Organic Contaminant Mixture Significantly Changes Microbenthic Community Structure and Increases the Expression of PAH Degradation Genes
- 1Department of Ecology, Environment and Plant Sciences, Stockholm University, Stockholm, Sweden
- 2Department of Environmental Science, Stockholm University, Stockholm, Sweden
- 3NordCEE, Department of Biology, University of Southern Denmark, Odense, Denmark
- 4Department of Marine Sciences, University of Gothenburg, Gothenburg, Sweden
- 5Stockholm University Baltic Sea Centre, Stockholm University, Stockholm, Sweden
Studying the effects of chemical contaminants on the structure and function of microbial and meiofauna communities have traditionally focused on specific effects of single contaminants on single species. This has left the complex interactions between mixtures of contaminants and its non-specific toxicity effects on the functions and structure of sediment microbial communities mostly overlooked. In order to improve our insights on such questions, we performed an experiment where Baltic Sea sediments were spiked with an ecologically relevant mixture of seven organic contaminants below specific toxicity levels and used 16S and 18S rRNA metabarcoding from RNA extracts to monitor changes in active microbial and meiofauna diversity and community structure in the spiked treatment compared to controls. In addition, we investigated the effects of exposure to this contaminant mixture on potential nitrification rates and on the expression of key-genes in the microbial nitrification and PAH degradation pathways with qPCR. There were significant differences in both eukaryotic and microbial community structures in sediments spiked with a mixture of organic contaminants. Nematoda showed a significant increase in overall relative abundance to the added contaminants (5.5 ± 1.1% higher in spiked), particularly taxa of the genus Leptolaimus (increased from 10.2 ± 5.4% in the controls to 32.5 ± 10.2% in the spiked treatment). Conversely, a significant decrease in relative abundance from 18.2 ± 5.6% in control to 7 ± 3.4% in of the genus Paraplectana was also detected. Additionally, while the abundance of active PAH degraders was significantly higher in spiked sediments than in the controls, no significant effect of our organic mixture was found on nitrification rates or the expression of AmoA (bacterial ammonia oxidizer gene). Our data indicate that mixtures of organic contaminants can have significant effects on microbenthic community structure even when its individual components are present at concentrations below its specific toxicity. In addition, we suggest that eRNA-based metabarcoding can offer important insights in microbenthic community structure and activities, and further empathizes the potential of meiofauna as bio-indicators of chemical contamination in benthic ecosystems.
Introduction
The marine benthos is the second largest ecosystem on Earth, harboring a substantial diversity of macro- and microorganisms (Snelgrove, 1999; Harley et al., 2006). These communities are crucial to global biogeochemical cycles, mineralizing organic matter and nutrients and serving as major nitrogen and carbon sinks through sedimentation processes (Kraaij et al., 2002; Sundbäck et al., 2004; Porubsky et al., 2009). The resilience and functioning of an ecosystem rely on its biodiversity, and highly diverse systems increase productivity by providing ecological niches and the ability to withstand disturbances through functional redundancy (Slootweg et al., 2009; Cardinale et al., 2012). In addition, benthic fauna like meiofauna (invertebrates smaller than 0.04 mm), which reside within sediments, continuously rework their habitat’s structures, transport nutrients, and promote resource availability in sediment layers in which they are most active, through burrowing, particle resuspension, and bioturbation (Bonaglia et al., 2014b; Zeppilli et al., 2015; Schratzberger and Ingels, 2018). Meiofauna are highly diverse and abundant microscopic benthic microeukaryotes and are regarded as crucial players in sediment ecosystems (Sheppard, 2006; Nascimento et al., 2012; Bonaglia et al., 2014b; Schratzberger and Ingels, 2018). They play a key role in food webs on nearly all trophic levels and are highly responsive to environmental disturbances (Giere, 2009; Bik et al., 2012).
The human impact on coastal benthic ecosystems has been significant for several decades and as a result, coastal benthic communities are often exposed to organic contaminants. Many organic contaminants are hydrophobic and thus have a high affinity to particulate organic matter, which can accelerate their vertical transport to the sediment (Nizzetto et al., 2010; Zhang et al., 2015). Therefore, organic contaminants such as polycyclic aromatic hydrocarbons (PAHs) and polychlorinated biphenyls (PCBs) can accumulate in marine sediments, leading to the exposure of sediment communities to complex mixtures of organic contaminants. Due to their hydrophobic nature, PAHs and PCBs are bioaccumulative (ECHA, 2017). They are often associated with higher mortality, lower reproduction (Lotufo, 1998) and feeding rates (Silva et al., 2009) of benthic populations and have been shown to interfere with oxygen and nutrient dynamics (Carman and Todaro, 1996; Fleeger et al., 2003; Quero et al., 2015). Acute exposure to high concentrations of PAHs can change the composition and ecological functions of benthic communities. For instance, Edlund and Jansson (2006) reported a rise in Gammaproteobacteria and Flavobacteria following bioremediation of polluted Baltic Sea sediments and Lindgren et al. (2012) found certain nematode taxa to decreased in abundance, in response to the addition of diesel, while conversely Foraminifera increased in relative abundance. Additionally, Lindgren et al. (2014) show that exposure of marine sediments to organic contaminants impacts not only meiofauna and bacterial communities, but also important ecological processes related to benthic nitrogen cycling. These authors found sediment-to-water fluxes of nitrite and nitrate, and potential nitrification and denitrification to be reduced in sediment exposed to PAHs.
Biological communities in the environment are rarely exposed to a single, isolated compound, instead they are exposed to complex mixtures of contaminants (Mustajärvi et al., 2017b; Dulio et al., 2018; Jahnke et al., 2018). Our understanding of the effects of such mixtures on benthos is limited, particularly regarding higher levels of biological organization. This poses a significant obstacle for risk assessment of chemical contaminants, as there is evidence of negative effects of mixtures even if each of its components is present below a no-effect concentration (Silva et al., 2002; Kortenkamp et al., 2009). In general, concentrations of different chemicals cannot be added up to derive the overall toxicity of the mixture, because the relationship between toxicity and concentration is different for every chemical. Concentrations of single chemicals are often below what would cause compound specific toxicity (Inostroza et al., 2017). However, chemicals occur in the environment in complex mixtures, and mixtures can have a non-specific toxicity (Escher et al., 2002; Inostroza et al., 2017). Non-specific toxicity is induced by partitioning of contaminants to cell membranes, causing disturbance of the membrane. It is governed by the chemical’s hydrophobicity, is additive and correlates to chemical activity (Mackay et al., 2011; Gobas et al., 2018). Chemical activity is a thermodynamic concept that relates the concentration (C; mol L–1) of chemical i to its maximum solubility (S; mol L–1) in the same environmental media (e.g., sediment porewater) (Schwarzenbach et al., 2002). Chemical activity can thus be used to quantify the total load and potency of environmental mixtures. In the case of non-specific toxicity, it is the chemical activity of the mixture that matters, and not the composition of the mixture (Mayer and Reichenberg, 2006; Mackay et al., 2009). However, how chemical activity as a proxy for non-specific toxicity, affects benthic community function and structure is not well understood, particularly regarding microbial and meiofauna communities. As previous work has demonstrated a baseline toxicity at the chemical activities level of 0.01 to 0.1 (Smith et al., 2013), we aimed for a total chemical activity of the contaminant mixture to be within that range.
Here we investigated the non-specific toxicity of a chemical mixture containing hexachlorobenzene (HCB), biphenyl, three PCB congeners (PCB52, PCB101, PCB153) and two PAHs (pyrene and phenanthrene), on the composition of pro-and eukaryotic microbial and meiofaunal communities. For this, we utilized a metabarcoding approach and sequenced both the 16S and 18S regions of the ribosomal RNA to capture the diversity of benthic prokaryotes and eukaryotes, respectively. As extracellular DNA is generally stable and might persist in the environment (Corinaldesi et al., 2008), we targeted RNA to better target the active diversity. In addition, we used RT-qPCR to quantify the expression of the microbial ammonia monooxygenase (AmoA) and PAH-ring hydroxylating dioxygenase (PAH-RHD) genes (Cébron et al., 2008) and determined potential nitrification rates by oxic slurry incubations (Bonaglia et al., 2014a). In brief, we hypothesized the following: i) the structure of benthic microbial and meiofauna communities is significantly changed in sediments containing a complex mixture of organic contaminants at non-specific toxicity levels; ii) exposure to this mixture of organic contaminants in sediments will significantly decrease the expression of microbial AmoA genes and nitrification rates, and iii) the expression of PAH-RHDα genes to significantly increase in contaminated sediments.
Materials and Methods
Sediment and Animal Collection
The sediment and animals used in this experiment were collected at a depth of 37 m from the Skvallran pristine sampling station (58°47′25.0″N 17°43′42.2″E) near the Stockholm University Marine Field Station on the island of Askö, in the Northwestern Baltic Sea proper (Figure 1) using a benthic sled during the late spring (May 2nd) of 2017.
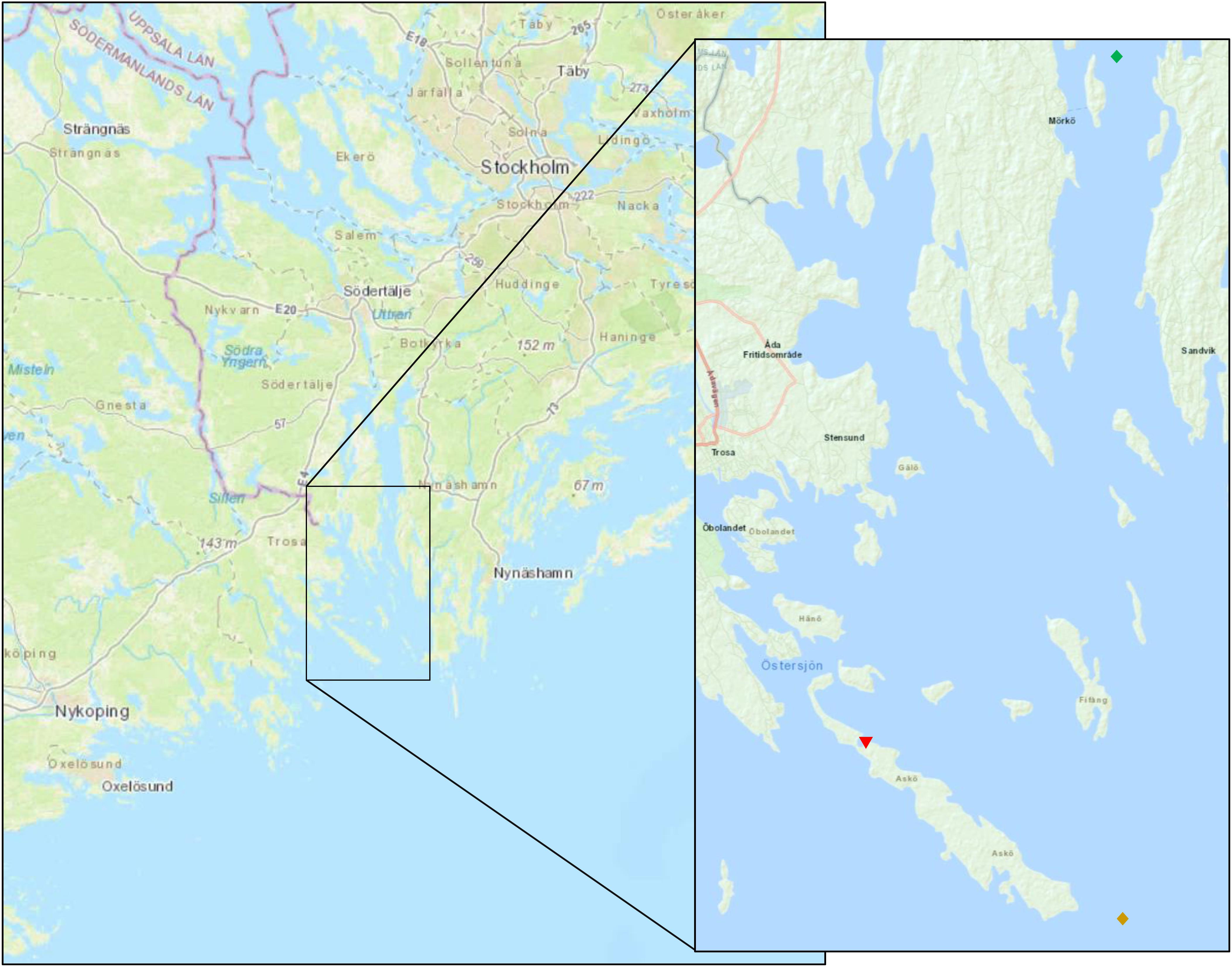
Figure 1. Sampling location of pristine sediment (brown diamond) near the Askö laboratory (red inverted triangle), South-West at the border of Stockholm County. Amphipods and diatoms were collected at station H4 (green diamond). Map was adjusted from ÅN Austin (2019) and OpenSeaMap (2019).
