Does the green finance reform and innovation experimental zone policy reduce carbon emission intensity? Evidence from Chinese cities
- 1School of Economics, Tianjin University of Commerce, Tianjin, China
- 2School of Management, National Defence University, Beijing, China
The reduction of carbon emission intensity cannot be achieved without policy support, of which green financial policy is a major component. The main aim of this study is to discuss the causality between the green finance reform and innovation (GFRI) experimental zone policy and the intensity of carbon emissions in China. Using data from 265 Chinese cities from 2005 to 2021, this study applies a time-varying difference-in-differences (DID) model to assess the effect of the GFRI experimental zone policy on the intensity of carbon emissions. The heterogeneity of impacts and the parallel-serial mediation effect of this experimental zone policy are discussed further. The following main findings are obtained. (1) The GFRI experimental zone policy dramatically reduces carbon emission intensity, and this effect is more prominent in cities with large sizes of credit and bond finance. (2) After considering the heterogeneity of government environmental concerns and fiscal pressures, this study reveals that the effect of the GFRI experimental zone policy on reducing carbon emission intensity is weaker in cities with high numbers of environmental protection penalties and fiscal pressures. As the number of environmental penalties and fiscal pressures increases, the effect of the GFRI experimental zone policy on reducing carbon emission intensity decreases; that is, the phenomenon of environmental protection paradoxes and policy conflicts has emerged. (3) Increasing financing for green projects and decreasing the energy intensity of the industry are the primary mediating channels for GFRI experimental zone policy to reduce carbon emission intensity, but the mediating channels for boosting green innovation and promoting energy structure adjustment are not prominent. Moreover, the total effect of the GFRI experimental zone policy on reducing carbon emission intensity is 72% direct and more than 20% indirect. This study concludes that in regions with high fiscal pressure, governments should rely more on green finance to decrease carbon emission intensity. Meanwhile, a green finance policy must be more supportive of zero-carbon energy production and reduce direct environmental penalties to decrease carbon emission intensity in the future.
1 Introduction
As carbon emissions are important contributors to global warming, sea level rise, and climate anomalies, interest in them has increased. In response to these challenges, various policy measures have been adopted to decrease carbon emission intensity, such as carbon taxes, carbon emission trading, and green finance. Particularly, green financial policies play a crucial function in reducing carbon emission intensity. Green finance is defined as increasing long-term funding support for green projects through green bonds, green funds, and green credit to realize the goals of sustainable development (Sachs et al., 2019; Yi et al., 2023). In fact, green finance can strengthen the financing restrictions of highly energy-consuming and heavily polluting firms (Qian and Yu, 2023) and facilitate the financing of green firms (Zhang, 2023), which is associated with a reduction in carbon emission intensity. As a key policy tool for promoting low-carbon and sustainable development, green finance has attracted widespread attention (Bai and Lin, 2024). To reduce carbon emission intensity, China has implemented the green finance reform and innovation (GFRI) experimental zone policy, which is an important practice in green finance policy. Given the potentially huge environmental risks posed by carbon emissions, the scientific assessment of the role of the GFRI experimental zone policy in reducing carbon emission intensity can provide not only empirical evidence for assessing whether this experimental zone policy is effective but also ideas and methods for the further improvement of this policy, which has significant theoretical value and practical implications.
To effectively decrease carbon emission intensity, the State Council of China held a government general conference in June 2017 on financing constraints and selected some cities in five provinces (self-administered regions) for the construction of GFRI experimental zones with distinguishing features; these provinces were Xinjiang, Zhejiang, Guizhou, Guangdong, and Jiangxi. In December 2019, Lanzhou in Gansu Province was also selected as a GFRI experimental zone. Although some studies have focused on the impact of the GFRI experimental zone policy on carbon reduction (Ran and Zhang, 2023), the empirical analyses have mainly been based on data obtained at the provincial level. The GFRI experimental zone policy is carried out in cities, which means that using provincial-level data to assess the magnitude of the policy’s effect on carbon emissions may be biased. In addition, the mediating effect of the GFRI experimental zone policy on carbon emissions is mainly estimated using the stepwise estimation method (Ran and Zhang, 2023; Wang et al., 2023), which may lead to variable omission problems. Finally, the degree of environmental protection and the fiscal situation of the government should be considered when studying the role of green finance in reducing the intensity of carbon emissions.
This study attempts to scientifically assess the impact of the GFRI experimental zone policy on reducing carbon emission intensity at the city level in China. The three main purposes of this study are as follows. First, given that the GFRI experimental zone policy was launched in Chinese cities rather than provinces, this study attempts to identify whether the causality between the GFRI experimental zone policy and the decline in carbon emission intensity still holds in Chinese city regions. Second, considering that the degrees of government environmental protection and fiscal pressure may affect the government’s policy support for carbon emission reduction, this study attempts to investigate whether the role of the GFRI experimental zone policy in reducing carbon emission intensity is heterogeneous because of differences in the degrees of environmental protection and the fiscal situation of the government. Third, given that the GFRI experimental zone policy support focuses on increasing green project finance and reducing industrial energy intensity, after introducing the mediating variables of green project finance and industrial energy intensity, this study seeks to explore whether traditional mediating effects, such as enhancing green innovation and promoting energy structure, still exist. If so, how large are each mediating effect?
To investigate whether causality exists between the GFRI experimental zone policy and the decline in carbon emission intensity, given the differences in the launch date of the GFRI experimental zone policy in different cities, this study uses a time-varying difference-in-differences (DID) model for causality identification based on data from 265 Chinese cities from 2005 to 2021. The results demonstrate that the GFRI experimental zone policy dramatically decreases carbon emission intensity. This effect remains robust after accounting for endogeneity issues, excluding expectancy effects, nonrandom selection effects, other relevant policy disturbances, and placebo tests. The GFRI experimental zone policy reduces carbon emission intensity more significantly with a larger credit financing scale and a larger bond financing scale. This confirms that green finance can help reduce financing constraints and costs (Shi et al., 2022; Liu et al., 2023; Zhang, 2023), thus contributing to the reduction of carbon emission intensity.
The degrees of government environmental protection and fiscal pressure are factors that cannot be ignored when analyzing the role of green finance in reducing carbon emission intensity. In this regard, we investigate whether there are any differences in the impact of the GFRI experimental zone project on reducing the intensity of carbon emissions across different degrees of government environmental protection and fiscal pressure. Heterogeneity analysis suggests that the GFRI experimental zone policy has a weaker effect on reducing carbon emission intensity in cities with a higher number of environmental penalties and greater fiscal pressures. In addition, with the increase in the number of environmental penalties and fiscal pressures, the effect of the GFRI experimental zone policy on reducing carbon emission intensity declines. This implies that the government will need to rely more on green financial policy rather than fiscal policy to achieve its goal of reducing carbon emission intensity. More frequent environmental penalties may become a way of generating revenue for some local governments rather than helping to reduce carbon emissions.
To investigate the existence and magnitude of the effects of transmission mechanisms for the GFRI experimental zone policy in decreasing the intensity of carbon emissions under the multimediation scenario, this study constructed a parallel-serial mediating effect model to examine the transmission mechanism. The results of the model reveal that increasing green project finance and reducing the energy intensity of the industry are the main mediating channels for the GFRI experimental zone policy to reduce carbon emission intensity but that facilitating energy structure adjustment and boosting green innovation have been ineffective. In addition, the GFRI experimental zone policy affects the energy intensity of the industry through green project financing, which in turn decreases the intensity of carbon emissions. The direct effect of the policy in reducing the intensity of carbon emissions accounted for 72% of the total effect, while the indirect effect (mediating effect) accounted for more than 20%. Finally, considering that the mediating variables may be correlated with one another because of economic linkages, we further conducted estimations using structural equation modeling and examined the magnitude of each mediating effect. The results still support the above conclusions that increasing financing for green projects and decreasing the intensity of industrial energy consumption are the main mediating channels. This provides insights into how green financial policy can be effectively utilized to help reduce carbon emission intensity.
This study contributes to the literature by highlighting that the effectiveness of the GFRI experimental zone policy in reducing carbon emission intensity is associated with the size of credit financing and bond financing. Previous explorations of the function of green finance have concentrated on the utilization efficiency of resources (Yan et al., 2022; Yao et al., 2023; Yi et al., 2023), green productivity (Lee and Lee, 2022; Wang, 2023; Zhang et al., 2023), green innovation (Irfan et al., 2022; Lin et al., 2022; He et al., 2023; Liu et al., 2023; Liu and Wang, 2023), pollution abatement (Li and Gan, 2021; Zhang et al., 2021; Lan et al., 2023; Wan et al., 2023; Xiong and Dai, 2023), energy structure (Wan et al., 2023; Zhang et al., 2023), and energy consumption per gross domestic product (Shen and Cao, 2020), as well as the link between green finance and carbon emission abatement, using provincial- or country level data scenarios (Bakry et al., 2023; Du, 2023; Ran and Zhang, 2023; Umar and Safi, 2023; Wang et al., 2023). Unlike previous literature that assesses the function of the GFRI experimental zone policy using province-level data, this study evaluates the effect of this experimental zone policy on reducing carbon emission intensity based on city-level data in China, which addresses the problem of bias in the estimation of the policy’s effects. The study also evaluates the effect of the GFRI experimental zone policy on reducing carbon emission intensity across different credit and bond financing scale sizes, which has not yet been explored in the existing literature. The results reveal that this experimental zone policy dramatically reduces carbon emission intensity and that this effect is more prominent in cities with large sizes of credit financing and bond financing, which further confirms the function of green finance in decreasing financing constraints on carbon emission abatement (Liu et al., 2023; Zhang, 2023) and reducing energy consumption per GDP (Shen and Cao, 2020).
Our study also contributes to the literature on how different levels of government environmental protection and fiscal pressure influence the effectiveness of the GFRI experimental zone policy in reducing carbon emission intensity. Previous literature examining the heterogeneity of the carbon-reducing effect of green finance has focused on geographic location, core environmental protection cities, and resource-dependent cities (Wang et al., 2023a; He et al., 2023; Ran and Zhang, 2023). By contrast, the present study examines the heterogeneity of the effect of the GFRI experimental zone policy on reducing carbon emission intensity according to differences in the degrees of government environmental protection and fiscal pressure. Heterogeneity analyses demonstrate that in cities with greater numbers of environmental penalties and fiscal pressures, the role of the policy in reducing carbon emission intensity is weaker. In addition, with the increase in the number of environmental penalties and fiscal pressures, the effect of the GFRI experimental zone policy on reducing carbon emission intensity declines. The possible reason is that the amount of research and development (R&D) subsidies and green subsidies available to enterprises gradually decreases as fiscal pressures increase; furthermore, to alleviate the fiscal pressure, the government may increase enterprise taxes and fees, which will weaken the ability of enterprises to use the GFRI experimental zone policy and will increase their financial constraints. This scenario is not favorable for enterprises’ provision and use of low- and zero-carbon technologies and equipment; as a result, the effect of the GFRI experimental zone policy on reducing carbon emission intensity is weakened, which implies that the phenomenon of environmental protection paradoxes and policy conflict exists.
