Can land-use and land-cover change explain reduced resilience in forests?
- 1Department of Geosciences and Geography, University of Helsinki, Helsinki, Finland
- 2Department of Economics and Rural Development, Gembloux Agro-Bio Tech, University of Liège, Gembloux, Belgium
- 3School of Research and Graduate Studies, Arcada University of Applied Sciences, Helsinki, Finland
Generating signals of reduced resilience in ecosystems is crucial for conservation and management endeavors. However, the practical implications of such systems are still limited due to the lack of high-frequency data and uncertainties associated with predicting complex systems such as ecosystems. This study aims to investigate the potential of time series analysis of remote sensing data in detecting signals of reduced resilience in mangrove forest ecosystems. Using time series analysis of remote sensing images, the resilience of mangrove forests was explored across two distinct study sites. One site (Qeshm Island) has been adversely affected by land-use and land-cover changes, while the other (Gabrik) serves as a reference ecosystem. The study uses data from the Moderate Resolution Imaging Spectroradiometer (MODIS) satellite to quantify three remotely sensed indices: the Normalized Difference Vegetation Index (NDVI), the Modified Normalized Difference Water Index (MNDWI), and the Modified Vegetation Water Ratio (MVWR). In addition, Landsat data has been used to explore temporal alterations in land-use and land-cover change. To identify signals of reduced resilience, trend analyses of indicators such as autocorrelation (acf (1)) and standard deviation (SD) are applied. The findings revealed a notable decrease in resilience, signaled by significant upward trends in NDVI statistical metrics for Qeshm Island (Kendall’s τ of acf (1): 0.50 and SD: 0.90), contrasting with the pattern observed in Gabrik (Kendall’s τ of acf (1): −0.19 and SD: −0.19). These results align with our expectations derived from previous studies. Despite MNDWI significantly indicating reduced resilience in Qeshm Island (Kendall’s τ of acf (1): 0.86 and SD: 0.90), it also signaled decreased resilience in Gabrik (Kendall’s τ of acf (1): 0.79 and SD: 0.90). Moreover, MVWR failed to indicate signals of reduced resilience in both sites, specifically in Qeshm (Kendall’s τ of acf (1): −0.10 and SD: −0.07) and in Gabrik (Kendall’s τ of acf (1): −0.72 and SD: −0.12). These findings may be explained through quantitative analyses of land-use and land-cover change. While Qeshm Island and Gabrik share similarities in climate, geography, and annual rainfall, the analysis of land-use and land-cover change revealed significant differences between the two study areas. Qeshm Island underwent drastic increases in the built-up class by a 64.40% change between 1996 and 2014, whereas the built-up class expanded modestly by a 4.04% change in the Gabrik site. This study contributes to advancing our understanding of ecosystem dynamics. The findings of this study can be integrated with ecosystem management tools to enhance the effectiveness of conservation efforts. This is the first report of the successful application of remote sensing in generating signals of reduced resilience within mangrove forests in the Middle East.
1 Introduction
Mangrove forests play a crucial role in providing valuable ecosystem services, contributing to a staggering annual value of at least US $1.6 billion (Polidoro et al., 2010). These services encompass a wide range of benefits that support coastal livelihoods on a global scale (Dahdouh-Guebas et al., 2005; Duke et al., 2007; Ellison, 2008; Abrantes et al., 2015). However, mangrove forests are disappearing worldwide by 1%–2% per year (Duke et al., 2007). Clearing for coastal development, expansion of aquaculture, logging for timber, and fuel production are among the primary drivers behind this concerning trend (Daru et al., 2013; Kirui et al., 2013; Yessoufou and Stoffberg, 2016). It has been shown that over 40% of the assessed vertebrate species endemic to mangrove forests are currently facing global threats to their survival (Luther and Greenberg, 2009). As a result, urgent attention is needed to monitor the state of mangrove forests and get a better understanding of mangrove forest dynamics in response to disturbances.
According to the critical slowing down theory, the response of an ecosystem, such as the mangrove forest ecosystem, to disturbance is not always a gradual process; it can lead to sudden and irreversible changes (Scheffer, 1990; Scheffer, 2001; Scheffer et al., 2001). In fact, even gradual changes in the environment may not result in a corresponding gradual response from the ecosystem. Instead, they can trigger sudden, unpredictable, and irreversible shifts known as regime shifts (Capon et al., 2015). Growing evidence of critical changes occurrences in different ecosystems around the world (Barbier et al., 2008; Guttal and Jayaprakash, 2008; Lenton, 2011; Verbesselt et al., 2016; Alibakhshi et al., 2017), emphasizes the importance of gaining a deeper understanding of changes in ecosystem states and developing early warning signals for critical transitions in these ecosystems.
Abrupt changes in the state of an ecosystem can occur when ecosystems are unable or slow to cope with the effects of disturbances, which is a sign of a state change or reduced resilience (Carpenter and Brock, 2006; Carpenter et al., 2008; Scheffer et al., 2009; Carpenter and Brock, 2011; Carpenter et al., 2011). Disturbances can push the state of an ecosystem to a state that is near a critical threshold. Once a critical threshold is reached, even a small disturbance can trigger a significant transition to a new state, where it is challenging and sometimes impossible to return to the previous state (Scheffer et al., 2001; Carpenter and Brock, 2006; Carpenter et al., 2008; Scheffer et al., 2009; Carpenter and Brock, 2011; Carpenter et al., 2011).
Various statistical analyses have been developed to quantify the state of an ecosystem (Carpenter and Brock, 2006; Dakos et al., 2012a; Kéfi et al., 2014). For example, an increasing trend in autocorrelation and standard deviation of a “state variable” over time can serve as early warning signals (Dakos et al., 2012a; Dakos et al., 2012b; Lenton et al., 2012; Dakos et al., 2015). A “state variable” is an indicator that can present the state of an ecosystem and measure the proximity of the ecosystem to critical conditions. Increased skewness can also be used to measure early warning signals of critical transition, as disturbances can lead to alterations in the asymmetrical distribution of the time series of a state variable before the state change occurrences (Guttal and Jayaprakash, 2008; Dakos et al., 2012a).
Despite the availability of various methods for assessing early warning signals, the anticipation of critical transitions in ecosystems poses significant challenges. Hence, the application of the methods to assess the state of ecosystems and identify early warning signals of reduced resilience is still limited. This limitation stems from the complexity of ecosystem processes, compounded by the requirement for high-frequency time series data of ecosystem state variables (Dakos et al., 2015). To address this problem, this study uses the high spatio-temporal resolution of satellite images. Satellite images offer high-frequency time series of data, comprehensive coverage, and multispectral capabilities, which are required for generating reliable early warnings.
This study aims to investigate the potential of time series analysis of remote sensing data in detecting signals of reduced resilience in mangrove forest ecosystems. Understanding the state of mangrove forests is particularly crucial, as it can inform conservation efforts for safeguarding the mangrove services, especially for locals (Martínez et al., 2007). Detecting the state of mangroves can prevent irreversible changes in the state of the ecosystem by facilitating early interventions (Lenton et al., 2008; Hirota et al., 2011; Alibakhshi, 2023). The application of the critical slowing down theory in monitoring mangrove forests has been rarely tested (Wang et al., 2023), especially in the Middle East. This is the first study that explores the potential of remote sensing to explore early warning signals of impending critical transition in mangrove forests in the Middle East.
To achieve the aim of this study, the selection of the right ecosystem state variables is crucial. A prior research study has demonstrated that utilizing remotely sensed indicators capable of simultaneously capturing variations in both water and vegetation can provide a more comprehensive understanding of ecosystem states compared to relying solely on either vegetation-based or water-based indices (Alibakhshi et al., 2017). Hence, in this study, we used a vegetation-based indicator, namely, the Normalized Difference Vegetation Index (NDVI), a water-based indicator, namely, the Modified Normalized Difference Water Index (MNDWI), and a vegetation-water-based indicator, namely, Modified Vegetation Water Ratio (MVWR). To assess the land-use and land-cover change, Landsat data has been used. Mangrove forest ecosystems in Qeshm Island and Gabrik are chosen as study sites, where Qeshm Island is selected as a representative of an unhealthy ecosystem, while Gabrik serves as the reference site for comparison.
2 Material and methods
2.1 Study sites
This study focuses on Qeshm Island (Mazraeh and Pazhouhanfar, 2018; Kourosh Niya et al., 2019), located in the southern region of Iran, between the Persian Gulf and the Oman Sea. Qeshm Island, with a total area of 1667 km2 has undergone substantial land-use and land-cover change (Kourosh Niya et al., 2019) (Figure 1). Another study site, Gabrik, with a total area of 2,496 km2, has been selected as a reference case study, representing a healthy ecosystem. Both study sites are characterized by the presence of mangrove forest ecosystems (Zahed et al., 2010; Naderloo et al., 2013) (Figure 1).
