Self-Similarity, Leaf Litter Traits, and Neighborhood Predicting Fine Root Dynamics in a Common-Garden Forest
- 1The Evergreen State College, Olympia, WA, United States
- 2Lab of Landscape Ecology and Conservation Biology, Landscape Conservation Initiative, Northern Arizona University, Flagstaff, AZ, United States
- 3Conservation Science Partners, Truckee, CA, United States
- 4Department of Biological Sciences, Northern Arizona University, Flagstaff, AZ, United States
- 5Merriam-Powel Center for Environmental Research, Northern Arizona University, Flagstaff, AZ, United States
- 6Department of Life and Environmental Sciences, University of California, Merced, Merced, CA, United States
- 7Sierra Nevada Research Institute, University of California, Merced, Merced, CA, United States
While individual tree genotypes are known to differ in their impacts on local soil development, the spatial genetic influence of surrounding neighboring trees is largely unknown. We examine the hypothesis that fine root dynamics of a focal tree is based on the genetics of the focal tree as well as the genetics of neighbor trees that together define litter inputs to soils of the focal tree. We used a common garden environment with clonal replicates of individual tree genotypes to analyze fine root production, turnover and allocation with respect to modeled neighborhood: (1) foliar mass, (2) foliar condensed tannins (CT), (3) genetic identity of trees, and (4) genetic dissimilarity of neighbors. In support of our central hypothesis, we found that the presence of genetically dissimilar trees and high leaf CT contributions to the soil predicted increased fine root production. In fact, the modeled effects of neighbors accounted for ~90% of the explanatory weight of all models predicting root production. Nevertheless, the ultimate fate of those roots in soil (turnover) and the balance of fine roots relative to aboveground tree mass were still more predictable based on the leaf traits and genetics of the individual focal trees (explaining 99% of the variation accounted for by models). Our data provide support for a method allowing a comparison of the relative effects of individuals vs. spatial neighborhood effects on soils in a genetic context. Such comparisons are important for placing plant-soil feedbacks in a genetic and evolutionary framework because neighbors can decouple feedbacks between an individual and the surrounding environment.
Introduction
Fine roots represent a fundamentally important component of ecosystem carbon (C) and nutrient cycles (Norby and Jackson, 2000; Litton et al., 2007). As such, the spatial influences of neighboring trees on any individual tree's fine root dynamics are important for understanding how ecosystems function. In a now classic reference, Zinke (1962) stated “The literature of forestry and soil science has frequent reference to the effect of different species on soil properties but there are relatively few papers dealing with the spatial variation in these soil properties relative to individual forest trees.” In more recent work, research has expanded from inter-specific effects on soil processes (e.g., Hobbie, 1992; Binkley and Giardina, 1998; Waring et al., 2015) to intra-specific effects (Schweitzer et al., 2008, 2011; LeRoy and Fischer, 2019). Many studies also examine the effects of neighbors on individual plant growth, facilitation, and competition (Aerts, 1999; Callaway, 2002; Maina et al., 2002; Cahill et al., 2010), and it is well-understood that individual plant effects on soils and plant-soil feedbacks have major effects on ecosystem function (Freschet et al., 2012; Bardgett and van der Putten, 2014; Waring et al., 2015). In combination, such studies placed within a genetics framework may allow researchers to evaluate plant-soil feedbacks within the context of evolution and spatially complex biotic environments.
The spatial context for how plants affects soils has major implications for feed-backs affecting plant survival and growth (Bever et al., 2010; Schweitzer et al., 2013). Niche construction can occur when genetically variable, but heritable, interactions between plants and abiotic and biotic components of soils results in differential plant survival, growth, and reproduction. For example, demonstrated genetic-based effects of tree foliar tannin concentration on soils can result in reduced N availability in the rooting zone of riparian trees due to a suppressed microbial decomposition of leaf litter or binding of nitrogen with tannin molecules (Schweitzer et al., 2004). Reduced N-availability may in-turn be associated with increased root proliferation among high foliar-tannin trees (Fischer et al., 2006), and differential offspring survival (Pregitzer et al., 2010; Smith et al., 2012). Similar feedbacks have been documented at the species level where above- and below-ground plant traits influence decomposers, mutualists, predators, and pathogens with strong implications for plant growth and survival (e.g., Hobbie, 1992; Binkley and Giardina, 1998; Wardle et al., 2004; Bever et al., 2010; van de Voorde et al., 2011). Nevertheless, a spatial approach to understanding plant-soil feedbacks at the genetic level is critical to placing plant niche construction and associated biotic interactions in a realistic ecosystem context.
Previous studies have found clear evidence of spatial patterns under individual trees, where tree influences on soils decline with distance from a tree (Zinke, 1962), and can be measured with C and nitrogen (N) isotopes (Weber et al., 2008). These spatial effects have not been documented at the genetic-scale, despite implications for feedbacks between plants and soils and the associated niche and biotic interactions of plant species. In aggregation across a stand, overlapping “zones of influence” (Casper et al., 2003) associated with specific trees should result in variable mosaic neighborhoods of tree influences on soils. Zones of influence should be variable based on the traits of a focal tree genotype (e.g., foliar condensed tannins, CTs, that slow decomposition) and the traits of neighboring tree genotypes where litter mixing occurs. Accordingly, spatial patterns in belowground processes in forests may be driven by neighborhoods of overlapping zones of influence, resulting in spatial mosaics of ecosystem function (Madritch et al., 2009). These mosaic neighborhoods should also represent variable mosaic environments for selection based on plant-soil interactions. A spatial perspective is required to model integrated neighborhood effects, which result from spatially integrated inputs from multiple trees proximal to any measurement location. In single-species stands, variation in genetic composition and size of neighbors should have implications for the nature of plant-soil feedbacks, especially when it has been shown that tree genetics have effects on soils, belowground processes, survival, and growth (Schweitzer et al., 2004; Fischer et al., 2006; Pregitzer et al., 2010; Smith et al., 2012).
The concept of a plant genetic neighborhood is important for describing the cumulative metagenomic influence of plants on ecosystems. Here, we define the plant genetic neighborhood as the metagenomic fingerprint of all plants within a given area, and the plant phenotypic neighborhood as the trait landscape associated with the plant genetic neighborhood. The concept of a genetic and phenotypic neighborhood is analogous to the concept of spatially integrated zones of influence (Casper et al., 2003), but has an additional advantage of allowing researchers to explore the dynamics of these neighborhoods in the context of selection and evolution. For example, if a plant-soil feedback has an influence on plant success, the feedback must persist in the face of neighborhood influences. If the plant genetic neighborhood overwhelms the individual genetic effects on soils, a feedback is less likely to affect selection (Figure 1). We are aware of no studies that have utilized spatial models to estimate how plant genetic neighborhoods compare to individual tree genetic identities in predicting ecosystem function under individual focal trees; a classic evaluation of the effects of “neighborhood” vs. “self” on ecosystem function belowground. Such a comparison is important, because it is increasingly recognized that the genetically defined traits of a foundation or dominant tree species can affect ecosystem function (Fischer et al., 2014).
