Simulation and economic analysis of the high-temperature heat storage system of thermal power plants oriented to the smart grid
- 1National Institute of Clean and Low Carbon Energy, Beijing, China
- 2CHN Energy Jinjie Energy Co. Ltd., Yulin, China
With the continuous increase of the grid-connected proportion of intermittent renewable energy, in order to ensure the reliability of smart grid operation, it is urgent to improve the operational flexibility of thermal power plants. Electric heat storage technology has broad prospects in terms of in-depth peak shaving of power grids, improving new energy utilization rates and improving the environment. It is an important means to promote electric energy substitution. In this study, the economics of technical application scenarios are compared and analyzed, the principle of solid heat storage technology is discussed, and its application in heating fields such as industrial steam, district heating, and deep peak regulation of congeneration units is expounded. The results indicate that in the scenario where the peak shaving subsidy and the heat storage duration are the same, as the unit output increases, the investment recovery period increases. Moreover, the results also indicate that in the 0.3 yuan/kW power market peaking subsidy scenario, only when the unit output is 0 and the heat storage time is greater than 8 h, the investment can be recovered in 5 years, while in the 0.7 yuan/kW power market peaking subsidy scenario, except for the scenario where the unit output is 40% and the heat storage time is 7 h, the investment cannot be recovered; in other scenarios, the investment can be recovered within 5 years.
1 Introduction
In 2000, a smart power system concept was established with the primary goal of integrating two-way communication into the infrastructure of a standard grid system (Riaz et al., 2020; Fu et al., 2020; National Energy Administration, 2021). In order to achieve the goal of carbon neutrality, clean, efficient, and flexible operation has become an important goal of the transformation and development of the thermal power industry, and more and more attention has been paid to the flexibility transformation technology of thermal power plants (Fu et al., 2015; Riaz et al., 2020). Among them, the cost of the flexible retrofit, operating costs, and peak shaving benefits under the market rules of electric auxiliary services are the keys to choosing the most suitable retrofit technology (State Grid Corporation, 2013).
The summarized literature overview is tabulated in Table 1Ahmad et al., 2020, Long et al., 2022, National energy administration, 2019, Pasta et al., 2012. This is explained in greater detail as follows. In the recently released “Northeast Electric Power Auxiliary Service Market Operating Rules (Interim)” (Yang et al., 2011; Pasta et al., 2012; National energy administration, 2019; Ahmad et al., 2020; Long et al., 2022), the market rules have been further improved and upgraded (Hughes, 2010; Singh et al., 2015). The new rules design a day-head bidding mechanism for the peak rotating reserve market to achieve full coverage of the auxiliary service market “trough lows and peak peaks” (Fu et al., 2017). Only the two-way peak shaving units that can “go up and down” can obtain all the benefits of auxiliary services (Chinese Government, 2020) and put forward a complete flexibility standard for thermal power units, which can motivate and guide thermal power plants to adopt appropriate flexibility transformation technologies and comprehensively improve the peak shaving capacity of units (Rong et al., 2008; Deng et al., 2016). Among many energy storage technologies, thermal energy storage is one of the most promising large-scale energy storage technologies (Singh et al., 2016). Compared with other energy storage technology routes such as electrochemical energy storage and electrical energy storage, thermal energy storage has obvious advantages in terms of installed capacity, energy storage density, technology cost, service life, etc., (Huang et al., 2021). Compared with these two mechanical energy storage technologies, thermal energy storage technology has many advantages, such as small footprint, low cost, high energy storage density, small impact on the environment, and is not restricted by geographical and environmental conditions; it has obvious scale effects (Desrues et al., 2010). According to the needs of users, it can realize the combined supply of cold, heat, electricity, and steam of various energy grades; it can realize peak shaving and valley filling, two-way adjustment, and absorb the installed output of intermittent new energy (wind power, photo voltaic, etc.,) for the regional power grid (Rodríguez et al., 2009). The best solution is to balance the peak-to-valley difference; a large number of cycles, long life, and the bidirectional regulation function of the energy storage power station will not lead to a decrease in efficiency with long-term heat storage cycles (Suárez et al., 2015); no chemical reaction in the storage and storage processes and technical parameters and processes can be controlled, with high system security (Wang et al., 2015). Thermal energy storage technology can be applied to the power supply side, the grid side, and the user side (Hall et al., 1979). On the user side, thermal energy storage technology can be applied to user cooling, heating, electricity-integrated energy services, seawater desalination, and other occasions; in the direct utilization of thermal energy, thermal energy storage technology has higher energy utilization efficiency than electricity storage technology (Yang et al., 2018a; Yang et al., 2018b; Zhang et al., 2020); thermal technology also includes the storage and utilization of thermal energy below ambient temperature, that is, cold storage technology has been maturely applied in cold chain-related fields, and the market size is also expanding (Tao and He, 2015). For the power supply and grid side, the current power system presents a “double-high” characteristic of a high proportion of renewable energy and a high proportion of power electronic equipment, the system’s moment of inertia continues to decline, and the frequency and voltage regulation capabilities are insufficient, posing severe challenges to grid security; solar thermal energy storage power generation can effectively realize frequency regulation through the rotational inertia of the steam turbine generator set; in the flexibility transformation of thermal power plants (Yaqub et al., 2016), the thermal energy storage power generation technology converts the excess steam heat that occurs when the unit operates with variable loads into a heat storage medium (Quan et al., 2013; Zhang et al., 2021b). The thermal energy is stored and released when needed, which can not only increase the peak shaving depth of the unit but can also increase the peak load capacity, with lower investment and operating costs, which has obvious advantages (Andersen, 2009). The Shapley value (SV) has been calculated to estimate the benefits of cooperative power suppliers (Tao et al., 2012). The presented case studies have verified that the proposed algorithm and the established bidding strategy exhibit higher effectiveness (Baños et al., 2011).