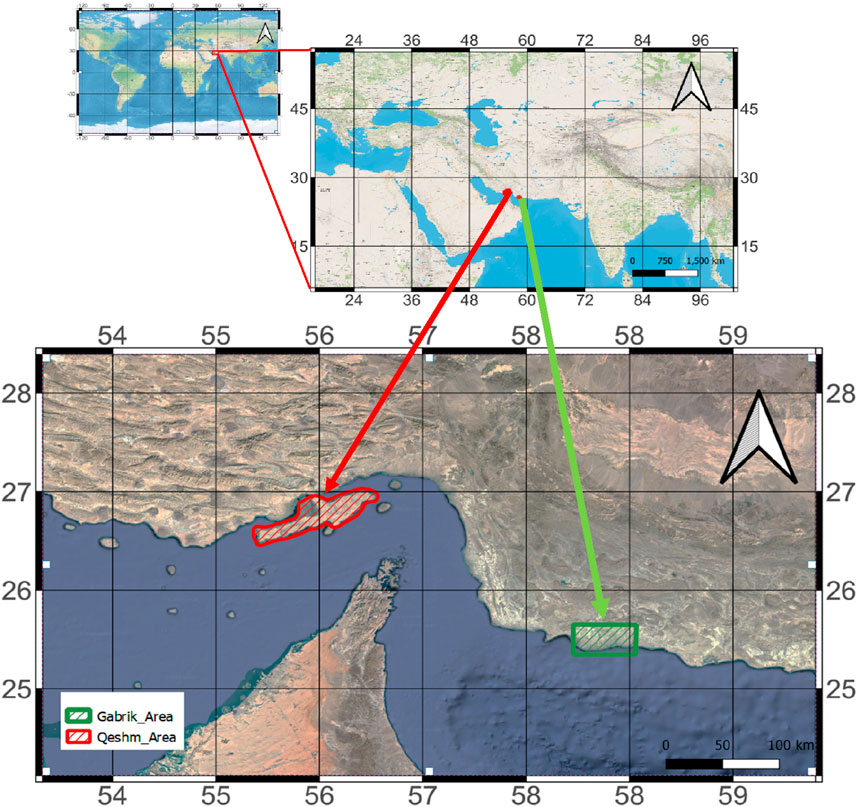
FIGURE 1. The geographic location of Qeshm Island and Kobi mangrove forests, using High-Resolution Esti Imagery and Google Maps overlay with the geographic coordinate system with an accuracy of at best 2-m spatial resolution. The images were accessed via ArcGIS online services. Data Source: Google Map, Esri, DigitalGlobe, GeoEye, Earthstar Geographics, CNES/Airbus DS, USDA, USGS, AeroGRID, IGN, and the GIS User Community. The red color polygon refers to the study site in Qeshm Island and the green colour polygon represents the Gabrik study site.
Based on ECMWF Reanalysis version 5 (ERA-5) data (Muñoz Sabater, 2019), the mean temperature in Qeshm Island is 301 K (28°C), and the mean precipitation is 36 mm between 18 February 2000 and 31 July 2021. In Gabrik, the mean temperature is 301 K (28°C), with an average precipitation of 28 mm between 18 February 2000 and 31 July 2021. These show the similarity of climatic conditions and local weather patterns of Qeshm Island and Gabrik study sites (Figure 2). According to the population dataset provided by WorldPop (Sorichetta et al., 2015), the estimated population residing in the region of Gabrik is reported to be remarkably low, with a mere 74 individuals. On the other hand, a significantly higher population count of 957 individuals are living in Qeshm Island.
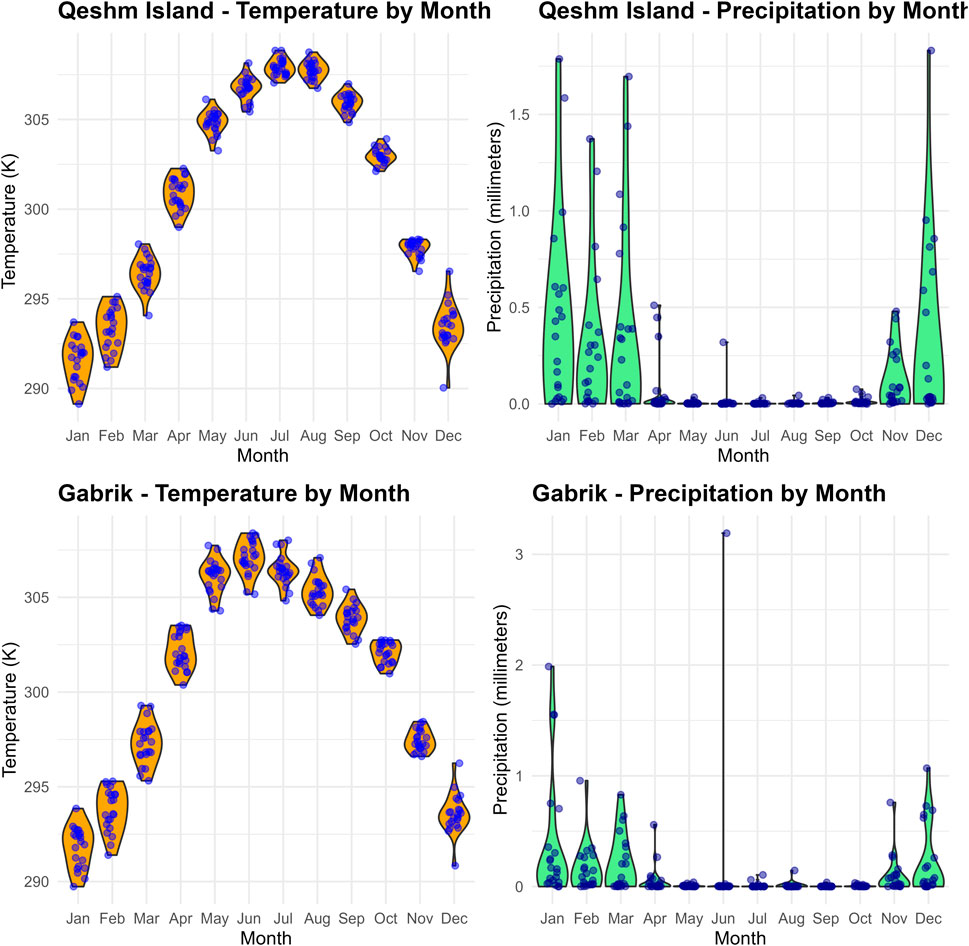
FIGURE 2. The monthly temperature in Kelvin (K) and precipitation in millimeters (mm) for Qeshm Island and Gabrik between 18 February 2000 and 31 July 2021.
In the context of Qeshm Island’s mangrove ecosystems, it is evident that they are subject to various stressors such as 1) eutrophication and pollution, and 2) biological stressors. More specifically, 1) eutrophication and pollution, from nutrient-rich wastewater effluent, have been identified as the main factors influencing mangrove health in the region (Akbarzadeh-Chomachaei et al., 2023). Heavy metal pollution in coastal sediments, as evidenced in Qeshm Island, raises concerns regarding mangrove ecosystem integrity (Akbarzadeh-Chomachaei et al., 2023). Petroleum pollution in sediments from Qeshm Island compounds these environmental challenges (Ebrahimi-Sirizi and Riyahi-Bakhtiyari, 2013; Hamzeh et al., 2021). Along with such anthropogenic disturbances, 2) biological stressors also play a significant role in disturbing mangrove forests. The proliferation of harmful algal blooms, a consequence of eutrophication, has emerged as a significant biological stressor impacting mangrove ecosystems in the region (Mirza Esmaeili et al., 2021). Additionally, the presence of a fungal pathogen and the documented damage on mangroves highlights the multifaceted biological threats faced by these mangroves (Goudarzi and Moslehi, 2020; Moslehi et al., 2023). The Gabrik site is largely protected and, unlike Qeshm Island, has been less impacted by pollution (Zarezadeh and Rezaee, 2016).
2.2 Materials and methods
2.2.1 State variables
The NDVI has been obtained from the latest version of the Moderate Resolution Imaging Spectroradiometer (MODIS) product, specifically MOD09A1 (version 006). The 006 version of MOD09A1 has undergone numerous enhancements in the algorithm (Didan, 2015). The data utilized in this study was acquired from the Google Earth Engine platform (Gorelick et al., 2017). The dataset utilized herein possesses a spatial resolution of 500 m with a temporal resolution of 16 days from 18 February 2000, to 31 July 2021. The MOD09A1 product is derived from atmospherically corrected bi-directional surface reflectance. The calculation of the NDVI (dimensionless) involves utilizing the Red and Near-Infrared (NIR) bands, particularly within the wavelengths of 0.620 μm–0.670 μm and 0.841 μm–0.876 μm, respectively (Eq. 1). The NDVI values range from −1 to +1, where a value of −1 indicates bare land, while a value of +1 indicates dense forest.
