The Importance of Modeling Carbon Dioxide Transportation and Geologic Storage in Energy System Planning Tools
- 1Geothermal Energy and Geofluids Group, Department of Earth Sciences, ETH Zurich, Zurich, Switzerland
- 2Carbon Solutions LLC, Bloomington, IN, United States
- 3Strategic Energy Analysis Center, National Renewable Energy Laboratory, Golden, CO, United States
- 4Indiana Geological and Water Survey, Indiana University, Bloomington, IN, United States
- 5Department of Earth and Environmental Sciences, University of Minnesota, Minneapolis, MN, United States
Energy system planning tools suggest that the cost and feasibility of climate-stabilizing energy transitions are sensitive to the cost of CO2 capture and storage processes (CCS), but the representation of CO2 transportation and geologic storage in these tools is often simple or non-existent. We develop the capability of producing dynamic-reservoir-simulation-based geologic CO2 storage supply curves with the Sequestration of CO2 Tool (SCO2T) and use it with the ReEDS electric sector planning model to investigate the effects of CO2 transportation and geologic storage representation on energy system planning tool results. We use a locational case study of the Electric Reliability Council of Texas (ERCOT) region. Our results suggest that the cost of geologic CO2 storage may be as low as $3/tCO2 and that site-level assumptions may affect this cost by several dollars per tonne. At the grid level, the cost of geologic CO2 storage has generally smaller effects compared to other assumptions (e.g., natural gas price), but small variations in this cost can change results (e.g., capacity deployment decisions) when policy renders CCS marginally competitive. The cost of CO2 transportation generally affects the location of geologic CO2 storage investment more than the quantity of CO2 captured or the location of electricity generation investment. We conclude with a few recommendations for future energy system researchers when modeling CCS. For example, assuming a cost for geologic CO2 storage (e.g., $5/tCO2) may be less consequential compared to assuming free storage by excluding it from the model.
Acronyms
All acronyms used in this paper are defined in Table 1.
Introduction
Motivation, Literature Review, and Research Gaps
Greenhouse gas (GHG) emissions, principally carbon dioxide (CO2), drive climate change and thus pose substantial risk to human health and economic growth (Intergovernmental Panel on Climate Change, 2018). GHGs are primarily emitted from human activities that burn fossil fuels for energy. For example, the energy system—electricity, transportation, heat—collectively emitted ∼90% of all GHG emissions in the United States in 2018 (Environmental Protection Agency, 2020). As a result, addressing climate change will require transitioning from the current energy system to one that is comprised of technologies that emit substantially fewer GHGs (Intergovernmental Panel on Climate Change, 2014; Rogelj et al., 2018).
Energy system planning tools are often used to gain insight into prospective energy transitions. For example, Integrated Assessment Models (IAMs) are increasingly being used as energy planning tools given their ability to link climate and energy systems together (Intergovernmental Panel on Climate Change, 2014; Rogelj et al., 2018; Vinca et al., 2018). Additionally, electricity sector Capacity Expansion Models (CEMs) are often used to investigate pathways to decarbonizing electricity specifically, because they can provide more targeted guidance on electricity infrastructure investment decisions. For example, CEMs can be used to determine which technologies should be deployed to supply electricity demand at least cost under a grid-wide CO2 emission limit or a CO2 price that increases the cost of technologies that emit CO2 to the atmosphere (Wise et al., 2007; Frew et al., 2016; MacDonald et al., 2016; Mileva et al., 2016; Pleßmann and Blechinger, 2017; Koltsaklis and Dagoumas, 2018; Sepulveda et al., 2018; Dagoumas and Koltsaklis, 2019; Bistline and Blanford, 2020; Jayadev et al., 2020).
Results from these tools generally suggest there is uncertainty on the extent to which any single technology will be deployed throughout an energy transition given the inherent uncertainties about the future. For example, the deployment of one technology may be affected by the availability and cost of another (Fais et al., 2016). Despite this uncertainty and complexity, results from both IAMs and CEMs suggest that the cost and feasibility of decarbonization transitions are sensitive to the cost and availability of CO2 capture and storage (CCS) processes (Krey et al., 2014; Kriegler et al., 2014; Yang et al., 2015; Dessens et al., 2016; Sepulveda et al., 2018; Gambhir et al., 2019; Bistline and Blanford, 2020; Jayadev et al., 2020; Baik et al., 2022). In CCS processes, CO2 that would otherwise be emitted to the atmosphere is instead captured and compressed, possibly transported, and then injected into the subsurface for permanent storage in deep geologic formations that are naturally porous and permeable (Intergovernmental Panel on Climate Change, 2005). Some studies suggest that climate-stabilizing energy transitions will require injecting up to ∼1,200 GtCO2 globally by 2,100 (Rogelj et al., 2018). And in the United States specifically, the Princeton Net Zero America study demonstrates that at a minimum, 0.9 GtCO2/yr of CO2 injection is required to decarbonize by 2050, which is 1.3 times larger than the country’s oil production on a volume equivalent basis (Larson et al., 2020; Jenkins et al., 2021).
While important, robustly representing CCS in energy system planning tools is challenging. For one, estimating the cost and capacity of geologic CO2 storage over the geographical scope of energy systems (e.g., state, continent, globe) is difficult. The subsurface properties that define the geology at any given CO2 storage site influence its capacity and cost but are always uncertain. These properties also vary geospatially, which means the capacity and cost of geologic CO2 storage can vary substantially by location. Moreover, the capacity and cost of geologic CO2 storage can also vary due to site-level factors that can be independent of geology. For example, the diameter of the well casing may constrain the maximum CO2 injection rate, thus the CO2 storage capacity, more than geology (Middleton et al., 2020b). But the cost and capacity implications of these site-level factors are understudied, and even though there are no substantial technical challenges to CCS deployment (Akerboom et al., 2021), there are very few geologic CO2 storage sites in existence for which to base site-level assumptions.
Additionally, representing CCS in energy system planning tools also requires assumptions about CO2 transportation because it is possible to transport captured CO2 long distances, via pipeline for example, before subsurface injection. The geologic CO2 storage formations below any given power plant, if any exist, may or may not be the least-cost location to store the captured CO2 when considering the geospatial variability of CO2 storage capacities and costs, the cost of CO2 transportation, and other costs like electricity transmission infrastructure (Hannon and Esposito, 2015). Further, determining a least-cost CO2 transportation network is non-trivial, even if all the CO2 point sources and potential sinks are identified (Middleton and Bielicki, 2009; Wu et al., 2015; Middleton et al., 2020c). Capturing the systems-level ramifications of CO2 transportation endogenously within an energy system planning tool is even more difficult because the power plants equipped with CO2 capture (i.e., the CO2 point sources) have not been identified, and are instead a possible option that the model may, or may not, deploy.
Due to these challenges, the representation of CCS in energy system planning tools varies (Table 2). For example, all “out of the box” CEMs by default include power plants equipped with CO2 capture as a technology option, but most do not represent CO2 transportation or geologic storage in any way. This exclusion is typically justified by assuming that 1) the cost of CO2 capture drives investment decisions because it accounts for the largest share of CCS-related costs and/or 2) the capacity and cost of geologic CO2 storage is driven by geologic factors that are outside the domain of the model. Regardless of the justification, this exclusion means the majority of CEMs implicitly assume that CO2 transportation and geologic storage is free, and therefore, results from these CEMs misrepresent the cost of deploying and operating CCS.
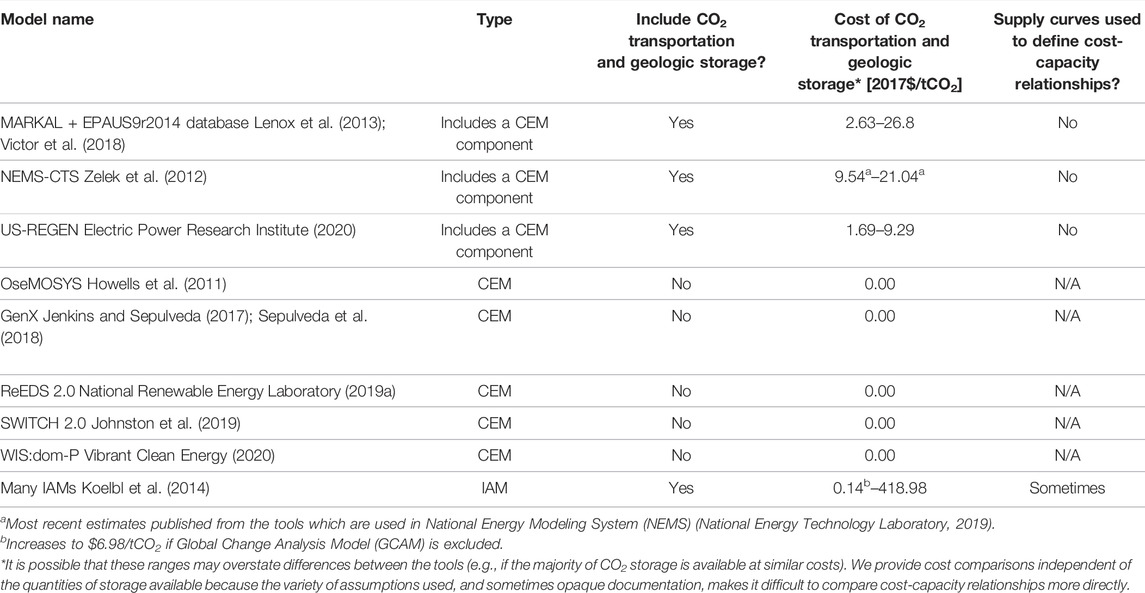
TABLE 2. Representation of CO2 Transportation and Geologic Storage in Integrated Assessment Model (IAM) and Electric Sector Capacity Expansion Model (CEM) Energy Planning Tools. It is possible to modify any CEM to include CO2 transportation and geologic storage representation, which has been done, for example with SWITCH (Sanchez et al., 2015), but this table lists the default representation. Costs were converted to 2017 dollars following the method published in prior work (Koelbl et al., 2014).