1.1 Motivation
From the aforementioned discussion, it is concluded that thermal energy storage already exists in a wide spectrum of applications. Sensible heat storage is used in pebble beds, packed beds, or molten salts for thermal solar power plants (Zhao and Wu, 2011; Li et al., 2017; Yin et al., 2020), in water heater storage (Denholm and Kulcinski, 2004; Denholm and Holloway, 2005; Sun et al., 2008; Zheng and Chen, 2008), and in blast or glass furnace regenerators (Carrasco et al., 2006), and it is the most used technology for heating and cooling of buildings Latent heat storage is used in buildings for passive storage systems such as phase change material walls, wall boards, and shutters (Bejan et al., 1996; Laing et al., 2006; Kuravi et al., 2013). As far as we know, solid heat storage devices with a thermal storage temperature of 900°C have not been considered for peak shaving in thermal power plants, and this study considers different peak shaving subsidy scenarios and peak shaving benefits of thermal power plants with high-temperature solid heat storage devices.
1.2 Contributions
The contributions of this study are summed up as follows.
1) A new type of high-temperature heat storage material is proposed, and its heat storage performance is compared with that of current main heat storage materials.
2) In the proposed model, according to the characteristics of the new heat storage material, THERMOFLEX simulation software was used to establish the deep peak-regulating model of the thermal power plant with a high-temperature heat storage device. The feasibility of applying the new heat storage material to deep peak regulation in thermal power plants is proved.
3) An economic analysis model is established considering the scenario of the peak-regulating subsidy, considering different load rates of units, heat storage duration, subsidies, and the corresponding capital recovery period. It provides a reference for the application of heat storage technology in practical projects.
1.2 Study organization
This study is organized as follows: Section 1 introduces the smart power system, its elements, and related research contribution, while Section 2 covers the architecture and flexible peak shaving technology for typical thermal power plants. The mathematical model and economic analysis of the high-temperature solid heat storage system are discussed in Section 3. The rest of the study is organized as follows. Simulation results are discussed in Section 4. Section 5 provides a brief summary of the whole article with concluding remarks.
2 Flexible peak-shaving technology for typical thermal power plants
At present, the flexibility of thermal power plants is mainly limited by the operation flexibility of “determining electricity by heat.” Therefore, improving the peak-shaving capacity of the heating unit is the main content of flexibility transformation. The flexibility transformation of the heating unit is mainly divided into three categories (Bai et al., 2019). One is to increase the heating capacity of the unit, reduce the boiler output, and reduce the forced output of the unit under the condition of meeting the heating load (Verda and Colella, 2011). Second is the electric heating peak regulation technology, which converts the electric energy generated by the unit into heat energy for external heating, such as the electrode boiler technology and electric boiler solid heat energy storage technology; third is the thermal energy storage peak shaving technology, which converts excess steam thermal energy in steam turbines into thermal energy of energy storage medium for storage, such as the hot water tank energy storage technology, phase change thermal energy storage technology, concrete thermal energy storage technology, and molten salt thermal energy storage technology (Tyagi and Buddhi, 2007; Morisson et al., 2008; Cao et al., 2019; Wang et al., 2019; Xing et al., 2019; Ling et al., 2020).
2.1 Increasing the unit heat supply peak regulation technology
The peak-shaving technology to increase the heating capacity of the unit is mainly to reduce the work share of the steam inside the steam turbine and convert it into heat energy for external heating, which can reduce the forced output of the steam turbine unit and show a strong peak-shaving capacity (Kocak and Paksoy, 2020). The steam turbine bypass heat supply extracts the high-temperature and high-pressure steam with a strong performance for heating and shows great potential for peak shaving, but there is a lot of thermal economic loss and higher operating cost. Used for heating, the loss of cold source is eliminated, and the operating cost is low; however, they generally need to replace the special low-pressure cylinder rotor, and the cost of transformation is high (Sowmy and Prado, 2008).
2.2 Electric heating peak regulation technology
Electric heating peak regulating technology mainly includes the electrode boiler and electric boiler solid thermal energy storage technology, which does not involve the transformation of the main equipment of the thermal power plant and has little influence on the normal operation of the thermal power plant (Lizarraga⁃Garcia and Mitsos, 2014). The solid electrode boiler and electric boiler thermal energy storage lead to electric consumption directly, external power supply, reduce the thermal power plant to increase the load capacity, low load with a load, and the large depth operation flexibility, which are good advantages, but the disadvantages are the high investment cost, operation cost is high, and suit the market early gains’ high load of the depth of the market demand, with the addition of more and more power plant auxiliary service markets (Miao et al., 2019). The demand for deep peak shaving is becoming less and less (Yin et al., 2020). In the market competition of cost sharing, it will be difficult for the heat supply peak-shaving technology with electric energy as the heat source to gain a competitive advantage (Laing et al., 2006).
