The impact of large language models on higher education: exploring the connection between AI and Education 4.0
- 1School of Humanities and Education, Tecnologico de Monterrey, Monterrey, Mexico
- 2School of Psychology, Universidad César Vallejo, Lima, Peru
- 3Institute for the Future of Education, Tecnologico de Monterrey, Monterrey, Mexico
The digital transformation has profoundly affected every facet of human life, with technological advancements potentially reshaping the economy, society, and our daily living and working modalities. Artificial Intelligence (AI), particularly Generative AI (GAI), has emerged as a pivotal disruption in education, showcasing the capability to produce diverse and context-relevant content. Generative Artificial Intelligence (GAI) has revolutionized natural language processing, computer vision, and creative arts. Large language models (LLMs) like GPT-4 and Open Assistant and tools like DALL-E and Midjourney for the visual and creative domain are increasingly used for various tasks by students and others with critical information needs. AI presents novel avenues for crafting effective learning activities and developing enhanced technology-driven learning applications in the educational sector. However, integrating AI with a pedagogical focus pose challenge. Education 4.0, which integrates emerging technologies and innovative strategies, aims to prepare new generations for a technologically fluid world. This systematic literature review aims to analyze the use of LLMs in higher education within the context of Education 4.0’s pedagogical approaches, identifying trends and challenges from a selection of 83 relevant articles out of an initial set of 841 papers. The findings underscore the significant potential of LLMs to enrich higher education, aligning with Education 4.0 by fostering more autonomous, collaborative, and interactive learning. It highlights the necessity for human oversight to ensure the quality and accuracy of AI-generated content. It addresses ethical and legal challenges to ensure equitable implementation, suggesting an exploration of LLM integration that complements human interaction while maintaining academic integrity and pedagogical foundation.
1 Introduction
The impact of digital transformation is felt in all aspects of people’s lives (Zaoui and Souissi, 2020). Recent technological advancements can transform the economy, society, and people’s lives and work (Rosalina et al., 2021). Around the world, there is a growing momentum toward technological and industrial transformation, driven by the widespread adoption of cutting-edge information and communication technologies (Zhang and Lu, 2021; Glasserman-Morales et al., 2023).
Digital transformation has also impacted education, as education needs to meet the demands and challenges of the digital and global society (Miranda et al., 2021). For instance, Suarez-Brito et al. (2022) argue that education must adapt to meet the needs of qualification and skills development in a 21st century with diverse challenges in problem solving along with creative thinking skills (CTS), as a means of long-lasting, significant learning (Forte-Celaya et al., 2021). This transformation is particularly evident in higher education, which has led to significant changes (Udovita, 2020). Factors driving this transformation include the accelerated introduction of emerging digital technologies, the creation of innovative systems for delivering education, revamped economic models, and the evolving educational expectations of a new generation of students who are digital natives (Gillpatrick, 2020).
Higher education institutions are now in a disruptive environment due to digital transformation, marked by new business models and greater interaction with digital technologies (Benavides et al., 2020). A disruptive technology that has demonstrated its potential to develop various sectors like the industry (Angelov et al., 2021) and education is Artificial Intelligence (AI) (Zmyzgova et al., 2020). AI offers a variety of benefits, including personalized learning, increased efficiency, and improved student outcomes (Zhai et al., 2021; Hariri, 2023).
The potential of AI in education is vast. It encompasses various areas that can transform the way teaching and learning are conducted (Shrivastava et al., 2023), creating new opportunities to design productive learning activities and develop better technology-enhanced learning applications or environments (Sanabria-Navarro et al., 2023). The availability of generative AI systems like GPT-4, Open Assistant, DALL-E, Midjourney, and many others has changed the landscape of information acquisition and learning (Biri et al., 2023; Walczak and Cellary, 2023).
The use of GAI has attracted the interest of education professionals, such as educators and researchers, generating significant debate, with particular concern for the correct incorporation of tools such as large language models (LLMs), as these models can perform a wide range of linguistic tasks (Ülkü, 2023). From a positive viewpoint, LLMs have the potential to enhance educational efficacy and are perceived as valuable tools in educational settings (Prentzas and Sidiropoulou, 2023). These models offer significant opportunities to improve education through teaching assistance, fostering creativity, democratizing access to educational technology, and adapting to specific educational contexts (Li et al., 2023).
From this scenario, integrating LLMs into education requires carefully considering how these tools can affect teaching and student learning (Huang et al., 2023a,b). Furthermore, it is essential to direct a pedagogical intent when implementing these emerging technologies. The integration of AI, especially LLMs, should not be based solely on excitement for technological innovation or operational efficiency they may provide. Instead, their implementation must be guided by a clearly defined educational objective to ensure these technologies enhance the academic experience. This approach guarantees that integrating LLMs aligns with pedagogical goals and effectively contributes to the teaching and learning process (Sanabria-Navarro et al., 2023).
Thus, the LLM revolution in higher education is creating a critical milestone in research (Huang et al., 2023b). It is necessary to understand which pedagogical approaches can support the correct integration of these LLMs, which should be seen as a complement that enriches the learning process, not as a substitute for human teaching (Bauer et al., 2023). Therefore, this research aims to provide an overview of current research related to implementing LLMs in higher education and to demonstrate their connections with Education 4.0 pedagogical principles. Furthermore, the study aims to outline the challenges and implications of this technology in higher education institutions.
2 Theoretical framework
Artificial Intelligence (AI) constitutes a branch of computer science dedicated to developing technologies capable of simulating human reasoning (Fox and Leake, 2001; Deng, 2018; Harika et al., 2022). This field focuses on knowledge as its main object of study, from acquisition to analysis and expression, utilizing these methods to simulate human intellectual activities (Da Xu et al., 2021). Integrating disciplines such as computational science, logic, biology, psychology, and philosophy, AI has made significant advances in areas like voice recognition, image processing, natural language processing, and intelligent robotics, playing a crucial role in social development, work efficiency, labor cost reduction, optimization of human resources, and the creation of new job demands (Zhang and Lu, 2021).
The formal exploration of AI began in the 1950s, specifically during the Dartmouth conference (Sanabria-Navarro et al., 2023), where it was established that “every aspect of learning or any other feature of intelligence can be so precisely described, that a machine can be made to simulate it” (McCarthy et al., 2006). The history of AI, spanning over 70 years, shows a development in stages from the artificial neuron model in 1943 to recent advances in deep learning and voice and image recognition (Zhang and Chen, 2020; Alhwaiti, 2023). Daily applications of AI include spam filters in emails, facial recognition systems to unlock mobile devices, recommendation algorithms on services like Netflix, Spotify, and Amazon (Fontes et al., 2021; Khandelwal, 2023), as well as virtual assistants (e.g., Siri and Amazon’s Alexa), among others. These applications are supported by underlying technologies that enable AI to function (Sikdar, 2018; Brill et al., 2019).
For AI to perform complex operations, it relies on various technologies that contribute to generating intelligence that seeks to emulate human intelligence. Among these technologies are: (a) Machine Learning, which focuses on developing statistical algorithms designed to learn from data and improve performance on specific tasks (Mühlhoff, 2020); (b) Neural networks, algorithms inspired by the structure and function of the human brain to recognize patterns, comprised of artificial neurons (nodes) organized in layers, processing input data through mathematical functions and generating output (Majewski and Kacalak, 2006); and (c) Deep Learning, which is based on neural networks with multiple data processing layers, allowing for higher levels of data abstraction and significantly contributing to voice, image, and natural language recognition (Bengio et al., 2021).
The latest advancements in Machine Learning and Deep Learning have extended traditional data-driven AI tasks, such as predictions, classifications, or recommendations, toward generating unique, realistic, and creative content. The concept of Generative Artificial Intelligence (GAI) emerges as a transformative force in the digital environment (Banh and Strobel, 2023). GAI represents a significant advancement in AI, focused on creating new and original content, including text, images, music, and videos (Shah, 2023; Zhuhadar and Lytras, 2023).
Deep Generative Models (DGMs) have emerged as a class of Deep Learning models for generating new content based on existing data, creating various possibilities for AI applications (Lehmann and Buschek, 2021). These models are trained to understand complex data distributions, allowing them to produce outcomes closely resembling real-world data (Tomczak, 2022), in contrast to generative models like hidden Markov models or Bayesian networks that attempt to model statistical problems involving time series or sequences (Harshvardhan et al., 2020).
The substantial improvement of DGMs is thanks to Generative Adversarial Networks (GANs), which have shown remarkable potential in generating content close to human production (Liu et al., 2021; Lamba et al., 2023). Among the most notable applications of GAI is the large language model (LLM), specifically designed to understand, interpret, generate, and manage text in natural language on a large scale. The scenario from AI to GAI concepts can be observed in Figure 1.
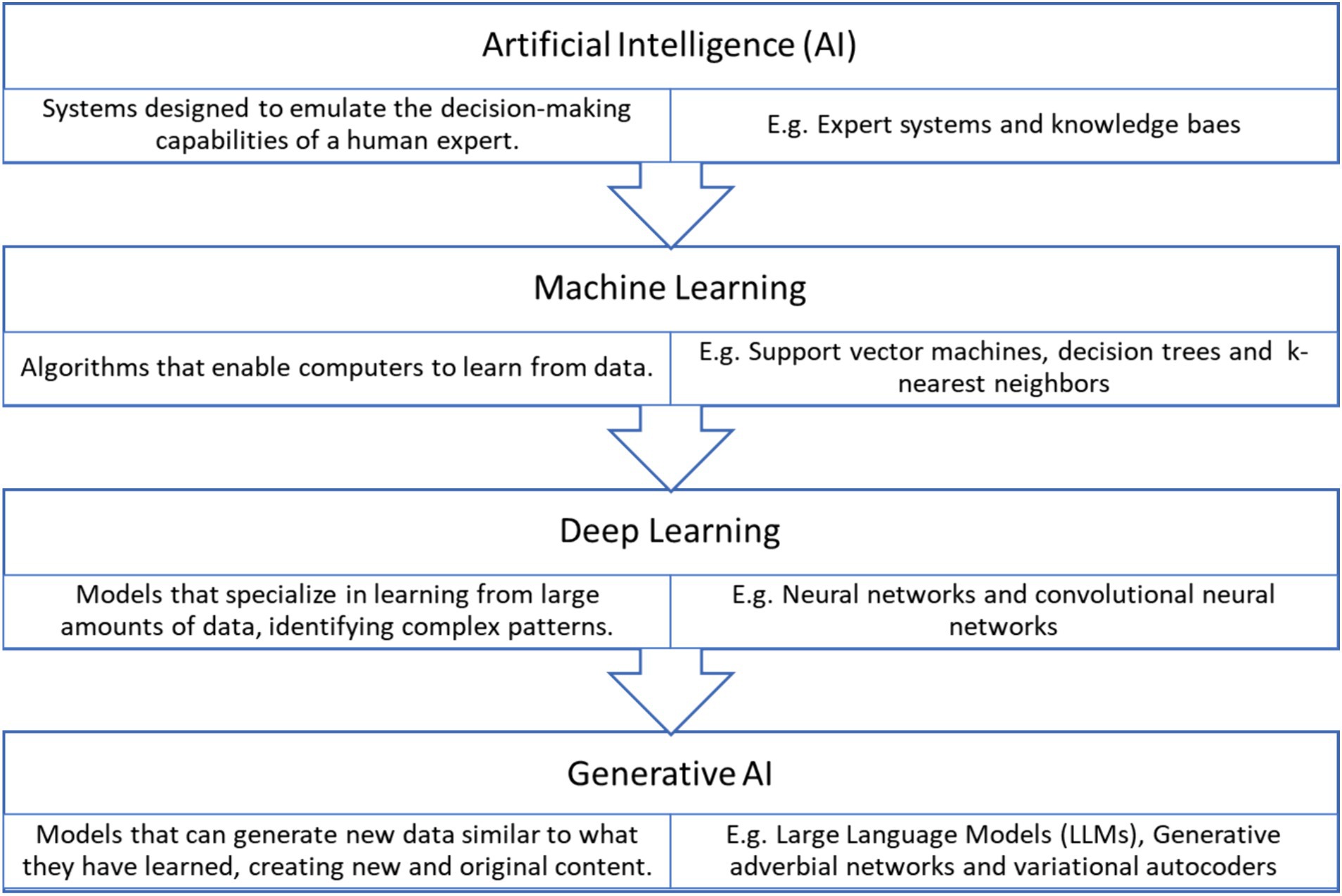
Figure 1. Scheme of the concepts of AI and GAI based on Banh and Strobel (2023) and Zhuhadar and Lytras (2023).
