“The sleep data looks way better than I feel.” An autoethnographic account and diffractive reading of sleep-tracking
- 1Cognitive Science Research Group, School of Electronic Engineering and Computer Science, Queen Mary University of London, London, United Kingdom
- 2Future Interactive Technologies Lab, Department of Computer Science, School of Mathematics and Computer Science, Swansea University, Swansea, United Kingdom
Sleep-tracking products are promising their users an improvement to their sleep by focusing on behavior change but often neglecting the contextual and individual factors contributing to sleep quality and quantity. Making good sleep for productive scheduling a personal responsibility does not necessarily lead to better sleep and may cause stress and anxiety. In an autoethnographic study, the first author of this paper tracked her sleep for one month using a diary, body maps and an Oura ring and compared her subjectively felt sleep experience with the data produced by the Oura app. A thematic analysis of the data resulted in four themes describing the relationship between the user-researcher and her wearable sleep-tracker: (1) good sleep scores are motivating, (2) experience that matches the data leads to sense-making, (3) contradictory information from the app leads to frustration, and (4) the sleep-tracker competes with other social agents. A diffractive reading of the data and research process, following Karen Barad's methodology, resulted in a discussion of how data passes through the analog and digital apparatus and what contextual factors are left out but still significantly impact sleep quality and quantity. We add to a canon of sleep research recommending a move away from representing sleep in terms of comparison and competition, uncoupling it from neoliberal capitalistic productivity and self-improvement narratives which are often key contributing factors to bad sleep in the first place.
1 Introduction
Sleep is a much-discussed topic today. The public discourse emphasizes both the benefits of sleep for physical and mental health, well-being and functioning and the risks associated with poor sleep quality and quantity. This discourse is also associated with an assumed widespread sleep deprivation and new, increasingly diagnosed sleep disorders. De Christofaro and Chiodo (2023) and others refer to this concurrence of emergent concerns the sleep crisis. This sleep crisis goes hand in hand with a growing industry of sleep-related products. According to an online survey from Statista Consumer Insights, nearly a quarter of the respondents in India and China said they had used sleep monitoring apps in the 12 months prior to the survey. In the United States, 15% of adults said they used apps such as Sleep Cycle and Sleep Time (Fleck, 2023) and it is estimated that 10% of US adults are using wearable fitness- and sleep-tracking devices regularly while up to 50% consider purchasing one (Baron et al., 2017).
Studies show that in the past half-century, the self-reported sleep quality and duration among adults have decreased (Kronholm et al., 2008; Ford et al., 2015). Among factors contributing to sub-optimal sleep duration, researchers have identified lower socioeconomic status (Stamatakis et al., 2007), lifestyle and stress (Vgontzas et al., 2008; Seixas et al., 2015). Behaviors such as smoking, heavy drinking and physical inactivity are also associated with lower sleep quality. While those behaviors are often perceived as attributable to individual differences, Crary (2014) argues in his influential book 24/7: Late Capitalism and the Ends of Sleep that they are linked to chronic emotional stress caused by socioeconomic pressures leading to working irregular schedules and less access to healthcare. Consumer-grade sleep-trackers, one available product category among many in the sleep industry, purport to address an individual's sleep goals by analysing their personal biodata and targeting individual behavior change without addressing any of the social or contextual factors contributing to sleep quality (Kroker, 2016). Normative categories of sleep are encoded into sleep-tracking devices, neglect subjective experience and situational factors and contribute to the commodification of people's lives (Sanches et al., 2022). Sleep-related products promise the optimization of the self by putting the positive, self-restorative effects of sleep to work. The adoption of a sleep-tracker transforms sleep from an unconscious, un-reflexive experience into an active, measurable performance. Using the device can turn sleep into a responsibility for the individual to improve upon (Lockton et al., 2020).
Personal informatics systems are based on the premise that the tracking of personal data increases awareness and motivates desired behavior changes (Choe et al., 2011). Tracking one's sleep does increase awareness about personal sleep patterns and has shown to improve sleep quality among people suffering from insomnia and in clinical settings (Altena et al., 2008; De Zambotti et al., 2019). Measuring sleep purely as a lifestyle choice has proven less useful. Users do not know how to act upon the presented data, as sleep depends on many internal (mental and physical) and external (social, technological, economic, stress-related, environmental) factors which are beyond the control of the individual (Liu et al., 2015; Liang and Ploderer, 2016; Ravichandran et al., 2017). Sleep-tracking technology can even become disruptive to sleep quality by reinforcing sleep-related anxiety and exacerbating insomnia: that is, the phenomenon of orthosomnia (Baron et al., 2017).
Commercially available wearable sleep-trackers collect biodata and calculate sleep stages, aiming to produce similar results to polysomnography, the time and cost-expensive gold-standard method of sleep-tracking. These devices are usually worn on the head, wrist or finger and, depending on the device, they include sensors to measure EEG, movement, heart rate, temperature and blood oxygen saturation (Pan et al., 2020). Biodata is produced by human bodies and behaviors, but it is not independent of its origins, context and temporal history. Human-computer interaction (HCI) research and design increasingly involves both human and non-human stakeholders and factors. Frauenberger (2020) labels this new wave of HCI research “entanglement HCI” which is based on posthuman theories. The physicist and Science and Technology Studies (STS) scholar Barad (2007) argues that all phenomena are emergent through the intra-action of all entangled components. She proposes diffraction as an analytical method for attending to differences and their effects as part of entangled processes. As a method, it is suitable for looking at the entanglement of sleep, sleep data, and a sleep-tracking device to understand their relationships and how they are mutually involved in the individual, social and contextual perception of the sleeping self.
In this paper we present an autoethnographic study to deepen the understanding of the relationship between a user's sleep and her wearable sleep-tracker by tracking and describing sleep other than in numbers and words alone. The methodology was chosen to gain insights into the contextual factors that sleep-tracking devices, which interpret sleep based on a set of biodata, do not consider in their evaluation and further ask what this implicates for the design of such devices. For data collection the first author engaged in two different forms of sleep-tracking for a month in summer 2022. She tracked her sleep with an Oura Ring Generation 3 1 alongside completing body maps and questionnaires about her sleep for 18 mornings and compared her notes to the sleep data generated by Oura.
In recent years, autoethnography and first-person research methods are increasingly utilized in design and HCI research (Lucero et al., 2019; Desjardins et al., 2021; Höök et al., 2021). An increased interest, which according to Lockton et al. (2020) coincides with an increase in self-tracking technologies (Lupton, 2014) and an expanding body of research in personal informatics (Rooksby et al., 2014; Ayobi et al., 2016). Commercially available self-tracking technology is built on a logic of knowing through data and linked to productivity. They aid self-reflection and autoethnographic enquiry, but the data leaves out much of what it is to be a (sleeping or tired) person. Knowing one's data and acting on it is situated and contextual and thus affects the experience.
For the analysis of this autoethnographic study, we follow diffractive design principles as described by Sanches et al. (2022). We pay attention to material entanglements and practices constituting everyday life and how these different elements interfere while tracking sleep, and how sleep data in return interferes with the everyday. The engagement with the data is open-ended and aimed at exploring practices around data. We hold space for messiness and ambiguity in the interpretation of the data. We engage with the data diffractively in a non-representational way through this autoethnographic study, arguing that data cannot straightforwardly represent or reflect the felt experience of sleeping and that attention to the context is necessary for interpreting personal sleep data.
2 Materials and methods
The purpose of autoethnographic research methods in HCI is to gain a deep and experiential understanding of the effect of technology on people, society and everyday life in the complex world in which it is used (Lucero et al., 2019). Human bodies express our “double status as object and subject—as something in the world and as a sensibility that experiences, feels, and acts in the world” (Shusterman, 2006). Autoethnography as a method acknowledges and appreciates the researcher's subjectivity and influence on the research. With it, we intend to call into question the objective observer position of the researcher and to disturb notions of the coherent individual self and its binaries of self/society and objective/subjective (Reed-Danahay, 1997). Breaking down binaries is a suitable approach to investigate sleep-tracking technology, as sleep itself is depending on various internal and environmental factors and so is sleep data.
A representation of sleep in numbers and words promotes a division of body and mind and establishes a data double that is at risk to be understood as objective information only (Viseu and Suchman, 2010). Body maps are suitable tools to capture autoethnographic exploration and to counteract the reliance on words and numbers alone when tracking sleep. They are visual documents where somatic experiences can be drawn onto a graphical representation of an outline of the human body. They can capture complex and non-explicit emotions and felt sensations, elaborating narratives that cannot simply be spoken (Mah et al., 2021).
By allowing non-verbal insight into bodily processes this research critically assesses “normal” functioning as a human and the participation in networks impacting sleep. These networks are external (between us and the world) as well as internal (between us and ourselves). For this study we produce visual references to compare the felt experience of sleeping with sleep-tracker data, which aids the analysis and discussion. As an autoethnographic study it is not intended to generate general evidence or results directly comparable to the experience of others. According to Williams (2015) the production of idiosyncratic accounts can create empathy for how one's own life is entangled with others. It can foster a rich understanding of the role of personal devices in our lives, how they evaluate sleep, what their limitations are and ultimately can aid in raising questions about potential design implications.
2.1 Study protocol
For this autoethnographic study we designed a qualitative workbook containing 30 copies of blank body maps, diary prompts, felt-tip markers and a pen (Figure 1). During the study period, within 30 minutes after waking up in the morning, each recording starts with sitting or lying down with closed eyes, drawing attention to the different body parts from head to toe and taking mental notes on how each part is feeling (Cochrane et al., 2022). Then the researcher opens her eyes and turns to the pages in the workbook, using the colored felt-tip markers, marking the body parts both at the front and the back of the body map which felt most present in colors she deems suitable to represent the feeling at that moment. There are no restrictions or guidelines on creating symbols or patterns to represent sensory information. Then the participant-researcher (Anna) turns the page and answers the four prompts in writing. The first diary prompt invites the researcher to annotate the drawings with key terms and then to describe the night's sleep in words. The second prompt asks to describe the sensations or external factors impacting the night's sleep quality. Those first two prompts are there to aid the interpretation of the visual data and make it accessible to people other than the researcher herself.
