Understanding the Causes and Implications of Endothelial Metabolic Variation in Cardiovascular Disease through Genome-Scale Metabolic Modeling
- 1Center for Systems Biology, University of Iceland, Reykjavik, Iceland
- 2Department of Pharmacology and Toxicology, School of Health Sciences, University of Iceland, Reykjavik, Iceland
- 3Sinopia Biosciences Inc., San Diego, CA, USA
- 4Section for Transfusion Medicine, Capital Region Blood Bank, Rigshospitalet, University of Copenhagen, Copenhagen, Denmark
- 5Department of Biochemistry and Molecular Biology, School of Health Sciences, University of Iceland, Reykjavik, Iceland
High-throughput biochemical profiling has led to a requirement for advanced data interpretation techniques capable of integrating the analysis of gene, protein, and metabolic profiles to shed light on genotype–phenotype relationships. Herein, we consider the current state of knowledge of endothelial cell (EC) metabolism and its connections to cardiovascular disease (CVD) and explore the use of genome-scale metabolic models (GEMs) for integrating metabolic and genomic data. GEMs combine gene expression and metabolic data acting as frameworks for their analysis and, ultimately, afford mechanistic understanding of how genetic variation impacts metabolism. We demonstrate how GEMs can be used to investigate CVD-related genetic variation, drug resistance mechanisms, and novel metabolic pathways in ECs. The application of GEMs in personalized medicine is also highlighted. Particularly, we focus on the potential of GEMs to identify metabolic biomarkers of endothelial dysfunction and to discover methods of stratifying treatments for CVDs based on individual genetic markers. Recent advances in systems biology methodology, and how these methodologies can be applied to understand EC metabolism in both health and disease, are thus highlighted.
Introduction
Cardiovascular disease (CVD) includes acute and chronic conditions, such as stroke and coronary heart disease (1). CVD results in a shortened life span and is the biggest cause of death worldwide (1–3). The endothelium is the single cell layer that lines blood vessels and lymphatic system and its dysfunction contributes to the development of CVD (4, 5). Endothelial cells (ECs) play an important role in controlling vascular tone and by secreting or expressing surface molecules, they ensure appropriate regulation of blood flow, counteracting intravascular activation of platelets, and coagulation (6, 7). Moreover, cardiac ECs have been shown to affect the ventricular myocardium. Thus, the force-frequency response of cardiac muscle in the presence of increased cardiac workload is blunted after damage to the cardiac endothelium (8).
A vascular surface that normally is thromboresistant, anti-inflammatory, vasodilatory, and antiproliferative can turn into a surface that is thrombogenic, proinflammatory, vasoconstricive, and stimulatory of smooth muscle cell proliferation. Often this change is reactive and transient restoring vascular homeostasis. However, in diseases such as atherosclerosis, hypertension, and diabetes mellitus (DM) such changes, known as endothelial dysfunction, may be prolonged and critical for disease progression. The extent of pathological metabolic perturbation is determined by an interaction of lifestyle factors, such as diet and exercise with underlying genetic factors (9–12). Consequently, health-care interventions may be more effective if adapted to an individual.
Metabolic modeling offers insights into cellular metabolism (13). Below, we consider endothelial metabolic alterations, their contribution to endothelial dysfunction, and integrated analysis of this information with genome-scale metabolic models (GEMs) to advance personalized health care.
Endothelial Metabolism
Endothelial cell metabolism has been investigated in multiple contexts including angiogenesis, hypoxia, shear stress, glycemia, and response to perturbations with mediators of vascular health including thrombin, sphingosine-1-phosphate, and more (14–19). The endothelium operates with variable nutrient availability and oxygen partial pressures in a manner that is EC subtype specific (20) and results in altered synergy in the oxidation of its core nutrients glucose, fatty acids, and amino acids (17, 21–23) that are reviewed specifically elsewhere (24, 25) but considered collectively here and illustrated in Figure 1.
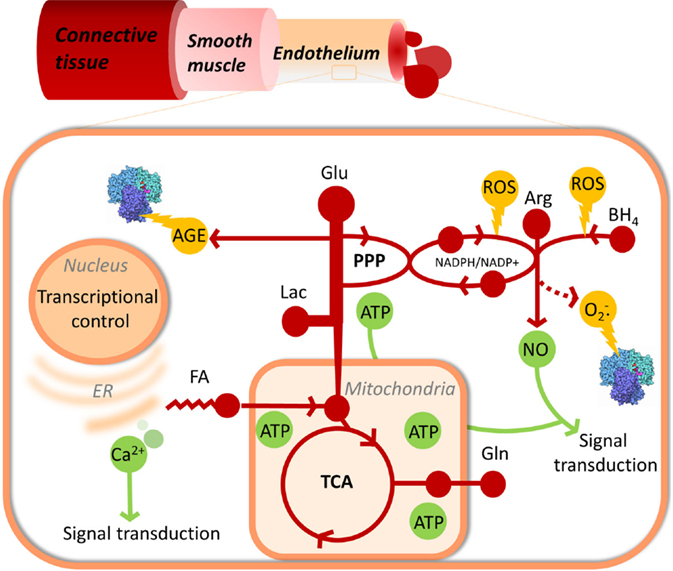
Figure 1. Endothelial metabolism and its links to cellular damage, function, and proliferation control. Metabolism, including glycolysis, pentose phosphate pathway, TCA cycle, fatty acid oxidation, and nitric oxide synthase are represented in red. Useful products of metabolism, NO, ATP, and Ca2+ signaling are shown in green. Damaging side products of metabolism are shown in yellow.
Glycolysis Affects Endothelial Proliferation and Angiogenesis
Endothelial cells oxidize glucose largely by glycolysis, allowing maximal availability of oxygen for transendothelial transport to perivascular cells (26–29). Carbons from glucose are primarily excreted as lactate with only 1 in 200 pyruvate equivalents contributing to oxidative phosphorylation (26). Laminar shear stress, the frictional force created by blood flow, promotes anti-inflammatory, anti-thrombotic, and anti-oxidative properties in ECs and helps to maintain quiescence largely via the transcription factor Kruppel-like factor 2 (30) that acts to repress phosphofructokinase-2/fructose-2,6-bisphosphatase-3 (PFKFB3) thereby promoting a quiescent phenotype (16).
In response to angiogenic factors induced by injury or in pathological conditions such as hypoxia, nutrient deprivation, or tissue damage, ECs quickly form new vasculature by sprouting. During vessel sprouting, glycolysis is increased further, mediated by increased activity of PFKFB3, the loss of which impairs vessel formation (26). Increased glycolysis without oxidation of pyruvate relies on lactate dehydrogenase to supply NAD+, and the activity of PFKFB3 is reflected in both intracellular and secreted lactate of ECs (31). Furthermore, lactate is involved in PFKFB3-mediated endothelial proliferation, tube formation, and Akt activation providing a plausible explanation for PFKFB3-mediated angiogenesis (31). Lactate dehydrogenase activity also increases with EC subtype proliferation rate. In pulmonary microvascular ECs, rapid angiogenesis is dependent on lactate dehydrogenase A expression (14).
Endothelial-dependent vascular function correlates with blood glucose levels (32–37). In hyperglycemia, glyceraldehyde-3-phosphate dehydrogenase is inactivated, impeding glycolysis (38). A build-up of fructose-6-phosphate, a glycolytic intermediate, impacts hexosamine biosynthesis generating N-acetylglucosamine that glycosylates and modifies angiogenic proteins including Notch and vascular endothelial growth factor receptor 2 (39–45) and, inhibits eNOS (46). Excess glucose also enters the polyol pathway, producing excess advanced glycation end products (AGEs) (47, 48). AGEs alter the binding of erythrocytes and platelets to the endothelium (49, 50), and clinical arterial responsiveness correlates negatively with the ratio of AGEs to soluble receptor of AGEs (51).