The MNDWI is calculated using MOD09A1 (version 006), which is described earlier. Among the various remotely sensed water indices (Mozumder et al., 2014; Rokni et al., 2014; Li et al., 2015), MNDWI has been acknowledged as one of the most accurate indicators for extracting water area variations (Ji et al., 2009; Chen et al., 2013). MNDWI, similar to NDVI, is a dimensionless index that ranges between −1 and 1. When MNDWI values are below 0, it indicates low water content, which includes soil and vegetation. On the other hand, MNDWI values above 0 indicate high water content and varying water levels. The calculation of the MNDWI involves utilizing the Green and shortwave infrared (SWIR) bands, specifically within the wavelengths of 0.55 μm–0.57 μm and 1.23 μm–1.25 μm, respectively (Eq. 2).
The MVWR (Eq. 3) is sensitive to changes in vegetation water content, which is the main component of mangrove forests (Alibakhshi et al., 2017). MVWR effectively captures variations in water availability and reflects hydrological dynamics, such as seasonal fluctuations and long-term shifts. Moreover, the MVWR is shown as a reliable indicator to assess vegetation health and stress levels (Tehrani and Janalipour, 2021), which can make it a powerful indicator for the aim of this study. The calculation of the MNDWI involves:
2.2.2 Map of mangrove forest ecosystems and land-use and land-cover change
The land-use and land-cover change maps of study sites were calculated with a spatial resolution of 30 m (Kourosh Niya et al., 2019), by using Landsat satellite imagery for the years 1996, 2002, 2008, and 2014. The maps provide a classification map of study sites, including six distinct land use classes: agriculture, bare-land, built-up, dense vegetation, mangrove forests, and waterbody. The maps exhibit a total accuracy and Kappa coefficient ranging from 88% to 91% (Kourosh Niya et al., 2019).
To delineate mangrove forests as training data, the global mangrove forest distribution dataset was employed for the year 2000 with a spatial resolution of approximately 30 m (Giri et al., 2011; Giri et al., 2013). The data compilation involved analyzing over 1,000 Landsat Thematic Mapper ™ scenes using a hybrid approach of classification techniques.
2.2.3 Exploring early warning signals of critical transition
First, 100 points were randomly selected at each study site. From each point, the time series of the state variables (Section 2.2.1) was extracted using a mean function for the period between 18 February 2000 and 31 July 2021. The utilization of random points stems from variations in the study extent. Subsequently, autocorrelation, skewness, and standard deviation were applied to detect early warning signals of critical transition (Dakos et al., 2012a). Autocorrelation (
Standard deviation (SD) measures the degree of variability or distribution for a set of data relative to the mean of the same data. SD is obtained from the variance as shown in Eq. 5:
Skewness is the third statistical index used in this study, which is calculated using Eq. 6:
Prior to applying metric-based models, the data were detrended and smoothed to mitigate the impact of nonstationary conditions on the leading indicators (Dakos et al., 2012a) using a Gaussian filter (Lenton et al., 2012). In this study, a sensitivity analysis was conducted using Kendall’s τ, a nonparametric statistic measuring the association between indicators and time, to identify the optimal size of the rolling window and bandwidth for the Gaussian filter (Bevan and Kendall, 1971). Kendall’s τ ranges from −1 to +1, where higher values indicate stronger trends, aiming to identify the detrending settings that best capture trends in the leading indicators. To achieve this, the leading indicators for various rolling window sizes (ranging from 25% to 75% of the time series length) and bandwidths (ranging from 25% to 75% of the time series length for the Gaussian filter) with increments of 10%, were calculated using Gaussian filter.
To ensure that the observed trends in the leading indicators were not due to random chance, 1000 surrogate datasets were generated. These datasets were created by fitting the best linear autoregressive moving average model (ARMA) based on Akaike Information Criterion (AIC) to the residuals obtained after detrending the data. AIC quantifies the trade-off between the goodness of fit of a model and its complexity by penalizing models in case there are too many parameters using the likelihood function. Each surrogate dataset had the same length as the residual time series. The probability of finding a trend by chance was measured by comparing Kendall’s τ of the original data with the number of cases in which the statistic was equal to or smaller than the estimates of the simulated records, denoted as P (τ∗≤τ) (Dakos et al., 2012a).
In this study, we used a large variety of freely available remotely sensed data. All statistical analyses and visualizations were performed in R statistical software (Pinheiro et al., 2000), QGIS (Qgis, 2016), and Google Earth Engine (Gorelick et al., 2017).
3 Results
The results in this section include analyses of land-use and land-cover change in Qeshm Island and Gabrik, representing changes in agriculture, bare land, built-up areas, mangroves, vegetation, and water bodies classes (Section 4.1). Time series of state variables, including NDVI, MNDWI, and MVWR are represented, which show temporal dynamics and seasonal variations of mangrove forests in study sites (Section 4.2). The early warning signals and the results of sensitivity analyses have been presented (Section 4.3 and Supplementary Material).
3.1 Land-use and land-cover change
Qeshm Island experienced various changes in land-use and land-cover change during the study period (Table 1; Figure 3). The area dedicated to agriculture witnessed a marginal increase of 338 units (unit: number of Landsat pixels with spatial resolution of 30-m) (0.65% change). However, there was a significant decrease in bare land, with a change of −57,360 units (−3.65% change). On the other hand, built-up areas expanded dramatically, showing an increase of 35,759 units (64.40% change). Mangroves and vegetation also exhibited positive growth, with changes of 6,049 units (9.07% change) and 6,880 units (70.30% change), respectively. Water bodies slightly expanded, with a change of 8,336 units (0.82% change).
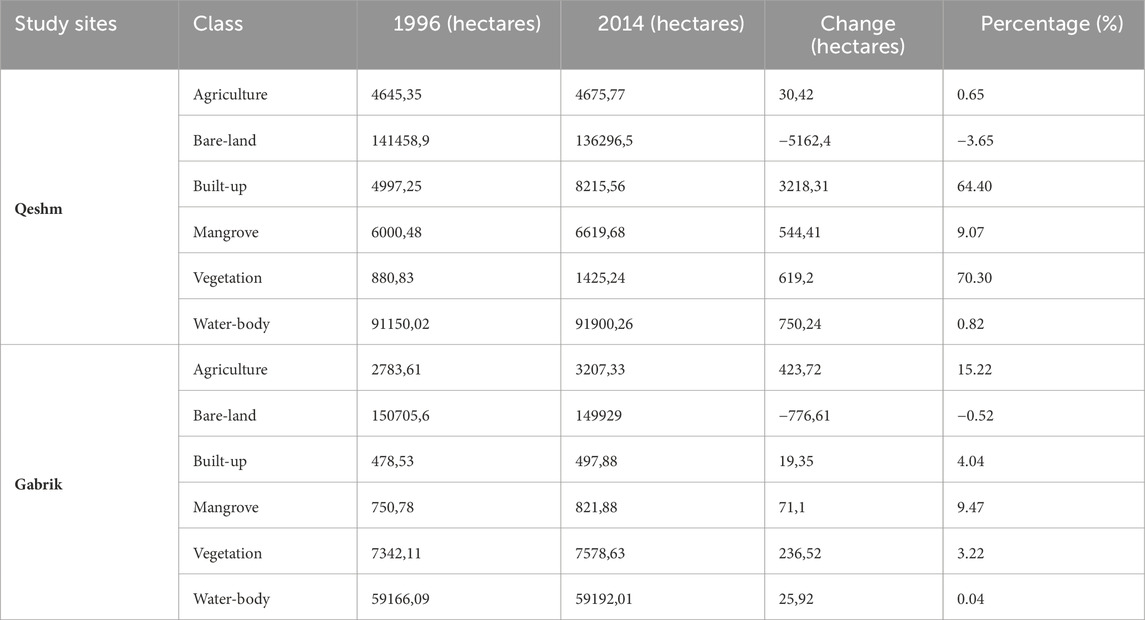
TABLE 1. Land-use and land-cover change in Qeshm Island and Gabrik obtained from Landsat images at 30-m spatial resolution between 1996 and 2014.
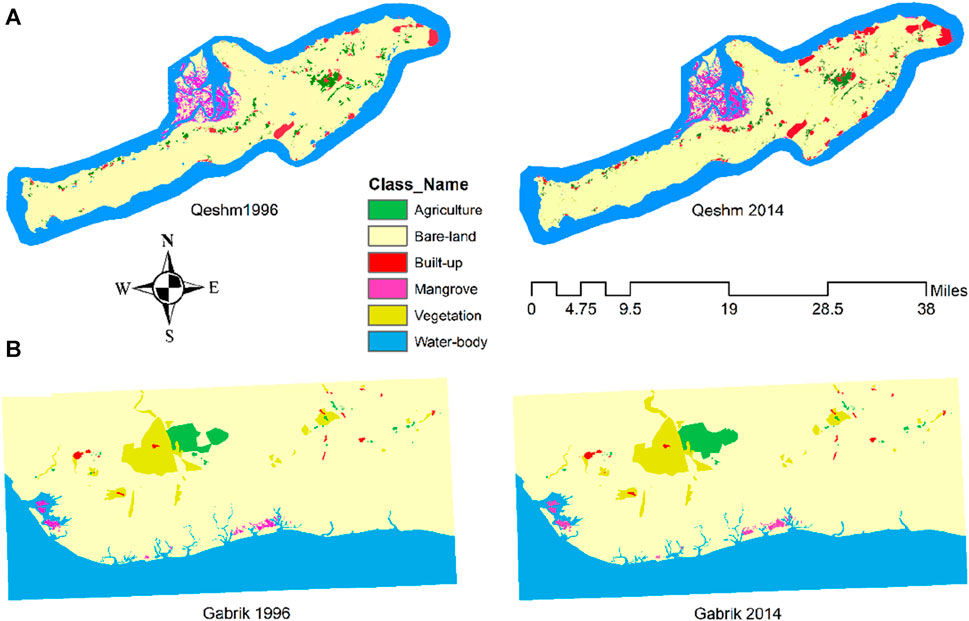
FIGURE 3. Land-use maps of Qeshm Island (A) and Gabrik (B) were calculated using Landsat images at 30-m spatial resolution between 1996 and 2014.
