Does Carbon Emissions, and Economic Expansion Induce Health Expenditure in China: Evidence for Sustainability Perspective
- 1School of Economics and Management, Fuzhou University of International Studies and Trade, Fuzhou, China
- 2School of Economics, Shandong Technology and Business University, Yantai, China
- 3Graduate school of Economics and Management, Ural Federal University, Yekaterinburg, Russia
- 4Department of Economics, Faculty of Economics, Administrative and Social Sciences, Nisantasi University, Istanbul, Turkey
The current paper assesses the drivers of health care expenditure such as urbanization, natural resources, economic expansion, and CO2 utilizing quarterly data from 2000Q1 to 2018Q4. The research applied the novel dual adjustment approach to identify the long run association between healthcare expenditure and urbanization, economic growth, natural resource and CO2 emissions. The main novelty of the dual adjustment approach is that the approach offers another way to cointegration analysis by relaxing the implicit assumption of the singular adjustment in cointegration analysis. The outcome of the dual adjustment approach affirmed cointegration among the variables in the long run. Furthermore, we applied fully modified ordinary least square (FMOLS), dynamic ordinary least square (DOLS) and canonical cointegrating regression (CCR) estimators and their results disclosed that economic growth, urbanization, and CO2 emissions increase health care expenditure while natural resource rent mitigates healthcare expenditure in China. Moreover, the spectral causality test uncovered that urbanization, economic growth, natural resource, and CO2 emissions can predict healthcare expenditure at various frequencies. Based on these findings, China’s policymakers should establish strategic environmental management policies that improve healthy and clean air to reduce healthcare costs. In addition, policymakers in China should reevaluate their urban development strategies to avoid negative externalities associated with fast urbanization.
Introduction
The pursuit of economic progress fueled by high amounts of energy usage has resulted in an increase in air pollution, which is hazardous to human health (Shahzad et al., 2020; Adebayo et al., 2021a; Güngör et al., 2021; Adebayo et al., 2021b). For several nations, developing a responsive health system to combat the growing health implications of environmental degradation is critical. All emerging countries such as China want to build a strong healthcare system, but rising health spending is eating up a large portion of their gross domestic product (Doğan et al., 2021; Fareed et al., 2021; Ghazouani et al., 2021; Shah et al., 2021). As a result, (Zaidi and Saidi, 2018) pointed out that the alarming rate at which health expenditures are rising is outpacing GDP growth in several economies, particularly in the emerging economies. According to (UNEP, 2016), emerging nations spend around 5% of their GDP on health-linked expenses caused by pollution (UNEP, 2016). As a result, the goal of universal healthcare access is jeopardized due to rising healthcare expenses (Atuahene et al., 2020; Yang et al., 2021; Zeeshan et al., 2021).
China’s health spending per capita has risen from less than US$42.11in 2000 to more than US$501 in 2019 (See Figure 1). The explanations for this expansion are numerous. The most significant factors are the rising ageing population and standard of living. China’s health expenditure per capita and total health expenditure has risen rapidly since 1978. The total health expenditure was roughly 3% of GDP in 1978, and by the 1990s, it had risen to around 4% of GDP (World Bank, 2021). Since the Chinese healthcare reform commenced in 2009, the rate has risen to over 5%, reaching 6% in 2016.
In the empirical literature, there are various factors such as income, energy use, CO2 emissions, population, economic growth, and health status of citizens and many others that can influence healthcare expenditure in various nations. For example, (Chaabouni et al., 2016) scrutinized the drivers of health expenditures in 51 nations and their findings unveiled that an upsurge in GDP contributes to health expenditures. Similarly, (Yang et al., 2021) assessed the drivers of healthcare expenditure based on the STIRPAT framework from 1995 to 2018. The authors employed AMG and CCEMG approaches to assess this connection and their outcomes disclosed that urbanization, industrialization, ecological footprint, and economic expansion triggers health expenditures while globalization mitigates health expenditure. Likewise, (Ampon-Wireko et al., 2021) applied the PMG, panel causality and DOLS approaches to assess the drivers of healthcare expenditure in emerging nations. Their outcomes show that natural resources, urbanization, economic growth and CO2 increase healthcare spending in addition, there is a feedback causal interrelationship between economic expansion and health spending. The literature pertinent to this work offers limited insight on drivers of healthcare expenditure such as income, urbanization, natural resource extraction, and CO2 emissions. As a result, we investigate the interrelationship between health spending and its determinants (economic growth, CO2, urbanization, and natural resources) in China, which is the most populated country in the world.