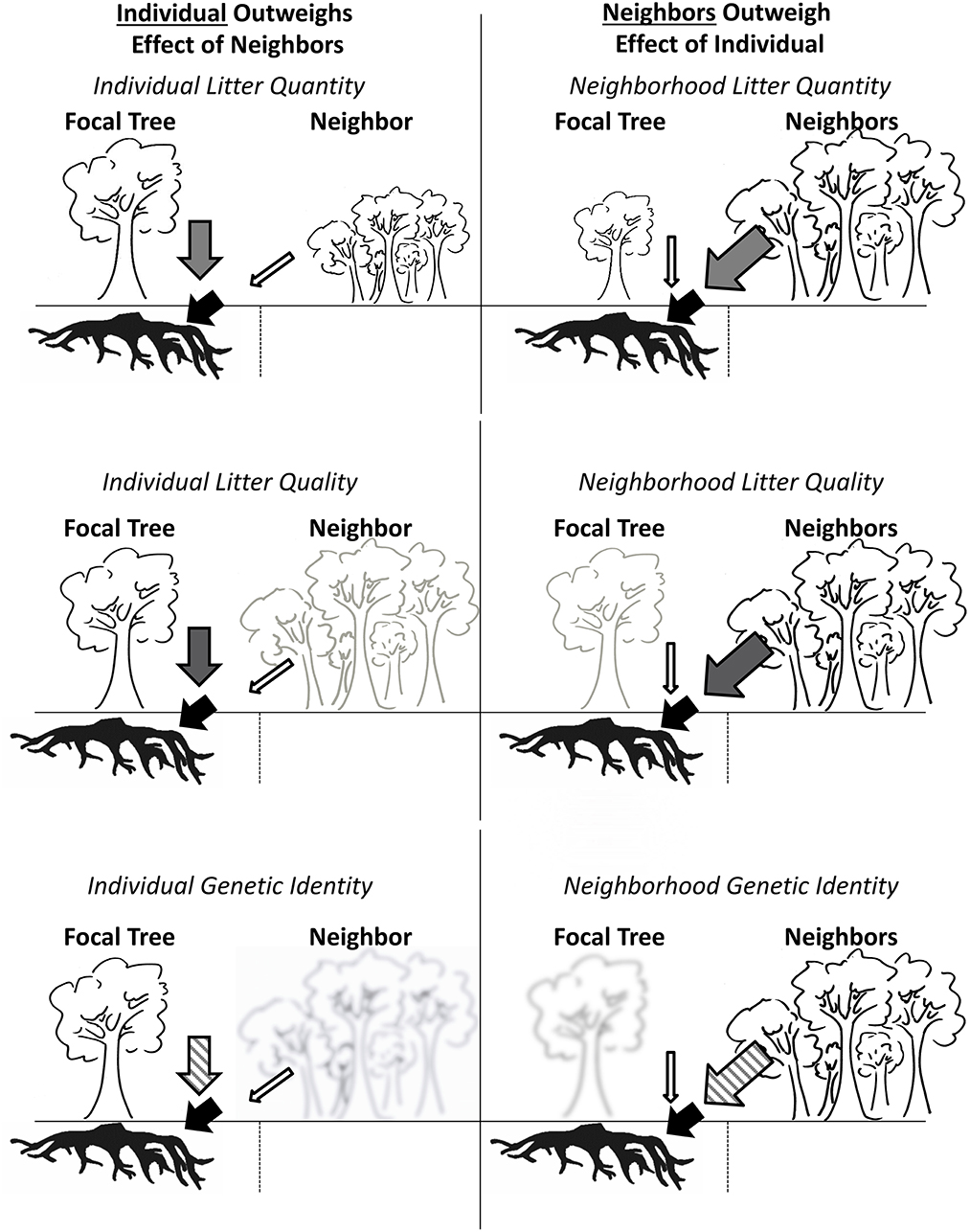
Figure 1. Conceptual diagram illustrating scenarios for plant-soil feedbacks in the context of genetically dissimilar neighbors that differ in quantity, quality, and identity of litter produced. Left: Influence of individual focal tree litter outweighs the effect of neighbor influence, resulting in a strong feedback to the focal tree. Right: Neighbor influence outweighs the effect of the individual focal tree resulting in a weak feedback from the focal tree, and lack of a detectable influence of the individual focal tree's on soils. Scenarios from top to bottom display differential effects in terms of litter quality (top; larger symbols represent larger leaf litter quantity), quality (middle; darker symbols represent leaf litter quality with a stronger effect on soils), and genetic identity (bottom; clearer icons and cross-hatching in arrows represents a stronger influence on soils based on genetic identity or dissimilarity with focal tree).
Based on the above logic, we address the central hypothesis that the genetic and phenotypic neighborhood has a significant impact on fine root dynamics through leaf-litter input to soils. Mechanistically, if belowground responses are more sensitive to neighbor effects than a given focal tree, sensitivity could vary depending on neighbor foliar mass inputs, neighbor foliar quality, neighbor genetic identity, and neighbor dissimilarity to the focal tree (e.g., Binkley and Giardina, 1998; Reynolds et al., 2018; Figure 1). Variable foliar mass inputs could alter the soil environment with implications for fine root dynamics even with no differences in leaf litter chemistry. Similarly, differential quality of aboveground inputs could alter fine root dynamics through effects on microbial communities, litter decomposition and nutrient mineralization (Northup et al., 1998; Smolander et al., 2012; LeRoy and Fischer, 2019). Genetic identity of neighbors and the traits they express may influence fine root dynamics through effects on leaf traits that affect the soil environment beyond the immediate footprint of individual trees. Focal trees may respond to closely related neighbors with similar traits differently than to more distantly related neighbors expressing different traits via facilitative (Maina et al., 2002) and competitive belowground interactions (Cahill et al., 2010). These responses could be manifested via aboveground volatile organic signaling in plants, aboveground light cues, or more complex belowground signaling processes (Baldwin et al., 2006; Fang et al., 2013; Gundel et al., 2014; Semchenko et al., 2014). Above- and belowground mechanisms are of course not mutually exclusive, especially because more genetically similar individuals are known to exhibit similar foliar traits (Bangert et al., 2006; Barbour et al., 2009; Compson et al., 2016).
Here, we use a new approach to compare models predicting the trait-based and genetic influence of tree neighborhoods relative to the traits of individual focal trees in an experimental common garden experimental forest in Northern Utah. Previously, we have identified correlations between individual tree genetics, foliar CTs, and fine root production—a pattern indicating a potential feedback effect where high foliar CTs are associated with low litter decomposability, low N-availability (Schweitzer et al., 2004; Fischer et al., 2010) and high fine root growth in trees (Fischer et al., 2006). We focus specifically on foliar CTs because they have been well-studied for their potential influences on soil nutrient status (Smolander et al., 2012), and their inputs can be readily predicted based on tree genetic identify and mass in this system (Schweitzer et al., 2004). We assess pattern in fine root growth, and turnover across genetic hybridization gradients associated with riparian forest trees (Populus spp. and naturally occurring hybrids) in comparison to modeled neighbor foliar input effects. Specifically, we divide our investigation into the comparison of the effects of individual genetic identity vs. effects of modeled contributions by neighbors in: (1) foliar quantity (litter mass); (2) foliar quality (foliar CTs); (3) genetic identity of neighbors; and (4) genetic dissimilarity of the tree neighborhood. We provide an analysis of each effect to determine comparative strength in the prediction of fine root production, turnover, and fine root production relative to aboveground tree mass (RFRP).
Materials and Methods
We utilized a common garden (Figure 2) consisting of natural genotypes of Populus fremontii, Populus angustifolia, and their genetically intermediate hybrids from along the Weber River, Utah (elev. 1,370 m; 41°11′N, 111°56′W). Because these species widely hybridize, producing offspring that successfully cross with the parent species, the intra- and inter-specific trait variation in leaf litter in this hybrid complex is high and perhaps similar to that observed across different plant genera. For example, foliar CTs expressed a dry leaf litter weight among individual tree genotypes range from near 0 to 33% (Schweitzer et al., 2004). The degree of hybridization and introgression within the complex has been previously characterized using the fraction of 35 species-specific restriction fragment length polymorphism (RFLP) genetic markers of P. fremontii found in each tree (Martinsen et al., 2001), allowing analysis of trees along a gradient of genetic relatedness. Here, we additionally use a metric of molecular dissimilarity between trees we define as the squared difference in the shared 35 species-specific RFLP markers between any two genotypes. The garden consists of 199 trees from 70 naturally occurring genotypes. Each genotype has been clonally replicated two or more times and randomly planted in the common garden (199 total clones). The genotypes represent parental species, F1 hybrids, and backcross hybrids between the parent species. All trees were randomly planted in 1991 on four-meter centers. The common garden is located at the low-elevation end of the 13 km hybrid zone along the Weber and Ogden Rivers. The soil at the common garden is classified as an Entisol USDA Soil Taxonomic order, composed of ~60% sand, ~30% silt, and ~10% clay. The site is a flattened former-field with little to no edaphic microtopography. Annual precipitation is approximately 440 mm.