This study contributes to the literature that focuses on green project finance as an important pathway toward reducing carbon intensity. Previous studies have explored the mediation effect of green finance on carbon emission abatement mainly in terms of single mediating mechanism effects, such as green innovation, industrial upgrading, and energy structure (Wang et al., 2023b; He et al., 2023; Ran and Zhang, 2023), rather than examining the roles of these mediating mechanisms simultaneously. By contrast, aside from selecting the energy structure and green innovation as mediating variables, we choose the energy intensity of the industry and green project financing as mediating variables and construct a parallel-serial mediation model to examine the mediating effect of the GFRI experimental zone policy on reducing carbon emission intensity. Unlike the findings of He et al. (2023) and Wang et al. (2023c), this study reveals that increasing green project financing and reducing industrial energy intensity are the main mediating mechanisms of the GFRI experimental zone policy to reduce carbon emission intensity. However, the mediating effect through the restructuring of energy and the promotion of green innovation is not obvious. This further confirms the finding that the GFRI experimental zone policy is not conducive to green innovation (Shen and Cao, 2020; Shi et al., 2022; Ran and Zhang, 2023). Thus, there is a need to expand the literature on the transmission mechanisms of the carbon abatement effect of green finance.
Previous studies that are linked to ours are those of He et al. (2023) and Ran and Zhang (2023), but our work differs in the following ways. First, unlike Ran and Zhang’s (2023) exploration of the effect of the GFRI experimental zone policy on carbon emissions using province-level data, given that the GFRI experimental zone policy is carried out in cities, we assess the effect of the policy on reducing the intensity of carbon emissions using city-level data. In contrast to He et al. (2023), who studied the effect of the GFRI experimental zone policy on both carbon and pollution abatement, we explore its effect on reducing the intensity of carbon emissions. Second, unlike Ran and Zhang (2023) and He et al. (2023), who examined the heterogeneity of the effect of the GFRI experimental zone policy on carbon reduction based on geographic location, core environmental protection cities, and resource-dependent cities, the current study explores the heterogeneity of the impact of the policy on reducing the intensity of carbon emissions based on the degrees of government environmental protection and fiscal pressure, which provides insights into the implementation of environmental protection and fiscal policies to reduce the intensity of carbon emissions. Third, in contrast to studies examining the single mediating effect of green finance alone, the present study adopts a parallel-serial mediating effect model to investigate the transmission mechanism of the impact of the GFRI experimental zone policy on reducing carbon emission intensity to address the issue of the omission of variables; doing so expands the pathway of the role of green finance in reducing carbon emission intensity.
The remainder of this paper is structured as follows. Section 2 reviews the relevant literature, and Section 3 introduces the policy background and proposes the research hypothesis. Section 4 describes the empirical model, variables, and data, and Section 5 presents the main empirical results and analyses. Section 6 offers further discussion, and Section 7 provides the conclusions and policy implications.
2 Literature review
To address the ecological damage and environmental pollution induced by human production and life, researchers have proposed various policies to decrease carbon and pollution emissions and reinforce sustainable socioeconomic development. As an important part of environmental pollution control policies, the function of green finance policy has drawn increased attention.
2.1 Green finance, green innovation, and resource efficiency
Green innovation is a key initiative to fulfill the goals of pollution and carbon abatement, but this is unachievable if not supported by green finance. Irfan et al. (2022) demonstrated that a green finance innovation reform policy has strongly contributed to green technology innovation and enhanced sustainable performance. Optimizing the structure of industries, increasing investment in R&D, and boosting economic growth are the core mediation mechanisms for green finance innovation and reform policy impacts on green innovation. A large body of micro-empirical evidence also indicates that the function of green finance is important in the process of green innovation (Lin et al., 2022; Liu and Wang, 2023; Ma et al., 2023; Zhang et al., 2023). The contribution of green finance to green innovation occurs not only in terms of the quantity of innovation but also in terms of its quality and performance. Liu et al. (2023) found that the expansion of green finance could enhance firms’ innovative performance. Green finance can decrease enterprises’ financing constraints, enhance their R&D investments, and increase their investments in environmental protection, thus improving their innovative performance. He et al. (2023) further indicated that the positive effect of green finance is amplified by improving the quality of green innovation.
Green finance is closely linked to resource allocation efficiency. Yan et al. (2022) indicated that firms’ inefficient investments and overinvestments are significantly reduced in the GFRI experimental zone. Reducing agency problems and increasing R&D investments are the mediating mechanisms through which the GFRI experimental zone policy improves firms’ investment efficiency. In China and Organization for Economic Co-operation and Development (OECD) countries, green finance would not only improve natural resource utilization efficiency (Yao et al., 2023; Yi et al., 2023) but also enhance factor allocation efficiency and support green process innovation (Liang et al., 2023). This is because green financing improves resource efficiency by facilitating technological progress (Yan et al., 2023). Thus, green financing instruments are a reliable means to achieve green economic growth and resource efficiency (Xu et al., 2023).
In addition to affecting the efficiency of resource usage, green finance significantly affects the productivity of green total factors. Lee and Lee (2022) developed indicators of green financial development at the province level and found that the development of green finance could effectively increase green productivity. As part of the specific practical policy of green finance, China’s GFRI experimental zone policy has significantly increased city green productivity (Zhang et al., 2023). Moreover, Wang (2023) measured green development efficiency by applying a super-efficiency slacks-based measure model for 30 regions in China and showed that green finance effectively enhances the efficiency of green development. Its impact channels are allocating green credit funds, supporting environmental projects, optimizing the structure of the industry, and facilitating technological progress.
2.2 Green finance, pollution reduction, and carbon emission abatement
The reduction of pollution is one of the primary objectives of green finance. With respect to macro-level evidence, Zhang et al. (2021) showed that green credit has improved environmental quality in China. Incentivizing firm innovation, upgrading the structure of industries, and improving firm performance are three transmission mechanisms for the impact of green credit on environmental pollution. In particular, the function of green finance in decreasing the pollution emissions of the industry, improving cities’ environmental quality, and enhancing the ecological environment of regions is obvious (Li and Gan, 2021; Li et al., 2022; Lan et al., 2023; Wan et al., 2023) and has positive spatial externalities (Zhou and Tang, 2022). In addition, green finance can enhance environmental sustainability (Zhang and Chen, 2023) and help build a more livable ecosystem (Hou et al., 2023). The reason for the considerably positive role of green finance regarding pollution and sustainable development is that green financial tools can be used to encourage environment-friendly technological progress, thereby decreasing environmental pollution (Xiong and Dai, 2023). In terms of micro evidence, He et al. (2022) suggested that green finance can decrease the environmental liability of severely polluting firms. Green credit policy, as an integral part of green financial policy, has significantly inhibited the investment behavior of high-energy-consuming firms (Zhang et al., 2021) and strengthened the financing constraints for high-polluting firms (Qian and Yu, 2023). At the same time, green finance has facilitated financing for green enterprises (Zhang, 2023) and enhanced regional environmental performance. However, there are regional differences in the impact of green finance on carbon emissions, and the development of green finance can reduce carbon emissions in East Asia but not in other regions (Sun, 2023).
Carbon emission reduction requires strong support from green finance. Utilizing Chinese province-level data, Ran and Zhang (2023) indicated that the GFRI experimental zone policy has a significant effect on carbon abatement; its mediating mechanism is the upgrading of the industrial structure and the promotion of green innovation. Wang et al. (2023a) demonstrated that there is a negative link between green credit and carbon emissions and that the transmission mechanism is an upgraded industry structure and an optimized energy consumption structure. As a new component of green financing, green property financing is inversely associated with carbon dioxide (CO2) emissions (Wan et al., 2022). In developing, Asian, and OECD countries, green finance can significantly contribute to carbon emission reductions (Bakry et al., 2023; Wang et al., 2023b; Du, 2023; Umar and Safi, 2023).
Energy structure adjustment is an essential pathway for carbon emission reduction and is associated with support for green finance. Wan et al. (2023) used data sourced from 30 Chinese provinces, measured green credit using the ratio of the total interest expenses of six major highly energy-intensive industry sectors to the interest expenses of the industrial sector, and found that green finance dramatically facilitates energy structure adjustment, while environmental regulatory and green technology innovation are channels for the low-carbon transition of the energy structure. In particular, green financing can substantially influence the restructuring of energy usage (Zhang et al., 2023). Green funds also have significant long-term effects on renewable energy development (Zhao et al., 2023). This partly reflects the fact that the function of green finance in reducing carbon emission intensity cannot be ignored.
In summary, existing studies have explored the influence of green finance on innovation, resource usage efficiency, pollution reduction, and carbon emission abatement. This allows us to further explore the impacts of the GFRI experimental zone policy on carbon emission intensity. However, some aspects of existing studies remain unclear, such as the following. First, most studies examining the effect of the GFRI experimental zone policy on carbon emissions use province-level data for their empirical analyses. Nevertheless, the experimental zone policy was launched in Chinese cities, and expanding the experimental area directly from the city to the provincial level may result in estimation bias. Second, despite the stepwise regression applied to examine the mediating effect of green finance on reducing carbon emission intensity, there may be a problem involving omitted variables, which can lead to biased estimations. Third, whether financing constraints affect the effectiveness of green finance in decreasing carbon emission intensity has not been investigated in existing studies. Finally, whether the degree of government environmental protection and fiscal pressure affects the role of green finance in reducing carbon emission intensity remains unclear.
3 GFRI experimental zone policy background and research hypotheses
3.1 GFRI experimental zone policy background
To improve the ecological environment, enhance resource usage efficiency, and decrease carbon emission intensity, in June 2017, the Chinese government held a State Council general conference and decided to select certain areas in Guizhou, Guangdong, Zhejiang, Xinjiang, Jiangxi, and other provinces (autonomous regions) to establish GFRI experimental zones, with their distinctive features (see https://www.gov.cn/premier/2017/06/14/content_5202560.htm). The GFRI experimental zone is tasked with supporting financial organizations in establishing green finance sectors, encouraging green credit issuance, developing markets for trading environmental rights, such as emission permits, and strengthening bond financing support for public green projects.
Subsequently, seven ministries affiliated with the State Council of China, including the Ministry of Finance and the People’s Bank of China, issued a notice on the general framework for the establishment of the GFRI experimental zone policy and performed the tasks outlined in this policy in late June 2017 (see https://www.gov.cn/xinwen/2017/06/27/content_5205752.htm). Specific measures include building a green financial service system, increasing green credit, conducting green equity financing, and issuing green bonds to raise the proportion of green financing. The specific cities selected were Ganzhou in Jiangxi; Guiyang in Guizhou; Hami, Changji, and Karamay in Xinjiang; Guangzhou in Guangdong; and Quzhou and Huzhou in Zhejiang. Subsequently, in November 2019, six Chinese ministries and commissions printed and released a general program on the construction of a GFRI experimental zone at Lanzhou New Area in Gansu, which marked the selection of Lanzhou as a GFRI experimental zone (see http://lanzhou.pbc.gov.cn/lanzhou/2927286/3945585/index.html). By the end of 2021, eight cities and one county were selected as GFRI experimental zones. The selection of cities is representative, with cities in the eastern and midwestern regions being selected as GFRI experimental zones.
3.2 Research hypotheses
Reducing carbon emission intensity relies on certain equipment and technology levels. An enterprise cannot reduce carbon emission intensity and achieve carbon reduction targets without the use of certain carbon reduction technologies and equipment. However, carbon reduction technologies and equipment are costly. To address this problem, the GFRI experimental zone policy can provide financing assistance for enterprises. In fact, the policy also encourages local governments to undertake green bond financing for green infrastructure, low-carbon transport, and energy projects to expand the provision and application of energy-efficient and carbon-reducing technologies and thus achieve carbon reduction targets.
First, enterprises are allowed to apply for green credit from banks to fulfill the goal of reducing carbon emission intensity, such as purchasing carbon emission reduction equipment and improving energy efficiency, thereby enhancing the diffusion and adoption of low-carbon technology (Zhang et al., 2021; Wang, 2023; Xiong and Dai, 2023), accelerating the implementation of carbon emission abatement tasks, and reducing the intensity of carbon emissions. Innovative mortgage financing, carbon emission rights pledges, and mortgage loans are also important in this regard. As a new type of mortgage financing instrument, carbon emission rights mortgage financing can provide green financing to enterprises for carbon neutrality and carbon capture and sequestration, with dual benefits for the environment and economy, thus achieving the goal of decreasing carbon emission intensity.
