakakis, 2007; Gokkaya et al., 2016). Furthermore, optimal design with the consideration of robustness is called robust optimal design. A robust structural optimization design means that there is less degradation of the structural response even in the worst-case variation. As one of the indexes to quantitatively evaluate robustness for optimal design, the robustness function was proposed by Ben-Haim (2006), where variation of uncertainty is assumed.
In recent years, seismic characteristics such as large-amplitude and long-period seismic motions have been clarified, and research on design methods for structures with additive dampers has been intensified (Zhang and Soong, 1992; Castaldo and De luliis 2014; Garivani et al., 2020; Nabid et al., 2020; Xiao et al., 2021). Structural design theory with additive dampers aims to improve damping performance with constraints on cost, worst-case response, and other factors. It is well-known that damper characteristics such as oil dampers may differ between actual and predetermined values due to various uncertainties such as temperature dependence, aging, and manufacturing errors. Fujita and Yasuda (2016) and Fujita et al. (2021) developed robust optimal damper placement problems considering variations in the damping performance of linear and nonlinear oil dampers, but they do not consider building plasticity.
On the other hand, it is important to consider not only the variation in structural properties but also the variation in inputs. Akehashi and Takewaki (2019) developed the optimal placement problem of oil dampers for a critical double impulse (DI) input for an elastoplastic multi-mass model. Critical DI is an input consisting of two impulses that simulate near-fault earthquake motion, and the upper limit of the displacement response can be obtained by acting on the second impulse at the most critical timing for the building. The upper limit of the acceleration response, as well as the displacement response, can be evaluated by back-substituting the critical DI to an equivalent one-cycle sine wave. When considering input variability, the critical DI is also useful in robust optimal design problems because it provides an upper limit of response for a given velocity amplitude
This paper proposes a new method to evaluate the robustness of elastoplastic buildings concerning uncertain damping coefficients of dampers, considering various variations across a wide range of input levels and performance criteria. The uncertain design variables are the damping coefficients of the oil damper added to the building. Compared to the previous study conducted by Fujita et al. (2021), this paper focuses on the influence of the input level variation on structural performance including elastoplastic behavior. In the proposed robustness index, the robustness function is extended to consider simultaneous variations in input levels and multiple design criteria. This new robustness index can be derived as a three-dimensional surface. This three-dimensional robustness index can comprehensively evaluate the relationship between the input level of the worst-case resonant seismic motion for buildings and the upper bound of structural responses, such as ductility ratio and floor acceleration. The input excitation is determined as an equivalent one-cycle sine wave according to the critical double impulse theory under the variation in the damping coefficient of the oil damper. By comprehensively and efficiently evaluating that, the risk of performance variability can be considered in more detail in the design. Furthermore, based on the proposed robustness index, a robust optimal design problem is presented to obtain the optimal damper placement while considering uncertainties and robustness evaluation under a wide range of input variations and corresponding performance criteria.
2 Critical double impulse and equivalent one-cycle sine wave
2.1 Critical double impulse
The fling-step seismic motion is known to be observed near surface fault raptures (Hisada and Tanaka, 2021). It is noted that the fling-step seismic motion is a pulsating seismic motion, and the waveform with the largest amplitude is similar to the sinusoidal shape. This kind of pulse-like seismic motions can also be extracted in the Pacific Earthquake Engineering Research Center (PEER) database, one of the world’s most famous seismic databases. Furthermore, long-period pulses, where the predominant period is approximately 3 s, were observed in the Kumamoto earthquake in 2016, and it is important to examine the safety of buildings against such pulsating seismic motions. In order to discuss the response characteristic of the building subjected to such pulse-like ground motion, Kojima and Takewaki (2015, 2016) proposed a method to approximate the one-cycle sine wave by composing a DI input, which is expressed as
where
In Eq. 2,
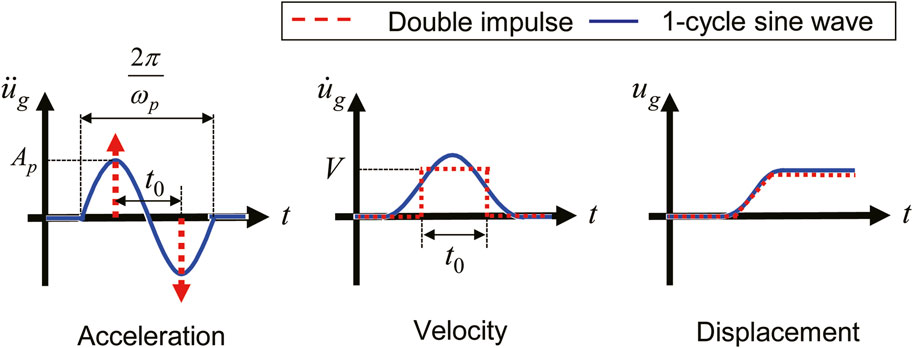
FIGURE 1. Relationship of the time–history of acceleration, velocity, and displacement between double impulse and the one-cycle sine wave.