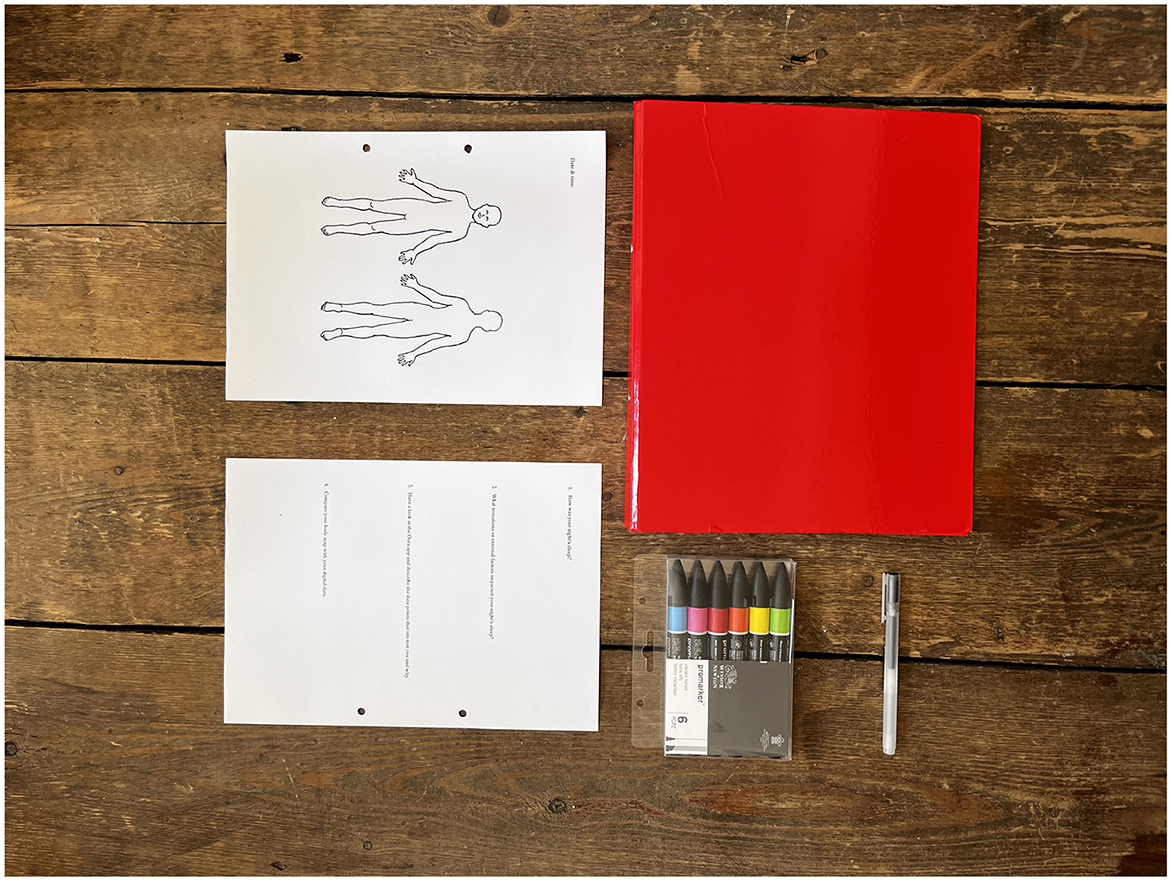
Figure 1. The materials for an autoethnographic diary-study, a folder containing 30 sheets of paper with body maps printed on one side, questions on the other side, six colored felt-tip markers and one black pen.
Then the researcher is prompted to open the Oura app on her smartphone, to look at the sleep data and to describe data points she is looking at and why they are interesting to her. For further analysis, the researcher takes screenshots of every data point that she comments on. The last prompt asks her to compare the completed body map with the sleep data from Oura.
This study ran for one month from 18 July 2022—18 August 2022. The first author of this paper recorded the felt experience of 18 nights' sleep in total by completing body maps, followed by the diary prompts. The goal was to complete at least 10 body maps within this month.
2.2 Data collection
The autoethnographic data collection was carried out by the first author of this paper. To highlight this subjective perspective, this sub-section is written in the first-person singular. This contextual information about the research apparatus and the researcher's subjective positionality in this process bears particular importance for the diffractive analysis of the data in section 3.2.
2.2.1 Positionality statement
I am both researcher and research participant in this autoethnographic study. I am also a PhD candidate in Media and Arts Technology at Queen Mary University of London and I have been studying wearable sleep-tracking technology since 2019. In previous empirical work on sleep-trackers I conducted a discourse analysis on commercially available, wearable sleep-tracking technology which resulted in an overview of the subjectivities - both human (users) and non-human (devices) - those discourses produce and some insights into the relationship between the two (Nagele et al., 2022). I do not consider myself experiencing any sleep disorder or chronic sleep disruption and I consider my sleep overall as good. My ideal sleep is consistent 8 hours of sleep per night. Anything below 6.5 hours of sleep has a significant impact on my normal functioning. Years before starting my research on sleep-tracking I experienced bouts of sleeping too much, which, as I know now, is considered an unhealthy behavior linked to emotional distress (Seixas et al., 2015). As part of a postgraduate-level course I undertook in 2021 I wrote some fragments about this period in my life which I would like to bring into this discussion:
7.6 At times I give myself up to diminishment. Then I prefer to not change my clothes at all between night and day, waking and sleeping. Long underwear is equally suitable for the bed. Sweatpants and sweatshirts can be used for yoga, sleep, work, and any other activity at home. The radius of home can be extended to the supermarket, the corner shop, the pharmacy or the post office.
[...]
8.2 There was a time in my life where I slept a lot, on average up until 5 pm everyday. Some nights I stayed up late, aimlessly scrolling through social media feeds, on others I simply went back to sleep after a short period of being awake. I did not see a point in waking up. I had nothing else to do, nothing to miss, nothing to lose if I just slept. Nobody missed me, nobody knew what I was doing. I had no job. I just graduated from university and having a job seemed to be the only meaning of life for everyone else. During the few hours I was awake everyday I applied for some positions or did not, took a shower or did not, ate something and sometimes met up with friends. I did not tell them about my sleeping habit.
8.3 When I finally found a job—for a two-week project in Berlin—my sleep-wake rhythm was far from normal and unsuitable to working life. When I arrived in Berlin on the Sunday morning before my work was about to begin, I lay down in my friend's flat for 20 h of uninterrupted, deep sleep only to wake up tired and worn out at 7 am on Monday morning to get to work. I cannot recall what happened in those two weeks, coffee kept me awake during the days and as soon as the working day was over I returned to the sofa I was sleeping on. I felt unsuitable to be a person. A working person.
I started researching sleep-trackers before having ever tracked my own sleep (nor my activity). As part of my research I came across an autoethnographic research paper by Salmela et al. (2019)—An autoethnographic study of (not) using a wearable sleep-tracking device. Up to this point I had read a lot about tracking biodata, privacy and ethical concerns e.g., Lupton (2014) and came across many voices of resistance to using such devices e.g., Crary (2014). In their paper Salmela et al. (2019) argue that the thing-power of the Oura ring as a research device is “threatening the limits and boundaries of what [they] consider as intimate-public-work-related lives”. I understand and empathize with this argument, but at the same time I felt uncomfortable with fact that the researchers are not familiar by experience with the devices they are studying. As I myself had never tracked my sleep before, on reading this paper I felt the urge to try it out; it felt necessary to create my own stance toward the practice of sleep-tracking.
In July 2020 I acquired a Fitbit Charge 4. At the time I started a page in my Notes app on my phone to document this experience of the first time ever tracking my sleep. I assumed that it might come in handy at some stage in my research. From the moment I received the Fitbit I was really excited about the new device, as it was the first time I was about to track my sleep:
It's one and a half days since I'm using the Fitbit and I'm still very excited about it. I check my data at least once every hour and try to comply with it's recommendation of walking 250 steps each hour between 9 and 6, the hours I most often spend sitting at a desk.
After the first night I slept with my Fitbit I woke up in the morning and the first thing I did was checking the app. For a second I got scared since I did not see any data as it was still updating and I was worried that I would have lost one day of data. I got a good sleep score and went through all the metrics to understand what they mean. I was surprised about the many wake-ups during the night and the little deep sleep I had.
[...]
Last night I expected to get a good night sleep since I did a lot of exercises during the day. Even though I was really tired I stayed up chatting to my boyfriend over a glass of wine and then struggled to fall asleep as quickly as I expected. Overall I slept less and with a worse sleep score than the night before. When I woke up I felt sleepy but both mornings I woke up faster than before the Fitbit because I was excited to see my scores. Strange, that this makes me associate sleeping with a performance score. (Notes, 29/07/2020)
In the beginning I wore it all the time and checked every piece of information I could get:
I've used the Fitbit now for two weeks in a row. I want to wear it all the time to get as much data as possible about myself. During the day I compete in step competitions with my partner and I get irritated when I have to charge it for an hour and thus don't wear it around the house. Generally it gives me the feeling I am quite fit and have good sleep. I've not received any negative feedback and I'm allegedly doing better than other women my age. I wonder if there is anyone who actually gets negative feedback. I noticed that my REM sleep is slightly above average, which makes sense since I feel like I dream a lot. (Notes, 11/08/2020)
After a few weeks I already noticed my excitement about sleep data fading. I raised concerns about the quality of Fitbit's sleep-tracking and describe how I found it more satisfying to hit daily goals such as walking 10,000 steps, as those could be met intentionally:
I sometimes check my sleep score but not daily, since I usually sleep well. When I do, I compare my sleep phases to my 30 day average and to the benchmark, this gives me a good indication of my sleep that night. I also look at the heart rate, since I noticed that it is directly affected by drinking alcohol or eating late.
One night I slept terrible. My partner was ill and kept me up all night. That next day the sleep data said that I slept for 2.5 h only, and that that's too little for sleep analysis. I would have expected more information since I was still in bed trying to sleep for the whole night.
It feels like for sleep the Fitbit is quite limited. It works better for tracking goals such as walking 10.000 steps a day or 250 steps every hour. (Notes, 10/09/2020)
During that period I was conducting interviews with users of wearable sleep-trackers. I found, that users of the Oura ring provided me with more details about their sleep-scores than users of other sleep-trackers. I ordered an Oura Ring Generation 3.
The golden Oura ring I chose looks almost like a piece of fine jewelry. It is very subtle, without a screen or any possibilities for interaction. As other fitness trackers it comes with a companion app for the phone. I have tracked my sleep and activity with the Oura ring on-and-off since August 2021, thus I am familiar with the ring and the sleep data it produces before engaging in this month of autoethnographic enquiry.