The few CEMs that include CO2 transportation and geologic storage assume a wide range of costs. For example, the cost estimates in the MARKet and ALlocation model (MARKAL) range over an order of magnitude, and the cost ranges in National Energy Modeling System—CO2 Capture, Transport, and Storage model (NEMS-CTS) and the US Regional Economy, Greenhouse Gas, and Energy model (US-REGEN) do not overlap (Table 2). These assumed costs are different from one another because different assumptions are made to address the previously discussed complexity and uncertainty around CCS representation. Some of these assumptions include: using cost and capacity relationships that were estimated independent from one another (Lenox et al., 2013; Victor et al., 2018); using cost estimates made for specific locations to represent the cost of CO2 transportation and geologic storage over entire regions (Zelek et al., 2012; National Energy Technology Laboratory, 2019); or assuming a CO2 pipeline of constant length and diameter for a given power plant type to estimate CO2 transportation cost (Electric Power Research Institute, 2020). Further, these are single-cost relationships for a given area (e.g., state, region) even though supply curves are the typical way cost-capacity relationships are defined for a given resource in energy system planning tools. In contrast, some IAMs do use supply curves to represent cost-capacity relationships, but the range of costs is even greater than in CEMs, with some IAMs assuming upper limits above $400/tCO2.
Contributions and Scope of This Paper
In this study, we address these knowledge gaps by 1) presenting a new approach for generating dynamic-simulation-based supply curves for geologic CO2 storage and 2) using these curves to investigate the grid-level ramifications, such as deployment decisions and CO2 emissions, of CO2 transportation and geologic storage assumptions. Our investigation is novel in multiple ways. For one, we present the first dynamic-simulation-based supply curves for geologic CO2 storage and the first investigation of how those supply curves may change based on site-level factors (e.g., number of monitoring wells per injection well). Prior work, for example Vikara et al. (2017), developed supply curves for geologic CO2 storage using volumetric approaches to estimate CO2 storage capacity. Volumetric-based assessments use algebraic equations to estimate the capacity of a potential CO2 storage site (i.e., multiply the pore volume of the rock by the density of CO2 and an assumed “efficiency” coefficient). In contrast, our method is based on an entirely different and novel way of estimating the capacity of CO2 storage that relies on dynamic reservoir simulation and machine learning algorithms. As a result, our supply curves are “dynamic-simulation-based” and do not rely on the assumptions required for volumetric methods in any way. Further, prior work has studied how geology may impact the cost and capacity of CO2 storage (Anderson, 2017; Vikara et al., 2017; Middleton et al., 2020b), but how site-level factors may affect the cost and capacity of geologic CO2 storage has, to our knowledge, not been previously investigated.
Additionally, we are the first to quantify the effect that CO2 transportation and geologic storage assumptions could have on a variety of energy system planning tool results (e.g., CO2 emissions, total system cost). We do this to gain a better understanding of what situations likely require a more robust representation of CCS compared to the current status-quo. There are many different reasons to use an energy system planning tool, and each application has many assumptions beyond those related to CCS that affect the results (e.g., natural gas price). Further, CO2 transportation and geologic storage are just two components of the CCS process, and in turn, CCS is just one of many options that a given tool may, or may not, deploy to supply energy. As a result, it is possible that current assumptions regarding CCS are sufficient for some applications, but it is also possible that there are situations in which more robust representations are warranted. As a result of this purpose and the dearth of studies in this area, we draw conclusions with the intent of guiding future energy system modelers when considering how to represent CCS in their tools.
Methods
As shown in Figure 1, our methodology consists of modifying two previously published tools and performing scenario analysis with each of them: the Sequestration of CO2 Tool (SCO2T) (Middleton et al., 2020a; Middleton et al., 2020b) and the 2019 open-access version of the Regional Energy Deployment System model (ReEDS) (National Renewable Energy Laboratory, 2019a). In this section, we provide a brief description of our modification and application of these two tools and provide more details in the Supplemental Information (SI). We adjust results from both tools to 2017 dollars because that was the dollar used in our prior work with SCO2T (Middleton et al., 2020a).
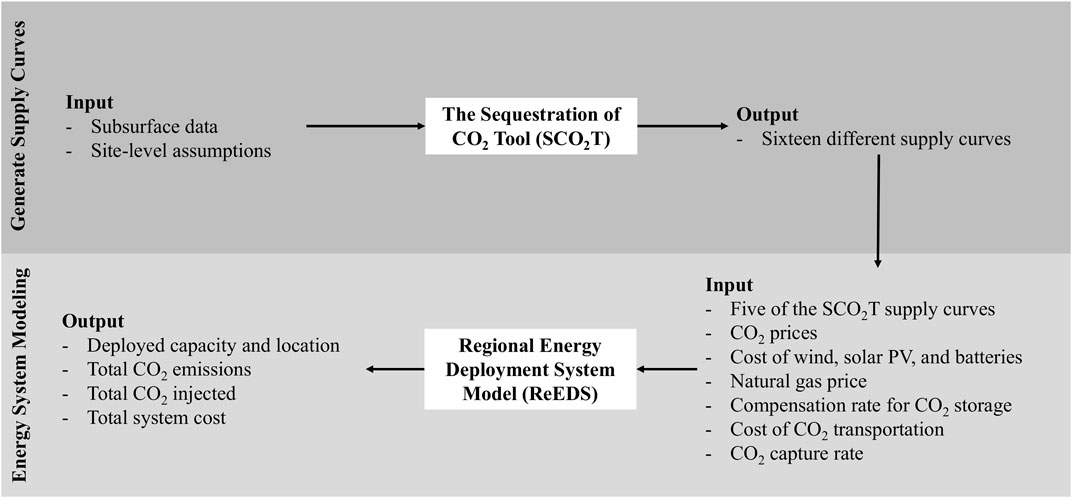
FIGURE 1. Framework for quantifying the effect that CO2 transportation and geologic storage assumptions could have on energy system planning tool results.
SCO2T is an Excel-based tool that estimates the capacity and cost of a geologic CO2 storage site given underlying geologic properties. To do this, SCO2T uses reduced-order models that replicate full-physics dynamic reservoir simulations (Chen et al., 2020). We modify SCO2T by 1) adding all site-level costs from the Environmental Protection Agency (EPA) geologic CO2 storage cost model (Environmental Protection Agency, 2010); 2) adding an Excel MACRO to generate supply curves; and 3) removing areas from the SCO2T subsurface dataset that prior work suggests cannot be developed for geothermal power plants or geologic CO2 storage sites (Young et al., 2019; Hoover et al., 2020). Below we list some assumptions made to apply the EPA cost model for SCO2T for this study. For example, the EPA provides some cost estimates in the units of $/site, which required adding inputs for the maximum site size. Section 1 of the SI contains more information on these modifications.
• In keeping with ReEDS scope that does not include decommissioning cost for power plants, we do not include a post-injection monitoring period or site closure costs in this study.
• We add two ways to constrain the maximum size of a single site within SCO2T: total injection capacity [MtCO2/yr] and number of CO2 injection wells [wells/site]. SCO2T estimates the CO2 injection rate for a single well, and then whichever of these two constraints is limiting determines how many sites are financially accounted for within a given SCO2T run.
• We assume the entire thickness of the formation is drilled for each stratigraphic well and that each core is 9 m long.
• We use a well and pump model from prior work to estimate the power required to inject CO2 across a range of depths and injection mass flowrates (Adams et al., 2015). We then use this data to regress an equation for pumping power, which is used to estimate the capacity, thus cost, of CO2 injection pumps. We assume the downhole pressure is 80% of the lithostatic pressure (this is the maximum downhole pressure allowable within SCO2T to eliminate potential of the formation fracturing from CO2 injection) to be conservative because this assumption will result in the largest pumping power, thus largest pump cost, estimate.
• In a separate calculation, we use the well and pump model to estimate the electricity required to inject CO2 across a few scenarios of more realistic downhole overpressures (e.g., only up to 10 MPa of additional pressure above hydrostatic). None of these scenarios resulted in positive pumping power, thus we do not account for a cost of electricity.
• While a single CO2 plume has the area of a circle, we assume the plume area has the shape of a square when estimating the “Active Monitoring Area” because the total plume size shape at a given site becomes more square-like as more injection wells are drilled (Middleton et al., 2020b).
ReEDS is a widely published CEM of the continental U.S. power system that simulates generation and transmission investment and operating decisions from 2010 to 2050. Out of the different energy system planning tools, we use ReEDS for two primary reasons. First, it has the regional resolution necessary to robustly explore grid-level effects of CCS representation. Other models, such as IAMs, do not easily lend themselves to considering regional and site-specific differences in the cost or capacity of geologic CO2 storage because they often have coarser spatial resolutions (e.g., continents, globe). Second, ReEDS comes “pre-packaged” with arguably the most thorough and respected sets of CEM input data (e.g., wind energy potential, projected future costs of batteries). As a result, using ReEDS enables us to execute many scenarios easily, and grounds our conclusions on a robust range of input data. The modifications that we make to ReEDS include adding constraint equations and additions to the objective function to 1) constrain the amount of CO2 that could be geologically stored; 2) incorporate CO2 transportation; and 3) account for the cost of CO2 transportation and geologic CO2 storage. While it is possible that sequestered CO2 could leak from the wells with time, prior work suggests this possibility has likely negligible impacts on CCS deployment in the energy system (Deng et al., 2017). As a result, we do not account for CO2 leakage in this study. Below we list a few of the key assumptions made to implement CO2 transportation and geologic storage in ReEDS for this study. Section 2 of the SI contains more information on these modifications.
• We use prior work to guide our financing assumptions for geologic CO2 storage sites (National Energy Technology Laboratory, 2017). For example, we use the 5-year depreciation schedule and 6-year construction time schedule options within ReEDS for geologic CO2 storage.
• We conservatively require ReEDS to deploy enough geologic CO2 storage capacity to hold the CO2 that would be captured over a 30-year power plant lifetime at a 100% capacity factor.
• We follow ReEDS convention and linearly interpolate CO2 captured in-between model years to estimate the amount of CO2 captured in gap years (i.e., years in-between ReEDS decision years).
Case Study and Description of Scenarios
We use the Electric Reliability Council of Texas (ERCOT) as a locational case study for several reasons. First, ERCOT is a simpler case study compared to the other options within ReEDS (i.e., Eastern Interconnect, Western Interconnect, or Nationwide), which is appropriate given the purpose of our study and the status-quo of 1) developing regional, dynamic-simulation-based, supply curves for CO2 storage and 2) CCS representation in energy system planning tools. Second, ERCOT manages approximately 90% of the electric load in Texas with a record peak demand of nearly 75 GW (Electricity Reliability Council of Texas, 2021) and is electrically isolated from the Eastern, Western Interconnections, and the Mexican Power Grid with only a small portion of demand being supplied with imports. As a result, ERCOT is a common electricity system case study, with much prior work using it to provide insights into electricity systems broadly (Denholm and Hand, 2011; Sepulveda et al., 2018; Ogland-Hand et al., 2019). Finally, much of the existing geologic CO2 storage infrastructure in the United States is in Texas for enhanced oil recovery.
