Successional Dynamics in the Gut Microbiome Determine the Success of Clostridium difficile Infection in Adult Pig Models
- 1Wageningen Bioveterinary Research, Lelystad, Netherlands
- 2iDiv – German Centre for Integrative Biodiversity Research, Leipzig, Germany
- 3TNO Innovation for Life, Zeist, Netherlands
- 4Wageningen Livestock Research, Wageningen, Netherlands
Clostridium difficile infections (CDI) are a major cause of antibiotic-associated diarrhea. It is hypothesized that CDI develops due to the antibiotic-induced disruption of the intestinal microbial community structure, which allows C. difficile to flourish. Here, we pre-treated weaned pigs with the antibiotics Clindamycin or Ciprofloxacin for 1 day, and subsequently inoculated them with a human and pig enteropathogenic C. difficile strain 078 spores. Body temperature, clinical signs of disease, and the fecal microbiome were monitored daily for 15 days. Clindamycin had a stronger effect on the pigs than Ciprofloxacin, resulting in drastic shifts in the fecal microbiome, decreases in microbial diversity and significant increases in body temperature, even in the absence of C. difficile. Fecal shedding of C. difficile was detectable for 3 and 9 days in Ciprofloxacin and Clindamycin treated pigs inoculated with C. difficile, respectively, and in both cases decreased cell proliferation rates were detected in colon tissue. The timing of C. difficile shedding coincided with the decrease in a large cluster of Firmicutes following Clindamycin treatment, a pattern which was also independent of C. difficile inoculation. The observed community patterns suggest that successional dynamics following antibiotic treatment facilitate C. difficile establishment. The similarities between the microbiome responses observed in our study and those previously reported in CDI-infected humans further support the utility of adult pigs as models for the study of CDI.
Introduction
Clostridium difficile infections (CDI) are a major cause of hospital-associated and antibiotic-associated diarrhea in humans (Monaghan, 2015). The clinical manifestations of CDI vary and range from mild diarrheal symptoms to severe colitis. Complications associated with CDI include bowel perforation, renal failure, sepsis, and systemic inflammatory response syndrome. CDI patients can be treated with specific antibiotics, although 20% encounter a recurrent episode (Laffan et al., 2006; Marsh et al., 2012). It is hypothesized that the residing gut microbiota is important in the maintenance of C. difficile colonization resistance in healthy individuals, and that CDI is associated with dysbiosis of the intestinal microbiota caused by antibiotic use (Reeves et al., 2011). Antibiotics disrupt the intestinal microbial community structure, decreasing its colonization resistance and promoting the germination and colonization of C. difficile spores in the colon (Paterson, 2004). The shift in microbial community structure that induces sensitivity to C. difficile infections has not been studied at a high temporal resolution due to lack of longitudinal studies which sample the intestinal microbiome prior to antibiotic administration and C. difficile infection.
The understanding of how colonization mechanisms by C. difficile precludes the development of therapeutic and preventive methods to treat infections (Kociolek and Gerding, 2016). Fecal microbiota transplantation is an established treatment for recurrent CDI, as it restores microbial homeostasis and diversity (Choi and Cho, 2016). However, there are numerous long-term safety and regulatory issues associated with this practice. Novel preventive prophylactic therapies, which increase the native microbiota's resistance to CDI are in diverse states of development (Best et al., 2012). Nutrition-based treatments may contribute toward increasing the native intestinal microbiota's resistance to colonization by C. difficile, but to date are underexplored, largely because the diets and digestive systems of current murine models differ from the human digestive system (Best et al., 2012; Schubert et al., 2015).
In order to rigorously examine the potential success of various treatments, it is crucial to have an animal model with a comparable digestive system and diet to humans. An alternative to murine models are pig (Sus scrofa domesticus) models. Recent literature indicates that pigs are a valuable translational model in developing therapies for human health, especially nutritional studies, intestinal microbiota associated diseases, and immune parameters (Litten-Brown et al., 2010; Meurens et al., 2012; Heinritz et al., 2013). The post-weaning gut development and nutritional requirements of pigs closely resemble those of humans (Andersen et al., 2011; Heinritz et al., 2013). After weaning, pigs exhibit similar physiology, morphology, and functionality of the gastro-intestinal tract (GIT) to humans, and microbial and immune homeostasis have already been established. Additionally, CDI has been documented to cause enteric disease in pigs (Goorhuis et al., 2008; Debast et al., 2009). In one study, the effects of CDI in germfree piglets and 1–10 day-old non-weaned conventional piglets included short periods of diarrhea in some piglets, while others were non-diarrheic but showed colitis at necropsy (Arruda et al., 2013).
Since the relationship between gastrointestinal microbial dysbiosis and CDI development has frequently been observed, it is hypothesized that specific microbial community structures may be indicative of an increased risk of CDI (Britton and Young, 2014). Studying the relationship between an individual's native gastrointestinal microbiome CDI requires the collection of longitudinal fecal samples before and immediately after the onset of CDI, which is not possible in humans. The objectives of this study were (1) to explore the dynamics of the gastrointestinal microbiome before and during CDI following antibiotic administration, using 8–10 week old pigs, and (2) to establish adult pigs as models for the study of CDI. To induce microbial dysbiosis and simulate the development of CDI in humans, we administered the pigs with Clindamycin or Ciprofloxacin, both of which are associated with increased risk for CDI in humans. Clindamycin has become the inducing agent of choice in small animal models (Best et al., 2012), while most C. difficile strains have been found to be resistant to Ciprofloxacin (Norman et al., 2014). Both antibiotics are commonly used to treat bacterial infections in humans. Following antibiotic administration, we challenged the pigs with C. difficile, and monitored various health parameters, as well as their fecal microbiome for the following 15 days. By monitoring the microbiome before and after antibiotic administration and C. difficile inoculation, we aim to provide further insight into the relationship between the native gut microbiome, the effect of antibiotic treatment, and susceptibility to CDI, and to establish the pig gut as a suitable model for the study of CDI in humans.
Materials and Methods
Experimental Design
All procedures were approved by the animal experimentation board at Wageningen University & Research Center (accession number AVD401002015141) and carried out according to the guidelines of the European Council Directive 86/609/EEC dated November, 1986. Briefly, 25 seven week-old pigs were obtained from a commercial breeder 2 weeks after weaning. The pigs were divided into five groups of five animals each, and housed in five separate pens. Prior to the beginning of the experiment, the pigs were allowed to adapt for 1 week (Figure S1). All pigs were fed the same controlled feed. At day −1 of the experiment, two groups (Clin C. diff and Clin) were orally administered with 50 mg/kg Clindamycin (TEVA Nederland BV, Haarlem, the Netherlands); two groups (Cip C.diff and Cip) with 15 mg/kg Ciprofloxacin (Pharmachemie B.V., Haarlem, the Netherlands); and one group served as a control group. At day 0 and 16 h later, the pigs in groups Clin C. diff and Cip C.diff were intra-gastrically inoculated with 2 × 109 CFUs of C. difficile ribotype 078. Groups Clin and Cip were intra-gastrically inoculated with sterile water. Feces were collected on days −5, 0, and daily between days 1 and 15 by taking rectal fecal samples. Blood was collected and body weights were measured on days −5, 0, 5, 10, and 15. Body temperature was measured once a day, starting on the day of arrival and twice daily after day 1 (Figure S1). During the experimental phase pigs were also monitored for clinical signs of disease, including diarrhea, dehydration, dyspnoea, weakness, lethargy, and anorexia. At the end of the experiment, animals were anesthetized with intravenous 20% 1 ml euthasol (AST Farma B.V. Oudewater, The Netherlands) and euthanized to collect tissue samples.