2.3 Thermal energy storage peak regulation technology
The thermal energy storage peak-shaving technology is a peak-shaving technology that converts the excess steam heat that occurs when the unit is running at variable loads into thermal energy of the thermal energy storage medium and releases the heat energy when needed, thereby increasing the flexibility of the unit (Reboussin et al., 2005; Wu et al., 2015; Hao et al., 2019). For example, when there is an excess of heating steam in the heating season, the excess heat energy is stored in the thermal energy storage equipment (Jian et al., 2015). When the power load is at a low valley, the boiler load and steam turbine output are reduced to meet the low-load peak regulation requirements of the unit (Zhang et al., 2021a). Part of it is supplemented by thermal energy storage equipment; when the power load is at its peak, the boiler load is increased, the external heat supply of the steam turbine is reduced, the peak load capacity of the unit is enhanced, and the insufficient heat supply is supplemented by thermal energy storage equipment (Igreen, 2019).
To sum up, there is no high-temperature thermal energy storage technology that uses the deep peak-shaving period of thermal power plants to combine the high-temperature steam of the system with valley electricity for cascade storage and utilization.
3 Modeling and economic analysis of the high-temperature solid heat storage system
3.1 System model composition
A subcritical intermediate reheating self-heating cycle drum furnace is used, and the furnace type is HG1025/17.4-YM28. The steam turbine has a capacity of 300MW, is of subcritical intermediate reheat type, possesses a high- and medium-pressure cylinder, and is of double-cylinder double-exhaust steam single-shaft condensing type (Igreen, 2019; Zhang et al., 2021a; Thermoflow, 2021). The unit model is N300-16.7/538/538. The external supply of industrial steam by the unit is mainly divided into two specifications, namely, 1) 4.2 MPa, 420°C, 60–70 t/h; and 2) 1.8MPa, 320°C, 240 t/h.
3.2 Thermal storage system materials and performance
In this study, carbon-based high-temperature heat storage materials are used, which have the following characteristics (Soprani et al., 2019; Zhang et al., 2020; Fu, 2022; Zhang et al., 2022): 1) good heat storage and thermal conductivity (as shown in Table 2); 2) excellent high-temperature resistance characteristics, can be used under oxygen-free conditions, and the temperature can be up to 3,000°C; also, it has thermal shock resistance at high temperature and good mechanical strength; and 4) good self-lubricating performance (Duan et al., 2018; Liu et al., 2018).
3.3 System simulation and economic analysis model
3.3.1 Simulation model
THERMOFLEX software is a process simulation software application, especially developed for thermodynamics (Carrasco et al., 2006; Soprani et al., 2019; Zhang et al., 2020; Zhang et al., 2022). In terms of simulating steam balance, THERMOFLEX can complete the basic material and energy balance, and set up various logic controls integrated into the steam balance model usually.
3.3 2 System economic analysis model
Here, Y is the net income, yuan/y;M is the income, yuan/y; and C is the cost, yuan/y.
Here, M1 is the peak-shaving subsidy, yuan/y;M2 is the heat sales revenue, yuan/y; andM3 is the peak electricity revenue increase, yuan/y.
Here, C1 is the electricity cost, yuan/y;C2 is the financing charges, yuan/y; andC3 is the operation and maintenance cost, yuan/y.
4 Results and discussion
In the proposed framework, the overall framework is presented in Figure 1.
According to the new high-temperature solid heat storage system designed in this study, it can be seen from the following Figure 2 that the minimum load of the unit is effectively reduced under the condition of the constant heating load. It can increase the low-load peak load capacity of the unit but cannot increase the peak load capacity of the unit during peak load, and even the high back pressure circulating water heating transformation will reduce the unit’s peak load capacity. According to the latest auxiliary service market rules, it belongs to the flexible transformation technology of “can go down but not go up” that cannot bring comprehensive peak-shaving benefits. High-temperature thermal energy storage enables thermal power plants to have “two-way” peak-shaving capabilities, which can increase the low-load operation capacity of thermal power plants and increase the top-load capacity during peak periods and can obtain complete peak-shaving benefits. Light (valley electricity) as a heat source and thermal energy storage have a good thermal economy and low operating costs. Therefore, the thermal energy storage peak-shaving technology has the best technical and economic competitive advantage.
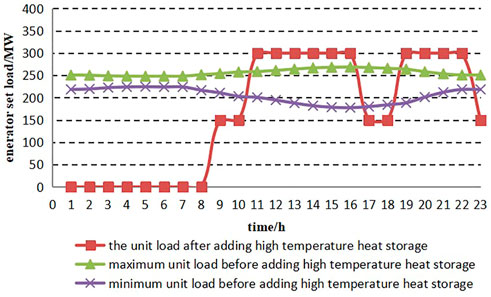
FIGURE 2. Output curves of units before and after adding high-temperature thermal energy storage devices.