We execute our study in two parts. First, we run SCO2T across ERCOT (13,601 10 × 10 km grid cells) for sixteen different scenarios of site-level assumptions to generate sixteen separate supply curves (i.e., each supply curve is generated from 13,601 SCO2T runs). These supply curves can be used to understand the effects that site-level assumptions may have on ERCOT-wide costs and capacities of geologic CO2 storage. The supply curve scenarios are further described in Section 3 of the SI. Second, we use five of these supply curves that cover the cost ranges of all sixteen as geologic CO2 storage scenarios in ReEDS within a larger electricity system analysis framework. Overall, we run 2,592 distinct combinations in ReEDS of different cost, price, CO2 capture rate, and policy scenario assumptions (Table 3) because there are many required inputs that affect results, and our goal is to provide future researchers with a better understanding of what situations likely require more robust assumptions around CCS compared to the current simple or non-existent representations.
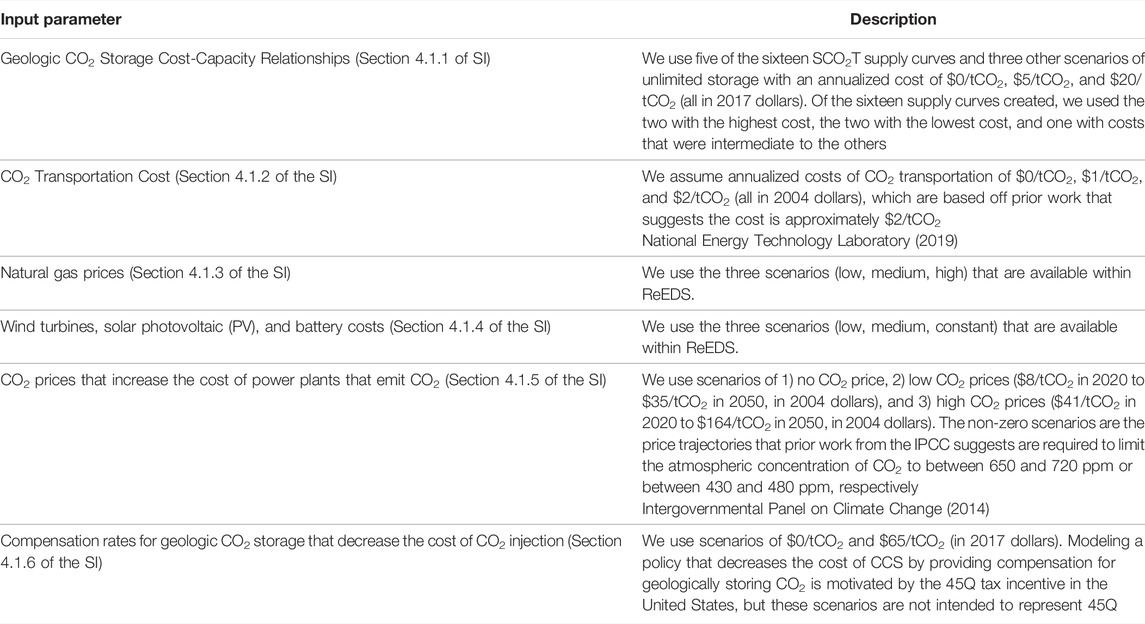
TABLE 3. Description of Parameter Space Used in Electricity System Analysis. We execute ReEDS for every combination of parameters listed when the CO2 capture rate is 90% (default capture rate in ReEDS), but only for a portion of the geologic CO2 storage cost-capacity relationship when the CO2 capture rate is 85% or 95%. The 90% default capture rate is common in many studies because it is a historical benchmark based on economic studies of CO2 capture (International Energy Agency Greenhouse Gas, 2019). Unless specified, we use ReEDS default inputs for the Mid case in the 2019 NREL Standard Scenarios report (National Renewable Energy Laboratory, 2019b).
Results and Discussion
Geologic CO2 Storage Supply Curves Produced With SCO2T
To our knowledge, Figure 2 shows the first supply curve for geologic CO2 across an energy system as large as ERCOT that is based on dynamic reservoir simulation results. First, it illustrates that there is a tremendous capacity available for geologic CO2 storage in ERCOT at low cost. For example, approximately 350 GtCO2 (30% of the possible 1,200 GtCO2 global maximum capacity needed (Rogelj et al., 2018)) of geologic CO2 storage capacity is available in ERCOT at or below $8/tCO2, and approximately 100 GtCO2 of this capacity is available at or below $3/tCO2. These costs are lower than most assumed estimates currently used in energy system planning tools (Table 2), but align with industry estimates and actual geologic CO2 storage projects that suggest that the cost of geologic CO2 storage is likely between $2/tCO2 and $4/tCO2 (Riestenberg et al., 2017; Holubnyak and Dubois, 2018).
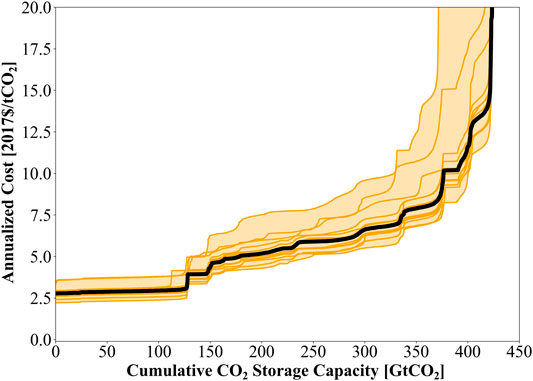
FIGURE 2. Supply Curve Variability Across All Sixteen SCO2T Scenarios. The black curve is the baseline SCO2T scenario, and the orange curves and area cover the range of how costs and capacities may change based on different site level assumptions. See Supplementary Figure S3 in the SI for SCO2T scenario labels.
Figure 2 also demonstrates that the site-level assumptions influencing the cost of geologic CO2 storage can change costs by a few dollars per tCO2, which is not negligible considering that the costs are only around $3/tCO2 for the least expensive 100 GtCO2 of capacity. Further, when compared against our prior work that demonstrates that reservoir depth, porosity, and thickness can change costs by ∼$2/tCO2, ∼$4/tCO2, or ∼$5/tCO2, respectively (Middleton et al., 2020b), the results in Figure 2 suggest that site-level factors may influence costs on a similar order of magnitude as geology. Given this level of sensitivity, and because this is the first study to consider the cost implications of site-level factors over a large area (i.e., ERCOT), we suggest future work continues to investigate how these factors may affect cost. Especially considering that some of these factors (e.g., monitoring costs) are a result of policies that could be changed.
Last, Figure 2 suggests that the baseline SCO2T inputs (black line) provide cost estimates that are intermediate to cost estimates from the more extreme SCO2T input assumption scenarios (orange lines and area). As a result of this and the overall sensitivity of cost to site-level factors, we suggest that future work use baseline SCO2T inputs for CO2 storage supply curves until there are more geologic CO2 storage projects deployed that can be used to guide site-level assumptions.
Figure 3 shows the geospatial distribution of geologic CO2 storage costs across ERCOT using the baseline SCO2T input scenario. There are geologic CO2 storage resources in every area that electricity supply and demand are matched within ReEDS (i.e., ReEDS balancing areas), but the costs of these resources vary. The least-expensive geologic CO2 storage resources are in West ERCOT (balancing areas p60, p61, and p62), while the more expensive resources are in the East (balancing areas p63, p64, p65, and p67). When considered with Figure 2, Figure 3 demonstrates the importance of the higher resolution estimates that SCO2T enables. For example, the NEMS-CTS model uses an estimated cost of geologic CO2 storage of $9/tCO2 (in 2018 dollars) for the region that includes Texas, which was developed assuming basin geology that is characteristic of East Texas (National Energy Technology Laboratory, 2019). Without the higher resolution SCO2T cost estimates, it would be difficult to know that this estimate is arguably not representative of costs in Texas.
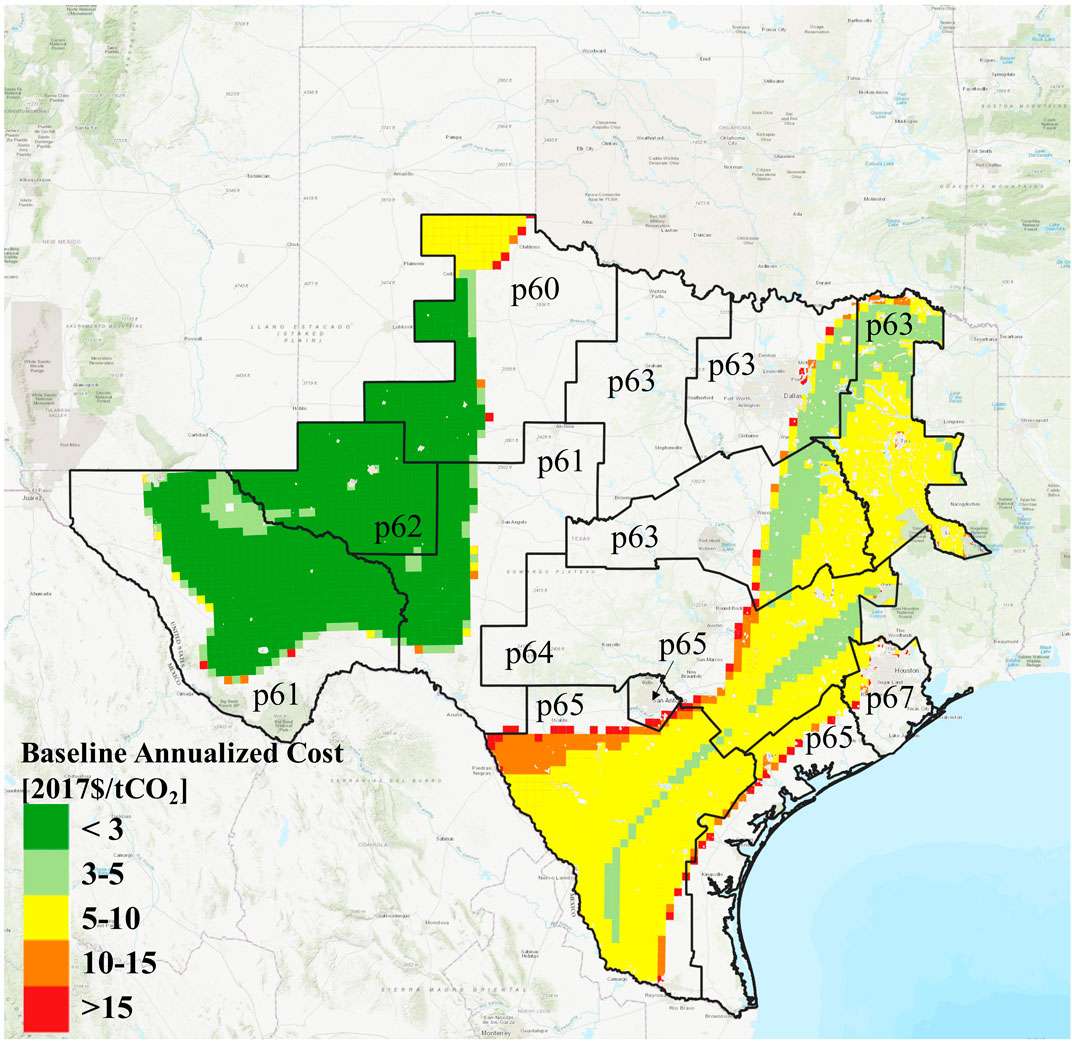
FIGURE 3. Annualized Cost of Geologic CO2 Storage in ERCOT for the Baseline SCO2T Inputs Scenario. The numbered labels (e.g., p61) are the ReEDS balancing areas and the black lines indicate the boundaries of wind and concentrating power resource regions that may be within a given balancing area (National Renewable Energy Laboratory, 2019a).