In Gabrik, agriculture experienced substantial growth (Table 1), with a change of 4,708 units (15.22% change). Bare land decreased, albeit to a lesser extent, with a change of −8,629 units (−0.52% change). The built-up areas expanded modestly, with a change of 215 units (4.04% change). Mangroves and vegetation also showed positive growth, with changes of 790 units (9.47% change) and 2,628 units (3.22% change), respectively. Water bodies remained relatively stable, with a minimal change of 288 units (0.04% change).
3.2 Time series of state variables
The NDVI values in study areas range from 0.01 to 0.36, MNDWI values range from −0.01 to 0.36, and MVWR values range from −0.242 to 0.263 in Qeshm Island and Gabrik (Figure 4). More specifically, Qeshm Island has a slightly higher average NDVI value of 0.20, indicating a relatively denser green vegetation cover compared to Gabrik, which has an average NDVI of 0.17. In terms of water presence, Gabrik exhibits a slightly lower average MNDWI value of 0.04 compared with Qeshm Island, which has an average MNDWI of 0.10. Regarding vegetation water content, Gabrik and Qeshm Island have similar average MVWR values of 0.10 and 0.09, respectively.
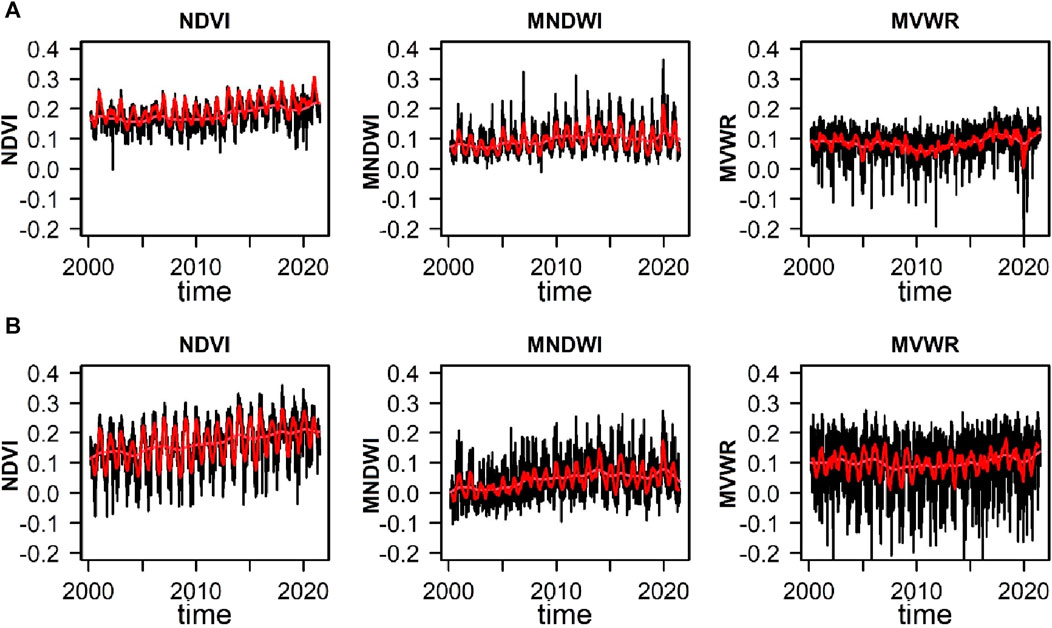
FIGURE 4. The time series of three remotely sensed indices. The x-axis in each graph represents the remotely sensed indicator and the y-axis represents time. The first column represents the time series of the Normalized Difference Vegetation Index (NDVI), the second column represents the Modified Normalized Water Index (MNDWI), and the third column represents the Modified Vegetation Water Ratio (MVWR) from 18 February 2000, to 31 July 2021, at 500-m spatial resolution in Qeshm Island (A) and Gabrik (B). The red line illustrates the trend obtained using a moving average with a window size of 20-time steps.
3.3 Early warning signals of a critical transition
In Qeshm Island, NDVI and MNDWI exhibited significant positive trends in autocorrelation (0.505, 0.864) and standard deviation (0.891, 0.903) (Figure 5 and Table 2). Notably, MVWR shows a negative trend in autocorrelation (−0.100) and standard deviation (−0.074). The trend of skewness in NDVI (0.288) indicates a more pronounced increasing trend compared to MNDWI (−0.584) and MVWR (−0.212). In Gabrik, the reference study site, NDVI exhibited a negative trend in autocorrelation (−0.192) and standard deviation (−0.186), while there was a positive trend in sweetness (0.431) (Figure 5 and Table 2). The MNDWI demonstrated an uptick in trends of both autocorrelation (0.789) and standard deviation (0.891) and a decrease in skewness (−0.576). MVWR in Gabrik exhibited corresponding declines in trends of all the indicators (acf (1): −0.722, SD: −0.118, and SK: −0.335).
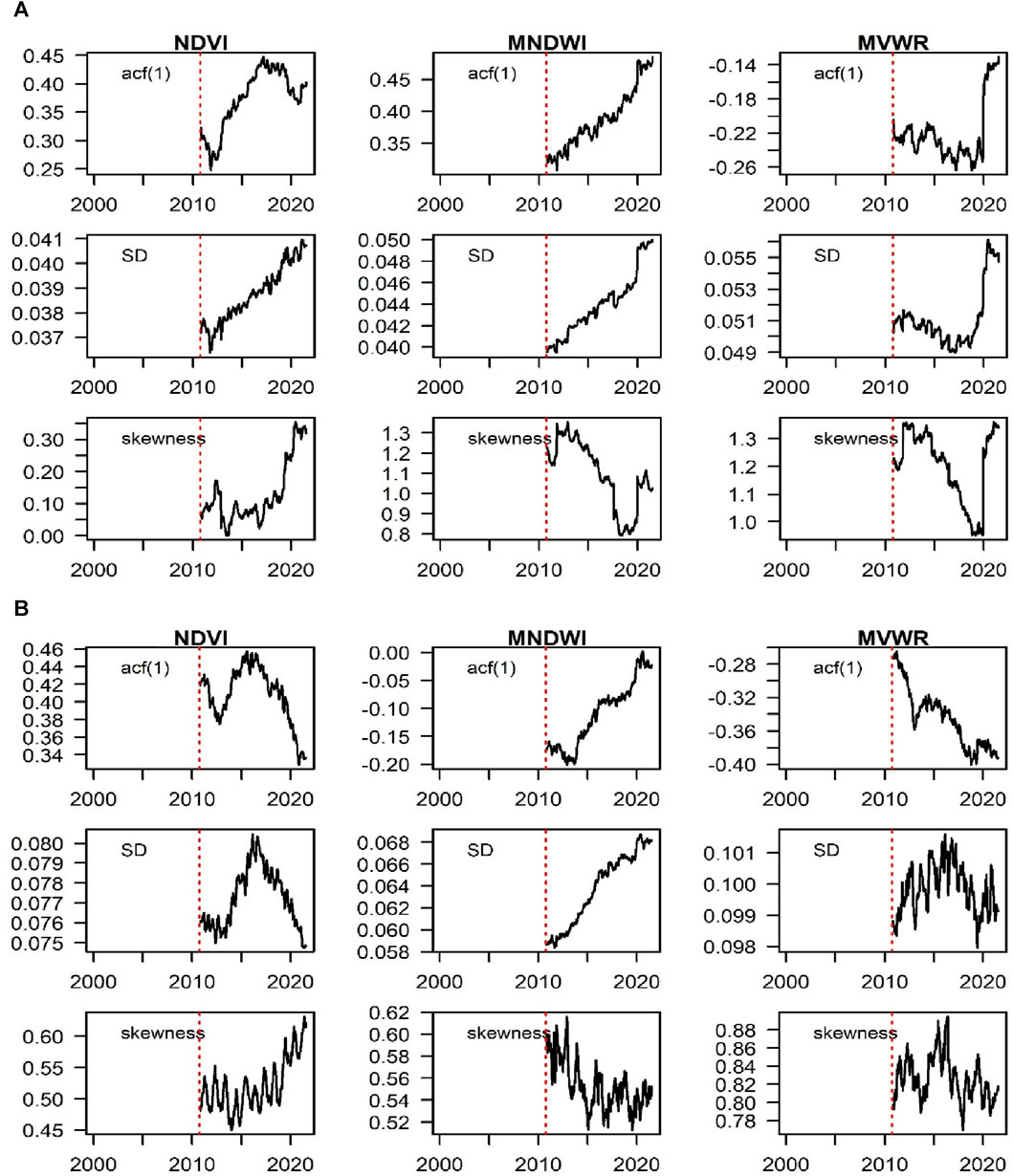
FIGURE 5. Early warning signals analysis using a time series of remotely sensed indices. The x-axis in each graph represents the remotely sensed indicator and the y-axis represents time. The first column represents the Normalized Difference Vegetation Index (NDVI), the second column represents the Modified Normalized Water Index (MNDWI), and the third column represents the Modified Vegetation Water Ratio (MVWR) from 18 February 2000, to 31 July 2021, at 500-m spatial resolution in Qeshm Island (A) and Gabrik (B). The red line illustrates the rolling window size of 50 percent which was used in the analysis. The acf (1) refers to autocorrelation at lag one, and SD refers to standard deviation.