Examining the relationships between these variables in China has become extremely relevant from a policy standpoint. In the absence of effective national policies, healthcare costs and the resulting deprivation of people are expected to rise, necessitating the urgency for this research. Moreover, natural resource exploitation caused health threats because of the numerous chemicals disposed at mining sites, which has not gotten the recognition it deserves in terms of human healthcare. The current paper differentiates itself by offering a vigorous analysis of the combined impact of economic growth, urbanization, natural resource, and CO2 on healthcare expenditure in China within the framework of advanced econometric approaches such as the dual adjustment method. The main novelty of the dual adjustment approach is that the approach offers another way to cointegration analysis by relaxing the implicit assumption of the singular adjustment in cointegration analysis. In addition, we applied spectral causality, fully modified ordinary least square (FMOLS), dynamic ordinary least square (DOLS) and canonical cointegrating regression (CCR) estimators to assess these interconnections.
The subsequent section of the research is as follows: Section 2 illustrates a summary of past studies which is followed by methodology and data in Section 3. Findings and discussion are presented in Section 4. The research concludes in Section 5.
Synopsis of Related Studies
Health spending encompasses all expenditures for the health services provision, nutrition activities, health-related emergency assistance and family planning activities, but it omits the provision of sanitation and drinkable water. Increased pollution of the environment, the spread of diseases, income disparities and overcrowding in cities in emerging and developed nations have drawn policymakers’ and researchers’ attention to the drivers of healthcare expenditures. Over the years, several studies (Chaabouni et al., 2016; Chaabouni and Saidi, 2017; Haseeb et al., 2018; Zaidi and Saidi, 2018; Shahzad et al., 2020) have been conducted on the drivers of health expenditure; however, findings have produced mixed results. For instance, utilizing a dataset from 1995 to 2013, (Chaabouni et al., 2016) assessed the determinants of health expenditures in 51 nations. The investigators utilized GMM estimator and panel causality to uncover the interrelationship and their findings uncovered that a rise in CO2 contributes to health expenditures in the selected nations.
Moreover, using China and India, (Atuahene et al., 2020) scrutinized the nexus between health expenditure, GDP and CO2 utilizing dataset between 1960 and 2019 and GMM estimator. Their outcomes uncovered that CO2, GDP and health spending all have a strong interconnection. For both nations, the empirical research unveiled that CO2 has a considerable positive influence on health spending, but economic expansion has a detrimental effect on health expenditure during the research period. In addition, no significant connection exists between urbanization and health expenditure in India while in China, urbanization impacts health expenditure positively. Likewise, the research of (Zeeshan et al., 2021) on the asymmetric nexus CO2 and health expenditure in China from 1990 to 2019 disclosed that positive (negative) shock in CO2 impacts health expenditure positively (negatively). Using 10 nations with the highest healthcare expenditures, (Yang et al., 2021) assessed the drivers of healthcare expenditure based on the STIRPAT framework from 1995 to 2018. The authors employed AMG and CCEMG approaches to assess this connection and their outcomes disclosed that urbanization, industrialization, ecological footprint, and economic expansion triggers health expenditures positively while globalization mitigates health expenditure.
Some studies have also incorporated natural resources into their model to assess drivers of healthcare expenditure. For example, using a dataset from 2000 to 2018, (Ampon-Wireko et al., 2021) applied the PMG, panel causality and DOLS approaches to assess the drivers of healthcare expenditure in emerging nations. Their outcomes showed that natural resources, urbanization, economic growth and CO2 increase healthcare spending. In addition, there is a feedback causal interrelationship between economic expansion and healthcare spending. Likewise, the research of (Nikzadian et al., 2019) for OPEC nations on the nexus between health care expenditure and natural resources using a dataset from 2002 to 2015 and panel OLS reported that natural resources impact healthcare expenditure positively. Similarly, the study of (Wang et al., 2019) using a dataset from 1975 to 2017 and bootstrap ARDL for the case of 18 OECD nations unveiled a long-run association among CO2, economic expansion and health expenditure. In addition, the authors observed feedback causal interconnectedness between healthcare expenditure and CO2 in New Zealand and Norway.
Based on the summarized studies above, there is no agreement on the impact of economic growth, urbanization, natural resource, and CO2 on healthcare expenditure. Thus, the current paper differentiates itself by offering a vigorous analysis of the combined impact of economic growth, urbanization, natural resource, and CO2 on healthcare expenditure in China within the framework of advanced econometric approaches such as the dual adjustment approach. The main innovation of this method is that it offers an option for analysis of cointegration by reducing the implicit assumption of a single modification. In addition, we applied spectral causality, DOLS, CCR and FMOLS approach to assess these interconnections. Investigating the combinations of various strategies serves to unlock the originality of the techniques, resulting in reliable estimates that assist effective policy decisions.