2.1 Large language models
Large language models represent a crucial advancement in GAI (Kaur et al., 2022). The effectiveness of LLMs is based on their training with extensive textual datasets, enabling them to understand and generate human language in a coherent and contextualized manner (Wang et al., 2023; Whitehouse et al., 2023). Capable of performing a wide variety of linguistic tasks, LLMs stand out as versatile and powerful tools for applications requiring a deep understanding of language (Gan et al., 2023).
These models began to gain prominence in 2017 with the emergence of a range of transformer-based machine-learning models that allow for better performance in language-based tasks compared to previous machine-learning techniques (Perkins, 2023). Google introduced BERT (Bidirectional Encoder Representations from Transformers) in 2018, which used bidirectional training in natural language processing to improve Google searches’ relevance of search results and suggestions (Devlin et al., 2019). This LLM can be fine-tuned with additional task-specific data to perform a wide range of Natural Language Processing tasks, learning complex relationships between words, and better understanding the structure of language (Martin et al., 2023).
In 2019, OpenAI released the Generative Pre-trained Transformer 2 (GPT-2), and in 2020, the Generative Pre-trained Transformer 3 (GPT-3) (Dale and Viethen, 2021). This LLM is described as an AI-powered writing assistant and is one of the most developed and widely known LLMs, noted for its ability to generate coherent and relevant content (Neumann et al., 2023). In the medical field, various LLMs such as BioBERT, PubMedBERT, and ClinicalBERT were developed from BERT, or Med-PaLM, fine-tuned from PaLM. Similarly, ChatDoctor or Hua Tuo are based on Meta’s LLaMa (Huang et al., 2023b).
Due to the staggering popularity of these LLMs, especially OpenAI’s GPT-3, teachers and researchers worldwide have begun to evaluate the potential impact of LLMs on education (Cao et al., 2023; Hariri, 2023; Prentzas and Sidiropoulou, 2023). Studies have been published discussing ways these intelligent systems can be useful in educational settings (Guleria et al., 2023; Prentzas and Sidiropoulou, 2023; Tsai et al., 2023).
In education, LLMs offer significant opportunities to innovate student’s learning process, promoting personalized learning, and democratizing access to knowledge (Gan et al., 2023). Capable of simulating interactive tutoring, these models can provide explanations tailored to the individual needs of each student, thereby improving understanding and retention of knowledge (Akiba and Fraboni, 2023). Moreover, by generating educational content in multiple languages, LLMs have the potential to overcome linguistic barriers, offering valuable resources to a global audience (Snoussi, 2019). This ability to personalize and universalize education access marks a step toward equal educational opportunities for students from diverse backgrounds (Böhm and Schedlberger, 2023).
However, implementing LLMs in in education faces significant challenges, such as ethical, accuracy, and access issues (Rafi et al., 2020; Cowling et al., 2023; Crawford et al., 2023). Dependence on these models raises concerns about the truthfulness of the content generated and its impact on the development of students’ critical thinking (Biri et al., 2023; Farah et al., 2023). Ethical questions arise regarding how these systems handle biases and data privacy, necessitating careful regulation to protect users (Al-Hunaiyyan et al., 2020). Moreover, the digital divide underscores an equity challenge, as not all students have equal access to these advanced technologies. Overcoming these obstacles requires collaboration among educators, technologists, and policymakers, ensuring that LLMs are utilized to enrich the educational experience while respecting ethical and equity principles (Snoussi, 2019).
On the other hand, there are various technical challenges to consider when implementing LLMs in higher education. LLMs are trained on vast datasets to generalize human language, which can lead to imprecise or inapplicable responses in specific contexts. LLMs can produce biased outcomes within this scenario, raising ethical and justice concerns, especially when used in sensitive contexts (Floridi and Chiriatti, 2020). Another study recognized that while LLMs can generate various challenges, such as academic integrity for teachers and students, as LLMs can generate detailed responses and complete essays that could limit the development of soft skills required by new professionals in this era of digital transformation (Kasneci et al., 2023).
Furthermore, it is crucial to strike a balance between the use of this technology in higher education institutions, as the constant use of LLMs may suppress student creativity or critical thinking (Bernabei et al., 2023). Many educators have expressed concern about overreliance and potential inaccuracies of LLMs, highlighting the need for careful planning and effective pedagogical integration (Biri et al., 2023). From this scenario, Escalante et al. (2023) showcase the need to balance human interaction with AI efficiency and explore how to effectively integrate these technologies to enhance student learning in higher education.
To identify effective educational practices and the use of LLMs in higher education, the concept of Education 4.0 has emerged. This approach emphasizes incorporating emerging technologies and innovative strategies to improve teaching methods in higher education. The goal is to prepare students to apply physical and digital resources to tackle current and future social challenges (Miranda et al., 2021). From a pedagogical basis, Education 4.0 proposes an innovative educational system focused on experience, integrating technology to meet the expectations of today’s world, centered on the student (González-Pérez and Ramírez-Montoya, 2022). Therefore, it is considered relevant to delve into this educational approach promoting disruptive technologies such as LLMs.
2.2 Education 4.0
Education 4.0 is a modern teaching methodology that focuses on equipping new generations with the skills and competencies required to adapt to a rapidly changing technological landscape (Mukul and Büyüközkan, 2023). This approach emphasizes problem-solving, integrating emerging technologies, and innovative strategies to develop students’ abilities (Matsumoto-Royo et al., 2021; Ramírez-Montoya et al., 2022b). This educational approach promotes emerging technologies in teaching and learning processes (Ciolacu et al., 2019), as its fundamental principles are innovation, flexibility, and adaptability to change (Kovaliuk and Kobets, 2021).
Education 4.0 aligns with the Fourth Industrial Revolution (4IR) through the evolution and reorientation of educational paradigms and innovative pedagogical approaches to meet the needs of a continuously changing technological society (Moraes et al., 2023). From this scenario, Education 4.0 is oriented toward increasing the flexibility of the various actors within the educational system with the support of innovative pedagogical paradigms and the backing of technology to guide the learning process. This integration encompasses connectivity and storage infrastructure, institutional guidelines, organizational procedures, and methods that promote innovation (González-Pérez and Ramírez-Montoya, 2022).
This innovative approach is a response to changes in society and technology in the 21st century, as it can offer advanced solutions to current educational challenges, including the implementation of hybrid and online education models, the use of digital tools and platforms to enhance learning and teaching, and the need for adequate training for teachers and students in the use of these technologies (Sabando Barreiro, 2021). One of the foundations of Education 4.0 is the incorporation of innovative pedagogies, mainly three pedagogical approaches that reflect the growing importance of digital technologies in education and the need for students to take an active role in their learning (Ramírez-Montoya et al., 2022a).
2.3 Pedagogical approaches of Education 4.0
Education 4.0 is built upon three pedagogical approaches: (a) Cyberpedagogy, (b) Heutagogy, and (c) Peeragogy (Ramírez-Montoya et al., 2022a). They are important to optimize learning and prepare students for the future, as these innovative pedagogies seek to change how the instructor, student, and resources interact in teaching and learning (Bizami et al., 2023).
Cyberpedagogy aims to connect the educational experience with ICT, considering the significant influence of the Internet and social media on learning. This educational paradigm emerges in response to rapid technological advances incorporating technologies for self-directed and interactive learning in a virtual world (Sumarsono, 2020; Rahma et al., 2021). Thus, new technologies seek to foster a virtual learning environment that supports the construction of knowledge and understanding among students, focusing on helping students learn autonomously and collaboratively (Tajudin et al., 2020).
Peeragogy promotes cooperation and collaboration in educational processes, as learning is a social act generated through interaction with others via technology (Revuelta-Domínguez and Pedrera-Rodríguez, 2020). This approach is based on peer learning, where students exchange information and enhance their learning strategies and communication skills to achieve educational goals together (Tajudin et al., 2020). Hence, the main patterns of Peeragogy are motivation, context, efforts of trust and inclusion, problem, solution, justification, and resolution (Rheingold et al., 2014). This approach addresses complex problems requiring multiple responses, achievable only through peer-to-peer pedagogy (Ramírez-Montoya et al., 2022a).
Heutagogy is grounded in humanistic and constructivist principles to foster self-learning practices (Miranda et al., 2021). Technological advancements allow students to explore and learn independently with digital tools and media. Pedagogy principles lead to self-determined learning with student autonomy, capacity, self-efficacy, reflection, metacognition, and non-linear learning (Blaschke and Marín, 2020). It is important to note that the student requires a level of personality, independence, and maturity for solid learning and good understanding. Heutagogy promotes lifelong learning and students’ independence, allowing education to transfer knowledge and foster responsible, honest, and fair citizens for life in the work and social fields (Sumarsono, 2020).
To summarize, Education 4.0 focuses on three key principles. Firstly, it promotes a student-centered approach to learning, emphasizing active engagement. Secondly, it seeks to provide students with real-world contexts, scenarios, and challenges, enabling them to apply their knowledge and skills in practice. Thirdly, it encourages the development of research skills and complex thinking, allowing students to propose innovative solutions to current societal issues. These principles are based on the Heutagogy, Peeragogy, and Cyberpedagogy approaches (Ramírez-Montoya et al., 2022a). A visual representation of these pedagogical approaches is presented in Figure 2.
From the description of the pedagogical approaches of Education 4.0, it is possible to find the relation of the pedagogical principles of Education 4.0 with the implementation of LLMs in higher education since Education 4.0 guides the use of emerging technologies such as LLMs based on the foundation of innovative pedagogies like autonomous and interactive learning (Ramírez-Montoya et al., 2022a). Similarly, current literature regarding the implications of LLMs shows a growing field that requires establishing the outcomes of recent studies and the future directions for implementing these models in higher education.
3 Methodology
A Systematic Literature Review (SLR) was implemented using the Scopus and Web of Science (WOS) databases to identify research focused on applying LLMs in the context of higher education between 2018 and 2023. It analyzed how these studies relate to the pedagogical principles of Education 4.0. The main purpose was to understand the interconnection between this emerging technology and the educational paradigm of Education 4.0 to obtain a comprehensive and profound insight within the realm of higher education (Hassan, 2023).
Firstly, we conducted a systematic mapping using the methodological framework that Petersen et al. (2015) suggested to investigate the use of LLMs in education. For example, Bernabei et al. (2023) conducted a case study in higher engineering education to explore students’ perceptions of the utility and acceptance of LLMs. On the other hand, Farah et al. (2023) examined the disruptive impact of LLMs and conversational AI on higher education pedagogy. We recognized that various authors have conducted preliminary studies on higher education and, therefore, conducted a thorough analysis of how implementing LLMs in this environment relates to the pedagogical principles of Education 4.0 in university settings. We followed the methodological guidelines that Kitchenham and Charters (2007) proposed.
This methodology allowed for an examination of how the application of LLMs reflects and enhances the educational approaches associated with Education 4.0 in a systematic and in-depth manner. This methodological approach, previously applied in academic research (Ramírez-Montoya and Lugo-Ocando, 2020; Baig and Yadegaridehkordi, 2023; Fariani et al., 2023), reinforces its validity and applicability in studies related to higher education. Furthermore, this SLR methodological proposal encompasses three specific phases addressing (a) the planning phase (the goal and scope of the research, research questions, search strategies, and inclusion, exclusion, and quality criteria), (b) the implementation phase (search phase, selection process through inclusion, exclusion, and quality assessment through quality, full-paper assessment, and inclusion of documents in SLR), and (c) the result reporting phase (reporting of results, discussion, and conclusions of the study). Figure 3 illustrates the methodology and phases of the research process, adapted from the works of Kitchenham (2004) and Kitchenham and Charters (2007), and each phase is detailed subsequently.
3.1 Phase 1: planning of the systematic literature review
The first phase consists of establishing (a) the objective and scope of the research, (b) the research questions, search strategies, and inclusion, exclusion, and quality criteria. This planning phase was established through the methodological proposal of Kitchenham and Charters (2007) (see Figure 4).
3.1.1 Objective and scope of the systematic literature review
A significant body of research in implementing LLMs has been identified through a systematic literature mapping. It was observed that a considerable portion of the research was focused on higher education. Therefore, this study aims to comprehensively and systematically explore the research field related to implementing LLMs in higher education and analyze its alignment with the pedagogical principles of Education 4.0.