TABLE 2. Trends in autocorrelation, standard deviation, and skewness changes for Qeshm island and Gabrik, notably significant at p < 0.1, in Normalized Difference Vegetation Index (NDVI), Modified Normalized Water Index (MNDWI), and Modified Vegetation Water Ratio (MVWR) from 18 February 2000, to 31 July 2021, analyzed using Kendall’s τ.
Contour plots (Figure 6) illustrate the impact of varying the width of the rolling window and applying Gaussian filtering on the discerned trend in the statistical metrics, as quantified by Kendall’s τ. Additionally, Supplementary Figures S1A, S2A depict histograms representing the frequency distribution of the trend statistic of the marked upside triangles in Figure 6. The results of the sensitivity analysis showed NDVI, MNDWI, and MVWR consistently presented low variation, indicating the robustness of the results (i.e., Kendall’s τ results) with different setting to perform the analysis such as rolling window widths and Gaussian filtering bandwidths.
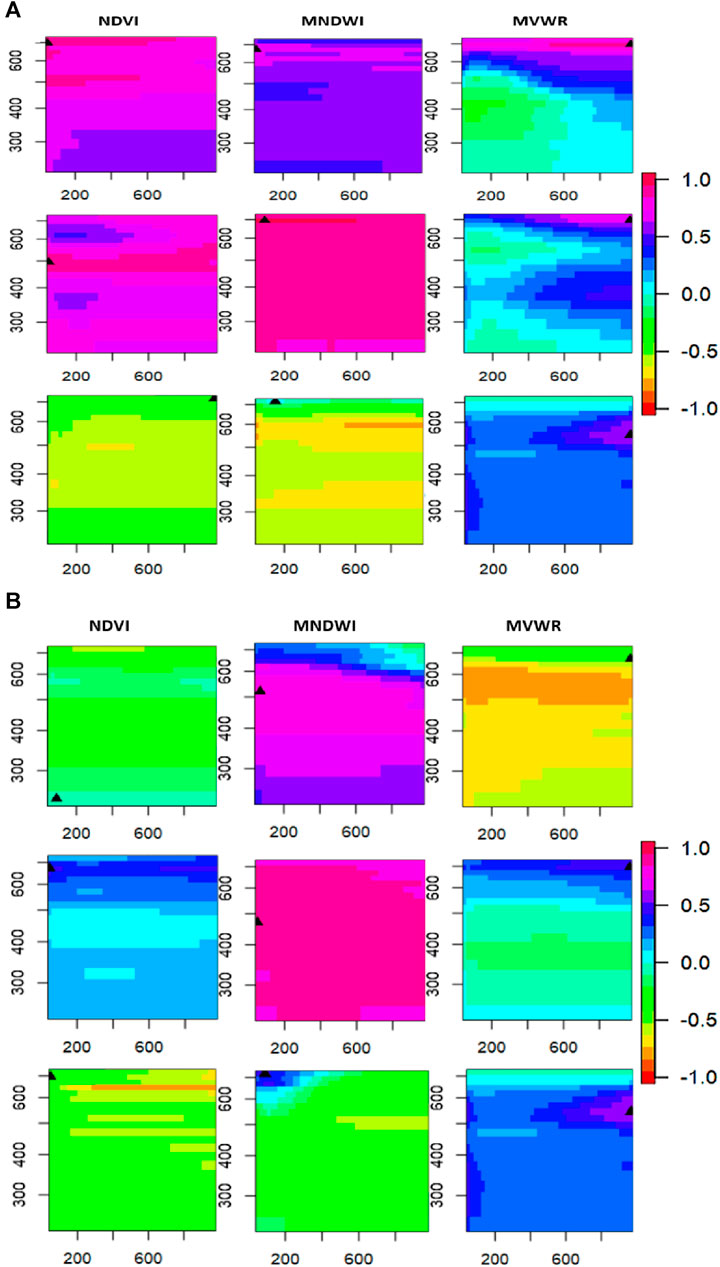
FIGURE 6. Sensitivity analysis of rolling window metrics. The x-axis in each graph represents the filtering bandwidth and the y-axis represents the rolling window size. The first column represents the Normalized Difference Vegetation Index (NDVI), the second column represents the Modified Normalized Water Index (MNDWI), and the third column represents the Modified Vegetation Water Ratio (MVWR) between 18 February 2000, and 31 July 2021, in Qeshm Island (A) and Gabrik (B). In each panel, the first row refers to autocorrelation (acf (1)), the first row refers to standard deviation, and the third row refers to skewness.
4 Discussion
This study illustrates the potential of remote sensing coupled with critical slowing down theory to explore the resilience of the mangrove forest ecosystem, which contributes to the state-of-the-art knowledge on monitoring of mangroves. While Qeshm Island and Gabrik share similarities in climate, geography, and annual rainfall (Figure 1 and Figure 2), the analysis of remote sensing indices, including NDVI, MNDWI, and MVWR, revealed significant differences between the two study areas (Figure 4). The time series of NDVI in Qeshm Island consistently showed increases in trends of change in autocorrelation, standard deviation, and skewness, opposite to Gabrik (Figure 5), which may signal a reduced resilience within the mangroves. Importantly, the increases in the trend of statistical metrics do not necessarily predict a state shift in the ecosystem’s state but rather suggest a potential decrease in resilience (Dakos et al., 2015). Although the results cannot directly warn of the upcoming transition in the mangroves’ state, the projection models anticipated the loss of up to half of the current mangrove areas (i.e., occurrence of tree less state) by the end of the 21st century due to the impending threats posed by disturbances (Mafi-Gholami et al., 2020). In addition, a comparison of three hybrid models to simulate land use change suggested a decline in the total area of mangrove forests (Kourosh Niya et al., 2020).
Qeshm Island’s mangroves face severe threats from pollution (including heavy metals and petroleum), biological stressors like algal blooms and fungi, and extensive land-use changes (Ebrahimi-Sirizi and Riyahi-Bakhtiyari, 2013; Hamzeh et al., 2021; Akbarzadeh-Chomachaei et al., 2023). In addition to all these stressors, we showed that the land-use and land-cover change presented by the expansion of built-up areas was significantly increased in Qeshm Island, compared with Gabrik (Table 1). Gabrik site is considered a protected area, and opposite to Qeshm Island, is shown to be less affected by pollution (Zarezadeh and Rezaee, 2016) and land-use land-cover-change (Table 1). Although the adverse impacts of the abovementioned disturbances (i.e., pollution and eutrophication, and biological stressors) on Qeshm Island are well documented, the area of mangroves exhibits an increase (Table 1). This observed expansion could be attributed to mangrove adaptation through landward migration and expansion (Etemadi et al., 2018) as well as reforestation projects in the regions (Mahmoudi et al., 2022; Farshid, Moradi Balef et al., 2023). Furthermore, the results showed not only has the area of mangroves increased during the past decade, but also the NDVI did not show declines (Figure 4). In fact, given previous reports of reduced resilience in the mangroves, one might have anticipated a decline in the NDVI time series. However, the absence of a decline in NDVI (Figure 4) does not necessarily indicate the health of the mangrove forest in the region. The elevation in eutrophication along with reported instances of algal blooming (Hamzeh et al., 2021; Mirza Esmaeili et al., 2021) can increase the NDVI (Rada et al., 2023). Hence, the pattern of NDVI trend (Figure 4) in the mangroves is most likely due to an increase in chlorophylls in water, following the increase in eutrophic level and algal blooming occurrences, not an increase in the tree covers or improvements in the mangrove forests health. NDVI is sensitive to greenness and vegetation health (Alatorre et al., 2016; Li et al., 2019; Alibakhshi, 2020; Cabello et al., 2021) and is widely utilized and validated in various studies. NDVI proves effective in assessing vegetation dynamics by capturing the reflectance of near-infrared (NIR) radiation and absorption of red light, indicative of healthy vegetation (Verbesselt et al., 2010a; Verbesselt et al., 2010b; Ruan et al., 2022; Tran et al., 2022).