Sediment Spiking and Chemical Analysis
Sediment was spiked with the chemical mixture (Table 1) by vertically rolling the sediment in 1 L amber glass spiking jars (Ditsworth et al., 1990). Spiking jars were prepared with chemicals by adding stock solutions of 1 mg mL–1 HCB in aceton:hexane 1:3 v/v to the empty jars, followed by a mixture of biphenyl 0.5 mg mL–1, PAHs 0.5 mg mL–1 and PCBs 0.3 mg mL–1 in acetone:dimethyl sulfoxide (DMSO) 10:1 v/v, after which the jars were left on a roller without lids to allow the solvent to evaporate. DMSO (1 mg kg–1 sediment fresh weight) was added as a keeper to the stock solution to retain the more volatile compounds. The control jars were treated similarly by adding equivalent amounts of solvents and DMSO to those used for chemical spiking. Wet sediment was then added (800 g/jar) and the jars were covered with aluminum foil, sealed with a lid, and rolled directly for 20 min. The sediment was allowed to equilibrate with the chemical mixtures sorbed to the inner glass walls of the jars during 20 days at 5°C. Due to a limited space on the roller mixer, jars were rolled in three batches and were cycled equally between the roller and a vertical shaking table every 24 h. Jars were opened daily to avoid gas building up. After 20 days, the 800 g sub-batches of sediment were combined (total of 5.6 kg spiked and control sediment) and sediment samples were collected for chemical analysis.
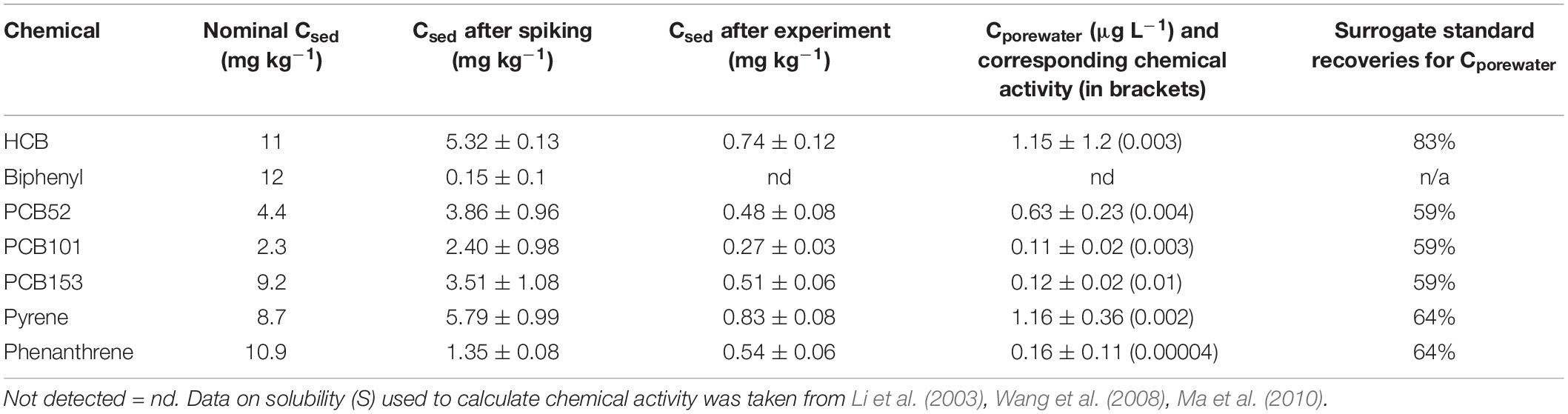
Table 1. Chemical mixture spiked to the sediment and analyzed sediment concentrations (Csed) before spiking and after the experiment (mg kg–1 sediment dry weight, average ± standard deviation, n = 3), as well concentrations in sediment porewater (Cporewater) and chemical activity.
Concentrations of the chemical mixture components were measured both in total sediment (Csed) and in sediment porewater (Cporewater). Extraction of sediment was performed with accelerated solvent extraction (ASE, Dionex 200), with acetone:hexane (1:1 v/v) using two extraction cycles per sample and the instrument was set-up according to Josefsson et al. (2006). Dry sediment samples (0.1 g, n = 3) were weighed to the extractions cells (34 mL), internal surrogate standards were added (PCB3, pyreneD10, phenanthreneD10, HCBC13), and the cells were filled with ISOLUTE® HM-N resin (furnished 450°C overnight prior to use). Method blanks were included, containing ISOLUTE® HM-N resin only. Sample extracts were then treated with activated Cu powder for sulfur removal. Due to the high expected concentrations in the extracts, the samples were diluted 1:20 with hexane before the second batch of surrogate standards were added (PCB52C13, PCB101C13, and PCB153C13). Dimethyl formamide (DMF) was used for sample cleanup, followed by 1 cm ø silica columns (10% deactivated). The cleanup method is described in detail by Mustajärvi et al. (2017a). Following clean-up, the samples were reduced in volume (200 μL), and internal recovery standards (PCB53 and aldrin) were added prior to GCMS analysis.
Porewater concentrations (Cporewater) were measured in sediment by using equilibrium passive sampling. Sediment samples for Cporewater analysis were collected just before the start of the experiment and frozen until analysis. Passive equilibrium sampling was carried out by using glass jars coated internally with a 1, 3, or 5 μm silicone layer (DC1-2577- silicone; Dow Corning®) (Reichenberg et al., 2008). Approximately 10 g of wet sediment was added to the coated 20 ml glass jars. The glass jars were rolled horizontally for two weeks in room temperature in darkness, to allow the analytes to equilibrate between the sediment and the silicone. The sediment was then discarded, and the inside part of the glass vials was rinsed with cold tap water and dried carefully with a lint-free tissue. For extraction of the silicone, 2 ml acetone:hexane (1:1, v:v) was added to the glass jars together with IS surrogate standards (CB3, pyreneD10, phenanthreneD10, HCBC13). The glass jars were rolled for 30 min and solvent was collected. The extraction of the silicone was repeated for a second time and the two extracts were pooled. The cleanup of the extracts followed the same procedure as described for the sediment samples. After cleanup all samples were reduced in volume (200 μl) and recovery standards (PCB53 and Aldrin) were added prior the gas chromatography mass spectrometry (GCMS) analysis.
Chemical concentrations in silicone (Cpol) at equilibrium were converted to porewater concentrations by using polymer-water partition coefficients (Kpol/w):
Kpol/w from Gilbert et al. (2016) for PCBs and HCB, Kpol/w for phenanthrene and pyrene were extrapolated by plotting published Kpol/w (Gilbert et al., 2016) for PCBs against octanol-water coefficients (Kow) from Hawker and Connell (1988).
Limits of detections (LOD) were calculated from the method blanks (average plus three times standard deviation), and all samples were blank corrected. Average blank levels for Cporewater were 0.61 ng for PCBs, 0.90 for HCB and 0.21 ng for PAHs. Average blank levels for sediment samples were 0.40 to 0.99 ng for PCBs, 0.54 ng for HCB, and 1.22 ng and 2.45 ng for phenanthrene and pyrene, respectively. Surrogate standard recoveries for Cporewater were 59% PCBs, 83% HCB and 64% PAHs. For sediment samples the recoveries were 39% to 60 % for PCBs, 39% for HCB, and 64% and 74% for phenanthrene and pyrene, respectively.
Microcosm Experiment
The experiment consisted of untreated sediments (control) and sediments spiked with chemical mixture (n = 5) (spiked). Microcosms were prepared in 500 ml glass beakers with an inner diameter of 8 cm, by adding ∼100 g of spiked or control sediment per replicate to create a 56 cm2 layer of sediment ∼3 cm high. Microcosm jars were then filled up to the 400 mL marker with filtered brackish water collected at the Skvallran pristine sampling station (Figure 1). Meiofauna were isolated from an equivalent volume of additional pristine sediment following the same method described in Nascimento et al. (2012), and added to each microcosm. To increase ecological relevance, 5 individuals of the amphipod Monoporeia affinis were added per microcosm. The collection of meiofauna, Monoporeia and diatoms are described in more detail in the Supplementary Material section.
Following the addition of both meiofauna and macrofauna individuals, each replicate received an input of food by adding 0.2 mg cm2 of diatom bloom material (dominated by Thalassiosira sp.) with a pipette tip to the sediment surface (evenly spread over the entire surface area). Microcosms were supplied with gentle air bubbling and the experiment was started. It ran for a total of 5 weeks in a dark controlled climate room at 5°C. To assess microbial community structure, PAH-RHDα and AmoA gene expression over time, a total of 2 g of sediment from each experimental unit was sampled with a micro-spoon at 1, 3, and 5 weeks after the initial microcosm setup. Subsamples were taken from multiple random spots of each replicate and pooled into a 2 mL Eppendorf, thoroughly homogenized, and stored at −80°C for later RNA extraction, qPCR, and 16S and 18S rRNA metabarcoding.
After 5 weeks, at the termination of the experiment, a small sediment sample (∼5 g in a sterile tube) was collected from each replicate for potential nitrification rate measurements. Following this, meiofauna were isolated by quickly sieving the sediment using a 40 μm mesh sieve, transferred to a screw-cap tube and further concentrated per replicate to a total volume of 10 mL. Collected meiofauna was then stored at −80°C until RNA extraction.
Pooled samples from spiked sediments and control sediments were collected at termination for chemical analysis, and the samples were stored at −20°C prior to analysis. The remaining sediment was sieved, M. affinis individuals were collected and counted. Oxygen conditions were stable during the duration of the experiment as indicated by the high survival rate of M. affinis (96.5%, Supplementary Figure S4), a hypoxia sensitive amphipod, in the control at the end of the experiment.
RNA Extraction and cDNA Synthesis
From the collected meiofauna and the 1, 3, and 5 weeks subsampled sediment for bacterial community structure analysis, a total of 2 g was transferred to a microcentrifuge tube. For the meiofauna suspension, a pipette with a cut-off tip was used. The procedure described below was followed for all samples. For total RNA extraction, the RNeasy PowerSoil (QIAGEN) kit was used with slight modifications to its provided instructions, namely, samples were mechanically lysed on a custom-made vortex adapter and incubation times following elution were increased to a minimum of 2 h. Following extraction, RNA was treated with DNAse (TURBO DNA-free Kit, Invitrogen). To control for DNA carry-over, PCR using universal 16S primers (968F; 1401R, Felske et al., 1996) was performed on untreated RNA extractions for 35 cycles and showed no amplification through gel electrophoresis. RNA concentrations were normalized to 15 ng μl–1 and total RNA reverse transcription was carried out using the AccuScript First Strand Synthesis Kit (Agilent) on a GeneAmp PCR System (Applied Biosystems) following manufacturer’s instructions. cDNA was then stored at −20°C until metabarcoding library preparation.
16S and 18S rRNA Metabarcoding Library Preparation and Sequencing
Two libraries targeting the 16S and 18S ribosomal RNA gene on the synthesized cDNA were prepared, following the dual-index amplification methods adapted from Nascimento et al. (2018) and Andersson et al. (2008). The hypervariable variable region of the 16S and 18S rRNA marker was amplified using the 341F / 805R primer-pair (Herlemann et al., 2011) for bacteria and the TAReuk454FWD1/TAReukREV3 primer-pair (Stoeck et al., 2010) for eukaryotes (Table 2). Thermocycler conditions for both the 16S and 18S primer-pairs in the first round were: 30 s at 98°C, followed by 12 cycles of 10 s at 98°C, 30 s at 50°C, 30 s at 72°C. All PCR reactions for the library preparation were carried out on a BioRad T100 Thermal Cycler (BioRad Laboratories) using Q5 HS High-Fidelity Master Mix (New England BioLabs) following the manufacturer’s instructions. First round amplicons were cleaned by adding 0.1 μL Exonuclease I (New England BioLabs) and 0.2 μL Thermosensitive Alkaline Phosphatase (Promega), incubating for 15 min at 37°C followed by 15 min at 74°C to terminate the reaction. The second round of PCR was carried out using indexing primers as described by Andersson et al. (2008) to barcode each sample with a unique combination of forward and reverse index sequences avoid cross-contamination (Esling et al., 2015). Thermocycler conditions for the second round were 3 min at 95°C, 15 cycles of 30 s at 95°C, 30 s at 55°C, 30 s at 72°C, and a final elongation of 5 min at 72°C. The final barcoded amplification products were then cleaned using Agencourt AMPure XP magnetic beads (Beckman Coulter). Amplicon concentrations were measured using a Qubit 2.0 Fluorometer (dsDNA BR Assay Kit, Invitrogen), samples were then standardized for cDNA concentration, pooled, and sequenced on an Illumina MiSeq V3 system using a 2 × 300 bp setup at the National Genomics Infrastructure in Stockholm, Sweden (NGI, Stockholm). The sequences are available in the NCBI BioProject repository (PRJNA641540).
Bioinformatics
Sequence reads were demultiplexed by the sequencing facility using bcl2fastq_v2.20.0.422 from the CASAVA software suite. further quality filtering and chimera removal was done in R (v3.5.2, R Core Team, 2014) using the DADA2 pipeline (Callahan et al., 2016). In more detail, forward and reverse paired-end reads were truncated at 290 and 210 bp, respectively, and trimmed at 8 bp using the following parameters: truncLen = c(290,210), maxEE = c(2,2), trimLeft = c(8,8), minFoldParentOverAbundance = 4, and allowoneoff = TRUE. Taxonomic assignment for 16S rRNA amplicon sequence variants (ASVs) was carried out using the SILVA SSU database (r132) and the DECIPHER package (v 2.10.2, Wright et al., 2012). The ASVs generated by the DADA2 pipeline are higher-resolution alternative to the operational taxonomic units (OTUs) and report the number of times each amplicon is observed per sample (Callahan et al., 2017).
For eukaryotic ASVs, sequences were aligned against the NCBI NT (January 2019) database using BLAST (v2.7.0, Altschul et al., 1990) with an e-value threshold of 0.001. The output file was imported to MEGAN (v 6.14.2, Huson et al., 2016) that links NCBI NT association numbers with taxonomic classifications and uses the lowest common ancestor algorithm to further estimate taxonomic classifications. Sequences belonging to Thalassiosirophycidae, which accounted for the majority of diatoms that were added as food, were excluded from the dataset. Singletons were removed (ASVs occurring only once in the dataset) and the final read counts were normalized between samples as relative abundances.