Second, enterprises are allowed to finance themselves through the issuance of green bonds to help achieve targets for reducing carbon emission intensity. (1) Allowing enterprises that provide carbon-reducing technologies and equipment to obtain green bond financing, such as energy-efficient equipment manufacturers, new energy vehicle and green ship manufacturers, and new energy and clean energy equipment manufacturers, accelerates zero- and low-carbon innovation (Wang et al., 2022; Lee et al., 2023). (2) Allowing enterprises that utilize carbon-reducing technologies and equipment to raise funds through the issuance of green bonds, such as for the energy-conserving renovation of electricity facilities, industrial energy conservation renovation, renewable energy infrastructure establishment and operations, clean energy-efficient operations, green consulting and technical services, and green operation and management, aids in the application of carbon emission abatement technologies and equipment, thus helping to realize the goal of carbon emission intensity reduction. (3) The GFRI experimental zone policy encourages local governments to undertake bond financing for green public projects, such as green infrastructure upgrades, low-carbon and clean transportation projects, and zero- and low-carbon energy programs. In particular, the issuance of special green local government bonds for clean and low-carbon transport and energy projects (Zhang and Guo, 2023) can help reduce carbon emission intensity.
Third, in addition to green bond and green credit financing, the GFRI experimental zone policy allows enterprises to carry out green equity financing, green fund financing, and green trust financing, which significantly expands the channels of green financing for enterprises and provides financing for the provision and application of carbon-reducing technologies and equipment. Green fund financing, in particular, has a lasting effect on renewable energy growth (Zhao et al., 2023) and helps realize the goal of reducing carbon emission intensity. In fact, the provision or application of carbon emission abatement technologies and equipment often faces financing constraints, and green finance can help enterprises address such finance constraints (Qian and Yu, 2023). This means that the stronger the green financing, the more effective the provision and application of carbon-reducing technologies and equipment and the greater the effect of the GFRI experimental zone policy on reducing carbon emission intensity. Correspondingly, Hypothesis 1 is proposed.
Hypothesis 1:. The GFRI experimental zone policy can decrease carbon emission intensity, but this reduction effect varies across financing size conditions.
The energy-saving and carbon-reducing technological innovations carried out by enterprises have the attributes of public goods and rely on certain R&D subsidies or tax incentives for R&D inputs. Thus, increasing fiscal support for R&D activities can effectively enhance green innovation (Bai et al., 2019) and curb carbon emissions (Chen et al., 2023). Nevertheless, as fiscal pressures increase, the impact of fiscal R&D expenditures on green technology innovation will diminish (Wei et al., 2023), which does not enhance energy efficiency (Jiang et al., 2022). Especially when local governments face high fiscal pressures, support for R&D subsidies may be insufficient, or the degree of tax incentives for R&D inputs may be reduced. To alleviate fiscal pressure, the government may also increase the burden of corporate tax and fees. Therefore, the influence of the GFRI experimental zone policy on decreasing carbon emission intensity is limited. In other words, when the government’s fiscal status is favorable, increasing fiscal support for enterprises’ R&D input can amplify the impact of the GFRI experimental zone policy on decreasing the intensity of carbon emissions.
Achieving the goal of reducing carbon emission intensity, in addition to depending on enterprises to provide and apply carbon emission abatement technologies and equipment, depends on the government’s concern for environmental protection. Strict environmental regulations and greater environmental pressure trigger technological innovations in firms, which can dramatically decrease the consumption of nonclean energy and improve the performance of environmental technology (You et al., 2019; Lin et al., 2022; Zhang and Zhao, 2023). Nevertheless, if the government’s goal in increasing its environmental protection efforts is to increase fiscal revenues, or if it directly closes down high-energy-consuming enterprises to achieve environmental protection goals, it may weaken the effect of the GFRI experimental zone policy on reducing carbon emission intensity. In particular, government environmental penalties have resulted in a dramatic decline in the quantity and quality of firms’ green innovations (Wang et al., 2023c), which may undermine the effectiveness of the GFRI experimental zone policy in reducing carbon emission intensity. Correspondingly, Hypothesis 2 is proposed.
Hypothesis 2:. There is heterogeneity in the effectiveness of the GFRI experimental zone policy in reducing carbon emission intensity, given different degrees of environmental protection and fiscal pressure.
The GFRI experimental zone policy, in addition to directly decreasing carbon emission intensity, will have an indirect effect on reducing carbon emission intensity. For enterprises that supply carbon-reducing technology and equipment, the GFRI experimental zone policy provides green financing support for their carbon-reducing technological innovations (Ran and Zhang, 2023; Zhang, 2023), which are technological measures to reduce carbon emission intensity and are the most crucial factors in this regard. At the same time, the GFRI experimental zone policy allows enterprises and the government to obtain green financing for projects, such as zero- and low-carbon transport and energy production (Zhang, 2023), thus facilitating the application and diffusion of low-carbon technological equipment and reducing energy intensity. In addition, the GFRI experimental zone policy allows industrial firms to obtain green financial resources for the adoption of more energy-efficient and carbon-reducing equipment, which helps decrease the energy intensity of the industry and accelerates the achievement of the objective of reducing carbon emission intensity.
Energy structure adjustment is the primary way to reduce carbon emission intensity. Low-carbon energy, especially zero-carbon energy production and clean and efficient energy usage (Sun and Zhang, 2023), contributes to radical reductions in carbon emission intensity. However, the provision and adoption of low- and zero-carbon energy technology and equipment require financial support. The GFRI experimental zone policy not only supports enterprises that manufacture power generation equipment, such as solar energy, wind energy, and nuclear energy, to obtain green financing but also allows them to obtain green financial resources for the construction and operation of ancillary facilities for power generation equipment, such as solar energy, wind energy, and nuclear energy, thus supporting the energy structure transition (Wan et al., 2023; Zhao et al., 2023). The construction and operation of facilities, such as high-efficiency energy storage facilities, smart electricity grids, and multi-energy complementary systems, can be aided by green financing support. At the same time, strengthening the financing constraints on traditional fossil fuel-based energy-consuming manufacturing enterprises and energy-intensive machinery and equipment application enterprises will curb the growth of carbon emissions (Zhang et al., 2021; Qian and Yu, 2023). Thus, the GFRI experimental zone policy can rapidly expand the production and supply of low- and zero-carbon energy and discourage the growth of traditional energy, such as thermal power and fossil fuels, which will significantly contribute to energy structure adjustment and thus reduce carbon emission intensity. Correspondingly, we propose Hypothesis 3:
Hypothesis 3:. Promoting green innovation, decreasing the energy intensity of the industry, increasing green project financing, and enhancing energy structure adjustment are the main channels through which the GFRI experimental zone policy reduces carbon emission intensity. Green project financing also meets the objectives of reducing carbon emission intensity by reducing the energy intensity of the industry.
4 Empirical model, variables, and data
4.1 Empirical model
The premise of the standard DID model for assessing policy effects is that the policy pilots for the treatment groups are implemented at the same point in time and that the policy intervention continues. If the policy pilots are not implemented at the same point in time for the treatment group and if the status of the policy intervention changes, estimation with the standard DID model will result in biased estimated coefficients for policy effectiveness (Callaway and Sant’Anna, 2021; Athey and Imbens, 2022; Roth et al., 2023). Compared to the standard DID model, the time-varying DID can solve the problem of bias in estimating policy effects in situations in which the start time of the pilot policy varies across individuals (regions). Athey and Imbens (2022) argued that utilizing the time-varying DID model yields unbiased estimators of the causal effects in cases of randomly assigned policy date time points. In practice, the GFRI experimental zone policy launched by China serves as a quasi-natural experiment; the start time of this pilot policy varies from one pilot city to another. Thus, the time-varying DID model is a suitable methodology for assessing the effects of the GFRI experimental zone policy. Referring to Athey and Imbens (2022) and Roth et al. (2023), we assess the effect of the GFRI experimental zone policy on reducing carbon emission intensity by using the time-varying DID model, which is constructed as follows:
In Eq. (1),
A valid prerequisite for applying the time-varying DID model to assess the impact of the GFRI experimental zone policy on reducing the intensity of carbon emission is that the parallel trend condition be met. Following the approaches of Baker et al. (2022) and Callaway and Li (2023), the event analysis method is utilized to investigate whether the parallel trend condition is satisfied. We construct the model as follows:
In Eq. (2),
Government environmental protection degrees and fiscal status affect corporate carbon abatement behavior (You et al., 2019; Lin et al., 2022; Wei et al., 2023), which may occur in association with the role of the GFRI experimental zone policy in reducing carbon emission intensity. To further examine whether there is heterogeneity in the impact of the GFRI on reducing carbon emission intensity under different degrees of governmental environmental protection and fiscal pressure, the model is set up as follows:
In Eq. (3),
4.2 Variables
4.2.1 Dependent variable
The dependent variable is
In Eq. (4),
4.2.2 Independent variable
The independent variable is
4.2.3 Control variable
The problem of estimation bias resulting from omitted variables must be controlled for when evaluating the effect of the GFRI experimental zone policy on reducing the intensity of carbon emissions. Referring to the studies of He et al. (2023) and Ran and Zhang (2023), the control variables selected are as follows: environmental regulation degree (ERD), measured by the ratio of environmental pollution control inputs to fiscal budget revenues; the number of taxis (lnNT), calculated by taking the natural logarithm of taxi numbers; levels of financial development (LFD), measured by using the ratio of loan stock to the GDP; the number of enterprises canceled (lnNEC), measured by counting the number of enterprises canceled and taking its natural logarithm; and the total number of patents (lnTNP), calculated by taking the sum of the number of the three types of patents granted and 1 and then taking the natural logarithm.
4.2.4 Instrumental variable
Considering that the city selection for the GFRI experimental zone policy may be related to the importance of a city’s historical industries and the energy consumption of these industries, a potential endogeneity problem exists. To address the issue of estimation bias induced by endogeneity, this study draws on the methodology of Acemoglu et al. (2001) and Cao et al. (2021) and selects instrumental variables (IVs) as follows: the ratio of the industry output to the GDP in 1986 (RIO) and the ratio of industrial electricity consumption to the industrial output in 1986 (RIE).
These IVs were chosen for the following reasons. First, cities with historically high shares of industrial output and industrial energy consumption are more likely to be chosen as GFRI experimental zones, which correlates with this experimental zone policy. Second, these IVs represent historical data that are uncorrelated with the other variables, thus satisfying the assumption of IV exogeneity. Because these variables involve cross-sectional data, they cannot be used directly for estimation. Drawing on the methodology of Liu et al. (2021), this study uses the interaction term between these ratios and the year relative to the launch date of the GFRI experimental zone policy as IVs, namely, RIOO and REII.
4.2.5 Moderating variables
The moderating variables include the degree of government environmental protection and fiscal pressure. The latter was measured using the frequency of the use of environmental protection vocabulary in government work reports (FEPV) and the natural logarithm of the sum of the number of environmental protection penalties and 1 (lnEPP). Fiscal pressure was measured using the fiscal gap (FG), namely, by subtracting the budget revenue from the budget expenditure and then calculating the ratio of the result to the GDP. As a substitute indicator, fiscal pressure is also measured by the tax gap (TG), namely, by subtracting the tax revenue from the budget revenue and then calculating the ratio of the result to the GDP.