Fatty Acid and Amino Acids Metabolism
Fatty acid-binding protein 4 (FABP4) is an intracellular fatty acid chaperone protein that impacts the peroxisome proliferator-activated receptor transcription pathway (52). Circulating levels of FABP4 are associated with endothelial dysfunction in DM patients (53) and increased risk of atherosclerosis and cerebrovascular malformations (54, 55).
Fatty acid oxidation (FAO) accounts for roughly 14% of ATP production in cultured EC (22). Carnitine palmitoyl transferase (CPT1A), a long-chain fatty acid shuttle protein regulated by AMP-activated protein kinase, is a key point of FAO regulation (22, 56, 57). Palmitate has been shown to contribute carbons to nucleotide formation via the tricarboxylic acid (TCA) cycle. When CPT1A was knocked down in vitro, vessel sprouting was impaired due to low levels of deoxy ribonucleotides. CPT1A knockdown in mice produced impaired retinal vessel formation (58).
In addition to glucose and fatty acids, amino acids contribute to EC metabolism and function (59). Specifically glutamine fuels anaplerotic reactions via the TCA cycle (23, 29, 60). Internalization of glutamine occurs via solute carrier family 1 member 5 (23, 29), and inhibition of glutaminase causes premature senescence and reduced proliferation in ECs (61). The most intensely investigated amino acid with respect to endothelial dysfunction is, however, arginine in the context of its conversion to the vasorelaxant nitric oxide (NO) by endothelial nitric oxide synthase (eNOS).
Endothelial Nitric Oxide Is Important to Vascular Function and Its Production Is Affected by Genetic and Metabolic Factors
In addition to causing vasorelaxation, NO affects smooth muscle cell proliferation, aggregation and adhesion of platelets and leukocytes, important processes to atherosclerosis and other CVD (62, 63). When eNOS has insufficient arginine, a result of competition with arginase, and/or lacks the cofactor tetrahydrobiopterin, it produces reactive oxygen species (ROS) instead of the products NO and citrulline – in a pathological state known as uncoupling (64–71). Furthermore, the pressure of causes rapid inactivation of endothelium-derived NO (72). Indeed, arginase and eNOS activities and genotypes in addition to tetrahydrobiopterin levels have all been linked to endothelial function (73–76).
Altered NOS activity due to inhibition by asymmetric dimethylarginine (ADMA) encourages NOS uncoupling leading to endothelial dysfunction. ADMA levels, and the ratio of ADMA to arginine, have been connected to several aspects of CVD risk (77–80).
Genetic variation in eNOS affects some measures of recovery of blood flow control in acute myocardial infarction (73). Inhibiting arginase activity, which reduces eNOS uncoupling, is helpful in restoring endothelial function in both coronary artery disease and after ischemia–reperfusion injury (64, 65). Genetic variation in NOS1 has also been linked with CVD in various studies (75, 76). Furthermore, the ROS scavenger methionine sulfoxide reductase A, important to reducing the effect of uncoupled NOS and other ROS, is affected by genetic variation relevant to coronary artery disease risk (81, 82).
Interestingly, the extracellular presence of certain amino acids – ornithine, l-lysine, l-homoarginine, l-glutamine, l-leucine, or l-serine – decreases NO and increases endothelium-dependent vascular resistance. This effect is reversible by adding arginine to the medium and was shown to be dependent on y+L and y+ family amino acid transporters (83).
Decoding Endothelial Metabolism and Function through Computational Modeling
The previous section highlights the complexity of the contribution of metabolism to endothelial dysfunction. Importantly, some of the most common human metabolic gene alterations impact enzymes that are of importance to endothelial metabolic phenotypes. These include pyruvate kinase and (84) glucose-6-phosphate dehydrogenase, which alters CVD risk (85), in addition to those already mentioned above. The variability of the effect of these mutations on cardiovascular phenotypes highlights the problem of untangling complex genetic diseases (12). This complexity is aggravated by lifestyle choices that impact the expression and activity of these genes (9, 86–89). How altered gene expression and the environment combine to advance CVD can, however, be explored on the metabolic level, through metabolic systems analysis using genome-scale models of endothelial metabolism. For CVD research, genome-scale modeling promises to contribute to the definition of endothelial metabolism under different physiological conditions, allow the differentiation of individual endothelial metabolic phenotypes that can be related to CVD states and ultimately contribute to individualized therapy. In the following sections, we explain the concept of GEMs, their current and potential applications toward increasing the understanding of endothelial metabolism, and how this could lead to novel discoveries to combat CVD on the individual level.
GEMs Provide Snapshots of Metabolism
Genome-scale metabolic models are computational models that can be used to describe and investigate the metabolic flux phenotype of a cell based on disparate biochemical information. GEMs are built from biochemical component knowledgebases, also termed biochemical network reconstructions (90). Reconstructions are organism specific and account for genetic, and biochemical components, and their interactions, based on annotated biological information sourced from literature. All metabolic reactions and metabolites contained within a reconstruction can be represented as a numerical matrix, which is comprised of the stoichiometric factors of reactants and products of each metabolic reaction. In this format, the metabolome is subject to computational research allowing metabolic reaction flux at steady state through metabolic pathways to be computed (91).
Genome-scale metabolic reconstructions aim to account for as many as possible biochemical interactions that have been described in an organism (e.g., a human). While reconstructions afford a mechanistic description of genotype–phenotype relationships, they are not context specific. However, when constrained with cell or context-specific data, for example gene expression information of ECs, reconstructions afford GEMs that are descriptive of the biological event and cell of interest. Gene expression data of a HUVEC cells at normoxia vs. hypoxia would for instance generate two GEMs based on the same reconstruction thereby providing two snapshots descriptive of metabolic flux through reactions as defined by the two expression datasets. Essentially, reconstructions define the biochemical components of an organism, while context-specific polyomic data are required to generate a GEM of a particular cell or cellular event. Genomic, proteomic, and/or metabolomic fingerprints can thus be analyzed and compared within the context of GEMs (92).
The methodology of building, curating, and analyzing reconstructions and GEMs is commonly referred to as constraint-based analysis. Various software has been developed to facilitate constraint-based analysis including the COBRA and RAVEN toolbox’s for Matlab, Merlin and CORDA (93–96). Detailed protocols describing the necessary stages of building and curation are established (90, 97–99). Ultimately, constraint-based analysis of GEMs allows holistic exploration of metabolic phenotypes in silico and affords realistic hypotheses of biochemical mechanisms (92). In the past 5 years, multiple applications of GEMs descriptive of human metabolism have materialized that may contribute to the understanding of how genetic and environmental factors collectively contribute to CVD disease phenotypes when applied to endothelial metabolic research.
GEMs Differentiate between Metabolic Phenotypes
In the context of CVD, GEMs that are descriptive of healthy and CVD endothelial metabolism can be produced. As recently reviewed in Väremo et al. (100), GEMs of various tissues have been built and applied to the investigation of CVD-related disorders, including DM and metabolic syndrome, although not yet endothelium (101–104). Transcriptional changes in cardiomyocytes of DM patients have been analyzed using the myocyte-specific GEM, iMyocyte2419, revealing deregulation of metabolic pathways ultimately linked to dihydro-lipoamide dehydrogenase, a unique characteristic of myocyte response in DM (101).
Genome-scale metabolic models serve as a biomarker discovery tool, and a tool to discover potentially “druggable” metabolic (105). Computational techniques exist that predict the pathways likely to be responsible for differences between two metabolic states, identifying these differences allows reactions, linked to genes in a GEM, to be selected as drug targets, for example in hepatocellular carcinoma and Alzheimer’s, or metabolites to be identified as potential biomarkers for example for drug resistance in ovarian cancer (100, 106–108). Changes due in FAO in ECs leading to alterations in EC permeability – clinically important to sepsis – have been detected using a GEM. Altering FAO using drugs was shown to alter permeability, which may be clinically useful (109), future discoveries of this type may be linked to NO synthesis or clotting factor production useful for modulating CVD risk factors.