3.1.2 Research questions
The study questions were posed in two dimensions: (a) to establish a broad and structured view of the studies related to the implementation of LLMs in higher education, and (b) to analyze in detail the interconnection between the pedagogical principles of Education 4.0 and LLMs, following the methodology proposed by Kitchenham (2004) and Kitchenham and Charters (2007).
These questions aim to cover essential aspects related to the progress of research over time, the distribution of studies across different regions, the dominant methodology employed, the most significant types of studies, the observed trends in publications, as well as the impact and connection between the pedagogical principles of Education 4.0 and the integration of LLMs. This comprehensive approach aims to thoroughly understand the interplay between emerging educational technology and the contemporary educational paradigm of Education 4.0, thereby contributing to advancing knowledge in this ever-evolving field of study. The Research Questions (RQ) were: (RQ1) How many studies have been published on implementing LLMs in higher education from 2018 to 2023?, (RQ2) Which countries have led the research on implementing LLMs in higher education within Education 4.0?, (RQ3) What research methodologies have predominated in studies on the subject?, (RQ4) What studies have been employed in research on the subject?, (RQ5) What trends and patterns are observed in the studies?, (RQ6) What trends and patterns are observed in the studies?, (RQ7) How do the three pedagogical principles of Education 4.0 relate to using LLMs in higher education?, (RQ8) What has been the impact of integrating LLMs in higher education?, and (RQ9) What emerging challenges must future research address to maximize the potential of LLMs in higher education? (see Figure 4).
3.1.3 Search strategies
Search strategies were established for conducting the SLR based on the following criteria: (a) databases, (b) search string and keywords, (c) time frame, (d) type of document, (e) language, (f) field of study, and (g) document access type (see Figure 4).
Scopus and Web of Science databases were chosen due to their broad recognition and prestige in the academic and scientific community. The choice of these platforms is based on their ability to provide access to a wide spectrum of quality academic literature, which is essential for an exhaustive and relevant exploration of the field of study (George Reyes and Glasserman-Morales, 2021).
Two main search strings were established to conduct the systematic literature review: (a) (“large language model*” OR “LLM”) AND (“higher education” OR “university” OR “college”), and (b) (“artificial intelligence” OR “AI”) AND (“large language model*” OR “LLM”) AND (“higher education” OR “university” OR “college”). The adoption of two search strings is based on the principle of comprehensiveness, which is fundamental in the systematic review methodology, to minimize the risk of omitting relevant studies that could significantly contribute to the proposed research objectives.
The search string (a) (“large language model*” OR “LLM”) AND (“higher education” OR “university” OR “college”) was focused on identifying studies that explicitly mention “large language models” in combination with terms related to higher education (“higher education,” “university,” and “college”). The purpose of this string was to obtain literature that directly addresses the implementation and impacts of LLMs within higher education institutions, ensuring that the selected studies are directly relevant to the focus of the analysis. On the other hand, the search string (b) (“artificial intelligence” OR “AI”) AND (“large language model*” OR “LLM”) AND (“higher education” OR “university” OR “college”) was established to identify studies that consider the influence of AI to provide another perspective not directly limited to LLMs, in higher education. This approach increases the likelihood of retrieving all pertinent studies and strengthens the integrity and depth of the literature analysis.
To define the time frame of the review, the range from 2018 to 2023 was selected, considering that Google launched the first LLM (BERT) in 2018 (Perkins, 2023). This interval covers the initial advancements and current trends in LLMs in higher education. Eligible documents were defined as research articles, systematic literature reviews, methodological papers, meta-analyses, conference proceedings, and papers. Two languages, English and Spanish, were selected for document selection. Furthermore, open and restricted access documents were selected to consider the study’s importance. On the other hand, the field of study delimited for the SLR was Education (see Figure 4).
3.1.4 Inclusion, exclusion, and quality criteria
To ensure a rigorous and focused systematic literature review on implementing LLMs in higher education within the framework of Education 4.0. The inclusion criteria for this study were as follows: (a) the research focus should be on the implementation, use, impact, and challenges of LLMs in higher education; (b) the documents should be scientific journal articles, books, and chapters published between 2018 and 2023; (c) works situated in the field of education should be considered; (d) the documents should be written in English or Spanish; and (e) the studies should be available in open access or through a subscription, ensuring a broad inclusion of relevant literature.
This review applied exclusion criteria to ensure that only relevant and significant studies were included in the analysis. The criteria included: (a) Thematic Relevance—studies that did not directly focus on the implementation or impact of LLMs in higher education were excluded to ensure that the analysis focused on the key topic of interest; (b) Type of Publications—conference proceedings; congress memories, regulatory documents, or publications were excluded as they were deemed less reliable sources of information; (c) Publication Period—works published outside the time range of 2018–2023 were excluded to maintain the relevance and timeliness of the review; (d) Disciplinary Relevance—only documents related to the specified disciplines of interest were included to limit the analysis to significant contributions to the field of study; and (e) Language and Duplication—publications in languages other than English or Spanish and duplicate documents from the same research were excluded to ensure the quality and uniqueness of the data used in this review.
The Quality Criteria have two main aspects. Firstly, the contribution to the field means that the study must significantly contribute to understanding the implementation and impact of LLMs in higher education, particularly regarding the principles and challenges of Education 4.0. Secondly, methodological rigor means that the coherence and appropriateness of the research design, methodology, analysis, and discussion of results will be key criteria for assessing the quality of the included studies.
3.2 Phase 2: implementation
The implementation of the SLR involved following the study selection and quality assessment procedure established in the planning phase. This process entailed an adaptation of the three stages of the selection process by Kitchenham and Charters (2007): (a) study search, (b) study selection, and (c) quality assessment, culminating in the documents included for analysis in the systematic review. It is recognized that this methodology establishes a flexible selection process that has allowed each systematic review to be customized to address specific research objectives and unique contexts, thereby ensuring the relevance and applicability of the results obtained (Kitchenham et al., 2010; Ramírez-Montoya and Lugo-Ocando, 2020; Santos et al., 2020; Pizard et al., 2021; Baig and Yadegaridehkordi, 2023; Fariani et al., 2023) (see Figure 5).
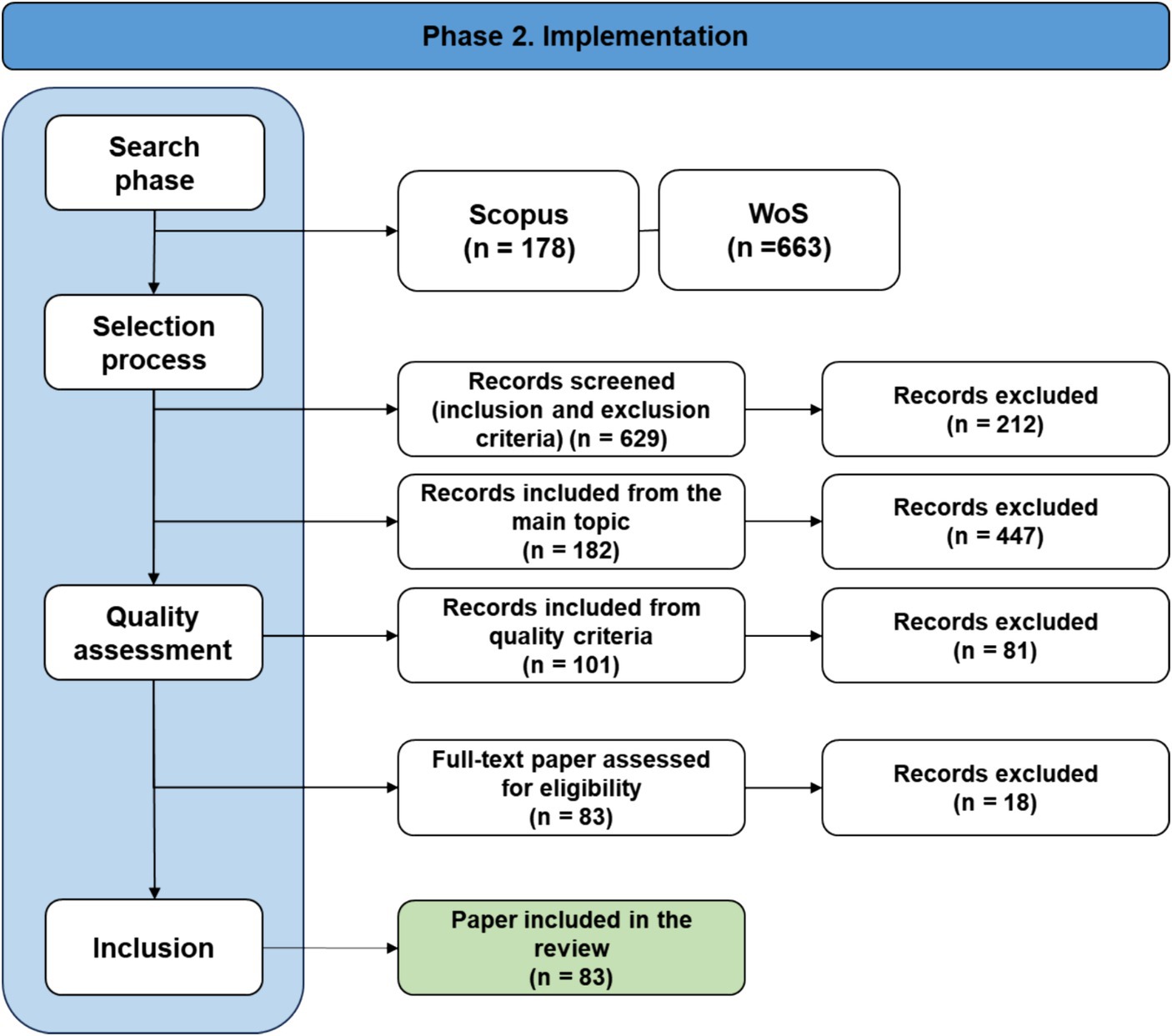
Figure 5. Selection process adapted from Kitchenham and Charters (2007).
During the search phase of the study, 841 documents were retrieved through the two search strings across the selected databases: Scopus (n = 178) and Web of Science (n = 663). For the Scopus database, the first search string [TITLE-ABS-KEY (“large language model*” OR “LLM”) AND TITLE-ABS-KEY (“higher education” OR “university” OR “college”)] yielded 175 documents. The second search string [TITLE-ABS-KEY (“large language model*” OR “LLM”) AND TITLE-ABS-KEY (“higher education” OR “university” OR “college”) AND TITLE-ABS-KEY (“artificial intelligence” OR “AI”)] yielded 113 documents. Between the two search strings, 288 initial documents were obtained. Through the platform, an initial screening of duplicate documents from both search strings was performed, saving the searches and downloading the final list with 178 documents. For the Web of Science database, the first search string considering all fields ALL = (“large language model*” OR “LLM”) AND ALL = (“higher education” OR “university” OR “college”) yielded a total of 663 documents. The search string ALL = (“large language model*” OR “LLM”) AND ALL = (“higher education” OR “university” OR “college”) AND ALL = (“artificial intelligence” OR “AI”) resulted in 203 documents. 834 documents from the two search strings were considered at the beginning of the identification. A list was created in WoS, resulting in a final list of 663 documents between the two search strings.
In the selection process, inclusion and exclusion filters were applied considering the document type, language, frequency, and duplication in the initial base downloaded from both databases. In this initial phase of the selection process, 212 documents from both databases were filtered out, resulting in an initial base of 629 documents without duplicates, in Spanish and English, and with the correct type of document to assess relevance to the topic through title, abstract, and keywords. 182 documents considered relevant to the topic were included, and 447 documents were excluded. Subsequently, quality criteria among the 182 documents were considered, assessing if these studies had a significant contribution to the understanding of the implementation and impact of LLMs in higher education, especially about the principles and challenges of Education 4.0 and whether the coherence and appropriateness of the research design, methodology, analysis, and discussion of results would be key criteria for evaluating the quality of the included studies. A final base of 101 documents was obtained through quality criteria, and 81 were excluded. The 101 documents were evaluated through a full-text analysis.
It is important to emphasize that the exclusion of articles after applying inclusion and exclusion criteria was carried out through a rigorous process detailed in the document. The exclusion criteria were established to ensure that only relevant and significant studies were included in the analysis. These criteria included thematic relevance, type of publications, publication period, disciplinary relevance, and language and duplication of documents. Only those documents that directly focused on the implementation or impact of large language models (LLMs) in higher education were included, excluding those that did not directly address this topic to maintain focus on the key interest of the study.