Alpha diversities based on the 16S rRNA and 18S rRNA metabarcoding datasets were estimated using the Observed, ACE, and Shannon indices as implemented in the phyloseq package (v1.24.2; McMurdie and Holmes, 2013). The ACE index provides a rough estimate of abundance−based richness of ASVs and considers the abundance of rare taxa. The Shannon index accounts for both richness and evenness of ASVs present. A dissimilarity matrix was generated with the vegdist function of the vegan package (Oksanen, 2015) in R (v 3.4.2) using relative read counts with Bray-Curtis distances.
Quantitative PCR
cDNA synthesized from each replicate was used for the gene expression quantification of AmoA, PAH-ring hydroxylating dioxygenase G-, and 16S rRNA. For this purpose, the primer sets PAH-RHD GN F and PAH-RHD GN R, R968, and F1401 and amoA1F and amoA2R were used (Table 2). The PAH-RHDα primer set was designed by Cébron et al. (2008) and targets a wider selection of PAH-degraders than other sets (Baldwin et al., 2003; Nyyssönen et al., 2006; Park and Crowley, 2006). This primer-set was shown to be highly specific toward the target bacteria and resulted in a clear single band on 1% agarose gels. Quantification of 16S and PAH-RHDα copy numbers were normalized through gBlocks gene fragments (IDT) at 4.48 × 106 and 4.70 × 106 copies μl–1, respectively. Quantitative PCR was then carried out in duplicate, on a Roche LightCycler 480 II Real-Time PCR System in reaction volumes of 20 μl, containing SYBR Green I Master Mix (Roche), 0.10 μM of each primer, and 1 μl of template at approximately 5 ng μL–1 DNA. Thermocycler conditions were set to 95°C for 10 min as an initial denaturation step, followed by 40 cycles of 95°C for 20 s, 55°C for 20 s annealing, and 72°C for 15 s, followed by a final continuous melt curve analysis step at 60°C. Standard curves for each primer set were generated in duplicates using gBlocks Gene Fragments (IDT) at concentrations of 5 × 108–5 × 101. Copy numbers and melting curves were validated through a standard curve for each primer set and the amplification efficiency of each run (1.95 ≥ 2) using the LC480 software. Data analysis was carried out using the LightCycler 480 device software (v1.5.1) and converted to Excel for further analysis and visualization. Gene copy numbers for PAH-RHDα and AmoA were normalized against 16S cDNA amplification data for each sample.
Potential Nitrification Rates
Potential nitrification rates (PNR) were measured by NO3– production in NH4+ amended sediment slurries at in situ temperature (5°C) with shaking as previously described by Bonaglia et al. (2014b). This was done by creating slurries and transferring 2 g of sediment from each replicate to a 50 mL centrifuge tube after the termination of the experiment using a cut-off syringe. This was followed by adding 40 mL of filtered in situ bottom water using a disposable 0.2 μm filter. All slurries were then amended with a stock solution of 20 mM NH4Cl to a final concentration of 100 μM NH4+ and incubated in the dark for 15 min on a shaker table at in situ temperature. Subsamples of 15 mL were then taken after 15 min (T0), 24 h (T1), and 48 h (T2) for analysis of dissolved inorganic nitrogen (DIN, i.e., NH4+, NO3– and NO2–). DIN was determined on a segmented flow AutoAnalyzer system (ALPKEM, Flow Solution IV). The precision was ±0.036 μmol L–1 for NH4+, ±0.021 μmol L–1 for NO3–, and ±0.014 μmol L–1 for NO2– NO3–. The concentrations in each slurry were plotted against the sampling time, and individual slopes of the linear regression were used to calculate the net PNR according to Caffrey et al. (2019).
Statistical Analysis
Repeated measures ANOVA was used to test the effects of the chemical mixture (i.e., treatment), time and interaction of the two factors on the alpha-diversity metrics for bacteria, more specifically ACE (Abundance-based Coverage Estimate) and Shannon diversity indices. In the cases where time and the interaction of time and treatment had significant effects, one-way ANOVA was used to test the effect of treatment on diversity at each time point. The differences in bacterial community composition between the treatments were visualized using Non-metric Multidimensional Scaling (NMDS) ordination based on Bray-Curtis dissimilarity matrix. Effects of the contaminant mixture (treatment), time and their interaction on bacterial community structure were analyzed using repeated measures PERMANOVA test with PERMANOVA+ package in PRIMERe (v6) (Clarke et al., 2006). As meiofauna were collected only at the end of the experiment, differences in alpha diversity and community structures for the 18S dataset were tested with One Way ANOVA. PERMANOVA was used to test the effects of treatment on the community composition with the adonis function of the vegan package (Oksanen, 2015) in R (v 3.4.2). Best-fit analyses based on the Bray-Curtis dissimilarity index and Spearman correlations were conducted using the BIO-ENV method (Clarke and Ainsworth, 1993). This made it possible to get an indication of the best subset of taxa that changed in relative abundance in response to the addition the contaminant mixture. Differences in AmoA and PAH-RHDα gene abundances and nitrification rates were tested using repeated measures ANOVA. Prior to all ANOVA tests, the assumptions of normality of distribution was tested with Shapiro Wilk tests and homogeneity of variances with the Levene test. Differences in relative abundances of the top 20 bacterial and eukaryotic taxa between treatments at the phylum and genus level were tested with Kruskall-Wallis non-parametric test as relative abundances were not normally distributed. The ‘betadisper’ function in the R vegan package was used to test for differences in multivariate homogeneity of beta diversity variance between treatments.
Results
Exposure Concentrations of the Contaminant Mixture in Sediment
The concentration of each single contaminant in sediment porewater (Cporewater) of the spiked sediment was 0.11 to 1.15 μg L–1, corresponding to chemical activities of 0.00004 to 0.01 and a total chemical activity of the mixture of 0.024 (Table 1). The total exposure of the contaminant mixture used in this study is similar to what has previously been observed in contaminated areas in the Baltic Sea (Löf et al., 2016), but is higher than at National monitoring stations in background areas (Swedish Geology Survey, apps.sgu.se/kartvisare). The exposure level is a tradeoff between having a mixture with a high enough chemical activity (i.e., 0.01 to 0.1 according to literature) that causes non-specific toxicity, alongside the concentration of each mixture-constituent below any concentration threshold for specific toxicity. The chemical analyses of the sediment showed a loss of chemicals during spiking (nominal spiked versus measured concentrations prior experiment), and during the experiment (measured concentrations prior and after the experiment). At the end of the experiment less than 15% of PCBs, HCB and pyrene, and 40% of phenanthrene were retained compared to the measured concentrations prior experiment (Table 1). Biphenyl was no longer detected at the end of the experiment. Despite the loss of chemicals during the spiking and the experiment, the concentrations in the spiked sediment were high compared to control. In control sediment, all chemicals were below detection limits (Table 1). The loss of chemicals during the experiment is likely due to the chemicals not reaching equilibrium with the sediment during spiking, which in turn, likely is an effect of the high mass of sediment (∼800 g) per jar.
Amplicon Sequencing Output
Amplicon sequencing yielded a total of 3,000,277 and 1,127,063 reads after quality trimming and filtering, with an average of 32,063 and 112,706 sequences per sample for the 16S rRNA and 18S rRNA marker, respectively. After removing singletons, a total of 5,369 bacterial ASVs and 4,543 eukaryotic ASVs remained.
Bacterial Diversity and Community Structure
Of the total number of ASVs from all samples, the majority belonged to the Proteobacteria (71.4 ± 5.59%, mean ± one standard deviation), Bacteroidetes (9.65 ± 2.6%), Actinobacteria (5.74 ± 1.65%) and Acidobacteria phyla (3.43 ± 1.4%) (Supplementary Figure S1A). Within the Proteobacteria phylum, ASVs belonged mainly to the classes Gammaproteobacteria (51 ± 9%) and Deltaproteobacteria (15 ± 3%). The top 20 genera with the highest relative abundance belonged to 17 distinct families (Figure 2). The relative abundance of Methylophagaceae was notably high in samples across all timepoints, averaging at 23 ± 14% and 26 ± 16% in control and spiked sediments, respectively. Desulfobacteraceae followed with 11 ± 1% in control and 10.4 ± 1.8% in spiked and Ilumatobacteraceae with 5.4 ± 0.5% and 6.4 ± 1.6%, respectively.
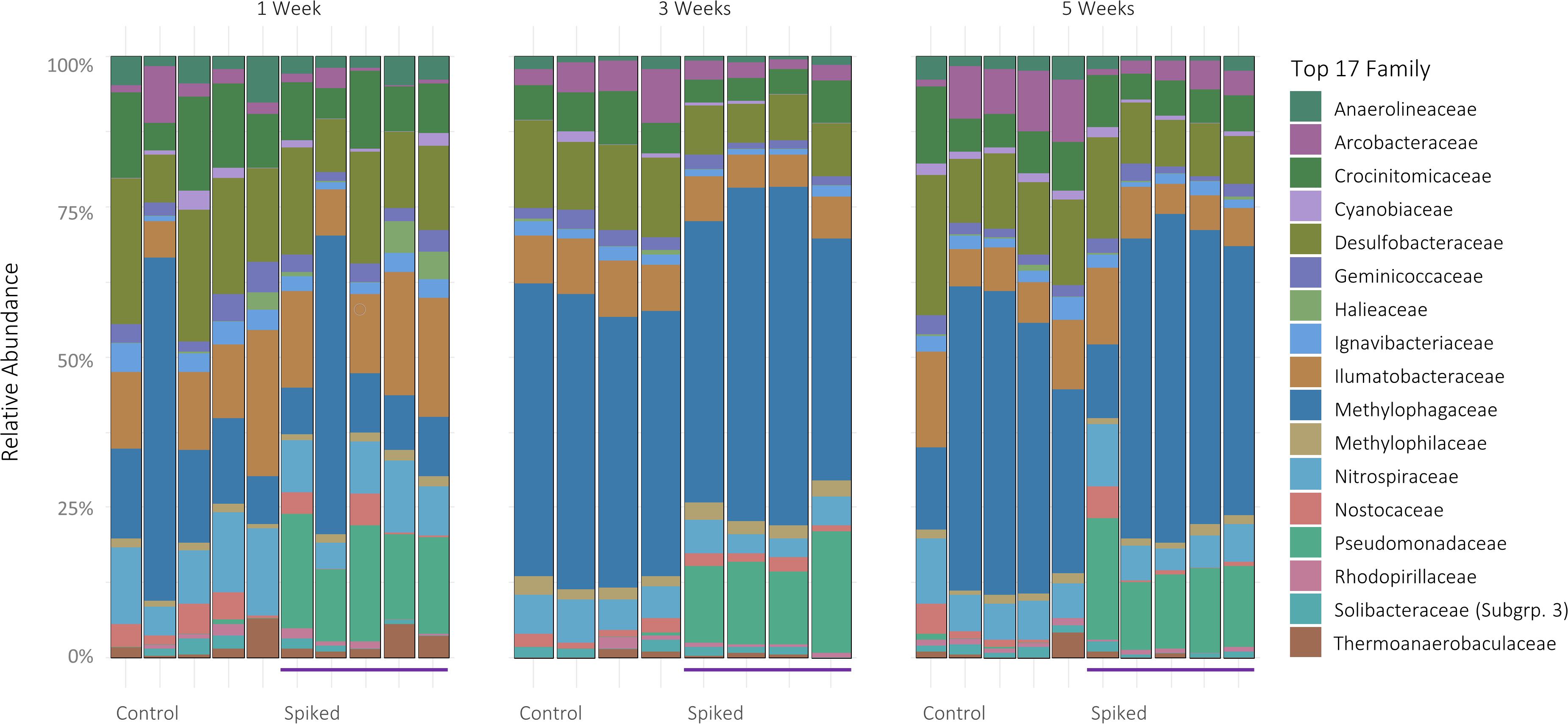
Figure 2. 16S rRNA relative abundance of the top 17 most common prokaryotic Families, facetted by timepoint. Each bar represents a sampling replicate (5 replicates per treatment for week 1 and 5, 4 replicates for week 3).
Focusing on the changes between treatments, our results show that the relative abundance of Gammaproteobacteria increased in the spiked sediment after 5 weeks of incubation (week 1, 41 ± 8%; compared to week 5, 53 ± 11%; n = 5 for both weeks, Kruskal-Wallis test, df = 1, H = 6,91, p = 0.031) (Supplementary Figure S1B). Notably, Pseudomonadaceae were significantly higher in the spiked treatment (5.2 ± 0.05% Pseudomonas spp., 1.4 ± 0.02% other unclassified Pseudomonadaceae) when compared to control (0.06 ± 0.01% and 0.02 ± 0.01%, respectively) (n = 5, KW, p < 0.001, df = 1, H = 21,2) across all timepoints. Finally, averaged over all timepoints, the relative abundances of Desulfobacteraceae, Crocinitomicaceae, and Anaerolineaceae, were significantly lower in the spiked sediment (KW, p < 0.05, df = 1, H = 13.8, 6.8, 7.4, respectively). The bio.env analysis indicated that changes in Pseudomonas and Desulfobacteraceae (Desulfobacterales) best explained the differences between treatments in community structure (Spearman’s rho 0.84; Figure 3).