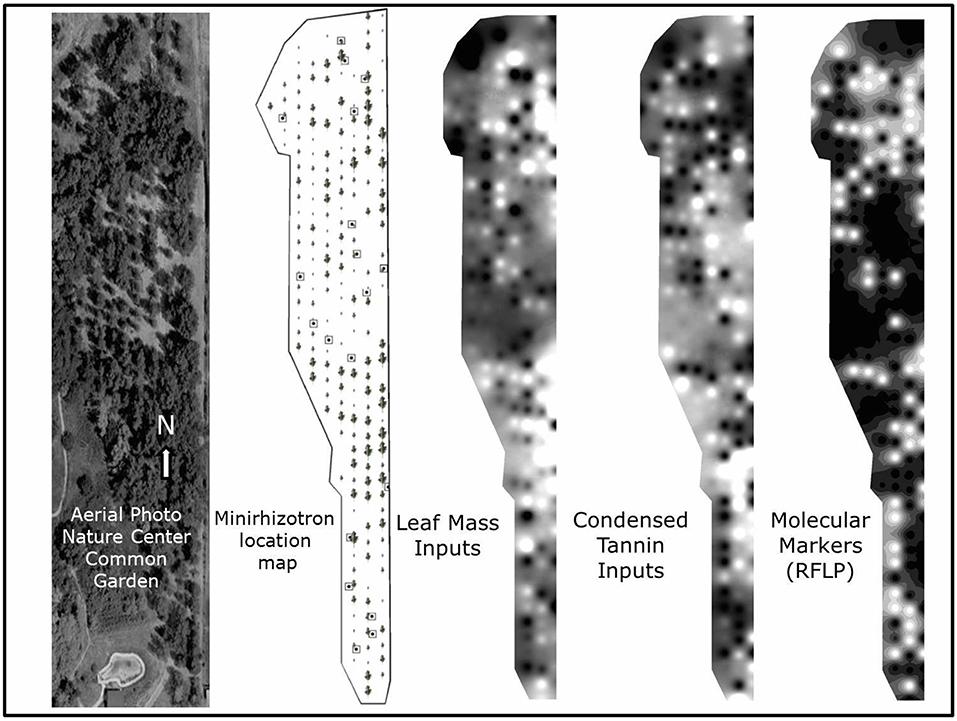
Figure 2. Aerial photo of study site (June 17, 2010) followed by a minirhizotron site map (with tree symbols scaled to reflect relative tree size), representative visualizations of leaf mass and foliar condensed tannin phenotype neighborhoods, and molecular marker genetic neighborhoods within the study site. Minirhizotron measurement locations are shown on the minirhizotron location map as squares with a single dot. The visualizations were created using kriging interpolation techniques in ARC GIS 10 based on values from all individual trees. For leaf mass inputs, light areas represent higher leaf mass inputs to soil, for foliar condensed tannins, lighter areas represent higher foliar condensed tannin inputs, and lighter areas on the RFLP marker map represent higher influence of trees with a higher proportion of Populus fremontii RFLP molecular markers.
We measured fine root dynamics using minirhizotrons under the canopies of 19 trees including P. angustofolia (3), P. fremontii (4), and hybrids (12). While our sample size was relatively small, our work takes place in a standardized experimental environment (a common garden), and our previous research (Fischer et al., 2006) has demonstrated that a sample size this small is large enough to detect meaningful patterns in root growth of our study trees when compared across a continuous gradient in genetic dissimilarity. In our study, we see our analysis as an opportunity to advance spatial methods for estimating the influence of neighbors on belowground processes (see below).
Methods for minirhizotron installation and placement were previously described in Fischer et al. (2006). Briefly, minirhizotron tubes (one per tree; 6.35 cm inner diameter and 1 m long) were placed in the soil, 1 m from the base of each tree in May, 2002, at an angle of −36° to the horizontal, to a depth of 55 cm, at randomized aspects with regard to the tree bases. Emergent portions of tubes were covered with black, followed by white paint to reduce light penetration. Foam padding was placed inside tubes to insulate them, and they were then covered with tin to reduce light and heat from solar radiation.
In order to isolate minirhizotron tube observations from belowground influences of neighbors, we used a partial trenching technique. Trenches (30 cm depth) were placed two meters from the base of the tree where each minirhizotron was placed, double-lined with 0.15 mm thick polyethylene plastic and back-filled. The 2 m distance represented the intermediate distance between trees based on 4 m spacing of trees in the garden. Previous work mapping root distributions with depth (Fischer et al., 2007) has demonstrated that the depth of 30 cm is sufficient to capture the majority of fine root activity in our study system. Trenching treatments could potentially influence root growth through releasing root competition with neighbors, or disturbance related effects on soils. While this potential existed for all trees in this study, Observations in previous studies suggested that patterns of root growth in the trenched common garden were similar to patterns in adjacent un-trenched field sites with the same genotypes (Fischer et al., 2006, 2007; also see below).
Minirhizotron image collection was also previously described in Fischer et al. (2006). Every 2 weeks from June 30th 2002 to November 18th 2002 images were collected using a CI-600 root scanner (CID, Inc., Camas, WA, USA) in order to measure root activity during peak of the growing season and the following fall (during and after leaf senescence). Three sequential image depths were measured at 0–12, 12–24, and 24–36 cm and averaged for all analyses. All images were analyzed using RooTracker imaging software (Duke University, Raleigh, NC, USA). Total root length was measured for all roots <2 mm diameter, and roots were counted as disappeared after they failed to appear for two consecutive images. Measurements within the same year as minirhizotron tube installation may not always represent the equilibrium ecosystem fine root production values in undisturbed ecosystems (Joslin and Wolfe, 1999). In disturbance-prone ecosystems like cottonwood floodplains though (Friedman and Lee, 2002), root growth is likely rapid and recovery from disturbance is common. Many minrihizotron studies have examined patterns in fine root dynamics in the same year as tube installation (Johnson et al., 2001). Additionally, comparisons of root growth among trees are unlikely to be biased, even if absolute values for root growth differ from non-disturbance conditions (Fischer et al., 2006).
In order to provide an estimate of amount of fine root production relative to tree biomass we estimate relative fine root production (hereafter, RFRP), by dividing estimates of fine root production by tree biomass. We used a locally derived biomass estimation equation,
as described in Fischer et al. (2006) to determine aboveground tree mass (also see Fischer et al., 2004; Cox et al., 2005; Lojewski et al., 2009). In earlier studies we found similar allometric relationships for P. fremontii, P. angustifolia, and hybrid tree types (Fischer et al., 2004; Cox et al., 2005), justifying the use of a shared biomass estimation equation. In order to estimate foliar mass of all trees in the garden, we used an additional locally derived biomass estimation equation described in Lojewski et al. (2009).
To estimate CT contributions from each tree in the garden, we took advantage of a previous study where tannin concentration in leaf litter was predicted for our garden trees based on molecular marker identity of individual tree genotypes. Schweitzer et al. (2004) found predictability of litter CTs (in 2001–2002) based on proportion of the 35 RFLP markers described in Martinsen et al. (2001) in the same common garden as our current study. We used these data (presented in Schweitzer et al., 2004) to derive a predictive equation for litter tannin concentrations based on litter molecular identity. We then applied this equation to all trees in the garden in order to estimate CT concentration in leaf litter for each tree. Concentration values where then multiplied by leaf mass estimates to provide an estimate of total condensed tannin amounts in the litter of every individual tree in the garden. We note that these values result from the combined influences of separate estimates for tree foliar mass and CT based on tree genetics, and so are not directly analogous to either.