In the quality assessment stage of the documents, 83 documents were obtained, and 18 documents were excluded. The quality evaluation of the documents considered a more detailed quality analysis that included reviewing abstracts and checking for significant contributions to the topic. The selected documents were analyzed in detail to respond to the research questions, ensuring that they reflected a deep and systematic understanding of the application of language models in higher education. These rigorous methods ensured that only the most relevant and high-quality studies were considered, allowing for a reliable and relevant evidence synthesis in the emerging field of Artificial Intelligence in higher education. At the end of the implementation phase, 83 documents were included to conduct this SLR (See the selection process at the link: https://bit.ly/selectionprocessLLM).
A database was created using Excel, containing information from various documents arranged alphabetically according to fields such as the database, author(s), document title, year, type of document, journal or publisher, DOI, abstract, keywords, language, and access type. The analysis of the documents to answer the research questions determined in the planning phase was initiated, as shown in Figure 2. The database was divided into 11 columns related to bibliographic data and five columns to answer questions and provide an overview of the theme. You can access the bibliographic database at the following link: Database.
The analysis results of the general overview of the theme were conducted through Tableau1 to visually identify the data obtained from the thematic area questions (RQ1, RQ2, RQ3, RQ4, and RQ5). Additionally, VOSVIEWER software2 was used to obtain the bibliometric network of the abstracts, keywords, and authors to identify trends and patterns of studies on the theme (RQ6). On the other hand, the documents were explored through MAXQDA 20223 to maintain an exhaustive and structured bibliographic analysis of the data (RQ7, RQ8, and RQ9).
4 Phase 3: reporting results
In the third phase of the SLR, the report of the results was prepared based on the nine research questions. This report is structured into (1) introduction, (2) method (Phase 1: Planning of the review and Phase 2: implementation), (3) results (Phase 3: reporting of the results), and (4) discussion and conclusions.
4.1 How many studies have been published on implementing LLMs in higher education from 2018 to 2023?
To respond to this inquiry, an exhaustive analysis of 83 selected documents was performed, evaluating aspects such as the type of document, access to it, and year of publication to outline a comprehensive panorama of the scientific production around the topic of study. It is crucial to highlight that this analysis covered 2018–2023, based on the bibliographic identification that the first Generative Pretraining Transformer (GPT) model was introduced by OpenAI in 2018 (Laato et al., 2023).
A detailed examination of related academic production reveals that in 2023, the highest volume of publications relevant to the topic was registered, totaling 81 documents. Generally, there is a marked preference for open access, with 70 research studies available under this modality, compared to 11 studies with restricted access. Although the initial launch of GPT dates back to 2018, it was not until 2022 that its application was significantly extended to the general public (Kuramitsu et al., 2023). This preliminary review of the works conducted in 2023 shows a substantial increase in research on the theme, especially highlighting those studies focused on LLMs in higher education (see Figure 6).
4.2 Which countries have led the research on implementing LLMs in higher education within Education 4.0?
The analysis of the geographical distribution of studies related to the implementation of LLMs in higher education shows a significant concentration of research in the United States (n = 24). It should be noted that two studies were conducted from a global perspective in the analysis. It is important to acknowledge that 12 studies had an international focus. In six of these studies, research was conducted in the United States and other countries such as Australia, Canada, Singapore, Ireland, Egypt, Chile, and China. Therefore, the United States shows significant participation, with multiple entries throughout the database, indicating a strong influence in this research field. Hence, they were not counted in the analysis by countries. On the other hand, Australia (n = 8) also features prominently, with several contributions, demonstrating its leadership in the Asia-Pacific region. Subsequently, Canada (n = 7) holds third place in producing studies on the topic (see Figure 7).
To delve deeper into the subject, performing an analysis by region is relevant. In America, two main countries with contributions to the topic, the United States and Canada, were previously mentioned. Thus, a predominant concentration in North America is revealed. However, the panorama changes about Latin America and the Caribbean, as studies focused on the topic were only located in Antigua and Barbuda, Colombia, and Chile (n = 1). From these results, a disparity in the scientific production of LLMs in higher education in the American continent is identified (see Figure 7).
In the European region, Germany is identified as the leading researcher on the topic (n = 9). Although the number of studies is less than in the country leading the research on the topic, a strong research concentration in this region is identified, as studies are observed in several European countries. The United Kingdom, Spain, and Ireland (n = 3) hold second place in academic production on the topic in Europe. Meanwhile, Norway and Poland (n = 2) show moderate interest, and Austria, Switzerland, and Finland have produced studies on the topic. Likewise, countries in Southern and Eastern Europe, such as Italy, Croatia, Serbia, Bulgaria, Greece, and Turkey (n = 1), show participation in LLM research, albeit to a lesser extent. This indicates a diversified panorama in Europe regarding LLM research, with Germany clearly at the forefront, followed by significant participation from the United Kingdom and Ireland and various contributions across the continent. This also reflects curiosity about the topic with 23 studies in 2 years of public use of these LLMs (see Figure 8).
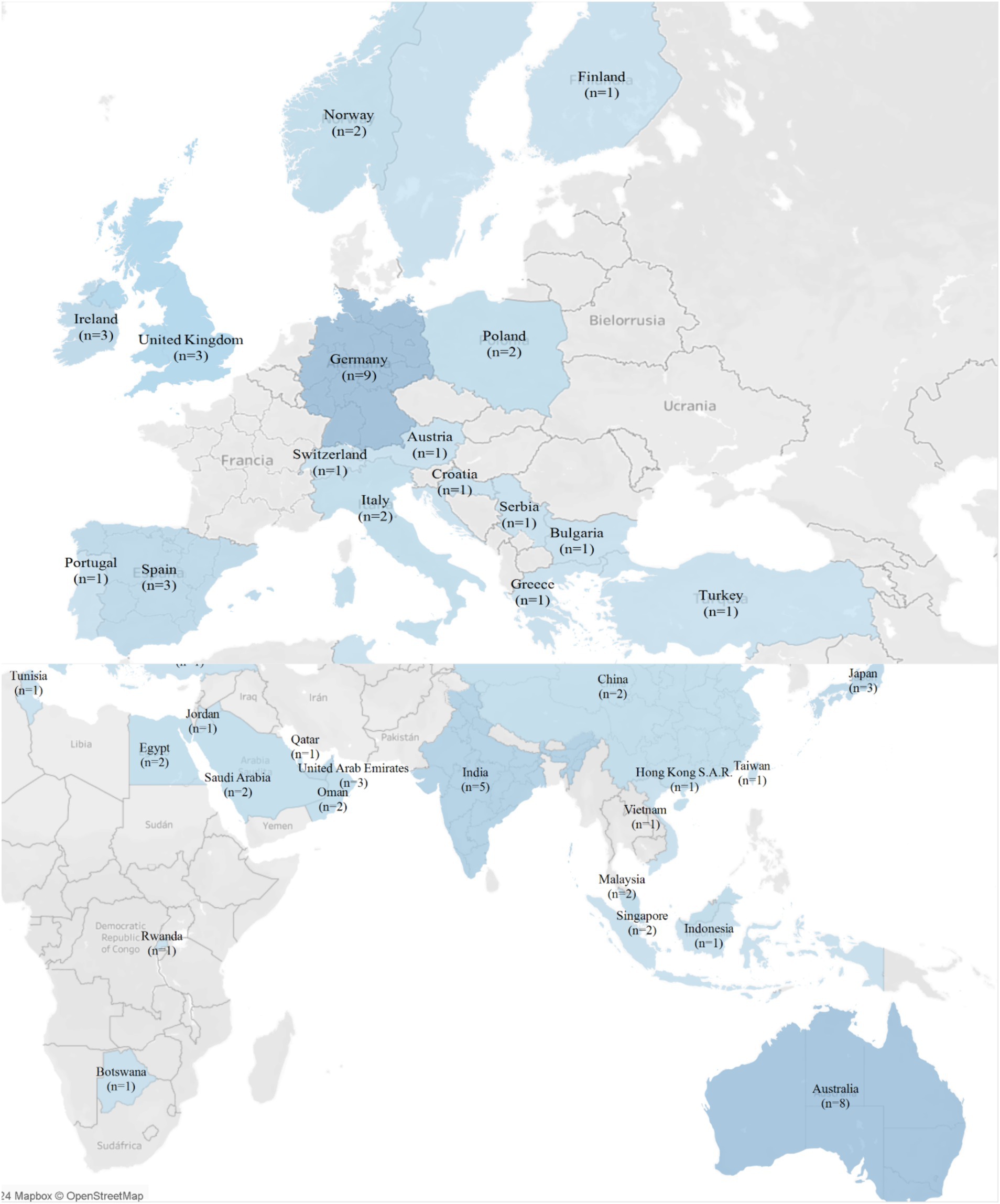
Figure 8. Geographic distribution of research on LLMs in higher education in Europe, Africa, and Asia.
On the other hand, Asia has shown a greater production of studies on the topic, with India leading the continent (n = 5). Japan and the United Arab Emirates are second (n = 3). China, Malaysia, Singapore, Oman, and Saudi Arabia are in third place (n = 2). Finally, Hong Kong, Taiwan, Vietnam, Indonesia, Qatar, and Jordan show initial interest in exploring the topic (n = 1). In Africa, only Egypt (n = 2), Tunisia, Rwanda, and Botswana (n = 1) have researched LLMs (see Figure 8).
4.3 What research methodologies have predominated in studies on the subject?
To answer the question, six categories of methodologies found in the studies were established: (a) quantitative methodology, (b) qualitative methodology, (c) mixed methods, (d) conceptual paper or literature review, (e) experimental methodology, and (f) design methodology. The analysis results show that quantitative methodology has been used in 27.71% (n = 23) of the studies, indicating a preference for approaches that rely on collecting and analyzing quantitative data. Mixed methods come in second place (n = 21), as 25.30% of the studies adopted a mixed methodology. In third place is a qualitative methodology (n = 18), with 21.69% of the studies. It is important to acknowledge that there are 16 studies related to literature reviews and conceptual works (19.28%). Although most studies sought to implement LLMs, a significant portion focused on synthesizing and analyzing existing literature to construct a robust theoretical or conceptual framework regarding the topic. It should be noted that four studies chose to use experimental methodology (4.82%), and one study was based on design methodology (1.20%) (see Figure 9).
4.4 What studies have been employed in research on the subject?
The review of the 83 documents considered 11 categories of study types: (a) experimental studies, (b) case studies, (c) literature reviews, (d) descriptive studies, (e) exploratory studies, (f) comparative studies, (g) design and development studies, and additionally found (h) exploratory and descriptive studies, (i) experimental and descriptive studies, (j) comparative and experimental studies, and (k) Delphi method. The predominant studies related to the topic were experimental studies, with 22 research studies, which is equivalent to 26.51%. Subsequently, research guided by case study guidelines was identified, representing 20.48% with 17 studies. Following that, 15 literature reviews (18.07%) were identified. On the other hand, the analysis found nine studies (10.84%) from a descriptive design, highlighting the importance of observing the topic. Regarding descriptive studies, five studies combined exploratory and descriptive approaches (6.02%). There were six exploratory studies only (7.23%). Additionally, four experimental studies combined with descriptive ones were identified (4.82%), as well as two comparative studies (2.41%). Finally, the least frequent approaches, such as the Delphi method, design and development, and comparative and experimental studies, were found in only one research (1.20%). From a general analysis, a diversity of approaches reflected in the research on LLMs in higher education can be identified, with a significant tendency toward experimental and case studies (see Figure 10).
4.5 What sample sizes are observed in studies on the subject?
In order to address the issue of sample size, the study established six different ranges, ranging from 1 to 50 to more than 250. The significance of this question lies in the fact that the size of the study population affects both the breadth and the potential applicability of the study’s results. Out of 83 documents reviewed, 46 did not provide information regarding the sample size. These documents focused on non-empirical data such as literature reviews, theoretical analyses, design studies, technological evaluations, or conceptual discussions, which did not require a sample of participants. Although these 46 documents are important for theoretical development, knowledge synthesis, and topic evaluation, they should have been considered when analyzing the sample size. As a result, the question was answered based on an analysis of 37 studies.
The analysis results show that sample sizes exceeding 250 subjects represent 38.89% of the studies (n = 14), highlighting a predominant focus on quantitative research, complementing the results of the types of methodologies where studies oriented toward quantitative methodology were predominant. Secondly, nine studies of 1–50 participants (25%) were identified; in third place, seven studies with sample sizes of 51–100 (19.44%) were located. These data may be reflected in qualitative or mixed methodologies, representing the studies’ second and third predominant places (see Figure 11). Finally, only four studies had samples within the ranges of 151–200 (n = 2) and 201–250 (n = 2) (see Figure 11).
