Risk preference and willingness to participate in the futures market: Evidence from cotton and jujube enterprises of China
- 1Wu Jinglian School of Economics, Changzhou University, Changzhou, China
- 2Institute of Agricultural Economics and Development, Chinese Academy of Agricultural Sciences, Beijing, China
- 3Business School, Shandong University of Technology, Zibo, China
Since the instability and uncertainty are mounting on the international landscape, it is urgent and necessary to explore the risk awareness and risk management capabilities of leading agricultural enterprises in China. Based on a survey of 219 enterprises, we build a logit model and estimate the impact of risk preference and price risk perception of leading cotton and jujube enterprises on their willingness to participate in the futures market. We further explore the moderating effect of the price risk perception on the relationship between risk preference and willingness to participate in the futures market. Results show that the risk preference and price risk perception of leading enterprises have a significant positive impact on their willingness to participate in the futures market, and the proportion of the mediating effect in the total effect is calculated to be 35.1%. Therefore, risk management instruments suitable for various types of leading enterprises and segmentation of training courses are suggested to optimize the effectiveness of China’s cotton and jujube futures market.
Introduction
With the promotion of rural revitalization and modernization of agriculture and rural areas in China, Chinese agricultural enterprises have continually improved their asset scale and operating capacity (Chen, 2020; Duan et al., 2020; Han, 2020; Zhang et al., 2022a; Zhang et al., 2022b). Chinese agricultural enterprises have become the driving force for agricultural industrialization: they have bridged the gap between small farmers and large markets, raised farmers’ incomes, and promoted innovation in agricultural management and practices (Liu and He, 2018).
However, due to the rapid rise in local living standards and food consumption of residents and the sharp decline in tariffs on agricultural products following China’s accession to the World Trade Organization, its agricultural products have become increasingly dependent on foreign trade (Huang et al., 2004; Carter et al., 2009; Han X. et al., 2019; Xue et al., 2021). Furthermore, agricultural products in the Chinese market are more sensitive to fluctuations in international market prices, resulting in increased operational risks for leading agricultural enterprises (Martin, 2001; Veeck et al., 2020). In this paper, leading agricultural enterprises are defined as those with a relatively large scale and a strong industrial driving force.
As a result, the loss probability of such enterprises has increased sharply because of their limited ability to cope with risks (Brandt et al., 2017; Peng et al., 2020b; Peng et al., 2021a; Zhao et al., 2021). Furthermore, a severe reduction has been observed in their capacity to achieve long-term, stable, and efficient development, to protect their own interests, and to expand production scales (Peng et al., 2020a; Liu et al., 2022; Zhao et al., 2022). Recently, the production and operation of several Chinese agricultural enterprises have been severely affected due to new developments, such as the Sino-US trade friction and the COVID-19 pandemic (Han J. et al., 2019; Li et al., 2020a; Zhang et al., 2020). Therefore, it is urgent and necessary to explore the risk awareness and risk management capabilities of leading agricultural enterprises in China.
In recent years, risk management instruments have become popular among agricultural enterprises (Just and Pope, 2002; Hao, 2010). Among these, futures are the only instruments that use standardized contracts as trading objects and employ open bidding to form a highly organized and professional market operation system, thereby keeping information centralized and accessible (Antoniou and Holmes, 1995). Therefore, the price discovery function of the agricultural product futures market can be effectively brought into play. Moreover, because the futures market has risk management functions, agricultural business entities often choose to use futures to manage their risks when the price of agricultural products fluctuates significantly.
China’s futures market has also developed continuously due to marketization (Peng et al., 2021b), and futures prices are increasingly being used for agricultural risk management. Agricultural commodity futures prices have emerged as the basis for the pricing of risk-averse agricultural commodity futures in China, as well as the creation of risk-compensated futures products. Agricultural commodity futures prices have provided new means for agricultural business entities to effectively manage risk (An, 2018).
Over the last few years, researchers have been increasingly interested in the field of risky decision-making behaviour (Elahi et al., 2021b; Han et al., 2021; Elahi et al., 2022a). Previous studies have been conducted from a variety of perspectives and have produced meaningful results. For instance, Menapace et al. (2015) tested the analysis methods for the effectiveness of risk decision behaviour and confirmed that the results obtained by designing a reasonable investigation mechanism to assess the effect of risk decision-making on farmers are stable and credible. Some studies also analysed the specific influencing factors of the risk decision-making behaviour of agricultural producers and concluded that risk preference, risk perception, weather changes, natural disasters, age, and education level can exert significant impacts (Leiserowitz, 2005; Harrison et al., 2007; Abid et al., 2018; Akhtar et al., 2018; Rizwan et al., 2020). Hellerstein et al. (2013) found that risk-averse agricultural producers were less willing to choose diversified operations and unwilling to use risk management instruments. van Winsen et al. (2016) found that the risk attitude of Belgian agricultural producers had a significant impact on their risk-hedging decision-making, but the impact of risk perception was not significant.