2.2.2 My practice of tracking
For the summer of 2022 I planned to stay in Austria for two months. It appeared to be a suitable time to conduct this study then, since life there is less predictable than my life as a PhD researcher in London, where I am moving between working at a desk at home, a desk at university, teaching, house-chores and seeing friends in the evenings or on weekends. Situating this study outside my regular routine we hoped to get more insights into different factors impacting sleep.
It was a hot and dry summer. My childhood home is embedded in a small village in rural Austria. It is mostly quiet and I sleep with the windows open in a south-east-facing room with no curtains to stop the sun from coming into the room each morning. There are no street-lights outside to disturb the darkness. Before the tracking began, I knew that some nights I would spend alone at home, other nights my mother would be in the house, or my boyfriend with me, and I was expecting a friend to visit me for several days during this time. There was some work on the farm I had agreed to do. There are lakes in the area where I was planning to spend some of my time off. Other than that I did not know what else might come up and impact my sleep during this time.
Each recording session is supposed to be preceded by a short body scanning activity within 30 min after waking up. I have gone through periods in my life where I meditated, so this is not new to me. I have completed a couple of body maps in preparation for this study. When looking back at my drawings I notice, that at the beginning of the study I was much more confined by the outlines of the body map. As I completed more and more body maps I can see that I started applying shapes on top of the body that go beyond and cross the outlines printed on the paper (i.e., Figures 3A, 12A, 13A).
At the start of the study period I completed five diary entries on five consecutive mornings. After that I was less regular with my entries. I do not complete the diary prompts on days with early commitments, over-sleeping or because I am away from home and do not have the time and space to complete my diary. A few times during this period I forgot to charge the Oura ring before going to sleep. On those days, the app only shows limited data. This is a lack of care from my side, but also linked to the fact that I do not always have my phone with me, thus missing the notifications from Oura reminding me to charge the ring before going to bed. Over one month 18 complete data sets including body maps, notes and screenshots from the Oura app were collected.
2.3 Data analysis
The initial analysis of the collected data follows the qualitative approach of thematic analysis (Braun and Clarke, 2012), looking for patterns in the marks, colors used, and symbols drawn, their comparison to the digitally generated data and the notes taken. In a first round, the participant-researcher analyzes the data for themes which are then in a second round of analysis shared and discussed alongside the raw data with the other researcher. In the analysis we pay particular attention to the entanglement of the sleeping body with external, environmental and internal, physical and psychological factors that affect the body and the night's sleep.
In a secondary meta-analysis we engage with the data diffractively, not focused on the specific attributes of an individual subject or object but on the ongoing phenomena emerging from entangled sleep-tracking. The field of design research is increasingly interested in diffraction as a method for working with data, as diffraction resists treating data as objective or neutral but views data as situated and entangled in the messiness of the lived world. It allows for insights into the ongoing transformation of meaning when living with biodata (Sanches et al., 2022). With the diffractive method of analysing data, Barad (2007) suggests an analysis of social and natural phenomena, material and discursive practices and the role of human and non-human subjects through one another in order to clarify the relationship between them. Knowledge-making practices such as datafication of sleep but also research methodologies such as this one are part of the phenomena we describe, they have material consequences and participate in shaping the world. By “reading insights through one another” we attune to the networks of producing knowledge about one's sleep rather than presuming boundaries and research subject and object in advance.
Looking at this autoethnographic data diffractively both gives insights into the entangling factors co-producing sleep data but also sleep itself. It allows to explore the differences between practices (Barad, 2007). We emphasize the moments where the two practices of body-mapping, narrating the participant-researcher's sleep and automatic sleep-tracking overlap.
For the analysis the first author of this paper prepared the data by transcribing her hand-written diary entries and notes into a digital format. The following Results section highlights the emergence of felt meaning by describing both the practice of sleep-tracking alongside a reflection. Diffraction patterns start to emerge and give insights into the entangled nature of both sleep and sleep-tracking.
3 Results
From the collected data, we present four themes about the relationships between the researcher and her sleep-tracker that emerged from the thematic analysis of the autoethnograhic data, including examples of each theme. The four themes we found are that good sleep-scores are motivating (Theme 1), experience that matches the data leads to sense-making (Theme 2), contradicting information from the app leads to frustration (Theme 3) and sleep-trackers compete with other social agents (Theme 4).
The study also aimed at trying to understand and describe the complex experience of sleeping other than in numbers and words as compared to the Oura sleep-tracking app. A diffractive reading of the materials and methods, the data and the four themes opens up perspectives on how sleep-tracking technology is involved in the experience of the participant-researchers' sleep and how it ties this fundamental bodily need to capitalist modes of production. It offers a look into the sociotechnical and nature-cultural relationships that “produce” sleep today.
3.1 Thematic insights into the relationship between user and sleep-tracker
The themes presented in this section emerged from a thematic analysis of the data gathered during the month of autoethnographic enquiry: 18 annotated body maps with diary entries and 127 screenshots from the Oura app. The entire dataset is available in the Supplementary material of this paper. We coded the body maps, diary entries and screenshots following an inductive, iterative approach. Four themes on how sleep-tracking technology is involved in the experience of the participant-researchers' sleep emerged.
3.1.1 Theme 1: good sleep scores are motivating
In several cases the participant-researcher (Anna) described not feeling refreshed after sleeping, not having had a good night sleep, the Sleep score and the Readiness score2 however being high, followed by a positive comment from the app. She described how seeing good scores on a bad morning are motivating to get through the day, overriding the initial bad feeling by improving it.
In her first diary entry Anna referred to a wedding she had attended two days before. The body map showed multiple uncomfortable feelings such as feeling sour in the stomach due to drinking alcohol and warm legs from dancing (Figure 2A). In the accompanying diary entry she described how this social occasion made her feel the night and morning following:
A wedding the two days and nights before left me quite exhausted, a bit anxious. I drank a bit too much and didn't know many people. I'm a bit achey overall, still a hangover from the wedding I went to. (18/7 - 8:25)
This felt experience was not represented in the sleep scores, it was worse than the data suggested (Figure 2B):
Wow, I'm very surprised to see a Readiness score of 92 and Sleep of 85, both with a little crown on. I don't feel as fresh yet, but it's good to see my heart-rate lowered to 65, for me that's as low as it gets. Seems I recovered well. I also had enough deep sleep. The HRV (Heart Rate Variability) is surprisingly high too with 54! My body doesn't feel as good as the Oura suggests but it is motivating—at least I feel confident for the day. (18/7 - 8:25)
Good data motivated the participant-researcher with confidence for the day. Similarly, on a different occasion (Figure 3) she wrote:
I must have slept deep I was so tired. In the morning it was hard to open my eyes and get up. It was hot and a bit loud in the room. We came home later than I thought or hoped and had more to drink than I like. In the morning the street got super busy. It woke me up. The [Sleep] data pretty much matches my experience. The good [Readiness] score for the day is better than expected but it motivates me to get up and do things. (14/8 - 10:22)
3.1.2 Theme 2: experience that matches the data leads to sense-making
Mornings where the felt experience matched the scores presented by Oura (i.e., feeling bad when waking up and the scores on the app are low, or when waking up feeling energized combined with high scores) Anna described how she was analysing the sleep phases in detail in order to establish her personal baseline. She described how feeling recovered often coincided with a longer than average (based on her memory alone) Deep Sleep phase and that vivid dreams or a busy mind often coincided with a long REM (Rapid Eye Movement) sleep phase:
I slept very well today, just once I remember waking up because of a cold draft of air. My dreams where very vivid and real tonight. I'm slightly confused and still deciphering what is real and what is not. [...] Sleep score is not as high as I would have thought - but I had a lot of REM sleep which probably correlates with my many dreams. It says deep sleep is low but 1 hour seems good to me. (20/7 - 8:30) (Figure 4)
I slept almost uninterrupted but had intense and annoying dreams, so much that I made myself wake up. I still feel confused and sleepy. [...] I'm not sure what triggered those dreams. [...] But more than 2h REM sleep - yes I dreamt a lot. [...] The REM sleep later on in the night makes sense. I feel like I dreamt for hours. [...] (21/7 - 7:32) (Figure 5)
I think I slept ok until I started having a terrible nightmare that I was told my cousin disappeared—I tried to find her and found out she was murdered but was trying to avoid seeing the images in my dream. The alarm was a relief but then I spent searching for her online. [...] Very little deep sleep but a lot of REM sleep makes sense. Those dreams! Quite distressing actually. (22/7 - 8:12) (Figure 6)
I had a lot of REM sleep that's good. My mind felt a bit too active at night. [...] REM also makes sense as it felt quite a deep sleep but active in my mind. (8/8 - 9:10) (Figure 7)
The data revealed that the participant-researcher paid most attention to two scores from the Oura app: REM Sleep in relation to dreams and mental health and Deep Sleep in relation to restlessness and physical health.
Finally it rained and was a bit cooler tonight. [...] Readiness is good again, up to 85. [...] Sleep was good - 82. Sleep duration 6:10 is on the low side. [...] Deep sleep is rising again—that's how it felt. I think It's for body repair. Low average heart rate again—I wonder why. HRV is high too. I remember a time where I only ever had 15 max. I see how I had more deep and less REM sleep than usual - sleep felt deep and calm without interruption. Even restfulness was good, usually this is where I struggle. (26/7- 6:45) (Figure 8)
It's a bit colder tonight. My readiness is great, 92. Maybe that gives me energy for the day. Sleep is good too, 86. [...] Lowest heart rate is interesting for me, it seems good. Maybe I'm improving? REM also makes sense as it felt quite a deep sleep but active in my mind. HRV is rising, that must be good. [...] (8/8 - 9:10) (Figure 7B)
My Readiness is good 88: optimal HRV balance let's see what that means. Enough recovery time—23 is a high average for me I think. Body-temp. -0.2C did I finally get over all the COVID? Also the Heart Rate is lowest in a while with 63. Sleep score is not as high as I would have thought—but I had a lot of REM sleep which probably correlates with my many dreams. It says Deep Sleep is low but 1 h seems good to me. (20/7 - 8:30) (Figure 4B)
The beliefs Anna held about certain aspects of her sleep data and their meaning (despite low accuracy of sleep phase detection from wearable devices as we will present in the Discussion) aided her sense-making between the felt sleep perception and that of the Oura app.