GEMs Can Define Endothelial Metabolism
Genome-scale metabolic models that are descriptive of core endothelial metabolism have already been produced. Patella et al recently used endothelial proteomic data to constrain the human reconstruction, Recon 1 (110), to generate a GEM that describes EC cell core metabolism during tube formation in matrigel (109). FAO was identified as an area of metabolism that is altered during tube formation. CPT1A inhibition affects ATP production via the TCA cycle and oxidative phosphorylation. Downstream, this alters Ca2+ signaling and junctional proteins via phospho-signaling to alter endothelial permeability, which were partially reversed by pyruvate supplementation (109). Automated GEMs have also been generated for colon and cerebral cortex ECs (111), though these models were not applied to CVD research.
Although automatically generated GEMs of EC metabolism have been used to reveal basal endothelial metabolic pathway usage, further curation and validation of EC GEMs would be beneficial. Investigations of vascular endothelial metabolism in different conditions and with different genetic backgrounds could be achieved, allowing genetic variation outside the context of core energy metabolism to be queried. For example, due to the inherent connectivity of metabolic reactions within GEMs, alterations in the release of sphingosine-1-P (a sphingolipid involved in vascular and immune signaling pathways) from ECs could be hypothesized and related to alterations in core energy metabolism induced by global metabolic expression profiles. The release of sphingosine-1-P from ECs and its contribution to individual vascular health could thus be proposed on biochemical alterations on the systems level as opposed to mutations in sphingosine kinase alone.
GEMs Can Be Personalized to Account for Individual Genetic Variation
Computational modeling can contribute to decisions regarding the suitability of a treatment for individual patients. GEMs could be produced for individuals based on genomics and subsequently used to stratify patients and personalize medical interventions for CVD. GEMs maybe based on generalized transcriptomic data from a pool of samples from a cell type (112) or a set of models may be created from individual samples and comparing the metabolic phenotypes predicted by each, allowing links between metabolism and broader phenotype, such as drug resistance in cancer cells, to be explored and may lead to insights about predictive biomarkers and druggable targets (108, 113, 114). Various algorithms for selecting active reactions for context-specific models based on transcriptomic and proteomic data are available including INIT and iMAT. These approaches have differing strengths and weaknesses that have been described and compared elsewhere (98).
Individualized hepatocellular carcinoma models have been used to predict patient outcomes based on the predicted production of acetate, identified as a key metabolic pathway for survival (114). Twenty-four individualized GEMs of erythrocytes were created based on genetic and metabolic data. These captured altered dynamics of erythrocyte metabolism and allowed the identification of individuals at risk to drug-induced anemia based upon their genomic sequence (115). These examples highlight a potential workflow, exemplified in Figure 2, to contribute to the personalization and stratification of medical treatments in the clinic. In the future, it is envisioned that an EC GEM could be used in a similar fashion by comparing GEMs CVD patients and healthy individuals to identify key metabolic changes to CVD for example those that increase production of atherosclerotic plaques.
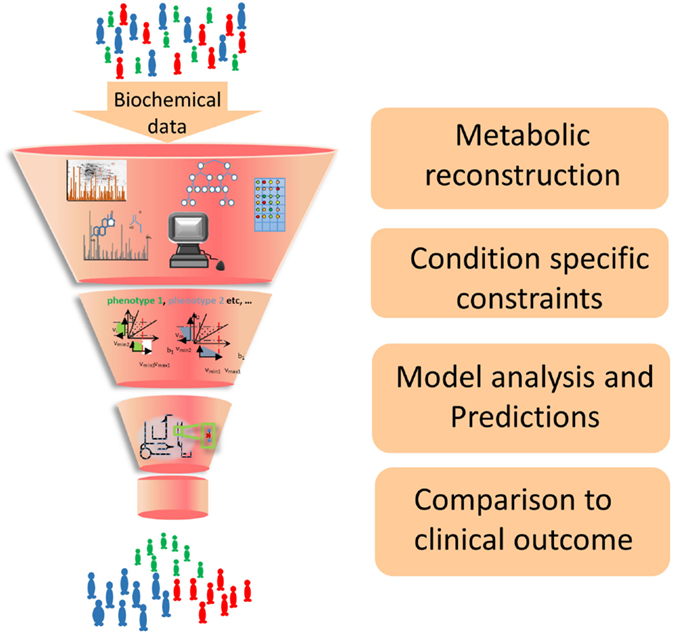
Figure 2. Workflow of GEM construction and contribution to developing new strategies for the clinic. Biochemical data from cell culture and clinical studies are combined to form a comprehensive metabolic reconstruction, which is constrained to form a context-specific GEM and produce biologically well-founded predictions that will suggest future clinical interventions.
Conclusion
Developing personalized CVD therapeutic interventions relies on the ability to account for genotypic and phenotypic variation. Variability in disease phenotypes can be captured and understood in the context of GEM’s to facilitate this process.
Genome-scale metabolic models provide an integrated approach in studying EC metabolism. They allow analysis of the multiple factors affecting ECs in the body, facilitating the exploration of the relationship of genotype to metabolic phenotype. This offers the possibility of producing personalized predictions of CVD risk and treatment, that account for both genetic and lifestyle factors. Currently, GEMs are the only biochemical model type that can account for both of these factors within a predictive modeling framework (92).
Genome-scale metabolic models are only one type of model used to account for EC function. Focused and mechanistic computational models of various aspects of vascular biology have also been made. These address some important biophysical parameters that are currently outside the scope of GEM modeling. This includes assessing the effects of shear stress on blood vessel reactivity and growth as well as the effects on blood cell/endothelium interactions of flow (116–122). Models describing the effects of circulation on endothelial metabolites have also been built (123). Endothelial NO interactions (124–126), Ca2+ signaling (127) along with protein (128) and mechanical (119) signaling have also been addressed with computational modeling. Models have been individualized using patient data and have explored the effects of stenting on blood flow (129–132).
Integrating biophysical and signaling parameters with GEMs would generate a more complete understanding of the role of endothelial metabolism for CVD. In addition, these future GEMs would allow retrospective analysis of biophysical and genomic data that have been generated in the last few decades from population studies (86, 133), whose analysis is currently confined to multivariate statistical and comparative analysis techniques for the identification of CVD risk factors. Such an effort could allow, for example, in silico querying of the effect of LDL deposition on global endothelial metabolism. Indeed, computational analysis of LDL metabolism has already proposed novel approaches to combat CVD (134–136).
Realistic computational predictions of the effects of genetic and environmental perturbations on endothelial metabolism are possible and beneficial. There has been some exploration of CVD with GEMs and analysis of EC metabolism with GEMs; however, the full potential of this technique is only just beginning to be explored. Existing and future models will allow clinicians and researchers to investigate variable endothelial function in silico in a data-driven manner, to optimize future clinical interventions.
Author Contributions
SM and OR wrote the manuscript and conceived the ideology. HH, SP, and PJ conceived the ideology and contributed to writing of the manuscript.
Conflict of Interest Statement
The authors declare that the research was conducted in the absence of any commercial or financial relationships that could be construed as a potential conflict of interest.
Acknowledgments
The authors would like to thank the Rigshospitalet Denmark for financial support. OR acknowledges RANNIS grant 130591-051. SP acknowledges European Research Council grant 641093.