Data and Methodology
Data and Model
In this research, we assess the impact of economic expansion (GDP) on healthcare expenditure (HE) in China. We also consider other drivers of health expenditure (HE) such as urbanization (URB), carbon emissions (CO2) and natural resources (NRR). The dataset utilized in the research span between 2001Q1 and 2018Q4 (76 observations). The dependent variable is health expenditure which is measured as domestic general government health expenditure (% of GDP). The independent variables are economic growth which is calculated as GDP per capita (constant US$ 2010), natural resource which is calculated as total natural resources rents (% of GDP), CO2 emissions are calculated as metric tons per capita and urbanization which is measured as Urban population (% of total population). The data for CO2, HE, GDP, NRR and URB are gathered from World Bank database. The research uses the natural logarithmic version of all indicators. The research econometric model is presented in Eq. 1 as follows:
Where, CO2, HE, GDP, NRR and URB stand for emissions of CO2, health expenditure, economic expansion, natural resource and urbanization. Furthermore, t and
Methodology
Unit Root Tests
The current paper aims to evaluate the long-run influence of economic expansion, CO2 emissions, natural resources, urbanization and urbanization on health expenditure in China. Nevertheless, it is vital to capture the stationarity attributes of the variables of investigation before assessing the long-run association. As a result, KPSS unit root test was initiated by (Kwiatkowski et al., 1992) to assess the variables' stationarity attribute. Nevertheless, with a structural break(s), the effectiveness of conventional methods such as KPSS decreases. As a result, the Zivot-Andrews (ZA) test proposed by (Zivot and Andrews, 2002) which considers a single break is used in this research. The time of structural break is determined endogenously in the ZA unit root test. Each series is allowed one structural break in the ZA test. One break in a series (Xt) is endogenized in the ZA model as follows:
Dual Adjustment Approach
The present study employs the dual adjustment approach which proposed by İsmihan. (2019). The benchmark case for the dual adjustment approach is the standard Engle-Granger (EG) cointegration method. Hence, this study uses both dual adjustment approach and EG cointegration approach. The main novelty of the dual adjustment approach is that the method gives an alternative to the cointegration analysis relaxing the implicit assumption of the singular adjustment in cointegration analysis. As advised by White and Granger (2011) and İsmihan. (2019), we follow the indirect method and use the Hodrick-Prescott (HP) filter to measure the trend and permanent component of the variables. Scilicet, HP is the favored method of decomposition in current analysis for numerous motives mentioned previously but this does not certainly mean that it is superior to others. Thus, the dual adjustment approach permits the present study to use other popular filters which are Baxter-King filter (BK) (suggested by (Baxter and King, 1999)) Ravn and Uhlig (RU) and Christiano and Fitzgerald (CF) filter (suggested by Christiano and Fitzgerald, 2003).
Following İsmihan. (2019), by employing the step-wise methodology of dual adjustment approach, the first phase in the dual adjustment approach is to decompose the variable in two distinct components. The HP approach is superior method for decomposition. However, by utilizing the HP method, the choice of smoothing parameter is the most important step. The lambda value for frequency of data is 100 in case of HP approach. However, RU proposes a value of 6.25 in the case of annual data. Hence, this study uses two different values of lambda, i.e., 100 and 6.25. The study also uses BK and CF for the choice of the smoothing parameter. Moreover, the study uses the critical values of EG approach in the case of Co-HP trend analysis. The Co-HP tend analysis has two steps. The following equation is estimated via OLS method first step:
Where,
Where
Where,
Long-Run Estimators (FMOLS, DOLS and DOLS)
Fully modified OLS (FMOLS) is one of the most viable choices for estimating long-run elasticities because it adjusts for autocorrelation and endogeneity issues in the data series (Kirikkaleli and Adebayo, 2020; Adebayo et al., 2021c). This method, developed by (Pedroni, 2001), is centered on the non-parametric method and is well-suited to achieving reliable outcomes in small samples. The FMOLS estimator is depicted as follows.
Where, the standard FMOLS estimator is
The distinctive association between the independent and dependent variables in this research was uncovered using this statistical method. To assess the FMOLS outputs’ robustness, the DOLS and CCR long-run estimators were used.
Breitung and Candelon Causality
The DOLS, CCR and FMOLS approaches can only assess the interrelationship between independent and dependent variables. As a result, we applied the Breitung and Candelon (BC) Causality test to identify the causal effect of CO2 emissions, natural resource, GDP and urbanization on health expenditure in China. According to (Alola et al., 2021) and (Adebayo and Rjoub, 2021), the main difference between the time-domain and frequency-domain methods is that the time-domain method notifies us where a particular adjustment appears within a time series, whilst the frequency-domain method tests the degree of a particular variance in the series. The BC uncovers causality at dissimilar frequencies.