Basically, since velocity amplitude
2.2 Equivalent one-cycle sine wave
For the response of a building to an impulsive input, from an energetic formulation point of view, kinetic energy is provided to the system at the moment the impulse acts. As one of the ways to take into account such a kinetic energy change in the framework of time history analysis, impulse amplitude
By using the one-cycle sine wave equivalent to the critical DI as the input, the input can also be regarded as resonant to the building. This means that the upper limit of the response to input uncertainty can be handled without searching for variations in the input characteristics. Furthermore, one-cycle sine waves are also appropriate for evaluating the maximum acceleration response of the building, because the input waves are continuously and smoothly varied that represent a portion of the actual pulse-like seismic wave. In this paper, the worst input timing
3 Evaluation of robustness against variations in uncertain parameters
3.1 Definition of the robustness function under varied input levels
Robustness is defined as a measure of the degree of variability of the responses or performance of the structure to various uncertainties derived by input and structural properties. In order to consider robustness during structural design and structural optimization, a quantitative measure of robustness is needed. As one of such quantitative robustness indices of a building against variations in uncertain parameters, Ben-Haim (2006) proposed a robustness function based on the info-gap model. Uncertainty parameters to account for variation can be categorized into probability-based and non-probability-based methods. The info-gap model belongs to the non-probabilistic uncertain model. An interval variable, one of the non-probabilistic uncertainty parameters, is defined as the upper- and lower-limit range of uncertain parameters, defined by the following equation:
where
The concept of the robustness function is shown in Figure 2. The left part of the figure represents the relationship between design variables and structural performance function. As the uncertain design domain represented by variable interval parameters is expanded by uncertainty degree
The authors previously proposed optimal design problems using this robustness function, but they conventionally assumed a single variable, such as input levels or performance criteria, and handled the other as a constant (Fujita et al., 2021; Hosoda and Fujita, 2023). However, the amplitude of input external disturbances varies based on the frequency of their occurrence, and it is common practice to set appropriate design criteria for such various input levels, so it is not appropriate to address only specific input levels. Therefore, in this paper, we also focus on changes in the magnitude of the input, i.e., the input velocity amplitude
where
3.2 Detailed definition of variables on the robustness function
The robustness function defined in Eq. 4 is a function whose variables are the nominal value
3.2.1 Nominal value of design variables assuming variation
In this paper, the design variable regarded as the uncertain parameter is the damping coefficient of oil dampers for each story added to the seismic vibration-controlled building, where
3.2.2 Performance function
The performance function
where
3.2.3 Performance criteria
Since the performance function in this paper is defined as the standardized multi-objective, as shown in Eq. 5, the performance criteria are given specified coefficients depending on
3.2.4 Input velocity amplitude
The one-cycle sine wave equivalent to the critical DI is used as the actual input seismic motion to the building in this paper. Since the input amplitude of the sine wave is dependent on the critical DI, the input seismic motion can be regarded as the function of the input velocity amplitude
3.3 Application of uncertainty analysis for the evaluation of the robustness function
In order to derive the robustness function defined in Eq. 4, an optimization problem is included that searches for the upper bound on the degree of uncertainty, where the uncertain parameters should be the worst combination to satisfy the specified performance criteria. Although it is difficult to determine the uncertainty bound corresponding to this implied optimization problem, it has been reported that the inverse problem of this optimization problem, i.e., finding the maximum performance function under a given uncertainty bound, can be useful for efficiently deriving the robustness function. This problem can be solved using the uncertainty analysis framework, where the upper and lower bounds of performance functions are derived. A flowchart for evaluating the robustness function in this paper is shown in Figure 3. This flowchart is a minor revision of the previously proposed uncertainty analysis method, called the NURP method. A detailed explanation of this flowchart is described in this section.
First, we consider the case to derive the robustness function of the relationship
Since the target building should be treated as an elastoplastic building model for various input levels including severe design loadings, it is concerning that there may be an unstable approximation where the performance function changes drastically due to uncertainties. This is mainly because, due to variations, the number of plasticizing stories increase or the maximum response stories for the worst response change. The NURP method uses a cubic function determined by the value of the evaluation point and its neighboring linear gradient when complementing between evaluation points, but if such points exist within the variation interval, a fairly large difference may exist between the critical variation calculated by the NURP method and the actual critical variation.
Therefore, in this paper, as a method to solve this issue without significantly increasing the computational time, we added a procedure to compare the actual response to the critical point calculated by the conventional NURP method with the response to the evaluation points at nominal, upper, and lower bounds of an uncertain parameter (phase 4 in Figure 3). That is, the point with the largest response among the four points is evaluated as the critical point to be updated.