The relative economic analysis is carried out using the static investment income. It calculated the return on investment years under the different outputs and heat storage time of the unit under the peak-shaving subsidy scenarios of 0.3 yuan/kW and 0.7 yuan/kW. It can be seen from Figure 3 that when the scenario of the peak-shaving subsidy in the power market is of 0.3 yuan/kW, all scenarios have static benefit years over 5 years, except that the output of the unit is 0 and the heat storage time is greater than 9 h. In addition, as shown in Figure 4, when the scenario of the peak-shaving subsidy in the power market is of 0.7 yuan/kW, all scenarios have static benefit years of less than 5 years, except that the output of the unit is 40% and the heat storage time is 7 h. Comparing Tables 3, 4, it can be seen that under the scenario of the peak-shaving subsidy in the power market of 0.3 yuan/kW, the investment can be recovered in 5 years only when the output of the unit is 0 and the heat storage time is greater than 8 h. In the scenario of the peak-shaving subsidy in the/kW power market, except for the scenario where the unit output is 40% and the heat storage time is 7 h, the investment cannot be recovered, and the investment can be recovered within 5 years in the other scenarios. Subsidies have a great impact on the payback period. In addition, in the scenario where the peak-shaving subsidy and the heat storage duration are the same, as the unit output increases, the investment recovery period increases.
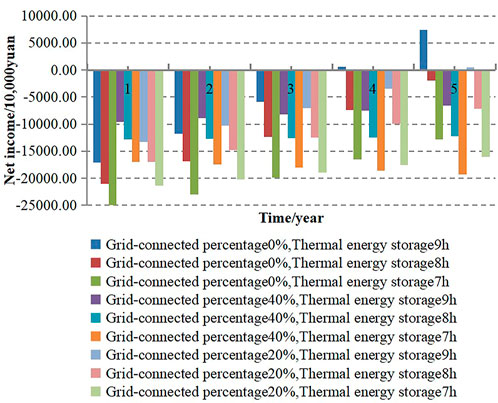
FIGURE 3. Comparison of investment returns under different outputs and heat storage duration of units (the peak-shaving subsidy is calculated at 0.3 yuan/kW).
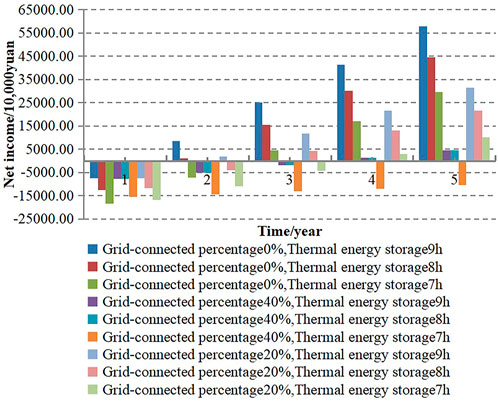
FIGURE 4. Comparison of investment returns under different outputs and heat storage duration of units (the peak-shaving subsidy is calculated at 0.7 yuan/kW).
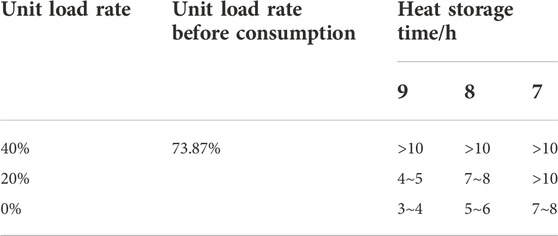
TABLE 3. Investment recovery period under different outputs and heat storage time of the unit (the peak-shaving subsidy is calculated at 0.3 yuan).
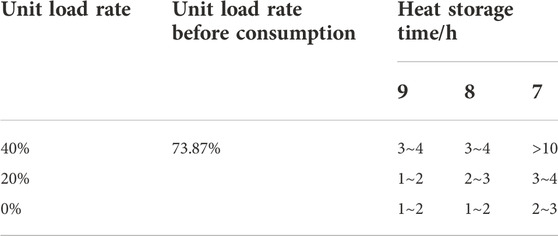
TABLE 4. Investment recovery period under different outputs and heat storage time of the unit (the peak-shaving subsidy is calculated at 0.7 yuan).
5 Conclusion
In this work, we have presented a mathematical model for simulation and economic analysis of a new high-temperature heat storage system for thermal power plants oriented to the smart grid.
1) In the flexible transformation technology of thermal power plants, the methods of increasing the heating capacity of the unit can effectively reduce the forced output of the unit and improve the power plant low-load operation flexibility, but it will reduce the peak load capacity of the unit during the peak load and face the loss of peak-shaving revenue under the new ancillary service rules. The thermal power plant adopts thermal energy storage peak regulation technology, which can not only increase the low-load operation capacity of the unit but can also increase the peak load capacity when the load is at its peak.
2) Simulation and comparison of actual data based on a thermal power plant is determined by THERMOFLEX thermal simulation software. The preliminary results show that in the model where the peak-shaving subsidy and the heat storage duration are the same, as the unit output increases, the investment recovery period increases.
3) The power market peak-shaving subsidy has a great impact on the investment recovery period. In the 0.3 yuan/kW power market peaking subsidy scenario, only when the unit output is 0 and the heat storage time is greater than 8 h, the investment can be recovered in 5 years, while in the 0.7 yuan/kW power market peaking subsidy scenario, except for the scenario where the unit output is 40% and the heat storage time is 7 h, the investment cannot be recovered; in other scenarios, the investment can be recovered within 5 years.