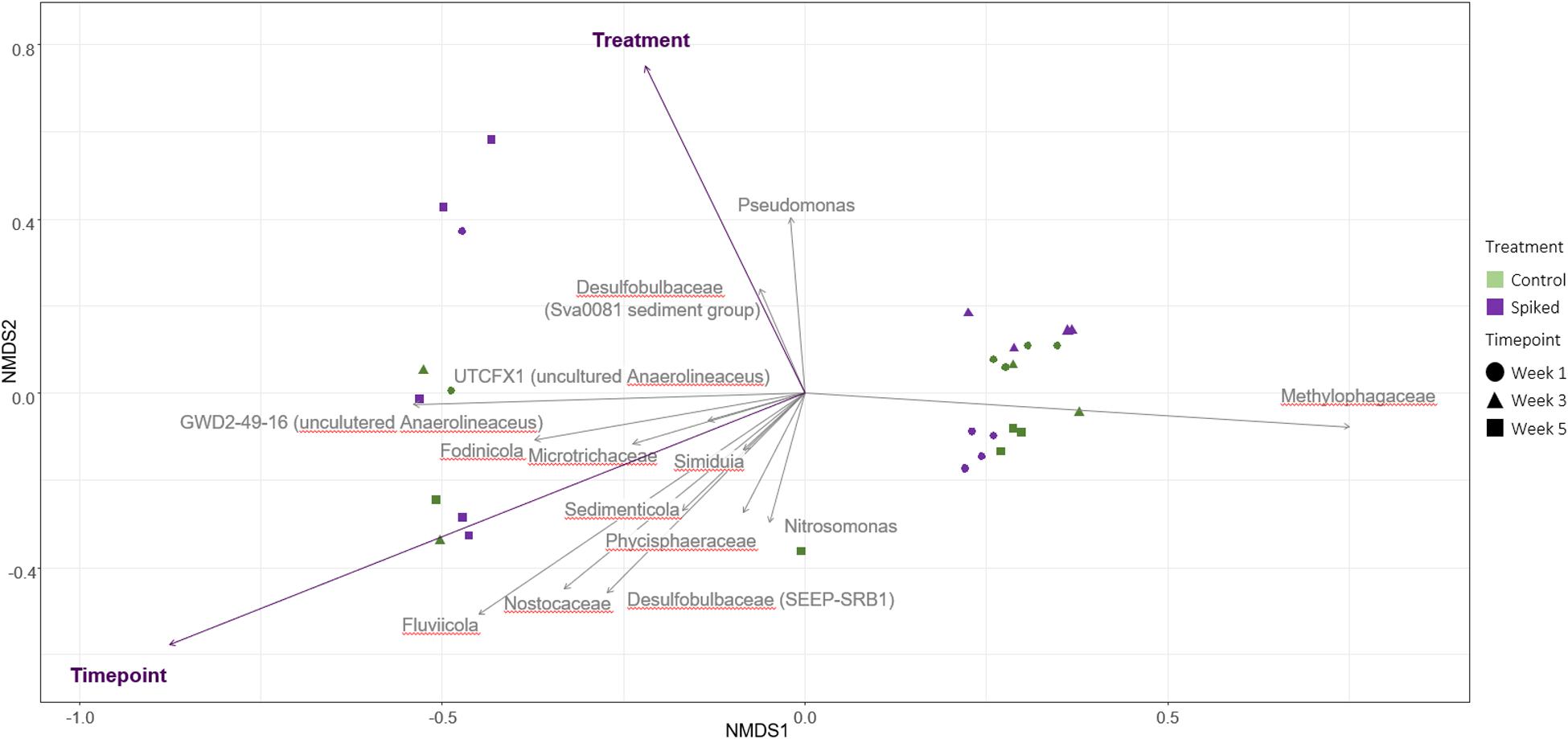
Figure 3. NMDS of the 16S rRNA data with Bray-Curtis distance, based on data for all timepoints. The overlying triplot shows the results of the bio.env analysis. The circles denote control replicates (green) and sediment spiked with the chemical mixture (purple), (stress = 0.07, Spearman’s rho = 0.84).
There was no effect of treatment on alpha diversity of bacterial communities calculated by ACE or Shannon indices (repeated measures ANOVA: F1,9 = 0.66, p = 0.44 and F1,9 = 0.32, p = 0.59, respectively) (Supplementary Figure S2). On the other hand, there was an effect of treatment on the bacterial community structure (repeated measures PERMANOVA: pseudoF1,9 = 4.68, p = 0.008). The NMDS ordination on bacterial ASVs showed that the majority of the sampling sites formed two significantly different clusters; one for control and one for the spiked replicates (Figure 4). Additionally, repeated measure PERMANOVA showed a significant difference of time on the bacterial community structure (pseudoF1,9 = 4.4, p = 0.0026) (Figure 4).
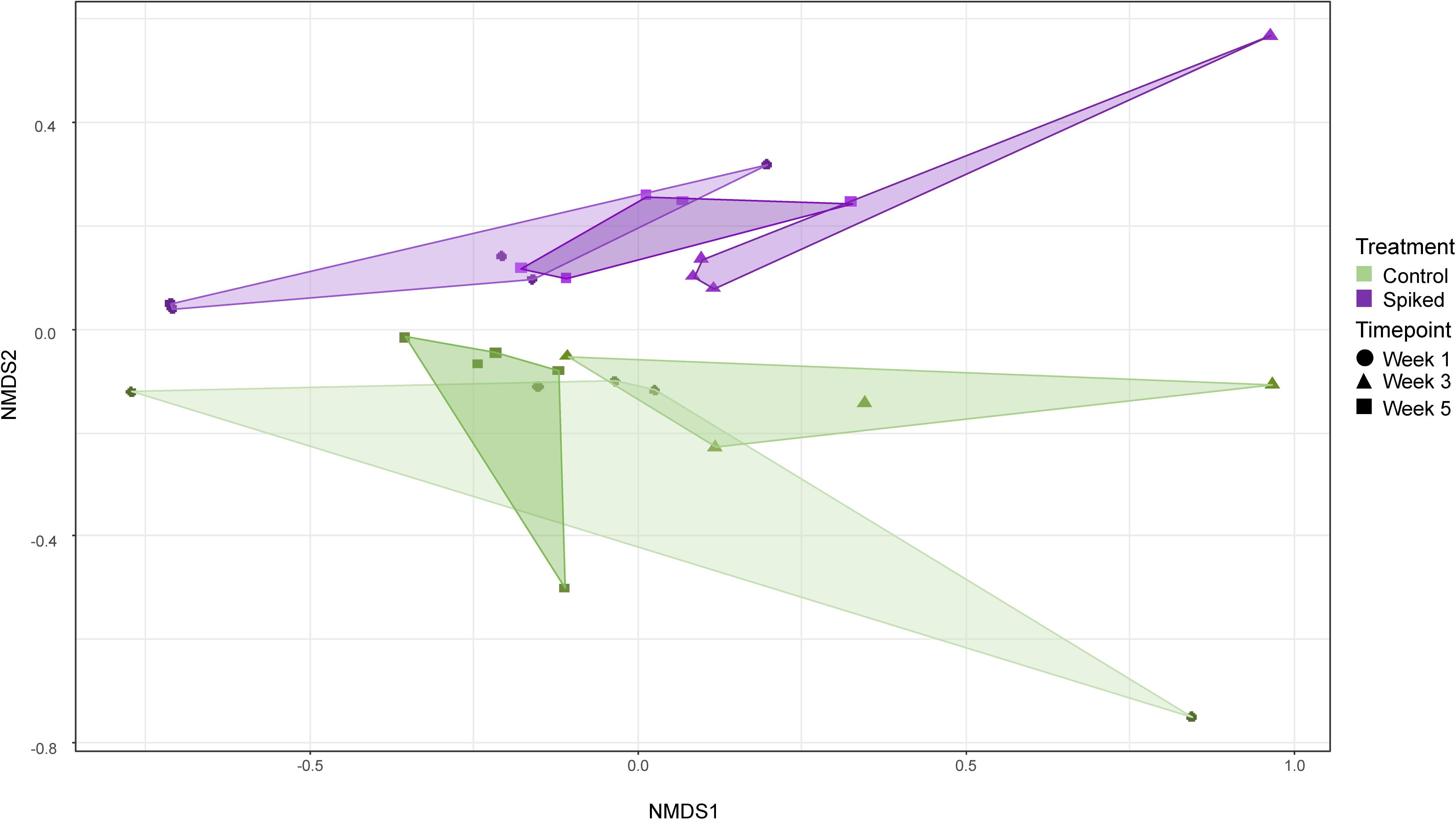
Figure 4. NMDS of Bray-Curtis dissimilarities based on the relative abundance of all ASVs classified as bacteria (stress = 0.02). Different colors represent the two experimental treatments (with green denoting controls, and purple sediment spiked with the chemical mixture). The shaped points denote samples taken in the different timepoints.
Microeukaryotic Diversity and Community Structure
Across all samples, Ciliophora (average read abundance 30.2 ± 11%), Metazoa (24.3 ± 6%) and Bacillariophyta (8 ± 2%) were the most represented taxa (Figure 5A). Zooming in the differences among treatments at the class level, our results show that the relative abundance of diatoms (Bacillariophyta) was higher in controls compared to spiked sediment (KW, p = 0.009, df = 1, H = 6.8). Conversely, ciliates were found at a lower relative abundance in the controls compared to spiked sediments (21 ± 1.2%, 27 ± 3.3%, respectively), with the class Prostomatea being dominant in this treatment (KW, p = 0.021, df = 1, H = 0.02).
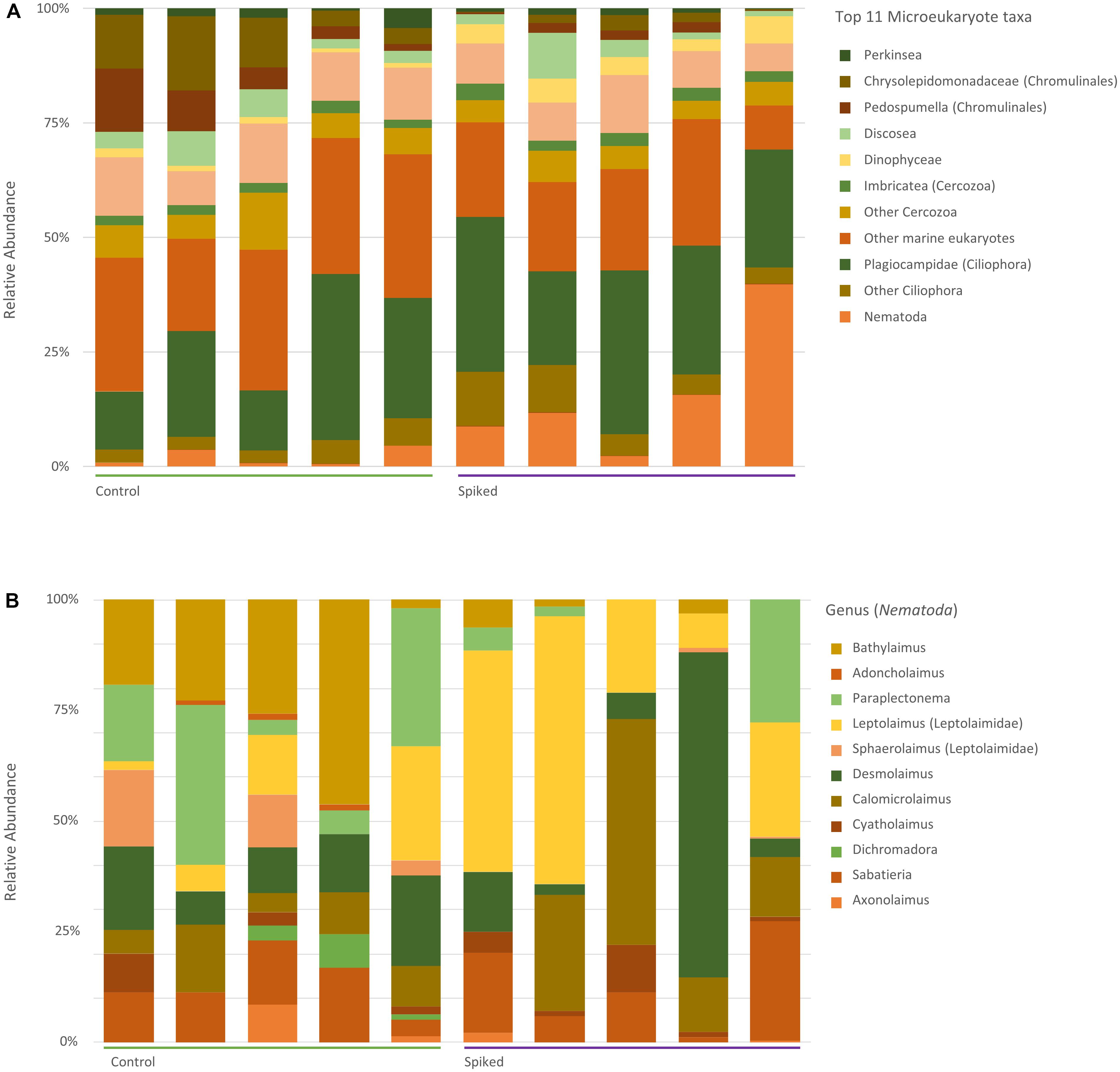
Figure 5. Relative abundance of the top 11 most common taxa reported for the 18S rRNA (A) and the most common genera amongst nematodes (B).