3.4 Robustness function to the simultaneous variation in input levels and performance criteria
Figure 4 shows a conceptual diagram of the
In order to evaluate the three-dimensional robustness relationship in
4 Three-dimension robustness function with performance criteria and input velocity amplitude
4.1 Building model and oil damper
This section shows an example of the evaluation of the
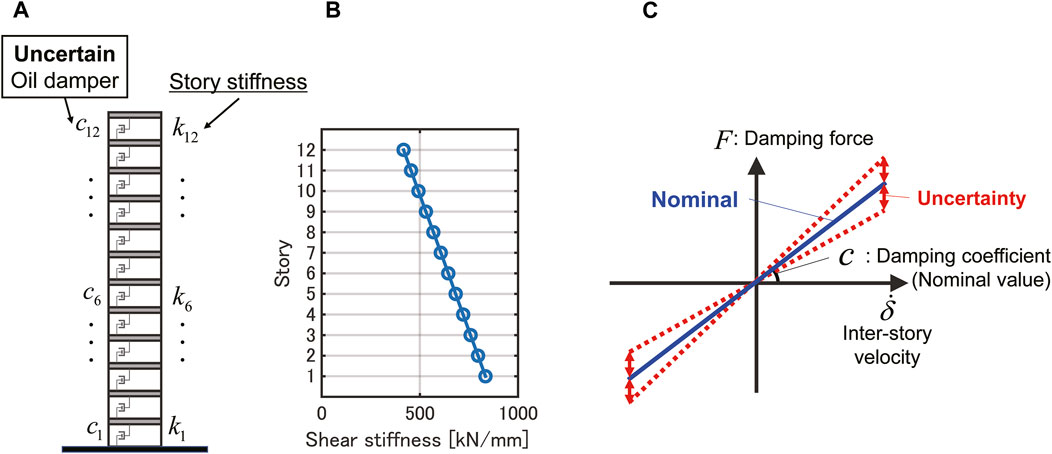
FIGURE 5. Structural properties of the 12 DOF model: (A) elevation, (B) story stiffness distribution, and (C) uncertainty of the damping coefficient.
The oil damper is assumed to be a linear damper, and in this section, the nominal damping coefficient of the damper at each story is proportional to the story stiffness, so that the added first-order damping factor due to the damper is 0.05. The variation in the damping coefficient is considered the uncertainty of the damping characteristics of the oil damper, as described above (Figure 5C). As a criterion for the range of the variation, we consider a variation of
4.2 An evaluation example of the relationship
Figure 6 shows a three-dimensional robustness function on the
In Figure 6, the
Next, to discuss the influence of uncertainties on the obtained
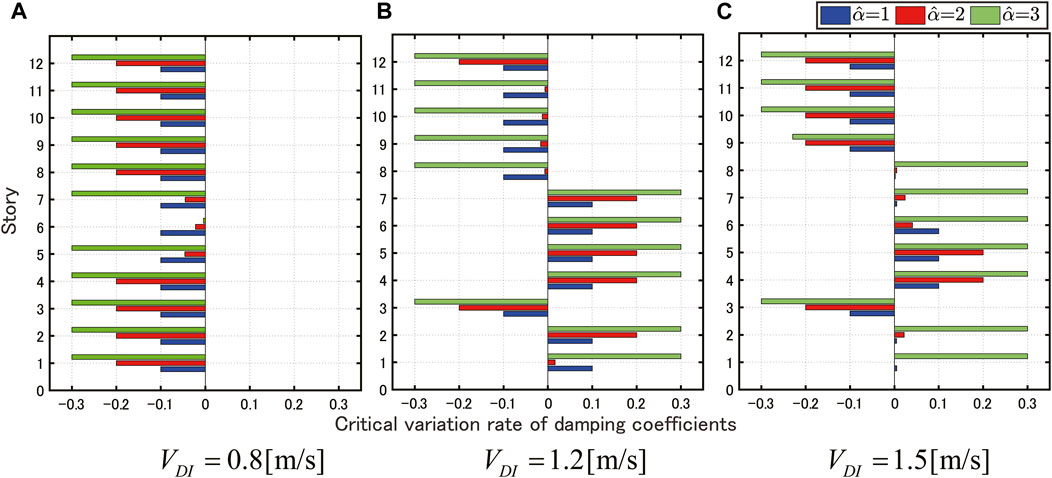
FIGURE 7. Worst combination of damping coefficients for each robustness function value: (A)
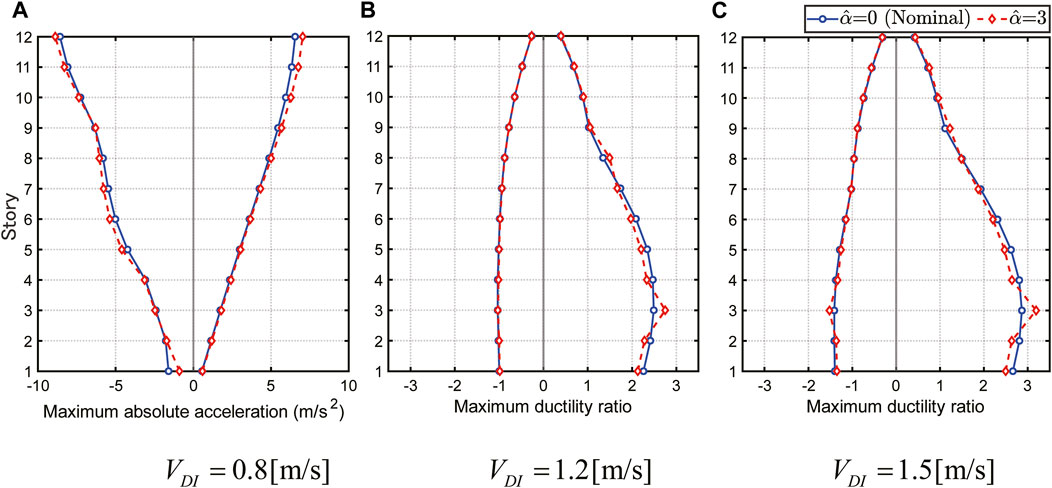
FIGURE 8. Positive and negative maximum performance functions for various input levels: (A)
In the case of
On the other hand, in the case of
In the case of
As shown in Figure 7, in the case of the structural design considering the variation in the damping coefficient of the damper, even if a uniform lower limit is adopted, it does not necessarily result in the worst-case response. This investigation and similar results were also discussed by Fujita et al. (2021).