4.2.6 Mediating variables
To identify the transmission channels for the impact of the GFRI experimental zone policy on reducing the intensity of carbon emissions and investigate the magnitude of the direct and indirect effects involved, the mediating variables selected in this study are as follows. The number of green innovations (lnNGP) is obtained by taking the sum of the number of green invention patent applications, the number of green utility patent applications, and 1, and then taking its natural logarithm. The energy intensity of the industry (EII) is measured using the ratio of electricity consumption in the secondary sector to the value added in the secondary sector. Green project finance (GPF) is determined by taking the sum of the amount of green bond financing and 1, and then taking its natural logarithm. Energy structure (ES) is calculated as 1 minus the ratio of renewable energy production to the aggregate energy production.
4.3 Data and processing
The data are primarily derived from the Economy Prediction System (EPS) database platform. Carbon dioxide emissions data are obtained from the Open-Data Inventory for Anthropogenic Carbon Dioxide Fossil Fuel Emission Database (https://db.cger.nies.go.jp/dataset/ODIAC/), and green project finance data are taken from the Wind Financial Terminal Database. The data for IVs are gathered from the China Economic and Social Big Data Research Platform, which is affiliated with China National Knowledge Internet. Data on the number of the three types of patents granted, green patent applications, and green invention patents are taken from the State Intellectual Property Office, while data on environmental penalties are obtained from the Mark Database (its original data come from the Laws and Regulations Database–Chinalawinfo). Some of the tax data before 2020 are taken from the CEIC database. The data for the GFRI experimental zone and the carbon emission trading pilot information come from the official websites of the National Development and Reform Commission of China and the State Council of China. The total number of enterprises canceled is obtained from the National Enterprise Credit Information Publicity System of China. Environmental protection tax policy information comes from the Institute for Global Decarbonization Progress (http://www.igdp.cn/).
Carbon dioxide is calculated as follows. As CO2 data are global picture raster data, we crop it according to the map of China, referring to the methodology of Chen et al. (2022). The monthly data are summed up to the annual data, and then the corresponding raster data for each city are summed up. Finally, the annual CO2 emission data for each city are obtained.
The energy structure (ES) is measured as follows. (1) Crude oil, fuel oil, coke, raw coal, paraffin, natural gas, gasoline, total electricity generation, and diesel are translated into standard coal and summarized in accordance with the methodology of translating all kinds of energy into standard coal in the China Energy Statistics Yearbook 2013. (2) Thermal electricity generation is subtracted from the total electricity generation to come up with clean energy electricity and convert this to standard coal. (3) One minus the ratio of clean energy standard coal to the total standard coal is used to obtain the ratio of nonclean energy, namely, the energy structure.
Green project financing (GPF) is measured as follows. Based on the bond information data, if the usage of funds collected in each bond has certain characteristics (energy saving, environmental protection, cleanliness, ecology, greenness, energy efficiency, sustainability, pollution, conservation, resource utilization, wind energy, solar energy, environment, carbon emissions, carbon capture, carbon sequestration, wastewater, environmental quality monitoring, and pollution source checking), then a dummy variable for green project financing is generated and assigned the value of 1, while the others are given a value of 0. In addition, if each bond’s scope of business items has the characteristics mentioned above, the dummy variable for green project financing is assigned a value of 1. The amount of bond financing is then totaled for each city for the corresponding year if the dummy value of green project financing is equal to 1, and we delete data duplicates based on city and year to obtain the corresponding data.
For data sourced from multiple databases, we merge the data by city name and year. In the case of provincial data, the data are merged according to the province and year corresponding to the city. Energy structure and environmental regulation degrees are provincial-level data. Because the data have missing values, the following approach is taken to interpolate the missing data, drawing on the methods of Yang and Hao (2023). (1) For the missing values on variables, including the number of environmental protection penalties, the frequency of the use of environmental protection vocabulary in government work reports, the number of green innovations, and green project financing, using 0 for interpolation. (2) For tax data obtained from the EPS database, which has missing values, these missing values are interpolated using tax data obtained from the CEIC database. (3) For missing values for tax and taxi data, the ratio of these variables to the GDP is calculated, and the mean of this ratio is taken by city; finally, the multiplication of the mean of this ratio with the GDP is used to interpolate the missing values. (4) For missing data on the ratio of industrial production to the GDP, as well as the ratio of industrial electricity consumption to the industrial output in 1986, we interpolate the missing values with 0. (5) If there are still missing data for other variables, the missing values are interpolated using a three-period moving average, and if there are data less than 0, the missing values are interpolated using nonzero values from the previous or subsequent period. (6) We delete samples in which the number of missing data for the city is greater than or equal to 1 year. Table 1 displays the statistical characteristics of the variables.
5 Empirical test and results analysis
This section begins with a parallel trends test and a heterogeneity treatment effects test to determine whether the time-varying DID model is reasonable. We then investigate the benchmark estimation results, the results under different financing sizes, issues of endogeneity, and the test of robustness.
5.1 Parallel trends test
Satisfying parallel trends is a precondition for the correct application of the time-varying DID model to estimate the impact of the GFRI experimental zone policy on reducing the intensity of carbon emissions. Drawing on the studies of Baker et al. (2022) and Yang and Hao (2023), the present study uses event analysis to test whether the parallel trends condition is fulfilled. Figures 1, 2 present the event estimation results without considering and considering the impacts of the other variables, respectively.
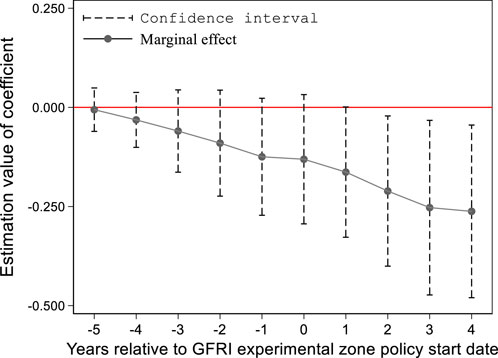
FIGURE 1. Dynamic effect results without considering the effects of other variables. Notes: The lengths of the dotted lines above and below the points indicate 95% confidence intervals.
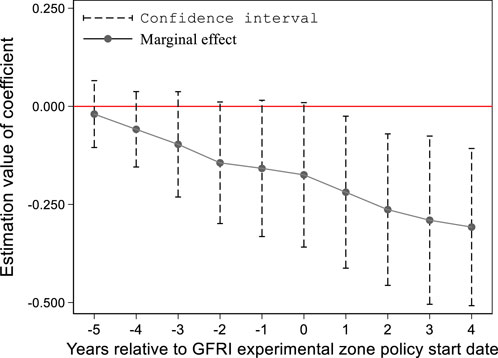
FIGURE 2. Dynamic effect results with consideration of the effects of other variables. Notes: The lengths of the dotted lines above and below the points indicate 95% confidence intervals.
Figures 1, 2 reveal that the coefficients of the GFRI were not significant before the GFRI experimental zone policy started, regardless of whether the effects of other variables are controlled for, implying that the assumption of parallel trends between GFRI and non-GFRI experimental zones is satisfied. The estimated coefficients of the GFRI are all dramatically different from zero after the GFRI experimental zone policy is initiated, implying that the GFRI experimental zone policy significantly reduces carbon emission intensity, with a cumulative impact of more than 0.25.
5.2 Heterogenous treatment effect
As of the sample deadline year, there were multiple pilot time points because of the two rounds of the GFRI experimental zone policy. De Chaisemartin and D’Haultfœuille (2020) argued that the negative weight weighting of coefficients could lead to incorrect estimation results in cases with different groups and periods, which would create the problem of heterogeneous treatment effects. For time-varying DID models (multiple group and period DID models), Goodman-Bacon (2021) decomposed the entire estimator into a weighted mean of all possible 2 × 2 estimators and computed the weights of the treatment variance. If the negative weights of the coefficients of the time-varying groups are large, this implies that there may be a problem with heterogeneous treatment effects. Referring to the methodology of Goodman-Bacon (2021), we use a Bacon decomposition to examine whether heterogeneous treatment effects exist for the effect of the GFRI experimental zone policy on reducing carbon emission intensity. Table 2 shows the Bacon decomposition results.
The decomposition results in Table 2 demonstrate that the estimation coefficients of the time-varying groups are less than 0.01, and their weights are less than 1%, regardless of whether the effects of other variables are controlled for; this implies that the heterogeneity of the treatment effect can be disregarded. Therefore, this study enables the use of a time-varying DID model to assess the impact of the GFRI experimental zone policy on reducing the intensity of carbon emissions.
5.3 Benchmark estimation
One of the objectives of this study is to explore the causality between the GFRI experimental zone policy and the reduction of the intensity of carbon emissions. For this purpose, a time-varying DID model is used for estimation. In addition, we divide the entire sample into midwestern subsamples and eastern subsamples and estimate them independently to examine whether there is a geographical difference in the carbon abatement effects of the GFRI experimental zone policy. In the eastern area, there are 11 provinces and municipalities (Shanghai, Shandong, Zhejiang, Beijing, Jiangsu, Guangdong, Liaoning, Tianjin, Hainan, Fujian, and Hebei), and the samples of their counterpart cities are the subsamples of the eastern region. GFRI experimental zone cities in eastern region include Huzhou, Quzhou, and Guangzhou. GFRI experimental zone cities in mid-western region include Hami, Kelamayi, Ganzhou, Guiyang, and Lanzhou. Table 3 reports the results of the benchmark regression.
Column (1) in Table 3 indicates that the coefficient of GFRI × Post is −0.205, which passes the significance test at the 1% level. This suggests that the GFRI experimental zone policy significantly reduces carbon emission intensity and that the causality between them is supported. In addition, the coefficients of GFRI × Post are significantly less than 0 for both the midwestern and eastern regions, which implies that the role of the GFRI experimental zone policy in reducing carbon emission intensity holds in all regions. Thus, Hypothesis 1 is confirmed.
The GFRI experimental zone policy can reduce carbon emission intensity significantly because this policy decreases the financial constraints on accessing carbon abatement technologies and equipment in multiple ways. First, the GFRI experimental zone policy gives financing support not only for enterprises that provide energy-saving and carbon-reducing technologies and equipment but also for enterprises that use them, thus decreasing the financing constraints confronted by firms attempting to fulfill the goal of carbon emission abatement (Qian and Yu, 2023; Zhao et al., 2023) and accelerating the dissemination of zero- and low-carbon technologies. Second, the GFRI experimental zone policy not only supports access to green financing for enterprises that manufacture power generation equipment, such as wind, solar, hydro, and nuclear energy equipment, but also allows enterprises that construct and operate ancillary facilities for wind, solar, hydro, and nuclear power generation equipment to access green financial resources, thus supporting the energy structure transition (Wan et al., 2023; Zhao et al., 2023). Third, the GFRI experimental zone policy increases financing constraints on traditional fossil fuel-based manufacturing enterprises, energy-intensive machinery, and equipment application enterprises to curb the growth of carbon and pollution emissions (Zhang et al., 2021; Qian and Yu, 2023). Thus, the GFRI experimental zone policy can significantly reduce carbon emission intensity.
5.4 Scale of financing and GFRI experimental zone policy effects
Reducing carbon emission intensity cannot be achieved without certain clean technologies and carbon reduction equipment, all of which require financial support. Especially when enterprises are constrained by financial resources, financial support for pollution reduction and carbon reduction can alleviate this financing constraint (Wang, 2023; Zhang, 2023), thereby promoting the use of cleaner production technologies and carbon-reducing equipment and improving energy efficiency. Simultaneously, expanding the scale of green finance can increase the efficiency of resource usage and enhance technological advancement (Yi et al., 2023). If there is a difference in the impact of the GFRI experimental zone policy on decreasing carbon emission intensity under different financing scale conditions, it can further prove the causal relationship between the two. For this purpose, we measure the size of financing by the size of credit financing and the size of new bond financing. The loan size data are sourced from the EPS database platform. Bond financing size data are obtained from the Wind Financial Terminal Database.