Grid-Level Effects of CO2 Transport and Geologic Storage Assumptions
Figure 4 shows the total new capacity deployed and total generation of each technology from 2020 to 2050, averaged across all scenarios of wind turbine, solar PV, and battery costs, natural gas prices, and CO2 transportation costs for each combination of CO2 policy and CO2 storage cost-capacity relationship. The 2020–2050 period was used to make differences across scenarios more apparent because the 2010–2019 deployment and generation is prescribed in ReEDS. We first present Figure 4 to facilitate a general discussion on the effects that the CO2 policies and geologic CO2 storage cost scenarios may have on deployment and dispatch decisions, because CEMs are primarily used to investigate such results. As energy system planning tools can also be used for other purposes, we follow by discussing the sensitivity of other grid-level results to assumptions about geologic CO2 storage (Section 3.2.1) and CO2 transportation (Section 3.2.2).
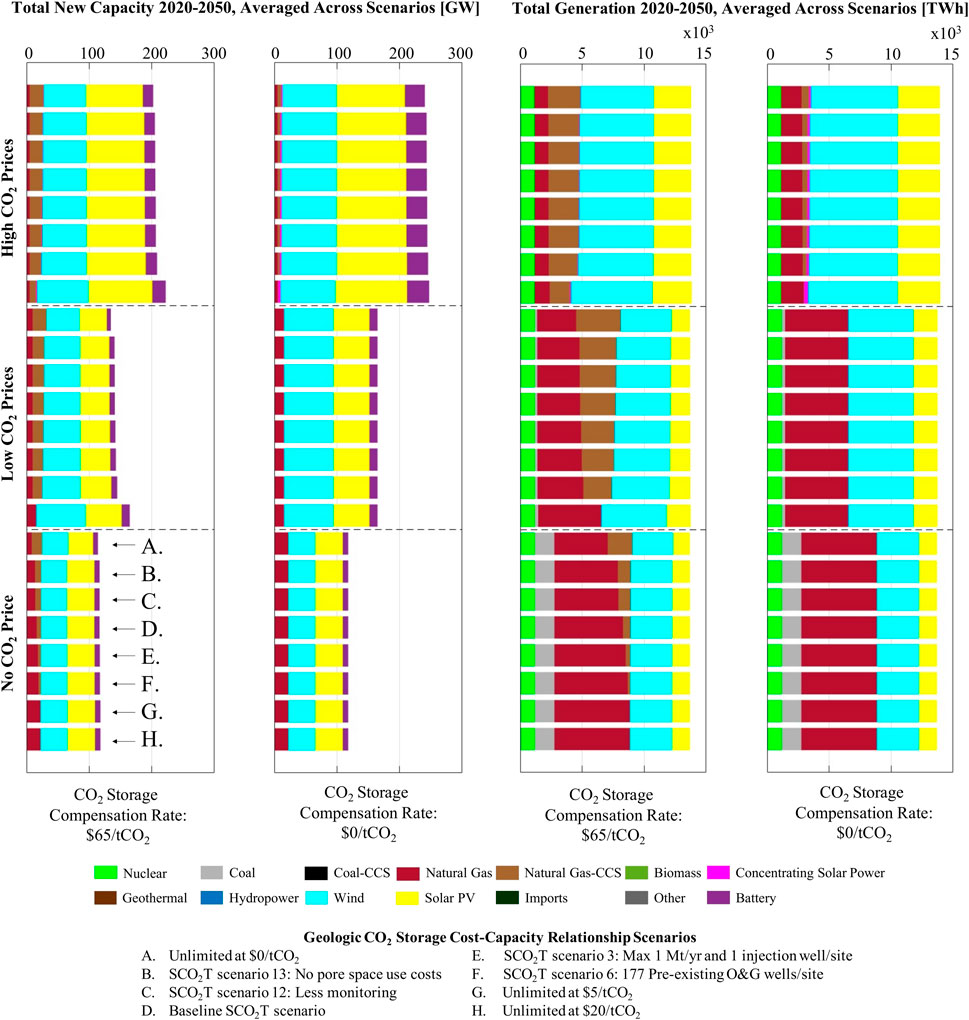
FIGURE 4. Total New Capacity and Generation 2020–2050 Averaged Across Scenarios for Wind Turbine, Solar PV, and Battery Costs, Natural Gas Prices, and CO2 Transportation Costs. These results assume a CO2 capture rate of 90% in all power plants equipped with CO2 capture. In each combination of CO2 policy (e.g., no CO2 price that increases the cost of emitting CO2 and a $0/tCO2 CO2 storage compensation rate), the SCO2T supply curve scenarios follow the same A.–H. order of increasing cost.
Across all combinations of CO2 policy and geologic CO2 storage that we consider, there is more investment in variable renewable energy technologies compared to any other technology. For example, approximately two-thirds or more of all deployment across all CO2 policy combinations is solar PV and wind turbines. No coal power plants with CO2 capture are deployed and the deployment of natural-gas power plants with CO2 capture is highly reliant on CO2 policies. Natural-gas power plants with CO2 capture are generally deployed at comparable capacities, if not less, than new natural-gas power plants without CO2 capture. More general discussion about these general results, including what services power plants with CO2 capture provide, is included in Section 6.2 of the SI.
The results in Figure 4 demonstrate that the assumed cost of geologic CO2 storage has the largest effect on deployment and dispatch decisions in policy scenarios that render CCS marginally competitive compared to other energy technologies. For example, when the CO2 policy renders natural gas power plants with CO2 capture less expensive (e.g., geologic CO2 storage compensation rate of $65/tCO2 and high CO2 prices) or more expensive (e.g., geologic CO2 storage compensation rate of $0/tCO2 and low CO2 prices) relative to other energy technologies, the average investment and average generation become relatively insensitive to the assumed cost of geologic CO2 storage. In contrast, when the CO2 policy renders natural gas power plants with CO2 capture only marginally competitive on cost (e.g., geologic CO2 storage compensation rate of $65/tCO2 and no CO2 price), small increases to the assumed cost of geologic CO2 storage (e.g., <$1/tCO2) can result in large changes to investment or generation because other energy technologies (i.e., natural gas without CO2 capture in this policy combination) become marginally less costly in comparison. This sensitivity of average investment in, and average generation of, natural-gas power plants with CO2 capture to CO2 policy occurs because the CO2 policy combination determines when natural gas power plants with CO2 capture compete closely with other energy technologies.
Sensitivity to Geologic CO2 Storage Cost
Figure 5 shows the variability of total CO2 injected across every combination of CO2 prices, wind, solar PV, and battery costs, natural gas prices, CO2 transportation costs, cost of geologic CO2 storage, and CO2 capture rate that we considered when the compensation rate for geologic CO2 storage is $65/CO2. We focus on this subset of scenarios because there is comparatively minor investment in, and dispatch of, CCS capacity in the other scenarios (Figure 4). The SI includes similar figures for different grid-level outcomes: total investment in natural gas power plants with CO2 capture (Supplementary Figure S6); total investment in wind and solar energy technologies (Supplementary Figure S7); total CO2 emissions (Supplementary Figure S8); total system cost (Supplementary Figure S9); and 2050 average CO2 emission rate (Supplementary Figure S10).
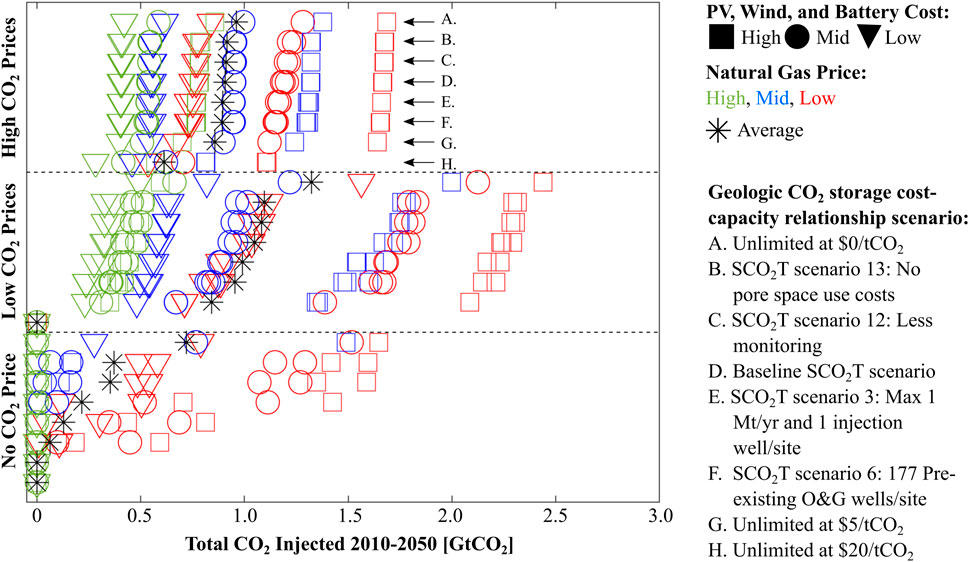
FIGURE 5. Total CO2 Injected Across Model Horizon When CO2 Storage Compensation Rate is $65/tCO2. Within a given geologic CO2 cost-capacity relationship scenario (i.e., row of data), differences between data points that have the same shape and color (e.g., red squares) are due to the different CO2 transportation costs: $0/tCO2, $1/tCO2, and $2/tCO2 (Table 3). These results assume a CO2 capture rate of 90% for any power plant equipped with CO2 capture. See the SI for results across all scenarios (Supplementary Figure S5).