References
1. WHO. Cardiovascular Diseases (CVDs). World Health Organization (2016). Available from: http://www.who.int/mediacentre/factsheets/fs317/en/
2. Field JM, Hazinski MF, Sayre MR, Chameides L, Schexnayder SM, Hemphill R, et al. Part 1: executive summary: 2010 American Heart Association guidelines for cardiopulmonary resuscitation and emergency cardiovascular care. Circulation (2010) 122(Suppl 3):640–57. doi: 10.1161/CIRCULATIONAHA.110.970889
3. Townsend N, Nichols M, Scarborough P, Rayner M. Cardiovascular disease in Europe – epidemiological update 2015. Eur Heart J (2015) 36(40):ehv428. doi:10.1093/eurheartj/ehv428
4. Landmesser U, Drexler H. The clinical significance of endothelial dysfunction. Curr Opin Cardiol (2005) 20(6):547–51. doi:10.1097/01.hco.0000179821.11071.79
5. Vanhoutte PM, Shimokawa H, Feletou M, Tang EHC. Endothelial dysfunction and vascular disease – a thirthieth anniversary update. Acta Physiol (Oxf) (2015). doi:10.1111/apha.12646
6. Kazmi R, Boyce S, Lwaleed B. Homeostasis of hemostasis: the role of endothelium. Semin Thromb Hemost (2015) 41(6):549–55. doi:10.1055/s-0035-1556586
7. Santillo M, Colantuoni A, Mondola P, Guida B, Damiano S. NOX signaling in molecular cardiovascular mechanisms involved in the blood pressure homeostasis. Front Physiol (2015) 6:194. doi:10.3389/fphys.2015.00194
8. Shen X, Tan Z, Zhong X, Tian Y, Wang X, Yu B, et al. Endocardial endothelium is a key determinant of force-frequency relationship in rat ventricular myocardium. J Appl Physiol (2013) 115(3):383–93. doi:10.1152/japplphysiol.01415.2012
9. Choi B, Steiss D, Garcia-Rivas J, Kojaku S, Schnall P, Dobson M, et al. Comparison of body mass index with waist circumference and skinfold-based percent body fat in firefighters: adiposity classification and associations with cardiovascular disease risk factors. Int Arch Occup Environ Health (2016) 89(3):435–48. doi:10.1007/s00420-015-1082-6
10. Hwang M-H, Kim S. Type 2 diabetes: endothelial dysfunction and exercise. J Exerc Nutrition Biochem (2014) 18(3):239–47. doi:10.5717/jenb.2014.18.3.239
11. Kwaśniewska M, Kozińska J, Dziankowska-Zaborszczyk E, Kostka T, Jegier A, Rębowska E, et al. The impact of long-term changes in metabolic status on cardiovascular biomarkers and microvascular endothelial function in middle-aged men: a 25-year prospective study. Diabetol Metab Syndr (2015) 7:81. doi:10.1186/s13098-015-0074-8
12. Hamrefors V. Common genetic risk factors for coronary artery disease: new opportunities for prevention? Clin Physiol Funct Imaging (2015). doi:10.1111/cpf.12289
13. Rolfsson O, Palsson B, Thiele I. The human metabolic reconstruction Recon 1 directs hypotheses of novel human metabolic functions. BMC Syst Biol (2011) 5:155. doi:10.1186/1752-0509-5-155
14. Parra-Bonilla G, Alvarez DF, Alexeyev M, Vasauskas A, Stevens T. Lactate dehydrogenase a expression is necessary to sustain rapid angiogenesis of pulmonary microvascular endothelium. PLoS One (2013) 8(9):e75984. doi:10.1371/journal.pone.0075984
15. Tuleta I, França CN, Wenzel D, Fleischmann B, Nickenig G, Werner N, et al. Intermittent hypoxia impairs endothelial function in early preatherosclerosis. Adv Exp Med Biol (2015) 858:1–7. doi:10.1007/5584_2015_114
16. Doddaballapur A, Michalik KM, Manavski Y, Lucas T, Houtkooper RH, You X, et al. Laminar shear stress inhibits endothelial cell metabolism via KLF2-mediated repression of PFKFB3. Arterioscler Thromb Vasc Biol (2015) 35(1):137–45. doi:10.1161/ATVBAHA.114.304277
17. Zhang Z, Apse K, Pang J, Stanton RC. High glucose inhibits glucose-6-phosphate dehydrogenase via cAMP in aortic endothelial cells. J Biol Chem (2000) 275(51):40042–7. doi:10.1074/jbc.M007505200
18. Halldórsson H, Thors B, Thorgeirsson G. Thrombin or Ca(++)-ionophore-mediated fall in endothelial ATP levels independent of poly(ADP-Ribose) polymerase activity and NAD levels – comparison with the effects of hydrogen peroxide. Nucleosides Nucleotides Nucleic Acids (2015) 34(4):246–57. doi:10.1080/15257770.2014.984072
19. Mahajan-Thakur S, Böhm A, Jedlitschky G, Schrör K, Rauch BH. Sphingosine-1-phosphate and its receptors: a mutual link between blood coagulation and inflammation. Mediators Inflamm (2015) 2015:831059. doi:10.1155/2015/831059
20. Viator RJ, Khader H, Hingorani N, Long S, Solodushko V, Fouty B. Hypoxia-induced increases in glucose uptake do not cause oxidative injury or advanced glycation end-product (AGE) formation in vascular endothelial cells. Physiol Rep (2015) 3(7):e12460. doi:10.14814/phy2.12460
21. Koziel A, Woyda-Ploszczyca A, Kicinska A, Jarmuszkiewicz W. The influence of high glucose on the aerobic metabolism of endothelial EA.hy926 cells. Pflugers Arch (2012) 464(6):657–69. doi:10.1007/s00424-012-1156-1
22. Dagher Z, Ruderman N, Tornheim K, Ido Y. Acute regulation of fatty acid oxidation and AMP-activated protein kinase in human umbilical vein endothelial cells. Circ Res (2001) 88(12):1276–82. doi:10.1161/hh1201.092998
23. Lohmann R, Souba WW, Bode BP. Rat liver endothelial cell glutamine transporter and glutaminase expression contrast with parenchymal cells. Am J Physiol (1999) 276(3 Pt 1):G743–50.
24. Eelen G, de Zeeuw P, Simons M, Carmeliet P. Endothelial cell metabolism in normal and diseased vasculature. Circ Res (2015) 116(7):1231–44. doi:10.1161/CIRCRESAHA.116.302855
25. Tabas I, García-Cardeña G, Owens G. Recent insights into the cellular biology of atherosclerosis. J Cell Biol (2015) 209(1):13–22. doi:10.1083/jcb.201412052
26. De Bock K, Georgiadou M, Schoors S, Kuchnio A, Wong B, Cantelmo A, et al. Role of PFKFB3-driven glycolysis in vessel sprouting. Cell (2013) 154(3):651–63. doi:10.1016/j.cell.2013.06.037
27. Dobrina A, Rossi F. Metabolic properties of freshly isolated bovine endothelial cells. Biochim Biophys Acta (1983) 762(2):295–301. doi:10.1016/0167-4889(83)90084-8
28. Krützfeldt A, Spahr R, Mertens S, Siegmund B, Piper HM. Metabolism of exogenous substrates by coronary endothelial cells in culture. J Mol Cell Cardiol (1990) 22(12):1393–404. doi:10.1016/0022-2828(90)90984-A
29. Leighton B, Curi R, Hussein A, Newsholme EA. Maximum activities of some key enzymes of glycolysis, glutaminolysis, Krebs cycle and fatty acid utilization in bovine pulmonary endothelial cells. FEBS Lett (1987) 225(1–2):93–6. doi:10.1016/0014-5793(87)81137-7
30. Parmar KM, Larman HB, Dai G, Zhang Y, Wang ET, Moorthy SN, et al. Integration of flow-dependent endothelial phenotypes by Kruppel-like factor 2. J Clin Invest (2006) 116(1):49–58. doi:10.1172/JCI24787
31. Xu Y, An X, Guo X, Habtetsion TG, Wang Y, Xu X, et al. Endothelial PFKFB3 plays a critical role in angiogenesis. Arterioscler Thromb Vasc Biol (2014) 34(6):1231–9. doi:10.1161/ATVBAHA.113.303041
32. Zhang X-G, Zhang Y-Q, Zhao D-K, Wu J-X, Zhao J, Jiao X-M, et al. Relationship between blood glucose fluctuation and macrovascular endothelial dysfunction in type 2 diabetic patients with coronary heart disease. Eur Rev Med Pharmacol Sci (2014) 18(23):3593–600.