Model Parameterization and Statistical Analysis
In order to examine the potential influences of neighbors on any given location in our study, we identified the spatial position of each point where measurements were made relative to all neighbor trees. We then used a model to integrate traits and characters of all neighbor trees (i.e., leaf mass, foliar CT content, molecular marker composition, etc.). Models estimating the influence of each neighborhood trait/character could then be compared for their relative influence on belowground dynamics. We created a set of spatially dependent, summative log-normal models based on total leaf mass, CT content, and molecular marker identity of each tree. These were used to distribute estimated zones of influence across 2-D horizontal space for individual trees and then aggregated to account for co-occurrence. We used a model form commonly applied in similar studies examining seed dispersal or leaf shedding (Greene et al., 2004; Canham and Uriarte, 2006; Gómez-Aparicio and Canham, 2008):
where Yk represents the neighborhood impact of a factor for a given location integrated across all trees within a specified buffer distance, B represents the parameter of interest (e.g., litter mass, foliar tannins, etc.) expressed on an individual tree basis, d represents distance of each tree within a buffer to the point of interest, and X0 and Xb are model form constants determined on-site based on an independent validation data set (see below). For our study, we chose a buffer distance of 7 m to define the neighborhood. This distance was chosen so that all immediate neighbors of each tree would be accounted for in all directions, and up to two neighbors would be accounted for each given direction from the focal tree (Figure 2; number of neighbors range = 2–6, = 4, CV = 0.3). This neighborhood included all trees, besides the focal tree, whose aggregated inputs overlap with the location of interest based on this buffer distance. Actual contributions of leaf litter from each tree within the buffer were then dependent on both litter mass and distance from the location of interest (with model form predicting that more distant trees had less influence). For computation of both genetic and genetic dissimilarity of the neighborhood, we used an average across all trees within the 7 m buffer in place of the summation indicated in the above equation. Genetic identity was estimated based on the number of P. fremontii RFLP markers in each neighbor genotype. Genetic dissimilarity was calculated based on the squared difference in P. fremontii RFLP marker scores (0–35) among focal and neighborhood trees. For both of these metrics, the above equation (Equation 1) was simply divided by the number of trees in the 7 m buffer radius.
In order to determine the best model form, and fit the model constants (X0 and Xb) to observed values, we collected a separate parameterization data-set of actual leaf litter inputs at 20 random locations in the common garden. In fall, 2010, we collected leaf litter fall inputs on 20, 0.0625 m2 mesh squares, placed on the soil surface prior to leaf abscission. From among a systematically defined set of possible constant values, we varied the model constants X0 and Xb, compared the predicted leaf mass to actual leaf mass collected, and then selected the most predictive combination of constant values. Briefly, the constant X0 reflects the mode of the litter dispersal (i.e., the distance that receives the most litter from each source tree—generally close to the base of the tree), and Xb reflects the spread of dispersal (where lower values reflect a steeper decline in litter contributions with distance from a source tree). Based on work evaluating leaf shedding in two other tree species (Gómez-Aparicio and Canham, 2008), we systematically varied the mode between values of 0.01 and 20 (0.01, 0.25, 0.5, 0.75, 0.8, 0.85, 0.9, 0.95, 1, 1.5, 2, 2.5, 3–10, 15, 20), and systematically varied the spread between 0.1 and 20 (0.1, 0.25, 0.5, 0.75, 1, 1.5, 2, 2.5, 3–10). We chose shorter increments near values of 1 based on observed litter depth values where leaf litter has a tendency to cluster near the base of the tree at our study site (data not shown), and also included models with extremely low and high modes and spreads. Accordingly, this range of models vary in their estimation of how leaf litter travels from the base of the tree to the ground surface where high value modes result in peak leaf litter dispersal distant from the tree, and high value spreads result in more even dispersal over a greater distance away from the tree (Figure 3).
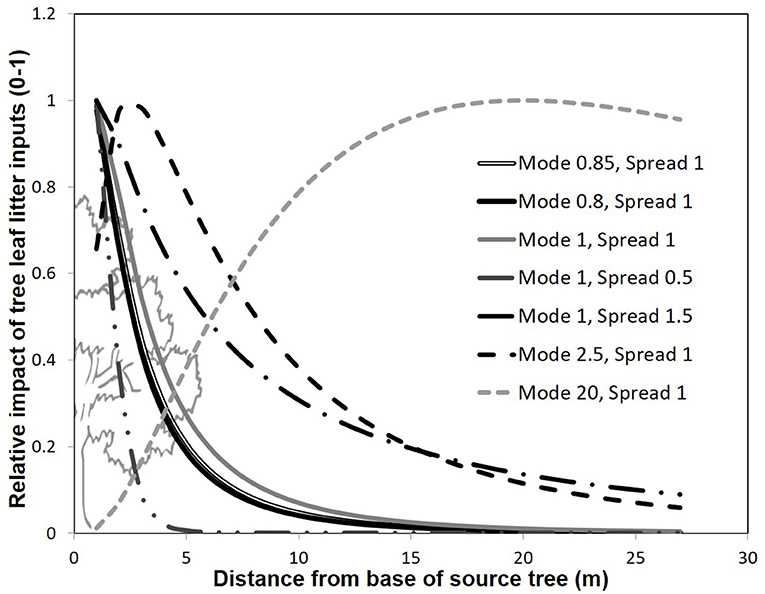
Figure 3. Representation of differing model forms for modeling tree foliar contributions to soils with distance. The y-axis denotes a scalar quantifying the contribution of a given tree's leaf litter inputs to soils, where 1 represents the maximum contribution of leaf litter from a given tree. Different models are represented by different constants (Xo and Xb) reflecting the mode and spread of leaf litter deposition with distance from a focal tree.
To identify a “best” combination of model and spread (constant) values, we used an information-theoretic approach to model selection and inference (Burnham and Anderson, 2002). This approach is widely used to rank and compare models, and is generally considered incompatible with parametric regression (Anderson, 2008). Candidate sets of models, each with systematically varying values assigned to the constants, were evaluated using Akaike's Information Criterion, adjusted for small sample size (AICc; Burnham and Anderson, 2002; see also below). Specifically, we used AICc difference values (ΔAICc), and AICc weights (wi) to simultaneously compare and rank multiple models from our set of candidate models (Burnham and Anderson, 2002). Although we considered any candidate model with a ΔAICc value ≥4.0 of an intercept-only model to be a good approximation of the data (see Anderson, 2008), we used the constant parameter values specified in best model (lowest ΔAICc) to model the response variables described below. All model parameters were estimated using maximum likelihood and a multiple linear regression analysis procedure in SAS JMP version 4.0.4 (SAS institute, Cary, N.C. USA).
Our best models in our independent model parameterization set had values of X0 and Xb near 1. The best model had an X0 value of 0.85, an Xb value of 1, but carried only 13.8% of all the weight presented by all other models (Table 1). Nevertheless, the first 7 models carried 91.5% of the weights in the candidate set, and all these models had X0 and Xb near 1. All other parameter combinations assumed a curve that was less steep (litter mass remained high with distance from the tree), or with an unrealistically distant maximum leaf litter mass (see Figure 3). We selected the best model in the candidate set (Mode 0.85, Spread = 1), and then used this model form in phenotypic neighborhood models for estimated leaf litter inputs (litter quantity), estimated CT inputs (litter quality), molecular marker composition (genetic identify of neighbors), and molecular dissimilarity of tree neighborhood.
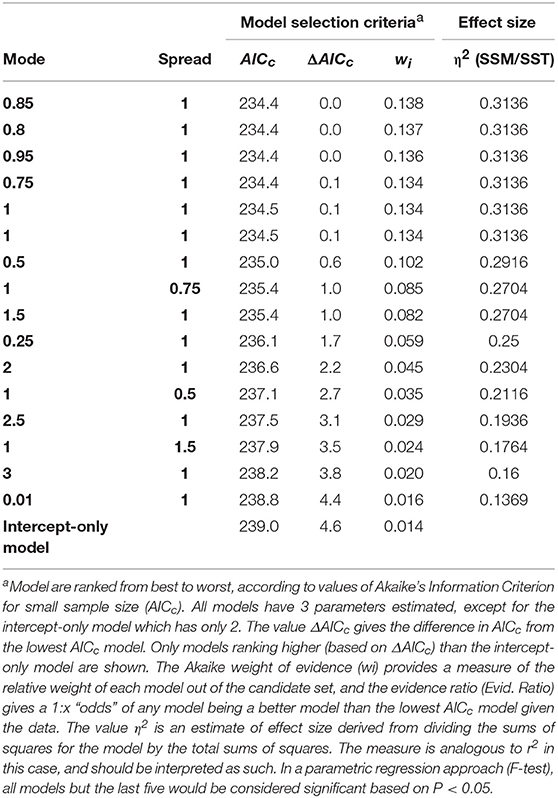
Table 1. Selection criteriaa (Italic) for models with systematically varying constant values (Bold) predicting litter fall mass per area at 20 randomly selected locations at the study site.