First, Figure 5 suggests that for our scenario assumptions, there are orders of magnitude more capacity for geologic CO2 storage in ERCOT than needed by the electricity system. For example, a maximum of about 2.8 GtCO2 are cumulatively injected by 2050, which is approximately 2.4% of the total geologic CO2 storage capacity available in ERCOT at or below $5/tCO2 (Figure 2).
Second, Figure 5 and the accompanying figures in the SI can be used to qualitatively compare the effects that the ranges of assumed inputs have on grid-level impacts. Overall, these figures suggest that the grid-level results are generally more sensitive to input assumptions (e.g., CO2 policy, the price of natural gas) than to the cost of geologic CO2 storage. For example, when the compensation rate for storing CO2 is $65/tCO2 in the low CO2 prices scenario, the amount of CO2 injected during any given geologic CO2 storage cost scenario varies between approximately 0.5 GtCO2 and 2.5 GtCO2, depending on the price of natural gas and the cost of solar PV, wind, and batteries. Similarly, changes in the assumed power plant CO2 capture rate generally result in smaller changes to other grid-level results compared to the CO2 policy scenario; the price of natural gas; or the cost of solar PV, wind, and batteries—all else constant (Figure 5; Supplementary Figure S4; Supplementary Figure S5; Supplementary Figure S6; Supplementary Figure S7; Supplementary Figure S8; Supplementary Figure S9).
Third, while other assumed inputs (e.g., the price of natural gas) drive any given grid-level result more than the cost of CO2 storage, the cost of geologic CO2 storage was the only input that could eliminate CO2 injection in some policy combinations (e.g., when the geologic CO2 storage compensation rate was $65/tCO2 in the low CO2 prices scenario). This finding is important to highlight because, like the other cost and price scenarios, all geologic CO2 storage cost scenarios used in this study are within the cost ranges that are currently assumed in energy planning tools (Table 2). As a result, it is possible that prior studies underestimated the deployment of power plants with CO2 capture by overestimating the cost of geologic CO2 storage, even in scenarios in which these power plants were more than marginally competitive on cost with other energy technologies.
Table 4 provides statistics that quantify the variability in grid-level results across geologic CO2 storage cost scenarios when the CO2 compensation rate was $65/tCO2 and no CO2 price. We use results from this specific policy combination because it is the one in which the average total investment and average total generation are most sensitive to the assumed cost of geologic CO2 storage (Figure 4).
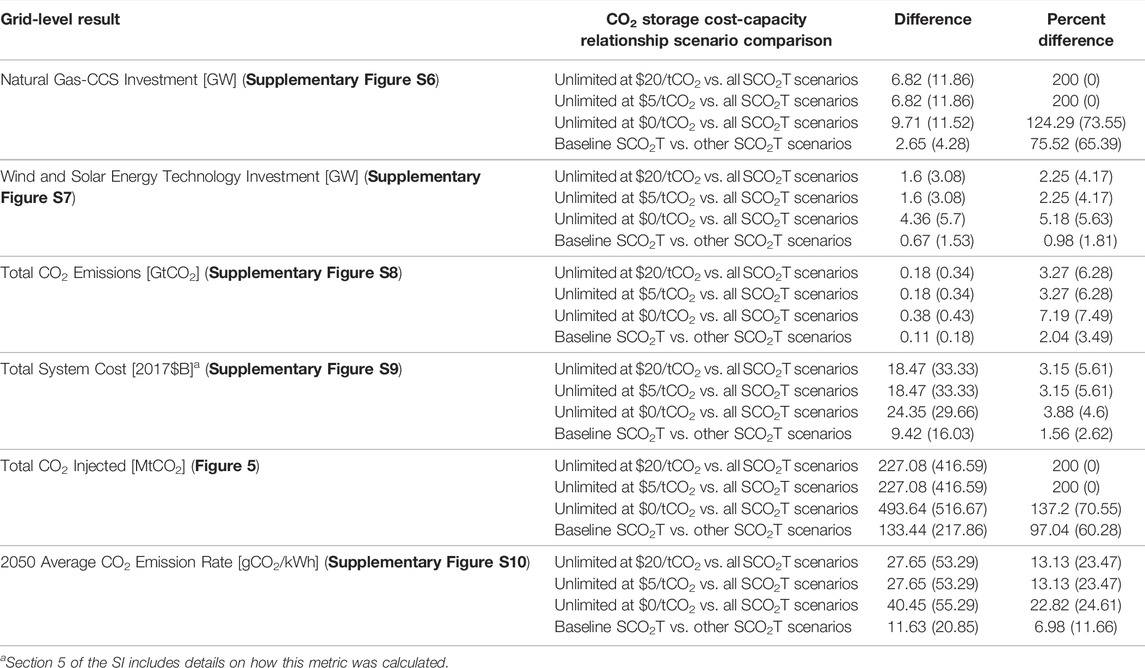
TABLE 4. Distribution of Differences Across CO2 Storage Cost-Capacity Scenarios When CO2 Storage Compensation Rate is $65/tCO2 in the No CO2 Price Scenario: Mean (Standard Deviation in Parentheses). These results are for a CO2 capture rate of 90%. All differences are between the same combination of inputs (e.g., natural gas price). The “all SCO2T scenarios” refers to the five SCO2T scenarios (labeled B, C, D, E, and F in Figure 5) that were used within ReEDS (Table 3). The final comparison (row four of each section) is between the baseline SCO2T scenario (labeled D in Figure 5) and the other four SCO2T scenarios (labeled B, C, E, and F in Figure 5). Please see the referenced Figures in the SI to see the distribution results across all scenarios.
Table 4 shows that in policy scenarios that render CCS marginally competitive, it is still possible that assumptions around the cost of geologic CO2 storage may have a small influence on results, depending on the metrics of interest. For example, outside of the total investment in natural gas power plants with CO2 capture and total amount of CO2 injected, the grid-level result that is most sensitive to the assumed cost of CO2 storage is the 2050 average CO2 emission rate. This occurs because natural-gas power plants without CO2 capture are deployed instead of natural-gas power plants with CO2 capture as the assumed cost of geologic CO2 storage increases (Figure 4). In turn, the grid-level result that is least sensitive to the cost of CO2 storage in this CO2 policy combination is the total investment in wind and solar energy technologies because as the cost of CO2 storage increases, the natural gas power plants with CO2 capture are generally not replaced with investment in wind and solar energy technologies. As a result, depending on the reason why a given energy system planning tool is used, non-robust assumptions around geologic CO2 storage representation may not substantially influence results.
Table 4 also suggests that it may be less consequential to overestimate the cost of geologic CO2 storage compared to assuming it is free: the mean and standard deviations between the $0/tCO2 cost scenario and the five SCO2T supply curve scenarios (third row in each section of Table 4) are generally larger compared to the mean and standard deviations between the $20/tCO2 or $5/tCO2 cost scenarios and the five SCO2T supply curve scenarios (first and second rows in each section of Table 4). This result occurs in this CO2 policy scenario because natural-gas power plants with CO2 capture are deployed when the cost of geologic CO2 storage is $0/tCO2, but there are some SCO2T supply curve scenarios that render natural-gas power plants with CO2 capture non-competitive on cost compared to natural-gas power plants without CO2 capture (Figure 4). As a consequence, when SCO2T supply curve scenarios are used, the grid-level results are more similar to when geologic CO2 storage is assumed to cost $20/tCO2 or $5/tCO2 than $0/tCO2. This finding suggests that if SCO2T supply curves cannot be used, overestimating the cost of geologic storage (i.e., $5/tCO2) may be a more justifiable choice compared to assuming it is free by excluding it from the model.
Lastly, Table 4 also shows the mean and standard deviations between the baseline SCO2T supply curve cost scenario and the four other SCO2T supply curve scenarios (fourth row in each section of Table 4) are smaller than the other comparisons (rows). This relationship occurs because the assumed costs of geologic CO2 storage are closer across this comparison, but these differences are non-zero. As a result, while we suggest future work use the baseline SCO2T inputs because they are intermediate to the supply curves generated with the more extreme input assumptions (Figure 2), future researchers should be wary that these baseline assumptions may still have non-zero grid-level effects, depending on the CO2 policy and the specific grid-level result.
Sensitivity to CO2 Transportation Cost
Figure 6 shows the total investment of natural-gas power plants with CO2 capture and the total CO2 injection, averaged across all scenarios of wind turbine, solar PV, and battery costs, and natural gas prices, in the high CO2 prices scenario and when the CO2 storage compensation rate is $65/tCO2.