For meiofauna, the relative abundance of nematodes was significantly higher in spiked replicates (53 ± 14%) when compared to control (22 ± 8%; Figure 5). There was a significant increase in relative abundance amongst taxa in the Leptolaimidae family (KW, p = 0.02, df = 1, H = 5.12) and. Particularly, Leptolaimus spp. showed a significant increase in abundance in spiked sediments (KW, p = 0.013, df = 1, H = 6.14), whereas Paraplectonema, Adoncholaimus, and Bathylaimus spp. showed a decrease (KW, p = 0.046, df = 1, H = 5.12; p = 0.05, H = 3.7, and p = 0.027, H = 4.8, respectively). The bio.env analysis of the top 30 reported metazoa, showed that changes in Leptolaimus and Microlaimidae relative abundance explained most of the variation between treatments (Spearman’s rho = 0.72). Similar to the 16S rRNA dataset, eukaryotic alpha diversity was not affected by the chemical mixture (One Way ANOVA, F1,9 = 0.5425, p = 0.48) (Supplementary Figure S3), but there was a significant effect on the microeukaryotic community structure (PERMANOVA, pseudoF1,9 = 2.77, p = 0.009). An NMDS plot based on relative abundances for the 18S rRNA showed separate clustering between the controls and spiked sediment (Figure 6). A similar pattern was found using presence/absence matrix and Sørensen differences (Supplementary Figure S5, PERMANOVA, pseudo F1,9 = 2.57, p = 0.03).
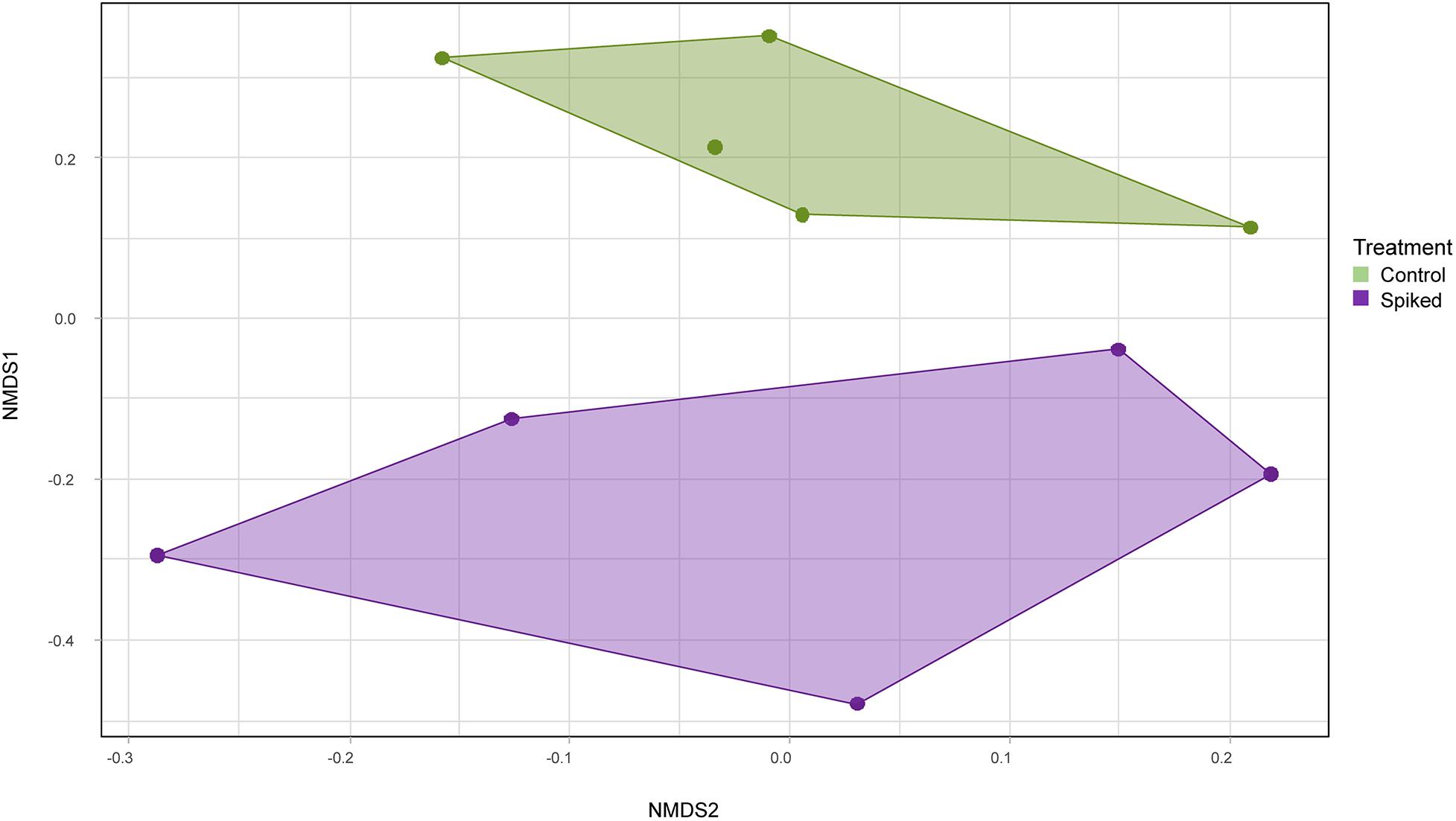
Figure 6. NMDS of Bray-Curtis dissimilarities based on the relative abundance of all ASVs in the 18S rRNA dataset. Spiked (purple) and control (green) grouped distinctly with no overlap between samples.
Quantification of PAH-RHD and AmoA cDNA
AmoA expression levels did not show any significant changes between treatment and timepoints (repeated measures ANOVA, F1, 9 = 0.55, p = 0.46 and, F1,9 = 2.35, p = 0.12, respectively) (Figure 7). However, there was a significant difference between treatments in the expression levels of the PAH-RHD gene (repeated measures ANOVA, F1, 9 = 42.98, p < 0.0001). It was detected in spiked sediment samples from all three timepoints but remained under detection limit in the control samples (Figure 7).
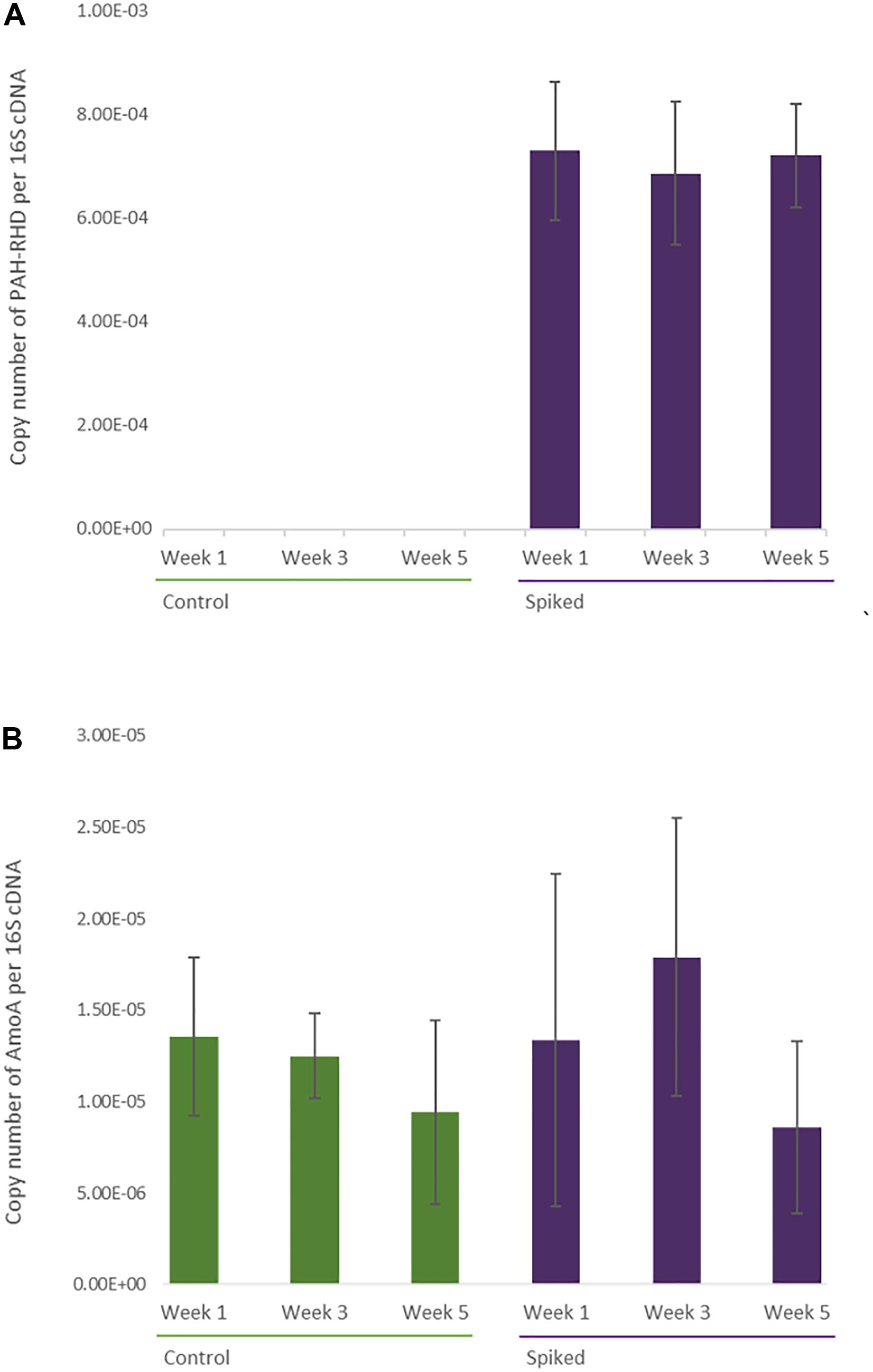
Figure 7. RT-qPCR Quantification of PAH-RHD (A) and AmoA cDNA gene copies (B). Relative abundance of PAH-RHD and AmoA copies were normalized by 16S gene copy count and averaged per treatment and timepoint. Error bars represent standard deviation from replicates.
Potential Nitrification Rates
All incubated sediment slurries showed linear nitrate production with time (with R2 ranging between 0.87-0.98). Average potential nitrification rates (PNR) calculated from these slopes were 21.1 ± 4.6 nmol cm–3 d–1 in control and 23.5 ± 1.5 nmol cm–3 d–1 in spiked treatment. Exposure to the contaminant mixture did not significantly affect PNR at the end of the experiment (Figure 8, ANOVA, F1,9 = 1.26; p = 0.293). However, average PNR was lower in the controls compared to spiked treatment.
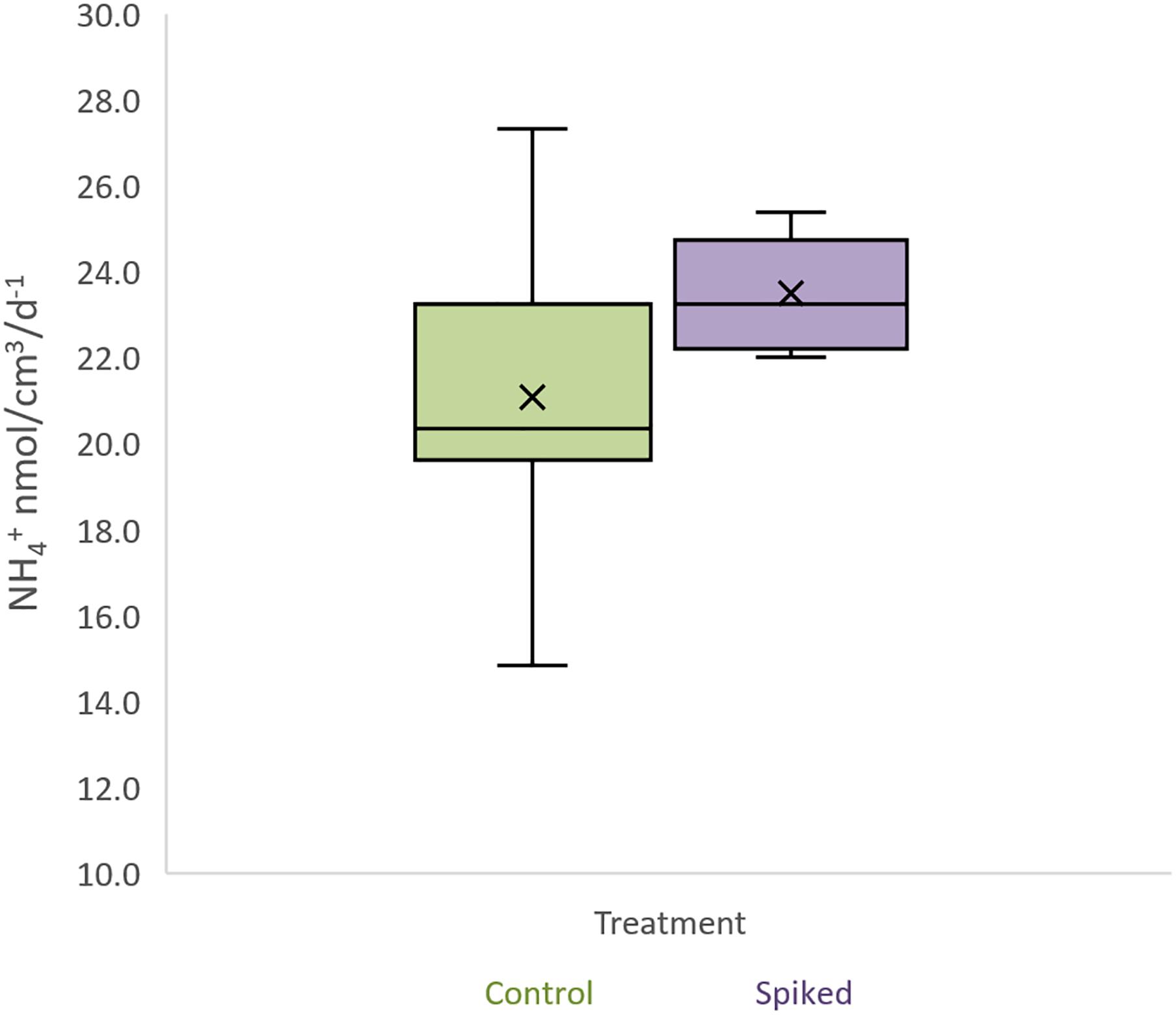
Figure 8. Potential nitrification rates for control and spiked samples. In the box plots, the boundary of the box closest to zero indicates the 25th percentile, a black line within the box marks the mean, and the boundary of the box farthest from zero indicates the 75th percentile. Whiskers above and below the box indicate the 10th and 90th percentiles.