Findings and Discussion
Pre-Estimation Outcomes
Table 1 displays the statistics summary for the studied variables. The mean of GDP (4361.5) is the highest which ranges from 1723.5 to 7984.3. This is accompanied by URB (47.707) which ranges from 35.446 to 59.592, CO2 (5.3817) which ranges from 2.5775 to 7.0459, NRR (2.3677) which ranges from 0.5718 to 5.9863 and HE (1.9947) which ranges from 0.9493 to 3.0725. The skewness value unveiled that CO2 and URB are negatively skewed while GDP, HE, and NRR are positively skewed. The value of the kurtosis further disclosed that all the variables (CO2, HE, GDP, NRR and URB) are leptokurtic.
In this research, we applied KPSS unit root test to identify the stationarity attribute of HE, GDP, URB, NRR and CO2. Table 2 reports the KPSS outcomes, and the result unveiled that HE, GDP, CO2, NRR and URB are stationary at level. Nevertheless, with a structural break(s), the effectiveness of conventional methods such as KPSS decreases. As a result, the Zivot-Andrews (ZA) test which considers a single break is used in this research. Table 2 reports the ZA outcomes and the result uncovered that all the variables are I(1) with HE, GDP, CO2, NRR and NRR having a break in 2010Q2, 2007Q2, 2011Q2, 2014Q2 and 2005Q2 respectively.
Dual Adjustment Approach Outcomes
The dual adjustment method is used in this investigation. We begin by checking for the existence of Co-HP Trend. The null hypothesis of “no common HP trend” is overruled in all cases, i.e. with four separate smoothing factors. The DAA outcome is reported in Table 3. In all cases, there is proof of long-run interrelationship between health expenditure and economic growth, natural resource, urbanization, and CO2 emissions as disclosed by both Co-HP trend and Engle-Granger CI respectively. The RU, HP, CF and BK smoothing parameters are used to examine the Co-HP trend. Moreover, the Engle-Granger and Co-HP trend cointegration outcomes are comparable. We dismissed the null hypothesis of “no cointegration” as a result of significant values of EG/Co-HPw. As a result, there is a consistent HP trend in all HP, RU, BK, and CF in all cases. The outcomes affirm that health expenditure is stationary around a common part of economic growth, CO2 emissions, natural resource, and urbanization (Co-HP trend).
The outcomes of the λBK, λRU, λCF and λHP discloses that economic growth, and CO2 emissions impact health expenditure positively in the long run. This infers that an upsurge in economic growth, urbanisation and CO2 contribute to an upsurge in health expenditure in China. Furthermore, we observed that natural resources rent impact health expenditure negatively in China. This demonstrates that an upsurge in natural resources rent mitigate health spending in China. In the short run, λHP and λRU outcomes show that CO2 emissions impact health expenditure positively. Furthermore, we observed a negative connection between economic growth and health expenditure as unveiled by λBK, λRU, λCF and λHP respectively. Moreover, we noticed negative interconnectedness between health expenditure and natural resources as disclosed by λHP and λRU respectively.
Long-Run Estimators (DOLS, CCR and FMOLS) Outcomes
We also utilized DOLS, CCR and FMOLS to catch the long-run association between health expenditure and its drivers (economic growth, natural resource, urbanization and CO2 emissions) in China. Table 4 reports FMOLS, DOLS and CCR outcomes. We observed positive interconnection between economic growth and health expenditure. This implies that keeping other indicators constant, a 1% upsurge in economic growth increases health expenditure by 1.3161% (FMOLS), 1.3785 (DOLS) and 1.3315% (CCR) respectively. Moreover, we established positive interconnectedness between health expenditure and CO2 in China. This demonstrates that keeping other factors constant, a 1% upsurge in CO2 increases health expenditure by 0.9454% (FMOLS), 1.9411% (DOLS) and 0.9012% (CCR) respectively. The studies of (Chaabouni et al., 2016; Chaabouni and Saidi, 2017; Shahzad et al., 2020) reported similar outcomes by establishing that economic growth and CO2 contribute to an increase in health expenditure. Economic expansion is critical for nations since it is the most reliable means of promoting social and economic development. Nevertheless, as a result of the related energy and other resources use, the pursuit of economic expansion increases CO2, resulting in increased health expenditure internationally. It underlines the fact that as a consequence of rising pollution and other ecologically linked diseases caused by economic expansion, the proportion of GDP assigned to health continues to rise. Moreover, CO2 is the key factor that has a negative impact on people’s health, according to this research. As a result, authorities should concentrate on CO2 and develop measures that will help to minimize CO2. Moreover, (Chaabouni and Saidi, 2017) noted that healthcare spending has a crucial role in stimulating a country’s economic expansion, stating that a high level of health spending may contribute to improved economies of scale, social security and effective resource allocation.