However, relatively few studies have examined the impact of risk decision-making on agricultural business entities in China. For instance, Fang et al. (2019) shed light on farmers’ willingness to participate in the “insurance and futures” pilot program. Shang et al. (2020) investigated the relationship between farmers’ perceptions of risk and their willingness to use risk management tools. Chen and Wei (2019) examined the impact of poor farmers’ risk preferences on their risk management strategies. However, similar studies are typically conducted from the perspective of individual farmers. They did not provide analysis from the perspective of agricultural business entities. Many other studies have focused on industrial sector for estimating risk and environment nexus (Elahi et al., 2019a; Zhao et al., 2020; Zhong et al., 2020; Cheng et al., 2021; Zhong et al., 2021). Chinese leading agricultural enterprises constitute the main body of risk management in the futures market, which should be addressed in full.
The lack of research on leading agricultural enterprises has made it difficult to objectively understand the risk preference and risk management practices of leading agricultural enterprises. Therefore, it is difficult to determine the factors influencing the willingness of China’s leading enterprises to participate in the futures market from the perspective of behavioural economics. It is evident that leading agricultural enterprises are highly efficient producers, but due to the complex circumstances of the market economy, it is not yet known whether these enterprises have adequate risk management capabilities. Therefore, it is necessary to enhance enterprise risk awareness and management so that they can contribute more actively to rural revitalization and agricultural modernization.
Northwest China is the main region for the production of cotton and jujube. In this region, the Chinese government has been conducting risk training activities in-depth for agricultural operators for many years. Further, cash crops, such as cotton and jujube, are effective instruments for poverty alleviation in China (Li et al., 2020b). Therefore, to compensate for the deficiencies in existing studies, leading domestic enterprises in the cotton and jujube industries were selected for the current investigation. This study makes two primary contributions to the literature. First, based on first-hand survey data, the current situation of leading Chinese enterprises’ risk preference, price risk perception, and willingness to participate in the futures market is assessed. Second, we identify the impacts of risk preference, price risk perception, and the interaction between the two on leading enterprises’ willingness to participate in the futures market and the possible mediating effect of price risk perception.
The remainder of this article is organized as follows. First, a logit model is used to empirically test the impact of leading enterprises’ risk preference and price risk perceptions on the willingness to participate in the futures market (Elahi et al., 2018b; Elahi et al., 2019c; Elahi et al., 2021a). Second, we discuss the interaction effect between risk preference and price risk perception on leading enterprises’ willingness to participate in the futures market. Third, the possible mediating effect of leading enterprises’ price risk perception on risk preference and willingness to participate in the futures market is empirically analysed. We find that the risk preference and price risk perceptions of leading enterprises have a significant positive impact on the willingness to participate in the futures market. Their price risk perception positively moderates the relationship between risk preference and willingness to participate in the futures market. Furthermore, their price risk perception has a mediating effect on the relationship between risk preference and willingness to participate in the futures market.
Materials and methods
Theoretical framework
Research on risk preference and risk decision-making behaviour originates from the investigation of individual behaviour. Prospect theory proposes that when people make risky decisions, they should not only rely on their analysis of assumptions based on the behaviour of rational people in traditional economics, but also consider people’s cognitive biases. On this basis, risk decision theory grounded on risk preference and other uncertain conditions was introduced into economic research (Kahneman and Tversky, 1979), providing a new perspective for better analysis and interpretation of the relationship between enterprise risk preference and risk decision-making behaviour.
Existing research results indicate that the decision-making process of enterprises is usually affected by many factors, such as enterprise scale and strength, business philosophy, risk attitude, and information availability (Zhang et al., 2021). Several studies have explored the relationship between risk preference and risk taking, and have reached different conclusions, as shown in Table 1 (Weber et al., 2013; Kandasamy et al., 2014; Cohn et al., 2015; Guiso et al., 2018; König-Kersting and Trautmann, 2018; Aragó et al., 2022). The results of these studies on correlation between risk preference and risk taking, however, are inconsistent. Therefore, we propose hypothesis 1 as follows.