Preparation of the C. difficile Inoculum
A toxigenic C. difficile strain of PCR Ribotype 078 (human isolate, kindly given by Prof. E. Kuijper, Centre for Infectious Diseases, Leiden University Medical Centre, Leiden, The Netherlands) was selected for the experimental C. difficile challenge because this strain has been found in both humans and in pigs (Goorhuis et al., 2008). Spores of C. difficile Ribotype 078 were prepared as follows. C. difficile was anaerobically grown in Schaedler Anaerobe broth (SAB, Oxoid CM0497) for 5 to 7 days at 37°C. Spores were harvested by centrifugation and washed with cold water at least three times. C. difficile spores were selected by heat treatment for 20 min at 65°C to ensure that any remaining vegetative cells were killed. Viable spores were calculated to determine the C. difficile inoculation dose by plating for colony forming units (CFU/ml). C. difficile strain 078 was shown to be vulnerable to Clindamycin (TEVA Nederland BV, Haarlem, The Netherlands) and Ciprofloxacin (Pharmachemie B.V., Haarlem, The Netherlands), allowing the use of both antibiotics in the subsequent experiments.
Histopathology and Immunohistochemistry
Histopathology was performed at the end of the experiment to detect CDI-related changes in the intestines of all pigs. Formalin-fixed colon and jejunum tissue sections (5 μm thick) were stained with haematoxylin and eosin (HE staining) and were examined morphologically by light microscopy for changes in the crypt depth and villus height as previously described (Zekarias et al., 2005).
Immunohistochemistry was performed to identify CDI-related changes in the colon and jejunum of pigs from all other treatments. Frozen colon and jejunum tissue sections (10 μm thick) were immunohistochemically stained for the detection of CD3+ T-cells (abcam ab16669) and Macophages (CVI-SwNL 517.2 ID nr: 107) by an indirect immunoperoxidase method as described before (Bianchi et al., 1992). The images were acquired and analyzed with Image-Pro Plus (version 5.1, DVC Machinevision Breda, The Netherlands). Proliferating cell nuclear antigen (PCNA) was used as an indicator for cell health; a decreased PCNA may indicate reduced cell renewal rates and intestinal lesions. PCNA was detected by immunohistochemistry in formalin-fixed duodenum and jejunum tissue sections (rat PCNA; PC-10, Dako, Glostrup, Denmark) in PBS containing 0.1% BSA. The cellular markers were visualized using Dako Real TM EnvisionTM Detection System-HRP (K5007ENV) according to the manufacturer's instructions. Examination of the slides under light microscopy and scoring were as described before (Zekarias et al., 2005). All animals were examined, and the mean scoring was calculated for the group at a given time period.
DNA Isolation and Quantification of Bacterial Community and C. difficile in Fecal Samples
DNA was isolated from snap-frozen fecal samples according to Ladirat et al. (2013) and used for quantitative PCR (qPCR) analysis as well as sequencing. The amount of C. difficile DNA was determined by quantitative qPCR. qPCR was performed on an ABI 7500 Fast System (Taqman-MGB) using in-house designed primer-probe combinations targeting C. difficile-specific portions of the 16S rRNA gene (Cdif Forward 5′-GCAACGCGAAGAACCTTACCTA-3′; Cdif Reverse 5′- GAAGGGAACTCTCCGATTAAGGA-3′; Cdif probe 5′- TGACATCCCAATGACA-3′; VIC-MGB). A standard curve spanning seven orders of magnitude (100-106 fg) was generated using total chromosomal DNA of the target strain. The amount of detected target sequence was calculated as femtograms according to this standard curve. Total bacterial DNA was determined by targeting the 16S rRNA gene, using the generic PCR primer-probe combination (16S uni-I-F 5′- CGA AAG CGT GGG GAG CAA A-3′; 16S uni-I-R 5′- GTT CGT ACT CCC CAG GCG G-3′; 16Suni-I 5′- ATT AGA TAC CCT GGT AGT CCA-3′; FAM-MGB). qPCR conditions for both reactions are detailed in Section S2. C. difficile counts were divided by total 16S rRNA counts per sample to account for differences in fecal swab content between samples. The detection limit was set as (mean 16S rRNA counts)−1.
Bacterial Community Sequencing and Processing
The V4 region of the 16S rRNA gene was amplified by PCR as described by Caporaso et al. (2012). Amplicons were confirmed by analysis on a fragment analyzer (Advanced Analytical, Germany), after which the pool was purified by the Qiaquick gel extraction kit from (QIAGEN) and sequenced by targeted-amplicon 16S sequencing on an Illumina MiSeq sequencer as previously described (Caporaso et al., 2012). All processing and analyses were performed in R 3.4.0 (R Core Team, 2014). The 16S rRNA gene sequencing reads were filtered, trimmed, dereplicated, chimera-checked, and merged using the dada2 package (Callahan et al., 2016) and reads were assigned with the RDP classifier (Wang et al., 2007). Downstream analyses were performed with the phyloseq (McMurdie and Holmes, 2013) package. Reads were rarefied to 4 686 reads per sample using the rarefy_even_depth function (seed = 1). The final dataset contained 10 604 745 reads distributed among 2 969 sequence variants (SVs). Good's coverage was >0.999.
Statistical Analyses
Statistical analyses were performed in R 3.4.0 (R Core Team, 2014) using the vegan (Oksanen et al., 2013) and phyloseq (McMurdie and Holmes, 2013) packages. Richness, or α-diversity was calculated using the Shannon-weiner index and total observed SV richness. Differences between time and treatment for 16S rRNA qPCR counts and α-diversity were evaluated using two-way ANOVAs or one-way ANOVAs followed by post-hoc Tukey tests for pairwise comparisons. Differences between genera and phyla on day −5 and day 0 within treatments were evaluated with Welch's t-tests. Taxon abundances are displayed as mean± standard deviation.
Pairwise distances between samples were calculated using the Bray-Curtis distance metric, and visualized using a principal coordinates analysis (PCoA). Differences between treatments and time were evaluated using adonis on Bray-Curtis distances. Homogeneity of dispersions between replicates were evaluated using betadisper on Bray-Curtis distances, with Tukey tests for the evaluation of pairwise distances. A principal response curve (Van den Brink and Ter Braak, 1999) was used to compare community responses over time. We selected SVs with a species score > |1|, which indicates a strong influence on the observed community dynamics, and agglomerated them at the genus level. For the heatmap, taxa abundances were standardized per SV over time using the decostand function, their Euclidean distances were calculated and taxa were clustered using Ward's method. Pearson correlations between individual SVs and C. difficile-specific qPCR data were performed corr.test from the R package psych (Revelle and Revelle, 2015). The identity of a C. difficile-like SV was confirmed using nucleotide BLAST (Johnson et al., 2008).