To further strengthen the evidence for a causal relationship between the GFRI experimental zone policy and the reduction of carbon emission intensity, we use the following strategy. First, we add 1 to the loan balance and the amount of new bond financing, take their natural logarithms, and calculate the average for each city over the sample period. Second, we sort the samples according to the size of the mean of the loan balances and divide the entire samples equally into three groups, retaining the second and third groups’ samples (group 1 lacks the samples of the treatment group) and labeling them the small loan sample group and large loan sample group, respectively. Third, we sort the means of new bond financing from smallest to largest and divide the sample equally into four groups, keeping samples from the third and the fourth groups (group 1 and group 2 lack samples of the treatment group) and labeling them as the small bond financing sample group and large bond financing sample group, respectively. Finally, the time-varying DID model is applied for group estimation. Table 4 reports the results.
Columns (1) and (2) in Table 4 indicate that the coefficients of GFRI × Post are insignificant in the sample of cities with small loan sizes, while the coefficient of GFRI × Post is significant for −0.159 in the sample of cities with large loan sizes, implying that the effect of the GFRI experimental zone policy on reducing carbon emission intensity is more likely to be fulfilled in the scenario with large loan financing. Columns (3) and (4) reveal that the coefficient of GFRI × Post fails to pass the significance test in the sample of cities with small bond financing sizes, while the coefficient of GFRI × Post is significant for −0.109 in the sample of cities with large bond financing sizes, implying that in the case of large bond financing sizes, the GFRI experimental zone policy reduces carbon emission intensity more remarkably. This further confirms that there is a financing constraint on carbon emissions (Liu et al., 2023; Zhang, 2023).
5.5 Exploration of endogeneity issues
Considering that the initial conditions of the cities in the GFRI experimental zone and the cities that are not in such zones are not identical, this may lead to measurements of treatment effects that suffer from endogeneity issues. The choice of cities for the GFRI experimental zone may consider their historical industrial development and industrial energy consumption, which generates a problem regarding the omission of potential variables. All of the above scenarios can lead to endogeneity problems.
This study addresses endogeneity issues by using IV estimation. Following the methodology of Acemoglu et al. (2001) and Liu et al. (2021), we select the ratio of industrial output to the GDP and the ratio of industrial electricity consumption to the industrial output in 1986 as instrumental variables (IVs) for the GFRI. Because these variables are cross-sectional, which cannot be used straightforwardly in panel data estimation, we need to generate the interaction terms between these variables and the length of time relative to the start date of the GFRI experimental zone policy as IVs to be applied in the estimation of the model. After the estimation of the DID with the IV model (IV-DID), following the methodology of Kleibergen and Paap (2006), the LM statistic is applied to perform the under-identification test. Referring to the method of Cragg and Donald (1993), we test for weak identification issues by using the Cragg–Donald Wald F statistic. Drawing on Hansen’s (1982) approach, the Hansen J statistic is used to perform the overidentification test. Table 5 presents the IV-DID model estimation results.
After the estimation of the IVs, columns (1)–(4) of Table 5 reveal that the p-value corresponding to the Kleibergen–Paap rk LM statistic is lower than 0.1, indicating that the model is identifiable. The value of the Cragg–Donald Wald F is higher than 10, indicating that the model does not have an issue with a weak IV. The p-value corresponding to Hansen’s J statistic is larger than 0.1, suggesting that the IVs chosen for the model are strictly exogenous. After estimation using the IV-DID model, the coefficients of the GFRI × Post are still significantly less than −0.2, which indicates that causality exists between the GFRI experimental zone policy and the reduction of the intensity of carbon emissions.
5.6 Robust test
5.6.1 Tests for the existence of expected effects
Before the launch date of the GFRI experimental zone policy, seven ministries and commissions of China released the Guidance Opinions on Constructing a System of Green Finance, which may have led to the expected effects of the GFRI experimental zone policy. Whenever the government releases policy information in advance, people’s behavior may change as a result of the policy information. If a reinforcing behavioral consequence (nondesirable outcome) exists before the policy is implemented, a peak or valley value is reached, so there is a significant downward or upward effect after the policy is implemented (desirable outcome). This is attributed to the role of expectations (Goodman-Bacon and Marcus, 2020) rather than the effects of the GFRI experimental zone policy itself. Therefore, we construct an empirical model to examine whether there is an expected effect.
In Eq. (5), k = 1 or 2, and
Columns (1) and (2) in Table 6 reveal that none of the coefficients of GFRI × Post(−1) pass the significance test; columns (3) and (4) also reveal that the coefficients of GFRI × Post(−2) are insignificant, implying that there is no dramatic effect on reducing the intensity of carbon emission in the scenario 1 and 2 years before the launch of the GFRI experimental zone policy. Thus, there is no expected effect, which demonstrates that the effect of the GFRI experimental zone policy remains robust.
5.6.2 Nonrandom selection of discussions
The ideal condition for applying the DID model to assess the effectiveness of the GFRI experimental zone policy is that the GFRI experimental zone cities and non-GFRI experimental zone cities are chosen randomly; however, the reality is that the selection of GFRI experimental zone cities is based on factors, such as the rank of the city, its geographic location, and the condition of its resources. These inherent differences change over time, which affects the reduction of carbon emission intensity and leads to biased estimates. Referring to the methodology of Li et al. (2016) and Xue and Chen (2022), the interaction terms between the selection criteria variable and the time trend and the interaction terms on the time trend variable and the control variable are added to the benchmark model. The time trend is the length of time since the start of the experimental zone city. We then construct the model as follows:
In Eq. (6),
Columns (1) and (2) in Table 7 demonstrate that the coefficients of GFRI × Post are both dramatically less than 0 after introducing interaction terms on the big city dummy variable and time trend (BC × Trend); columns (3) and (4) show that the coefficients of GFRI × Post are still significantly less than 0 after introducing interaction terms on the capital city dummy variable and time trend (CC × Trend). Compared to the baseline estimates, the coefficients of GFRI × Post were essentially similar, which suggests that the estimation results are robust.
5.6.3 Excluding the effects of other relevant policy interferences
Multiple policies are often introduced to achieve a particular economic or social goal, leading to cross-cutting or parallel policies, which in turn affect the assessment of the effectiveness of a particular policy (Goodman-Bacon and Marcus, 2020). When the GFRI experimental zone policy is launched, other pollution and carbon reduction policies may also reduce carbon emissions, which may result in bias in the estimated coefficients for the GFRI. For example, to meet the goal of decreasing carbon emission intensity, the Chinese government implemented two batches of carbon emission trading pilot policies in 2011 and 2017 and an environmental protection fee-to-tax policy in 2017, in which 12 provinces raised their minimum environmental tax rates. We add dummy variables for these two policies to the base model to further control for the impacts of other pollution and carbon reduction policies, and we construct the model as follows:
In Eq. (7), OPCR represents other pollution and carbon reduction policies, including the policy on the conversion of environmental protection fees into taxes (EPFT) and the carbon emission trading (CET) pilot policy. EPFT is a dummy variable, which equals 1 if the corresponding province of the city
Columns (1) and (2) in Table 8 demonstrate that the coefficients of GFRI × Post have not changed dramatically after accounting for the impacts of the environmental fee-to-tax policy. Columns (3) and (4) demonstrate that the coefficients of GFRI × Post do not markedly differ from the baseline model after accounting for the effect of the CET pilot policy, which implies that the effect of the GFRI experimental zone policy on reducing carbon emission intensity is not disturbed by other relevant policies. Thus, the impact of the GFRI experimental zone policy on decreasing the intensity of carbon emissions remains robust.
5.6.4 Placebo test
In the previous analyses, the effect of random factors has not yet been discussed. If the control and treatment groups are disturbed by random factors after the launch of the GFRI experimental zone policy, resulting in the same trend in terms of change, this means that there is bias in assessing the effect of the GFRI experimental zone policy on reducing carbon emission intensity. Ferrara et al. (2012) and Cai et al. (2016) argued that a placebo test can identify the effect of the random factor. In the case of the pilot policy, individuals (cities) are randomly selected, or the policy pilot individuals (cities) and the start date of the policy pilots are randomly selected simultaneously. If the coefficients are identical for the control and treatment groups after estimation by applying the DID model, this implies that the impact of the pilot policy is interfered with by a stochastic factor, so the estimation results are biased.
Drawing on the methodology of Ferrara et al. (2012) and Cai et al. (2016), we apply a placebo test to examine whether the effect of the GFRI experimental zone policy on reducing carbon emission intensity is disturbed by other random effects, which are divided into two scenarios. In Scenario 1, there is a random selection of pseudo-GFRI experimental zone cities. First, we keep the sample of 2021, randomly selecting six cities as pseudo-GFRI experimental zone cities, and we keep the codes of the cities. The pseudo-GFRI experimental zone city data are then merged with the original sample data according to the city codes, and the matched city samples are the pseudo-GFRI experimental zone city sample group, while the unmatched samples are the pseudo-non-GFRI experimental zone city sample group. Finally, dummy variables for the pseudo-GFRI experimental zone policy are created by comparing each period with the point in time when the GFRI experimental zone policy was initiated and are estimated using the DID model.
In Scenario 2, both pseudo-GFRI experimental zone policy years and cities are randomly selected. First, we randomly select a year as the pseudo-GFRI experimental zone policy year according to each city, and then randomly select six cities as pseudo-GFRI experimental zone cities and keep the city’s code and year. Again, the pseudo-GFRI experimental zone city data are merged with the raw sample data according to city code and year. Finally, dummy variables for the pseudo-GFRI experimental zone policy are generated, and the DID model is applied to assess the influence of the pseudo-GFRI experimental zone policy on reducing carbon emission intensity. This process is performed 1,000 times to obtain a placebo test result. Figures 3, 4 present the placebo test results.
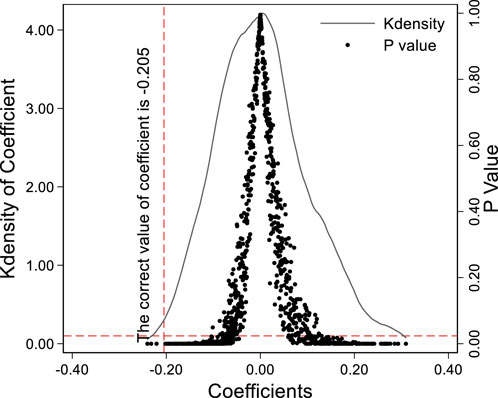
FIGURE 3. Results under random selection only of GFRI experimental cities. Notes: The red vertical dashed line represents the real coefficient estimation of the GFRI. The red horizontal dashed line represents that the p-value corresponding to the coefficient is 0.1. The distributions of the coefficient densities and p-values are the results of performing 1,000 times of random sampling.
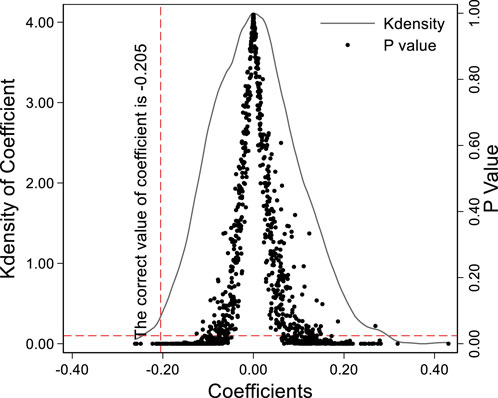
FIGURE 4. Results under simultaneous random selection of GFRI experimental cities and years. Notes: The red vertical dashed line represents the real coefficient estimation of the GFRI. The red horizontal dashed line represents that the p-value corresponding to the coefficient is 0.1. The distributions of the coefficient densities and p-values are the results of performing 1,000 times of random sampling.