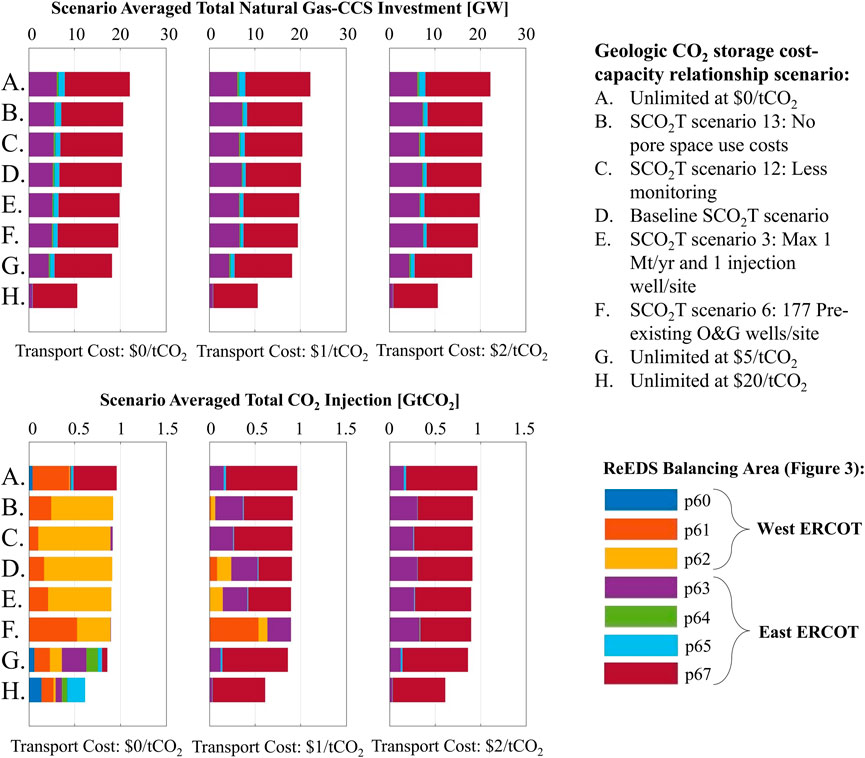
FIGURE 6. Total Investment in Natural Gas Power Plants with CO2 Storage (TOP) and Total CO2 Injection (BOTTOM) Averaged Across Scenarios for Wind Turbine, Solar PV, and Battery Costs, and Natural Gas Prices When the CO2 Storage Compensation Rate is $65/tCO2 and in the High CO2 Prices Scenario. These results assume a CO2 capture rate of 90% for all power plants equipped with CO2 capture. Supplementary Figures S11, S10 in the SI show the results for every CO2 policy combination.
Similar to Figure 5, Figure 6 and the accompanying Figures in the SI suggest the cost of CO2 transportation can affect deployment capacity decisions (e.g., on average, differences of up to about 500 MW of natural-gas power plants with CO2 capture and up to about 0.1 GtCO2 of total CO2 injected), especially when CCS is marginally competitive. But this relationship is less general compared to the changes that occur in the deployment location. As shown in Figure 6 and Supplementary Figures S11, S12, on average, the cost of CO2 transportation has less effect on the location of investment in natural-gas power plants with CO2 capture and more effect on the location of geologic CO2 storage. Power plant capacity is built in East ERCOT primarily because that is where most electricity is demanded. When CO2 transportation is free, the captured CO2 from these power plants is transported from the eastern balancing areas to the least expensive geologic CO2 storage resources in West ERCOT. But on average, less CO2 is transported across ERCOT when the CO2 transportation cost is $1/tCO2, and no transportation occurs when the cost is $2/tCO2, which is possible because there are orders of magnitude more storage resources available than needed across all ERCOT balancing areas (Figure 2; Figure 5). Therefore, our findings primarily suggest that when there is bountiful geologic storage available and CCS is more than marginally competitive on cost, the grid-level result that is most affected by the cost of CO2 transportation is the location of geologic CO2 storage.
Figure 6 also suggests that the site-level factors that influence the cost and capacity of a geologic CO2 storage site can affect the location of geologic CO2 storage. In other words, site-level assumptions can change the cost or capacity of geologic CO2 storage differently, depending on the geologic CO2 storage resource, and these differences may influence the optimal location of CO2 injection. For example, when the price of CO2 is not zero and the CO2 transportation cost is $1/tCO2, more CO2 is injected in balancing areas p61 and p62, and less in p67, when the SCO2T supply curves from the 177 pre-existing oil and gas wells scenario (labeled as F.) are used compared to other SCO2T supply curve scenarios. This difference occurs because the cost of geologic CO2 storage increases more in that SCO2T scenario for the geologic CO2 storage resources located in p67 compared to those in other balancing areas (Supplementary Figure S13). This finding further demonstrates the importance of studying these site-level factors and their impact on geologic CO2 storage costs and optimal injection locations.
Conclusion, Implications, and Future Work
Conclusion
We present the first study to our knowledge that 1) develops supply curves for geologic CO2 storage across an energy system as large as ERCOT that are based on dynamic reservoir simulation; 2) investigates how those supply curves may change based on site-level assumptions; and 3) quantifies the effect that CO2 transportation and geologic storage assumptions may have on a variety of energy system planning tool results. Given the current status-quo of CO2 transportation and geologic storage representation in energy system planning tools, our study is conducted to provide guidance to future energy system modelers by investigating what effects a more robust representation of CCS has on electric sector planning outcomes. For this reason, we interpret our results generally, so our findings apply as broadly to energy systems as possible. We find that:
1. Site-level assumptions (e.g., number of monitoring wells per injection well) may increase or decrease the cost of geologic CO2 storage by up to a few dollars per tonne of CO2 (similar order of magnitude as geologic variations) and can change the cost differently in different locations (Figure 2; Supplementary Figure S13).
2. The assumed cost of geologic CO2 storage has generally small effects at the grid-level compared to other inputs (e.g., natural gas price) (Figure 5), but these effects may be non-negligible when policy renders CCS marginally competitive (Figure 4; Table 4). When power plants with CO2 capture are only marginally competitive on cost, the grid-level results can be sensitive enough to the cost of geologic CO2 storage that site-level assumptions have non-zero effects on the results (Table 4).
3. When power plants with CO2 capture are only marginally competitive on cost, overestimating the cost of geologic CO2 storage (e.g., $5/tCO2) generally produces more similar grid-level results to using SCO2T supply curves compared to assuming sequestration is free (Table 4).
4. Specific to ERCOT, there are orders of magnitude more capacity for geologic CO2 storage available than is needed by the electricity system (Figure 2; Figure 5). In this situation, the cost of CO2 transportation generally affects where geologic CO2 storage investment occurs more than how much generation investment occurs or where that generation investment occurs (Figure 3; Figure 6).
Implications for Future Energy System Modelers
In general, the appropriateness of robustly representing, or not representing, any component of the energy system depends on the reason an energy system planning tool is being used, and our findings suggest CCS representation is no exception. As a result, there are situations in which current assumptions around CO2 transportation and geologic CO2 storage are likely sufficient, and there are other situations where they are insufficient. Based on our conclusions, we provide three recommendations for future researchers considering CCS representation in their modeling efforts:
• Energy system modelers should primarily be concerned about CO2 transportation and geologic storage representations if they are modeling scenarios in which CCS is marginally competitive. Our findings suggest the assumed costs of CO2 transportation and geologic storage are less consequential at the grid level if policies that incentivize decarbonization are not being investigated (e.g., CO2 storage compensation rate of $0/tCO2 and no CO2 price), or if enough policy support exists that CCS is more than marginally competitive on cost (e.g., CO2 storage compensation rate of $65/tCO2 and high CO2 prices).
• Until more geologic CO2 storage sites are deployed that can guide site-level assumptions, future researchers concerned with robustness across uncertainty in site-level factors should consider using supply curves produced with baseline SCO2T inputs because the baseline inputs produce comparatively “average” supply curves that are aligned with cost estimates from actual CO2 storage sites. At the very least, our results suggest that assuming a cost for geologic CO2 storage (e.g., $5/tCO2) may be less consequential than assuming a zero cost by excluding it from the model.
• A more robust characterization of CO2 transportation in energy planning tools may not be necessary in studies primarily concerned with capacity investment decisions across areas with many low-cost geologic CO2 storage resources. This implication is particularly important for energy systems planning tools that model continent-scale, if not global-scale, energy systems (e.g., IAMs).
Study Limitations and Suggestions for Future Work
While our conclusions and recommendations are grounded in a very large parameter space of scenarios, they are dependent on our assumptions. Relaxing or changing these limitations is outside the scope of this study but could be the focus of future work. Key suggestions include:
• Investigate scenarios with a lower assumed cost of CO2 capture. We incorporate future projections for low-cost wind turbines, solar PV, and battery energy storage technologies into our ReEDS parameter space but not for power plants with CO2 capture because these future costs are not available as default ReEDS inputs in the 2019 version. Lowering the cost of CO2 capture would make CCS more competitive on cost, thus, modeling lower CO2 capture costs could decrease the importance of robustly representing geologic CO2 storage, depending on the region and scenarios under investigation.
• Investigate locations with less geologic CO2 storage capacity and locations with less favorable wind and solar energy resources. While our ERCOT case study is well endowed with high-quality wind energy, solar energy, and geologic CO2 storage resources, there are other locations where this is not the case. Under decarbonization policy scenarios, it is likely that the cost of geologic CO2 storage would have less of an effect on grid-level results in locations with poor wind and solar energy resources because there would be few alternatives to investing in CCS processes. Additionally, CO2 transportation costs would likely play a larger role in investment capacity decisions in locations with less geologic CO2 storage potential. If warranted, a more robust representation of CO2 transportation could be achieved by iterating an energy system planning tool with SimCCS (Middleton and Bielicki, 2009; Middleton et al., 2020c), which can be used to determine optimal CO2 pipeline networks.
• Investigate scenarios in which bioenergy power plants with CO2 capture (BECCS) are also available to be deployed. While outside the scope of this study, it is increasingly understood that negative emission technologies like BECCS could play a key role in addressing climate change (Fuss et al., 2018; Minx et al., 2018; National Academies of Sciences, 2019; Fuss and Johnsson, 2021). It is likely that scenarios exist in which this technology is only marginally competitive on cost because its deployment is dependent on strong policy support, like fossil-fuel power plants with CO2 capture. As a result, robustly representing geologic CO2 storage could be important for such future work.
Data Availability Statement
The only new data that this study generated were the creation of supply curves for the ERCOT region. The supply curve data generated for this study can be found in the on GitHub: https://github.com/GEG-ETHZ/ReEDS/tree/main/reeds_and_sco2t. Additionally, the primary General Algebraic Modeling System (GAMS) files that were modified for this study to add CO2 transportation and geologic storage to ReEDS have also been deposited to the same GitHub repository. These GAMS files complement the full description of the ReEDS modifications that are included in the Supplementary Material.
Author Contributions
JO-H: Conceptualization, Methodology, Software, Validation, Formal analysis, Investigation, Data curation, Writing—Original Draft, Visualization. SC: Conceptualization, Methodology, Resources, Writing—Review and Editing, Visualization. RK: Software, Resources, Writing—Review and Editing. KE: Writing—Review and Editing. MS: Writing—Review and Editing, Funding Acquisition. JB: Writing—Review and Editing. RM: Conceptualization, Resources, Writing—Review and Editing, Visualization, Funding Acquisition.