Discussion
16S rRNA Microbial Community Structure Analysis
Our results showed that there was a notable shift in the relative abundance of several microbial taxa in spiked sediments. Pseudomonas (amongst the Gammaproteobacteria) showed a clear increase in relative abundance in treated sediments (from 0.23 ± 0.13% to 11 ± 2.7%). Species belonging to the Pseudomonas genus have regularly been found at various contaminated sites (Ridgway et al., 1990; Aitken et al., 1998; Romero et al., 1998) and shown to be able to degrade a variety of PAHs (amongst other contaminants) in previous pure-culture studies (Müller et al., 1999; Rockne et al., 2000; Wasi et al., 2011). This increase in abundance of PAH-degrading taxa was again visible from the high PAH-dioxygenase expression levels measured in the contaminated sediments. Active PAH degradation, degradation pathways of biphenyl, PCB and other halogenated aromatic degradation, by Pseudomonas spp. has been widely reported (Hofer et al., 1993; Commandeur and Parsons, 1994), even under aerobic conditions (Abramowicz, 1990). This action by Pseudomonas spp. could partially explain the lower contaminant levels measured at the end of our experiment. Several strains of Pseudomonas have been suggested as viable candidates for use in bioremediation or bioaugmentation scenarios (Wasi et al., 2013; Kahlon, 2016; Huang et al., 2017; Liu et al., 2017). The high Pseudomonas relative abundance as well as PAH-RHD gene expression in our contaminated microcosms further suggests its viability in such applications. Additionally, Epsilonproteobacteria, particularly Helicobacteraceae, have been reported to increase in relative abundance in sediments contaminated with a complex mixture of PCBs, PAHs and heavy metals (Quero et al., 2015), but contrary to Pseudomonas, Heliobacteria do not seem to be able to metabolize PAHs or PCBs. They appear to be abundant in contaminated sediments, but not essential for the degradation of organic contaminants (Starke et al., 2016). Obi et al. (2016) suggested that Epsilonproteobacteria may be synthrophs of potential PAH and PCB metabolizing species. Nevertheless, the most common Epsilonproteobacteria in our spiked sediment, Arcobacteraceae, did not increase but in fact significantly decreased in relative abundance in the spiked treatment when compared to the controls. Alongside Pseudomonas, Desulfobacteraceae has also been associated with anaerobic degradation of naphthalene (Kümmel et al., 2015; Bonaglia et al., 2020). Accordingly, in our study the genus Sva0081 of the Desulfobacteraceae family was shown by the bio.env analysis to drive an important portion of the differences between treatments in microbial community composition. This minor shift in our findings may be due to the well-aerated conditions, partially aided by the M. affninis (Karlson et al., 2010) in our microcosms, that tends to favor aerobic degraders like Pseudomonas (Lu et al., 2011).
Microeukaryotic Community Structure Analysis
Among the microeukaryotes, nematodes and ciliates, with the majority belonging to the Chromadorea class and Plagiocampidae family, respectively, were found in higher relative abundances in the contaminated sediments compared to the controls. The relative abundances of a number of taxa at the family level within Nematoda (Desmodoridae, Monhysteridae, Plectidae, and Araeolaimidae) did not differ significantly between control and spiked sediments. The lack of response of benthic metazoans to exposure to organic contaminants contrasts with previous results reported by Bik et al. (2012) that found nematodes to decrease in relative abundance in response to the Deep-Water Horizon Oil spill. The exposure of nematodes to organic contaminants in this study was not measured, but it is likely to have been much higher than what was seen in our experimental sediments, providing a possible explanation for the contrasting responses. Nevertheless, at the genus level we found in our study important shifts in some taxa, namely Leptolaimus and Bathylaimus, and to a lesser extent, Paraplectonema, showed a higher relative abundance in spiked sediments, indicating that these nematode taxa were tolerant to non-specific toxicity of contaminant mixture at the levels here tested. The striking dominance of Leptolaimus in our sediments contaminated with PCBs/PAHs detected with metabarcoding, is in accordance with previous morphological studies by Wang et al. (2009) and Moreno et al. (2008a), despite the different methods utilized. From a wide range of contaminants tested, Moreno et al. (2008a) found Leptolaimus to only be present in highly contaminated areas where PAH content measured up to ∼145 mg kg–1. Moreover, Leptolaimus appears to be particularly resilient toward a wider variety of environmental stressors, from physical disturbances (Schratzberger and Warwick, 1998), hypoxia/anoxia (Modig and Ólafsson, 1998) to heavy metals (Somerfield et al., 1994; Moreno et al., 2008b) and other organic contaminants (Moreno et al., 2008a; Wang et al., 2009). As such, their general resilience might allow them to avoid food competitors and, in this case, thrive in relatively toxic shallow sediment. Nematodes of the genus Sabatieria have also been found in our study to be either unaffected by the exposure to contaminated sediment or to even increase in relative abundance therein (Louati et al., 2015). They have been reported for their tolerance to petrochemical and heavy metal contamination (Hedfi et al., 2007; Beyrem et al., 2010) or high levels of PAHs. The PAH/PCB content in the sediment most likely exerted a negative pressure on potential food competitors, benefitting the more tolerant and opportunistic feeders. As in most studies using high throughput DNA sequencing to investigate changes in bacterial communities our data is compositional, presenting data in relative abundances. Detecting changes in microbial communities with compositional data has been shown to present a number of important drawbacks (Morton et al., 2019). Alternative methods of normalization like log-ratio or reference frames (Gloor et al., 2017; Quinn et al., 2019) should be considered in the future to facilitate the detection of reproducible changes of microbial communities from compositional data.
Potential Nitrification Rates (PNR) and Expression of Ammonia Monooxygenase (AmoA) and PAH-Dioxygenase (PAH-RHD) Marker Genes
PNR rates were several orders of magnitude lower when compared to those found in previous works in the Baltic Sea (Bonaglia et al., 2014a; Caffrey et al., 2019). Our rates ranged only between 14.8 and 27.3 nmol N mL–1 d–1, while the cited studies reported rates ranged between 283.2-640.8 nmol N mL–1 d–1 (Bonaglia et al., 2014a) and 108-1154 nmol N mL–1 h–1 (Caffrey et al., 2019). The lower nitrification rates found in our study compared to the enormous ones reported by Caffrey and co-authors were likely due to differences in temperature between our experimental conditions (5 °C) and the referenced study (room temperature, approximately 21 °C). Additionally, the manipulation of our sediments might have contributed to such differences. Our microcosms were well aerated, and the sieving of the sediment prior to the microcosm setup might have resulted in substrate limitation and consequent lower nitrifying activity (Bernhard and Bollmann, 2010). Expression of the bacterial ammonia monooxygenase marker gene (AmoA) measured through qPCR and PNR did not show a significant change between contaminated and control sediment. However, although not statistically significant, both AmoA expression levels and PNR-rates were indicative of a net increase of nitrification activity in spiked sediment. This appears to conflict with recent work by Lindgren et al., 2014, 2012, which showed a reduced capability for nitrification as a response to PAH exposure. While many Pseudomonas members (Gammaproteobacteria) are known denitrifiers, some species (e.g., P. stutzeri, P. tolaasii, P. aeruginosa) exhibit heterotrophic nitrification capabilities (i.e., He et al., 2015). Paired with the high expression of PAH-RHD, their nitrifying activity, consequentially, may have been inhibited. In addition, our experimental setup only allowed us to assess effects on PNR at the end of the experiment. It is possible that exposure to contaminants had an initial effect on this benthic process that was not detectable at the termination of our experiment. Nevertheless, our results suggest that such a potential negative effect on nitrification was not present 5 weeks after exposure. Future work should aim to investigate such temporal dynamics of effects of non-specific toxicity on benthic ecosystem processes.
Conclusion
Our results reveal a complex response of benthic interactions to non-specific toxicity of organic contaminant mixtures and calls for further research with a broader range of chronic chemical exposure to identify potential taxa that can serve as bioindicators of non-specific toxicity. Nevertheless, we report on effects of non-specific toxicity of organic contaminant mixtures at a community level of biological organization for both bacteria and microeukaryotes. We found that the tested levels of the chemical mixture can significantly decrease the relative abundance of sensitive taxa like Paraplectonema and increase the dominance of resistant nematodes like Leptolaimus. In addition, our results indicate a functional response of bacterial communities with the levels of microbial PAH-degradation gene expression increasing in spiked microcosms aligned clearly with a significant rise in the relative abundance of active Pseudomonas genus. Furthermore, our study indicates that utilizing metabarcoding, next-generation sequencing and quantitative methods on RNA gene expression has shown to be an effective approach to assess effects of disturbance on active meiofauna and microbial community structures in experiments with a short time frame. As (e)DNA-based metabarcoding has become a versatile and robust method for community-wide studies and assessing biodiversity, using eRNA can provide additional insights to how benthic communities can respond to environmental changes over a short period of time.
Data Availability Statement
The datasets presented in this study can be found in online repositories. The names of the repository/repositories and accession number(s) can be found below: NCBI BioProject, accession no: PRJNA641540.
Author Contributions
SI performed the experiment, molecular analysis and bioinformatics, and drafted the manuscripts. IN performed the sediment spiking and chemical analysis, and provided feedback on the manuscript. SB performed biogeochemistry and data analysis, and provided feedback on the manuscript. AK and AS helped to design the experiment and provided feedback on the manuscript. FN designed the experiment, finances and coordinated the project and helped draft the manuscript. All authors contributed to the article and approved the submitted version.
Funding
Experimental work was financed by the Swedish Research Council Formas to FN (Grant no. 2016-00804), and Stockholm University faculty funding to AS.
Conflict of Interest
The authors declare that the research was conducted in the absence of any commercial or financial relationships that could be construed as a potential conflict of interest.
Acknowledgments
We are very grateful to Matias Ledesma, Serena Albert, Léonie Le Bouille, and Nicolas Joulia for their support during sampling and setting up the microcosms and our thanks to Karin Ek for her help with the sediment spiking and Dr. Elias Broman for his help on this manuscript. Furthermore, we would like to acknowledge the staff of Askö Laboratory for their assistance with the sediment and animal sampling.
Supplementary Material
The Supplementary Material for this article can be found online at: https://www.frontiersin.org/articles/10.3389/fenvs.2020.00128/full#supplementary-material
References
Abramowicz, D. A. (1990). Aerobic and anaerobic biodegradation of PCBs: a review. Crit. Rev. Biotechnol. 10, 241–251. doi: 10.3109/07388559009038210
Aitken, M. D., Stringfellow, W. T., Nagel, R. D., Kazunga, C., and Chen, S.-H. (1998). Characteristics of phenanthrene-degrading bacteria isolated from soils contaminated with polycyclic aromatic hydrocarbons. Can. J. Microbiol. 44, 743–752. doi: 10.1139/w98-065
Altschul, S. F., Gish, W., Miller, W., Myers, E. W., and Lipman, D. J. (1990). Basic local alignment search tool. J. Mol. Biol. 215, 403–410.