The results in Figures 3, 4 demonstrate that the coefficients of pseudo-GFRI experimental zone policy are mostly distributed around 0 after the implementation of the placebo test, and the p-values corresponding to the coefficients of the pseudo-GFRI experimental zone policy are higher than 0.1, which is dissimilar to the real coefficient of GFRI experimental zone policy. This implies that the coefficient estimates in the time-varying DID model are not affected by stochastic factors, so the influence of the GFRI experimental zone policy on reducing carbon emission intensity remains robust.
6 Further discussion
Notably, green finance is an essential tool for reducing financing restraints (Liu et al., 2023; Zhang, 2023), and government environmental protection degrees and fiscal status affect corporate carbon abatement behavior (You et al., 2019; Lin et al., 2022; Wei et al., 2023), which may occur in association with the effect of the GFRI experimental zone policy in decreasing carbon emission intensity. Although some studies consider energy structural adjustment and green innovation as channels through which green finance affects carbon abatement (Ran and Zhang, 2023), reducing industrial energy intensity and increasing green project financing are also important pathways for green finance to reduce carbon emission intensity. Accordingly, this section further explores the heterogeneity of the impacts of the GFRI experimental zone policy on carbon emission intensity and then explores their mediating effects.
6.1 Heterogeneity analysis
6.1.1 Heterogeneity based on different environmental protection degrees
Strict environmental regulations and greater environmental pressures have prompted firms to engage in technological innovations that lead to significant reductions in the consumption of nonclean energy and raise environmental performance (You et al., 2019; Lin et al., 2022; Zhang and Zhao, 2023). However, if the government strengthens the degree of environmental protection to alleviate its own fiscal pressure or if it directly closes down high-energy-consuming enterprises to fulfill its political goals, the increase in the degree of environmental protection may undermine the effectiveness of the pilot zone’s policy in reducing carbon emission intensity. At this point, the government’s role in environmental protection becomes particularly important.
To analyze whether there is a difference in the influence of the GFRI experimental zone policy on reducing the intensity of carbon emissions under different degrees of environmental protection, we take the following measures. First, we calculate the mean values of the environmental protection word frequency and the number of environmental protection penalties according to the sample period for each city. Second, according to the size of the mean value of the environmental protection word frequency, we divide the entire sample equally into a sample group with a low frequency of environmental words and one with a high frequency of environmental words. Third, according to the size of the mean value of the number of environmental protection penalties, we divide the sample equally into a sample group with a low number of environmental protection penalties and one with a high number of environmental protection penalties. Finally, the estimation is performed for the groups. Table 10 reports the estimated results.
To further verify whether there is heterogeneity in the effect of the experimental zone policy on reducing carbon emission intensity under different degrees of government environmental protection and fiscal pressure, we introduce an interaction term between the GFRI experimental zone policy and the frequency of environmental protection words and the number of environmental protection penalties and then estimate it. The results are shown in Table 9.
Columns (1) and (2) in Panel A of Table 9 demonstrate that the coefficients of
Columns (1) and (2) in Panel B in Table 9 show that the coefficients of
6.1.2 Heterogeneity based on different statuses of fiscal pressure
Increasing government subsidies to enterprises for their green R&D is an effective way to achieve carbon emission abatement. However, with increasing fiscal pressures, the contribution of fiscal R&D expenditures to green technology innovation is diminished (Wei et al., 2023). This implies that green finance policy, as an important complement to fiscal policy, is likely to play an essential role in reducing carbon emissions.
To further test whether there is a difference in the effect of the GFRI experimental zone policy on reducing the intensity of carbon emissions under different levels of fiscal pressure, the following strategy is adopted. First, the average of the fiscal gap and the tax gap during the sample period for each city is calculated. Second, the means of the fiscal gap are sorted, and the entire sample is divided equally into two groups, namely, the sample group with a low fiscal gap and one with a high fiscal gap. Third, based on the size of the mean value of the tax gap, the entire sample is divided into a sample group with a small tax gap and one with a large tax gap. Finally, regressions are performed on the various groups. Table 10 displays the estimated results.
Additionally, to further examine whether the effect of the GFRI experimental zone policy on reducing carbon emission intensity is heterogeneous because of differences in fiscal pressures, we introduce the interaction terms of the GFRI experimental zone policy with the fiscal gap and the tax gap in our model and then estimate them. The specific results are shown in Table 10.
Columns (1) and (2) in Panel A of Table 10 demonstrate that in cases of a low fiscal gap, the coefficient of is −0.213, while in cases of a high fiscal gap, the coefficient of
Columns (1) and (2) in Panel B of Table 10 demonstrate that the coefficients of
The reason for this is that as fiscal pressures increase, the R&D and green subsidies available to enterprises gradually decrease; furthermore, the government may increase the tax and fee burdens of enterprises to alleviate the fiscal pressure, which is not helpful for green technological innovation in enterprises (Bai et al., 2019; Wei et al., 2023). This step is also not conducive to the acquisition and application of low- and zero-carbon technology and equipment, thus weakening the effects of the GFRI experimental zone policy on reducing carbon emission intensity.
6.2 Mechanism
The research hypothesis suggests that the GFRI experimental zone policy decreases the intensity of carbon emissions by boosting green technology advancement, improving the energy structure, promoting green project financing, and lowering the energy intensity of the industry. In addition, green project financing decreases carbon emissions by reducing the energy intensity of the industry. For this reason, we further test whether these mediating effects hold.
A common method to test for the existence of mediating mechanisms is the mediation effects model approach proposed by Baron and Kenny (1986), which uses stepwise regression. However, Jiang et al. (2022) emphasized that the application of the stepwise estimation method to test for mediation effects may cause endogeneity issues because of the omission of variables. To address the problem of omitted variables, as well as multi-mediation effects, Taylor et al. (2008), Cugno et al. (2021), and Zhou and Yamamoto (2023) proposed a parallel-serial multiple mediation effects model, which is estimated using a structural equation model approach. Bootstrap methods are then applied to test for direct and indirect effects. Drawing on the methodology of Taylor et al. (2008), Cugno et al. (2021), and Zhou and Yamamoto (2023), the mediating effects model is constructed as follows:
In Eqs (8–10),
Column (1) of Panel A in Table 11 shows that the coefficient of the GFRI × Post fails the significance test, implying that the GFRI experimental zone policy does not affect green innovation. Column (2) reveals that the coefficient of the GFRI × Post also fails the significance test, suggesting that the GFRI experimental zone policy does not affect the energy structure transition. Column (3) demonstrates that the coefficient of the GFRI × Post is dramatically different from 0, indicating that the GFRI experimental zone policy can significantly contribute to green project financing. Column (4) reveals that the coefficients of both GFRI × Post and GPF are significantly less than 0, implying that the GFRI experimental zone policy and green project finance can dramatically decrease the energy intensity of the industry. Column (5) indicates that the GFRI experimental zone policy, green innovation, the energy structure, green project financing, and the energy intensity of the industry all dramatically affect carbon emission intensity.
After the bootstrap method is applied to examine the significance of the direct and indirect impact coefficients of the variables, Panel B in Table 11 indicates that the direct effect of the GFRI experimental zone policy is −0.104. The individual mediating effects via green innovation, the energy structure, green project finance, and industrial carbon emission intensity are −0.006, 0.001, −0.022, and −0.012, respectively. The serial mediation effect of the GFRI experimental zone policy on carbon emission intensity via increased green project finance to reduce industrial energy intensity is −0.001. Moreover, the direct effect of the GFRI experimental zone policy in terms of reducing carbon emission intensity is 72%, and the indirect effect is more than 20%. Thus, the mediating mechanism of the GFRI experimental zone policy can decrease carbon emission intensity via green project financing and the reduction of industrial energy intensity, which supports Zhang’s (2023) and Qian and Yu’s (2023) findings that green financial instruments alleviate financing constraints, thereby achieving the goal of reducing carbon emission intensity.
As the mediating variables of the GFRI experimental zone policy that affect carbon emission intensity are correlated by economic linkages, accurately distinguishing the magnitude of the effects of these mediating mechanisms may not be possible in a parallel-serial multiple mediation effects model. For this reason, the effect of the GFRI experimental zone policy on each mediating variable is estimated while controlling for the effects of other mediating variables and economic growth, and a structural equation model is used to estimate the magnitude of the mediating effect. The estimation model is set up as follows:
In Eqs (11–12),
Columns (1) and (8) in Table 12 show that the effect of the GFRI experiment zone policy on reducing carbon emission intensity through green innovation is −0.006, accounting for 15.4% of the total mediating effect; the effect of the GFRI experiment zone policy on reducing carbon emission intensity through the energy structure is 0.002, accounting for −5.2% of the total mediating effect; the effect of the GFRI experiment zone policy on reducing carbon emission intensity through green project financing −0.023, accounting for 59.0% of the total mediating effect; the effect of the GFRI experiment zone policy on reducing carbon emission intensity through industrial energy intensity is −0.012, accounting for 30.8% of the total mediating effect. We still found that the mediating channels for promoting green innovation and facilitating energy restructuring are insignificant and that the mediating roles of increasing green project financing and reducing industrial energy intensity are significantly existent. Thus, part of the inference in Hypothesis 3 is tested. The mediating role of green project financing is the strongest. This further reinforces the conclusions in Table 11.
In contrast to the findings of Zhang (2023), our findings fail to establish that the GFRI experimental zone policy promotes green innovation and improves the energy structure, which is consistent with the findings of Shen and Cao (2020), Shi et al. (2022), and Ran and Zhang (2023). The possible reasons are as follows. First, firms consider environmental protection pressure and use green finance funds more for energy-saving and carbon-reducing equipment and technologies rather than for green innovation and green energy production. Second, in the early stages of the implementation of the GFRI experimental zone policy, enterprises lacked endogenous incentives to innovate and turned to end-of-pipe pollution control because of the lack of detailed regulations and supporting policies, thereby further depleting the resources needed for green innovation (Shi et al., 2022). Third, under the condition of strict environmental protection pressure, enterprises will expend on environmental protection to meet the corresponding environmental regulatory standards, which will reduce the original green R&D investment of enterprises and thus offset the impact of the green finance pilot policy on green innovation (Ran and Zhang, 2023).
7 Conclusion and policy implications
Carbon emission intensity reduction cannot be separated from support for green financial policy, and as an essential complement to green fiscal policy, its role cannot be ignored. Viewing the GFRI experimental zone policy as a quasi-natural experiment, this study evaluates the impact of the policy on reducing the intensity of carbon emissions by applying a time-varying DID model with data from Chinese cities from 2005 to 2021. Meanwhile, we explore the heterogeneity of the impact of the GFRI experimental zone policy on reducing carbon emission intensity according to differences in the degrees of government environmental protection and fiscal pressure. Finally, a parallel-serial mediation effects model and a structural equation model are utilized to test the mediation mechanism of the GFRI experimental zone policy.
The following are the primary conclusions of this study. (1) The GFRI experimental zone policy can effectively decrease the intensity of carbon emissions, and this effect is more prominent in cities with high credit and bond financing scales, thus further strengthening evidence of causality. (2) The role of the GFRI experimental zone policy in reducing the intensity of carbon emissions is weaker in cities with higher degrees of government environmental protection and fiscal pressure. Moreover, as the degrees of government environmental protection and fiscal pressure increase, the effect of the GFRI experimental zone policy on reducing carbon emission intensity decreases, which provides insights into how governments face high fiscal pressures to reduce the intensity of carbon emissions. (3) Enhancing green project finance and reducing industrial energy intensity are mediating channels through which the GFRI experimental zone policy reduces carbon emission intensity, but their mediating roles in promoting green innovation and improving the energy structure are not obvious. In addition, the magnitude of the direct effect is about 72%, and the magnitude of the indirect effect is more than 20% of the overall effects of the GFRI experimental zone policy in terms of reducing carbon emission intensity.