Results
Phenotypic Observations and Pathology Associated With C. difficile Germination and Multiplication
Pigs from all treatments except for the Cip group exhibited significantly higher temperatures on average compared to the control group (P < 0.01 for all Tukey HSD comparisons of a one-way ANOVA on the effect of treatment, Figure 1). In addition, pigs in the Cip C. dif group exhibited significantly higher temperatures than pigs in the Cip treatment on average (p < 0.0001), while pigs in the Clin treatment did not significantly differ from those in the Clin C. diff treatment (p = 0.47). The mean temperature for pigs from both the Cip C. dif and Clin C. dif treatments was highest on day 1 (39.71and 39.69°C, respectively), but neither was significantly different from temperatures exhibited before the C. difficile challenge (day 0).
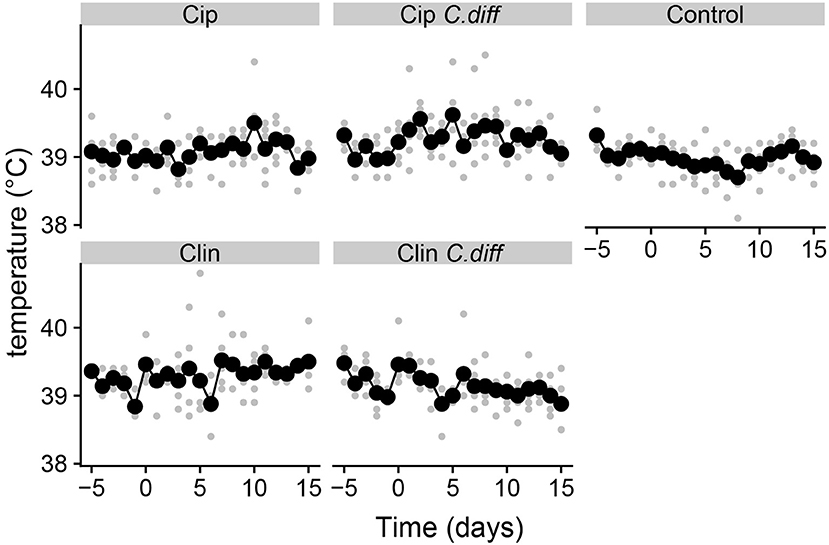
Figure 1. Elevated temperatures in Clindmycin and C. difficile-treated pigs. Rectal temperatures for pigs were significantly higher in the Clin, Clin C. diff, and Cip C. diff treatments relative to controls, on average (p < 0.01). A single diurnal sample is shown per pig, per day in gray. Daily means per treatment are displayed in black and connected with lines. Temperature was measured at the same time each day.
Visual inspection of the intestine pack at necropsy showed no signs of pathological changes in any of the treatment groups. Weight gains did not differ significantly between any treatment group (data not shown).
At the end of the experiment, PCNA staining of colon tissue demonstrated significant differences in cell proliferation rates between Clin C. diff and Cip C. diff groups and the control group (Mann-Whitney U-test, p = 0.0159 and 0.0556, respectively, Figure 2), but not between Clin and Cip groups and the Control group, indicating that this was a C. difficile-specific outcome (Figure 2). No differences between groups could be observed for the number of macrophages and CD3+ cells in colon sections or C. difficile-associated cell proliferation crypte/villus ratios and the number of macrophages and CD3+ in jejunal tissue (data not shown).
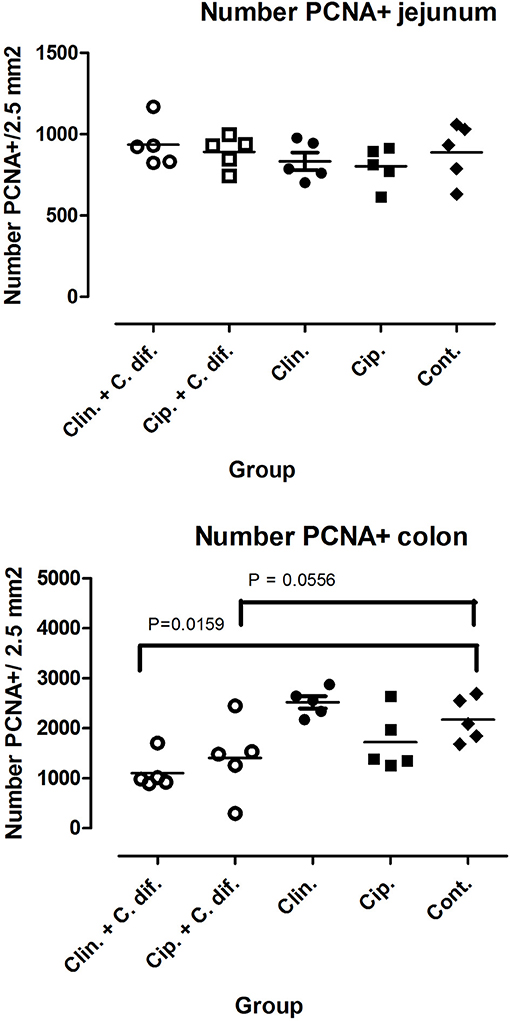
Figure 2. Decreased cell proliferation rates in the colons of C. difficile-inoculated pigs. Number of PCNA positive cells in jejunal (Top) and colon (Bottom) tissue. Significance of differences between treatments was determined using Mann-Whitney tests.
Effects of Antibiotic Treatments on the Fecal Microbiome
We performed 16S rRNA gene amplicon sequencing and quantification of C. difficile-specific genes on the same fecal samples used for gene quantification in order to identify whether shifts in bacterial community structures induced by Clindamycin and Ciprofloxacin treatments increased the colonization success of C. difficile. In the absence of antibiotics and throughout the experiment, the fecal microbiomes contained an average of 237 ± 99 different SVs. The communities were heavily dominated by the Firmicutes (71.2±7.8% of the community on average) and Bacteroidetes (23.2±8.1% of the community on average). The dominant genera were Prevotella (17.1±7.6%), Blautia (6.6±2.9%), Roseburia (5.7±4.6%), a Ruminococcaceae SV (7.7±3.0%), and a Lachnospiraceae SV (5.0±2.6%).
We measured α-diversity in terms of the Shannon richness index. In the control microbiomes, α-diversity showed no clear patterns, but fluctuated significantly over time (p < 0.001, ANOVA, Figure 3), possibly reflecting the ongoing growth of the pigs or their adaptation to the experimental environment, or intrinsic variability. Similarly, a PCoA showed a weak, but not significant, effect of time on the community structures of Control samples (PERMANOVA, pseudo F = 1.16, R2 = 0.24, p = 0.085, Figure 4).