Andersson, A. F., Lindberg, M., Jakobsson, H., Bäckhed, F., Nyrén, P., and Engstrand, L. (2008). Comparative analysis of human gut microbiota by barcoded pyrosequencing. PLoS One 3:e2836. doi: 10.1371/journal.pone.0002836
Baldwin, B. R., Nakatsu, C. H., and Nies, L. (2003). Detection and enumeration of aromatic oxygenase genes by multiplex and real-time PCR. Appl. Environ. Microbiol. 69, 3350–3358. doi: 10.1128/AEM.69.6.3350-3358.2003
Bernhard, A. E., and Bollmann, A. (2010). Estuarine nitrifiers: new players, patterns and processes. Estuar. Coast. Shelf Sci. 88, 1–11. doi: 10.1016/j.ecss.2010.01.023
Beyrem, H., Louati, H., Essid, N., Aïssa, P., and Mahmoudi, E. (2010). Effects of two lubricant oils on marine nematode assemblages in a laboratory microcosm experiment. Mar. Environ. Res. 69, 248–253. doi: 10.1016/j.marenvres.2009.10.018
Bik, H. M., Halanych, K. M., Sharma, J., and Thomas, W. K. (2012). Dramatic shifts in benthic microbial eukaryote communities following the deepwater horizon oil spill. PLoS One 7:e38550. doi: 10.1371/journal.pone.0038550
Bonaglia, S., Broman, E., Brindefalk, B., Hedlund, E., Hjorth, T., Rolff, C., et al. (2020). Activated carbon stimulates microbial diversity and PAH biodegradation under anaerobic conditions in oil-polluted sediments. Chemosphere 248:126023. doi: 10.1016/j.chemosphere.2020.126023
Bonaglia, S., Deutsch, B., Bartoli, M., Marchant, H. K., and Brüchert, V. (2014a). Seasonal oxygen, nitrogen and phosphorus benthic cycling along an impacted Baltic Sea estuary: regulation and spatial patterns. Biogeochemistry 119, 139–160. doi: 10.1007/s10533-014-9953-6
Bonaglia, S., Nascimento, F. J., Bartoli, M., Klawonn, I., and Bruchert, V. (2014b). Meiofauna increases bacterial denitrification in marine sediments. Nat. Commun. 5:5133. doi: 10.1038/ncomms6133
Caffrey, J. M., Bonaglia, S., and Conley, D. J. (2019). Short exposure to oxygen and sulfide alter nitrification, denitrification, and DNRA activity in seasonally hypoxic estuarine sediments. FEMS Microbiol. Lett. 366, 1–10. doi: 10.1093/femsle/fny288
Callahan, B. J., Mcmurdie, P. J., and Holmes, S. P. (2017). Exact sequence variants should replace operational taxonomic units in marker-gene data analysis. ISME J. 11, 2639–2643. doi: 10.1038/ismej.2017.119
Callahan, B. J., McMurdie, P. J., Rosen, M. J., Han, A. W., Johnson, A. J. A., and Holmes, S. P. (2016). DADA2: high-resolution sample inference from Illumina amplicon data. Nat. Methods 13, 581–583. doi: 10.1038/nmeth.3869
Cardinale, B. J., Duffy, J. E., Gonzalez, A., Hooper, D. U., Perrings, C., Venail, P., et al. (2012). Biodiversity loss and its impact on humanity. Nature 486, 59–67. doi: 10.1038/nature11148
Carman, K. R., and Todaro, M. A. (1996). Influence of polycyclic aromatic hydrocarbons on the meiobenthic-copepod community of a Louisiana salt marsh. J. Exp. Mar. Bio. Ecol. 198, 37–54. doi: 10.1016/0022-0981(95)00168-9
Cébron, A., Norini, M. P., Beguiristain, T., and Leyval, C. (2008). Real-time PCR quantification of PAH-ring hydroxylating dioxygenase (PAH-RHDα) genes from gram positive and gram negative bacteria in soil and sediment samples. J. Microbiol. Methods 73, 148–159. doi: 10.1016/j.mimet.2008.01.009
Clarke, K. R., and Ainsworth, M. (1993). A method of linking multivariate community structure to environmental variables. Mar. Ecol. Prog. Ser. 92, 205–219. doi: 10.3354/meps092205
Clarke, K. R., Clarke, K. R., Gorley, K. N., Clarke, K., and Gorley, R. (2006). PRIMER v6: User Manual/Tutorial.
Commandeur, L. C. M., and Parsons, J. R. (1994). “Biodegradation of halogenated aromatic compounds,” in Biochemistry of Microbial Degradation, ed. C. Ratledge (Berlin: Springer), 423–458. doi: 10.1007/978-94-011-1687-9_13
Corinaldesi, C., Beolchini, F., and Dell’Anno, A. (2008). Damage and degradation rates of extracellular DNA in marine sediments: implications for the preservation of gene sequences. Mol. Ecol. 17, 3939–3951. doi: 10.1111/j.1365-294X.2008.03880.x
Ditsworth, G. R., Schults, D. W., and Jones, J. K. P. (1990). Preparation of benthic substrates for sediment toxicity testing. Environ. Toxicol. Chem. 9, 1523–1539. doi: 10.1002/etc.5620091211
Dulio, V., van Bavel, B., Brorström-Lundén, E., Harmsen, J., Hollender, J., Schlabach, M., et al. (2018). Emerging pollutants in the EU: 10 years of NORMAN in support of environmental policies and regulations. Environ. Sci. Eur. 30:5. doi: 10.1186/s12302-018-0135-3
ECHA (2017). Guidance on Information Requirements and Chemical Safety Assessment, r. 11: PBT/vPvB Assessment. Helsinki: ECHA.
Edlund, A., and Jansson, J. K. (2006). Changes in active bacterial communities before and after dredging of highly polluted Baltic Sea sediments. Appl. Environ. Microbiol. 72, 6800–6807. doi: 10.1128/AEM.00971-06
Escher, B. I., Eggen, R. I. L., Schreiber, U., Schreiber, Z., Vye, E., Wisner, B., et al. (2002). Baseline toxicity (Narcosis) of organic chemicals determined by in vitro membrane potential measurements in energy-transducing membranes. Environ. Sci. Technol. 36, 1971–1979. doi: 10.1021/es015844c
Esling, P., Lejzerowicz, F., and Pawlowski, J. (2015). Accurate multiplexing and filtering for high-throughput amplicon-sequencing. Nucleic Acids Res. 43, 2513–2524. doi: 10.1093/nar/gkv107
Felske, A., Engelen, B., Nübel, U., and Backhaus, H. (1996). Direct Ribosome Isolation from Soil To Extract Bacterial rRNA for Community Analysis. Available online at: https://aem.asm.org/content/62/11/4162 (accessed February 22, 2020).
Fleeger, J. W., Carman, K. R., and Nisbet, R. M. (2003). Indirect effects of contaminants in aquatic ecosystems. Sci. Total Environ. 317, 207–233. doi: 10.1016/S0048-9697(03)00141-4
Giere, O. (2009). Meiobenthology, The Microscopic Motile Fauna of Aquatic Sediments. Berlin: Springer Science and Business Media.
Gilbert, D., Witt, G., Smedes, F., and Mayer, P. (2016). Polymers as reference partitioning phase: polymer calibration for an analytically operational approach to quantify multimedia phase partitioning. Anal. Chem. 88, 5818–5826. doi: 10.1021/acs.analchem.6b00393
Gloor, G. B., Macklaim, J. M., Pawlowsky-Glahn, V., and Egozcue, J. J. (2017). Microbiome datasets are compositional: and this is not optional. Front. Microbiol. 8:2224. doi: 10.3389/fmicb.2017.02224
Gobas, F. A. P. C., Mayer, P., Parkerton, T. F., Burgess, R. M., van de Meent, D., and Gouin, T. (2018). A chemical activity approach to exposure and risk assessment of chemicals: Focus articles are part of a regular series intended to sharpen understanding of current and emerging topics of interest to the scientific community. Environ. Toxicol. Chem. 37, 1235–1251. doi: 10.1002/etc.4091
Harley, C. D. G., Randall Hughes, A., Hultgren, K. M., Miner, B. G., Sorte, C. J. B., Thornber, C. S., et al. (2006). The impacts of climate change in coastal marine systems. Ecol. Lett. 9, 228–241. doi: 10.1111/j.1461-0248.2005.00871.x
Hawker, D. W., and Connell, D. W. (1988). Octanol-water partition coefficients of polychlorinated biphenyl congeners. Environ. Sci. Technol. 22, 382–387. doi: 10.1021/es00169a004
He, T., Li, Z., Sun, Q., Xu, Y., and Ye, Q. (2015). Heterotrophic nitrification and aerobic denitrification by Pseudomonas tolaasii Y-11 without nitrite accumulation during nitrogen conversion. Bioresour. Technol. 200, 493–499. doi: 10.1016/j.biortech.2015.10.064
Hedfi, A., Mahmoudi, E., Boufahja, F., Beyrem, H., and Aïssa, P. (2007). Effects of increasing levels of nickel contamination on structure of offshore nematode communities in experimental microcosms. Bull. Environ. Contam. Toxicol. 79, 345–349. doi: 10.1007/s00128-007-9261-0
Herlemann, D. P. R., Labrenz, M., Jürgens, K., Bertilsson, S., Waniek, J. J., and Andersson, A. F. (2011). Transitions in bacterial communities along the 2000 km salinity gradient of the Baltic Sea. ISME J. 5, 1571–1579. doi: 10.1038/ismej.2011.41
Hofer, B., Eltis, L. D., Dowiing, D. N., and Timmis, K. N. (1993). Genetic analysis of a Pseudomonas locus encoding a pathway for biphenyl/polychlorinated biphenyl degradation (Recombinant DNA; bph locus; nucleotide sequence; amino acid sequence comparisons; gene expression). Gene 130, 47–55. doi: 10.1016/0378-1119(93)90345-4
Huang, D., Hu, C., Zeng, G., Cheng, M., Xu, P., Gong, X., et al. (2017). Combination of Fenton Processes and Biotreatment for Wastewater Treatment and Soil Remediation. Amsterdam: Elsevier, doi: 10.1016/j.scitotenv.2016.08.199
Huson, D. H., Beier, S., Flade, I., Górska, A., El-Hadidi, M., Mitra, S., et al. (2016). MEGAN community edition – interactive exploration and analysis of large-scale microbiome sequencing data. PLoS Comput. Biol. 12:e1004957. doi: 10.1371/journal.pcbi.1004957
Inostroza, P. A., Massei, R., Wild, R., Krauss, M., and Brack, W. (2017). Chemical activity and distribution of emerging pollutants: insights from a multi-compartment analysis of a freshwater system. Environ. Pollut. 231, 339–347. doi: 10.1016/j.envpol.2017.08.015
Jahnke, A., Sobek, A., Bergmann, M., Bräunig, J., Bräunig, B., Landmann, M., et al. (2018). Emerging investigator series: effect-based characterization of mixtures of environmental pollutants in diverse sediments. Environ. Sci. Process. Impacts 20, 1667–1679. doi: 10.1039/c8em00401c
Josefsson, S., Westbom, R., Mathiasson, L., and Björklund, E. (2006). Evaluation of PLE exhaustiveness for the extraction of PCBs from sediments and the influence of sediment characteristics. Anal. Chim. Acta 560, 94–102. doi: 10.1016/j.aca.2005.12.044
Kahlon, R. S. (ed.). (2016). “Pseudomonas for industrial biotechnology,” in Pseudomonas: molecular and applied biology (New York, NY: Springer), 282–327.
Karlson, A. M. L., Nascimento, F. J. A., Näslund, J., and Elmgren, R. (2010). Higher diversity of deposit-feeding macrofauna enhances phytodetritus processing. Ecology 91, 1414–1423. doi: 10.1890/09-0660.1
Kortenkamp, A., Backhaus, T., and Faust, M. (2009). State of the art report on mixture toxicity. Contract 70307, 94–103.
Kraaij, R., Seinen, W., Tolls, J., Cornelissen, G., and Belfroid, A. C. (2002). Direct evidence of sequestration in sediments affecting the bioavailability of hydrophobic organic chemicals to benthic deposit-feeders. Environ. Sci. Technol. 36, 3525–3529. doi: 10.1021/es0102787
Kümmel, S., Herbst, F.-A., Bahr, A., Duarte, M., Pieper, D. H., Jehmlich, N., et al. (2015). Anaerobic naphthalene degradation by sulfate-reducing Desulfobacteraceae from various anoxic aquifers. FEMS Microbiol. Ecol. 91:fiv006. doi: 10.1093/femsec/fiv006
Li, N., Wania, F., Lei, Y. D., and Daly, G. L. (2003). A comprehensive and critical compilation, evaluation, and selection of physical-chemical property data for selected polychlorinated biphenyls. J. Phys. Chem. Ref. Data 32, 1545–1590. doi: 10.1063/1.1562632
Lindgren, J. F., Hassellöv, I.-M., and Dahllöf, I. (2012). Meiofaunal and bacterial community response to diesel additions in a microcosm study. Mar. Pollut. Bull. 64, 595–601. doi: 10.1016/J.MARPOLBUL.2011.12.014
Lindgren, J. F., Hassellöv, I.-M., and Dahllöf, I. (2014). PAH effects on meio- and microbial benthic communities strongly depend on bioavailability. Aquat. Toxicol. 146, 230–238. doi: 10.1016/j.aquatox.2013.11.013
Liu, S. H., Zeng, G. M., Niu, Q. Y., Liu, Y., Zhou, L., Jiang, L. H., et al. (2017). Bioremediation mechanisms of combined pollution of PAHs and heavy metals by bacteria and fungi: a mini review. Bioresour. Technol. 224, 25–33. doi: 10.1016/j.biortech.2016.11.095
Löf, M., Sundelin, B., Bandh, C., and Gorokhova, E. (2016). Embryo aberrations in the amphipod Monoporeia affinis as indicators of toxic pollutants in sediments: a field evaluation. Ecol. Indic. 60, 18–30. doi: 10.1016/j.ecolind.2015.05.058
Lotufo, G. R. (1998). Lethal and sublethal toxicity of sediment-associated fluoranthene to benthic copepods: application of the critical-body-residue approach. Aquat. Toxicol. 44, 17–30. doi: 10.1016/S0166-445X(98)00071-X
Louati, H., Said, O. B., Soltani, A., Cravo-Laureau, C., Duran, R., Aissa, P., et al. (2015). Responses of a free-living benthic marine nematode community to bioremediation of a PAH mixture. Environ. Sci. Pollut. Res. 22, 15307–15318. doi: 10.1007/s11356-014-3343-4
Lu, X.-Y., Zhang, T., and Fang, H. H.-P. (2011). Bacteria-mediated PAH degradation in soil and sediment. Appl. Microbiol. Biotechnol. 89, 1357–1371. doi: 10.1007/s00253-010-3072-7
Ma, Y. G., Lei, Y. D., Xiao, H., Wania, F., and Wang, W. H. (2010). Critical review and recommended values for the physical-chemical property data of 15 polycyclic aromatic hydrocarbons at 25 °C. J. Chem. Eng. Data 51, 819–825. doi: 10.1021/je900477x
Mackay, D., Arnot, J. A., Petkova, E. P., Wallace, K. B., Call, D. J., Brooke, L. T., et al. (2009). The physicochemical basis of QSARs for baseline toxicity. SAR QSAR Environ. Res. 20, 393–414. doi: 10.1080/10629360902949153
Mackay, D., Arnot, J. A., Wania, F., and Bailey, R. E. (2011). Chemical activity as an integrating concept in environmental assessment and management of contaminants. Integr. Environ. Assess. Manag. 7, 248–255. doi: 10.1002/ieam.139
Mayer, P., and Reichenberg, F. (2006). Can highly hydrophobic organic substances cause aquatic baseline toxicity and can they contribute to mixture toxicity? Environ. Toxicol. Chem. 25, 2639–2644. doi: 10.1897/06-142R.1
McMurdie, P. J., and Holmes, S. (2013). phyloseq: an R package for reproducible interactive analysis and graphics of microbiome census data. PLoS One 8:e61217. doi: 10.1371/journal.pone.0061217
Modig, H., and Ólafsson, E. (1998). Responses of Baltic benthic invertebrates to hypoxic events. J. Exp. Mar. Bio. Ecol. 229, 133–148. doi: 10.1016/S0022-0981(98)00043-4
Moreno, M., Ferrero, T. J., Gallizia, I., Vezzulli, L., Albertelli, G., and Fabiano, M. (2008a). An assessment of the spatial heterogeneity of environmental disturbance within an enclosed harbour through the analysis of meiofauna and nematode assemblages. Estuar. Coast. Shelf Sci. 77, 565–576. doi: 10.1016/J.ECSS.2007.10.016
Moreno, M., Vezzulli, L., Marin, V., Laconi, P., Albertelli, G., and Fabiano, M. (2008b). The use of meiofauna diversity as an indicator of pollution in harbours. ICES J. Mar. Sci. 65, 1428–1435. doi: 10.1093/icesjms/fsn116
Morton, J. T., Marotz, C., Washburne, A., Silverman, J., Zaramela, L. S., Edlund, A., et al. (2019). Establishing microbial composition measurement standards with reference frames. Nat. Commun. 10:2719.