Furthermore, natural resources impact health spending negatively. This demonstrates that keeping other factors constant, 1% upsurge in natural resource rent decreases health expenditure by 4.1404% (FMOLS), 4.3055% (DOLS) and 0.1418% (CCR) respectively. The findings of the study revealed that increasing natural resource exploitation decreases health spending. This is encouraging because the impact of natural resources on health spending has received little attention in the literature. As a result, scholars will be motivated to investigate the impact of natural resources on health spending in greater depth. The study of (Shahzad et al., 2020) reported a similar finding by establishing a negative interconnection between natural resources and health expenditure. Lastly, urbanization impacts health spending negatively suggesting that keeping other factors constant, 1% upsurge in urbanization increase health expenditure by 3.667% (FMOLS), 3.861% (DOLS) and 3.725% (CCR) respectively. This finding is unsurprising given the fact that China has witnessed significant urbanization in recent years. Thereby, an increase in urban population is anticipated to increase China’s healthcare expenditure. The study of (Çetin and Bakırtaş, 2019) in developing nations reported similar findings. Moreover, the graphical findings is illustrated by Figure 2.
Breitung and Candelon Causality Outcomes
The present research applied the Breitung and Candelon (BC) to identify the causal effect of CO2, natural resource, economic growth, and urbanization on health expenditure in China. The BC uncovers causality at dissimilar frequencies. Figures 3A–D presents the outcome of the BC causality test. The broken-thick blue line depicts the Test-statistic, and red (green) thick line depicts a 5 and 10% level of significance respectively. Figure 3A presents spectral causality from economic growth to health expenditure. At 5% and 10% levels of significance, the null hypothesis of “no causality” is dismissed in the long term, which implies that economic growth can predict health expenditure in China. Moreover, Figure 3B depicts causality from natural resource to health expenditure and the outcome shows that in the long-term, at 5 and 10% level of significance, the null hypothesis of “no causality from natural resource to health expenditure” is rejected. Furthermore, we observed causality from CO2 emissions to health expenditure in the middle-term at a 10% significance level (see Figure 3C). Lastly, in the long-term and short-term, there is proof of causal linkage from urbanization to health expenditure at a significance level of 5 and 10% respectively. In summary, natural resources, economic growth, urbanization and CO2 emissions can predict health expenditure. Thus, any policy initiatives channeled towards natural resources, economic growth, urbanization and CO2 emissions will impact health expenditure.
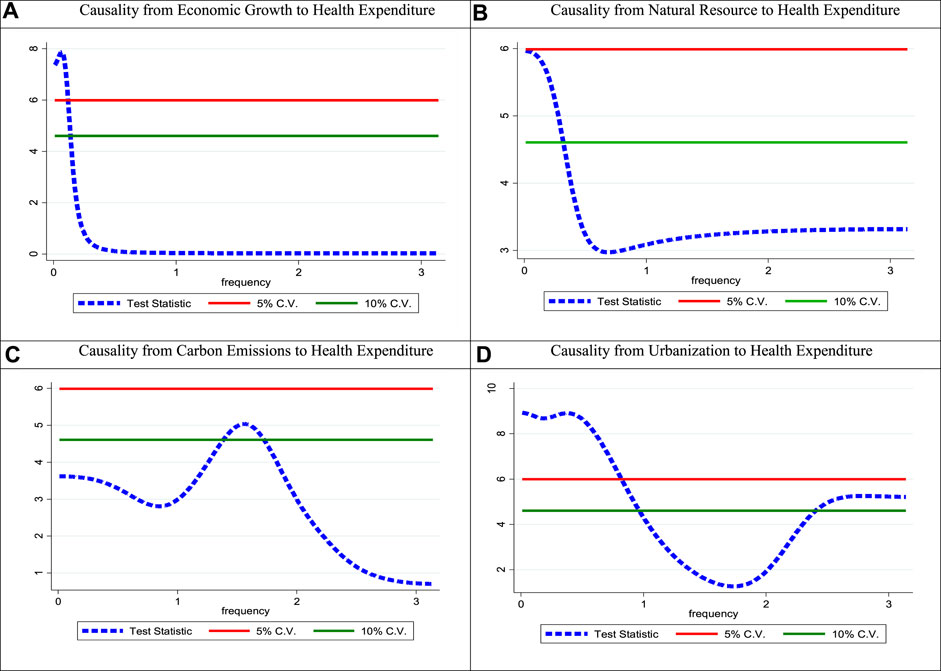
FIGURE 3. (A) causality from economic growth to health expenditure. (B) Causality from natural resource to health expenditure. (C) Causality from carbon emissions to health expenditure. (D) Causality from urbanization to health expenditure.