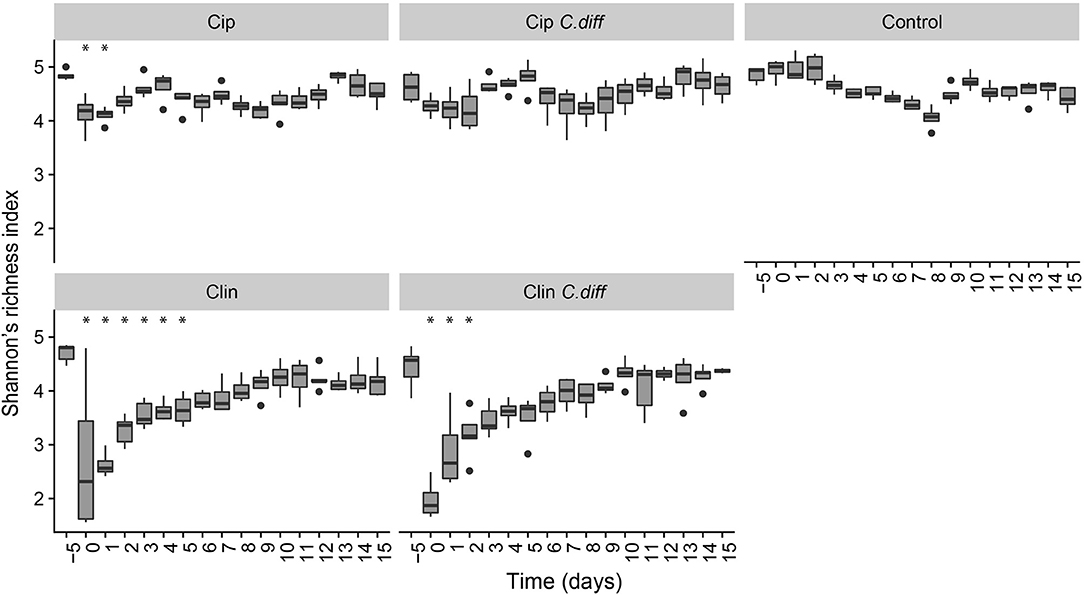
Figure 3. Recovery of bacterial diversity following antibiotic administration. Diversity is displayed as Shannon's H. For each treatment, asterisks represent time points which were significantly different from day −5 microbiomes (pairwise comparisons of a one way ANOVA using Tukeys's HSD, p < 0.01). Error bars represent standard error.
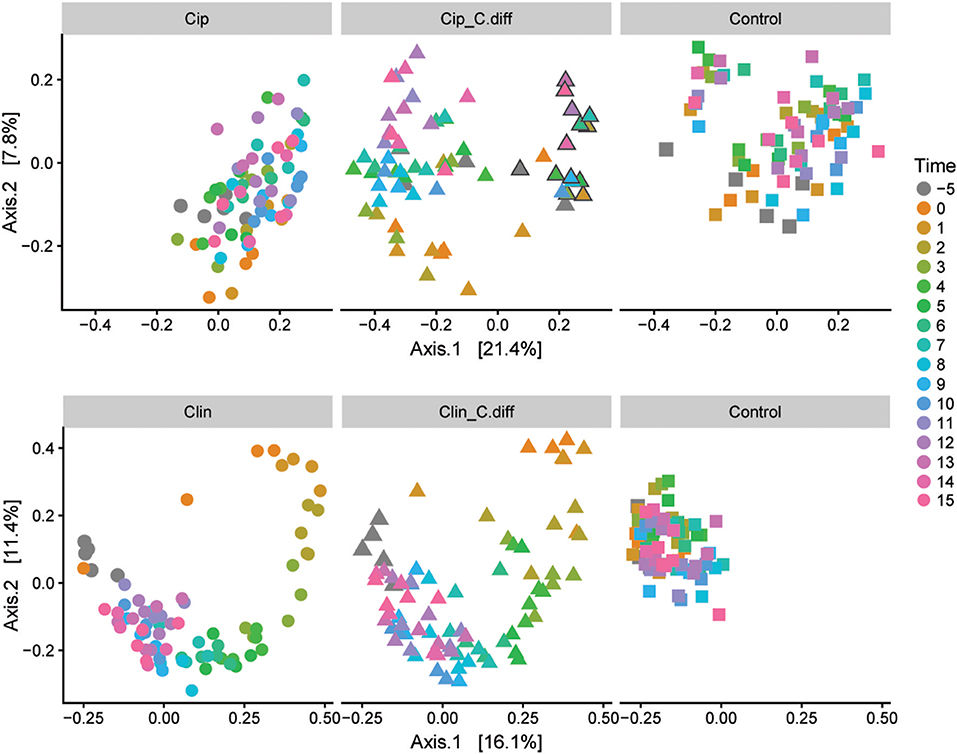
Figure 4. Microbial community responses to treatments. Separate Bray-Curtis PCoA ordinations of microbial community dynamics of Ciprofloxacin (Top) and Clindamycin (Bottom) treated pigs over time. Each ordination is faceted by treatment: antibiotic (left), antibiotic+ C.difficile (middle), and control (right). Gray outlines in the Cip. C.diff treatment surround samples from a single animal which exhibited a differing response.
Ciprofloxacin treatment (Cip) resulted in significantly lower relative abundances of Proteobacteria, Bacteroidetes, Actinobacteria, Actinobacteria taxa, and unclassified Bacteria; and relative increases in Firmicutes taxa (p < 0.05, Welch's t-test). Notably, in response to treatment, members of the Blautia genus increased from 9.05% on day −5 to 25.2% of the community on day 0, on average (p = 0.07, Welch's t-test). Following Cip treatment on day 0, richness fell to 85.15 ± 7.62% of day −5 values and remained significantly lower than day −5 samples until day 1 (p < 0.01, ANOVA, pairwise comparisons; Figure 3). By day 2, richness recovered to day −5 levels. The community structure exhibited strong temporal dynamics following Cip treatment, resulting in an altered community structure (PERMANOVA, pseudo-F = 2.04, R2 = 0.36, p < 0.001, Figure 4). Notably, Cip treated communities were not more variable than control communities (p > 0.05, Tukey's HSD pairwise test on Betadisper, Figure S3).
Clindamycin treatment (Clin) resulted in a high variability between Clin samples on day 0, and only the reduction in unclassified Bacterial taxa was significantly different (p = 0.01, Welch's t-test), but the relative reduction of Firmicutes was observed, as well as the relative increase in Bacteroidetes and Proteobacteria. Within Proteobacteria, members of the Escherichia/Shigella genus increased from 0.1% of the community on day −5 to 35.97% on day 0, on average. α-diversity in clindamycin-treated samples remained significantly below that of untreated samples until day 5 (p < 0.05, ANOVA; Figure 3). The recovering community exhibited clear temporal dynamics, gradually shifting its composition over time (PERMANOVA, pseudo-F = 4.01, R2 = 0.52, p < 0.001, Figure 4). Relative to control communities, samples from the Clin treatment were more variable (p < 0.001, Tukey's HSD pairwise test on homogeneity of dispersions; Figure 4, Figure S3).
Effects of C. difficile Inoculation on the Fecal Microbiome
The proportion of C. difficile in the bacterial community, as measured by qPCR, increased over time in the fecal samples of C. difficile-inoculated pigs, and was undetectable in the Control, Cip, or Clin treatments (Figure 5). The temporal pattern of this surge in C. difficile was dependent on the antibiotic used: fecal shedding of C. difficile in members of the Cip C. diff group was detectable on days 2 and 3, peaking on day 2 (0.026 ± 0.035% of the bacterial community on average). One animal also exhibited high levels of C. difficile on day 1. Fecal shedding of C. difficile in members of the Clin C. diff group was higher and more variable between animals, and was detected in all animals between days 1 and 9, peaking on days 3 and 4 (2.9 ± 6.2% and 2.2 ± 3.5% of the bacteria on average, respectively). A single C. difficile SV from the amplicon sequencing data was highly correlated with the qPCR data (Clostridium XI, r = 0.90, p = 0; species identity confirmed on BLAST).