4) From the aforementioned discussion, we can conclude that there are still certain research gaps that need to be filled in the future. The proposed scheme is based on the simulation method. In the future, this work can be extended to integrated energy, such as cold, hot, and compressed air. More comprehensive power consumption is considered, and the proposed scheme was implemented to contribute to grid stability and better utilization of the grid energy. Similarly, in the proposed mathematical model, we did not consider the cold and compressed air; therefore, the addition of them is another research direction. Moreover, our proposed peak-shaving subsidy is based on data given for 0.3 Y/kWh and 0.7 Y/kWh; however, it can be extended for real-time scenarios.
Data availability statement
The original contributions presented in the study are included in the article/Supplementary Material; further inquiries can be directed to the corresponding author.
Author contributions
HZ organized case studies and wrote the manuscript. JL contributed to the theoretical research of this study. All authors contributed to the manuscript and approved the submitted version.
Conflict of interest
MZ and YL were employed by the company CHN Energy Jinjie Energy Co. Ltd.
The remaining authors declare that the research was conducted in the absence of any commercial or financial relationships that could be construed as a potential conflict of interest.
Publisher’s note
All claims expressed in this article are solely those of the authors and do not necessarily represent those of their affiliated organizations, or those of the publisher, the editors, and the reviewers. Any product that may be evaluated in this article, or claim that may be made by its manufacturer, is not guaranteed or endorsed by the publisher.
References
Ahmad, S., Alhaliioni, M. M., Naeem, M., Ahmad, A., and Altaf, M. (2020). Joint energy management and energy trading in residential microgrid system. IEEE Access 8, 123334–123346. doi:10.1109/access.2020.3007154
Andersen, T. V. (2009). Integration of 50% wind power in a CHP-based power system: A model-based analysis of flexible power generation. Denmark: Technical University of Denmark, Kongens Lyngby.
Bai, Z., Miao, C., and Wang, W. (2019). The value of thermal storage technology in integrated energy system. Distribution Util. 36 (3), 20–26.
Baños, R., Manzano-gugliaro, F., Montoya, F., Gil, C., Alcayde, A., and Gomez, J. (2011). Optimization methods applied to renewable and sustainable energy: A review. Renew. Sustain. Energy Rev. 15 (4), 1753–1766. doi:10.1016/j.rser.2010.12.008
Bejan, A., Tsatsaronis, G., and Moran, M. (1996). Thermal design and optimization. New Jersey, United States: John Wiley & Sons.
Cao, M., Xu, J., and Ju, J. (2019). Technical and economic analysis of user side energy storage equipment participating in power grid ancillary services[J]. Power Demand Side Manag. 21 (1), 52–55.
Carrasco, J. M., Franquelo, L. G., Bialasiewicz, J. T., Galvan, E., PortilloGuisado, R., Prats, M., et al. (2006). Power-electronic systems for the grid integration of renewable energy sources: A survey. IEEE Trans. Ind. Electron. 53 (4), 1002–1016. doi:10.1109/tie.2006.878356
Chinese Government (2020) The ceremony of presenting flower baskets to people's heroes on martyr's day will be held on september 30. Party and state leaders including xi jinping will attend. Available at: http://www.gov.cn/zhengce/zhengceku/2020-06/18/content_5520311.html (Accessed september 20, 2020).
Deng, J., Hu, L., and Li, J. (2016). Analysis on mechanism of curtailed wind power accommodation and its economic operation based on electric boiler for peak-load regulation at secondary heat supply network. Automation Electr. Power Syst. 40 (18), 41–47.
Denholm, P., and Holloway, T. (2005). Improved accounting of emissions from utility energy storage system operation. Environ. Sci. Technol. 39, 9016–9022. doi:10.1021/es0505898
Denholm, P., and Kulcinski, G. L. (2004). Life cycle energy requirements and greenhouse gas emissions from large scale energy storage systems. Energy Convers. Manag. 45, 2153–2172. doi:10.1016/j.enconman.2003.10.014
Desrues, T., Ruer, J., Marty, P., and Fourmigue, J. (2010). A thermal energy storage process for large scale electric applications. Appl. Therm. Eng. 30, 425–432. doi:10.1016/j.applthermaleng.2009.10.002
Duan, C-T., Zheng, D-F., Junqing, L., Wang, Q-S., Liang, P., and Gong, X-Y. (2018). Research progress on the characterization of mesophase pitch. New Carbon Mater. 33 (3), 193–202.