Based on an accumulation of experiences with various patterns in sleep scores and data Anna held a belief about her personal baselines, even if they contradicted an interpretation from Oura: for Anna one hour of Deep Sleep was long, despite Oura considering it to be low. Similarly, Anna described that she considered a resting heart rate below 70 and HRV over 20 to be above her personal average and thus to be good, even if Oura suggests that these values would be better lower for RHR and higher for HRV. In those cases Anna rejected Oura's interpretation, overriding it with her own beliefs.
I don't feel as fresh yet, but it's good to see my heart-rate lowered to 65, for me that's as low as it gets. Seems I recovered well. I also had enough Deep Sleep. The HRV is surprisingly high too with 54! (18/7 - 8:45) (Figure 2)
Over an hour of deep sleep feels good to me despite the app. (19/7 - 7:50) (Figure 9)
I'm surprised it says I fell into Deep Sleep so quickly - it feels ages until I fell asleep. Was I sleeping on my arm and stopped the blood flow? My lowest heart rate and average are really low (60/66). I've never seen that. What does that mean? HRV seems good too. Maybe I'm better than I feel. (25/7 - 6:28) (Figure 10)
3.1.3 Theme 3: Contradicting information from the app leads to frustration
Whenever Oura pointed out specific events in the sleep scores, describing them in words with positive or negative connotation that were not coherent with each other, or that contradicted the participant-researcher's real experience, Anna described feelings of confusion or annoyance.
In one instance the app presented Anna with her “Ouraview”—a meta-overview of personal statistics since using the Oura ring (Figure 11). The app highlighted the longest night's sleep ever recorded on 10 May 2022. It showed how Anna had slept for 13 h and 49 min and stated “Oh what a night... Think back to that evening. Whatever you did, it worked! Bottle that sleep magic.” (27/7 - 8:15) However, Anna annotated that:
Ouraview shows me the longest night, but that night I had COVID so it wasn't that great. (27/7 - 8:15)
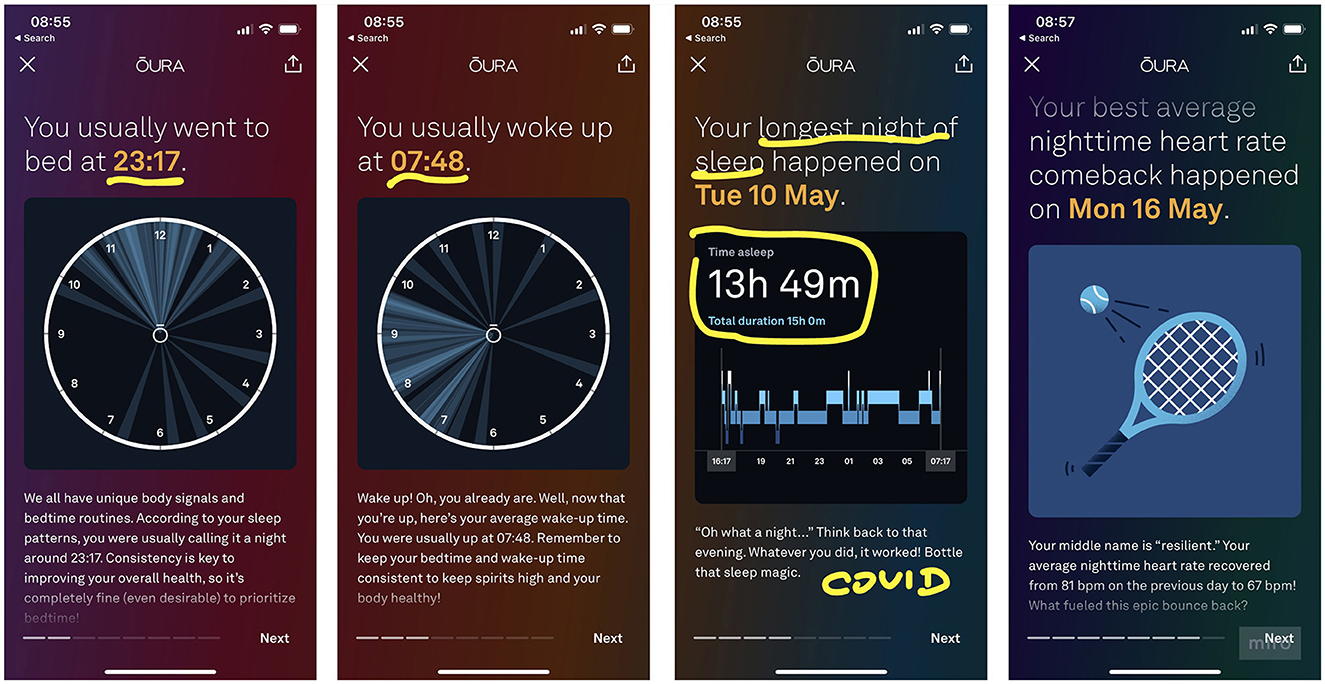
Figure 11. The “Ouraview” of my sleep since starting to use the device in 2021, presenting me with all-time averages and highlights.
In another instance, as presented in Figures 3B, 12B, the tracker told Anna to watch out because her Readiness score was low at 75. The text read “Not all nights are perfect. Something disturbed your sleep last night, but don't worry, you'll get back on track. Switch to lighter activities today, and try do something relaxing in the evening.” (13/8 - 8:36) Anna described how this information had primed her for the day and made her think that she needed to rest and to cut down on her activities:
Readiness and Sleep [scores] are lower than I expected. I was very restless at night. It suggests taking care of my body can work wonders - that's probably true. I don't do that much rest days. My Deep Sleep is very low. Maybe I go back to bed after this. I'm a bit surprised. (13/8 - 8:36)
The following day, however, her Readiness score was even lower (72), but the words from the app much more positive “Looking good. Your readiness is at a nice level, what's your plan for today? To boost your mood during the day, can you take time for something special that makes you smile?” (14/8 - 10:22) This left her confused:
My Readiness is low but the app says “looking good”. That's strange as the score was lower yesterday and said it was bad. (14/8 - 10:22)
We found that with increasing experience of using the Oura ring the participant-researcher learned to interpret and make sense of her data herself, not having to rely on Oura's verbal interpretations of it—particularly in cases where Oura is missing contextual information.
3.1.4 Theme 4: sleep-trackers compete for attention with other social agents
For the participant-researcher Anna social commitments played a big role in how she organized her sleep time and duration during the data collection period. From the notes we found that they also had an effect on perceived sleep quality and on on how she felt the next day, physically and/or mentally. Events in the evening involving other people tended to delay the time Anna went bed, while she interpreted that eating late, large dinners or drinking alcohol negatively impacted the Sleep and Readiness scores:
I didn't sleep much on Friday and not so well on Saturday. Today my [Sleep] score is kind of low too (76). Weekends are bad for sleep if you go out and try to be active. Overall the sleep factors don't even seem so bad. (25/7 - 6:28) (Figure 10B)
As a social agent itself, the sleep-tracker posed its own demands on when Anna should have been going to sleep by sending bed-time reminders or drawing attention to sleep data that could be improved by adjusting her sleep patterns. This led to conflicts between social commitments to other people vs. the Oura ring.
Restorative time per day is very low. I need more. I go to sleep a bit late these days - need to go earlier but it's summer and I see more friends. (8/8 - 9:10) (Figure 7B)
The presence of other people or pets in the same home where Anna was sleeping also had an impact on sleep duration, sleep onset and sleep quality. For example in the mornings, a shared breakfast with others was often mentioned as the main reason for getting up, even if she did not feel rested enough:
Sleep was good - 82. Sleep duration 6:10 [hours] is on the low side. Maybe I need more but I'm getting breakfast. (26/7 - 6:45) (Figure 8)
Anna also sometimes felt stress due to social commitments, which negatively affected her sleep perception:
It was the first night this week I could sleep longer in the morning, but we stayed up and out very late. [...] Yesterday my body felt already very tired and despite not wanting to stay out late I did and had a few drinks (with alcohol). My friend Z is also staying with me, so waking up feels a bit stressful, I need to make breakfast. (6/8 - 10:25) (Figure 13)
In return, sleep data alters how the participant-researcher perceived the time she spent with friends - despite feeling relaxed after a night out or a weekend with friends, if the scores told otherwise it created a conflict in what Anna wanted to do compared to what Oura told her would be better to do.
3.2 A diffractive reading of entangled sleep data
In this section we undertake a diffractive reading of the data set, including the positionality statement, the findings from thematic analysis and the overall methodology as an alternative way to gather further insights in how sleep-trackers and sleeping interact. Diffractive reading recognizes how the apparatus (in this article: the autoethnographic methodology including body-mapping activities and sleep-tracking technology as well as the thematic analysis of the results) is “part of the making of boundaries and distinctions that we as researchers apply in our empirical descriptions” (Barad, 2007). The goal of this analysis was to learn more about what does or does not ripple through the apparatus, to better understand the phenomena being studied and the apparatus itself. We organized the findings into several readings, presented in the following subsections.
3.2.1 What passes through the apparatus: making feelings and emotions visible
Diffractive reading can be used to examine the apparatus itself, the instruments through which the data, which is the basis for our analysis, passes. In our case the apparatus was primarily the Oura ring, its companion app and mobile phone; but also the folder for the diary study involving sheets of paper with body maps and questionnaires, felt-tip marker and a pen; a laptop used to collect, transcribe and analyze the data; Miro, a digital whiteboard that was used to organize and annotate the data; the thematic analysis (Braun and Clarke, 2012) and finally the diffractive analysis itself. Data passed through different tools, in different formats and mediums until it made its way into this paper.
Barad (2007) suggests that the apparatus used plays a role in determining what is visible and what is not. During this study, the Oura ring measured bio-signals day and night and interpreted the subject's sleep quality based on the data it captured and the normative ideals of sleep for the subject's age and sex reference group, which are encoded in its algorithms. It made visible the heart-rate, the time Anna spent in bed, the time she was asleep, restlessness (and moving), variations in her body-temperature, her blood-oxygen saturation and various combinations of those data-points to give a detailed, numerical overview of the participant-researcher's sleep. Not all internal workings of algorithms calculating the scores are known to us. The app curated an overview of each nights' sleep, presenting it as percentages, numbers, timings, degrees, trends—just enough to be comprehensible and that they could be learned over time. The raw data generated each second the ring was worn would be too overwhelming for a single user to analyze manually. Pre-coded text in the app provided a verbal interpretation of sleep data. It presented signals about the sleeping body that were not directly observable back to the subject, and used various functions of behavior change to try and support better sleep or better productivity during the day. It made conclusions about Anna's sleep merely on the signals her body sent and that it was able to pick up.