Funding
Martin Saar thanks the Werner Siemens Foundation (Werner Siemens-Stiftung, WSS) for its support of the Geothermal Energy and Geofluids (GEG) group (GEG.ethz.ch) at ETH Zurich. ETH Zurich is also thanked for its support of the GEG group. This material is also based upon work supported by the U.S. Department of Energy, Office of Science, Small Business Innovations Research program under Award DE-SC0021570. The content is solely the responsibility of the authors and does not necessarily represent the official views of the Werner Siemens Foundation, ETH Zurich, or the U.S. Department of Energy.
Conflict of Interest
Authors JO-H, KE, JB, and RM were employed by the company Carbon Solutions LLC.The remaining authors declare that the research was conducted in the absence of any commercial or financial relationships that could be construed as a potential conflict of interest.
Publisher’s Note
All claims expressed in this article are solely those of the authors and do not necessarily represent those of their affiliated organizations, or those of the publisher, the editors, and the reviewers. Any product that may be evaluated in this article, or claim that may be made by its manufacturer, is not guaranteed or endorsed by the publisher.
Acknowledgments
Many thanks to Isamu Naets for all the computer and python support required to get ReEDS up and running at ETH Zurich.
Supplementary Material
The Supplementary Material for this article can be found online at: https://www.frontiersin.org/articles/10.3389/fenrg.2022.855105/full#supplementary-material
References
Adams, B. M., Kuehn, T. H., Bielicki, J. M., Randolph, J. B., and Saar, M. O. (2015). A Comparison of Electric Power Output of CO2 Plume Geothermal (CPG) and Brine Geothermal Systems for Varying Reservoir Conditions. Appl. Energ. 140, 365–377. doi:10.1016/j.apenergy.2014.11.043
Akerboom, S., Waldmann, S., Mukherjee, A., Agaton, C., Sanders, M., and Kramer, G. J. (2021). Different This Time? the Prospects of CCS in the Netherlands in the 2020s. Front. Energ. Res. 9, 1–17. doi:10.3389/fenrg.2021.644796
Anderson, S. T. (2017). Cost Implications of Uncertainty in CO2 Storage Resource Estimates: A Review. Nat. Resour. Res. 26, 137–159. doi:10.1007/s11053-016-9310-7
Baik, E., Siala, K., Hamacher, T., and Benson, S. M. (2022). California's Approach to Decarbonizing the Electricity Sector and the Role of Dispatchable, Low-Carbon Technologies. Int. J. Greenhouse Gas Control. 113, 103527. doi:10.1016/j.ijggc.2021.103527
Bistline, J. E. T., and Blanford, G. J. (2020). Value of Technology in the U.S. Electric Power Sector: Impacts of Full Portfolios and Technological Change on the Costs of Meeting Decarbonization Goals. Energ. Econ. 86, 104694. doi:10.1016/j.eneco.2020.104694
Chen, B., Harp, D. R., Pawar, R. J., Stauffer, P. H., Viswanathan, H. S., and Middleton, R. S. (2020). Frankenstein's ROMster: Avoiding Pitfalls of Reduced-Order Model Development. Int. J. Greenhouse Gas Control. 93, 102892. doi:10.1016/j.ijggc.2019.102892
Dagoumas, A. S., and Koltsaklis, N. E. (2019). Review of Models for Integrating Renewable Energy in the Generation Expansion Planning. Appl. Energ. 242, 1573–1587. doi:10.1016/j.apenergy.2019.03.194
Deng, H., Bielicki, J. M., Oppenheimer, M., Fitts, J. P., and Peters, C. A. (2017). Leakage Risks of Geologic CO2 Storage and the Impacts on the Global Energy System and Climate Change Mitigation. Climatic Change 144, 151–163. doi:10.1007/s10584-017-2035-8
Denholm, P., and Hand, M. (2011). Grid Flexibility and Storage Required to Achieve Very High Penetration of Variable Renewable Electricity. Energy Policy 39, 1817–1830. doi:10.1016/j.enpol.2011.01.019
Dessens, O., Anandarajah, G., and Gambhir, A. (2016). Limiting Global Warming to 2 °C: What Do the Latest Mitigation Studies Tell Us about Costs, Technologies and Other Impacts? Energ. Strategy Rev. 13-14, 67–76. doi:10.1016/j.esr.2016.08.004
Electric Power Research Institute (2020). US-REGEN Model Documentation. [WWW Document]. Available at: https://www.epri.com/research/products/000000003002016601 Accessed: September 29, 2020.
Electricity Reliability Council of Texas (2021). ERCOT Fact Sheet. [WWW Document]. Available at: https://www.ercot.com/files/docs/2021/11/23/ERCOT Fact Sheet.pdf Accessed: February 24, 2022.
Environmental Protection Agency (2010). Geologic CO2 Sequestration Technology and Cost Analysis. Washington, DC: EPA Office of Ground Water and Drinking Water
Environmental Protection Agency (2020). Sources of Greenhouse Gas Emissions. [WWW Document]. Available at: https://www.epa.gov/ghgemissions/sources-greenhouse-gas-emissions Accessed: September 29, 2020.
Fais, B., Keppo, I., Zeyringer, M., Usher, W., and Daly, H. (2016). Impact of Technology Uncertainty on Future Low-Carbon Pathways in the UK. Energ. Strategy Rev. 13-14, 154–168. doi:10.1016/j.esr.2016.09.005
Frew, B. A., Becker, S., Dvorak, M. J., Andresen, G. B., and Jacobson, M. Z. (2016). Flexibility Mechanisms and Pathways to a Highly Renewable US Electricity Future. Energy 101, 65–78. doi:10.1016/j.energy.2016.01.079
Fuss, S., and Johnsson, F. (2021). The BECCS Implementation Gap-A Swedish Case Study. Front. Energ. Res. 8, 1–18. doi:10.3389/fenrg.2020.553400
Fuss, S., Lamb, W. F., Callaghan, M. W., Hilaire, J., Creutzig, F., Amann, T., et al. (2018). Negative Emissions-Part 2: Costs, Potentials and Side Effects. Environ. Res. Lett. 13, 063002. doi:10.1088/1748-9326/aabf9f
Gambhir, A., Rogelj, J., Luderer, G., Few, S., and Napp, T. (2019). Energy System Changes in 1.5 °C, Well below 2 °C and 2 °C Scenarios. Energ. Strategy Rev. 23, 69–80. doi:10.1016/j.esr.2018.12.006
Hannon, M. J., and Esposito, R. A. (2015). Screening Considerations for Caprock Properties in Regards to Commercial-Scale Carbon-Sequestration Operations. Int. J. Greenhouse Gas Control. 32, 213–223. doi:10.1016/j.ijggc.2014.11.016
Holubnyak, Y., and Dubois, M. (2018). Integrated CCS for Kansas (ICKan). [WWW Document]. Available at: https://www.osti.gov/biblio/1491482 Accessed: September 29, 2020.
Hoover, B., Yaw, S., and Middleton, R. (2020). CostMAP: An Open-Source Software Package for Developing Cost Surfaces Using a Multi-Scale Search Kernel. Int. J. Geographical Inf. Sci. 34, 520–538. doi:10.1080/13658816.2019.1675885
Howells, M., Rogner, H., Strachan, N., Heaps, C., Huntington, H., Kypreos, S., et al. (2011). OSeMOSYS: The Open Source Energy Modeling System. Energy Policy 39, 5850–5870. doi:10.1016/j.enpol.2011.06.033
Intergovernmental Panel on Climate Change (2014). Climate Change 2014: Mitigation of Climate Change, Contribution of Working Group III to the Fifth Assessment Report of the Intergovernmental Panel on Climate Change. Cambridge, United Kingdom and New York, NY, USA: Cambridge University Press.
Intergovernmental Panel on Climate Change (2005). IPCC Special Report on Carbon Dioxide Capture and Storage. doi:10.1557/mrs2008.63
Intergovernmental Panel on Climate Change (2018). Summary for Policymakers In: Global Warming of 1.5C. An IPCC Special Report on the Impacts of Global Warming of 1.5C above Pre-industrial Levels and Related Global Greenhouse Gas Emission Pathways, in the Context of Strengthening the Global Response to. Available at: https://www.ipcc.ch/sr15/ Accessed: September 7, 2020. doi:10.1016/j.oneear.2019.10.025
International Energy Agency Greenhouse Gas (2019). Towards Zero Emissions CCS from Power Stations Using Higher Capture Rates or Biomass. [WWW Document]. Available at: http://documents.ieaghg.org/index.php/s/CLIZIvBI6OdMFnf/download Accessed: September 29, 2020.
Jayadev, G., Leibowicz, B. D., and Kutanoglu, E. (2020). U.S. Electricity Infrastructure of the Future: Generation and Transmission Pathways through 2050. Appl. Energ. 260, 114267. doi:10.1016/j.apenergy.2019.114267
Jenkins, J. D., Mayfield, E. N., Larson, E. D., Pacala, S. W., and Greig, C. (2021). Mission Net-Zero America: The Nation-Building Path to a Prosperous, Net-Zero Emissions Economy. Joule 5, 2755–2761. doi:10.1016/j.joule.2021.10.016
Jenkins, J., and Sepulveda, N. (2017). Enhanced Decision Support for a Changing Electricity Landscape: the GenX Configurable Electricity Resource Capacity Expansion Model. MIT Energ. Initiat. Work. Pap., 1–40.
Johnston, J., Henriquez-Auba, R., Maluenda, B., and Fripp, M. (2019). Switch 2.0: A Modern Platform for Planning High-Renewable Power Systems. SoftwareX 10, 100251. doi:10.1016/j.softx.2019.100251
Koelbl, B. S., van den Broek, M. A., Faaij, A. P. C., and van Vuuren, D. P. (2014). Uncertainty in Carbon Capture and Storage (CCS) Deployment Projections: A Cross-Model Comparison Exercise. Climatic Change 123, 461–476. doi:10.1007/s10584-013-1050-7
Koltsaklis, N. E., and Dagoumas, A. S. (2018). State-of-the-art Generation Expansion Planning: A Review. Appl. Energ. 230, 563–589. doi:10.1016/j.apenergy.2018.08.087
Krey, V., Luderer, G., Clarke, L., and Kriegler, E. (2014). Getting from Here to There - Energy Technology Transformation Pathways in the EMF27 Scenarios. Climatic Change 123, 369–382. doi:10.1007/s10584-013-0947-5
Kriegler, E., Weyant, J. P., Blanford, G. J., Krey, V., Clarke, L., Edmonds, J., et al. (2014). The Role of Technology for Achieving Climate Policy Objectives: Overview of the EMF 27 Study on Global Technology and Climate Policy Strategies. Climatic Change 123, 353–367. doi:10.1007/s10584-013-0953-7
Larson, E., Greig, C., Jenkins, J., Mayfield, E., Pascale, A., Zhang, C., et al. (2020). Net-Zero America: Potential Pathways, Infrastructure, and Impacts Interim Report. [WWW Document]. Available at: https://netzeroamerica.princeton.edu/the-report Accessed: September 29, 2020.