Conclusion and Policy Path
Conclusion
Emerging economies are increasingly confronted with healthcare difficulties as their urban populations rise at a rapid rate. Infrastructure deficiencies, inadequacies in social change, rapid urbanization, and challenges in providing healthcare services, among other issues, result in considerable economic consequences for emerging countries. Though academics and scholars have studied the causes of healthcare expenditures for several different samples since the 1970s, studies that focus on the probable effects of urbanization and natural resources on health expenditures are scant. Thus, we assess the effect of natural resources, economic growth, urbanization, and CO2 emissions on health expenditure. Moreover, the research utilizes a quarterly dataset spanning from 2000 to 2018 for the case of china. We applied both KPSS and ZA unit root tests to capture variables’ stationarity features and the outcomes show that the variables are I(1) and I(0) respectively. Moreover, we utilized the novel dual adjustment approach to scrutinize the cointegration interrelationship between the independent and dependent variables. The dual adjustment approach’s main novelty is that it gives an option to the analysis of cointegration by decreasing the implicit assumption of single adjustment. The outcomes of the dual adjustment approach affirmed cointegration among the variables. Furthermore, we utilized the FMOLS, DOLS and CCR approach to catch the long-run association and the outcomes show that economic growth, CO2 and urbanization increase health expenditure while natural resource decreases health expenditure. Lastly, BC test outcomes show that all the variables (natural sources, economic growth, urbanization and CO2 emissions at various frequencies.
Policy Suggestion
Furthermore, China should implement environmental quality-linked policies and strategies to reduce health-related ailments, as environmental quality has been linked to an increase in healthcare spending. First and foremost, policymakers in China should reassess their urbanization strategies in order to minimize the negative externalities that may result from urban expansion population increase. As a result, authorities in China should raise their investments in the sustainable restructuring of their cities’ current infrastructure. Furthermore, decision-makers should establish fiscal measures that take into account the potential effects of urban expansion. Second, officials should enact new legislative measures to prevent unsustainable migration from rural to urban regions. Lastly, the outcomes suggest that China should develop environmental management initiatives to improve clean, and healthy air in order to reduce the burden on healthcare costs. Several policy recommendations could be made based on the research’s empirical outcomes.
Data Availability Statement
Publicly available datasets were analyzed in this study. This data can be found here: https://data.worldbank.org/country/china.
Author Contributions
All authors contributed equally.
Conflict of Interest
The authors declare that the research was conducted in the absence of any commercial or financial relationships that could be construed as a potential conflict of interest.
Publisher’s Note
All claims expressed in this article are solely those of the authors and do not necessarily represent those of their affiliated organizations, or those of the publisher, the editors and the reviewers. Any product that may be evaluated in this article, or claim that may be made by its manufacturer, is not guaranteed or endorsed by the publisher.
Abbreviations
ADF, Augmented Dickey-Fuller; CCR, canonical cointegrating regression; CO2, Carbon emissions; DOLS, Dynamic Ordinary Least Square; FMOLS, fully modified ordinary least square; GHGs, Greenhouse gas emissions; HE, Health Expenditure; KPSS, Kwiatkowski–Phillips–Schmidt–Shin; NRR, Natural Resource Rent; SB, Structural Break; ZA, Zivot Andrews.