To compare candidate sets of neighborhood models that predicted fine root production, fine root turnover, or fine root production as a proportion of tree mass, we again used an information-theoretic approach to model selection and inference. Model parameters based on leaf mass (see above) were held constant for all models. For each response variable, candidate neighborhood models were simple linear models with the independent variables of: neighborhood leaf litter inputs, individual leaf litter inputs, neighborhood leaf CT inputs, individual leaf CT inputs, neighborhood molecular marker composition (based on RFLP), average neighborhood molecular marker dissimilarity to each overstory tree, individual tree molecular marker composition (based on RFLP), and an intercept only model (a null model). As above, we calculated AICc, ΔAICc, and wi to compare and rank multiple models from our set of a priori candidate models (Burnham and Anderson, 2002; Anderson, 2008). We considered models with ΔAICc values within ≤4.0 of the best model as those that best approximated the data (Anderson, 2008). As above, all results were estimated using maximum likelihood and a stepwise multiple linear regression analysis procedure in SAS JMP version 4.0.4 (SAS institute, Cary, N.C. USA). Finally, we calculated an estimate of effect size, η2, as the proportion of the total sums of squares divided by the model sums of squares.
Results
Fine Root Production
In support of our central hypothesis that plant genetic and phenotypic neighborhoods should affect ecosystem function, the best two models predicting fine root production were: (1) a model based on neighbor genetic dissimilarity, where fine root production increased as neighbors were on average genetically more dissimilar to the focal tree (as visualized in scatter plots shown in Figure 4A); and (2) a model where fine root production increased as neighbor foliar CT inputs to soils increased (as visualized in scatter plots shown in Figure 4B; Table 2). The model based on neighbor molecular genetic dissimilarity accounted for 60% of the weight in the candidate set, and the model based on neighbor leaf CT accounted for 30%. These two models ranked more highly than all other models, but where not clearly discernable from each other in their relative importance based on similar AICc values (i.e., ΔAICc values ≤4.0; Anderson, 2008). Even though neighbor genetic dissimilarity ranked highly in our candidate set, individual tree genetic identity and neighborhood genetic identity were less important. In other words, it was important how related neighborhood trees were to a focal tree, but specific genetic identity was less important. Individual tree P. fremontii RFLP markers (indicating the importance of individual identity over neighborhood) and the modeled RFLP molecular genetics based identity of the neighborhood (accounting for the importance of the neighborhood identity over the individual) accounted for 4 and 1% of the weight among all models, respectively. All other models in the candidate set predicting fine root production accounted for >1% of the model weights and are not reported.
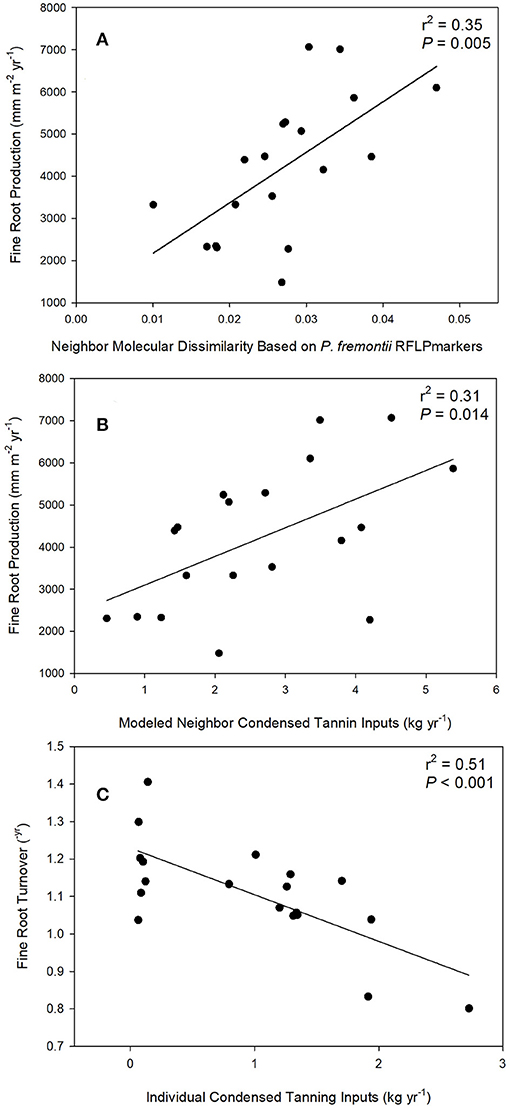
Figure 4. Linear regressions between fine root production and average neighbor dissimilarity (squared difference in RFLP molecular markers) (A), fine root production and neighborhood condensed tannin inputs (B), and individual foliar condensed tannin content and fine root turnover (C).
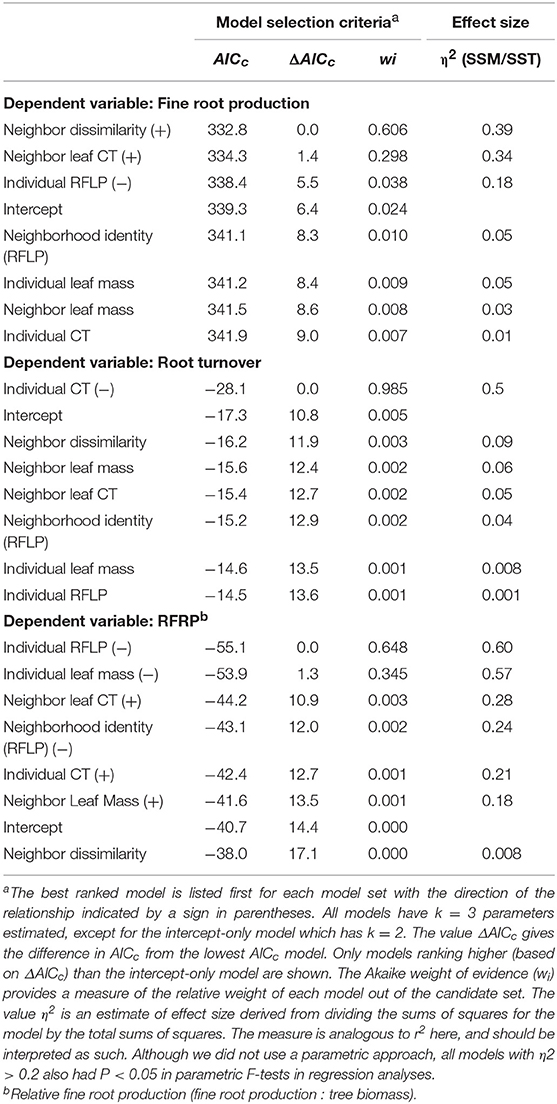
Table 2. Selection criteriaa (Italic) for distinct simple models predicting fine root production, fine root turnover, and fine root production: tree mass (the dependent variable for each suite of models is indicated in Bold).
Fine Root Turnover
We did not find support for overriding effects of the plant genetic and phenotypic neighborhoods on fine root turnover. Comparison of models for fine root turnover demonstrated that the data best supported a model where fine root turnover in the soil decreased with increasing individual foliar CT (Table 2; visualized as a scatter plot and simple linear regression in Figure 4C). This model was by far the best explanatory model for turnover as it was more than four ΔAICc points from the nearest model, and accounted for 99% of the weight of evidence in the candidate set. All other models were much less predictive of the data (Table 2). We note that fine root turnover was generally negatively (weakly) correlated with fine root production (Pearson's r = −0.21), and positively correlated with individual tree biomass (Pearson's r = −0.21).
Fine Root Production as a Function of Tree Mass
Individual aboveground tree biomass was negatively correlated with fine root production in our data (Pearson's r = −0.58), and fine root production in proportion to tree mass (RFRP) could provide interesting insight into variation root activity among trees that differ in mass aboveground. Nevertheless, we did not find support for overriding effects of the plant genetic and phenotypic neighborhoods on fine root production as a proportion of aboveground focal tree mass. Comparison of models for RFRP demonstrated that the data best supported two models. First, the individual RFLP genetic model was supported as the best predictor of RFRP (Table 2). This relationship was identical to the pattern described by Fischer et al. (2006) where RFRP increased in individuals more closely related to high-foliar-tannin genotypes of P. angustifolia. This model was only 1.3 ΔAICc points different than the next best model, Individual Leaf Mass. Nevertheless, because individual leaf mass and tree mass where both calculated from allometric equations based on tree diameter, leaf mass is auto-correlated with RFRP and so the predictive value of this measure is inherently limited. The Individual RFLP Molecular Genetics model accounted for 63% of the explanatory weight provided by the candidate set of models, and the Individual Leaf Mass model accounted for 35% of the weight provided by all models. All other models were much less predictive of the data (Table 2), and thus no neighborhood-based models strongly predicted RFRP.