33. Temelkova-Kurktschiev TS, Koehler C, Henkel E, Leonhardt W, Fuecker K, Hanefeld M. Postchallenge plasma glucose and glycemic spikes are more strongly associated with atherosclerosis than fasting glucose or HbA1c level. Diabetes Care (2000) 23(12):1830–4. doi:10.2337/diacare.23.12.1830
34. Azuma K, Kawamori R, Toyofuku Y, Kitahara Y, Sato F, Shimizu T, et al. Repetitive fluctuations in blood glucose enhance monocyte adhesion to the endothelium of rat thoracic aorta. Arterioscler Thromb Vasc Biol (2006) 26(10):2275–80. doi:10.1161/01.ATV.0000239488.05069.03
35. Torimoto K, Okada Y, Mori H, Tanaka Y. Relationship between fluctuations in glucose levels measured by continuous glucose monitoring and vascular endothelial dysfunction in type 2 diabetes mellitus. Cardiovasc Diabetol (2013) 12:1. doi:10.1186/1475-2840-12-1
36. Jiao X-M, Zhang X-G, Xu XU-P, Yi C, Bin C, Cheng Q-P, et al. Blood glucose fluctuation aggravates lower extremity vascular disease in type 2 diabetes. Eur Rev Med Pharmacol Sci (2014) 18(14):2025–30.
37. Service FJ, Molnar GD, Rosevear JW, Ackerman E, Gatewood LC, Taylor WF. Mean amplitude of glycemic excursions, a measure of diabetic instability. Diabetes (1970) 19(9):644–55. doi:10.2337/diab.19.9.644
38. Du X, Matsumura T, Edelstein D, Rossetti L, Zsengellér Z, Szabó C, et al. Inhibition of GAPDH activity by poly(ADP-ribose) polymerase activates three major pathways of hyperglycemic damage in endothelial cells. J Clin Invest (2003) 112(7):1049–57. doi:10.1172/JCI18127
39. Kim DH, Seok YM, Kim IK, Lee I-K, Jeong SY, Jeoung NH. Glucosamine increases vascular contraction through activation of RhoA/Rho kinase pathway in isolated rat aorta. BMB Rep (2011) 44(6):415–20. doi:10.5483/BMBRep.2011.44.6.415
40. Rajapakse AG, Ming X-F, Carvas JM, Yang Z. O-linked beta-N-acetylglucosamine during hyperglycemia exerts both anti-inflammatory and pro-oxidative properties in the endothelial system. Oxid Med Cell Longev (2009) 2(3):172–5. doi:10.4161/oxim.2.3.8482
41. Rajapakse AG, Ming X-F, Carvas JM, Yang Z. The hexosamine biosynthesis inhibitor azaserine prevents endothelial inflammation and dysfunction under hyperglycemic condition through antioxidant effects. Am J Physiol Heart Circ Physiol (2009) 296(3):H815–22. doi:10.1152/ajpheart.00756.2008
42. Wu G, Haynes TE, Yan W, Meininger CJ. Presence of glutamine:fructose-6-phosphate amidotransferase for glucosamine-6-phosphate synthesis in endothelial cells: effects of hyperglycaemia and glutamine. Diabetologia (2001) 44(2):196–202. doi:10.1007/s001250051599
43. Slawson C, Copeland RJ, Hart GW. O-GlcNAc signaling: a metabolic link between diabetes and cancer? Trends Biochem Sci (2010) 35(10):547–55. doi:10.1016/j.tibs.2010.04.005
44. Benedito R, Roca C, Sörensen I, Adams S, Gossler A, Fruttiger M, et al. The notch ligands Dll4 and Jagged1 have opposing effects on angiogenesis. Cell (2009) 137(6):1124–35. doi:10.1016/j.cell.2009.03.025
45. Vaisman N, Gospodarowicz D, Neufeld G. Characterization of the receptors for vascular endothelial growth factor. J Biol Chem (1990) 265(32):19461–6.
46. Federici M, Menghini R, Mauriello A, Hribal ML, Ferrelli F, Lauro D, et al. Insulin-dependent activation of endothelial nitric oxide synthase is impaired by O-linked glycosylation modification of signaling proteins in human coronary endothelial cells. Circulation (2002) 106(4):466–72. doi:10.1161/01.CIR.0000023043.02648.51
47. Du XL, Edelstein D, Dimmeler S, Ju Q, Sui C, Brownlee M. Hyperglycemia inhibits endothelial nitric oxide synthase activity by posttranslational modification at the Akt site. J Clin Invest (2001) 108(9):1341–8. doi:10.1172/JCI11235
48. Wautier J-L, Schmidt AM. Protein glycation: a firm link to endothelial cell dysfunction. Circ Res (2004) 95(3):233–8. doi:10.1161/01.RES.0000137876.28454.64
49. Wautier J-L, Wautier M-P. Erythrocytes and platelet adhesion to endothelium are mediated by specialized molecules. Clin Hemorheol Microcirc (2004) 30(3–4):181–4.
50. Vlassara H, Fuh H, Donnelly T, Cybulsky M. Advanced glycation endproducts promote adhesion molecule (VCAM-1, ICAM-1) expression and atheroma formation in normal rabbits. Mol Med (1995) 1(4):447–56.