The app offers the option to add tags to describe certain external factors that might have an impact on sleep (e.g., Caffeine, Hot shower, Late meal, Nightmares, Supplements, Wine etc.) which allow to contextualize sleep. We did not make use of this feature during this study. Upon a closer look at this feature we identified that more than half of the tags are behavior-related and thus offer themselves for behavior-change interventions as opposed to recognizing the external influences on sleep that are out of individual control. Overall, in its interpretations and recommendations, the app zoomed in on Anna's body and behaviors and provided remarks to help her establish a better sleep hygiene. It made her the sole responsible of her own sleep, putting it into her hands to improve.
The diary study including body maps and a questionnaire required active input from the participant-researcher's side. Each morning she started by taking a few minutes to perform a body scan to notice how she felt and to reflect on her night's sleep. Then she turned to the page and took note of the date and time of the observation, and then she made use of the available colors (green, yellow, orange, red, pink, and blue) to try and visualize her feelings and emotions, and how they manifest in her body, by drawing them onto the body maps. Red and orange were mostly used in association with heat, or pain and inflammation. Green and yellow were often linked to ‘sour' or burning feelings in the stomach such as after eating too heavy at night. Blue was used either in connection with feeling confused or concerned, on days with longer REM sleep as well as any physical sensations relating to breathing or fresh/cold air by marking areas around the chest. A mix of colors outside the outlines of the body map were used to elicit a busy mind, excitement and high energy. Most of the shapes Anna drew were abstract, but sometimes they were more defined to represent something or someone directly such as the flow of air, the paws of a cat or stinging pain from nettles. Heavy or big feelings were often represented in geometrical shapes, such as a ball around the head, a rectangle on the shoulders or triangles over painful hands.
For the thematic analysis we read and re-read the data multiple times. Using Miro as a digital whiteboard we made use of digital post-it notes, sticky dots and color-coding to interpret the data and find patterns. At some point we had to move from working on our computers to taking notes on paper as the screens were too small to look at all the different points at once. We looked for instances where subjective experience and Oura's interpretation matched and for instances where they did not and then drew conclusions on what that meant for her relationship with her sleep-tracker. Our shared experience having done user-research for sleep-tracking technologies before influenced what we found interesting to look at and guided us on where to look closer. Since this is an autoethnographic study, those themes might not be applicable to other users but some of the observations, such as that good sleep scores motivated Anna for the day (Theme 1), or that sleep-trackers compete for attention with other social relationships (Theme 4) echo things that users had told us in previous studies.
Theme 4 offered itself for an explanation of the boundary-making apparatus at hand. In Theme 4 we argued that sleep-trackers compete for attention with other social agents. In one example that presented this pattern, on Monday, 25 July 2022, Anna woke up feeling stressed and not well rested. She drew a cross-eyed face, noting that it was difficult to focus. Her stomach felt heavy from the food she ate at the weekend, which she drew as an orange ball in the center of the figure, and her skin felt good from the sun, which she noted down as yellow streaks along the outside of the arms and legs on the map (Figure 10). Anna recounted, that on this morning she woke up at home early (6:25, as the time on her phone told her) to do some work after a weekend she spent with her partner in a different city. On Friday night they went out late, saw an exhibition, had dinner and spent time with friends. They could not sleep very long on Saturday morning because the room got heated up by the sun very early. On Sunday they spent the day by a lake and in the evening Anna drove back home, for over two hours, keeping herself alert by drinking Red Bull and going to bed at 23:28 (as the Oura app recorded). Oura did not know any of these specific and personal circumstances and suggested that Anna was doing well with a sleep score of 76 (which for Anna was lower than average), a lower than average heart rate (which is considered good) and enough restorative sleep; but it also made the remark that Anna should “prioritize sleep this week. Your average sleep score for last week is 74, which is on the low side. No worries, weeks can vary when it comes to sleep quality. To stop the downward trend, pay attention to your sleep schedule this week.” To which Anna commented on in a diary entry:
I didn't sleep much on Friday and not so well on Saturday. Today my [sleep] score is kind of low too (76). Weekends are bad for sleep if you go out and try to be active. Overall the sleep factors don't even seem so bad. (25/7 - 6:28)
Anna rationalized her bad sleep score by relating it back to the facts that she had spent the weekend being active, doing things she liked with her partner and friends. We found a few similar examples where Oura suggested alternative behaviors than Anna's schedule involving other people had catered for. During the thematic analysis we identified those occurrences as a theme because we were already aware of this behavior from previous research, where other users of sleep-trackers expressed that they would listen to their tracker more than they listened to their friends.
3.2.2 Sleep is entangled with life
Reading sleep data provided by the Oura ring through body maps and notes not only made visible what passes through one or the other as illustrated above, but it provided insights into the entanglement of sleep with the sleep-tracker, other agents and the environment. From analysing the different data-points on the self-assessment of sleep it emerged that Anna had never thought about her own sleep without also thinking about the social context she was in, her mental and physical health, the weather and temperature, work and time off as a PhD student, time constraints, emotions and desires among others. The apparatus we had built for this study, an autoethnographic diary study involving body maps and note-taking alongside the automatic sleep-tracking with the Oura ring, therefore made visible how entangled the sleeping bodymind is with the world around, something the sleep-tracker alone did not capture. In this second diffractive reading we paid attention to the intra-action of different human and non-human agencies in the co-constitutive production of the matter and meaning of sleep.
The body maps represented feelings and sensations on the body, and the words used to annotate the maps often included external factors that had caused those feelings manifesting in the body. The body maps together with the diary entries made visible how sleep is relational, situated and cannot be separated from its context. The external factors Anna referred to when reflecting on her sleep quality in the diary can be grouped into social, environmental, physiological and technological. Compared to the sleep data from the Oura ring, the body maps elicited an interpretation of sleep through its context and the lived experience.
Noise, for example, is a category that always emanated from external sources, permeating the sense of hearing during sleep, sleep onset or waking up, leaving a mark on how sleep had been perceived. This auditory stimulation was often the first or last thing Anna sensed around her sleep and thus was often represented in the body maps. Other people (or pets) in the house made noise which affected sleep:
My mum woke me up at some stage when she went to the bathroom. (19/7 - 7:50)
My alarm rings at 6am to feed Oskar [the kitten]. He was already screaming. (27/7 - 8:15) (Figure 14)
My mum listened to an opera next door [last night], that was nice. (26/7 - 6:45)
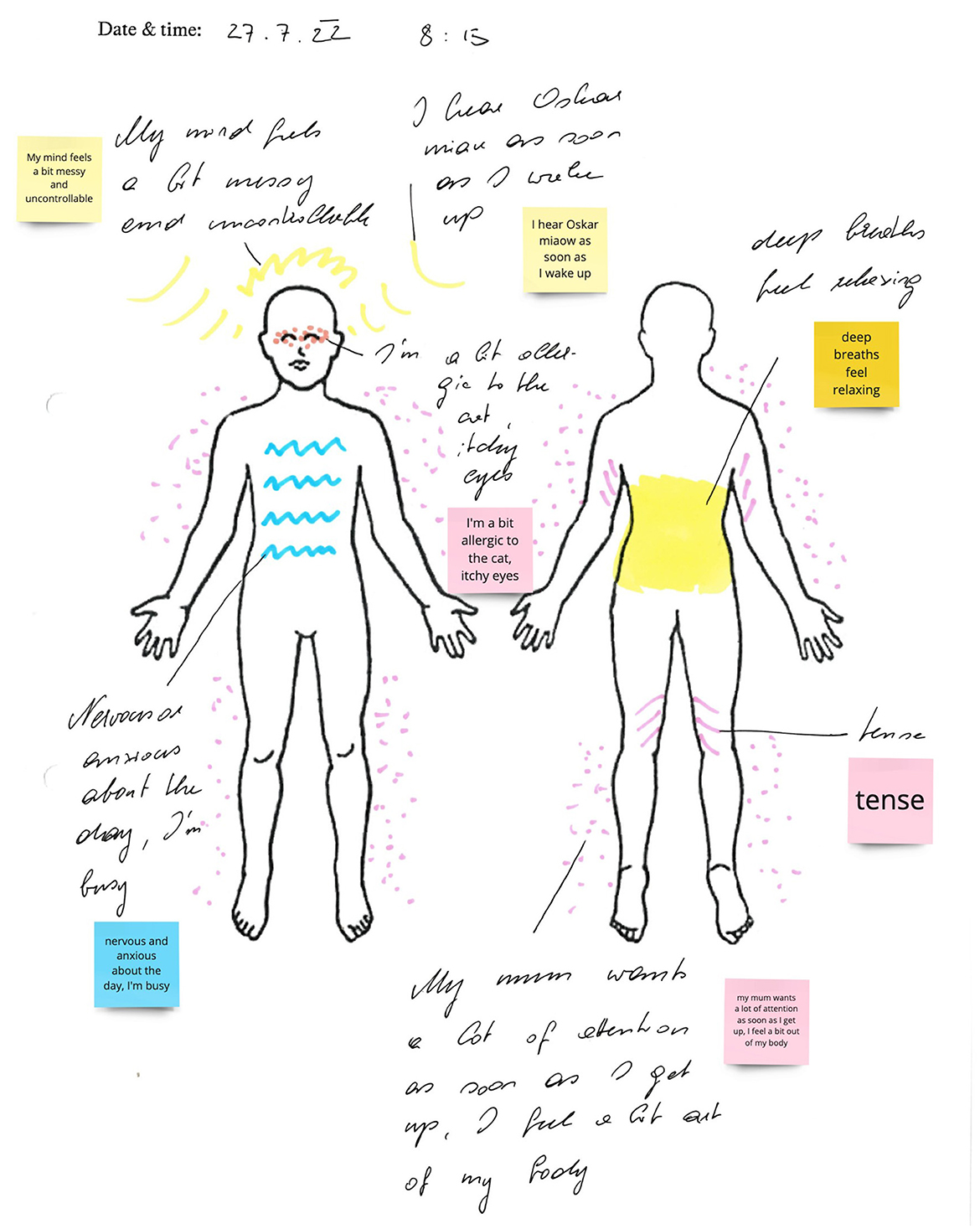
Figure 14. Body map on 27 July 2022, 8:15. The battery of my ring was not charged and thus the data from Oura is missing for that day.