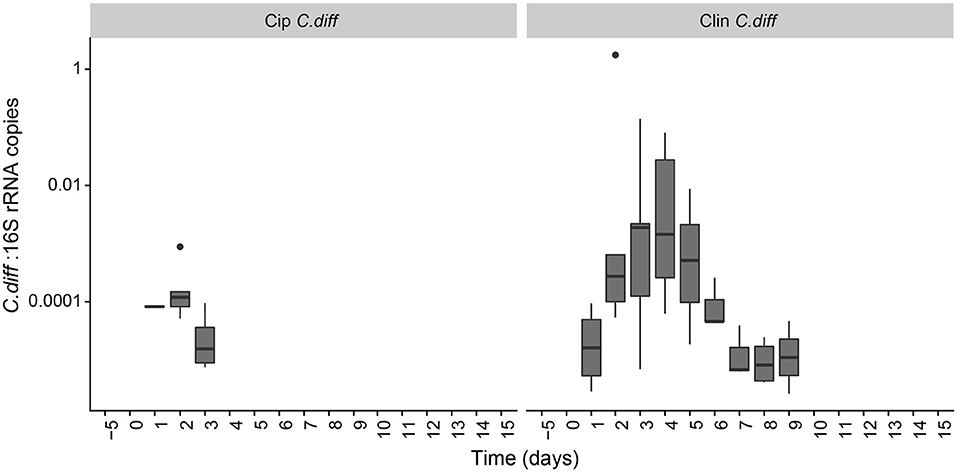
Figure 5. Persistence of C. difficile in the pig microbiome depended on the antibiotic pre-treatment. Ratio of C. difficile-specific 16S rRNA copies to total bacterial 16S rRNA copies in C. difficile treatments. Samples from other treatment groups had no detectable levels of C. difficile-specific 16S rRNA.
C. difficile inoculation resulted in a significant shift in both Clin C. diff and Cip C. diff microbiomes relative to the Clin and Cip groups respectively (PERMANOVA, pseudo-F = 15.04, R2 = 0.12, p < 0.001 and pseudo-F = 18.55, R2 = 0.11, p < 0.001, respectively; Figure 4). However, while time was the most discriminating factor in a PCoA including Clin, Clin C. diff and Control samples, C. difficile inoculation was the main discriminating factor for the PCoA including Cip, Cip C. diff and Control samples (with the exception of one animal), suggesting that the C. difficile inoculation had a larger impact on the fecal microbiomes of Ciprofloxacin-treated pigs relative to the antibiotic application than samples from Clindamycin-treated pigs. Notably, inoculation with C. difficile resulted in significantly higher community dissimilarity between samples from the same Group x Time combination than in control Clin, or Cip treatments (p < 0.001, TukeyHSD comparison of Bray-Curtis distances between groups; Figure S3).
A principal response curve (PRC) was used to compare the effects of each treatment on the fecal microbiomes over time (Figure 6). For the whole experiment, time explained 13.6% of the variance, and group explained 35.1% of the variance. Clindamycin treatment had an overwhelming effect on community composition: relative to controls, samples from the Clin and Clin C.diff groups exhibited much more drastic changes in community composition than the Cip and Cip C.dif.
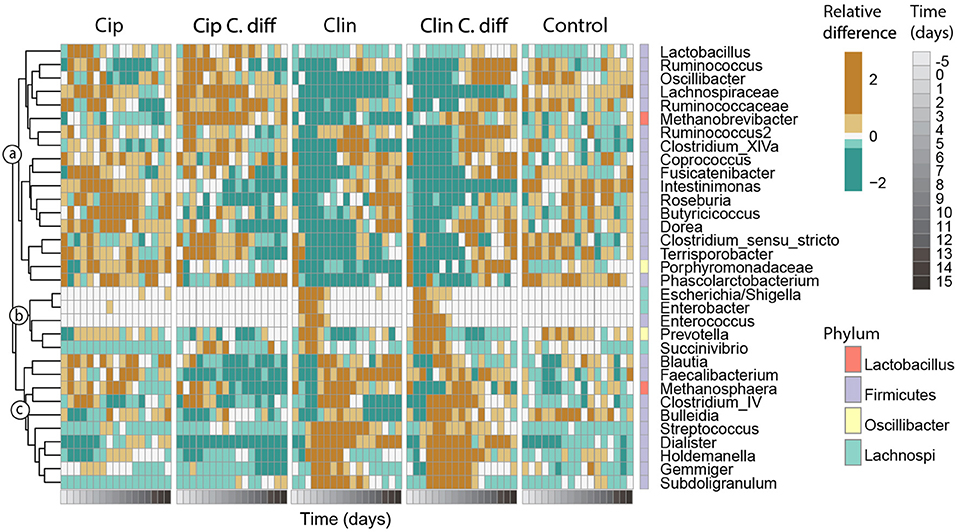
Figure 6. Responses of key taxa to antibiotic and C. difficile treatments. Thirty-three genera which exhibited high species scores in a principal response curve (bottom) were selected. Abundances were averaged per time point for each treatment, and centered and scaled prior to plotting. The phylum membership of each taxon is displayed on the right column, and the lowest classification of each taxon is shown on the right. Taxa were clustered into groups a–c according to their temporal response pattern using Ward's clustering method.
Genera with species scores greater than |1| were expected to have a larger impact on the observed community dynamics, and were selected for further investigation (Figure 6). These 33 genera represented 55.1 ± 12.1% of the community, and belonged to the Firmicutes (26 genera), Proteobacteria (3 genera), Bacteroidetes (2 genera) phyla, and 2 were Archaea. According to their patterns of abundance over time, these taxa clustered into three groups (a–c, Figure 6). Taxa in cluster a were more heavily affected by exposure to Clindamycin than to Ciprofloxacin within the first 5 days of recovery, increasing in relative abundance by day 8 and remaining high for the rest of the experiment. Within cluster a and among Ciprofloxacin-treated samples, those inoculated with C. difficile had a lower relative abundance of Intestinimonas, Roseburia, Lactobacillus, and Butyricicoccus; and a higher relative abundance of Ruminococcus, Oscillibacter, Lachnospiraceae sp., and Methanobrevibacter sp. (cluster a, p < 0.01 for all pairwise Cip:Cip C.diff comparisons of ANOVA). For Clindamycin-treated samples, those inoculated with C. difficile had significantly higher relative abundances of Dorea, Methanobrevibacter, and Ruminococcaceae sp.
Cluster b included taxa which were mostly absent in Control samples and became dominant in Clindamycin-treated samples between days 0 and 5. In C. difficile inoculated samples, this pattern was more pronounced: on day 0, Escherichia/Shigella exhibited a relative abundance of 42.1 ± 26.9 and 35.7 ± 27.2%, and on day 2, Enterococcus achieved a relative abundance of 4.0 ± 2 and 2.3 ± 0.4% in Clin C.diff and Clin samples, respectively (cluster b, Figure 6).