Discussion
Relative Contributions of Focal Trees and Neighbors
In the study system we use, previous work has demonstrated that foliar tannins can reduce soil N availability via reduced leaf decomposition and complexation of N in the soil, and such effects are correlated with increased plant root growth, performance, and survival (Schweitzer et al., 2004; Fischer et al., 2006; Pregitzer et al., 2010). However, when the cumulative influence of neighbors is stronger than the individual, feedbacks could be decoupled (Figure 1). In this case, the individual may have some extended effects on the environment, but the individual's performance is more dependent on responses to neighbors, with implications for coevolution (Callaway, 2002; Ehlers and Thompson, 2004; Thompson, 2005).
In our data, neighborhood traits were associated with fine root production in soils, but individual focal tree traits and genetic identity were better associated with fine root turnover and RFRP in the soil, respectively. Thus, while neighborhood traits may play a role in determining carbon inputs belowground in the form of fine roots, individual traits were moderately better associated with how quickly fine root carbon turns over in soil. Fine root production relative to tree biomass also had higher fidelity to tree individuals. Our data of both turnover and RFRP have implications for reinforcing plant-soil feedbacks where individual phenotypes modify their environments despite the effects of neighbors. Below, we explore these findings in the context of previous work and plant-soil feedbacks. We note that all results should be interpreted in light of the limited sample size we used.
Genetic Similarity and the Phenotypic Neighborhood
Other recent work on neighborhood effects has suggested that belowground responses can be dependent on the dissimilarity of an individual with its neighbors (Maina et al., 2002; Cahill et al., 2010; Fang et al., 2013; Semchenko et al., 2014). For example, trees may compete more for belowground nutrient patches via over-proliferation of fine roots when in the presence of individuals who are less genetically related (e.g., Maina et al., 2002; Semchenko et al., 2014). The mechanisms for these effects are potentially diverse and could have both below- and above-ground components (Bais et al., 2004; Baldwin et al., 2006; Cahill et al., 2010; Fang et al., 2013; Gundel et al., 2014; Semchenko et al., 2014). In our study, where trenching reduced belowground interactions, fine root production increased as neighbors were more distantly related and as foliar CT inputs increased, suggesting potential integration of responses to both soil inputs and neighbor identity (sensu Cahill et al., 2010). The mechanism, though, is still unclear—how do plants detect genetic dissimilarity in litter inputs?
The presence of CT in neighboring tree litter may provide a clearer mechanism for belowground responses due to the known effects of CT on soils where CT binds N and reduces decomposition (Schweitzer et al., 2004). Foliar CT inputs in our common garden seem to be related to fine root dynamics at the ecosystem scale through both neighborhoods and individual genetics. Where foliar CT inputs of neighbors were high, fine root production by individual trees was high; and when CT of the individual focal tree was high, root turnover was low. For fine root production, the influence of neighbors was greater than the influence of the individual focal tree (but see Fischer et al., 2006), yet for fine root turnover the reverse was true. These data suggest that high CT neighborhoods could result in higher soil C inputs through increasing root production, and when individuals with high foliar CT are considered, higher fine root production may result in more C in the soil due to reduced turnover rates. Individual effects on turnover of the fine root C pool into soil C could potentially be related to fine root chemistry, but this is not clear from the broader literature. While correlations between turnover and foliar CT could simply reflect higher fine root CT in trees with high foliar CT, this correlation is not supported by recent work with aspen trees (Dettlaff et al., 2018). In a meta-analysis, Kaplan et al. (2008) found that constitutive and inducible defensive chemistry was generally similar for leaves and roots across 74 studies. However, induced defense chemistry might not be reflected in roots (Kaplan et al., 2008), and tannin content in roots may often be low in Populus (Stevens et al., 2014). In our study, individual C allocation to fine roots (proportional to aboveground mass) was associated with genotype of the individual tree (Fischer et al., 2006), but not individual predicted CT. Instead, after individual genetics, the next best model for fine root C allocation was neighborhood foliar CT. Overall, this pattern suggests that the genetics of individual trees could influence root growth and turnover in soils, but neighborhood foliar chemistry also plays a role with implications for plant-soil feedbacks (see Schweitzer et al., 2004; Fischer et al., 2006).
Of course, it is difficult to separate the myriad of real-world soils interactions that govern belowground processes, and neighborhood and individual feedbacks are clearly not mutually independent. Nevertheless, plant phenotypic neighborhoods (as defined above) may decouple feedbacks associated with individual tree genetics through modification of the soil environment. For example, even if individual litter quality has effects on individual tree performance in the soil (e.g., Kosola et al., 2004; Pregitzer et al., 2010; Smith et al., 2012), neighbor effects through litter inputs may overwhelm these effects on soil processes. Although RFRG may be partially genetically specific to a phenotype, neighborhood foliar inputs may increase fine root production at the ecosystem scale by altering soil conditions. Specifically, in N-limited systems, chemical contributions from neighbors including terpenes and phenolics (and not just CT) that alter the N-availability environment (Smolander et al., 2012) are thus likely to affect fine roots-consistent with the broad literature on this topic (Nadelhoffer et al., 1985; Yuan and Chen, 2012). In our limited study, foliar CT was an important variable moderately associated with both fine root production and turnover. Such findings may be consistent with key plant chemical traits driving patterns in fine root dynamics, regardless if patterns arise from the trait of an individual or a neighborhood of trees. Similar patterns should exist for other foliar traits known to effect ecosystem processes such as litter C:N, lignin content, Lignin:N, other defensive compounds, and foliar phosphorus (Yuan and Chen, 2012). Interactions among traits in the plant-soil matrix are likely to further complicate feedback effects. For each trait though, the relative balances of individual and neighborhood influences, and trait interactions, determine the plant-soil feedback potential of individual traits under genetic control.
Concluding Remarks
Modeling the spatial influence of neighbors is critical to understanding the relative influences of individual focal trees vs. neighbors on plant-soil feedbacks, and hence, the potential for these feedbacks to affect selection and plant evolution. Here, we present a spatially dependent approach that can help untangle the relative influence of neighbors vs. the genetics-based traits of focal trees on soil processes. In our small data set, it was apparent that different measures of belowground dynamics were related to spatial models of aboveground neighborhood litter inputs and the genetic-based traits of individual focal trees. The aboveground effects of neighbors on fine root processes may be measurable using this approach, which may provide a mechanism to model plant-soil feedbacks in a neighborhood genetic and evolutionary framework.
Data Availability Statement
The datasets generated for this study are available on request to the corresponding author.
Author Contributions
DF conceived, conducted field work, and conducted all analysis for this work. TW designed the broader common garden experiment where this work took place, contributed heavily to the experimental design, and contributed to all genetic data analysis and interpretations. SH contributed heavily to the original experimental design and field methods. BD advised on statistical techniques, modeling, and analysis. DF wrote the initial drafts of the manuscript. All authors contributed editorial comments and revisions to the manuscript.
Funding
This study was funded by NSF IRCEB, FIBR, and Macrosystems grants DEB-0078280, DEB-0425908, and DEB-1340852, BOR grants 04FC300039, CESU-06FC300025, and CESU-06FC300025, The Murdock Charitable Foundation, and sabbatical funding to DF from the Evergreen State College, WA, USA.
Conflict of Interest
The authors declare that the research was conducted in the absence of any commercial or financial relationships that could be construed as a potential conflict of interest.
Acknowledgments
We thank The Evergreen State College and the NAU School of Forestry for logistical and financial support. We also thank the Ogden Nature Center for partnering with our research in support of the common gardens. For extensive help in the laboratory and in the field, we thank A. Martin, N. Lojewski, D. Guido, G. Cox, P. Cox, R. Ivens, P. Townsend, J. Bailey, G. Wimp, A. Haden, A. Gitlin, D. Weber, and D. Baumley. Thomas Kolb, A. Martin, and C. Gehring provided important feedback on earlier drafts of this work. We also thank the members of the NAU Hart and Whitham laboratories, and the Evergreen Ecosystem Ecology laboratory, who also provided helpful comments on the ideas presented here.