Street-noise was also a factor that woke Anna up on few occasions, when she stayed in a city:
It was hot and a bit loud in the room. [...] In the morning the street got super busy. It woke me up. (14/8 - 10:22)
In terms of social factors, the example we discussed in chapter 3.2.1 elicited, how spending time with friends and family was one of the main factors in how Anna organized her time during this period of summer, often to the disappointment of the Oura ring. But also merely the presence of other people or pets caused stress through their perceived demands for attention:
Knowing this little cat was next door I woke up excited and eager to get up. (26/7 - 6:45) (Figure 8A)
Restorative time per day is very low. I need more. I go to sleep a bit late these days - need to go earlier but it's summer and I see more friends. (8/8 - 9:10) (Figure 7)
Work is another social context that impacted Anna's sleep. During the observation Anna felt torn between working on her PhD research and working on the farm. On various occasions she described feeling stressed. This often also manifested as a tense feeling in the body such as clenching her jaw or fidgeting with the feet (i.e., Figures 8A, 9A, 13A and 14). The stress of feeling like she had to do her own work but also the physical strain of doing physical work affected her body, her sleep and her sleep data differently:
I worked until late last night. Because of the heat I find the days difficult to work in. I was a bit stressed by all the things I have to do. Then I don't want to sleep. (22/7 - 8:12)
Before sleeping [...] I felt stressed because I wanted to work at night but didn't really. (28/7 - 8:02)
I couldn't fall asleep for long—felt quite preoccupied about all I have to do this week and also a bit sad. I remember rolling around long before falling asleep. I was hot and then cold. [...] I have to be up early but drove long last evening. [...] I had a Red Bull before driving, maybe that kept me up. I feel stressed because I didn't work at the weekend and have to go in the forest this morning. (25/7 - 6:28)
The data collection took place during a particularly hot period of summer and high night-time temperatures affected Anna's sleep quality and quantity. The duration Anna spent outside during the days also impacted the sleep experience. When reporting about hot weather or temperatures in the room Anna often associated them with feeling less recovered, which was not necessarily reflected in the sleep-scores. We found that slight drop in temperature however did have positive effects on both sleep perception and scores.
Physical pain or discomfort affected the restlessness during the night. Physical, straining work sometimes caused pain to Anna's body which she experienced as impacting her sleep, which Oura often recorded as restlessness during the night.
The researcher-participant's period was another physiological factor that had an effect on how she perceived her sleep. We did not find this effect to be represented in any change of sleep scores and data:
I'm not sure I dreamt but felt a bit sad before sleeping—I'm getting my period + hangover.(18/7 - 8:45) (Figure 2)
For privacy-concerns about personal health data Anna chose not to use the inbuilt Oura period tracker—thus we cannot know how this might have affected the scores differently.
The Oura app had a narrow focus of its sleep interpretation, on the body and behavior, suggesting personal responsibility to sleep better by making behavior-changes like taking it easy or going to sleep earlier to make up for lost sleep. While Anna considered herself to have been in the privileged position that she might have had the freedom to make such choices in the moment of the study—since it was technically a summer-break—these recommendations made her think of people who do not have this freedom, for example due to caring responsibilities, shift work or other economic dependencies. This reproduced findings of and resonated with other digital health research, suggesting that biodata-tracking technologies work to promote disciplinary self-surveillance practices and neoliberal notions of the responsible, self-optimizing citizen (Foucault, 1988; Lupton, 2014; Ruckenstein, 2014; Fotopoulou and O'Riordan, 2017; Sanders, 2017).
4 Discussion
In our autoethnographic study we set out to deepen the understanding of the relationship between sleep, the sleeper and wearable sleep-trackers in terms of the context of personal sleep and the data a sleep-tracker produces. Insights into these relationships raise a series of implications for the design of sleep-tracking technology.
We used body maps and diary prompts to explore the impact of sleep-tracking technology on the participant-researcher's own sleep experiences. After a thematic analysis we also conducted a diffractive analysis, focusing on the entanglement of sleep-tracking practices and the researcher's sleep. The study set out to generate a rich understanding of personal experiences with sleep-tracking technology, rather than producing generalizable results. Four themes about the user's relationship with her sleep-tracker emerged from the analysis: (1) The user finds motivation in seeing high sleep scores on the app, even if she does not feel refreshed; the scores give her confidence for the day ahead. (2) The user examines specific data points to make sense of her felt sleep experience; she finds correlations between feeling recovered and long deep sleep phases or between vivid dreams/a busy mind and long REM sleep phases. (3) When the app provides contradictory descriptions or interpretations of sleep events that do not align with the user's experience, it causes confusion and annoyance. Finally, (4) social commitments and events influence the user's sleep time and quality; the sleep-tracker's reminders and recommendations can conflict with those social commitments, creating a tension between meeting Oura's sleep goals and fulfilling social obligations.
The diffractive reading of the data allowed us to present an alternative to representationalism. Rather than accepting data as separate from the act of measuring we were interested in how data is produced through the act of measuring and thus entangled with the instrument and the world. The data we collect and make visible in this report exists at the boundary, where the apparatus and the rest of the world meet (Barad, 2007). We first looked at the research apparatus (the body maps, diary entries and Oura app) and paid attention to how information passes through each instrument. In a second reading we looked closer at what impacts how sleep is perceived and conceptualized, discovering a range of factors manifesting in the researcher-participant's waking body and turning the focus from the personal and behavioral, which sleep-trackers are built on, to the context of sleep in the world. Our knowledge-making practice becomes part of the phenomena of sleep that we describe. Sleep data influences how sleep is perceived in hindsight.
Our findings are consistent with those of the case study by Edinger and Krystal (2003), which describes subjective insomnia or sleep state misperception, clinical cases of patients who report not being able to sleep but showing “normal” results of a sleeping person in polysomnography. In one case the patient, after attending a sleep lab and discussing the results that according to the lab she had a good night of sleep, said: “I don't really care what your [sleep] machine says. All I know is that I used to be able to sleep,... I know what that's like,... and I am not doing it now.” This closely matches experiences where the participant-researcher woke up, not feeling fresh often due to period pain or physical exhaustion, but being met with good sleep scores (see Theme 1). While the scores were motivating for the day ahead, making her feel slightly better, it does not alter the overall perception of how the night's sleep went.
Our findings are also supported by Viseu and Suchman (2010), who argue that the representation of biodata, and thus sleep, in numbers and words promotes a division of body and mind and establishes a data double that is at risk to be understood as objective information only. In Theme 2 we describe scenarios where the user over time learns to make sense of her data. Specifically, the participant-researcher reflected on REM sleep corresponding to nights with vivid dreams and Deep sleep being linked to physical restoration, as if this data was taken at face value. According to several studies (Liang and Chapa Martell, 2018; De Zambotti et al., 2023) sleep phase detection in wearable sleep-tracking is not accurate. The data on sleep phases does not necessarily confirm whether or not a user did indeed have a lot of REM or Deep sleep. Despite the participant-researcher's awareness of this issue and lack of accuracy she repeatedly considered those sleep phases as important to her data interpretation. In a previous study the participant-researcher analyzed blog posts, newspaper and magazine articles on wearable sleep-tracking. Upon reflection, the discourse on wearable-sleep-trackers as presented in popular media and the daily comments from the Oura app influenced the believes the first author of this paper held about the importance of sleep phases. Despite the inaccuracy of sleep phase detection, Deep sleep and REM sleep phases oftentimes matched with the felt experience of the paricipant's sleep.
By tracking her sleep for this study, but also by tracking her sleep with the Oura ring in the year before this study, the researcher-participant was not trying to improve or optimize her sleep, but merely to track and monitor it and to spot anomalies. The tracker raised the user's awareness of her own biodata and sleep behaviors, however, information on sleep phases per se or detailed data overall had limited usefulness for the participant-researcher. As discussed in the findings in Theme 3, over time the participant-researcher made sense of the data and then lost interest in the language description of the app. The Oura app taught her over several months how to read and interpret data herself in conjunction with how she felt.
The results of our study support the idea sleep is much less normative and “natural” compared to what a popular discourse suggests, but it is social and cultural. We as humans need sleep biologically, but how much and when is constructed by the sociocultural frameworks each person lives within, and there is considerable variability between these. The normative ideals encoded in the Oura ring are at best motivational if we stay within the Euro-American analytic mode of comparison. Sleep-trackers are enrolled in a neoliberal discourse of self-surveillance to maximize capitalistic extraction and they put sleep to work for increased productivity. Rather than alleviating the issues at the core of the so-called sleep crisis in the West, which is chronic emotional stress (Crary, 2014), they add to it by causing more stress. Our findings support and extend Baron et al. (2017)'s cases of orthosomnia where users of devices present their doctors with a form of sleeplessness caused by anxiety around not sleeping well enough or not being able to meet good enough sleep scores. Our study shows less a lack of sleep induced by the sleep-tracker but rather added stress in negotiating sleep and societal commitments (Theme 4). While the first author conducted the study during the summer with mostly her own choice of how to plan her time, the personal circumstances of shift-workers or carers who do not have a choice in changing their sleep schedules and their sleep become even more problematized. Findings from this study about negotiating what one wants to do or has to do, and what the tracker suggests doing, match findings from our previous study, where several users of wearable sleep-tracking devices have mentioned they prioritized good sleep over socializing (Nagele et al., 2022).
With this study we show an example of a sleep-tracking apparatus that moves beyond existing practices of sleep data measurement and representation, indebted to historical ways of inscribing the human body through scientific frameworks. The study challenges the notion that the sleeping body can be reduced to bio-signals and opens up opportunities for the design of sleep-trackers to engage with bodies from a holistic perspective including mind, body, emotions and values. As suggested by Tsaknaki et al. (2022), the body-scanning and body-mapping activity each morning during the study period was an attempt to turn abstract biodata into a material form that can be experienced with multiple senses, creating new meaning to support embodied understanding of biodata. Illustrating the felt experience of ones sleep on the body maps was an attempt to visualize the embodied sensations of sleep without categorisation, attending to and making visible the richness and variety of lived, and thus slept, experiences. It might be a first step to working with biodata for sleep beyond imposing normative categories as Sanches et al. (2022) suggests.