References
Adebayo, T. S., Akadiri, S. S., Adedapo, A. T., and Usman, N. (2021a). Does Interaction Between Technological Innovation and Natural Resource Rent Impact Environmental Degradation in Newly Industrialized Countries? New Evidence from Method of Moments Quantile Regression. Environ. Sci. Pollut. Res. Int., 1–8. doi:10.1007/s11356-021-17631-y
Adebayo, T. S., Oladipupo, S. D., Adeshola, I., and Rjoub, H. (2021b). Wavelet Analysis of Impact of Renewable Energy Consumption and Technological Innovation on CO2 Emissions: Evidence from Portugal. Environ. Sci. Pollut. Res. doi:10.1007/s11356-021-17708-8
Adebayo, T. S., and Rjoub, H. (2021). A New Perspective into the Impact of Renewable and Nonrenewable Energy Consumption on Environmental Degradation in Argentina: A Time-Frequency Analysis. Environ. Sci. Pollut. Res. doi:10.1007/s11356-021-16897-6
Adebayo, T. S., Rjoub, H., Akadiri, S. S., Oladipupo, S. D., Sharif, A., and Adeshola, I. (2021c). The Role of Economic Complexity in the Environmental Kuznets Curve of MINT Economies: Evidence from Method of Moments Quantile Regression. Environ. Sci. Pollut. Res. doi:10.1007/s11356-021-17524-0
Alola, A. A., Adebayo, T. S., and Onifade, S. T. (2021). Examining the Dynamics of Ecological Footprint in China with Spectral Granger Causality and Quantile-On-Quantile Approaches. Int. J. Sustain. Dev. World Ecol., 1–14. doi:10.1080/13504509.2021.1990158
Ampon-Wireko, S., Zhou, L., Xu, X., Dauda, L., Mensah, I. A., Larnyo, E., et al. (2021). The Relationship Between Healthcare Expenditure, CO2 Emissions and Natural Resources: Evidence from Developing Countries. J. Environ. Econ. Pol., 1–15. doi:10.1080/21606544.2021.1979101
Atuahene, S. A., Yusheng, K., and Bentum-Micah, G. (2020). Health Expenditure, CO2 Emissions, and Economic Growth: China vs. India. Preprints, 2020090384. doi:10.20944/preprints202009.0384.v1
Baxter, M., and King, R. G. (1999). Measuring Business Cycles: Approximate Band-Pass Filters for Economic Time Series. Rev. Econ. Stat. 81 (4), 575–593. doi:10.1162/003465399558454
Çetin, M. A., and Bakırtaş, İ. (2019). Does Urbanization Induce the Health Expenditures? A Dynamic Macro-Panel Analysis for Developing Countries. Available at: http://openaccess.dpu.edu.tr/xmlui/handle/20.500.12438/2530.
Chaabouni, S., and Saidi, K. (2017). The Dynamic Links Between Carbon Dioxide (CO2) Emissions, Health Spending and GDP Growth: A Case Study for 51 Countries. Environ. Res. 158, 137–144. doi:10.1016/j.envres.2017.05.041
Chaabouni, S., Zghidi, N., and Ben Mbarek, M. (2016). On the Causal Dynamics Between CO 2 Emissions, Health Expenditures and Economic Growth. Sustain. Cities Soc. 22, 184–191. doi:10.1016/j.scs.2016.02.001
Christiano, L. J., and Fitzgerald, T. J. (2003). The Band Pass Filter. Int. Econ. Rev 44 (2), 435–465. doi:10.1111/1468-2354.t01-1-00076
Doğan, B., Driha, O. M., Balsalobre Lorente, D., and Shahzad, U. (2021). The Mitigating Effects of Economic Complexity and Renewable Energy on Carbon Emissions in Developed Countries. Sustain. Dev. 29 (1), 1–12. doi:10.1002/sd.2125
Fareed, Z., Salem, S., Pata, U. K., and Shahzad, F. (2021). Role of export Diversification and Renewable Energy on the Load Capacity Factor in Indonesia. Front. Environ. Sci. 9, 770152. doi:10.3389/fenvs.2021.770152
Ghazouani, A., Jebli, M. B., and Shahzad, U. (2021). Impacts of Environmental Taxes and Technologies on Greenhouse Gas Emissions: Contextual Evidence from Leading Emitter European Countries. Environ. Sci. Pollut. Res. 28 (18), 22758–22767. doi:10.1007/s11356-020-11911-9
Güngör, H., Kirikkaleli, D., and Adebayo, T. S. (2021). Consumption‐based Carbon Emissions, Renewable Energy Consumption, Financial Development and Economic Growth in Chile. Business Strategy Environ. 2 (3), 33–42. doi:10.1002/bse.2945
Haseeb, A., Xia, E., DanishBaloch, M. A., Baloch, M. A., and Abbas, K. (2018). Financial Development, Globalization, and CO2 Emission in the Presence of EKC: Evidence from BRICS Countries. Environ. Sci. Pollut. Res. 25 (31), 31283–31296. doi:10.1007/s11356-018-3034-7
İsmihan, M. (2019). The Dual Adjustment Approach with an Application to the Consumption Function. Cent. Bank Rev. 19 (1), 1–8. doi:10.1016/j.cbrev.2019.02.001