5 Robust optimal design considering uncertainty in damping characteristics of oil dampers
5.1 Optimal design index using robustness functions with performance criteria and input velocity amplitude as variables
In this section, the robust optimal design problem is proposed to determine the oil damper placement that improves the structural performance with robustness using the proposed robustness surface based on the
As mentioned in the evaluation example in Section 4, in the
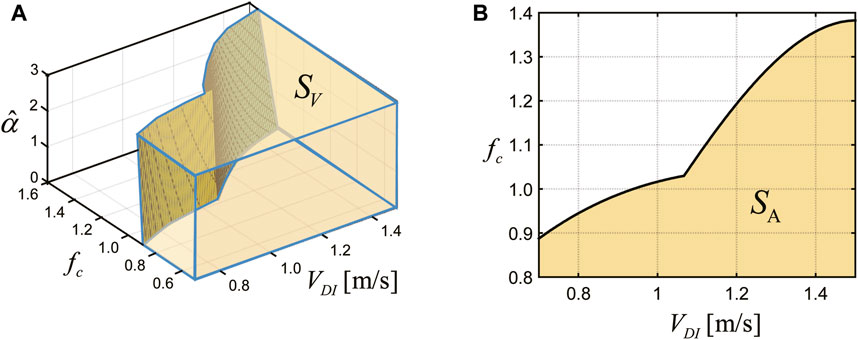
FIGURE 9. Objective function used in optimization problems based on the three-dimensional robustness function: (A) robust optimization problem and (B) normal optimization problem.
5.2 Robust optimal design problem
The robust optimal design problem based on the robustness index presented in Section 5.1 can be expressed as follows:
[Robust optimal design problem]
where
where
5.3 Optimal design problem without considering uncertainties
The robust optimal design problem presented in Section 5.2 aims to suppress the performance deterioration considering variations in the design variables by taking robustness into account. On the other hand, robust optimization may result in a smaller performance value when variation is not considered (at
[Normal optimal design problem]
In this paper, the design problem in Eq. 8 is referred to as a “normal optimal design problem.” In order to optimize structural performance for various input levels, an objective function is set up with the intention of obtaining response values to the nominal values for each input level. This objective function is based on ideas similar to those treated in the robust optimization problem, as shown in Eq. 6. The objective function
In this problem, the optimal design is to improve the performance at various input levels without considering the variation in the damping coefficient of the oil damper.