At the start of this paper we argue that body-mapping is a suitable methodology to explore and describe the experience of sleep other than in numbers and words and yet we focus a lot on numbers and words for analysis and description of the data. The patterns we found within the drawings did not produce meaningful insights beyond for example heat and inflammation represented with red colors, blue with cold and air etc., which might be learned associations that could be different for each person conducting the same study. We found that at the beginning of the study the participant-researcher colored the body maps more within the outlines and as the time went on and the month passed, at the end the outlines were less important and colorful shapes were drawn on top of the bodies. A longer duration of the study might have resulted in more meaningful interpretations of the drawings themselves.
4.1 Implications for the design of wearable sleep-trackers
We intend that the results of this autoethnography add to a canon of sleep research recommending a move away from representing sleep in terms of comparison and competition, uncoupling it from neoliberal capitalistic productivity and self-improvement narratives. We suggest moving beyond existing practices of sleep data measurement and representation, indebted to historical ways of inscribing the human body through scientific frameworks. The study challenges the notion that the sleeping body can be reduced to bio-signals and opens up opportunities for the design of sleep-trackers to engage with bodies from a holistic perspective including mind, body, emotions and values. We briefly outline some concrete design recommendations along these lines.
We found several instances where the verbal descriptions of data by the Oura app contradicted what had happened in reality. While those verbal interpretations can support learning of data we recommend that after a period of on-boarding the app focuses more on aiding subjective and personal interpretations rather than making conclusions.
Since sleep-phase detection does not accurately represent sleep but was considered important by the participant we want to introduce the idea that instead of sticking to sleep-medical terms such as REM and deep sleep, the app could allow users to label their data with their own words following the felt experience of sleep.
The numerical data from the app supported the analysis of sleep alongside the subjectively reported experience. However, as we saw at several points in the discussion, the data at times felt too prescriptive and judgemental. Future work might engage more with the idea of mediating internal and external factors' contribution to sleep (beyond activity), representing them other than in scales, binaries and competition with the self or others.
Abstract biodata might for example be turned into a material form that can be experienced with multiple senses, creating new meaning to support embodied understanding of biodata. The body maps presented in this study visualize the embodied sensations of sleep without categorisation, attending to and making visible the richness and variety of lived, and thus slept, experiences. Exploring embodied visualizations for data-representation might be a first step to working with biodata for sleep beyond imposing normative categories as Sanches et al. (2022) suggests.
Finally, we accept the social agency a sleep-tracker holds and our study exemplifies this in situations where the user has to negotiate between the sleep-tracker's and other societal demands in regards to her sleep pattern and duration. Allowing a user to reflect and decide on the social role and level of agency a sleep-tracker should take in their life could give agency back to the user and could personalize the approach to sleep-tracking.
4.2 Recommendations for future research
Sleep-tracking technology can cause stress for its user, either by creating health concerns, supporting unhealthy, obsessive behavior (Baron et al., 2017) or by adding pressure to negotiate the sleep schedule between ones sleep data and other social commitments (Theme 4). A move away from this narrative around self-improvement or analytical mode of comparison inherent to the design of consumer-grade wearable sleep-trackers could mean to consider sleep data as a tool for social justice by making inequalities and extractive systems visible and empowering people to rest, pushing back against extractive capitalism (Hersey, 2022).
An area we have not addressed here is dream research. Dreams play an integral part in how a night's sleep is subjectively experienced and perceived. A split in sleep- and dream research occurred with the medicalization of sleep and the properties of dreams that do not allow measurement with any medical or technological apparatus. While the REM sleep stage is associated with the phase of sleep in which most dreams occur, interpretation does not go beyond that. Dream recall relies on the dreamer to remember the dream and to record it before it slips memory. In the study at hand, dreams are often referred to and attempted to be found in the data; and we found that vivid dreams often coincide with long REM phases. Dreams, REM and the subjective feeling when waking up are closely correlated and meaningful. Similar findings emerged in another study by the same authors, where interviewees and users of sleep-tracking technologies referred to an interest in trying to spot the moment at night where they are dreaming. Future studies or applications of sleep-tracking technologies could look at dreams and the role they play in sleep quality and quantity; another opportunity could be to use sleep data to support dream recall and dream-tracking technologies as previously suggested by Hoefer et al. (2022).
In this study we also find that the interpretations of sleep data by the app become redundant over time (Theme 2). The user learns where her experience matches the data and makes the interpretation herself, such as one hour of deep sleep being enough, despite the app suggesting that this is on the low side. While at the beginning those texts were helpful to learn from, after a while they can even lead to contradictions and frustration (Theme 3). This raises the question whether accuracy is overrated. In a sentiment analysis as part of the discourse analysis in our previous study, accuracy is the most sought-after feature of sleep-tracking technology, expressed from users, experts, the media and even academic research. Whether or not the data is accurate might, however, be secondary for an end user once the meaning of one's data compared to one's experience has been established. This might also be a way to address situations such as the aforementioned sleep stage misperception (Edinger and Krystal, 2003). Future research could investigate the quest for accuracy in sleep-tracking outside of medical sleep research, and what benefits moving away from it could bring to new technologies.
4.3 Limitations
Our study had several limitations in terms of being unrepresentative of marginalized populations. Bad sleep is often linked to behavior resulting from chronic emotional stress caused by socioeconomic pressures such as working irregular schedules or less access to healthcare (Crary, 2014). The participant-researcher herself does have access to healthcare and is in a financially secure position. We furthermore chose to conduct this study outside of a regular work schedule during the summer. This choice was made to secure the experience of a wide range of situations and external factors that might impact sleep rather than with a more regulated daily schedule during the university term-time. While the analysis made the researchers think about those who do not have comparable privilege and flexibility for their sleep, this study does not do the experience of marginalized sleepers justice.
For personal and political reasons, in the context of the overturn of the Roe vs. Wade decision by the U.S. Supreme Court only weeks before conducting this study (Weitz, 2022), the participant-researcher chose to turn off automatic period tracking in the Oura app due to privacy concerns. This decision might have had an impact on sleep-data in cases where the participant-researcher discusses the influence of her period on her perceived sleep quality without any noticeable change in the data.
Data availability statement
The original contributions presented in the study are included in the article/Supplementary material, further inquiries can be directed to the corresponding author.
Ethics statement
The studies involving humans were approved by School of Electronic Engineering and Computer Science Peter Landin Building, London, Devolved School Research Ethics Committee; Email: eecs-ethics@qmul.ac.uk. The studies were conducted in accordance with the local legislation and institutional requirements. The participants provided their written informed consent to participate in this study. Written informed consent was obtained from the individual(s) for the publication of any potentially identifiable images or data included in this article.
Author contributions
AN: Conceptualization, Data curation, Formal analysis, Funding acquisition, Investigation, Methodology, Project administration, Visualization, Writing – original draft. JH: Conceptualization, Methodology, Supervision, Validation, Writing – review & editing.
Funding
The author(s) declare financial support was received for the research, authorship, and/or publication of this article. AN's work was funded by the EPSRC and AHRC Centre for Doctoral Training in Media and Arts Technology at Queen Mary University of London [grant number EP/L01632X/1]. JH's work was partly funded by UKRI EPSRC's FLUIDITY project [grant number EP/X009343/1].
Acknowledgments
Thank you to Zara Dinnen for supervising this research project and providing continuous feedback and support. Thanks to the Sociability of Sleep research creation project for inviting AN to the Somanambulations 2 Graduate Colloquium in Montreal, 2023, and providing valuable feedback on the findings of this study.
Conflict of interest
The authors declare that the research was conducted in the absence of any commercial or financial relationships that could be construed as a potential conflict of interest.
Publisher's note
All claims expressed in this article are solely those of the authors and do not necessarily represent those of their affiliated organizations, or those of the publisher, the editors and the reviewers. Any product that may be evaluated in this article, or claim that may be made by its manufacturer, is not guaranteed or endorsed by the publisher.
Supplementary material
The Supplementary Material for this article can be found online at: https://www.frontiersin.org/articles/10.3389/fcomp.2024.1258289/full#supplementary-material
Footnotes
2. ^These are two of the three key figures in the Oura app and not independent of each other - Readiness depends on Sleep and Activity scores.
References
Altena, E., Van Der Werf, Y. D., Strijers, R. L. M., and Van Someren, E. J. W. (2008). Sleep loss affects vigilance: effects of chronic insomnia and sleep therapy. J. Sleep Research 17:335–343. doi: 10.1111/j.1365-2869.2008.00671.x
Ayobi, A., Marshall, P., and Cox, A. L. (2016). “Reflections on 5 years of personal informatics: rising concerns and emerging directions,” in Proceedings of the 2016 CHI Conference Extended Abstracts on Human Factors in Computing Systems (San Jose California USA: ACM), 2774–2781.
Barad, K. M. (2007). Meeting the Universe Halfway: Quantum Physics and the Entanglement of Matter and Meaning (Durham: Duke University Press).
Baron, K. G., Abbott, S., Jao, N., Manalo, N., and Mullen, R. (2017). Orthosomnia: are some patients taking the quantified self too far? J. Clini. Sleep Med. 13, 351–354. doi: 10.5664/jcsm.6472
Braun, V., and Clarke, V. (2012). “Thematic analysis,” in APA Handbook of Research Methods in Psychology, Vol 2: Research Designs: Quantitative, Qualitative, Neuropsychological, and Biological, Cooper, H., Camic, P. M., Long, D. L., Panter, A. T., Rindskopf, D., and Sher, K. J., editors (Washington: American Psychological Association), 57–71.
Choe, E. K., Consolvo, S., Watson, N. F., and Kientz, J. A. (2011). “Opportunities for computing technologies to support healthy sleep behaviors,” in Proceedings of the 2011 Annual Conference on Human Factors in Computing Systems - CHI '11 (Vancouver, BC, Canada. ACM Press), 3053.
Cochrane, K. A., Mah, K., Ståhl, A., Núnez-Pacheco, C., Balaam, M., Ahmadpour, N., et al. (2022). “Body maps: a generative tool for soma-based design,” in Sixteenth International Conference on Tangible, Embedded, and Embodied Interaction. (Daejeon: ACM), 1–14.