Although the trend analyses of time series of NDVI cannot reflect the dynamics of mangroves in the study sites, the results of statistical metrics of NDVI provided valuable knowledge on the state of the mangroves’ resilience (Table 2). The increases in the trend of autocorrelation, standard deviation, and skewness (Table 2) in Qeshm Island may suggest mangrove forests are slow in recovering themself in Qeshm Island which is in line with previous studies (Ghanbarzad Dashti et al., 2021). The negative trend of change in autocorrelation, standard deviation, and skewness observed in the NDVI index in Gabrik suggests reliable signals and interpretations. Decreases in the trend of MVWR statistical metrics in Qeshm Island revealed that MVWR did not signal changes in mangroves’ resilience. Furthermore, the positive trend of autocorrelation and standard deviation values observed in the MNDWI index in Gabrik show limitations in the application of water indicators in presenting the state of the mangroves in the reference site. These findings might be attributed to the complexity of mangrove forest structures and also the adaptability of mangroves, particularly in response to variations in water areas (as indicated by MNDWI in this study) through landward migration (Etemadi et al., 2018).
In selecting the satellite data to perform the analyses, we strategically selected MODIS due to its advantages in terms of both temporal frequency and historical data availability. MODIS offers a longer archive of data along with high temporal resolution compared to other widely used satellite images such as Landsat and Sentinel, enabling us to capture dynamic changes in environmental conditions. The relatively low sensitivity of NDVI, MNDWI, and MVWR as early warning signal generators in mangrove forests has been depicted in Figure 6. More specifically, the consistently high Kendall’s τ observed in NDVI, even under varying conditions of rolling window widths and Gaussian filtering bandwidths may suggest its stability and reliability in presenting the state of the ecosystem. This robustness may be attributed to NDVI’s sensitivity to vegetation changes, making it less prone to fluctuations introduced by the applied methodologies.
Despite the significance of the results presented in this study, it is essential to acknowledge certain limitations. Firstly, the analysis solely relies on remote sensing data, which may have limitations in accurately capturing certain ecological processes and dynamics at a finer spatial scale. Additionally, the study focused on a specific region (Qeshm Island and Gabrik), limiting the generalizability of the results to other mangrove forest ecosystems. However, it should be noted that, due to the current political situation in some countries such as Iran, accessing field data is difficult, and thus the common understanding of ecosystem dynamics is strongly based on satellite data.
The results of this study have critical implications for biodiversity, ecosystem resilience, and socio-economic factors. Mangrove forest ecosystems are crucial for biodiversity conservation, harbouring a diverse range of plant and animal species and providing vital habitats and breeding grounds for numerous marine and avian organisms, contributing to the overall ecological resilience of coastal ecosystems (Nagelkerken et al., 2000; Lugendo et al., 2007; Kathiresan, 2012). Early warning signals offer opportunities for proactive conservation to maintain biodiversity and ecosystem stability. By providing a robust approach that can effectively evaluate the state of the mangrove forests, combined with a thorough conservation and management system, it is possible to effectively monitor and enhance the state of mangrove forests and safeguard the wellbeing of the communities dependent on these forests.
5 Conclusion
This study contributes to the state-of-the-art knowledge on the monitoring of mangroves and provides new information on the early warning signals of reduced resilience in mangrove forests. Utilizing remote sensing analysis and time series analysis offers substantial opportunities to enhance the global capacity for monitoring the state of mangrove ecosystems. The utilization of remote sensing indices, particularly NDVI, emerges as a robust indicator of ecosystem dynamics. NDVI outperforms MVWR and MNDWI in generating early warning signals of critical transition. Furthermore, the study highlights significant differences between the mangrove forests of Qeshm Island and Gabrik, with Qeshm Island exhibiting more pronounced fluctuations and variability in ecosystem dynamics. The practical implications of this study involve informing policy frameworks and international initiatives for the conservation and sustainable management of mangrove forest ecosystems. To promote the conservation of mangrove forest ecosystems, several policy recommendations can be made. Strict regulations and land use planning should control urban expansion, particularly in sensitive coastal regions like Qeshm Island. Buffer zones and protected areas must be established. Sustainable agricultural practices and reduced use of harmful chemicals are essential. Raising public awareness through education programs is crucial, along with fostering collaboration between governmental organizations, research institutions, and local communities to develop integrated management plans. The findings highlight the need for region-specific conservation approaches and highlight the value and vulnerability of these ecosystems.
Data availability statement
The raw data supporting the conclusion of this article will be made available by the authors, without undue reservation. The land-use and land-cover change data utilized in this study were obtained from Kourosh Niya (Kourosh Niya et al., 2019).
Author contributions
SA: Conceptualization, Formal Analysis, Validation, Visualization, Writing–original draft, Writing–review and editing. HA: Writing–review and editing. LE-L: Writing–review and editing.
Funding
The author(s) declare financial support was received for the research, authorship, and/or publication of this article. This research was supported by the Finnish Research Impact Foundation.
Acknowledgments
This study is inspired by a previous study conducted by SA (Alibakhshi et al., 2017). We extend our sincere gratitude to Kourosh Niya for generously providing the data and background knowledge about study sites. Additionally, we would like to express our appreciation to Prof. Jinliang Huang and Xiamen University for their support, assistance, and contributions to this study. This research was supported by the Finnish Research Impact Foundation.
Conflict of interest
The authors declare that the research was conducted in the absence of any commercial or financial relationships that could be construed as a potential conflict of interest.
Publisher’s note
All claims expressed in this article are solely those of the authors and do not necessarily represent those of their affiliated organizations, or those of the publisher, the editors and the reviewers. Any product that may be evaluated in this article, or claim that may be made by its manufacturer, is not guaranteed or endorsed by the publisher.
Supplementary material
The Supplementary Material for this article can be found online at: https://www.frontiersin.org/articles/10.3389/feart.2024.1317188/full#supplementary-material
References
Abrantes, K. G., Johnston, R., Connolly, R. M., and Sheaves, M. (2015). Importance of mangrove carbon for aquatic food webs in wet–dry tropical estuaries. Estuaries Coasts 38, 383–399. doi:10.1007/s12237-014-9817-2
Akbarzadeh-Chomachaei, G., Koohkan, H., Dehghani, R., Mortazavi, M., and Gozari, M. (2023). Comparison of heavy metals pollution in coastal sediments of bandar abbas, Qeshm island and hormuz-lark, Persian Gulf. Int. J. Environ. Sci. Technol. 20 (10), 10861–10876. doi:10.1007/s13762-023-05098-y
Alatorre, L. C., Sánchez-Carrillo, S., Miramontes-Beltrán, S., Medina, R. J., Torres-Olave, M. E., Bravo, L. C., et al. (2016). Temporal changes of NDVI for qualitative environmental assessment of mangroves: shrimp farming impact on the health decline of the arid mangroves in the Gulf of California (1990–2010). J. Arid Environ. 125, 98–109. doi:10.1016/j.jaridenv.2015.10.010
Alibakhshi, S. (2020). Remotely sensed monitoring of land surface albedo and ecosystem dynamics. Aalto University publication series DOCTORAL DISSERTATIONS, 183/2020.