Fu, X. (2022). Statistical machine learning model for capacitor planning considering uncertainties in photovoltaic power. Prot. Control Mod. Power Syst. 7, 5. doi:10.1186/s41601-022-00228-z
Fu, X., Chen, H., Cai, R., and Yang, P. (2015). Optimal allocation and adaptive VAR control of PV-DG in distribution networks. Appl. Energy 137, 173–182. doi:10.1016/j.apenergy.2014.10.012
Fu, X., Guo, Q., and Sun, H. (2020). Statistical machine learning model for stochastic optimal planning of distribution networks considering a dynamic correlation and dimension reduction. IEEE Trans. Smart Grid 11 (4), 2904–2917. doi:10.1109/tsg.2020.2974021
Fu, X., Sun, H., Guo, Q., Pan, Z., Wen, X., and Wang, L. (2017). Uncertainty analysis of an integrated energy system based on information theory. Energy 122, 649–662. doi:10.1016/j.energy.2017.01.111
Fukahori, R., Nomura, T., Zhu, C., Sheng, N., Okinaka, N., and Akiyama, T. (2016). Macro-encapsulation of metallic phase change material using cylindrical-type ceramic containers for high-temperature thermal energy storage. Appl. Energy 170, 324–328. doi:10.1016/j.apenergy.2016.02.106
Hall, E. W., Hausz, W., Anand, R., LaMarche, N., Oplinger, J., and Katzer, M. (1979) Conceptual design of thermal energy storage systems for near term electric utility applications. Department of Energy, office of Energy Technology, Division of Storage Systems, 2: 79–84. doi:10.2172/5777283
Hao, J., Chen, Q., Ge, W., Chen, L., Xu, F., and Min, Y. (2019). Influence of thermal characteristics on the synthetic scheduling of an integrated heat and power supply system with thermal storage. Energy Syst. Optim. 39 (29), 5063–5072. doi:10.13334/j.0258-8013.pcsee.181157
Huang, X., Qin, Q., and Yu, Q. (2021). Simulation on structure optimization and heat storage and release characteristics of solid electric heat storage device[J]. J. Mater. Metallurgy 20 (4), 290–296.
Hughes, L. (2010). The technical potential for off-peak electricity to serve as backup in wind-electric thermal storage systems. Int. J. Green Energy 7, 181–193. doi:10.1080/15435071003673690
Igreen (2019). The latest and most complete peak-valley electricity price map in the country: The peak-valley price difference in 14 regions exceeds 0.7 yuan/kwh. Available at: http://www.igreen.org/index.php?m=content&c=index&a=show&catid=18&id=9201 (Accessed Sep 30, 2019).
Jian, Y., Falcoz, Q., Neveu, P., Bai, F., Wang, Y., and Wang, Z. (2015). Design and optimization of solid thermal energy storage modules for solar thermal power plant applications. Appl. Energy 139, 30–42. doi:10.1016/j.apenergy.2014.11.019
Kandasamy, R., Wang, X., and Mujumdar, A. S. (2008). Transient cooling of electronics using phase change material (PCM)-based heat sinks. Appl. Therm. Eng. 28, 1047–1057. doi:10.1016/j.applthermaleng.2007.06.010
Kocak, B., and Paksoy, H. (2020). Performance of laboratory scale packed-bed thermal energy storage using new demolition waste based sensible heat materials for industrial solar applications. Sol. Energy 211 (17), 1335–1346. doi:10.1016/j.solener.2020.10.070
Kuravi, S., Trahan, J., Goswami, D. Y., Rahman, M. M., and Stefanakos, E. K. (2013). Thermal energy storage technologies and systems for concentrating solar power plants. Prog. Energy Combust. Sci. 39 (4), 285–319. doi:10.1016/j.pecs.2013.02.001
Laing, D., Steinmann, W. D., Tamme, R., and Richter, C. (2006). Solid media thermal storage for parabolic trough power plants. Sol. Energy 80 (10), 1283–1289. doi:10.1016/j.solener.2006.06.003
Li, D. C., Hu, Y. K., and He, W. (2017). “Dynamic modelling and simulation of a combined-cycle power plant integration with thermal energy storage,” in 23rd International Conference on Automation and Computing (ICAC), Huddersfield, United Kingdom, 2017, Huddersfield, United Kingdom, 7-8 Sept. 2017 (IEEE).
Ling, H., He, J., Xu, Y., Liang, W., and Haisheng, C. (2020). Status and prospect of thermal energy storage technology for clean heating[J]. Energy Storage Sci. Technol. 9 (3), 861. doi:10.19799/j.cnki.2095-4239.2019.0246
Liu, J., Duan, C., Zheng, D., Peng, L., and Qiushi, W. (2018). Research on phase separation of mesophase pitch and its carbon fiber. New Chem. Mater. 46 (10), 165–169.
Lizarraga⁃Garcia, E., and Mitsos, A. (2014). Effect of heat transfer structures on thermoeconomic performance of solid thermal storage. Energy 68 (3), 896–909. doi:10.1016/j.energy.2014.02.015
Long, H., Fu, X., Kong, W., Chen, H., Zhou, Y., and Yang, F. (2022). Key technologies and applications of rural energy internet in China. Inf. Process. Agric. doi:10.1016/j.inpa.2022.03.001
Miao, X. Y., Kolditz, O., and Nagel, T. (2019). Modelling thermal performance degradation of high and low-temperature solid thermal energy storage due to cracking processes using a phase-field approach. Energy Convers. Manag. 180 (2), 977–989. doi:10.1016/j.enconman.2018.11.042
Morisson, V., Rady, M., Palomo, E., and Arquis, E. (2008). Thermal energy storage systems for electricity production using solar energy direct steam generation technology. Chem. Eng. Process. Process Intensif. 47 (3), 499–507. doi:10.1016/j.cep.2007.01.025
National energy administration (2019). Grid-connected operation of wind power in 2018. Available at: http://www.nea.gov.cn/2019-01/28/c_137780779.htm (accessed January 28, 2019).
National Energy Administration (2021) Win the tough battle of low-carbon transition. Available at: http://www.nea.gov.cn/2021-04/09/c_139869435.htm (Accessed April 9, 2021).