4.6 What trends and patterns are observed in the studies?
A bibliometric network analysis was conducted to identify the trends in studies focused on implementing LLMs in higher education to analyze (a) the keywords and (b) the titles and abstracts of the documents by VOSViewer. The analysis showed a total of 952 items and 13,083 links. The total number of clusters was 47. The most significant term was “systematic review,” and a link of this term with “report,” “language,” “positive attitude,” and “vlogging” (red cluster) was shown. Through this result, it can be recognized that this topic has many documents focused on literature reviews as it is a topic of recent origin. Another interesting cluster (green) is “Student submission,” as students have the possibility of automatic evaluation and also the opportunity to inquire and be autonomous learners as proposed in the literature (Prentzas and Sidiropoulou, 2023) (see Figure 12).
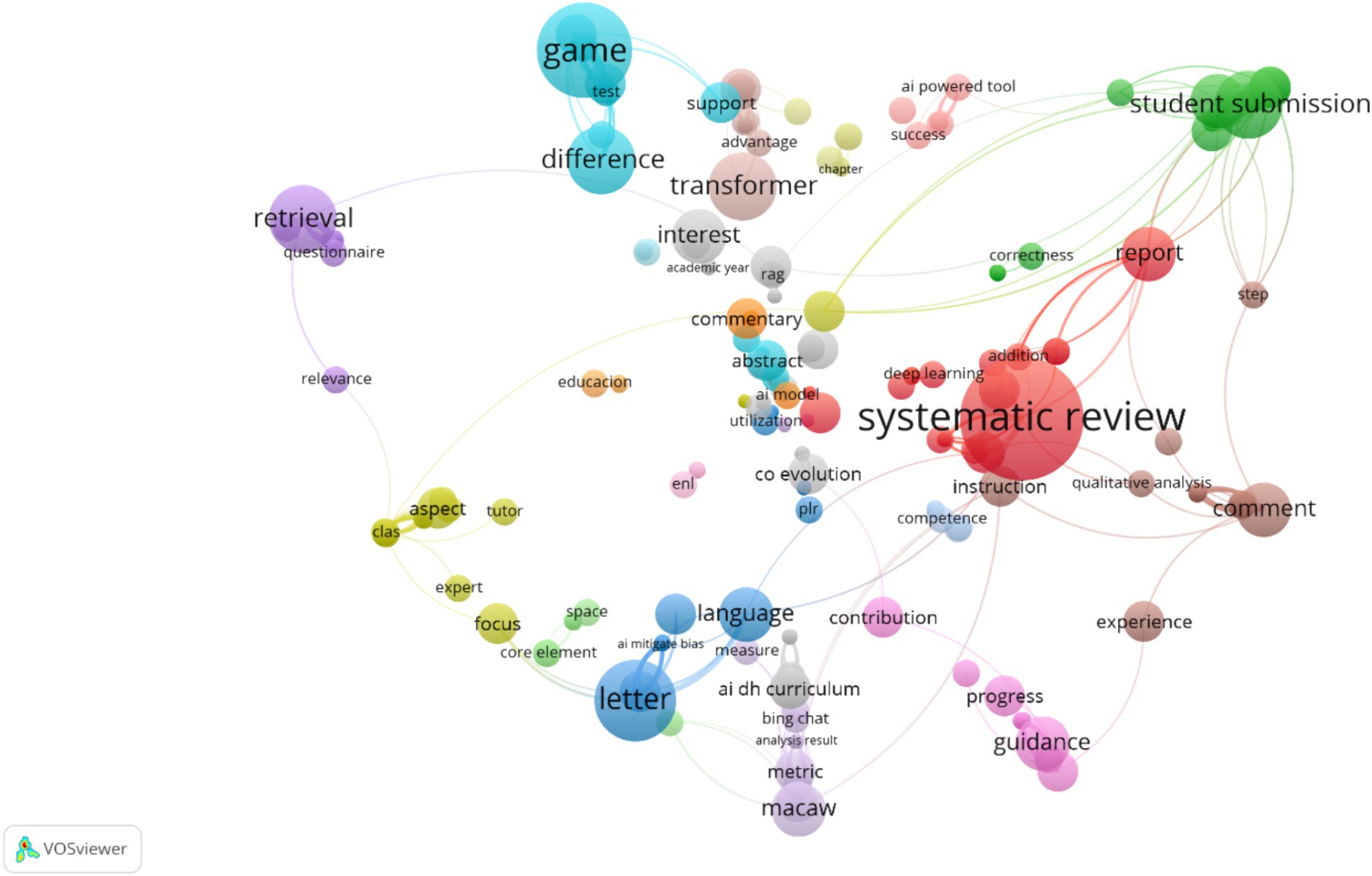
Figure 12. Bibliometric network of abstracts and keywords (http://tinyurl.com/2apzptx5).
4.7 How do the three pedagogical principles of Education 4.0 relate to using LLMs in higher education?
To analyze the documents and link the study findings with the pedagogical principles of Education 4.0, three main categories (a) Cyberpedagogy, (b) Peeragogy, and (c) Heutagogy, and 12 subcategories were established through MAXQDA software.
The analysis of implementing these models in higher education shows that LLMs lead to two key principles of cyberpedagogy: autonomous learning (18%) and collaborative learning (19%). According to Alqahtani et al. (2023), these models can support autonomous learning and provide personalized assessment to the student. On the other hand, LLMs can pose questions for the student to continue learning autonomously (Cheung et al., 2023; Sallam et al., 2023). It was also identified that LLMs can support peer assessment (Bauer et al., 2023; Böhm and Schedlberger, 2023). Directly, interactive learning in the studies (15%) is another principle of cyberpedagogy when students exchange knowledge with the model and their peers if these models are used from student-centered pedagogical bases (Chun and Elkins, 2023). It is important to recognize that the most observable pedagogical principles in implementing these models are cyberpedagogy and heutagogy. However, the principles of peeragogy were identified less frequently in the analyzed studies (2.1. Context, 2.2. Self-confidence and inclusion, 2.3. Problem and solution). Self-determined learning was the most observable of the five principles of peeragogy (14%), as it was recognized that LLMs can provide immediate feedback and offer a wide range of knowledge to students (Williams and Fadda, 2023) (see Figure 13).
4.8 What has been the impact of integrating LLMs in higher education?
A comprehensive review of the 83 documents was conducted to answer this question. After the qualitative data analysis, eight indispensable categories were classified through MAXQDA. The impact of LLMs in the studies was primarily positive, identifying that the studies show that these models have improved Learning Efficiency and Effectiveness (23%), as models like CHATGPT have helped students to understand better and generate an autonomous learning environment (Dale and Viethen, 2021; Jankovic et al., 2023). Likewise, the studies have recognized that LLMs can be a potential educational tool to complement task and exam assessments without eliminating teachers’ important role in the teaching-learning process (Biri et al., 2023). Another significant impact of these models is that the studies show the potential of LLMs to provide preliminary feedback before supervisor review, which supports the psychological needs of students and improves the quality of feedback from Advances in Automated Feedback code (16%) (see Figure 14).
4.9 What emerging challenges must future research address to maximize the potential of LLMs in higher education?
To identify the challenges higher education faces in implementing these LLMs, a categorization of the 10 main challenges for future research was done through MAXQDA. After analyzing the 83 documents related to the topic, the main challenge identified in the studies was ethical and legal considerations in using AI technologies like ChatGPT, as educational stakeholders show genuine concern for these models’ ethical and legal implications (20%). After analyzing 83 documents related to the topic, the primary challenge identified in the studies pertains to the ethical and legal considerations in using AI technologies like ChatGPT, reflecting genuine concern among educational stakeholders about these models’ ethical and legal implications (20%). Additionally, the importance of adapting educational frameworks to effectively incorporate AI technologies and align AI-generated content with learning objectives is acknowledged (Integration of AI in Pedagogical Content = 16%). Moreover, the findings revealed significant concern among higher education institutions regarding the quality of the content produced by such models (Accuracy and Reliability of AI-generated content = 16%).
This analysis underscores the dominance of ethical and legal challenges and the pedagogical integration of AI as focal points in higher education. It emphasizes the urgency of outlining future research directions to deepen knowledge in these areas, exploring strategies for responsible incorporation of LLMs that balance technological efficacy with integrity and human values (Killian et al., 2023; Moya Figueroa and Eaton, 2023; Vicente-Yagüe-Jara et al., 2023). Furthermore, it is suggested that clear regulatory frameworks and control mechanisms for producing and using AI-generated content be developed, ensuring its reliability and alignment with educational and ethical principles (Fecher et al., 2023).
To address these concerns, a research agenda is proposed, including the development of methodologies to evaluate and enhance the quality of AI-generated content, strategies to bolster digital literacy among students and educators, and studies examining the long-term impact of AI on learning outcomes (Kostka and Toncelli, 2023; Mills et al., 2023). This comprehensive approach should reflect a synthesis of emerging technology and pedagogy to inform best practices and policies in higher education with AI (Sohail et al., 2023) (see Figure 15).
5 Discussion
The results of this SLR provides evidence that LLMs can play a significant role in enhancing interactive, collaborative, and autonomous learning, aligning with the principles of cyberpedagogy and supported within the framework of Education 4.0. It was found that LLMs can support autonomous learning by 18%, collaborative learning by 19%, and interactive learning by 15%, underscoring their potential as online pedagogical tools to deliver personalized content and real-time responses to student inquiries. This information underscores the relevance of focusing education on the learner and aiding in their comprehensive development, aligning with the findings of Tajudin et al. (2020), who promote transformative learning through pedagogical approaches such as heutagogy and cyberpedagogy. These findings can be situated in various studies of this research, pointing toward a significant integration of LLMs in higher education (Crawford et al., 2023; Dwivedi et al., 2023; Farah et al., 2023).
Although this study focused on finding the interconnection between pedagogical approaches of Education 4.0 and the use of LLMs, this emerging technology can support the development of specific skills and competencies that require exploration, such as critical thinking. Various studies have shown that using LLMs can stimulate critical thinking (Guo and Lee, 2023; Hackl et al., 2023; Sridhar et al., 2023). Specifically, one study explored the potential to enhance equity, personalization, and critical thinking skills (Ivanov and Soliman, 2023). Another study observed that these models can motivate students by allowing them to engage in interactive problem-solving and support the development of critical thinking skills and a deep understanding of the principles behind LLMs, taking responsibility for their use and creations (Kostka and Toncelli, 2023). From this scenario, various studies envision that LLMs present numerous opportunities to enrich learning and instruction in higher education. However, educators and students must know their limitations (Laato et al., 2023). Thus, the vast field of research on implementing LLMs in higher education is acknowledged.
Furthermore, incorporating AI in educational contexts is a fundamental pillar in fostering the advancement of skills based on critical and creative thinking by creating dynamic and adaptive learning environments. Findings indicate a significant increase in students’ ability to tackle complex problems and generate innovative solutions when AI tools are integrated into the curriculum. This progress aligns with pedagogical theories highlighting the importance of stimulating learning environments for developing creativity and critical thinking (Ramírez-Montoya et al., 2022b; Bernabei et al., 2023). In summary, AI offers tools for personalizing learning and catalyzes the development of essential skills in the digital age, thus enriching the educational experience.
On the other hand, the study exhibited that implementing AI technologies in higher education through LLMs poses significant ethical and legal challenges, especially regarding privacy. The results indicate that, despite the perceived benefits, concerns about ethical conduct and legal implications account for 20%, in addition to 14% for information privacy, of the total concerns regarding their full incorporation in higher education. This finding concurs with previous research that highlights the need for implementing stringent regulations and guidelines to protect users from potential harm (Alqahtani et al., 2023), the importance of establishing solid ethical and legal frameworks that guide the incorporation of Artificial Intelligence in educational realms (Al-Hunaiyyan et al., 2020), and the creation of discipline-specific consensuses on the ethical and appropriate application of Large Language Models (Killian et al., 2023; Moya Figueroa and Eaton, 2023; Vicente-Yagüe-Jara et al., 2023). In conclusion, while AI offers unprecedented opportunities to personalize and enrich the educational experience, addressing these ethical and legal challenges is imperative to ensure a fair, safe, and respectful learning environment. From this context, it can be identified that mitigating these ethical and legal issues can support using LLMs in higher education.