51. Kajikawa M, Nakashima A, Fujimura N, Maruhashi T, Iwamoto Y, Iwamoto A, et al. Ratio of serum levels of AGEs to soluble form of RAGE is a predictor of endothelial function. Diabetes Care (2015) 38(1):119–25. doi:10.2337/dc14-1435
52. Makowski L, Brittingham KC, Reynolds JM, Suttles J, Hotamisligil GS. The fatty acid-binding protein, aP2, coordinates macrophage cholesterol trafficking and inflammatory activity. Macrophage expression of aP2 impacts peroxisome proliferator-activated receptor gamma and IkappaB kinase activities. J Biol Chem (2005) 280(13):12888–95. doi:10.1074/jbc.M413788200
53. Aragonès G, Ferré R, Lázaro I, Cabré A, Plana N, Merino J, et al. Fatty acid-binding protein 4 is associated with endothelial dysfunction in patients with type 2 diabetes. Atherosclerosis (2010) 213(1):329–31. doi:10.1016/j.atherosclerosis.2010.07.026
54. Yeung DCY, Xu A, Cheung CWS, Wat NMS, Yau MH, Fong CHY, et al. Serum adipocyte fatty acid-binding protein levels were independently associated with carotid atherosclerosis. Arterioscler Thromb Vasc Biol (2007) 27(8):1796–802. doi:10.1161/ATVBAHA.107.146274
55. Cataltepe S, Arikan MC, Liang X, Smith TW, Cataltepe O. Fatty acid binding protein 4 expression in cerebral vascular malformations: implications for vascular remodelling. Neuropathol Appl Neurobiol (2015) 41(5):646–56. doi:10.1111/nan.12159
56. Dagher Z, Ruderman N, Tornheim K, Ido Y. The effect of AMP-activated protein kinase and its activator AICAR on the metabolism of human umbilical vein endothelial cells. Biochem Biophys Res Commun (1999) 265(1):112–5. doi:10.1006/bbrc.1999.1635
57. Zabielska MA, Borkowski T, Slominska EM, Smolenski RT. Inhibition of AMP deaminase as therapeutic target in cardiovascular pathology. Pharmacol Rep (2015) 67(4):682–8. doi:10.1016/j.pharep.2015.04.007
58. Schoors S, Bruning U, Missiaen R, Queiroz KCS, Borgers G, Elia I, et al. Fatty acid carbon is essential for dNTP synthesis in endothelial cells. Nature (2015) 520(7546):192–7. doi:10.1038/nature14362
59. Pan M, Fischer CP, Wasa M, Lukaszewicz G, Stevens BR, Bode BP, et al. Amino acid metabolism and the vascular endothelium: regulation and disease implications. Shock (1995) 4(2):79–88. doi:10.1097/00024382-199508000-00001
60. Wu G, Haynes TE, Li H, Meininger CJ. Glutamine metabolism in endothelial cells: ornithine synthesis from glutamine via pyrroline-5-carboxylate synthase. Comp Biochem Physiol Part A Mol Integr Physiol (2000) 126(1):115–23. doi:10.1016/S1095-6433(00)00196-3
61. Unterluggauer H, Mazurek S, Lener B, Hütter E, Eigenbrodt E, Zwerschke W, et al. Premature senescence of human endothelial cells induced by inhibition of glutaminase. Biogerontology (2008) 9(4):247–59. doi:10.1007/s10522-008-9134-x
62. Kawashima S, Yokoyama M. Dysfunction of endothelial nitric oxide synthase and atherosclerosis. Arterioscler Thromb Vasc Biol (2004) 24(6):998–1005. doi:10.1161/01.ATV.0000125114.88079.96
63. Yang Y, Wu Z, Meininger CJ, Wu G. l-Leucine and NO-mediated cardiovascular function. Amino Acids (2015) 47(3):435–47. doi:10.1007/s00726-014-1904-y
64. Kovamees O, Shemyakin A, Eriksson M, Angelin B, Pernow J. Arginase inhibition improves endothelial function in patients with familial hypercholesterolaemia irrespective of their cholesterol levels. J Intern Med (2015). doi:10.1111/joim.12461
65. Kövamees O, Shemyakin A, Pernow J. Effect of arginase inhibition on ischemia-reperfusion injury in patients with coronary artery disease with and without diabetes mellitus. PLoS One (2014) 9(7):e103260. doi:10.1371/journal.pone.0103260
66. Daff S. NO synthase: structures and mechanisms. Nitric Oxide (2010) 23(1):1–11. doi:10.1016/j.niox.2010.03.001
67. Vásquez-Vivar J, Kalyanaraman B, Martásek P, Hogg N, Masters BS, Karoui H, et al. Superoxide generation by endothelial nitric oxide synthase: the influence of cofactors. Proc Natl Acad Sci U S A (1998) 95(16):9220–5. doi:10.1073/pnas.95.16.9220
68. Vásquez-Vivar J, Martásek P, Whitsett J, Joseph J, Kalyanaraman B. The ratio between tetrahydrobiopterin and oxidized tetrahydrobiopterin analogues controls superoxide release from endothelial nitric oxide synthase: an EPR spin trapping study. Biochem J (2002) 362(Pt 3):733–9. doi:10.1042/bj3620733
69. Ali ZA, Rinze R, Douglas G, Hu Y, Xiao Q, Qi W, et al. Tetrahydrobiopterin determines vascular remodeling through enhanced endothelial cell survival and regeneration. Circulation (2013) 128(11 Suppl 1):S50–8. doi:10.1161/CIRCULATIONAHA.112.000249
70. Xia Y, Tsai AL, Berka V, Zweier JL. Superoxide generation from endothelial nitric-oxide synthase. A Ca2+/calmodulin-dependent and tetrahydrobiopterin regulatory process. J Biol Chem (1998) 273(40):25804–8. doi:10.1074/jbc.273.40.25804
71. Landmesser U, Dikalov S, Price SR, McCann L, Fukai T, Holland SM, et al. Oxidation of tetrahydrobiopterin leads to uncoupling of endothelial cell nitric oxide synthase in hypertension. J Clin Invest (2003) 111(8):1201–9. doi:10.1172/JCI200314172
72. Paolocci N, Biondi R, Bettini M, Lee C-I, Berlowitz CO, Rossi R, et al. Oxygen radical-mediated reduction in basal and agonist-evoked NO release in isolated rat heart. J Mol Cell Cardiol (2001) 33(4):671–9. doi:10.1006/jmcc.2000.1334
73. Machado-Silva W, Alfinito-Kreis R, Carvalho LSF, Quinaglia-E-Silva JC, Almeida OLR, Brito CJ, et al. Endothelial nitric oxide synthase genotypes modulate peripheral vasodilatory properties after myocardial infarction. Gene (2015) 568(2):165–9. doi:10.1016/j.gene.2015.05.042
74. Umman B, Cakmakoglu B, Cincin ZB, Kocaaga M, Emet S, Tamer S, et al. Identification of gene variants related to the nitric oxide pathway in patients with acute coronary syndrome. Gene (2015) 574(1):76–81. doi:10.1016/j.gene.2015.07.081
75. Levinsson A, Olin A-C, Björck L, Rosengren A, Nyberg F. Nitric oxide synthase (NOS) single nucleotide polymorphisms are associated with coronary heart disease and hypertension in the INTERGENE study. Nitric Oxide (2014) 39:1–7. doi:10.1016/j.niox.2014.03.164
76. Manso H, Krug T, Sobral J, Albergaria I, Gaspar G, Ferro JM, et al. Variants within the nitric oxide synthase 1 gene are associated with stroke susceptibility. Atherosclerosis (2012) 220(2):443–8. doi:10.1016/j.atherosclerosis.2011.11.011
77. Stamboul K, Lorin J, Lorgis L, Guenancia C, Beer J-C, Touzery C, et al. Atrial fibrillation is associated with a marker of endothelial function and oxidative stress in patients with acute myocardial infarction. PLoS One (2015) 10(7):e0131439. doi:10.1371/journal.pone.0131439
78. Xuan C, Tian Q-W, Li H, Zhang B-B, He G-W, Lun L-M. Levels of asymmetric dimethylarginine (ADMA), an endogenous nitric oxide synthase inhibitor, and risk of coronary artery disease: a meta-analysis based on 4713 participants. Eur J Prev Cardiol (2016) 23(5):502–10. doi:10.1177/2047487315586094
79. Shivkar RR, Abhang SA. Ratio of serum asymmetric dimethyl arginine (ADMA)/nitric oxide in coronary artery disease patients. J Clin Diagn Res (2014) 8(8):CC04–6. doi:10.7860/JCDR/2014/7849.4665
80. Masaki N, Hakuno D, Toya T, Shiraishi Y, Kujiraoka T, Namba T, et al. Association between brachial-ankle pulse wave velocity and the ratio of l-arginine to asymmetric dimethylarginine in patients undergoing coronary angiography. J Cardiol (2015) 65(4):311–7. doi:10.1016/j.jjcc.2014.06.005
81. Tabas I, Garcia-Cardena G, Owens GK. The cell biology of disease: recent insights into the cellular biology of atherosclerosis. J Cell Biol (2015) 209(1):13–22. doi:10.1083/jcb.201412052
82. García-Bermúdez M, López-Mejías R, González-Juanatey C, Castañeda S, Miranda-Filloy JA, Blanco R, et al. Association of the methionine sulfoxide reductase A rs10903323 gene polymorphism with cardiovascular disease in patients with rheumatoid arthritis. Scand J Rheumatol (2012) 41(5):350–3. doi:10.3109/03009742.2012.677063
83. Kakoki M, Kim H-S, Edgell C-JS, Maeda N, Smithies O, Mattson DL. Amino acids as modulators of endothelium-derived nitric oxide. Am J Physiol Renal Physiol (2006) 291(2):F297–304. doi:10.1152/ajprenal.00417.2005
84. Carey PJ, Chandler J, Hendrick A, Reid MM, Saunders PW, Tinegate H, et al. Prevalence of pyruvate kinase deficiency in northern European population in the north of England. Northern Region Haematologists Group. Blood (2000) 96(12):4005–6.