Kirikkaleli, D., and Adebayo, T. S. (2020). Do renewable Energy Consumption and Financial Development Matter for Environmental Sustainability? New Global Evidence. Sustain. Dev. 29 (4), 583–594. doi:10.1002/sd.2159
Kwiatkowski, D., Phillips, P. C. B., Schmidt, P., and Shin, Y. (1992). Testing the Null Hypothesis of Stationarity Against the Alternative of a Unit Root: How Sure Are We that Economic Time Series Have a Unit Root? J. Econom. 54 (1), 159–178. doi:10.1016/0304-4076(92)90104-Y
Nikzadian, A., Agheli, L., Arani, A. A., and Sadeghi, H. (2019). The Effects of Resource Rent, Human Capital and Government Effectiveness on Government Health Expenditure in Organization of the Petroleum Exporting Countries. Int. J. Energ. Econ. Pol. 9 (2), 381–389. doi:10.32479/ijeep.7575
Pedroni, P. (2001). “Fully Modified OLS for Heterogeneous Cointegrated Panels,” in Nonstationary Panels, Panel Cointegration, and Dynamic Panels. Editors B. H. Baltagi, T. B. Fomby, and R. Carter Hill (Bingley, UK: Emerald Group Publishing Limited), 15, 93–130. doi:10.1016/S0731-9053(00)15004-2
Shah, S. M. A., Jiang, Y., Wu, H., Ahmed, Z., Ullah, I., and Adebayo, T. S. (2021). Linking Green Human Resource Practices and Environmental Economics Performance: The Role of Green Economic Organizational Culture and Green Psychological Climate. Ijerph 18 (20), 10953. doi:10.3390/ijerph182010953
Shahzad, K., Jianqiu, Z., Hashim, M., Nazam, M., and Wang, L. (2020). Impact of Using Information and Communication Technology and Renewable Energy on Health Expenditure: A Case Study from Pakistan. Energy 204, 117956. doi:10.1016/j.energy.2020.117956
UNEP (2016). UNEPUNEP DTU Clean Development Mechanism/Joint Implementation Pipeline Analysis and Database. Nairobi: United Nations Environment Program and Danish Technical University.
Wang, C.-M., Hsueh, H.-P., Li, F., and Wu, C.-F. (2019). Bootstrap ARDL on Health Expenditure, CO2 Emissions, and GDP Growth Relationship for 18 OECD Countries. Front. Public Health 7, 324. doi:10.3389/fpubh.2019.00324
White, H., and Granger, C. W. (2011). Consideration of Trends in Time Series. J. Time Ser. Econom. 3 (1), 23–36.
World Bank (2021). World Development Indicators. Available at: http://data.worldbank.org/country.
Yang, B., Usman, M., and jahanger, A. (2021). Do Industrialization, Economic Growth and Globalization Processes Influence the Ecological Footprint and Healthcare Expenditures? Fresh Insights Based on the STIRPAT Model for Countries with the Highest Healthcare Expenditures. Sustainable Prod. Consumption 28, 893–910. doi:10.1016/j.spc.2021.07.020
Zaidi, S., and Saidi, K. (2018). Environmental Pollution, Health Expenditure and Economic Growth in the Sub-saharan Africa Countries: Panel ARDL Approach. Sustain. Cities Soc. 41, 833–840. doi:10.1016/j.scs.2018.04.034
Zeeshan, M., Han, J., Rehman, A., Ullah, I., and Afridi, F. E. A. (2021). Exploring Asymmetric Nexus Between CO2 Emissions, Environmental Pollution, and Household Health Expenditure in China. Rmhp 14, 527–539. doi:10.2147/RMHP.S281729
Keywords: health expenditure, economic growth, urbanization, environmental sustainability, dual adjustment approach, China
Citation: Xiu J, Ameer W, Abbas S and Altuntaş M (2022) Does Carbon Emissions, and Economic Expansion Induce Health Expenditure in China: Evidence for Sustainability Perspective. Front. Environ. Sci. 9:838734. doi: 10.3389/fenvs.2021.838734
Received: 18 December 2021; Accepted: 21 December 2021;
Published: 17 February 2022.
Edited by:
Gagan Deep Sharma, Guru Gobind Singh Indraprastha University, IndiaReviewed by:
Magdalena Radulescu, University of Pitesti, RomaniaWenjie Zou, Fujian Normal University, China
Copyright © 2022 Xiu, Ameer, Abbas and Altuntaş. This is an open-access article distributed under the terms of the Creative Commons Attribution License (CC BY). The use, distribution or reproduction in other forums is permitted, provided the original author(s) and the copyright owner(s) are credited and that the original publication in this journal is cited, in accordance with accepted academic practice. No use, distribution or reproduction is permitted which does not comply with these terms.
*Correspondence: Waqar Ameer, waqar.ameer@yahoo.com; Shujaat Abbas, Shujaat.abbas@urfu.ru