After analyzing the results, it has become evident that educators have a crucial role in the Artificial Intelligence (AI) era. This highlights the need to redefine teaching through technology, transforming educators into facilitators of learning who emphasize pedagogical and emotional competencies. The findings suggest that integrating Large Language Models (LLMs) into the classroom expands educators’ capabilities, enabling them to offer more personalized instruction focused on the critical and creative development of the student (15%) and empowering students to pursue autonomous learning (32%). The research supports this approach, which emphasizes mentoring and personalized guidance as key components of modern education to achieve student autonomy. The role of educators within an educational environment enhanced by LLMs requires careful consideration, focusing on strategies for their adaptation and success. It is necessary to recognize educators not just as transmitters of knowledge but as facilitators of learning, capable of integrating advanced technologies to enrich the educational experience. This reassessment involves continuous training of educators in digital tools and innovative pedagogical methodologies. The educator’s role expands to include mentoring and personalized support, ensuring that technology serves as a complement that enriches and not as a substitute for the human educational process. Thus, integrating LLMs in education involves technological implementation, pedagogical evolution, and professional development.
The quality and accuracy of GAI content are crucial for effective education. Studies by Cowling et al. (2023), Crawford et al. (2023), and Rafi et al. (2020) have highlighted the importance of accuracy, as current research has shown that Language Models (LLMs) can produce inaccurate or irrelevant responses in certain contexts (Floridi and Chiriatti, 2020). While LLMs can provide detailed and relevant information, further analysis and improvement are required to ensure accuracy. Therefore, future investigations are necessary to validate the information generated by LLMs, which accounts for around 16% of the research. To ensure academic integrity in the educational community, monitoring and evaluating the quality of AI-generated content is crucial, as suggested by Biri et al. (2023) and Farah et al. (2023). In conclusion, even though technological advancements have been made, human supervision is still essential to ensure that the educational content generated by AI is of high quality and integrity.
In addition to the ethical and accuracy challenges of implementing LLMs, studies have identified an equity issue, as not all students have equal access to such advanced technologies (Cabero-Almenara and Ruiz-Palermo, 2017; Snoussi, 2019). The digital divide is a significant challenge in implementing LLMs, as students from different socioeconomic backgrounds still face disparities in access to technologies like AI (Dwivedi et al., 2023; Sarker et al., 2023). Moreover, paid LLMs like ChatGPT-4 may limit the free use of high-quality programs for the entire population, resulting in disparities in access, support, usage, and competencies of students, as well as a range of secondary effects (Lacey and Smith, 2023). While LLMs have the potential to offer vast educational opportunities, the reality is that disparity in access to these technologies remains a significant obstacle. Therefore, it is crucial to continue the debate and research on ensuring the benefits of LLMs are accessible to all students, promoting true democratization of education in the digital age.
6 Conclusion
This SLR has demonstrated the significant impact of LLMs in higher education, which aligns with the principles of Education 4.0. These models facilitate more interactive, collaborative, and self-directed learning, promoting access to knowledge, overcoming linguistic and cultural barriers, and emphasizing the importance of personalized learning. LLMs have the potential to enhance pedagogical approaches, improve educational outcomes, and encourage holistic development and student-centered education through advanced technologies.
It is essential to acknowledge the challenges brought about by the rapid evolution of the LLM field and its impact on education. The most critical challenge in the fast-paced integration of LLMs into education is the ethical and legal issues. It requires thorough regulation and deep ethical reflection to protect students and educators regarding data privacy, informed consent, intellectual authorship, and equity in access. It is imperative to establish robust regulatory frameworks and effective control mechanisms for AI-generated content to ensure its reliability and adherence to fundamental ethical and legal principles in the educational realm. Lastly, despite the challenges, it is crucial to recognize the unparalleled potential LLMs offer to personalize and enrich the educational experience. Balancing technological innovation with fundamental ethical and human values, human supervision, and guidance will remain crucial to ensuring a fair, safe, and respectful learning environment.
It is important to note some limitations of this study, one of which is the rapid evolution of GAI. This advancement may overshadow the current conclusions, emphasizing the need for constant updates on the state of the art and research surrounding LLMs. It is crucial to continuously adapt to technological advances to ensure the relevance and applicability of our conclusions and recommendations over time. Another significant limitation is the specificity and availability of the analyzed data. The study only focused on applying LLMs in higher education, excluding other educational levels that could benefit from or be affected by integrating these technologies. Future research must encompass a wider range of educational contexts to gain a broader understanding of the impact of LLMs across the complete educational spectrum. This holistic approach will provide valuable insights into how these technologies can serve as pedagogical tools in diverse environments and levels of education.
In conclusion, future research should focus on exploring how advanced technologies can facilitate the development of specific competencies such as emotional, collaborative, complex problem-solving skills, and critical thinking. This study has established a connection between Education 4.0 and the implementation of LMMs in higher education and has shown that LLMs can promote critical thinking skills. However, incorporating LLMs in higher education also poses several ethical and legal challenges regarding privacy. Therefore, studies are needed to develop ethical and legal frameworks to guide the safe and fair integration of artificial intelligence into education. Furthermore, further research is necessary to understand how LLMs change the role of educators from knowledge transmitters to learning facilitators. It is essential to explore how this transition affects pedagogical practices and the professional competencies that teachers need to develop.
Author contributions
IP-S: Conceptualization, Data curation, Formal analysis, Investigation, Methodology, Writing – original draft, Writing – review & editing. DV-C: Conceptualization, Formal analysis, Investigation, Writing – review & editing. LG-M: Funding acquisition, Supervision, Visualization, Writing – review & editing.
Funding
The author(s) declare financial support was received for the research, authorship, and/or publication of this article. The authors acknowledge the financial and technical support from Tecnologico de Monterrey, through the “ChallengeBased Research Funding Program 2022” (Project ID # I005-IFE001- C2-T3-T). Also, academic support comes from the Writing Lab, the Institute for the Future of Education, Tecnologico de Monterrey, Mexico.
Conflict of interest
The authors declare that the research was conducted in the absence of any commercial or financial relationships that could be construed as a potential conflict of interest.
Publisher’s note
All claims expressed in this article are solely those of the authors and do not necessarily represent those of their affiliated organizations, or those of the publisher, the editors and the reviewers. Any product that may be evaluated in this article, or claim that may be made by its manufacturer, is not guaranteed or endorsed by the publisher.
Supplementary material
The Supplementary material for this article can be found online at: https://www.frontiersin.org/articles/10.3389/feduc.2024.1392091/full#supplementary-material
Footnotes
References
Akiba, D., and Fraboni, M. C. (2023). AI-supported academic advising: exploring ChatGPT’s current state and future potential toward student empowerment. Educ Sci 13:885. doi: 10.3390/educsci13090885
Al-Hunaiyyan, A., Al-Sharhan, S., and AlHajri, R. (2020). Prospects and challenges of learning Management Systems in Higher Education. Int. J. Adv. Comput. Sci. Appl. 11:2020. doi: 10.14569/IJACSA.2020.0111209
Alhwaiti, M. (2023). Acceptance of artificial intelligence application in the post-Covid era and its impact on faculty members’ occupational well-being and teaching self efficacy: a path analysis using the UTAUT 2 model. Appl. Artif. Intell. 37. doi: 10.1080/08839514.2023.2175110
Alqahtani, T., Badreldin, H. A., Alrashed, M., Alshaya, A. I., Alghamdi, S. S., bin Saleh, K., et al. (2023). The emergent role of artificial intelligence, natural learning processing, and large language models in higher education and research. Res. Soc. Adm. Pharm. 19, 1236–1242. doi: 10.1016/j.sapharm.2023.05.016
Angelov, P. P., Soares, E. A., Jiang, R., Arnold, N. I., and Atkinson, P. M. (2021). Explainable artificial intelligence: an analytical review. WIREs Data Mining Knowledge Discov. 11:e1424. doi: 10.1002/widm.1424
Baig, M. I., and Yadegaridehkordi, E. (2023). Flipped classroom in higher education: a systematic literature review and research challenges. Int. J. Educ. Technol. High. Educ. 20:61. doi: 10.1186/s41239-023-00430-5
Banh, L., and Strobel, G. (2023). Generative artificial intelligence. Electron. Mark. 33:63. doi: 10.1007/s12525-023-00680-1
Barrett, A., and Pack, A. (2023). Not quite eye to a.I.: student and teacher perspectives on the use of generative artificial intelligence in the writing process. Int. J. Educ. Technol. High. Educ. 20:59. doi: 10.1186/s41239-023-00427-0
Bauer, E., Greisel, M., Kuznetsov, I., Berndt, M., Kollar, I., Dresel, M., et al. (2023). Using natural language processing to support peer-feedback in the age of artificial intelligence: a cross-disciplinary framework and a research agenda. Br. J. Educ. Technol. 54, 1222–1245. doi: 10.1111/bjet.13336
Benavides, L., Tamayo Arias, J., Arango Serna, M., Branch Bedoya, J., and Burgos, D. (2020). Digital transformation in higher education institutions: a systematic literature review. Sensors 20:3291. doi: 10.3390/s20113291
Bengio, Y., Lecun, Y., and Hinton, G. (2021). Deep learning for AI. Commun. ACM 64, 58–65. doi: 10.1145/3448250
Bernabei, M., Colabianchi, S., Falegnami, A., and Costantino, F. (2023). Students’ use of large language models in engineering education: a case study on technology acceptance, perceptions, efficacy, and detection chances. Comput. Educ. Artific. Intellig. 5:100172. doi: 10.1016/j.caeai.2023.100172
Biri, S. K., Kumar, S., Panigrahi, M., Mondal, S., Behera, J. K., and Mondal, H. (2023). Assessing the utilization of large language models in medical education: insights from undergraduate medical students. Cureus 15:e47468. doi: 10.7759/cureus.47468
Bizami, N. A., Tasir, Z., and Kew, S. N. (2023). Innovative pedagogical principles and technological tools capabilities for immersive blended learning: a systematic literature review. Educ. Inf. Technol. 28, 1373–1425. doi: 10.1007/s10639-022-11243-w
Blaschke, L. M., and Marín, V. I. (2020). Applications of heutagogy in the educational use of e-portfolios. Rev. Educ. Distan. 20. doi: 10.6018/RED.407831
Böhm, K., and Schedlberger, L.-M. (2023). The use of generative AI in the domain of human creations-a case for co-evolution? CEUR Workshop Proc.
Bradley, P., Nordheim, L., De La Harpe, D., Innvær, S., and Thompson, C. (2005). A systematic review of qualitative literature on educational interventions for evidence-based practice. Learn. Health Soc. Care 4, 89–109. doi: 10.1111/j.1473-6861.2005.00095.x
Brill, T. M., Munoz, L., and Miller, R. J. (2019). Siri, Alexa, and other digital assistants: a study of customer satisfaction with artificial intelligence applications. J. Mark. Manag. 35, 1401–1436. doi: 10.1080/0267257X.2019.1687571
Cabero-Almenara, J., and Ruiz-Palermo, J. (2017). Las Tecnologías de la Información y Comunicación para la inclusión: reformulando la brecha digital. Int. J. Educ. Res. Innov. 9, 16–30.
Cao, Y., Li, S., Liu, Y., Yan, Z., Dai, Y., Yu, P.S., et al. (2023). A comprehensive survey of AI-generated content (AIGC): A history of generative AI from GAN to ChatGPT.
Cheung, B. H. H., Lau, G. K. K., Wong, G. T. C., Lee, E. Y. P., Kulkarni, D., Seow, C. S., et al. (2023). ChatGPT versus human in generating medical graduate exam multiple choice questions—a multinational prospective study (Hong Kong S.A.R., Singapore, Ireland, and the United Kingdom). PLoS One 18:e0290691. doi: 10.1371/journal.pone.0290691
Chun, J., and Elkins, K. (2023). The crisis of artificial intelligence: a new digital humanities curriculum for human-Centred AI. Int. J. Hum. Arts Comp. 17, 147–167. doi: 10.3366/ijhac.2023.0310
Ciolacu, M.I., Binder, L., Svasta, P., Tache, I., and Stoichescu, D. (2019). “Education 4.0—jump to innovation with IoT in higher education” in 2019 IEEE 25th International Symposium for Design and Technology in Electronic Packaging (SIITME). IEEE, pp. 135–141.