De Christofaro, D., and Chiodo, S. (2023). Quantified sleep: self-tracking technologies and the reshaping of 21st-century subjectivity. Hist. Soc. Res. 48, 2. doi: 10.12759/hsr.48.2023.21
De Zambotti, M., Cellini, N., Goldstone, A., Colrain, I. M., and Baker, F. C. (2019). Wearable sleep technology in clinical and research settings. Med. Sci. Sports Exerc. 51, 1538–1557. doi: 10.1249/MSS.0000000000001947
De Zambotti, M., Goldstein, C., Cook, J., Menghini, L., Altini, M., Cheng, P., et al. (2023). State of the science and recommendations for using wearable technology in sleep and circadian research. Sleep. 27, 1255–1273. doi: 10.1093/sleep/zsad325
Desjardins, A., Tomico, O., Lucero, A., Cecchinato, M. E., and Neustaedter, C. (2021). Introduction to the Special Issue on First-Person Methods in HCI. ACM Trans. Comput. Hum. Interact. 28, 1–12. doi: 10.1145/3492342
Edinger, J. D., and Krystal, A. D. (2003). Subtyping primary insomnia: Is sleep state misperception a distinct clinical entity? Sleep Med. Rev. 7, 203–214. doi: 10.1053/smrv.2002.0253
Fleck, A. (2023). How Popular Are Sleep Tracking Apps. Available online at: https://www.statista.com/chart/29518/share-of-people-that-say-they-have-used-sleep-monitoring-apps/#:~text=According%20to%20data%20from%20Statista,Sleep%20Cycle%20and%20Sleep%20Time (accessed February 8, 2024).
Ford, E. S., Cunningham, T. J., and Croft, J. B. (2015). Trends in self-reported sleep duration among US adults from 1985 to 2012. Sleep 38, 829–832. doi: 10.5665/sleep.4684
Fotopoulou, A., and O'Riordan, K. (2017). Training to self-care: fitness tracking, biopedagogy and the healthy consumer. Health Sociol. Rev. 26, 54–68. doi: 10.4324/9781315108285-5
Foucault, M. (1988). Technologies of the Self: A Seminar with Michel Foucault. Amherst: University of Massachusetts Press.
Frauenberger, C. (2020). Entanglement HCI the next wave? ACM Trans. Comput. Hum. Interact. 27:1–27. doi: 10.1145/3364998
Hoefer, M. J. D., Schumacher, B. E., and Voida, S. (2022). “Personal Dream Informatics: A Self-Information Systems Model of Dream Engagement,” in CHI Conference on Human Factors in Computing Systems (New Orleans LA USA: ACM), 1–16.
Höök, K., Benford, S., Tennent, P., Tsaknaki, V., Alfaras, M., Avila, J. M., et al. (2021). Unpacking non-dualistic design: the soma design case. ACM Trans. Comput. Hum. Interact. 28, 1–36. doi: 10.1145/3462448
Kroker, K. (2016). The Sleep of Others and the Transformation of Sleep Research. Toronto: University of Toronto Press.
Kronholm, E., Partonen, T., Laatikainen, T., Peltonen, M., Härmä, M., Hublin, C., et al. (2008). Trends in self-reported sleep duration and insomnia-related symptoms in Finland from 1972 to 2005: a comparative review and re-analysis of finnish population samples. J. Sleep Res. 17, 54–62. doi: 10.1111/j.1365-2869.2008.00627.x
Liang, Z., and Chapa Martell, M. A. (2018). Validity of consumer activity wristbands and wearable EEG for measuring overall sleep parameters and sleep structure in free-living conditions. J. Healthc. Inform. Res. 2, 152–178. doi: 10.1007/s41666-018-0013-1
Liang, Z., and Ploderer, B. (2016). “Sleep tracking in the real world: A qualitative study into barriers for improving sleep,” in Proceedings of the 28th Australian Conference on Computer-Human Interaction - OzCHI '16 (Launceston: ACM Press), 537–541.
Liu, W., Ploderer, B., and Hoang, T. (2015). “In bed with technology: challenges and opportunities for sleep tracking,” in Proceedings of the Annual Meeting of the Australian Special Interest Group for Computer Human Interaction on - OzCHI '15 (Parkville, VIC: ACM Press), 142–151.
Lockton, D., Zea-Wolfson, T., Chou, J., Song, Y. A., Ryan, E., and Walsh, C. (2020). “Sleep ecologies: tools for snoozy autoethnography”, in Proceedings of the 2020 ACM Designing Interactive Systems Conference (Eindhoven: ACM), 1579–1591.
Lucero, A., Desjardins, A., Neustaedter, C., Höök, K., Hassenzahl, M., and Cecchinato, M. E. (2019). “A sample of one: first-person research methods in HCI,” in Companion Publication of the 2019 on Designing Interactive Systems Conference 2019 Companion (San Diego: ACM), 385–388.
Lupton, D. (2014). “Self-tracking cultures: towards a sociology of personal informatics,” in Proceedings of the 26th Australian Computer-Human Interaction Conference on Designing Futures: The Future of Design (Sydney: ACM), 77–86.
Mah, K., Loke, L., and Hespanhol, L. (2021). Towards a contemplative research framework for training self-observation in HCI: a study of compassion cultivation. ACM Trans. Comput. Hum. Interact. 28, 1–27. doi: 10.1145/3471932
Nagele, A. N., Hough, J., and Dinnen, Z. (2022). “The Subjectivities of Wearable Sleep-Trackers - A Discourse Analysis,” in CHI Conference on Human Factors in Computing Systems Extended Abstracts (New Orleans LA: ACM), 1–8.
Pan, Q., Brulin, D., and Campo, E. (2020). Current status and future challenges of sleep monitoring systems: systematic review. JMIR Biomed. Eng. 5, e20921. doi: 10.2196/20921
Ravichandran, R., Sien, S.-W., Patel, S. N., Kientz, J. A., and Pina, L. R. (2017). “Making sense of sleep sensors: how sleep sensing technologies support and undermine sleep health,” in Proceedings of the 2017 CHI Conference on Human Factors in Computing Systems (Denver: ACM), 6864–6875.
Reed-Danahay, D. (1997). “Auto/ethnography: rewriting the self and the social,” in Explorations in Anthropology (New York: Berg, Oxford).
Rooksby, J., Rost, M., Morrison, A., and Chalmers, M. (2014). “Personal tracking as lived informatics,” in Proceedings of the SIGCHI Conference on Human Factors in Computing Systems (Toronto: ACM), 1163–1172.
Ruckenstein, M. (2014). Visualized and interacted life: personal analytics and engagements with data doubles. Societies 4, 68–84. doi: 10.3390/soc4010068
Salmela, T., Valtonen, A., and Lupton, D. (2019). The affective circle of harassment and enchantment: reflections on the ŌURA ring as an intimate research device. Qual. Inquiry 25, 260–270. doi: 10.1177/1077800418801376
Sanches, P., Howell, N., Tsaknaki, V., Jenkins, T., and Helms, K. (2022). “Diffraction-in-action: Designerly Explorations of Agential Realism Through Lived Data,” in CHI Conference on Human Factors in Computing Systems (New Orleans: ACM), 1–18.
Sanders, R. (2017). Self-tracking in the Digital era: biopower, patriarchy, and the new biometric body projects. Body & Soc. 23, 36–63. doi: 10.1177/1357034X16660366
Seixas, A., Nunes, J., Airhihenbuwa, C., Williams, N., James, C., Jean-Louis, G., et al. (2015). Linking emotional distress to unhealthy sleep duration: analysis of the 2009 National Health Interview Survey. Neuropsychiat. Dis. Treatm. 11, 2425–2430. doi: 10.2147/NDT.S77909
Shusterman, R. (2006). Thinking through the body, educating for the humanities: a plea for somaesthetics. J. Aesthetic Educ. 40, 1–21. doi: 10.2307/4140215
Stamatakis, K. A., Kaplan, G. A., and Roberts, R. E. (2007). Short sleep duration across income, education, and race/ethnic groups: population prevalence and growing disparities during 34 years of follow-up. Ann. Epidemiol. 17, 948–955. doi: 10.1016/j.annepidem.2007.07.096
Tsaknaki, V., Sanches, P., Jenkins, T., Howell, N., Boer, L., and Bitzouni, A. (2022). “Fabulating biodata futures for living and knowing together,” in Designing Interactive Systems Conference (Virtual Event: ACM), 1878–1892.
Vgontzas, A. N., Lin, H.-M., Papaliaga, M., Calhoun, S., Vela-Bueno, A., Chrousos, G. P., et al. (2008). Short sleep duration and obesity: the role of emotional stress and sleep disturbances. Int. J. Obes. 32, 801–809. doi: 10.1038/ijo.2008.4
Viseu, A., and Suchman, L. (2010). “Wearable augmentations: imaginaries of the informed body,” in Technologized Images, Technologized Bodies: Anthropological Approaches to a New Politics of Vision (New York: Berghahn Books).
Weitz, T. (2022). Roe vWade Overturned: What It Means, What's Next. Washington, DC: American University. Available online at: https://www.american.edu/cas/news/roe-v-wade-overturned-what-it-means-whats-next.cfm (accessed February 8, 2024).
Keywords: sleep, sleep-tracking, autoethnography, personal informatics, design research, biodata, wearable technology, diffraction
Citation: Nagele AN and Hough J (2024) “The sleep data looks way better than I feel.” An autoethnographic account and diffractive reading of sleep-tracking. Front. Comput. Sci. 6:1258289. doi: 10.3389/fcomp.2024.1258289
Received: 13 July 2023; Accepted: 31 January 2024;
Published: 21 February 2024.
Edited by:
Maria Normark, Södertörn University, SwedenReviewed by:
Mattias Rost, University of Gothenburg, SwedenMattias Jacobsson, Södertörn University, Sweden
Copyright © 2024 Nagele and Hough. This is an open-access article distributed under the terms of the Creative Commons Attribution License (CC BY). The use, distribution or reproduction in other forums is permitted, provided the original author(s) and the copyright owner(s) are credited and that the original publication in this journal is cited, in accordance with accepted academic practice. No use, distribution or reproduction is permitted which does not comply with these terms.
*Correspondence: Anna Nolda Nagele, a.n.nagele@qmul.ac.uk