The following insights are derived from this study to further decrease carbon emission intensity. (1) There is a need to further expand the range of cities selected as GFRI experimental zones. Given that the GFRI experimental zone policy has significantly reduced carbon emission intensity, it needs to be replicated on a wider scale to enhance the effect of reducing carbon emission intensity. (2) There is also a need to innovate regarding green financial products and services, such as establishing carbon bank accounts, carrying out carbon emission rights mortgage financing, setting up carbon-neutral funds, granting green credits, and issuing green bonds, to activate market opportunities for green finance and empower carbon abatement. (3) Green finance policies must enhance financing support for enterprises that provide and use zero- and low-carbon technologies, such as solar and wind energy, thus contributing to reducing carbon emission intensity. (4) Governments need more effective green finance policies to facilitate the structural transformation of clean energy and encourage green technological innovation, as well as to prevent companies from obtaining green financing under the pretext of green energy and green innovation but are not engaging in such activities. (5) Regions with high fiscal pressure should rely more on green financial policies to reduce carbon emission intensity. As the reduction of carbon emission intensity relies on certain carbon-reducing equipment and technologies, this requires a large amount of financial investment. However, fiscal support policies for reducing carbon emission intensity face budget constraints, especially when governments experience substantial fiscal pressures that may weaken the effectiveness of the GFRI experiment zone policy in reducing carbon emission intensity. The green finance policy plays a crucial role in easing constraints on financing and thus contributes to reducing carbon emission intensity.
Although this study provides important insights into utilizing the GFRI experimental zone policy to reduce carbon emission intensity, its limitations are as follows. First, given constraints in data accessibility, this study has not examined the link between the size of green finance and the reduction of carbon emission intensity of firms from a micro perspective. Second, the effect of specific green financial products on reducing carbon emission intensity has not also been explored because of the lack of associated data. Third, in analyzing the contribution of the GFRI experimental zone policy to decreasing carbon emission intensity, its spatial spillover effects have not been considered. Cities in neighboring regions that have also carried out the policy may affect the effectiveness of green finance policies in local areas. In the future, we will continue to explore the causality between the GFRI experimental zone policy and the reduction of carbon emission intensity from a micro perspective, as well as examine spatial spillovers, to provide empirical insights into the better utilization of green finance to decrease carbon emission intensity.
Data availability statement
The original contributions presented in the study are included in the article/supplementary material, further inquiries can be directed to the corresponding author.
Author contributions
YY: Conceptualization, Data curation, Formal Analysis, Funding acquisition, Writing–original draft. CP: Methodology, Supervision, Validation, Writing–review and editing.
Funding
The author(s) declare financial support was received for the research, authorship, and/or publication of this article. This work is supported by the Scientific Research Program of Tianjin Municipal Education Commission of China in 2022 (Grant No. 2022SK034).
Acknowledgments
The authors of this article express their sincere gratitude to the editors as well as reviewers for their professional, timely, and efficient services and helpful suggestions.
Conflict of interest
The authors declare that the research was conducted in the absence of any commercial or financial relationships that could be construed as a potential conflict of interest.
Publisher’s note
All claims expressed in this article are solely those of the authors and do not necessarily represent those of their affiliated organizations, or those of the publisher, the editors and the reviewers. Any product that may be evaluated in this article, or claim that may be made by its manufacturer, is not guaranteed or endorsed by the publisher.
References
Acemoglu, D., Johnson, S., and Robinson, J. A. (2001). The colonial origins of comparative development: an empirical investigation. Am. Econ. Rev. 91 (5), 1369–1401. doi:10.1257/aer.91.5.1369
Athey, S., and Imbens, G. W. (2022). Design-based analysis in difference-in-differences settings with staggered adoption. J. Econ. 226 (1), 62–79. doi:10.1016/j.jeconom.2020.10.012
Bai, R., and Lin, B. (2024). Green finance and green innovation: theoretical analysis based on game theory and empirical evidence from China. Int. Rev. Econ. 89, 760–774. doi:10.1016/j.iref.2023.07.046
Bai, Y., Song, S., Jiao, J., and Yang, R. (2019). The impacts of government R&D subsidies on green innovation: evidence from Chinese energy-intensive firms. J. Clean. Prod. 233, 819–829. doi:10.1016/j.jclepro.2019.06.107
Baker, A. C., Larcker, D. F., and Wang, C. C. (2022). How much should we trust staggered difference-in-differences estimates? J. Financial Econ. 144 (2), 370–395. doi:10.1016/j.jfineco.2022.01.004
Bakry, W., Mallik, G., Nghiem, X. H., Sinha, A., and Vo, X. V. (2023). Is green finance really “green”? Examining the long-run relationship between green finance, renewable energy and environmental performance in developing countries. Renew. Energy 208, 341–355. doi:10.1016/j.renene.2023.03.020
Baron, R. M., and Kenny, D. A. (1986). The moderator–mediator variable distinction in social psychological research: conceptual, strategic, and statistical considerations. J. Personality Soc. Psychol. 51 (6), 1173–1182. doi:10.1037/0022-3514.51.6.1173
Cai, X., Lu, Y., Wu, M., and Yu, L. (2016). Does environmental regulation drive away inbound foreign direct investment? Evidence from a quasi-natural experiment in China. J. Dev. Econ. 123, 73–85. doi:10.1016/j.jdeveco.2016.08.003
Callaway, B., and Li, T. (2023). Policy evaluation during a pandemic. J. Econ. 236 (1), 105454. doi:10.1016/j.jeconom.2023.03.009
Callaway, B., and Sant’Anna, P. H. (2021). Difference-in-differences with multiple time periods. J. Econ. 225 (2), 200–230. doi:10.1016/j.jeconom.2020.12.001
Cao, X., Deng, M., and Li, H. (2021). How does e-commerce city pilot improve green total factor productivity? Evidence from 230 cities in China. J. Environ. Manag. 289, 112520. doi:10.1016/j.jenvman.2021.112520
Chen, J., Li, Y., Xu, Y., Vardanyan, M., Shen, Z., and Song, M. (2023). The impact of fiscal technology expenditures on innovation drive and carbon emissions in China. Technol. Forecast. Soc. Change 193, 122631. doi:10.1016/j.techfore.2023.122631
Chen, S., Oliva, P., and Zhang, P. (2022). The effect of air pollution on migration: evidence from China. J. Dev. Econ. 156, 102833. doi:10.1016/j.jdeveco.2022.102833
Cragg, J. G., and Donald, S. G. (1993). Testing identifiability and specification in instrumental variable models. Econ. Theory 9 (2), 222–240. doi:10.1017/S0266466600007519
Cugno, M., Castagnoli, R., and Büchi, G. (2021). Openness to Industry 4.0 and performance: the impact of barriers and incentives. Technol. Forecast. Soc. Change 168, 120756. doi:10.1016/j.techfore.2021.120756
De Chaisemartin, C., and d’Haultfoeuille, X. (2020). Two-way fixed effects estimators with heterogeneous treatment effects. Am. Econ. Rev. 110 (9), 2964–2996. doi:10.1257/aer.20181169
Du, G. (2023). Nexus between green finance, renewable energy, and carbon intensity in selected Asian countries. J. Clean. Prod. 405, 136822. doi:10.1016/j.jclepro.2023.136822
Ferrara, E. L., Chong, A., and Duryea, S. (2012). Soap operas and fertility: evidence from Brazil. Am. Econ. J. Appl. Econ. 4 (4), 1–31. doi:10.1257/app.4.4.1
Goodman-Bacon, A. (2021). Difference-in-differences with variation in treatment timing. J. Econ. 225 (2), 254–277. doi:10.1016/j.jeconom.2021.03.014
Goodman-Bacon, A., and Marcus, J. (2020). Using difference-in-differences to identify causal effects of COVID-19 policies. Surv. Res. Methods 14 (2), 153–158. Southampton: European Survey Research Association. doi:10.2139/ssrn.3603970
Hansen, L. P. (1982). Large sample properties of generalized method of moments estimators. Econometrica 50 (2), 1029–1054. doi:10.2307/1912775
He, L., Zhong, T., and Gan, S. (2022). Green finance and corporate environmental responsibility: evidence from heavily polluting listed enterprises in China. Environ. Sci. Pollut. Res. 29, 74081–74096. doi:10.1007/s11356-022-21065-5
He, N., Zeng, S., and Jin, G. (2023). Achieving synergy between carbon mitigation and pollution reduction: does green finance matter? J. Environ. Manag. 342, 118356. doi:10.1016/j.jenvman.2023.118356
Hou, H., Zhu, Y., Wang, J., and Zhang, M. (2023). Will green financial policy help improve China’s environmental quality? The role of digital finance and green technology innovation. Environ. Sci. Pollut. Res. 30, 10527–10539. doi:10.1007/s11356-022-22887-z
Irfan, M., Razzaq, A., Sharif, A., and Yang, X. (2022). Influence mechanism between green finance and green innovation: exploring regional policy intervention effects in China. Technol. Forecast. Soc. Change 182, 121882. doi:10.1016/j.techfore.2022.121882
Jiang, W., Li, X., Liu, R., and Song, Y. (2022). Local fiscal pressure, policy distortion and energy efficiency: micro-evidence from a quasi-natural experiment in China. Energy 254, 124287. doi:10.1016/j.energy.2022.124287
Kleibergen, F., and Paap, R. (2006). Generalized reduced rank tests using the singular value decomposition. J. Econ. 133 (1), 97–126. doi:10.1016/j.jeconom.2005.02.011
Lan, J., Wei, Y., Guo, J., Li, Q., and Liu, Z. (2023). The effect of green finance on industrial pollution emissions: evidence from China. Resour. Policy 80, 103156. doi:10.1016/j.resourpol.2022.103156
Lee, C. C., and Lee, C. C. (2022). How does green finance affect green total factor productivity? Evidence from China. Energy Econ. 107, 105863. doi:10.1016/j.eneco.2022.105863
Lee, C. C., Wang, F., and Chang, Y. F. (2023). Towards net-zero emissions: can green bond policy promote green innovation and green space? Energy Econ. 121, 106675. doi:10.1016/j.eneco.2023.106675
Li, C., and Gan, Y. (2021). The spatial spillover effects of green finance on ecological environment—empirical research based on spatial econometric model. Environ. Sci. Pollut. Res. 28, 5651–5665. doi:10.1007/s11356-020-10961-3
Li, C., Sampene, A. K., Agyeman, F. O., Brenya, R., and Wiredu, J. (2022). The role of green finance and energy innovation in neutralizing environmental pollution: empirical evidence from the MINT economies. J. Environ. Manag. 317, 115500. doi:10.1016/j.jenvman.2022.115500
Li, P., Lu, Y., and Wang, J. (2016). Does flattening government improve economic performance? Evidence from China. J. Dev. Econ. 123, 18–37. doi:10.1016/j.jdeveco.2016.07.002
Liang, S., Wang, P., Jia, C., and Zhu, J. (2023). Studying green financing, factor allocation efficiency, and regional productivity growth in renewable energy industries. Renew. Energy 214, 130–139. doi:10.1016/j.renene.2023.05.072
Lin, W., Xiao, Y., Yu, H., and Shen, S. (2022). Does vertical environmental protection pressure promote convergence of urban air pollution? J. InnovationKnowledge 7 (2), 100186. doi:10.1016/j.jik.2022.100186
Liu, C., Dai, C., Chen, S., and Zhong, J. (2023). How does green finance affect the innovation performance of enterprises? Evidence from China. Environ. Sci. Pollut. Res. 30, 84516–84536. doi:10.1007/s11356-023-28063-1
Liu, N., Deng, M., and Cao, X. (2021). Does the E-commerce transformation of cities promote green and high-quality development? Evidence from a quasi-natural experiment based on national E-commerce demonstration cities. J. Finance Econ. 47 (04), 49–63. doi:10.16538/j.cnki.jfe.20201115.401
Liu, S., and Wang, Y. (2023). Green innovation effect of pilot zones for green finance reform: evidence of quasi natural experiment. Technol. Forecast. Soc. Change 186, 122079. doi:10.1016/j.tech-fore.2022.122079
Ma, L., Iqbal, N., Bouri, E., and Zhang, Y. (2023). How good is green finance for green innovation? Evidence from the Chinese high-carbon sector. Resour. Policy 85, 104047. doi:10.1016/j.resourpol.2023.104047
Qian, S., and Yu, W. (2023). Green finance and environmental, social, and governance performance. Int. Rev. Econ. Finance 89, 1185–1202. doi:10.1016/j.iref.2023.08.017
Ran, C., and Zhang, Y. (2023). Does green finance stimulate green innovation of heavy-polluting enterprises? Evidence from green finance pilot zones in China. Environ. Sci. Pollut. Res. 30, 60678–60693. doi:10.1007/s11356-023-26758-z
Ran, C., and Zhang, Y. (2023). The driving force of carbon emissions reduction in China: does green finance work. J. Clean. Prod. 421, 138502. doi:10.1016/j.jclepro.2023.138502
Roth, J., Sant’Anna, P. H., Bilinski, A., and Poe, J. (2023). What’s trending in difference-in-differences? A synthesis of the recent econometrics literature. J. Econ. 235 (2), 2218–2244. doi:10.1016/j.jeconom.2023.03.008
Sachs, J. D., Woo, W. T., Yoshino, N., and Taghizadeh-Hesary, F. (2019). “Importance of green finance for achieving sustainable development goals and energy security,” in Handbook of green finance: energy security and sustainable development. Editors J. D. Sachs, W. T. Woo, N. Yoshino, and F. Taghizadeh-Hesary (Singapore: Springer), 1–10.