5.4 Results and discussion of the robust optimal design
In this section, the results of the robust optimal design problem for finding the optimal damper placement added to the elastoplastic 12-story shear degree of freedoms model treated in Section 4 are shown, and the difference from the normal optimal design is discussed. In the figure legend and following discussions, the mass system model treated in Section 4 is referred to as the “standard model,” the model with dampers where Eq. 8 is applied as the “normal optimization model,” and the model with dampers where Eq. 6 is applied as the “robust optimization model.” The total amount of damping coefficients of the damper
As a result of the optimal design problem, the damping coefficient distributions of the oil damper for each model are shown in Figure 10. In addition, for each model, the values of
Comparing the values of
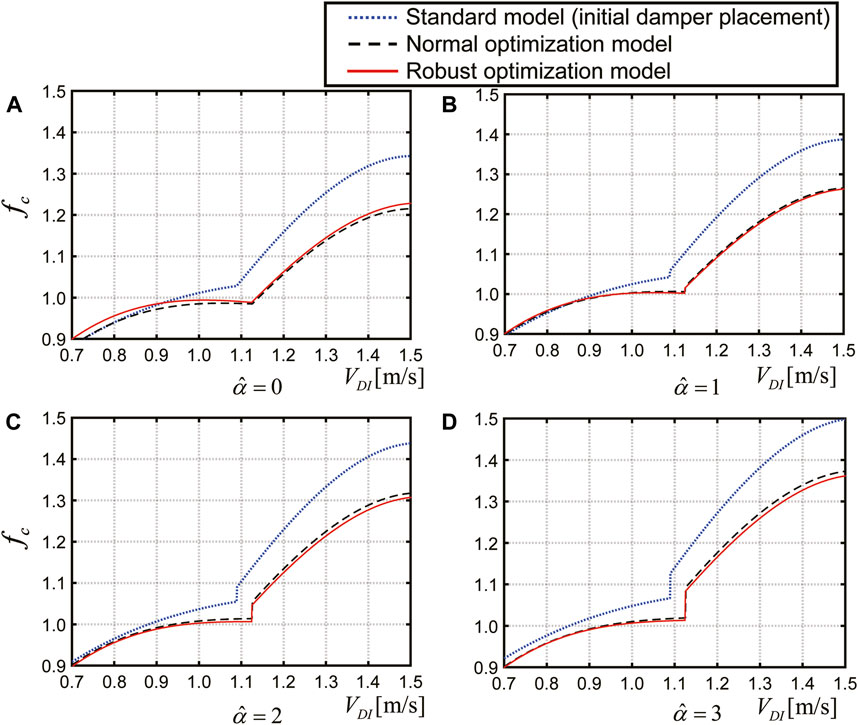
FIGURE 11. Three-dimensional robustness function for various robustness degrees: (A)
From Figure 10, in the robust optimization model, it can be observed that more dampers are placed in the lower and upper stories, and fewer dampers are placed in the middle stories, especially in seventh and eighth stories. This is because, under a constant total damping coefficient of the damper, the response increase can be suppressed even if the damping coefficient varies, by placing more dampers in and around the stories, where the response displacement or acceleration increases due to variations in the damping coefficient. The normal optimization model has a similar placement to the robust optimization model, but the amount of dampers is approximately 22% and 49% larger for the seventh and eighth stories, respectively. It may be pointed out that there is not much difference between the damper placement of the normal and robust optimization models, but it is important to note that the normal optimal design, which is used as a comparison in this paper, takes into account the variability in input levels. It can be said that the normal optimization model has a certain degree of high robustness. Although the damping coefficient difference between both models is small, Table 2 shows that it is possible to improve the
In order to compare the characteristics of each model, the distributions of the maximum response at a given input level
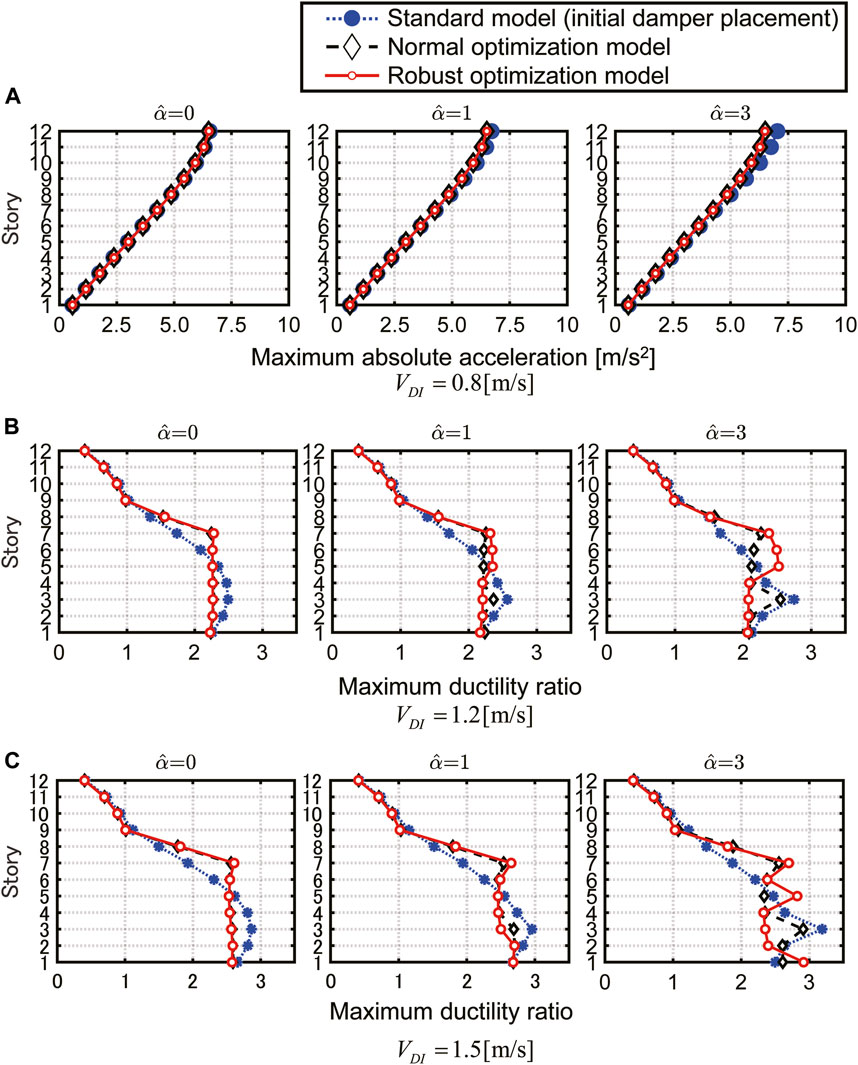
FIGURE 12. Comparison of performance functions for various input levels and robustness degree
Figure 12A shows the case of
Figure 12B shows the case of
Figure 12C shows the case of
As a common feature observed in both the cases of
The performance difference between each model is not so significant when