Cluster c consisted of taxa which became transiently abundant in Clindamycin-treated samples between days 1 and 8. Relative to samples from the Clin treatment, samples from the Clin C.diff treatment exhibited higher abundances of Bulleidia (p < 0.01 for all pairwise Clin:Clin C.diff comparisons of ANOVA).
Within the C. difficile-inoculated treatments, the abundance of C. difficile was positively correlated (pearson correlation, r > 0.43, p < 0.01) with the relative abundances of Ruminococcus, Lachnospiraceae sp., Roseburia, and Porphyromonadaceae sp., in the Cip C.diff treatment, and with Oscillibacter, Intestinimonas, Enterococcus, Gemmiger, and Subdoligranulum in the Clin C.diff treatment. Ruminococcaceae sp. ,Butyricicoccus, Prevotella, Blautia, Faecalibacterium, and Streptococcus were positively correlated with both treatments (pearson correlation, r > 0.21, p < 0.01).
Discussion
Multiple studies have shown that an altered intestinal microbial community structure may allow the germination and colonization of indigenous or externally-derived C. difficile, which results in CDI, although the specific mechanisms behind this phenomenon are unknown (Britton and Young, 2014). In order to further the study of the relationship between CDI and native microbiota, the gut microbiome should be monitored from its pre-infection state through its infected state, which cannot be done in humans, as it requires stimulating a controlled infection. We therefore examined the colonization success of C. difficile in 8–10 week-old pigs following Clindamycin or Ciprofloxacin treatment.
We inoculated the pigs with strain C. difficile 078. In humans, this strain is regarded as mildly pathogenic, and the clinical manifestations of CDI ranges from no symptoms to severe colitis. In particular, C. difficile ribotype 078 toxinotype V has been isolated from affected humans as well as diarreal pigs (Goorhuis et al., 2008; Debast et al., 2009). Fecal shedding of C. difficile cells began 24 h after inoculation, and lasted up to 3 days in the Cip. C. diff treatment and up to 9 days in the Clin C. diff treatment, in agreement with previous findings for murine models (Reeves et al., 2011). It is possible that at the time of peak C. difficile shedding, histopathological changes would have been more pronounced; unfortunately no histopathological samples were taken at this time.
We did not test the effect of C. difficile inoculation in the absence of antibiotics. The association of CDI with prior exposure to antibiotics has been extensively studied over several decades [starting with (Bartlett et al., 1977)], consistently showing that an altered gut microbiome (i.e., through disease, aging, medication, or medical procedures) is a pre-condition for CDI (Britton and Young, 2012; Samarkos et al., 2018). In light of these findings, the omission of this experimental group is common in studies which employ animal models, as the sacrifice of additional animals is hard to justify given the available research (Reeves et al., 2011; Buffie et al., 2012; Schubert et al., 2015). Nevertheless, we do acknowledge that further study of the effect of C. difficile inoculation in the absence of antibiotics may aid in the understanding of the role of microbial interactions in the success of C. difficile.
We selected the antibiotics Ciprofloxacin and Clindamycin because Ciprofloxacin is one of the most common antibiotics used for humans worldwide, and Clindamycin has been reported to induce CDI (Britton and Young, 2014). Increased susceptibility to CDI due to Clindamycin and Ciprofloxacin administration has been reported (Best et al., 2012), although a study comparing the effects of several antibiotics in susceptibility to CDI in mice found that Clindamycin increased the risk of CDI, while no effect was detected for Ciprofloxacin-treated mice (Schubert et al., 2015). Our findings largely align with those of Schubert and colleagues. In our study, Ciprofloxacin-treated pigs were not significantly different from control pigs in terms of body temperature, and the microbiomes of Ciprofloxacin-treated pigs returned to Control diversity levels within 2 days after antibiotic administration. This is likely a result of the antibiotic's target populations: Ciprofloxacin-treated pigs exhibited relative decreases in Actinobacteria, Bacteroidetes and Proteobacterial phyla in their fecal microbiomes, and relative increases in the Firmicutes phylum, particularly the Blautia genus. Increases in the relative abundance of Blautia in response to Ciprofloxacin have been previously reported (Stewardson et al., 2015). Despite the increase in body temperature and the decrease in colon cell proliferation rates in the Cip C. diff group, the rapid recovery of α-diversity combined with the lack of major community dynamics within 2 days after antibiotic treatment suggests that the treatment was not strong or prolonged enough to cause major changes in the pig gut microbiome.
In contrast, Clindamycin treatment had a much more severe effect on the pigs and their fecal microbiomes. The fecal microbiomes of members of both Clin and Clin C. diff remained distinct from controls for 5 days after antibiotic administration, and while community composition for these treatments exhibited a trend toward recovery, it did not recover within the period studied. Body temperature was significantly higher than in controls for Clin and Clin C. diff, suggesting this was an effect of the antibiotic, and not of C. difficile administration. Notably, the relative abundance of Proteobacteria increased 1 day after disturbance, particularly Escherichia/Shigella, Enterobacter, Enterococcus, Prevotella, and Succinivibrio (members of cluster b) for both treatments. These taxa are rapid-growing opportunists (Fierer et al., 2007), and their dominance suggests the availability of resources following the antibiotic treatment. The increase in the relative abundance of Blautia, Bulleidia, Holdemanella, and other members of cluster c on day 2 occurred as members of cluster b decreased, suggesting that the latter were displaced.
Community dynamics in the fecal microbiomes of Clindamycin-treated pigs were similar regardless of whether C. difficile was present. For example, the Escherichia/Shigella genus, which is often positively correlated to C. difficile colonization (Schubert et al., 2015; Theriot and Young, 2015), increased in abundance in our experiment following Clindamycin treatment, even in the absence of C. difficile inoculation. Furthermore, while we did not find significant correlations between specific clusters of taxa and C. difficile abundances, the timing of growth of cluster a in the Clin and Clin C.diff treatments coincided with the end of C. difficile shedding in the Clin. C. diff treatment.
The community dynamics observed in both Clindamycin-treated groups—in particular displacement of taxa over time—are consistent with the patterns of secondary succession in microbial communities (Jurburg et al., 2017). Secondary succession, the process of recovery of disturbed communities, has been shown in a wide variety of microbial communities, from soil to gut microbiomes (Shade et al., 2012). We suggest that the competitive dynamics which arise following antibiotic treatment in the microbiome facilitate C. difficile establishment. This is consistent with a previous study, which showed that the time between antibiotic administration and C. difficile inoculation had a strong effect on the incidence of CDI (Schubert et al., 2014). The temporal sampling patterns of CDI-related microbiome research are generally relative to the patient (i.e., months), not the microbiome (i.e., days), however. Future research may consider treatments which accelerate successional dynamics, thereby reducing the window during which a C. difficile may become dominant.