Shen, T., and Cao, M. (2020). Does the green finance pilot reduce the energy intensity? J. Financial Dev. Res. 2, 3–10. doi:10.19647/j.cnki.37-1462/f.2020.02.001
Shi, J., Yu, C., Li, Y., and Wang, T. (2022). Does green financial policy affect debt-financing cost of heavy-polluting enterprises? An empirical evidence based on Chinese pilot zones for green finance reform and innovations. Technol. Forecast. Soc. Change 179, 121678. doi:10.1016/j.techfore.2022.121678
Sun, C. (2023). How are green finance, carbon emissions, and energy resources related in Asian sub-regions? Resour. Policy 83, 103648. doi:10.1016/j.resourpol.2023.103648
Sun, C., and Zhang, Y. (2023). Banking sectors and carbon neutrality goals: mediating concern of financial inclusion. Environ. Sci. Pollut. Res. 30 (23), 64637–64650. doi:10.1007/s11356-023-26302-z
Taylor, A. B., MacKinnon, D. P., and Tein, J. Y. (2008). Tests of the three-path mediated effect. Organ. Res. Methods 11 (2), 241–269. doi:10.1177/1094428107300344
Umar, M., and Safi, A. (2023). Do green finance and innovation matter for environmental protection? A case of OECD economies. Energy Econ. 119, 106560. doi:10.1016/j.eneco.2023.106560
Wan, Q., Qian, J., Baghirli, A., and Aghayev, A. (2022). Green finance and carbon reduction: implications for green recovery. Econ. Analysis Policy 76, 901–913. doi:10.1016/j.eap.2022.09.022
Wan, Y., Sheng, N., Wei, X., and Su, H. (2023). Study on the spatial spillover effect and path mechanism of green finance development on China's energy structure transformation. J. Clean. Prod. 415, 137820. doi:10.1016/j.jclepro.2023.137820
Wan, Y., Sheng, N., Wei, X., Tan, M., and Ling, J. (2023). Effect of green finance reform and innovation pilot zone on improving environmental pollution: an empirical evidence from Chinese cities. Environ. Sci. Pollut. Res. 30, 75058–75077. doi:10.1007/s11356-023-27657-z
Wang, A., Si, L., and Hu, S. (2023). Can the penalty mechanism of mandatory environmental regulations promote green innovation? Evidence from China's enterprise data. Energy Econ. 125, 106856. doi:10.1016/j.eneco.2023.106856
Wang, J., Tian, J., Kang, Y., and Guo, K. (2023). Can green finance development abate carbon emissions: evidence from China. Int. Rev. Econ. 88, 73–91. doi:10.1016/j.iref.2023.06.011
Wang, T., Liu, X., and Wang, H. (2022). Green bonds, financing constraints, and green innovation. J. Clean. Prod. 381, 135134. doi:10.1016/j.jclepro.2022.135134
Wang, T., Umar, M., Li, M., and Shan, S. (2023). Green finance and clean taxes are the ways to curb carbon emissions: an OECD experience. Energy Econ. 124, 106842. doi:10.1016/j.eneco.2023.106842
Wang, X. (2023). Exploring the role of resource industry dependence and green finance in green development efficiency in the context of post-Covid-19 period. Resour. Policy 85, 103866. doi:10.1016/j.resourpol.2023.103866
Wei, L., Lin, B., Zheng, Z., Wu, W., and Zhou, Y. (2023). Does fiscal expenditure promote green technological innovation in China? Evidence from Chinese cities. Environ. Impact Assess. Rev. 98, 106945. doi:10.1016/j.eiar.2022.106945
Xiong, Y., and Dai, L. (2023). Does green finance investment impact on sustainable development: role of technological innovation and renewable energy. Renew. Energy 214, 342–349. doi:10.1016/j.renene.2023.06.002
Xu, J., She, S., Gao, P., and Sun, Y. (2023). Role of green finance in resource efficiency and green economic growth. Resour. Policy 81, 103349. doi:10.1016/j.resourpol.2023.103349
Xue, F., and Chen, X. (2022). The carbon emission reduction effect of green fiscal policy: evidence from the “national comprehensive demonstration city of energy saving and emission reduction fiscal policy”. J. Finance Econ. 48 (7), 79–93. doi:10.16538/j.cnki.jfe.20211114.204
Yan, C., Mao, Z., and Ho, K. C. (2022). Effect of green financial reform and innovation pilot zones on corporate investment efficiency. Energy Econ. 113, 106185. doi:10.1016/j.eneco.2022.106185
Yan, X., Yang, C., and Zhang, R. (2023). How does green finance derive the resource efficiency and decarbonization of the economy? Resour. Policy 85, 103934. doi:10.1016/j.resourpol.2023.103934
Yang, Y., and Hao, F. (2023). Does the carbon emission rights trading pilot policy aggravate local government fiscal pressure? Evidence from China. Environ. Sci. Pollut. Res. 30, 65217–65236. doi:10.1007/s11356-023-26914-5
Yao, N., Fabus, M., Hu, L., and Qian, F. (2023). Resource efficiency and economic sustainability in APEC: assessing the financial sector's role. Resour. Policy 85, 103963. doi:10.1016/j.resourpol.2023.103963
Yi, H., Hao, L., Liu, A., and Zhang, Z. (2023). Green finance development and resource efficiency: a financial structure perspective. Resour. Policy 85, 104068. doi:10.1016/j.resourpol.2023.104068
You, D., Zhang, Y., and Yuan, B. (2019). Environmental regulation and firm eco-innovation: evidence of moderating effects of fiscal decentralization and political competition from listed Chinese industrial companies. J. Clean. Prod. 207, 1072–1083. doi:10.1016/j.jclepro.2018.10.106
Zhang, H., Wang, Y., Li, R., Si, H., and Liu, W. (2023). Can green finance promote urban green development? Evidence from green finance reform and innovation experimental zone in China. Environ. Sci. Pollut. Res. 30, 12041–12058. doi:10.1007/s11356-022-22886-0
Zhang, K., Li, Y., Qi, Y., and Shao, S. (2021). Can green credit policy improve environmental quality? Evidence from China. J. Environ. Manag. 298, 113445. doi:10.1016/j.jenvman.2021.113445
Zhang, M., and Zhao, Y. (2023). Does environmental regulation spur innovation? Quasi-natural experiment in China. World Dev. 168, 106261. doi:10.1016/j.worlddev.2023.106261
Zhang, P., and Guo, Q. (2023). Carbon emission reduction effects of China's green local government special bonds under ‘dual carbon goals. Finance Res. Lett. 58, 104348. doi:10.1016/j.frl.2023.104348
Zhang, S., and Chen, K. (2023). Green finance and ecological footprints: natural resources perspective of China’s growing economy. Resour. Policy 85, 103898. doi:10.1016/j.resourpol.2023.103898
Zhang, S., Wu, Z., Wang, Y., and Hao, Y. (2021). Fostering green development with green finance: an empirical study on the environmental effect of green credit policy in China. J. Environ. Manag. 296, 113159. doi:10.1016/j.jenvman.2021.113159
Zhang, T. (2023). Can green finance policies affect corporate financing? Evidence from China's green finance innovation and reform experimental zones. J. Clean. Prod. 419, 138289. doi:10.1016/j.jclepro.2023.138289
Zhang, X., Jiang, F., Liu, H., and Liu, R. (2023). Green finance, managerial myopia and corporate green innovation: evidence from Chinese manufacturing listed companies. Finance Res. Lett. 58, 104383. doi:10.1016/j.frl.2023.104383
Zhang, Z., Fu, H., Xie, S., Cifuentes-Faura, J., and Urinov, B. (2023). Role of green finance and regional environmental efficiency in China. Renew. Energy 214, 407–415. doi:10.1016/j.renene.2023.05.076
Zhao, S., He, X., and Faxritdinovna, K. U. (2023). Does industrial structure changes matter in renewable energy development? Mediating role of green finance development. Renew. Energy 214, 350–358. doi:10.1016/j.renene.2023.05.088
Zhou, X., and Tang, X. (2022). Spatiotemporal consistency effect of green finance on pollution emissions and its geographic attenuation process. J. Environ. Manag. 318, 115537. doi:10.1016/j.jenvman.2022.115537
Keywords: GFRI, carbon emission intensity, heterogeneity effect, parallel-serial mediation effect, environmental protection paradox, policy conflicts
Citation: Yang Y and Peng C (2024) Does the green finance reform and innovation experimental zone policy reduce carbon emission intensity? Evidence from Chinese cities. Front. Energy Res. 11:1337594. doi: 10.3389/fenrg.2023.1337594
Received: 13 November 2023; Accepted: 27 December 2023;
Published: 12 January 2024.
Edited by:
Zequn Yang, Central South University, ChinaReviewed by:
Chenghao Sun, Shandong Management University, ChinaChenyang Ran, Dalian University of Technology, China
Copyright © 2024 Yang and Peng. This is an open-access article distributed under the terms of the Creative Commons Attribution License (CC BY). The use, distribution or reproduction in other forums is permitted, provided the original author(s) and the copyright owner(s) are credited and that the original publication in this journal is cited, in accordance with accepted academic practice. No use, distribution or reproduction is permitted which does not comply with these terms.
*Correspondence: Chunli Peng, pcl-1990@163.com; Yun Yang, yangyunpaper@126.com