85. Meloni L, Manca MR, Loddo I, Cioglia G, Cocco P, Schwartz A, et al. Glucose-6-phosphate dehydrogenase deficiency protects against coronary heart disease. J Inherit Metab Dis (2008) 31(3):412–7. doi:10.1007/s10545-008-0704-5
86. Tsao CW, Vasan RS. Cohort profile: the Framingham Heart Study (FHS): overview of milestones in cardiovascular epidemiology. Int J Epidemiol (2015) 44(6):1800–13. doi:10.1093/ije/dyv337
87. Jankovic N, Geelen A, Streppel MT, de Groot LC, Kiefte-de Jong JC, Orfanos P, et al. WHO guidelines for a healthy diet and mortality from cardiovascular disease in European and American elderly: the CHANCES project. Am J Clin Nutr (2015) 102(4):745–56. doi:10.3945/ajcn.114.095117
88. Miao C, Bao M, Xing A, Chen S, Wu Y, Cai J, et al. Cardiovascular health score and the risk of cardiovascular diseases. PLoS One (2015) 10(7):e0131537. doi:10.1371/journal.pone.0131537
89. Eckel N, Meidtner K, Kalle-Uhlmann T, Stefan N, Schulze MB. Metabolically healthy obesity and cardiovascular events: a systematic review and meta-analysis. Eur J Prev Cardiol (2015) 1–11. doi:10.1177/2047487315623884
90. Thiele I, Palsson B. A protocol for generating a high-quality genome-scale metabolic reconstruction. Nat Protoc (2010) 5(1):93–121. doi:10.1038/nprot.2009.203
91. Rolfsson Ó, Palsson BO. Decoding the jargon of bottom-up metabolic systems biology. Bioessays (2015) 37(6):588–91. doi:10.1002/bies.201400187
92. Bordbar A, Monk J, King Z, Palsson B. Constraint-based models predict metabolic and associated cellular functions. Nat Rev Genet (2014) 15(2):107–20. doi:10.1038/nrg3643
93. Becker S, Feist A, Mo M, Hannum G, Palsson B, Herrgard M. Quantitative prediction of cellular metabolism with constraint-based models: the COBRA toolbox. Nat Protoc (2007) 2(3):727–38. doi:10.1038/nprot.2007.99
94. Agren R, Liu L, Shoaie S, Vongsangnak W, Nookaew I, Nielsen J. The RAVEN toolbox and its use for generating a genome-scale metabolic model for Penicillium chrysogenum. PLoS Comput Biol (2013) 9(3):e1002980. doi:10.1371/journal.pcbi.1002980
95. Dias O, Rocha M, Ferreira EC, Rocha I. Reconstructing genome-scale metabolic models with merlin. Nucleic Acids Res (2015) 43(8):3899–910. doi:10.1093/nar/gkv294
96. Schultz A, Qutub AA. Reconstruction of tissue-specific metabolic networks using CORDA. PLoS Comput Biol (2016) 12(3):e1004808. doi:10.1371/journal.pcbi.1004808
97. Pfau T, Pacheco MP, Sauter T. Towards improved genome-scale metabolic network reconstructions: unification, transcript specificity and beyond. Brief Bioinform (2015):bbv100. doi:10.1093/bib/bbv100
98. Machado D, Herrgård M. Systematic evaluation of methods for integration of transcriptomic data into constraint-based models of metabolism. PLoS Comput Biol (2014) 10(4):e1003580. doi:10.1371/journal.pcbi.1003580
99. Mardinoglu A, Gatto F, Nielsen J. Genome-scale modeling of human metabolism – a systems biology approach. Biotechnol J (2013) 8(9):985–96. doi:10.1002/biot.201200275
100. Väremo L, Nookaew I, Nielsen J. Novel insights into obesity and diabetes through genome-scale metabolic modeling. Front Physiol (2013) 4:92. doi:10.3389/fphys.2013.00092
101. Väremo L, Scheele C, Broholm C, Mardinoglu A, Kampf C, Asplund A, et al. Proteome- and transcriptome-driven reconstruction of the human myocyte metabolic network and its use for identification of markers for diabetes. Cell Rep (2015) 11(6):921–33. doi:10.1016/j.celrep.2015.04.010
102. Kumar A, Harrelson T, Lewis NE, Gallagher EJ, LeRoith D, Shiloach J, et al. Multi-tissue computational modeling analyzes pathophysiology of type 2 diabetes in MKR mice. PLoS One (2014) 9(7):e102319. doi:10.1371/journal.pone.0102319
103. Mardinoglu A, Kampf C, Asplund A, Fagerberg L, Hallström BM, Edlund K, et al. Defining the human adipose tissue proteome to reveal metabolic alterations in obesity. J Proteome Res (2014) 13(11):5106–19. doi:10.1021/pr500586e
104. Edwards LM, Sigurdsson MI, Robbins PA, Weale ME, Cavalleri GL, Montgomery HE, et al. Genome-scale methods converge on key mitochondrial genes for the survival of human cardiomyocytes in hypoxia. Circ Cardiovasc Genet (2014) 7(4):407–15. doi:10.1161/CIRCGENETICS.113.000269
105. Yizhak K, Gabay O, Cohen H, Ruppin E. Model-based identification of drug targets that revert disrupted metabolism and its application to ageing. Nat Commun (2013) 4:2632. doi:10.1038/ncomms3632
106. Stempler S, Yizhak K, Ruppin E. Integrating transcriptomics with metabolic modeling predicts biomarkers and drug targets for Alzheimer’s disease. PLoS One (2014) 9(8):e105383. doi:10.1371/journal.pone.0105383
107. Shlomi T, Cabili MN, Ruppin E. Predicting metabolic biomarkers of human inborn errors of metabolism. Mol Syst Biol (2009) 5(1):263. doi:10.1038/msb.2009.22
108. Agren R, Mardinoglu A, Asplund A, Kampf C, Uhlen M, Nielsen J. Identification of anticancer drugs for hepatocellular carcinoma through personalized genome-scale metabolic modeling. Mol Syst Biol (2014) 10(3):721. doi:10.1002/msb.145122
109. Patella F, Schug Z, Persi E, Neilson L, Erami Z, Avanzato D, et al. Proteomics-based metabolic modeling reveals that fatty acid oxidation (FAO) controls endothelial cell (EC) permeability. Mol Cell Proteomics (2015) 14(3):621–34. doi:10.1074/mcp.M114.045575
110. Duarte N, Becker S, Jamshidi N, Thiele I, Mo M, Vo T, et al. Global reconstruction of the human metabolic network based on genomic and bibliomic data. Proc Natl Acad Sci U S A (2007) 104(6):1777–82. doi:10.1073/pnas.0610772104
111. Agren R, Bordel S, Mardinoglu A, Pornputtapong N, Nookaew I, Nielsen J. Reconstruction of genome-scale active metabolic networks for 69 human cell types and 16 cancer types using INIT. PLoS Comput Biol (2012) 8(5):e1002518. doi:10.1371/journal.pcbi.1002518
112. Fouladiha H, Marashi S-A, Shokrgozar M. Reconstruction and validation of a constraint-based metabolic network model for bone marrow-derived mesenchymal stem cells. Cell Prolif (2015) 48(4):475–85. doi:10.1111/cpr.12197
113. Motamedian E, Ghavami G, Sardari S. Investigation on metabolism of cisplatin resistant ovarian cancer using a genome scale metabolic model and microarray data. Iran J Basic Med Sci (2015) 18(3):267–76.