Our finding that C. difficile abundances correlate positively with Gemminger, Roseburia, Subdoligranulum, Blautia, Prevotella, Intestinimonas, Ruminococcaceae sp., and Enterococcus is in agreement with previous findings, both in murine models and in human samples (Skraban et al., 2013; Schubert et al., 2015; Theriot and Young, 2015), indicating that our pig model is suitable for the study of CDI infections in humans. This experiment further provides evidence that weaned pigs can be colonized by the human pathogenic C. difficile strain 078, after disruption of the normal microbial community structure by pre-treatment with antibiotics. Since the post-weaning gut development and nutritional requirements of pigs closely resemble those of humans in many aspects (Litten-Brown et al., 2010; Meurens et al., 2012; Heinritz et al., 2013) antibiotic-treated pigs may be a preferred model to the currently used murine models to study the effect of nutritional-based intervention on the prevention of C. difficile infections in humans. Furthermore, since the gastrointestinal tract of pigs shows substantial similarities to that of humans and the pig is very similar to humans in terms of anatomy, genetics and physiology, the model described here offers an attractive intermediate for pre-clinical testing of preventive and/or therapeutic interventions for human CDI.
Data Availability
Data is available in the NCBI SRA under accession number PRJNA528235.
Ethics Statement
All procedures were approved by the animal experimentation board at Wageningen University & Research Center (accession number AVD401002015141) and carried out according to the guidelines of the European Council Directive 86/609/EEC dated November, 1986.
Author Contributions
SJ and JC performed sequence and statistical analyses and wrote the manuscript. JR, MS, and JC planned and performed the experiment. PdB performed molecular analyses and sequencing. All authors contributed substantially to revisions.
Funding
This research has been funded by the Dutch Ministry of Economic Affairs, TO-Flex project Intestinal Health Screening (BO-34-002-002).
Conflict of Interest Statement
The authors declare that the research was conducted in the absence of any commercial or financial relationships that could be construed as a potential conflict of interest.
Acknowledgments
We would like to thank S. Tem for their helpful comments.
Supplementary Material
The Supplementary Material for this article can be found online at: https://www.frontiersin.org/articles/10.3389/fcimb.2019.00271/full#supplementary-material
References
Andersen, A. D., Mølbak, L., Thymann, T., Michaelsen, K. F., and Lauritzen, L. (2011). Dietary long-chain n-3 PUFA, gut microbiota and fat mass in early postnatal piglet development—exploring a potential interplay. Prostaglandins Leukot. Essent. Fat. Acids 85, 345–351. doi: 10.1016/j.plefa.2011.08.004
Arruda, P. H. E., Madson, D. M., Ramirez, A., Rowe, E., Lizer, J. T., and Songer, J. G. (2013). Effect of age, dose and antibiotic therapy on the development of Clostridium difficile infection in neonatal piglets. Anaerobe 22, 104–110. doi: 10.1016/j.anaerobe.2013.04.010
Bartlett, J. G., Onderdonk, A. B., Cisneros, R. L., and Kasper, D. L. (1977). Clindamycin-associated colitis due to a toxin-producing species of Clostridium in hamsters. J. Infect. Dis. 136, 701–705. doi: 10.1093/infdis/136.5.701
Best, E. L., Freeman, J., and Wilcox, M. H. (2012). Models for the study of Clostridium difficile infection. Gut Microbes 3, 145–167. doi: 10.4161/gmic.19526
Bianchi, A. T. J., Zwart, R. J., Jeurissen, S. H. M., and Moonen-Leusen, H. W. M. (1992). Development of the B-and T-cell compartments in porcine lymphoid organs from birth to adult life: an immunohistological approach. Vet. Immunol. Immunopathol. 33, 201–221. doi: 10.1016/0165-2427(92)90182-P
Britton, R. A., and Young, V. B. (2012). Interaction between the intestinal microbiota and host in Clostridium difficile colonization resistance. Trends Microbiol. 20, 313–319. doi: 10.1016/j.tim.2012.04.001
Britton, R. A., and Young, V. B. (2014). Role of the intestinal microbiota in resistance to colonization by Clostridium difficile. Gastroenterology 146, 1547–1553. doi: 10.1053/j.gastro.2014.01.059
Buffie, C. G., Jarchum, I., Equinda, M., Lipuma, L., Gobourne, A., Viale, A., et al. (2012). Profound alterations of intestinal microbiota following a single dose of clindamycin results in sustained susceptibility to Clostridium difficile-induced colitis. Infect. Immun. 80, 62–73. doi: 10.1128/IAI.05496-11
Callahan, B. J., McMurdie, P. J., Rosen, M. J., Han, A. W., Johnson, A. J. A., and Holmes, S. P. (2016). DADA2: high-resolution sample inference from Illumina amplicon data. Nat. Methods 13:581. doi: 10.1038/nmeth.3869
Caporaso, J. G., Lauber, C. L., Walters, W. A., Berg-Lyons, D., Huntley, J., Fierer, N., et al. (2012). Ultra-high-throughput microbial community analysis on the Illumina HiSeq and MiSeq platforms. ISME J. 6, 1621–1624. doi: 10.1038/ismej.2012.8
Choi, H. H., and Cho, Y.-S. (2016). Fecal microbiota transplantation: current applications, effectiveness, and future perspectives. Clin. Endosc. 49, 257–265. doi: 10.5946/ce.2015.117
Debast, S. B., Van Leengoed, L. A. M. G., Goorhuis, A., Harmanus, C., Kuijper, E. J., and Bergwerff, A. A. (2009). Clostridium difficile PCR ribotype 078 toxinotype V found in diarrhoeal pigs identical to isolates from affected humans. Environ. Microbiol. 11, 505–511. doi: 10.1111/j.1462-2920.2008.01790.x
Fierer, N., Bradford, M. A., and Jackson, R. B. (2007). Toward an ecological classification of soil bacteria. Ecology 88, 1354–1364. doi: 10.1890/05-1839
Goorhuis, A., Bakker, D., Corver, J., Debast, S. B., Harmanus, C., Notermans, D. W., et al. (2008). Emergence of Clostridium difficile infection due to a new hypervirulent strain, polymerase chain reaction ribotype 078. Clin. Infect. Dis. 47, 1162–1170. doi: 10.1086/592257
Heinritz, S. N., Mosenthin, R., and Weiss, E. (2013). Use of pigs as a potential model for research into dietary modulation of the human gut microbiota. Nutr. Res. Rev. 26, 191–209. doi: 10.1017/S0954422413000152
Johnson, M., Zaretskaya, I., Raytselis, Y., Merezhuk, Y., McGinnis, S., and Madden, T. L. (2008). NCBI BLAST: a better web interface. Nucleic Acids Res. 36, W5–W9. doi: 10.1093/nar/gkn201
Jurburg, S. D., Nunes, I., Stegen, J. C., Le Roux, X., Prieme, A., Sørensen, S. J., et al. (2017). Autogenic succession and deterministic recovery following disturbance in soil bacterial communities. Sci. Rep. 7:45691. doi: 10.1038/srep45691