Cowling, M., Crawford, J., Allen, K.-A., and Wehmeyer, M. (2023). Using leadership to leverage ChatGPT and artificial intelligence for undergraduate and postgraduate research supervision. Australas. J. Educ. Technol. 39, 89–103. doi: 10.14742/ajet.8598
Crawford, J., Cowling, M., and Allen, K.-A. (2023). Leadership is needed for ethical ChatGPT: character, assessment, and learning using artificial intelligence (AI). J. Univ. Teach. Learn. Pract. 20. doi: 10.53761/1.20.3.02
Dale, R., and Viethen, J. (2021). The automated writing assistance landscape in 2021. Nat. Lang. Eng. 27, 511–518. doi: 10.1017/S1351324921000164
Deng, L. (2018). Artificial intelligence in the rising wave of deep learning: the historical path and future outlook [perspectives]. IEEE Signal Process. Mag. 35, 180–177. doi: 10.1109/MSP.2017.2762725
Devlin, J., Chang, M.-W., Lee, K., and Toutanova, K. (2019). “BERT: pre-training of deep bidirectional transformers for language understanding” in Proceedings of the 2019 Conference of the North. Association for Computational Linguistics, Stroudsburg, PA, USA, pp. 4171–4186.
Dwivedi, Y. K., Kshetri, N., Hughes, L., Slade, E. L., Jeyaraj, A., Kar, A. K., et al. (2023). “So what if ChatGPT wrote it?” multidisciplinary perspectives on opportunities, challenges and implications of generative conversational AI for research, practice and policy. Int. J. Inf. Manag. 71:102642. doi: 10.1016/j.ijinfomgt.2023.102642
Escalante, J., Pack, A., and Barrett, A. (2023). AI-generated feedback on writing: insights into efficacy and ENL student preference. Int. J. Educ. Technol. High. Educ. 20:57. doi: 10.1186/s41239-023-00425-2
Farah, J. C., Ingram, S., Spaenlehauer, B., Lasne, F. K.-L., and Gillet, D. (2023). Prompting Large Language Models to Power Educational Chatbots. in Advances in Web-Based Learning – ICWL 2023. ICWL 2023. Lecture Notes in Computer Science. (Eds.) Xie, H., Lai, CL., Chen, W., Xu, G., and Popescu, E. 14409. Singapore: Springer. doi: 10.1007/978-981-99-8385-8_14
Fariani, R. I., Junus, K., and Santoso, H. B. (2023). A systematic literature review on personalised learning in the higher education context. Technol. Knowl. Learn. 28, 449–476. doi: 10.1007/s10758-022-09628-4
Fecher, B., Hebing, M., Laufer, M., Pohle, J., and Sofsky, F. (2023). Friend or foe? Exploring the implications of large language models on the science system. AI & Soc. 1–36. doi: 10.1007/s00146-023-01791-1
Floridi, L., and Chiriatti, M. (2020). GPT-3: its nature, scope, limits, and consequences. Minds Mach 30, 681–694. doi: 10.1007/s11023-020-09548-1
Fontes, F.D., Cabral, G.R.E., and Ramalho, G.L. (2021). “An open source platform to assist the creation of group playlists through artificial intelligence algorithms” in Anais Do XVIII Simpósio Brasileiro de Computação Musical (SBCM 2021). Sociedade Brasileira de Computação—SBC, pp. 166–169.
Forte-Celaya, J., Ibarra, L., and Glasserman-Morales, L. D. (2021). Analysis of creative thinking skills development under active learning strategies. Educ Sci 11:621. doi: 10.3390/educsci11100621
Fox, S., and Leake, D. B. (2001). Introspective reasoning for index refinement in case-based reasoning. J. Exp. Theor. Artific. Intellig. 13, 63–88. doi: 10.1080/09528130010029794
Gan, W., Qi, Z., Wu, J., and Lin, J.C.-W. (2023). “Large language models in education: vision and opportunities” in 2023 IEEE International Conference on Big Data (BigData). IEEE, pp. 4776–4785.
George Reyes, C. E., and Glasserman-Morales, L. D. (2021). Research competencies mediated by technologies: a systematic mapping of the literature. Educ. Knowledge Soc. 22, 1–16. doi: 10.14201/EKS.23897
Gillpatrick, T. (2020). Innovation and the digital transformation of education. Sınırsız Eğitim Araştırma Dergisi 5, 194–201. doi: 10.29250/sead.797372
Glasserman-Morales, L. D., Carlos-Arroyo, M., Ruiz-Ramirez, J. A., and Alcantar-Nieblas, C. (2023). Use of wearable devices in the teaching-learning process: a systematic review of the literature. Front Educ 8:1220688. doi: 10.3389/feduc.2023.1220688
Harshvardhan, G. M., Gourisaria, M. K., Pandey, M., and Rautaray, S. S. (2020). A comprehensive survey and analysis of generative models in machine learning. Comput. Sci. Rev. 38:100285. doi: 10.1016/j.cosrev.2020.100285
González-Pérez, L. I., and Ramírez-Montoya, M. S. (2022). Components of education 4.0 in 21st century skills frameworks: systematic review. Sustainability 14:1493. doi: 10.3390/su14031493
Guleria, A., Krishan, K., Sharma, V., and Kanchan, T. (2023). ChatGPT: ethical concerns and challenges in academics and research. J. Infect. Dev. Countries 17, 1292–1299. doi: 10.3855/jidc.18738
Guo, Y., and Lee, D. (2023). Leveraging ChatGPT for enhancing critical thinking skills. J. Chem. Educ. 100, 4876–4883. doi: 10.1021/acs.jchemed.3c00505
Hackl, V., Müller, A. E., Granitzer, M., and Sailer, M. (2023). Is GPT-4 a reliable rater? Evaluating consistency in GPT-4’s text ratings. Front Educ 8:1272229. doi: 10.3389/feduc.2023.1272229
Harika, J., Baleeshwar, P., Navya, K., and Shanmugasundaram, H. (2022). “A review on artificial intelligence with deep human reasoning” in 2022 International Conference on Applied Artificial Intelligence and Computing (ICAAIC). IEEE, pp. 81–84.
Hariri, W. (2023). Unlocking the potential of ChatGPT: A comprehensive exploration of its applications, advantages, limitations, and future directions in natural language processing.
Hassan, R. H. (2023). Educational vlogs: a systematic review. SAGE Open 13:215824402311524. doi: 10.1177/21582440231152403
Huang, Y., Gomaa, A., Semrau, S., Haderlein, M., Lettmaier, S., Weissmann, T., et al. (2023a). Benchmarking ChatGPT-4 on a radiation oncology in-training exam and red journal gray zone cases: potentials and challenges for ai-assisted medical education and decision making in radiation oncology. Front. Oncol. 13:1265024. doi: 10.3389/fonc.2023.1265024
Huang, R. S., Lu, K. J. Q., Meaney, C., Kemppainen, J., Punnett, A., and Leung, F.-H. (2023b). Assessment of resident and AI Chatbot performance on the University of Toronto Family Medicine Residency Progress Test: comparative study. JMIR Med. Educ. 9:e50514. doi: 10.2196/50514
Ivanov, S., and Soliman, M. (2023). Game of algorithms: ChatGPT implications for the future of tourism education and research. J. Tour. Futures 9, 214–221. doi: 10.1108/JTF-02-2023-0038
Jankovic, A., Mincic, D., Petrovic, N., and Tosic, M. (2023). “ChatGPT assisted development of Laravel applications” in 2023 16th International Conference on Advanced Technologies, Systems and Services in Telecommunications (TELSIKS). 340–343.
Kasneci, E., Kathrin, S., Stefan, K., Maria, B., Daryna, D., Frank, F., et al. (2023). ChatGPT for Good? On Opportunities and Challenges of Large Language Models for Education. Learning and Individual Differences 103:102274. doi: 10.1016/j.lindif.2023.102274
Kaur, J., Bhatia, S., Aggarwal, M., Bansal, R., and Krishnamurthy, B. (2022). “LM-CORE: language models with contextually relevant external knowledge” in Findings of the Association for Computational Linguistics: NAACL 2022. Association for Computational Linguistics, Stroudsburg, PA, USA, 750–769.
Khandelwal, K. (2023). A study to know—use of AI for personalized recommendation, streaming optimization, and original content production at Netflix. Int. J. Sci. Res. Eng. Trends 9, 1738–1743. doi: 10.61137/ijsret.vol.9.issue6.119
Killian, C. M., Marttinen, R., Howley, D., Sargent, J., and Jones, E. M. (2023). “Knock, Knock … Who’s there?” ChatGPT and artificial intelligence-powered large language models: reflections on potential impacts within health and physical education teacher education. J. Teach. Phys. Educ. 42, 385–389. doi: 10.1123/jtpe.2023-0058
Kitchenham, B., and Charters, S. (2007). Guidelines for performing systematic literature reviews in software engineering. Keele. Available at: https://www.elsevier.com/__data/promis_misc/525444systematicreviewsguide.pdf
Kitchenham, B., Pretorius, R., Budgen, D., Pearl Brereton, O., Turner, M., Niazi, M., et al. (2010). Systematic literature reviews in software engineering—a tertiary study. Inf. Softw. Technol. 52, 792–805. doi: 10.1016/j.infsof.2010.03.006
Kostka, I., and Toncelli, R. (2023). Exploring Applications of ChatGPT to English Language Teaching: Opportunities, Challenges, and Recommendations. Teaching English as a Second or Foreign Language--TESL-EJ 27.
Kovaliuk, T., and Kobets, N. (2021). “The Concept of an Innovative Educational Ecosystem of Ukraine in the Context of the Approach ‘Education 4.0 for Industry 4.0,’” in CEUR Workshop Proceedings.
Kuramitsu, K., Obara, Y., Sato, M., and Obara, M. (2023). “KOGI: a seamless integration of ChatGPT into Jupyter environments for programming education” in Proceedings of the 2023 ACM SIGPLAN International Symposium on SPLASH-E. 50–59.
Laato, S., Morschheuser, B., Hamari, J., and Björne, J. (2023). “AI-assisted learning with ChatGPT and large language models: implications for higher education” in 2023 IEEE International Conference on Advanced Learning Technologies (ICALT). 226–230.
Lacey, M. M., and David, P. S. (2023). Teaching and Assessment of the Future Today: Higher Education and AI. Microbiology Australia 44, 124–126. doi: 10.1071/MA23036
Lamba, S. H., Singh, R. K., and Soni, E. K. (2023). Generative adversarial network (GAN) to generate realistic images. Int. J. Res. Appl. Sci. Eng. Technol. 11, 2190–2196. doi: 10.22214/ijraset.2023.50306
Lehmann, F., and Buschek, D. (2021). Examining autocompletion as a basic concept for interaction with generative AI. I-com 19, 251–264. doi: 10.1515/icom-2020-0025
Li, Y., Sha, L., Yan, L., Lin, J., Raković, M., Galbraith, K., et al. (2023). Can large language models write reflectively. Comput. Educ. Artific. Intellig. 4:100140. doi: 10.1016/j.caeai.2023.100140
Liu, M.-Y., Huang, X., Yu, J., Wang, T.-C., and Mallya, A. (2021). Generative adversarial networks for image and video synthesis: algorithms and applications. Proc. IEEE 109, 839–862. doi: 10.1109/JPROC.2021.3049196
Majewski, M., and Kacalak, W. (2006). Intelligent system for natural language processing. pp. 742–747.
Martin, P. P., Kranz, D., Wulff, P., and Graulich, N. (2023). Exploring new depths: applying machine learning for the analysis of student argumentation in chemistry. J. Res. Sci. Teach. 1–36. doi: 10.1002/tea.21903
Matsumoto-Royo, K., Ramírez-Montoya, M. S., and Conget, P. (2021). Opportunities to develop lifelong learning tendencies in practice-based teacher education: getting ready for education 4.0. Future Internet 13:292. doi: 10.3390/fi13110292
McCarthy, J., Minsky, M., Rochester, N., and Shannon, C. (2006). A proposal for the Dartmouth summer research project on artificial intelligence, august 31, 1955. AI Mag. 27:12. doi: 10.1609/aimag.v27i4.1904
Mills, A., Bali, M., and Eaton, L. (2023). How do we respond to generative AI in education? Open educational practices give us a framework for an ongoing process. J. Appl. Learn. Teach. 6. doi: 10.37074/jalt.2023.6.1.34
Miranda, J., Navarrete, C., Noguez, J., Molina-Espinosa, J. M., Ramírez-Montoya, M. S., Navarro-Tuch, S. A., et al. (2021). The core components of education 4.0 in higher education: three case studies in engineering education. Comput. Electr. Eng. 93:107278. doi: 10.1016/j.compeleceng.2021.107278
Moraes, E. B., Kipper, L. M., Hackenhaar Kellermann, A. C., Austria, L., Leivas, P., Moraes, J. A. R., et al. (2023). Integration of industry 4.0 technologies with education 4.0: advantages for improvements in learning. Interact. Technol. Smart Educ. 20, 271–287. doi: 10.1108/ITSE-11-2021-0201
Moya Figueroa, B. A., and Eaton, S. E. (2023). Examining recommendations for artificial intelligence use with integrity from a scholarship of teaching and learning lens. Rev. Electrón. Investig. Eval. Educ. 29. doi: 10.30827/relieve.v29i2.29295
Mühlhoff, R. (2020). Human-aided artificial intelligence: or, how to run large computations in human brains? Toward a media sociology of machine learning. New Media Soc. 22, 1868–1884. doi: 10.1177/1461444819885334
Mukul, E., and Büyüközkan, G. (2023). Digital transformation in education: a systematic review of education 4.0. Technol forecast. Soc Change 194:122664. doi: 10.1016/j.techfore.2023.122664
Neumann, M., Rauschenberger, M., and Schön, E.-M. (2023). “We Need To Talk About ChatGPT”: The Future of AI and Higher Education in 2023 IEEE/ACM 5th International Workshop on Software Engineering Education for the Next Generation (SEENG). 29–32.