References
Aerts, R. (1999). Interspecific competition in natural plant communities: mechanisms, trade-offs and plant-soil feedbacks. J. Exp. Bot. 50, 29–37. doi: 10.1093/jxb/50.330.29
Anderson, D. R. (2008). Model Based Inference in the Life Sciences: A Primer on Evidence. Berlin: Springer.
Bais, H. P., Park, S.-W., Weir, T. L., Callaway, R. M., and Vivanco, J. M. (2004). How plants communicate using the underground information superhighway. Trends Plant Sci. 9, 26–32. doi: 10.1016/j.tplants.2003.11.008
Baldwin, I. T., Halitschke, R., Paschold, A., Dahl, C. C. V., and Preston, C. A. (2006). Volatile signaling in plant-plant in the genomics era. Science 311, 812–815. doi: 10.1126/science.1118446
Bangert, R. K., Turek, R. J., Rehill, B., Allan, G. J., Wimp, G. M., Schweitzer, J. A., et al. (2006). A genetic similarity rule determines arthropod community structure. Mol. Ecol. 15, 1379–1392. doi: 10.1111/j.1365-294X.2005.02749.x
Barbour, R. C., O'Reilly-Wapstra, J. M., De Little, D. W., Jordan, G. J., Steane, D. A., Humphreys, J. R., et al. (2009). A geographic mosaic of genetic variation within a foundation tree species and its community-level consequences. Ecology 90, 1762–1772. doi: 10.1890/08-0951.1
Bardgett, R. D., and van der Putten, W. H. (2014). Belowground biodiversity and ecosystem functioning. Nature 515, 505–511. doi: 10.1038/nature13855
Bever, J. D., Dickie, I. A., Facelli, E., Facelli, J. M., Klironomos, J., Moora, M., et al. (2010). Rooting theories of plant community ecology in microbial interactions. Trends Ecol. Evol. 25, 468–478. doi: 10.1016/j.tree.2010.05.004
Binkley, D., and Giardina, C. (1998). Why do tree species affect soils? The warp and woof of tree-soil interactions. Biogeochemistry 42, 89–106. doi: 10.1023/A:1005948126251
Burnham, K. P., and Anderson, D. R. (2002). Model Selection and Multimodel Inference; a Practical Information-Theoretic Approach. New York, NY: Springer-Verlag.
Cahill, J. F. Jr., Mcnickle, G. G., Haag, J. J., Lamb, E. G., Nyanumba, S. M., Cassady, S. T., et al. (2010). Plants integrate information about nutrients and neighbors. Science 328:1657. doi: 10.1126/science.1189736
Callaway, R. M. (2002). The detection of neighbors by plants. Trends Ecol. Evol. 17, 104–105. doi: 10.1016/S0169-5347(01)02438-7
Canham, C. D., and Uriarte, M. (2006). Analysis of neighborhood dynamics of forest ecosystems using likelihood methods and modeling. Ecol. Appl. 16, 62–73. doi: 10.1890/04-0657
Casper, B. B., Schenk, H. J., and Jackson, R. B. (2003). Defining a plant's belowground zone of influence. Ecology 84, 2313–2321. doi: 10.1890/02-0287
Compson, Z. G., Hungate, B. A., Whitham, T. G., Meneses, N., Busby, P. E., Wojtowicz, T., et al. (2016). Plant genotype influences aquatic-terrestrial ecosystem linkages through timing and composition of insect emergence. Ecosphere 7:e01331. doi: 10.1002/ecs2.1331
Cox, G., Fischer, D. G., Hart, S. C., and Whitham, T. G. (2005). Nonresponse of native cottonwood trees to water additions during summer drought. West. N. Am. Nat. 65, 175–185.
Dettlaff, M. A., Marshall, V., Erbilgin, N., and Cahill, J. F. Jr. (2018). Root condensed tannins vary over time, but are unrelated to leaf tannins. AoB Plants 10:ply044. doi: 10.1093/aobpla/ply044
Ehlers, B. K., and Thompson, J. (2004). Do co-occurring plant species adapt to one another? The response of Bromus erectus to the presence of different Thymus vulgaris chemotypes. Oecologia 141, 511–518. doi: 10.1007/s00442-004-1663-7
Fang, S., Clark, R. T., Zheng, Y., Iyer-Pascuzzi, A. S., Weitz, J. S., Kochian, L. V., et al. (2013). Genotypic recognition and spatial responses by rice roots. Proc. Natl. Acad. Sci. U.S.A. 110, 2670–2675. doi: 10.1073/pnas.1222821110
Fischer, D. G., Chapman, S. K., Classen, A. T., Gehring, C. A., Grady, K. C., Schweitzer, J. A., et al. (2014). Plant genetic effects on soils under climate change. Plant Soil 379, 1–19. doi: 10.1007/s11104-013-1972-x
Fischer, D. G., Hart, S. C., LeRoy, C. J., and Whitham, T. G. (2007). Variation in belowground carbon fluxes along a Populus hybridization gradient. New Phytol. 176, 415–425. doi: 10.1111/j.1469-8137.2007.02167.x
Fischer, D. G., Hart, S. C., Rehill, B. J., Lindroth, R. L., Keim, P., and Whitham, T. G. (2006). Do high-tannin leaves require more roots? Oecologia 149, 668–675. doi: 10.1007/s00442-006-0471-7
Fischer, D. G., Hart, S. C., Schweitzer, J. A., Selmants, P. C., and Whitham, T. G. (2010). Soil nitrogen availability varies with plant genetics across diverse river drainages. Plant and Soil 331, 391–400. doi: 10.1007/s11104-009-0260-2
Fischer, D. G., Hart, S. C., Whitham, T. G., Martinsen, G. D., and Keim, P. (2004). Ecosystem implications of genetic variation in water-use of a dominant riparian tree. Oecologia 139, 288–297. doi: 10.1007/s00442-004-1505-7
Freschet, G. T., Aerts, R., and Cornelissen, J. H. C. (2012). Multiple mechanisms for trait effects on litter decomposition: moving beyond home-field advantage with a new hypothesis. J. Ecol. 100, 619–630. doi: 10.1111/j.1365-2745.2011.01943.x
Friedman, J. M., and Lee, V. J. (2002). Extreme floods, channel change, and riparian forests along ephemeral streams. Ecol. Monogr. 72, 409–425. doi: 10.1890/0012-9615(2002)072[0409:EFCCAR]2.0.CO;2
Gómez-Aparicio, L., and Canham, C. D. (2008). Neighborhood models of the effects of invasive tree species on ecosystem processes. Ecol. Monogr. 78, 69–86. doi: 10.1890/06-2036.1
Greene, D. F., Canham, C. D., Coates, K. D., and LePage, P. T. (2004). An evaluation of alternative dispersal functions for trees. J. Ecol. 92, 758–766. doi: 10.1111/j.0022-0477.2004.00921.x
Gundel, P. E., Pierik, R., Mommer, L., and Ballaré, C. L. (2014). Competing neighbors: light perception and root function. Oecologia 176, 1–10. doi: 10.1007/s00442-014-2983-x
Hobbie, S. E. (1992). Effects of plant species on nutrient cycling. Trends Ecol. Evol. 7, 336–339. doi: 10.1016/0169-5347(92)90126-V
Johnson, M. G., Tingey, D. T., Phillips, D. L., and Storm, M. J. (2001). Advancing fine root research with minirhizotrons. Environ. Exp. Bot. 45, 263–289. doi: 10.1016/S0098-8472(01)00077-6
Joslin, J. D., and Wolfe, M. H. (1999). Disturbance during minirhizotron installation can affect root observation data. Soil Sci. Soc. Am. J. 63, 218–221. doi: 10.2136/sssaj1999.03615995006300010031x
Kaplan, I., Halitschke, R., Kessler, A., Sardanelli, S., and Denno, R. F. (2008). Constitutive and induced defenses to herbivory in above- and belowground plant tissues. Ecology 89, 392–406. doi: 10.1890/07-0471.1
Kosola, K. R., Dickmann, D. I., Hall, R. B., and Workmaster, B. A. A. (2004). Cottonwood growth rate and fine root condensed tannin concentration. Tree Physiol. 24, 1063–1068. doi: 10.1093/treephys/24.9.1063