Since it is known that the initial solution dependence in gradient-based optimization like the interior point method in fmincon, several optimization results based on different initial solutions in fmincon are compared in the proposed optimization problems. The optimization solver fmincon function uses the sensitivity of the objective function to successively update the solution until the objective function converges within a specified error range. Therefore, if an inappropriate initial solution is given, the obtained solution may be a local solution or the number of computational iterations required until the objective function converges may be very large. Furthermore, in the example problem, the computational load required to evaluate the objective function
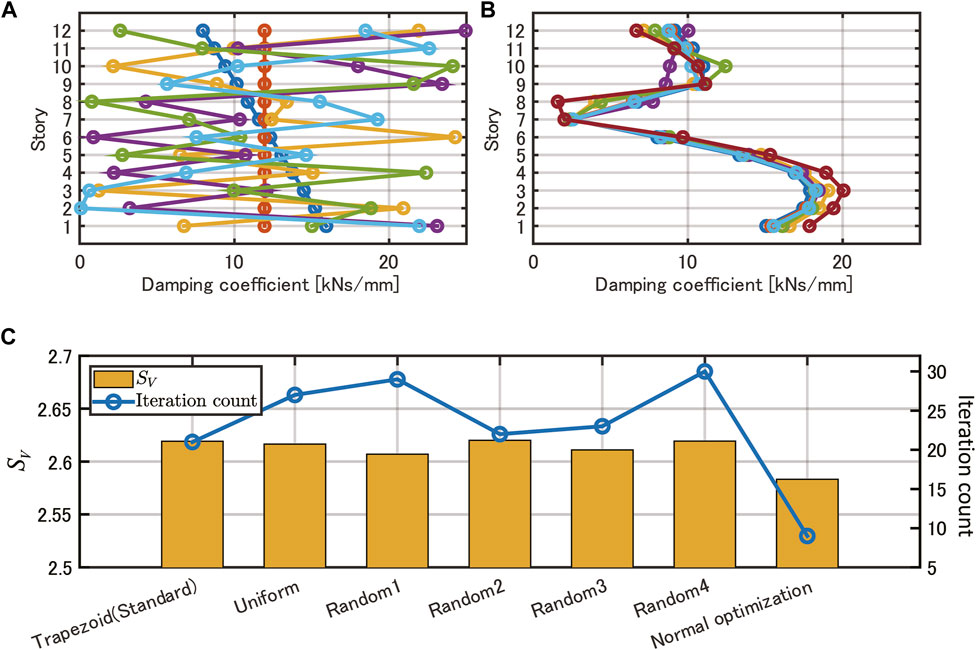
FIGURE 13. Investigation of initial solution dependence for robust optimization: (A) initial solution for random combinations, (B) optimal damping coefficient distribution, and (C) objective function in robust optimization and number of iterations.
6 Conclusion
A robustness evaluation method considering a wide range of input levels and performance criteria was presented for a structural design with various uncertainties. To address seismic input uncertainty in structural design, this paper focused on a worst-case scenario by utilizing a critical one-cycle sine wave, which establishes an upper bound on the structural response. In addition to the design constraint of the maximum response, the proposed robustness index evaluates the change in the robustness function with respect to variations in input amplitude as a multidimensional function. Furthermore, using the proposed robustness index, a robust optimal design problem and its solution method are presented to find a design that can retain high performance under uncertain parameters and large fluctuations in input levels. By solving numerical examples, the solution to the proposed robust optimization problem was presented using an elastoplastic shear mass system model with oil dampers. The detailed results obtained by this paper are as follows.
1) A simplified double impulse method is employed, where the main part of the near-fault seismic motions is expressed in terms of amplitude and impulse interval parameters. Based on the pre-analysis of the critical double impulse for the worst seismic input to the building structure, it is possible to take into account the uncertainty of the input. The effectiveness of the one-cycle sine wave, equivalent to the critical double impulse, in evaluating the structural response of inter-story ductility and floor accelerations was confirmed.