Müller, J. A., Galushko, A. S., Kappler, A., and Schink, B. (1999). Anaerobic degradation of m-cresol by Desulfobacterium cetonicum is initiated by formation of 3-hydroxybenzylsuccinate. Arch. Microbiol. 172, 287–294. doi: 10.1007/s002030050782
Mustajärvi, L., Eek, E., Cornelissen, G., Eriksson-Wiklund, A. K., Undeman, E., and Sobek, A. (2017a). In situ benthic flow-through chambers to determine sediment-to-water fluxes of legacy hydrophobic organic contaminants. Environ. Pollut. 231, 854–862. doi: 10.1016/j.envpol.2017.08.086
Mustajärvi, L., Eriksson-Wiklund, A.-K., Gorokhova, E., Jahnke, A., and Sobek, A. (2017b). Transferring mixtures of chemicals from sediment to a bioassay using silicone-based passive sampling and dosing. Environ. Sci. Process. Impacts 19, 1404–1413. doi: 10.1039/C7EM00228A
Nascimento, F. J. A., Lallias, D., Bik, H. M., and Creer, S. (2018). Sample size effects on the assessment of eukaryotic diversity and community structure in aquatic sediments using high-throughput sequencing. Sci. Rep. 8:11737. doi: 10.1038/s41598-018-30179-1
Nascimento, F. J. A., Näslund, J., Elmgren, R., Naslund, J., and Elmgren, R. (2012). Meiofauna enhances organic matter mineralization in soft sediment ecosystems. Limnol. Oceanogr. 57, 338–346. doi: 10.4319/lo.2012.57.1.0338
Nizzetto, L., Macleod, M., Borgå, K., Cabrerizo, A., Dachs, J., Di Guardo, A., et al. (2010). Past, present, and future controls on levels of persistent organic pollutants in the global environment. Environ. Sci. Technol. 44, 6526–6531. doi: 10.1021/es100178f
Nyyssönen, M., Piskonen, R., and Itävaara, M. (2006). A targeted real-time PCR assay for studying naphthalene degradation in the environment. Microb. Ecol. 52, 533–543. doi: 10.1007/s00248-006-9082-4
Obi, C. C., Adebusoye, S. A., Ugoji, E. O., Ilori, M. O., Amund, O. O., and Hickey, W. J. (2016). Microbial communities in sediments of Lagos Lagoon, Nigeria: elucidation of community structure and potential impacts of contamination by municipal and industrial wastes. Front. Microbiol. 7:1213. doi: 10.3389/fmicb.2016.01213
Oksanen, J. (2015). Multivariate Analysis of Ecological Communities in R: Vegan Tutorial. doi: 10.1016/0169-5347(88)90124-3
Park, J. W., and Crowley, D. E. (2006). Dynamic changes in nahAc gene copy numbers during degradation of naphthalene in PAH-contaminated soils. Appl. Microbiol. Biotechnol. 72, 1322–1329. doi: 10.1007/s00253-006-0423-5
Porubsky, W. P., Weston, N. B., and Joye, S. B. (2009). Benthic metabolism and the fate of dissolved inorganic nitrogen in intertidal sediments. Estuar. Coast. Shelf Sci. 83, 392–402. doi: 10.1016/j.ecss.2009.04.012
Quero, G. M., Cassin, D., Botter, M., Perini, L., and Luna, G. M. (2015). Patterns of benthic bacterial diversity in coastal areas contaminated by heavy metals, polycyclic aromatic hydrocarbons (PAHs) and polychlorinated biphenyls (PCBs). Front. Microbiol. 6:1053. doi: 10.3389/fmicb.2015.01053
Quinn, T., Erb, I., Gloor, G., Notredame, C., Richardson, M. F., and Crowley, T. M. (2019). A field guide for the compositional analysis of any-omics data. Giga Sci. 8:giz107.
Reichenberg, F., Smedes, F., Jönsson, J. -Å, and Mayer, P. (2008). Determining the chemical activity of hydrophobic organic compounds in soil using polymer coated vials. Chem. Cent. J. 2:8. doi: 10.1186/1752-153X-2-8
Ridgway, H. F., Safarik, J., Phipps, D., Carl, P., and Clark, D. (1990). Identification and catabolic activity of well-derived gasoline-degrading bacteria from a contaminated aquifer. Appl. Environ. Microbiol. 56, 3565–3575. doi: 10.1128/aem.56.11.3565-3575.1990
Rockne, K. J., Chee-Sanford, J. C., Sanford, R. A., Hedlund, B. P., Staley, J. T., and Strand, S. E. (2000). Anaerobic naphthalene degradation by microbial pure cultures under nitrate-reducing conditions. Appl. Environ. Microbiol. 66, 1595–1601. doi: 10.1128/AEM.66.4.1595-1601.2000
Romero, M. C., Cazau, M. C., Giorgieri, S., and Arambarri, A. M. (1998). Phenanthrene degradation by microorganisms isolated from a contaminated stream. Environ. Pollut. 101, 355–359. doi: 10.1016/S0269-7491(98)00056-6
Rotthauwe, J. H., Witzel, K. P., and Liesack, W. (1997). The ammonia monooxygenase structural gene amoA as a functional marker: molecular fine-scale analysis of natural ammonia-oxidizing populations. Appl. Environ. Microbiol. 63, 4704–4712.
Schratzberger, M., and Ingels, J. (2018). Meiofauna matters: the roles of meiofauna in benthic ecosystems. J. Exp. Mar. Bio. Ecol. 502, 1–14. doi: 10.1016/j.jembe.2017.01.007
Schratzberger, M., and Warwick, R. M. (1998). Effects of physical disturbance on nematode communities in sand and mud: a microcosm experiment. Mar. Biol. 130, 643–650. doi: 10.1007/s002270050286
Schwarzenbach, R. P., Gschwend, P. M., and Imboden, D. M. (2002). Environmental Organic Chemistry. Hoboken, NJ: John Wiley & Sons, Inc, doi: 10.1002/0471649643
Sheppard, C. (2006). The muddle of’biodiversity’. Mar. Pollut. Bull. 52:123. doi: 10.1016/j.marpolbul.2005.12.004
Silva, E., Rajapakse, N., and Kortenkamp, A. (2002). Something from “Nothing” - eight weak estrogenic chemicals combined at concentrations below NOECs produce significant mixture effects. Environ. Sci. Technol. 36, 1751–1756. doi: 10.1021/ES0101227
Silva, S. J., Carman, K. R., Fleeger, J. W., Marshall, T., and Marlborough, S. J. (2009). Effects of phenanthrene- and metal-contaminated sediment on the feeding activity of the harpacticoid copepod, Schizopera knabeni. Arch. Environ. Contam. Toxicol. 56, 434–441. doi: 10.1007/s00244-008-9197-3
Slootweg, R., Rajvanshi, A., Mathur, V. B., and Kolhoff, A. (2009). Biodiversity in Environmental Assessment: Enhancing Ecosystem Services for Human Well-Being (Ecology, Biodiversity and Conservation). Cambridge: Cambridge University Press.
Smith, K. E. C., Schmidt, S. N., Dom, N., Blust, R., Holmstrup, M., and Mayer, P. (2013). Baseline toxic mixtures of non-toxic chemicals: “solubility addition” increases exposure for solid hydrophobic chemicals. Environ. Sci. Technol. 47, 2026–2033. doi: 10.1021/es3040472
Snelgrove, P. V. R. (1999). Getting to the bottom of marine biodiversity: sedimentary habitats ocean bottoms are the most widespread habitat on Earth and support high biodiversity and key ecosystem services. Bioscience 49, 129–138. doi: 10.2307/1313538
Somerfield, P. J., Gee, J. M., and Warwick, R. M. (1994). Soft sediment meiofaunal community structure in relation to a long-term heavy metal gradient in the Fal Estuary system. Mar. Ecol. Prog. Ser. 105, 79–88. doi: 10.3354/meps105079
Starke, R., Keller, A., Jehmlich, N., Vogt, C., Richnow, H. H., Kleinsteuber, S., et al. (2016). Pulsed 13C2-acetate protein-SIP unveils epsilonproteobacteria as dominant acetate utilizers in a sulfate-reducing microbial community mineralizing benzene. Microb. Ecol. 71, 901–911. doi: 10.1007/s00248-016-0731-y
Stoeck, T., Bass, D., Nebel, M., Christen, R., Jones, M. D. M., Breiner, H. W., et al. (2010). Multiple marker parallel tag environmental DNA sequencing reveals a highly complex eukaryotic community in marine anoxic water. Mol. Ecol. 19, 21–31. doi: 10.1111/j.1365-294X.2009.04480.x
Sundbäck, K., Linares, F., Larson, F., Wulff, A., and Engelsen, A. (2004). Benthic nitrogen fluxes along a depth gradient in a microtidal fjord: The role of denitrification and microphytobenthos. Limnol. Oceanogr. 49, 1095–1107. doi: 10.4319/lo.2004.49.4.1095
Wang, J., Wu, Y., Jiang, H., Li, C., Dong, H., Wu, Q., et al. (2008). High beta diversity of bacteria in the shallow terrestrial subsurface. Environ. Microbiol. 10, 2537–2549. doi: 10.1111/j.1462-2920.2008.01678.x
Wang, Y., Chen, H., Chen, H., and Wu, J. (2009). Influences of chronic contamination of oil field exploitation on soil nematode communities at the Yellow River Delta of China. Front. Biol. China 4:376–383. doi: 10.1007/s11515-009-0030-9
Wasi, S., Tabrez, S., and Ahmad, M. (2011). Suitability of Immobilized Pseudomonas fluorescens SM1 Strain for remediation of phenols, heavy metals, and pesticides from water. Water Air Soil Pollut. 220, 89–99. doi: 10.1007/s11270-010-0737-x
Wasi, S., Tabrez, S., and Ahmad, M. (2013). Use of Pseudomonas spp. for the bioremediation of environmental pollutants: a review. Environ. Monit. Assess. 185, 8147–8155. doi: 10.1007/s10661-013-3163-x
Wright, E. S., Yilmaz, L. S., and Noguera, D. R. (2012). DECIPHER, a search-based approach to chimera identification for 16S rRNA sequences. Appl. Environ. Microbiol. 78, 717–725. doi: 10.1128/AEM.06516-11
Zeppilli, D., Sarrazin, J., Leduc, D., Arbizu, P. M., Fontaneto, D., Fontanier, C., et al. (2015). Is the meiofauna a good indicator for climate change and anthropogenic impacts? Mar. Biodivers. 45, 505–535. doi: 10.1007/s12526-015-0359-z
Keywords: non-specific toxicity, meiobenthos, microbial, metabarcoding, polychlorinated biphenyl, polycyclic aromatic hydrocarbons
Citation: Iburg S, Nybom I, Bonaglia S, Karlson AML, Sobek A and Nascimento FJA (2020) Organic Contaminant Mixture Significantly Changes Microbenthic Community Structure and Increases the Expression of PAH Degradation Genes. Front. Environ. Sci. 8:128. doi: 10.3389/fenvs.2020.00128
Received: 13 March 2020; Accepted: 16 July 2020;
Published: 18 August 2020.
Edited by:
C. Marjorie Aelion, University of Massachusetts Amherst, United StatesReviewed by:
Bhabananda Biswas, University of South Australia, AustraliaFranck Lejzerowicz, University of California, San Diego, United States
Copyright © 2020 Iburg, Nybom, Bonaglia, Karlson, Sobek and Nascimento. This is an open-access article distributed under the terms of the Creative Commons Attribution License (CC BY). The use, distribution or reproduction in other forums is permitted, provided the original author(s) and the copyright owner(s) are credited and that the original publication in this journal is cited, in accordance with accepted academic practice. No use, distribution or reproduction is permitted which does not comply with these terms.
*Correspondence: Sven Iburg, sven.iburg@su.se; Francisco J. A. Nascimento, Francisco.nascimento@su.se