Perkins, M. (2023). Academic integrity considerations of AI large language models in the post-pandemic era: ChatGPT and beyond. J. Univ. Teach. Learn. Pract. 20. doi: 10.53761/1.20.02.07
Petersen, K., Vakkalanka, S., and Kuzniarz, L. (2015). Guidelines for conducting systematic mapping studies in software engineering: an update. Inf. Softw. Technol. 64, 1–18. doi: 10.1016/j.infsof.2015.03.007
Pizard, S., Acerenza, F., Otegui, X., Moreno, S., Vallespir, D., and Kitchenham, B. (2021). Training students in evidence-based software engineering and systematic reviews: a systematic review and empirical study. Empir. Softw. Eng. 26:50. doi: 10.1007/s10664-021-09953-9
Prentzas, J., and Sidiropoulou, M. (2023). “Assessing the use of open AI chat-GPT in a University Department of Education” in 2023 14th International Conference on Information, Intelligence, Systems & Applications (IISA). 1–4.
Rafi, I., Nurjannah, F. F., Fabella, I. R., and Andayani, S. (2020). Peluang dan Tantangan Pengintegrasian Learning Management System (LMS) dalam Pembelajaran Matematika di Indonesia. J. Tadris Matemat. 3, 229–248. doi: 10.21274/jtm.2020.3.2.229-248
Rahma, R. A., Sucipto, A., Affriyenni, Y., and Widyaswari, M. (2021). Cybergogy as a digital media to facilitate the learning style of millennial college students. World J. Educ. Technol. Curr. Issues 13, 223–235. doi: 10.18844/wjet.v13i2.5691
Ramírez-Montoya, M. S., Castillo-Martínez, I. M., Sanabria-Z, J., and Miranda, J. (2022a). Complex thinking in the framework of education 4.0 and open innovation—a systematic literature review. J. Open Innov.: Technol. Mark. Complex. 8:4. doi: 10.3390/joitmc8010004
Ramírez-Montoya, M.-S., and Lugo-Ocando, J. (2020). Systematic review of mixed methods in the framework of educational innovation. Comunicar 28, 9–20. doi: 10.3916/C65-2020-01
Ramírez-Montoya, M. S., McGreal, R., and Obiageli-Agbu, J. F. (2022b). Horizontes digitales complejos en el futuro de la educación 4.0: luces desde las recomendaciones de UNESCO. Rev. Iberoam. Educ. Distan. 25, 09–21. doi: 10.5944/ried.25.2.33843
Revuelta-Domínguez, F.I., and Pedrera-Rodríguez, M.I. (2020). Retos y evidencias en la investigación con videojuegos en educación. Ediciones Octaedro, S. L.
Rheingold, H., Brett, G., Corneli, J., Danoff, C. J., Larson, K., Pierce, C., et al. (2014). The Peeragogy Handbook : Pierce Press and PubDomEd, 287.
Rosalina, D., Kartika, Y., Dias, S., and Muhammad, R. Z. (2021). Factors Influencing the Digital Literacy Competency of College Students in the Industrial Revolution Era 4.0. International Journal of Economics, Business and Applications 6:81. doi: 10.31258/ijeba.6.2.81-92
Sabando Barreiro, A. V. (2021). Educación 4.0 y su impacto en el sistema educativo durante la pandemia y post pandemia Covid 19 en el Ecuador. Sinerg. Educ. 7. doi: 10.37954/se.v7i1.332
Sallam, M., Salim, N. A., Barakat, M., Al-Mahzoum, K., Al-Tammemi, A. B., Malaeb, D., et al. (2023). Assessing health students’ attitudes and usage of ChatGPT in Jordan: validation study. JMIR Med. Educ. 9:e48254. doi: 10.2196/48254
Sanabria-Navarro, J.-R., Silveira-Pérez, Y., Pérez-Bravo, D.-D., and de-Jesús-Cortina-Núñez, M. (2023). Incidences of artificial intelligence in contemporary education. Comunicar 77, 97–107. doi: 10.3916/C77-2023-08
Santos, S., Tedesco, P., Borba, M., and Brito, M. (2020). “Innovative approaches in teaching programming: a systematic literature review’ in Proceedings of the 12th International Conference on Computer Supported Education, 205–214.
Sarker, D., Eiaz-Ur-Rahman, A. F. M., Sakib, A. R., Terano, H. J. R., and Rahman, M. M. (2023). ChatGPT’s applications in higher education: unmasking opportunities and challenges. J. Educ. Manag. Dev. Stud. 3, 37–47. doi: 10.52631/jemds.v3i4.250
Shah, C. (2023). “Generative AI and the future of information access” in Proceedings of the 32nd ACM International Conference on Information and Knowledge Management. ACM, New York, NY, USA, pp. 3–3.
Shrivastava, A. S. J., Suji, P., Ajay, R. Y., Mani, P., Pooja, N., and Abhay, C. (2023). IoT Based RFID Attendance Monitoring System of Students Using Arduino ESP8266 & Adafruit.Io on Defined Area. Cybernetics and Systems, 1–12. doi: 10.1080/01969722.2023.2166243
Sikdar, S. (2018). Artificial intelligence, its impact on innovation, and the Google effect. Clean Techn. Environ. Policy 20, 1–2. doi: 10.1007/s10098-017-1478-y
Snoussi, T. (2019). Learning management system in education: opportunities and challenges. Int. J. Innov. Technol. Explor. Eng. 8, 664–667. doi: 10.35940/ijitee.L1161.10812S19
Sohail, S. S., Farhat, F., Himeur, Y., Nadeem, M., Madsen, D. Ø., Singh, Y., et al. (2023). Decoding ChatGPT: a taxonomy of existing research, current challenges, and possible future directions. J. King Saud Univ. 35:101675. doi: 10.1016/j.jksuci.2023.101675
Sridhar, P., Doyle, A., Agarwal, A., Bogart, C., Savelka, J., and Sakr, M. (2023). Harnessing LLMs in curricular design: Using GPT-4 to support authoring of learning objectives. Empir. Softw. Eng. 26:50.
Suarez-Brito, P., Baena-Rojas, J. J., López-Caudana, E. O., and Glasserman-Morales, L. D. (2022). Academic literacy as a component of complex thinking in higher education: a scoping review. Eur. J. Contemp. Educ. 11, 931–945. doi: 10.13187/ejced.2022.3.931
Sumarsono, (2020). The paradigms of heutagogy and cybergogy in the transdisciplinary perspective. J. Pendid. Pengajaran 52, 172–182. doi: 10.23887/jpp.v52i3.22882
Tajudin, M. N., Suhaimi, N. A., Adnan, M., and Puteh, M. (2020). Promoting transformative mathematical learning through heutagogy, paragogy and cybergogy approaches. Palarch’s J. Archaeol. Egypt/Egyptology 17, 481–497.
Tsai, M.-L., Ong, C. W., and Chen, C.-L. (2023). Exploring the use of large language models (LLMs) in chemical engineering education: building core course problem models with chat-GPT. Educ. Chem. Eng. 44, 71–95. doi: 10.1016/j.ece.2023.05.001
Udovita, V. (2020). Conceptual Review on Digital Transformation of Higher Education. SSRN Electronic Journal. 13. doi: 10.2139/ssrn.3850993
Ülkü, A. (2023). Artificial intelligence-based large language models and integrity of exams and assignments in higher education: the case of tourism courses. Tour. Manag. Stud. 19, 21–34. doi: 10.18089/tms.2023.190402
Vicente-Yagüe-Jara, M. I., López-Martínez, O., Navarro-Navarro, V., and Cuéllar-Santiago, F. (2023). Writing, creativity, and artificial intelligence. ChatGPT in the university context. Comunicar 77, 47–57. doi: 10.3916/C77-2023-04
Walczak, K., and Cellary, W. (2023). Challenges for higher education in the era of widespread access to generative AI. Econ. Bus. Rev. 9. doi: 10.18559/ebr.2023.2.743
Wang, L., Lyu, C., Ji, T., Zhang, Z., Yu, D., Shi, S., et al. (2023). Document-level machine translation with large language models. arXiv [Preprint]. doi: 10.48550/arXiv.2304.02210
Whitehouse, C., Choudhury, M., and Aji, A.F., (2023). LLM-powered data augmentation for enhanced cross-lingual performance. arXiv [Preprint]. doi: 10.48550/arXiv.2305.14288
Williams, D. O., and Fadda, E. (2023). Can ChatGPT pass Glycobiology? Glycobiology 33, 606–614. doi: 10.1093/glycob/cwad064
Xu, L. D., Lu, Y., and Li, L. (2021). Embedding blockchain technology into IoT for security: a survey. IEEE Internet Things J. 8, 10452–10473. doi: 10.1109/JIOT.2021.3060508
Zaoui, F., and Nissrine, S. (2020). Roadmap for Digital Transformation: A Literature Review. Procedia Computer Science. 175, 621–628. doi: 10.1016/j.procs.2020.07.090
Zhai, X., Xiaoyan, C., Ching, S. C., Morris, S. Y. J., Andreja, I., Michael, S., et al. (2021). A Review of Artificial Intelligence (AI) in Education from 2010 to 2020. Complexity, 1–18. doi: 10.1155/2021/8812542
Zhang, C., and Chen, Y. (2020). A review of research relevant to the emerging industry trends: industry 4.0, IoT, Blockchain, and business analytics. J. Indus. Integ. Manag. 5, 165–180. doi: 10.1142/S2424862219500192
Zhang, C., and Lu, Y. (2021). Study on artificial intelligence: the state of the art and future prospects. J. Ind. Inf. Integr. 23:100224. doi: 10.1016/j.jii.2021.100224
Zhuhadar, L. P., and Lytras, M. D. (2023). The application of AutoML techniques in diabetes diagnosis: current approaches, performance, and future directions. Sustain. For. 15:13484. doi: 10.3390/su151813484
Zmyzgova, T. R., Polyakova, E. N., and Karpov, E. K., (2020). Digital Transformation of Education and Artificial Intelligence. Proceedings of the 2nd International Scientific and Practical Conference “Modern Management Trends and the Digital Economy: From Regional Development to Global Economic Growth” (MTDE 2020).
Keywords: Artificial Intelligence (AI), large language model (LLM), generative artificial intelligence (GAI), Education 4.0, higher education, innovation in education
Citation: Peláez-Sánchez IC, Velarde-Camaqui D and Glasserman-Morales LD (2024) The impact of large language models on higher education: exploring the connection between AI and Education 4.0. Front. Educ. 9:1392091. doi: 10.3389/feduc.2024.1392091
Edited by:
Diana Hernández Montoya, Universidad Estatal a Distancia, Costa RicaReviewed by:
Janika Leoste, Tallinn University, EstoniaAlicia García-Holgado, University of Salamanca, Spain
Copyright © 2024 Peláez-Sánchez, Velarde-Camaqui and Glasserman-Morales. This is an open-access article distributed under the terms of the Creative Commons Attribution License (CC BY). The use, distribution or reproduction in other forums is permitted, provided the original author(s) and the copyright owner(s) are credited and that the original publication in this journal is cited, in accordance with accepted academic practice. No use, distribution or reproduction is permitted which does not comply with these terms.
*Correspondence: Iris Cristina Peláez-Sánchez, cristina.pelaez@tec.mx