2) The uncertainty analysis method, obtaining the upper bound of the objective function under the specified uncertainty degrees, was applied to the robustness evaluation by varying the uncertainty range in a variable manner. The uncertainty analysis method was applicable for deriving the proposed robustness function considering both input velocity amplitude and performance criteria. The three-dimensional robustness surface was continuously derived by applying the interpolation of the cubic surface function.
3) The robust optimal damper placement problem, aiming to determine the damping coefficients of the oil dampers for each story, for the purpose of improving the volume value for the robustness surface was proposed. In order to consider the wide range of the input level and structural performance criteria, the volume integration value of the robustness surface was employed as the objective function of the optimal design problem.
4) The numerical examples were presented using an elastoplastic 12-degrees-of-freedom model for several optimization problems. The robust optimal design was compared with the normal optimal design where variations in oil dampers are not considered. It was shown that robust optimization can successively enhance the robustness by rearranging the damping coefficient under a constant constraint of the sum of damping coefficients. It was confirmed that in robust optimization, the damping coefficients are increased at specific floors with larger maximum ductility ratios when the degree of damper variation is increased. The investigation into the initial solution dependence revealed that a more robust solution can be achieved with a smaller computational load by using the normal optimal design as the initial solution.
Data availability statement
The original contributions presented in the study are included in the article/Supplementary Material; further inquiries can be directed to the corresponding author.
Author contributions
MH: conceptualization, data curation, formal analysis, investigation, and writing–original draft. KF: resources, supervision, validation, visualization, and writing–review and editing.
Funding
The author(s) declare financial support was received for the research, authorship, and/or publication of this article. This research was supported by JSPS, KAKENHI Grant No. 21K04334.
Conflict of interest
The authors declare that the research was conducted in the absence of any commercial or financial relationships that could be construed as a potential conflict of interest.
Publisher’s note
All claims expressed in this article are solely those of the authors and do not necessarily represent those of their affiliated organizations, or those of the publisher, the editors, and the reviewers. Any product that may be evaluated in this article, or claim that may be made by its manufacturer, is not guaranteed or endorsed by the publisher.
References
Akehashi, H., and Takewaki, I. (2019). Optimal viscous damper placement for elastic-plastic MDOF structures under critical double impulse. Front. Built Environ. 5, 20. doi:10.3389/fbuil.2019.00020
Ben-Haim, Y. (2006). Info-gap decision theory: decisions under severe uncertainty. Amsterdam, Netherlands: Elsevier.
Ben-Haim, Y., and Elishakoff, I. (1990). Convex models of uncertainty in applied mechanics. New York, NY, USA: Elsevier.
Castaldo, P., and De Iuliis, M. (2014). Optimal integrated seismic design of structural and viscoelastic bracing-damper systems. Earthq. Eng. Struct. Dyn. 43 (12), 1809–1827. doi:10.1002/eqe.2425
Chen, S. H., Ma, L., Meng, G. W., and Guo, R. (2009). An efficient method for evaluating the natural frequencies of structures with uncertain-but-bounded parameters. Comput. Struct. 87 (9-10), 582–590. doi:10.1016/j.compstruc.2009.02.009
Doltsinis, I., and Kang, Z. (2004). Robust design of structures using optimization methods. Comput. Methods Appl. Mech. Eng. 193 (23-26), 2221–2237. doi:10.1016/j.cma.2003.12.055
Elishakoff, I., and Ohsaki, M. (2010). Optimization and anti-optimization of structures under uncertainty. London: Imperial College Press.
Faes, M., and Moens, D. (2020). Recent trends in the modeling and quantification of non-probabilistic uncertainty. Archives Comput. Methods Eng. 27, 633–671. doi:10.1007/s11831-019-09327-x
Fragiadakis, M., and Papadrakakis, M. (2008). Performance-based optimum seismic design of reinforced concrete structures. Earthq. Eng. Struct. Dyn. 37 (6), 825–844. doi:10.1002/eqe.786
Fujita, K., and Takewaki, I. (2011). An efficient methodology for robustness evaluation by advanced interval analysis using updated second-order Taylor series expansion. Eng. Struct. 33 (12), 3299–3310. doi:10.1016/j.engstruct.2011.08.029
Fujita, K., Wataya, R., and Takewaki, I. (2021). Robust optimal damper placement of nonlinear oil dampers with uncertainty using critical double impulse. Front. Built Environ. 7, 744973. doi:10.3389/fbuil.2021.744973