Pasta, M., Wessells, C. D., Huggins, R. A., and Cui, Y. (2012). A high-rate and long cycle life aqueous electrolyte battery for grid-scale energy storage. Nat. Commun. 3, 1149. doi:10.1038/ncomms2139
Qarnia, H. E. (2009). Numerical analysis of a coupled solar collector latent heat storage unit using various phase change materials for heating the water. Energy Convers. Manag. 50, 247–254. doi:10.1016/j.enconman.2008.09.038
Quan, L. V., Chen, T., and Wang, H. (2013). Review and perspective of integrating wind power into CHP power system for peak regulation. Electr. Power 46 (11), 129–136. doi:10.3969/j.issn.1004-9649.2013.11.026
Reboussin, Y., Fourmigué, J. F., Marty, P., and Citti, O. (2005). A numerical approach for the study of glass furnace regenerators. Appl. Therm. Eng. 25, 2299–2320. doi:10.1016/j.applthermaleng.2004.12.012
Regin, A. F., Solanki, S., and Saini, J. (2006). Latent heat thermal energy storage using cylindrical capsule: Numerical and experimental investigations. Renew. Energy 31, 2025–2041. doi:10.1016/j.renene.2005.10.011
Riaz, M., Ahmad, S., and Hussain, I. (2020). Probabilistic optimization techniques in smart power system. Energies 11 (5), 357–361. doi:10.3390/en15030825
Rodríguez, I., Pérez-Segarra, C. D., Oliva, A., and Jaramillo, J. E. (2009). Numerical study of the transient cooling process of water storage tanks under heat losses to the environment. Numer. Heat. Transf. Part A Appl. 55 (12), 1051–1074. doi:10.1080/10407780903014418
Rong, A., Lahdelma, R., and Luh, P. B. (2008). Lagrangian relaxation based algorithm for trigeneration planning with storages. Eur. J. Operational Res. 188 (1), 240–257. doi:10.1016/j.ejor.2007.04.008
Singh, D., Kim, T., Zhao, W., Yu, W., and France, D. M. (2016). Development of graphite foam in- filtrated with MgCl2 for a latent heat based thermal energy storage (LHTES) system. Renew. Energy 94, 660–667. doi:10.1016/j.renene.2016.03.090
Singh, D., Zhao, W., Yu, W., France, D. M., and Kim, T. (2015). Analysis of a graphite foam–NaCl latent heat storage system for supercritical CO2 power cycles for concentrated solar power heat storage system for supercritical CO2 power cycles for concentrated solar power. Sol. Energy 118, 232–242. doi:10.1016/j.solener.2015.05.016
Soprani, S., Marongiu, F., Christensen, L., Alm, O., Petersen, K. D., Ulrich, T., et al. (2019). Design and testing of a horizontal rock bed for high temperature thermal energy storage. Appl. Energy, 251 113345. doi:10.1016/j.apenergy.2019.113345
Sowmy, D. S., and Prado, R. T. (2008). Assessment of energy efficiency in electric storage water heaters. Energy Build. 40, 2128–2132. doi:10.1016/j.enbuild.2008.06.007
State Grid Corporation (2013). Electricity replacement scheme of state grid corporation. China: State Grid Coporation.
Suárez, C., Iranzo, A., Pino, F. J., and Guerra, J. (2015). Transient analysis of the cooling process of molten salt thermal storage tanks due to standby heat loss. Appl. Energy 142, 56–65. doi:10.1016/j.apenergy.2014.12.082
Sun, F., Yan, X., and Gao, M. (2008). Technology and management of combined heat and power. Beijing: China Electric Power Press, 45–49.
Tao, Y. B., and He, Y. L. (2015). Effects of natural convection on latent heat storage performance of salt in a horizontal concentric tube salt in a horizontal concentric tube. Appl. Energy 143, 38–46. doi:10.1016/j.apenergy.2015.01.008
Tao, Y. B., He, Y. L., and Qu, Z. G. (2012). Numerical study on performance of molten salt phase change thermal energy storage system with enhanced tubes. Sol. Energy 86, 1155–1163. doi:10.1016/j.solener.2012.01.004
Thermoflow (2021). Thermoflex - general purpose program - heat balance software. Available at: https://www.thermoflow.com/products_generalpurpose.html (Accessed April 8, 2021).
Tian, H., Wang, W., Ding, J., and Wei, X. (2021). Thermal performance and economic evaluation of NaCl–CaCl2 eutectic salt for high-temperature thermal energy storage. Energy 227, 120412. doi:10.1016/j.energy.2021.120412
Tyagi, V. V., and Buddhi, D. (2007). PCM thermal storage in buildings: A state of art. Renew. Sustain. Energy Rev. 11 (6), 1146–1166. doi:10.1016/j.rser.2005.10.002
Verda, V., and Colella, F. (2011). Primary energy savings through thermal storage in district heating networks. Energy 36 (7), 4278–4286. doi:10.1016/j.energy.2011.04.015
Wang, J., Shi, Y., Zong, Y., Cai, H., Træholt, C., and Dong, Z. Y. (2019). Investigation of real-time flexibility of combined heat and power plants in district heating applications. Appl. Energy 237, 196–209. doi:10.1016/j.apenergy.2019.01.017
Wang, X., Palazoglu, A., and El-Farra, N. H. (2015). Operational optimization and demand response of hybrid renewable energy systems. Appl. Energy 143, 324–335. doi:10.1016/j.apenergy.2015.01.004
Wu, B., Shao, Z., and Hu, X. (2015). Heat supply transformation for four 215 MW units using steam extraction. Therm. Power Gener. 44 (5), 87–90.