Kociolek, L. K., and Gerding, D. N. (2016). Breakthroughs in the treatment and prevention of Clostridium difficile infection. Nat. Rev. Gastroenterol. Hepatol. 13:150. doi: 10.1038/nrgastro.2015.220
Ladirat, S. E., Schols, H. A., Nauta, A., Schoterman, M. H. C., Keijser, B. J. F., Montijn, R. C., et al. (2013). High-throughput analysis of the impact of antibiotics on the human intestinal microbiota composition. J. Microbiol. Methods 92, 387–397. doi: 10.1016/j.mimet.2012.12.011
Laffan, A. M., Bellantoni, M. F., Greenough, W. B., and Zenilman, J. M. (2006). Burden of Clostridium Difficile-associated diarrhea in a long-term care facility. J. Am. Geriatr. Soc. 54, 1068–1073. doi: 10.1111/j.1532-5415.2006.00768.x
Litten-Brown, J. C., Corson, A. M., and Clarke, L. (2010). Porcine models for the metabolic syndrome, digestive and bone disorders: a general overview. Animal 4, 899–920. doi: 10.1017/S1751731110000200
Marsh, J. W., Arora, R., Schlackman, J. L., Shutt, K. A., Curry, S. R., and Harrison, L. H. (2012). Association of relapse of Clostridium difficile disease with BI/NAP1/027. J. Clin. Microbiol. 50, 4078–4082. doi: 10.1128/JCM.02291-12
McMurdie, P. J., and Holmes, S. (2013). phyloseq: an R package for reproducible interactive analysis and graphics of microbiome census data. PLoS ONE 8:e61217. doi: 10.1371/journal.pone.0061217
Meurens, F., Summerfield, A., Nauwynck, H., Saif, L., and Gerdts, V. (2012). The pig: a model for human infectious diseases. Trends Microbiol. 20, 50–57. doi: 10.1016/j.tim.2011.11.002
Monaghan, T. M. (2015). New perspectives in Clostridium difficile disease pathogenesis. Infect. Dis. Clin. 29, 1–11. doi: 10.1016/j.idc.2014.11.007
Norman, K. N., Scott, H. M., Harvey, R. B., Norby, B., and Hume, M. E. (2014). Comparison of antimicrobial susceptibility among Clostridium difficile isolated from an integrated human and swine population in Texas. Foodborne Pathog. Dis. 11, 257–264. doi: 10.1089/fpd.2013.1648
Oksanen, J., Blanchet, F. G., Kindt, R., Legendre, P., Minchin, P. R., O'Hara, R. B., et al. (2013). Package ‘vegan.’ Community Ecolology Package. version 2, 1–295.
Paterson, D. L. (2004). “Collateral damage” from cephalosporin or quinolone antibiotic therapy. Clin. Infect. Dis. 38, S341–S345. doi: 10.1086/382690
R Core Team (2014). R: A Language and Environment for Statistical Computing. Vienna: R Foundation for Statistical Computing 2012. Available online at: https://cran.r-project.org
Reeves, A. E., Theriot, C. M., Bergin, I. L., Huffnagle, G. B., Schloss, P. D., and Young, V. B. (2011). The interplay between microbiome dynamics and pathogen dynamics in a murine model of Clostridium difficile infection. Gut Microbes 2, 145–158. doi: 10.4161/gmic.2.3.16333
Samarkos, M., Mastrogianni, E., and Kampouropoulou, O. (2018). The role of human gut microbiota in Clostridium difficile infection. Acta Microbiol. Hell. 63, 89–100. doi: 10.1016/j.ejim.2018.02.006
Schubert, A. M., Rogers, M. A. M., Ring, C., Mogle, J., Petrosino, J. P., Young, V. B., et al. (2014). Microbiome data distinguish patients with Clostridium difficile infection and non-C. difficile-associated diarrhea from healthy controls. MBio 5, e01021–e01014. doi: 10.1128/mBio.01021-14
Schubert, A. M., Sinani, H., and Schloss, P. D. (2015). Antibiotic-induced alterations of the murine gut microbiota and subsequent effects on colonization resistance against Clostridium difficile. MBio 6, 1–10. doi: 10.1128/mBio.00974-15
Shade, A., Peter, H., Allison, S. D., Baho, D. L., Berga, M., Bürgmann, H., et al. (2012). Fundamentals of microbial community resistance and resilience. Front. Microbiol. 3:417. doi: 10.3389/fmicb.2012.00417
Skraban, J., Dzeroski, S., Zenko, B., Mongus, D., Gangl, S., and Rupnik, M. (2013). Gut microbiota patterns associated with colonization of different Clostridium difficile ribotypes. PLoS ONE 8:e58005. doi: 10.1371/journal.pone.0058005
Stewardson, A. J., Gaïa, N., Francois, P., Malhotra-Kumar, S., Delemont, C., de Tejada, B. M., et al. (2015). Collateral damage from oral ciprofloxacin versus nitrofurantoin in outpatients with urinary tract infections: a culture-free analysis of gut microbiota. Clin. Microbiol. Infect. 21, 344.e1–344.e11. doi: 10.1016/j.cmi.2014.11.016
Theriot, C. M., and Young, V. B. (2015). Interactions between the gastrointestinal microbiome and Clostridium difficile. Annu. Rev. Microbiol. 69, 445–461. doi: 10.1146/annurev-micro-091014-104115
Van den Brink, P. J., and Ter Braak, C. J. F. (1999). Principal response curves: analysis of time-dependent multivariate responses of biological community to stress. Environ. Toxicol. Chem. 18, 138–148. doi: 10.1002/etc.5620180207
Wang, Q., Garrity, G. M., Tiedje, J. M., and Cole, J. R. (2007). Naive Bayesian classifier for rapid assignment of rRNA sequences into the new bacterial taxonomy. Appl. Environ. Microbiol. 73, 5261–5267. doi: 10.1128/AEM.00062-07
Zekarias, B., Stockhofe-Zurwieden, N., Post, J., Balk, F., van Reenen, C., Gruys, E., et al. (2005). The pathogenesis of and susceptibility to malabsorption syndrome in broilers is associated with heterophil influx into the intestinal mucosa and epithelial apoptosis. Avian Pathol. 34, 402–407. doi: 10.1080/03079450500268328
Keywords: Clostridium difficile, pig, microbiome, animal models, bacteria
Citation: Jurburg SD, Cornelissen JJBWJ, de Boer P, Smits MA and Rebel JMJ (2019) Successional Dynamics in the Gut Microbiome Determine the Success of Clostridium difficile Infection in Adult Pig Models. Front. Cell. Infect. Microbiol. 9:271. doi: 10.3389/fcimb.2019.00271
Received: 15 May 2019; Accepted: 15 July 2019;
Published: 06 August 2019.
Edited by:
Shai Bel, Bar-Ilan University, IsraelReviewed by:
Avi Peretz, The Baruch Padeh Medical Center, Poriya, IsraelGiovanni Widmer, Cummings School of Veterinary Medicine, Tufts University, United States
Copyright © 2019 Jurburg, Cornelissen, de Boer, Smits and Rebel. This is an open-access article distributed under the terms of the Creative Commons Attribution License (CC BY). The use, distribution or reproduction in other forums is permitted, provided the original author(s) and the copyright owner(s) are credited and that the original publication in this journal is cited, in accordance with accepted academic practice. No use, distribution or reproduction is permitted which does not comply with these terms.
*Correspondence: Johanna M. J. Rebel, annemarie.rebel@wur.nl