114. Björnson E, Mukhopadhyay B, Asplund A, Pristovsek N, Cinar R, Romeo S, et al. Stratification of hepatocellular carcinoma patients based on acetate utilization. Cell Rep (2015) 13(9):2014–26. doi:10.1016/j.celrep.2015.10.045
115. Bordbar A, McCloskey D, Zielinski DC, Sonnenschein N, Jamshidi N, Palsson BO. Personalized whole-cell kinetic models of metabolism for discovery in genomics and pharmacodynamics. Cell Syst (2015) 1(4):283–92. doi:10.1016/j.cels.2015.10.003
116. Kirby P, Buerk DG, Parikh J, Barbee KA, Jaron D. Mathematical model for shear stress dependent NO and adenine nucleotide production from endothelial cells. Nitric Oxide (2015) 52:1–15. doi:10.1016/j.niox.2015.10.004
117. Koo A, Nordsletten D, Umeton R, Yankama B, Ayyadurai S, García-Cardeña G, et al. In silico modeling of shear-stress-induced nitric oxide production in endothelial cells through systems biology. Biophys J (2013) 104(10):2295–306. doi:10.1016/j.bpj.2013.03.052
118. Kang HG, Shim EB, Chang K-S. A new multiphysics model for the physiological responses of vascular endothelial cells to fluid shear stress. J Physiol Sci (2007) 57(5):299–309. doi:10.2170/physiolsci.RP005407
119. Lim YC, Cooling MT, Long DS. Computational models of the primary cilium and endothelial mechanotransmission. Biomech Model Mechanobiol (2015) 14(3):665–78. doi:10.1007/s10237-014-0629-x
120. Nordgaard H, Swillens A, Nordhaug D, Kirkeby-Garstad I, Van Loo D, Vitale N, et al. Impact of competitive flow on wall shear stress in coronary surgery: computational fluid dynamics of a LIMA-LAD model. Cardiovasc Res (2010) 88(3):512–9. doi:10.1093/cvr/cvq210
121. McDougall SR, Anderson ARA, Chaplain MAJ. Mathematical modelling of dynamic adaptive tumour-induced angiogenesis: clinical implications and therapeutic targeting strategies. J Theor Biol (2006) 241(3):564–89. doi:10.1016/j.jtbi.2005.12.022
122. Pontrelli G, Halliday I, Spencer TJ, König CS, Collins MW. Modelling the glycocalyx-endothelium-erythrocyte interaction in the microcirculation: a computational study. Comput Methods Biomech Biomed Engin (2015) 18(4):351–61. doi:10.1080/10255842.2013.799146
123. Comerford A, David T, Plank M. Effects of arterial bifurcation geometry on nucleotide concentration at the endothelium. Ann Biomed Eng (2006) 34(4):605–17. doi:10.1007/s10439-005-9046-8
124. Kar S, Kavdia M. Modeling of biopterin-dependent pathways of eNOS for nitric oxide and superoxide production. Free Radic Biol Med (2011) 51(7):1411–27. doi:10.1016/j.freeradbiomed.2011.06.009
125. Kar S, Kavdia M. Endothelial NO and production rates differentially regulate oxidative, nitroxidative, and nitrosative stress in the microcirculation. Free Radic Biol Med (2013) 63:161–74. doi:10.1016/j.freeradbiomed.2013.04.024
126. Ghonaim NW, Fraser GM, Ellis CG, Yang J, Goldman D. Modeling steady state SO2-dependent changes in capillary ATP concentration using novel O2 micro-delivery methods. Front Physiol (2013) 4:260. doi:10.3389/fphys.2013.00260
127. Munaron L, Scianna M. Multilevel complexity of calcium signaling: modeling angiogenesis. World J Biol Chem (2012) 3(6):121–6. doi:10.4331/wjbc.v3.i6.121
128. Wei XN, Han BC, Zhang JX, Liu XH, Tan CY, Jiang YY, et al. An integrated mathematical model of thrombin-, histamine-and VEGF-mediated signalling in endothelial permeability. BMC Syst Biol (2011) 5(1):112. doi:10.1186/1752-0509-5-112
129. Sakellarios AI, Papafaklis MI, Siogkas P, Athanasiou LS, Exarchos TP, Stefanou K, et al. Patient-specific computational modeling of subendothelial LDL accumulation in a stenosed right coronary artery: effect of hemodynamic and biological factors. Am J Physiol Heart Circ Physiol (2013) 304(11):H1455–70. doi:10.1152/ajpheart.00539.2012
130. Nicolás M, Peña E, Malvè M, Martínez MA. Mathematical modeling of the fibrosis process in the implantation of inferior vena cava filters. J Theor Biol (2015) 387:228–40. doi:10.1016/j.jtbi.2015.09.028
131. Kagadis GC, Skouras ED, Bourantas GC, Paraskeva CA, Katsanos K, Karnabatidis D, et al. Computational representation and hemodynamic characterization of in vivo acquired severe stenotic renal artery geometries using turbulence modeling. Med Eng Phys (2008) 30(5):647–60. doi:10.1016/j.medengphy.2007.07.005
132. Cunnane EM, Mulvihill JJE, Barrett HE, Walsh MT. Simulation of human atherosclerotic femoral plaque tissue: the influence of plaque material model on numerical results. Biomed Eng Online (2015) 14(Suppl 1):S7. doi:10.1186/1475-925X-14-S1-S7
133. Harris TB, Launer LJ, Eiriksdottir G, Kjartansson O, Jonsson PV, Sigurdsson G, et al. Age, Gene/Environment Susceptibility-Reykjavik Study: multidisciplinary applied phenomics. Am J Epidemiol (2007) 165(9):1076–87. doi:10.1093/aje/kwk115
134. Holzinger ER, Dudek SM, Frase AT, Krauss RM, Medina MW, Ritchie MD. ATHENA: a tool for meta-dimensional analysis applied to genotypes and gene expression data to predict HDL cholesterol levels. Pac Symp Biocomput (2013):385–96. doi:10.1142/9789814447973_0038
135. Metts B, Thatcher S, Lewis E, Karounos M, Cassis L, Smith R, et al. DDDAS design of drug interventions for the treatment of dyslipidemia in ApoE(-/-) mice. J Dev drugs (2013) 2(2):107. doi:10.4172/2329-6631.1000107
Keywords: endothelium, metabolism, personalized/precision medicine, metabolomics, metabolic modeling, genetics
Citation: McGarrity S, Halldórsson H, Palsson S, Johansson PI and Rolfsson Ó (2016) Understanding the Causes and Implications of Endothelial Metabolic Variation in Cardiovascular Disease through Genome-Scale Metabolic Modeling. Front. Cardiovasc. Med. 3:10. doi: 10.3389/fcvm.2016.00010
Received: 29 January 2016; Accepted: 03 April 2016;
Published: 18 April 2016
Edited by:
Matteo Vatta, Indiana University. USAReviewed by:
Nazareno Paolocci, Johns Hopkins University, USAMete Civelek, University of Virginia, USA
Andrew James Murphy, Baker IDI Heart and Diabetes Institute, Australia
Copyright: © 2016 McGarrity, Halldórsson, Palsson, Johansson and Rolfsson. This is an open-access article distributed under the terms of the Creative Commons Attribution License (CC BY). The use, distribution or reproduction in other forums is permitted, provided the original author(s) or licensor are credited and that the original publication in this journal is cited, in accordance with accepted academic practice. No use, distribution or reproduction is permitted which does not comply with these terms.
*Correspondence: Óttar Rolfsson, o.rolfsson@gmail.com