Fujita, K., and Yasuda, K. (2016). Robust optimization for damper placement under structural uncertainties using robustness function. J. Struct. Eng. AIJ 62B, 387–394. (in Japanese). doi:10.3130/aijjse.69B.0_181
Garivani, S., Askariani, S. S., and Aghakouchak, A. A. (2020). “Seismic design of structures with yielding dampers based on drift demands,” in Structures (Amsterdam, Netherlands: Elsevier). doi:10.1016/j.istruc.2020.10.019
Gholizadeh, S. (2015). Performance-based optimum seismic design of steel structures by a modified firefly algorithm and a new neural network. Adv. Eng. Softw. 81, 50–65. doi:10.1016/j.advengsoft.2014.11.003
Gokkaya, B. U., Baker, J. W., and Deierlein, G. G. (2016). Quantifying the impacts of modeling uncertainties on the seismic drift demands and collapse risk of buildings with implications on seismic design checks. Earthq. Eng. Struct. Dyn. 45 (10), 1661–1683. doi:10.1002/eqe.2740
Henriques, A. A., Veiga, J. M. C., Matos, J. A. C., and Delgado, J. M. (2008). Uncertainty analysis of structural systems by perturbation techniques. Struct. Multidiscip. Optim. 35 (3), 201–212. doi:10.1007/s00158-007-0218-z
Hisada, Y., and Tanaka, S. (2021). What is fling step? Its theory, simulation method, and applications to strong ground motion near surface fault ruptures. Bull. Seismol. Soc. Am. 111 (5), 2486–2506. doi:10.1785/0120210046
Hosoda, M., and Fujita, K. (2023). Robust Optimal Design based on Story ductility ratio of elastic-plastic building structures with uncertain oil dampers subjected to critical double impulse. J. Struct. Eng. B 69B, 181–189. (in Japanese). doi:10.3130/aijjse.69B.0_181
Kaveh, A., Azar, B. F., Hadidi, A., Sorochi, F. R., and Talatahari, S. (2010). Performance-based seismic design of steel frames using ant colony optimization. J. Constr. Steel Res. 66 (4), 566–574. doi:10.1016/j.jcsr.2009.11.006
Kojima, K., and Takewaki, I. (2015). Critical earthquake response of elastic–plastic structures under near-fault ground motions (Part 1: fling-step input). Front. Built Environ. 1, 12. doi:10.3389/fbuil.2015.00012
Kojima, K., and Takewaki, I. (2016). Closed-form critical earthquake response of elastic-plastic structures with bilinear hysteresis under near-fault ground motions. J. Struct. Constr. Eng. 726, 1209–1219. (in Japanese). doi:10.3130/aijs.81.1209
Lagaros, N. D., and Papadrakakis, M. (2007). Robust seismic design optimization of steel structures. Struct. Multidiscip. Optim. 33, 457–469. doi:10.1007/s00158-006-0047-5
Moens, D., and Hanss, M. (2011). Non-probabilistic finite element analysis for parametric uncertainty treatment in applied mechanics: recent advances. Finite Elem. Analysis Des. 47 (1), 4–16. doi:10.1016/j.finel.2010.07.010
Moens, D., and Vandepitte, D. (2004). An interval finite element approach for the calculation of envelope frequency response functions. Int. J. Numer. Methods Eng. 61 (14), 2480–2507. doi:10.1002/nme.1159
Nabid, N., Hajirasouliha, I., Margarit, D. E., and Petkovski, M. (2020). Optimum energy based seismic design of friction dampers in RC structures. Structures 27, 2550–2562. doi:10.1016/j.istruc.2020.08.052
Papavasileiou, G. S., and Charmpis, D. C. (2016). Seismic design optimization of multi–storey steel–concrete composite buildings. Comput. Struct. 170, 49–61. doi:10.1016/j.compstruc.2016.03.010
Takewaki, I., and Ben-Haim, Y. (2005). Info-gap robust design with load and model uncertainties. J. Sound Vib. 288 (3), 551–570. doi:10.1016/j.jsv.2005.07.005
Wang, C., Qiang, X., Fan, H., Wu, T., and Chen, Y. (2022). Novel data-driven method for non-probabilistic uncertainty analysis of engineering structures based on ellipsoid model. Comput. Methods Appl. Mech. Eng. 394, 114889. doi:10.1016/j.cma.2022.114889
Xiao, Y., Zhou, Y., and Huang, Z. (2021). Efficient direct displacement-based seismic design approach for structures with viscoelastic dampers. Structures 29, 1699–1708. doi:10.1016/j.istruc.2020.12.067
Keywords: robust optimal design, damper placement, uncertainty analysis, critical double impulse, oil damper
Citation: Hosoda M and Fujita K (2024) Robust optimal damper placement based on robustness index simultaneously considering variation of elastoplastic design criteria and input level. Front. Built Environ. 10:1353827. doi: 10.3389/fbuil.2024.1353827
Received: 11 December 2023; Accepted: 26 January 2024;
Published: 14 February 2024.
Edited by:
Abbas Moustafa, Minia University, EgyptCopyright © 2024 Hosoda and Fujita. This is an open-access article distributed under the terms of the Creative Commons Attribution License (CC BY). The use, distribution or reproduction in other forums is permitted, provided the original author(s) and the copyright owner(s) are credited and that the original publication in this journal is cited, in accordance with accepted academic practice. No use, distribution or reproduction is permitted which does not comply with these terms.
*Correspondence: Kohei Fujita, fm.fujita@archi.kyoto-u.ac.jp