Alibakhshi, S. (2023). A robust approach and analytical tool for identifying early warning signals of forest mortality. Ecol. Indic. 155, 110983. doi:10.1016/j.ecolind.2023.110983
Alibakhshi, S., Groen, T. A., Rautiainen, M., and Naimi, B. (2017). Remotely-sensed early warning signals of a critical transition in a wetland ecosystem. Remote Sens. 9 (4), 352. doi:10.3390/rs9040352
Barbier, E. B., Koch, E. W., Silliman, B. R., Hacker, S. D., Wolanski, E., Primavera, J., et al. (2008). Coastal ecosystem-based management with nonlinear ecological functions and values. science 319 (5861), 321–323. doi:10.1126/science.1150349
Bevan, J. M., and Kendall, M. G. (1971). Rank correlation methods. Statistician 20 (3), 74. doi:10.2307/2986801
Cabello, K., Germentil, M., Blanco, A., Macatulad, E., and Salmo, S. (2021). Post-disaster assessment of mangrove forest recovery in lawaan-balangiga, eastern samar using ndvi time series analysis. Remote Sens. Spatial Inf. Sci. 3, 243–250. ISPRS Annals of the Photogrammetry. doi:10.5194/isprs-annals-v-3-2021-243-2021
Capon, S. J., Lynch, A. J. J., Bond, N., Chessman, B. C., Davis, J., Davidson, N., et al. (2015). Regime shifts, thresholds and multiple stable states in freshwater ecosystems; a critical appraisal of the evidence. Sci. Total Environ. 534, 122–130. doi:10.1016/j.scitotenv.2015.02.045
Carpenter, S. R., and Brock, W. A. (2006). Rising variance: a leading indicator of ecological transition. Ecol. Lett. 9 (3), 311–318. doi:10.1111/j.1461-0248.2005.00877.x
Carpenter, S. R., and Brock, W. A. (2011). Early warnings of unknown nonlinear shifts: a nonparametric approach. Ecology 92 (12), 2196–2201. doi:10.1890/11-0716.1
Carpenter, S. R., Brock, W. A., Cole, J. J., Kitchell, J. F., and Pace, M. L. (2008). Leading indicators of trophic cascades. Ecol. Lett. 11 (2), 128–138. doi:10.1111/j.1461-0248.2007.01131.x
Carpenter, S. R., Cole, J. J., Pace, M. L., Batt, R., Brock, W. A., Cline, T., et al. (2011). Early warnings of regime shifts: a whole-ecosystem experiment. Science 332 (6033), 1079–1082. doi:10.1126/science.1203672
Chen, Y., Huang, C., Ticehurst, C., Merrin, L., and Thew, P. (2013). An evaluation of MODIS daily and 8-day composite products for floodplain and wetland inundation mapping. Wetlands 33 (5), 823–835. doi:10.1007/s13157-013-0439-4
Dahdouh-Guebas, F., Jayatissa, L. P., Di Nitto, D., Bosire, J. O., Seen, D. L., and Koedam, N. (2005). How effective were mangroves as a defence against the recent tsunami? Curr. Biol. 15 (12), R443–R447. doi:10.1016/j.cub.2005.06.008
Dakos, V., Carpenter, S. R., Brock, W. A., Ellison, A. M., Guttal, V., Ives, A. R., et al. (2012a). Methods for detecting early warnings of critical transitions in time series illustrated using simulated ecological data. PLoS ONE 7 (7), e41010. doi:10.1371/journal.pone.0041010
Dakos, V., Carpenter, S. R., van Nes, E. H., and Scheffer, M. (2015). Resilience indicators: prospects and limitations for early warnings of regime shifts. Philosophical Trans. R. Soc. B Biol. Sci. 370 (1659), 20130263. doi:10.1098/rstb.2013.0263
Dakos, V., Van Nes, E. H., D'Odorico, P., and Scheffer, M. (2012b). Robustness of variance and autocorrelation as indicators of critical slowing down. Ecology 93 (2), 264–271. doi:10.1890/11-0889.1
Daru, B. H., Yessoufou, K., Mankga, L. T., and Davies, T. J. (2013). A global trend towards the loss of evolutionarily unique species in mangrove ecosystems. PloS one 8 (6), e66686. doi:10.1371/journal.pone.0066686
Duke, N. C., Meynecke, J.-O., Dittmann, S., Ellison, A. M., Anger, K., Berger, U., et al. (2007). A world without mangroves? Science 317 (5834), 41–42. doi:10.1126/science.317.5834.41b
Ebrahimi-Sirizi, Z., and Riyahi-Bakhtiyari, A. (2013). Petroleum pollution in mangrove forests sediments from Qeshm island and khamir port—Persian Gulf, Iran. Environ. Monit. Assess. 185, 4019–4032. doi:10.1007/s10661-012-2846-z
Ellison, A. M. (2008). Managing mangroves with benthic biodiversity in mind: moving beyond roving banditry. J. Sea Res. 59 (1-2), 2–15. doi:10.1016/j.seares.2007.05.003
Etemadi, H., Smoak, J. M., and Sanders, C. J. (2018). Forest migration and carbon sources to Iranian mangrove soils. J. Arid Environ. 157, 57–65. doi:10.1016/j.jaridenv.2018.06.005
Farshid, Z., Moradi Balef, R., Zendehboudi, T., Dehghan, N., Mohajer, F., Kalbi, S., et al. (2023). Reforestation of grey mangroves (Avicennia marina) along the northern coasts of the Persian Gulf. Wetl. Ecol. Manag. 31 (1), 115–128. doi:10.1007/s11273-022-09904-1
Ghanbarzad Dashti, S., Farzingohar, M., and Souri, A. (2021). Temperature and salinity effects in sensitive area of Qeshm island: mangrove forests. Int. J. Coast. Offshore Environ. Eng. 6 (4), 13–18. doi:10.22034/IJCOE.2021.150792
Giri, C., Ochieng, E., Tieszen, L., Zhu, Z., Singh, A., Loveland, T., et al. (2013)."Global mangrove forests distribution, 2000." NASA Socioeconomic Data and Applications Center (SEDAC), Palisades. doi 10: H4J67DW68.
Giri, C., Ochieng, E., Tieszen, L. L., Zhu, Z., Singh, A., Loveland, T., et al. (2011). Status and distribution of mangrove forests of the world using earth observation satellite data. Glob. Ecol. Biogeogr. 20 (1), 154–159. doi:10.1111/j.1466-8238.2010.00584.x
Gorelick, N., Hancher, M., Dixon, M., Ilyushchenko, S., Thau, D., and Moore, R. (2017). Google earth engine: planetary-scale geospatial analysis for everyone. Remote Sens. Environ. 202, 18–27. doi:10.1016/j.rse.2017.06.031
Goudarzi, A., and Moslehi, M. (2020). Distribution of a devastating fungal pathogen in mangrove forests of southern Iran. Crop Prot. 128, 104987. doi:10.1016/j.cropro.2019.104987
Guttal, V., and Jayaprakash, C. (2008). Changing skewness: an early warning signal of regime shifts in ecosystems. Ecol. Lett. 11 (5), 450–460. doi:10.1111/j.1461-0248.2008.01160.x
Hamzeh, M. A., Koochaknejad, E., and Hamzei, S. (2021). Historical eutrophication and pollution records off Bandar Abbas coast (North of Strait of Hormuz) using benthic foraminiferal ecology and geochemistry of trace elements from a sediment core. Regional Stud. Mar. Sci. 47, 101929. doi:10.1016/j.rsma.2021.101929
Hirota, M., Holmgren, M., Van Nes, E. H., and Scheffer, M. (2011). Global resilience of tropical forest and savanna to critical transitions. Science 334 (6053), 232–235. doi:10.1126/science.1210657
Ji, L., Zhang, L., and Wylie, B. (2009). Analysis of dynamic thresholds for the normalized difference water index. Photogrammetric Eng. Remote Sens. 75 (11), 1307–1317. doi:10.14358/pers.75.11.1307
Kathiresan, K. (2012). Importance of mangrove ecosystem. Int. J. Mar. Sci. 2 (10). doi:10.5376/ijms.2012.02.0010
Kéfi, S., Guttal, V., Brock, W. A., Carpenter, S. R., Ellison, A. M., Livina, V. N., et al. (2014). Early warning signals of ecological transitions: methods for spatial patterns. PLoS ONE 9 (3), e92097. doi:10.1371/journal.pone.0092097
Kirui, K., Kairo, J. G., Bosire, J., Viergever, K. M., Rudra, S., Huxham, M., et al. (2013). Mapping of mangrove forest land cover change along the Kenya coastline using Landsat imagery. Ocean Coast. Manag. 83, 19–24. doi:10.1016/j.ocecoaman.2011.12.004
Kourosh Niya, A., Huang, J., Karimi, H., Keshtkar, H., and Naimi, B. (2019). Use of intensity analysis to characterize land use/cover change in the biggest Island of Persian Gulf, Qeshm Island, Iran. Sustainability 11 (16), 4396. doi:10.3390/su11164396
Kourosh Niya, A., Huang, J., Kazemzadeh-Zow, A., Karimi, H., Keshtkar, H., and Naimi, B. (2020). Comparison of three hybrid models to simulate land use changes: a case study in Qeshm Island, Iran. Environ. Monit. Assess. 192, 302–319. doi:10.1007/s10661-020-08274-6
Lenton, T. M. (2011). Early warning of climate tipping points. Nat. Clim. Change 1 (4), 201–209. doi:10.1038/nclimate1143
Lenton, T. M., Held, H., Kriegler, E., Hall, J. W., Lucht, W., Rahmstorf, S., et al. (2008). Tipping elements in the Earth's climate system. Proc. Natl. Acad. Sci. U. S. A. 105 (6), 1786–1793. doi:10.1073/pnas.0705414105
Lenton, T. M., Livina, V. N., Dakos, V., Van Nes, E. H., and Scheffer, M. (2012). Early warning of climate tipping points from critical slowing down: comparing methods to improve robustness. Philosophical Trans. R. Soc. A Math. Phys. Eng. Sci. 370, 1185–1204. doi:10.1098/rsta.2011.0304
Li, H., Jia, M., Zhang, R., Ren, Y., and Wen, X. (2019). Incorporating the plant phenological trajectory into mangrove species mapping with dense time series Sentinel-2 imagery and the Google Earth Engine platform. Remote Sens. 11 (21), 2479. doi:10.3390/rs11212479
Li, W., Chen, Q., Cai, D., and Li, R. (2015). Determination of an appropriate ecological hydrograph for a rare fish species using an improved fish habitat suitability model introducing landscape ecology index. Ecol. Model. 311, 31–38. doi:10.1016/j.ecolmodel.2015.05.009
Lugendo, B. R., Nagelkerken, I., Kruitwagen, G., Van Der Velde, G., and Mgaya, Y. D. (2007). Relative importance of mangroves as feeding habitats for fishes: a comparison between mangrove habitats with different settings. Bull. Mar. Sci. 80 (3), 497–512.