LeRoy, C. J., and Fischer, D. G. (2019). Do genetically-specific tree canopy environments feed back to affect genetically specific leaf decomposition rates? Plant Soil 437, 1–10. doi: 10.1007/s11104-019-03952-y
Litton, C. L., Raich, J. W., and Ryan, M. G. (2007). Carbon allocation in forest ecosystems. Global Change Biol. 13, 2089–2109. doi: 10.1111/j.1365-2486.2007.01420.x
Lojewski, N. R., Fischer, D. G., Bailey, J. K., Schweitzer, J. A., Whitham, T. G., and Hart, S. C. (2009). Genetic basis of aboveground productivity in two native Populus species and their hybrids. Tree Physiol. 29, 1133–1142. doi: 10.1093/treephys/tpp046
Madritch, M. D., Greene, S. L., and Lindroth, R. L. (2009). Genetic mosaics of ecosystem functioning across aspen-dominated landscapes. Oecologia 160, 119–127. doi: 10.1007/s00442-009-1283-3
Maina, G. G., Brown, J. S., and Gersani, M. (2002). Intra-plant versus inter-plant root competition in beans: avoidance, resource matching or tragedy of the commons. Plant Ecol. 160, 235–247. doi: 10.1023/A:1015822003011
Martinsen, G. D., Whitham, T. G., Turek, R. J., and Keim, P. (2001). Hybrid populations selectively filter gene introgression between species. Evolution 55, 1325–1335. doi: 10.1111/j.0014-3820.2001.tb00655.x
Nadelhoffer, N. J., Aber, J. D., and Melillo, J. M. (1985). Fine roots, net primary production, and soil nitrogen availability: a new hypothesis. Ecology 66, 1377–1390. doi: 10.2307/1939190
Norby, R., and Jackson, R. B. (2000). Root dynamics and global change: seeking an ecosystem perspective. New Phytol. 147, 3–12. doi: 10.1046/j.1469-8137.2000.00676.x
Northup, R. R., Dahlgren, R. A., and McColl, J. G. (1998). Polyphenols as regulators of plant-litter-soil interactions in northern California's pygmy forest: a positive feedback? Biogeochemistry 42, 189–220. doi: 10.1007/978-94-017-2691-7_10
Pregitzer, C. C., Bailey, J. K., Hart, S. C., and Schweitzer, J. A. (2010). Soils as agents of selection: feedbacks between plants and soils alter seedling survival and performance. Evol. Ecol. 24, 1045–1059. doi: 10.1007/s10682-010-9363-8
Reynolds, L., Lajtha, K., Bowden, R. D., Tfaily, M. M., Johnson, B. R., and Bridgham, S. (2018). The path from litter to soil: insights into soil C cycling from long-term input manipulation and high-resolution mass spectrometry. J. Geophys. Res. Biogeosci. 123, 1486–1497. doi: 10.1002/2017JG004076
Schweitzer, J. A., Bailey, J. K., Rehill, B. J., Hart, S. C., Lindroth, R. L., Keim, P., et al. (2004). Genetically based trait in dominant tree affects ecosystem processes. Ecol. Lett. 7, 127–134. doi: 10.1111/j.1461-0248.2003.00562.x
Schweitzer, J. A., Fischer, D. G., Rehill, B. J., Wooley, S. C., Woolbright, S. A., Lindroth, R. L., et al. (2011). Forest gene diversity is correlated with the composition and function of soil microbial communities. Popul. Ecol. 53, 35–46. doi: 10.1007/s10144-010-0252-3
Schweitzer, J. A., Madritch, M. D., Bailey, J. K., LeRoy, C. J., Fischer, D. G., Rehill, B. J., et al. (2008). From genes to ecosystems: the genetic basis of condensed tannins and their role in nutrient regulation in a Populus model system. Ecosystems 11, 1005–1020. doi: 10.1007/s10021-008-9173-9
Schweitzer, J. A., Madritch, M. D., Felker-Quinn, E., and Bailey, J. K. (2013). “From genes to ecosystems: plant genetics as a link between above-and belowground processes,” in Soil Ecol. Ecosystem Services, eds D. H. Wall, R. D. Bardgett, J. E. Herrick, and T. H. Jones (Oxford: Oxford University Press), 82–97.
Semchenko, M., Saar, S., and Lepik, A. (2014). Plant root exudates mediate neighbour recognition and trigger complex behavioural changes. New Phytol. 204, 631–637. doi: 10.1111/nph.12930
Smith, D. S., Schweitzer, J. A., Turk, P., Bailey, J. K., Hart, S. C., Shuster, S. M., et al. (2012). Soil-mediated local adaptation alters seedling survival and performance. Plant Soil 352, 243–251. doi: 10.1007/s11104-011-0992-7
Smolander, A., Kanerva, S., Adamczyk, B., and Kitunen, V. (2012). Nitrogen transformations in boreal forest soils—does composition of plant secondary compounds give any explanations? Plant Soil 350, 1–26. doi: 10.1007/s11104-011-0895-7
Stevens, M. T., Gusse, A. C., and Lindroth, R. L. (2014). Root chemistry in Populus tremuloides: effects of soil nutrients, defoliation, and genotype. J. Chem. Ecol. 40, 31–38. doi: 10.1007/s10886-013-0371-3
Thompson, J. N. (2005). The Geographic Mosaic of Coevolution. Chicago, IL: University of Chicago Press.
van de Voorde, T. F. J., van der Putten, W. H., and Bezemer, T. M. (2011). Intra- and interspecific plant-soil interactions, soil legacies and priority effects during old-field succession. J. Ecol. 99, 945–953. doi: 10.1111/j.1365-2745.2011.01815.x
Wardle, D. A., Bardgett, R. D., Klironomos, J. N., Setälä, H., der Putten, D. H., and Wall, D. H. (2004). Ecological linkages between aboveground and belowground biota. Science 304, 1629–1633. doi: 10.1126/science.1094875
Waring, B. G., Alvarez-Cansino, L., Barry, K., Becklund, K., Dale, S., Gei, M., et al. (2015). Pervasive and strong effects of plants on soil chemistry: a meta-analysis of individual plant ‘Zinke' effects. Proc. R. Soc. Lond. B Biol. Sci. 282:20151001. doi: 10.1098/rspb.2015.1001
Weber, P., Bol, R., Dixon, L., and Bardgett, R. D. (2008). Large old trees influence patterns of δ13C and δ 15N in forests. Rapid Commun. Mass Spectrom. 22, 1627–1630. doi: 10.1002/rcm.3433
Yuan, Z. Y., and Chen, H. Y. H. (2012). A global analysis of fine root production as affected by soil nitrogen and phosphorus. Proc. R. Soc. Lond. B Biol. Sci. 279, 3796–3802. doi: 10.1098/rspb.2012.0955
Keywords: ecosystem genetics, genes-to-ecosystems, root production, Populus, condensed tannins
Citation: Fischer DG, Dickson BG, Whitham TG and Hart SC (2019) Self-Similarity, Leaf Litter Traits, and Neighborhood Predicting Fine Root Dynamics in a Common-Garden Forest. Front. Environ. Sci. 7:142. doi: 10.3389/fenvs.2019.00142
Received: 08 March 2019; Accepted: 10 September 2019;
Published: 24 September 2019.
Edited by:
Dionisios Gasparatos, Aristotle University of Thessaloniki, GreeceReviewed by:
Yuntao Hu, Lawrence Berkeley National Laboratory, United StatesShalom D. Addo-Danso, Forest Research Institute of Ghana, Ghana
Copyright © 2019 Fischer, Dickson, Whitham and Hart. This is an open-access article distributed under the terms of the Creative Commons Attribution License (CC BY). The use, distribution or reproduction in other forums is permitted, provided the original author(s) and the copyright owner(s) are credited and that the original publication in this journal is cited, in accordance with accepted academic practice. No use, distribution or reproduction is permitted which does not comply with these terms.
*Correspondence: Dylan G. Fischer, fischerd@evergreen.edu