Lenox, C., Dodder, R., Gage, C., Kaplan, O., Loughlin, D., and Yelverton, W. (2013). EPA U .S. Nine-Region MARKAL Database Database Documentation. [WWW Document]. Available at: https://nepis.epa.gov/Adobe/PDF/P100I4RX.pdf Accessed: September 29, 2020.
MacDonald, A. E., Clack, C. T. M., Alexander, A., Dunbar, A., Wilczak, J., and Xie, Y. (2016). Future Cost-Competitive Electricity Systems and Their Impact on US CO2 Emissions. Nat. Clim Change 6, 526–531. doi:10.1038/nclimate2921
Middleton, R. S., and Bielicki, J. M. (2009). A Scalable Infrastructure Model for Carbon Capture and Storage: SimCCS. Energy Policy 37, 1052–1060. doi:10.1016/j.enpol.2008.09.049
Middleton, R. S., Chen, B., Harp, D. R., Kammer, R. M., Ogland-Hand, J. D., Bielicki, J. M., et al. (2020a). Great SCOT! Rapid Tool for Carbon Sequestration Science, Engineering, and Economics. Appl. Comput. Geosciences 7, 100035. doi:10.1016/j.acags.2020.100035
Middleton, R. S., Ogland-Hand, J. D., Chen, B., Bielicki, J. M., Ellett, K. M., Harp, D. R., et al. (2020b). Identifying Geologic Characteristics and Operational Decisions to Meet Global Carbon Sequestration Goals. Energy Environ. Sci. 13, 5000–5016. doi:10.1039/d0ee02488k
Middleton, R. S., Yaw, S. P., Hoover, B. A., and Ellett, K. M. (2020c). SimCCS: An Open-Source Tool for Optimizing CO2 Capture, Transport, and Storage Infrastructure. Environ. Model. Softw. 124, 104560. doi:10.1016/j.envsoft.2019.104560
Mileva, A., Johnston, J., Nelson, J. H., and Kammen, D. M. (2016). Power System Balancing for Deep Decarbonization of the Electricity Sector. Appl. Energ. 162, 1001–1009. doi:10.1016/j.apenergy.2015.10.180
Minx, J. C., Lamb, W. F., Callaghan, M. W., Fuss, S., Hilaire, J., Creutzig, F., et al. (2018). Negative Emissions-Part 1: Research Landscape and Synthesis. Environ. Res. Lett. 13, 063001. doi:10.1088/1748-9326/aabf9b
National Academies of Sciences (2019). Negative Emissions Technologies and Reliable Sequestration, Negative Emissions Technologies and Reliable Sequestration. Washington, DC: The National Academies Press. doi:10.17226/25259
National Energy Technology Laboratory (2019). Carbon Dioxide Transport and Storage Costs in NETL Studies 1–31. Washington, DC: United States Department of Energy.
National Energy Technology Laboratory (2017). FE/NETL CO2 Saline Storage Cost Model: User’s Manual. [WWW Document]. Available at: https://edx.netl.doe.gov/dataset/fe-netl-co2-saline-storage-cost-model-2017 Accessed: September 29, 2020.
National Renewable Energy Laboratory, 2019a. Regional Energy Deployment System (ReEDS) Model Documentation: Version 2019. [WWW Document]. Available at: NREL/TP-6A20-67067. Accessed: September 29, 2020.
National Renewable Energy Laboratory, (2019b). 2019 Standard Scenarios Report: A U.S. Electricity Sector Outlook. [WWW Document]. Available at: https://www.nrel.gov/docs/fy20osti/75798.pdf Accessed: September 29, 2020.
Ogland-Hand, J. D., Bielicki, J. M., Wang, Y., Adams, B. M., Buscheck, T. A., and Saar, M. O. (2019). The Value of Bulk Energy Storage for Reducing CO2 Emissions and Water Requirements from Regional Electricity Systems. Energ. Convers. Manage. 181, 674–685. doi:10.1016/j.enconman.2018.12.019
Pleßmann, G., and Blechinger, P. (2017). How to Meet EU GHG Emission Reduction Targets? A Model Based Decarbonization Pathway for Europe's Electricity Supply System until 2050. Energ. Strategy Rev. 15, 19–32. doi:10.1016/j.esr.2016.11.003
Riestenberg, D., Esposito, R., and Gray, K. (2017). Establishing an Early Carbon Dioxide Storage Complex in Kemper County, Mississippi: Project ECO2S. [WWW Document]. Available at: https://www.osti.gov/servlets/purl/1617036 Accessed: September 29, 2020.
Rogelj, J., Shindell, D., Jiang, K., Fifita, S., Forster, P., Ginzburg, V., et al. (2018). Mitigation Pathways Compatible with 1.5°C in the Context of Sustainable Development In: Global Warming of 1.5°C. An IPCC Special Report on the Impacts of Global Warming of 1.5°C above Pre-industrial Levels and Related Global Greenhouse Gas Emission Pathw. [WWW Document]. Available at: https://www.ipcc.ch/site/assets/uploads/sites/2/2019/05/SR15_Chapter2_Low_Res.pdf Accessed: September 29, 2020.
Sanchez, D. L., Nelson, J. H., Johnston, J., Mileva, A., and Kammen, D. M. (2015). Biomass Enables the Transition to a Carbon-Negative Power System across Western North America. Nat. Clim Change 5, 230–234. doi:10.1038/nclimate2488
Sepulveda, N. A., Jenkins, J. D., de Sisternes, F. J., and Lester, R. K. (2018). The Role of Firm Low-Carbon Electricity Resources in Deep Decarbonization of Power Generation. Joule 2, 2403–2420. doi:10.1016/j.joule.2018.08.006
Vibrant Clean Energy (2020). Technical Documentation: Weather-Informed Energy Systems: For Design, Operation and Markets (Planning Version). [WIS:dom -P (v9.0)] [WWW Document]. Available at: https://vibrantcleanenergy.com/wp-content/uploads/2020/08/WISdomP-Model_Description(August2020).pdf Accessed: September 29, 2020.
Victor, N., Nichols, C., and Zelek, C. (2018). The U.S. Power Sector Decarbonization: Investigating Technology Options with MARKAL Nine-Region Model. Energ. Econ. 73, 410–425. doi:10.1016/j.eneco.2018.03.021
Vikara, D., Shih, C. Y., Lin, S., Guinan, A., Grant, T., Morgan, D., et al. (2017). U. S. DOE's Economic Approaches and Resources for Evaluating the Cost of Implementing Carbon Capture, Utilization, and Storage (CCUS). J Sustain. Energy Engng 5, 307–340. doi:10.7569/jsee.2017.629523
Vinca, A., Emmerling, J., and Tavoni, M. (2018). Bearing the Cost of Stored Carbon Leakage. Front. Energ. Res. 6, 1–11. doi:10.3389/fenrg.2018.00040
Wise, M., Dooley, J., Dahowski, R., and Davidson, C. (2007). Modeling the Impacts of Climate Policy on the Deployment of Carbon Dioxide Capture and Geologic Storage across Electric Power Regions in the United States. Int. J. Greenhouse Gas Control. 1, 261–270. doi:10.1016/S1750-5836(07)00017-5
Wu, Q., Lin, Q. G., Wang, X. Z., and Zhai, M. Y. (2015). An Inexact Optimization Model for Planning Regional Carbon Capture, Transportation and Storage Systems under Uncertainty. Int. J. Greenhouse Gas Control. 42, 615–628. doi:10.1016/j.ijggc.2015.09.017
Yang, C., Yeh, S., Zakerinia, S., Ramea, K., and McCollum, D. (2015). Achieving California’s 80% Greenhouse Gas Reduction Target in 2050: Technology, Policy and Scenario Analysis Using CA-TIMES Energy Economic Systems Model. Energy Policy 77, 118–130. doi:10.1016/j.enpol.2014.12.006
Young, K., Levine, A., Cook, J., Heimiller, D., and Ho, J. (2019). GeoVision Analysis Supporting Task Force Report: Barriers an Analysis of Non-technical Barriers to Geothermal Deployment and Potential Improvement Scenarios. [WWW Document]. Available at: https://www.nrel.gov/docs/fy19osti/71641.pdf Accessed: September 29, 2020.doi:10.2172/1524766
Zelek, C., Geisbrecht, R., Grant, T., Goudarzi, L., Callahann, K., and Babuich, M. (2012). “NEMS-CTS: A Model and Framework for Comprehensive Assessment of CCS and Infrastructure,” in 2012 Carbon Management Technology Conference. February 7-9, 2012, Orlando, Florida, USA [WWW Document]. Available at: https://www.onepetro.org/download/conference-paper/CMTC-150377-MS?id=conference-paper%2FCMTC-150377-MS Accessed: September 29, 2020.
Keywords: energy system planning, ReEDS, SCO2T, CCS, supply curve, geologic CO2 storage
Citation: Ogland-Hand JD, Cohen SM, Kammer RM, Ellett KM, Saar MO, Bennett JA and Middleton RS (2022) The Importance of Modeling Carbon Dioxide Transportation and Geologic Storage in Energy System Planning Tools. Front. Energy Res. 10:855105. doi: 10.3389/fenrg.2022.855105
Received: 14 January 2022; Accepted: 25 February 2022;
Published: 08 April 2022.
Edited by:
Greeshma Gadikota, Cornell University, United StatesReviewed by:
Laura Dalton, North Carolina State University, United StatesNikolaos Koltsaklis, Czech Technical University in Prague, Czechia
Hassnain Asgar, Cornell University, United States
Copyright © 2022 Ogland-Hand, Cohen, Kammer, Ellett, Saar, Bennett and Middleton. This is an open-access article distributed under the terms of the Creative Commons Attribution License (CC BY). The use, distribution or reproduction in other forums is permitted, provided the original author(s) and the copyright owner(s) are credited and that the original publication in this journal is cited, in accordance with accepted academic practice. No use, distribution or reproduction is permitted which does not comply with these terms.
*Correspondence: Jonathan D. Ogland-Hand, jonathan.ogland-hand@carbonsolutionsllc.com