Xing, Z., Xiang, S., and Xu, J. (2019). Design and experimental study on energy conversion system for solid electric heat generation and storage[J]. Exp. Technol. Manag. 36 (5), 89–93.
Xu, C., Li, X., Wang, Z., He, Y., and Bai, F. (2013). Effects of solid particle properties on the thermal performance of a packed-bed molten-salt thermocline thermal storage system. Appl. Therm. Eng. 57 (1), 69–80. doi:10.1016/j.applthermaleng.2013.03.052
Xu, X., Jin, X., Jia, H., Yu, X., and Li, K. (2015). Hierarchical management for integrated community energy systems. Appl. Energy 60, 231–243. doi:10.1016/j.apenergy.2015.08.134
Yang, T., Wang, H., Aziz, S., Jiang, H., and Peng, J. (2018a). “A novel method of wind speed prediction by peephole LSTM,” in 2018 International Conference on Power System Technology (POWERCON), Guangzhou, China, 6-8 November 2018, 364–369. doi:10.1109/POWERCON.2018.8601550
Yang, T., Wang, H., and Aziz, S. (2018b). “Long short-term memory network, peephole, Wavelet decomposition, wind speed prediction,” in International conference on power system Technology, Guangzhou, China, 6-8 November 2018.
Yang, Z., Zhang, J., Kintner-Meyer, C. W., Lu, X., Choi, D., Lemmon, J. P., et al. (2011). Electrochemical energy storage for green grid. Chem. Rev. 111 (5), 3577–3613. doi:10.1021/cr100290v
Yaqub, R., Ahmad, S., Ahmad, A., and Amin, M. (2016). Smart energy-consumption management system considering consumers’ spending goals (SEMS-CCSG). Int. Trans. Electr. Energy Syst. 26, 1570–1584. doi:10.1002/etep.2167
Yin, S., Shi, Y., Tong, L., Liu, C., Wang, L., and Ding, Y. (2020). Heating characteristics and economic analysis of a controllable on-demand heating system based on off-peak electricity energy storage. J. Therm. Sci. 29 (2), 343–351. doi:10.1007/s11630-019-1258-6
Zhang, H., and Liu, J. (2019). Analysis on energy saving potential for pumped-storage and two-shift peaking of coal-fired units. IOP Conf. Ser. Earth Environ. Sci. 295, 052021. doi:10.1088/1755-1315/295/5/052021
Zhang, H., Liu, J., Liang, W., Shan, H., and Wang, J. (2022). Design and simulation analysis of high-temperature heat-storage combined-circulation system. Clean. Energy 6 (1), 77–88. doi:10.1093/ce/zkab047
Zhang, H., Liu, J., Liang, W., and Wang, J. (2020). Modeling and energy efficiency analysis of thermal power plant with high temperature thermal energy storage (HTTES). J. Therm. Sci. 29 (4), 1025–1035. doi:10.1007/s11630-020-1310-6
Zhang, L., Yue, L., Zhang, H., Xu, X., Yang, Z., and Xu, W. (2021a). A review of the potential of district heating system in northern China. Appl. Therm. Eng. 188 (16), 116605. doi:10.1016/j.applthermaleng.2021.116605
Zhang, R., Aziz, S., Farooq, M. U., Hasan, K. N., Mohammed, N., Ahmad, S., et al. (2021b). A wind energy supplier bidding strategy using combined EGA-inspired HPSOIFA optimizer and deep learning predictor. Energies 14, 3059. doi:10.3390/en14113059
Zhao, C. Y., and Wu, Z. G. (2011). Thermal property characterization of a low melting-temperature ternary nitrate salt mixture for thermal energy storage systems. Sol. Energy Mat. Sol. Cells 95, 3341–3346. doi:10.1016/j.solmat.2011.07.029
Keywords: smart grid (SG), high temperature, thermal energy storage (TES), economic analysis (EA), thermal power plant (TPP)
Citation: Zhang H, Zhao M, Li Y, Liu J and Liang W (2023) Simulation and economic analysis of the high-temperature heat storage system of thermal power plants oriented to the smart grid. Front. Energy Res. 10:1006972. doi: 10.3389/fenrg.2022.1006972
Received: 29 July 2022; Accepted: 28 September 2022;
Published: 31 January 2023.
Edited by:
Xueqian Fu, China Agricultural University, ChinaReviewed by:
Sadiq Ahmad, COMSATS University Islambad, Wah Campus, PakistanJinliang Zhang, China Chemical Technology Research Institute, China
Copyright © 2023 Zhang, Zhao, Li, Liu and Liang. This is an open-access article distributed under the terms of the Creative Commons Attribution License (CC BY). The use, distribution or reproduction in other forums is permitted, provided the original author(s) and the copyright owner(s) are credited and that the original publication in this journal is cited, in accordance with accepted academic practice. No use, distribution or reproduction is permitted which does not comply with these terms.
*Correspondence: Hongwei Zhang, hongwei19851215@126.com