Luther, D. A., and Greenberg, R. (2009). Mangroves: a global perspective on the evolution and conservation of their terrestrial vertebrates. BioScience 59 (7), 602–612. doi:10.1525/bio.2009.59.7.11
Mafi-Gholami, D., Zenner, E. K., Jaafari, A., and Bui, D. T. (2020). Spatially explicit predictions of changes in the extent of mangroves of Iran at the end of the 21st century. Estuar. Coast. Shelf Sci. 237, 106644. doi:10.1016/j.ecss.2020.106644
Mahmoudi, B., Mafi-Gholami, D., and Ng, E. (2022). Evaluation of mangrove rehabilitation and afforestation in the southern coasts of Iran. Estuar. Coast. Shelf Sci. 277, 108086. doi:10.1016/j.ecss.2022.108086
Martínez, M. L., Intralawan, A., Vázquez, G., Pérez-Maqueo, O., Sutton, P., and Landgrave, R. (2007). The coasts of our world: ecological, economic and social importance. Ecol. Econ. 63 (2-3), 254–272. doi:10.1016/j.ecolecon.2006.10.022
Mazraeh, H. M., and Pazhouhanfar, M. (2018). Effects of vernacular architecture structure on urban sustainability case study: Qeshm Island, Iran. Front. Archit. Res. 7 (1), 11–24. doi:10.1016/j.foar.2017.06.006
Mirza Esmaeili, F., Mortazavi, M. S., Dehghan Banadaki, A., Saraji, F., and Mohebbi Nozar, S. L. (2021). Algal blooms historical outbreaks in the northern coastal waters of the Persian Gulf and Oman Sea (1980–2015). Environ. Monit. Assess. 193, 648–712. doi:10.1007/s10661-021-09413-3
Moslehi, M., Bernier, L., Zakeri, O., and Ahmadi, A. (2023). First report of Streblote solitaria (Lepidoptera: lasiocampidae) damage on Avicennia marina trees in southern mangroves of Iran. Acta Ecol. Sin. doi:10.1016/j.chnaes.2023.08.008
Mozumder, C., Tripathi, N. K., and Tipdecho, T. (2014). Ecosystem evaluation (1989–2012) of Ramsar wetland Deepor Beel using satellite-derived indices. Environ. Monit. Assess. 186 (11), 7909–7927. doi:10.1007/s10661-014-3976-2
Muñoz Sabater, J. (2019). ERA5-land monthly averaged data from 1981 to present, copernicus climate change service (C3S) climate data store (CDS). Earth Syst. Sci. Data 55, 5679–5695.
Naderloo, R., Türkay, M., and Sari, A. (2013). Intertidal habitats and decapod (Crustacea) diversity of Qeshm Island, a biodiversity hotspot within the Persian Gulf. Mar. Biodivers. 43, 445–462. doi:10.1007/s12526-013-0174-3
Nagelkerken, I., Van der Velde, G., Gorissen, M., Meijer, G., Van't Hof, T., and Den Hartog, C. (2000). Importance of mangroves, seagrass beds and the shallow coral reef as a nursery for important coral reef fishes, using a visual census technique. Estuar. Coast. shelf Sci. 51 (1), 31–44. doi:10.1006/ecss.2000.0617
Pinheiro, J., Bates, D., DebRoy, S., and Sarkar, D. (2000). “The R development core team, 2013,” in NLME: linear and nonlinear mixed effects models, 1–3.
Polidoro, B. A., Carpenter, K. E., Collins, L., Duke, N. C., Ellison, A. M., Ellison, J. C., et al. (2010). The loss of species: mangrove extinction risk and geographic areas of global concern. PloS one 5 (4), e10095. doi:10.1371/journal.pone.0010095
Qgis, S. (2016). QGIS geographic information system v.2.16.3. Open Source Geospatial Foundation. Available at: http://qgis.osgeo.org.
Rada, A., Nikitina, O., and Syrova, M. (2023). “Estimation of the level of eutrophication of coastal waters of the Baltic Sea on the basis of Earth remote sensing data,” in E3S Web of Conferences, EDP Sciences.
Rokni, K., Ahmad, A., Selamat, A., and Hazini, S. (2014). Water feature extraction and change detection using multitemporal landsat imagery. Remote Sens. 6 (5), 4173–4189. doi:10.3390/rs6054173
Ruan, L., Yan, M., Zhang, L., Fan, X., and Yang, H. (2022). Spatial-temporal NDVI pattern of global mangroves: a growing trend during 2000–2018. Sci. Total Environ. 844, 157075. doi:10.1016/j.scitotenv.2022.157075
Scheffer, M. (1990). Multiplicity of stable states in freshwater systems. Hydrobiologia, 200-201. Springer, 475–486.
Scheffer, M. (2001). Alternative attractors of shallow lakes. TheScientificWorldJournal 1, 254–263. doi:10.1100/tsw.2001.62
Scheffer, M., Bascompte, J., Brock, W. A., Brovkin, V., Carpenter, S. R., Dakos, V., et al. (2009). Early-warning signals for critical transitions. Nature 461 (7260), 53–59. doi:10.1038/nature08227
Scheffer, M., Carpenter, S., Foley, J. A., Folke, C., and Walker, B. (2001). Catastrophic shifts in ecosystems. Nature 413 (6856), 591–596. doi:10.1038/35098000
Sorichetta, A., Hornby, G. M., Stevens, F. R., Gaughan, A. E., Linard, C., and Tatem, A. J. (2015). High-resolution gridded population datasets for Latin America and the Caribbean in 2010, 2015, and 2020. Sci. data 2 (1), 150045–150112. doi:10.1038/sdata.2015.45
Tehrani, N. A., and Janalipour, M. (2021). Predicting ecosystem shift in a Salt Lake by using remote sensing indicators and spatial statistics methods (case study: lake Urmia basin). Environ. Eng. Res. 26 (4). doi:10.4491/eer.2020.225
Tran, T. V., Reef, R., and Zhu, X. (2022). A review of spectral indices for mangrove remote sensing. Remote Sens. 14 (19), 4868. doi:10.3390/rs14194868
Verbesselt, J., Hyndman, R., Newnham, G., and Culvenor, D. (2010a). Detecting trend and seasonal changes in satellite image time series. Remote Sens. Environ. 114 (1), 106–115. doi:10.1016/j.rse.2009.08.014
Verbesselt, J., Hyndman, R., Zeileis, A., and Culvenor, D. (2010b). Phenological change detection while accounting for abrupt and gradual trends in satellite image time series. Remote Sens. Environ. 114 (12), 2970–2980. doi:10.1016/j.rse.2010.08.003
Verbesselt, J., Umlauf, N., Hirota, M., Holmgren, M., Van Nes, E. H., Herold, M., et al. (2016). Remotely sensed resilience of tropical forests. Nat. Clim. Change 6 (11), 1028–1031. doi:10.1038/nclimate3108
Wang, F., Zhang, J., Cao, Y., Wang, R., Kattel, G., He, D., et al. (2023). Pattern changes and early risk warning of Spartina alterniflora invasion: a study of mangrove-dominated wetlands in northeastern Fujian, China. J. For. Res. 34, 1447–1462. doi:10.1007/s11676-023-01604-7
Yessoufou, K., and Stoffberg, G. (2016). Biogeography, threats and phylogenetic structure of mangrove forest globally and in South Africa: a review. South Afr. J. Bot. 107, 114–120. doi:10.1016/j.sajb.2015.11.002
Zahed, M. A., Rouhani, F., Mohajeri, S., Bateni, F., and Mohajeri, L. (2010). An overview of Iranian mangrove ecosystems, northern part of the Persian Gulf and Oman Sea. Acta Ecol. Sin. 30 (4), 240–244. doi:10.1016/j.chnaes.2010.03.013
Keywords: land-use and land-cover change, monitoring ecosystem dynamics, remote sensing, mangrove forests, reduced resilience
Citation: Alibakhshi S, Azadi H and Espinosa-Leal L (2024) Can land-use and land-cover change explain reduced resilience in forests?. Front. Earth Sci. 12:1317188. doi: 10.3389/feart.2024.1317188
Received: 10 October 2023; Accepted: 07 February 2024;
Published: 08 March 2024.
Edited by:
Wenkai Li, Sun Yat-sen University, ChinaReviewed by:
Mohamed Elhag, King Abdulaziz University, Saudi ArabiaZhen Gao, Guangdong Academy of Sciences, China
Copyright © 2024 Alibakhshi, Azadi and Espinosa-Leal. This is an open-access article distributed under the terms of the Creative Commons Attribution License (CC BY). The use, distribution or reproduction in other forums is permitted, provided the original author(s) and the copyright owner(s) are credited and that the original publication in this journal is cited, in accordance with accepted academic practice. No use, distribution or reproduction is permitted which does not comply with these terms.
*Correspondence: Sara Alibakhshi, sara.alibakhshi@gmail.com