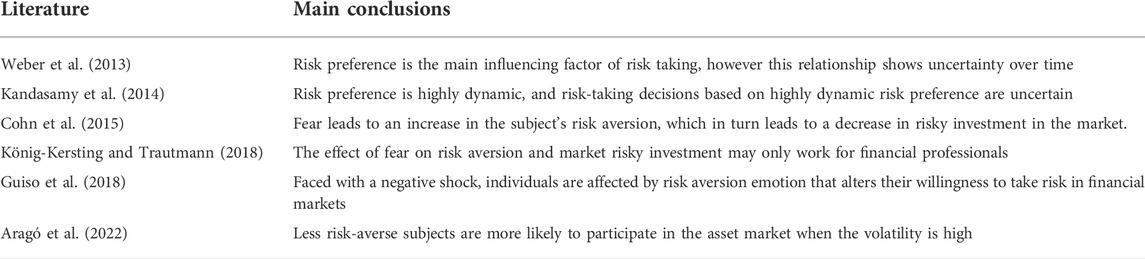
TABLE 1. Summary of research conclusions on the relationship between risk preference and willingness to take risks.
Hypothesis 1: The risk preference of leading enterprises has a significant impact on their willingness to participate in the futures market, but the direction of the impact is uncertain.
Under market economy conditions, to maximize profits, managers usually make production and operation decisions based on changes in market price signals (Ahmad et al., 2021a). In reality, enterprises are affected by various factors, such as their own perceptions, business objectives, and the external environment; thus, different enterprises often pay different levels of attention to price risk (Ahmad et al., 2021b). In this study, leading enterprises are divided into two groups: those that consider price risk as the most important risk factor and those that do not.
Hypothesis 2: Leading enterprises that regard price risk as the most important risk factor are more willing to participate in the futures market.
The recognition of possible risks by the risk subject is called risk perception (Bauer, 1960). Studies have shown that the relationship between risk preference and individual behavioural decision-making is affected by risk perception (Lv, 2014). In other words, individual behavioural decision-making is affected not only by risk preference and risk perception, but also by the interaction between the two. Based on this, risk-loving enterprises maybe also be considered more willing to participate in the futures market when leading enterprises are more sensitive to price risk.
Hypothesis 3: Price risk perception can positively moderate the impact of leading enterprises’ risk preference on their willingness to participate in the futures market.
Palich and Bagby (1995) argued that risk preference could influence the final decision through the mediation of risk perception. He et al. (2018) empirically studied farmers’ willingness to adopt water-saving irrigation technology and confirmed that risk preference could influence farmers’ decision-making through the mediation of risk perception. From the perspective of leading enterprises, since price fluctuations bring risks and opportunities, risk-loving enterprises are more concerned about price changes. When the ideal price is reached, such enterprises can choose to enter the risk management market at the right time.
Hypothesis 4: The risk preference of leading enterprises can influence their willingness to participate in the futures market through the mediation of price risk perception.
Methodology
Analysis of the influencing factors of leading enterprises’ willingness to participate in the futures market
First, a logit model is used to analyze the factors influencing leading cotton and jujube enterprises’ willingness to participate in the futures market. The logit model is one of the most widely used discrete choice models (Kuo et al., 2021), and its basic form can be written as:
where
Test of the moderating effect
If the relationship of two variables is influenced by a third variable, then the third variable is called the moderator variable (James and Brett, 1984).To test whether price risk perception can moderate the impact of the risk preference of leading cotton and jujube enterprises on their willingness to participate in the futures market, a regression analysis of the interaction effect between the price risk perception and the risk preference of enterprises is conducted, in accordance with Wen et al. (2005) conclusion. It is for the moderating effect analysis of explicit variables, when the independent variable and the moderating variable are both categorical variables.
where
Test of the mediating effect
A mediating effect model is used to explore whether the risk preference of leading cotton and jujube enterprises can influence the willingness to participate in the futures market through the mediation of price risk perception. In general, when the independent variable
First, the logit regression equation is:
where
To ensure comparability of the coefficients,
Second, by substituting Eqs 10–12 into Eqs 6–9, the estimated coefficients after standardization can be obtained, where
Finally, the product of
Source of data
The research object selected in this paper is the leading domestic cotton and jujube enterprises. The reason for not distinguishing between the two data obtained is that cotton and jujube are both representative agricultural products in western China, and the external risk environment faced by leading enterprises related to them and the various risk management trainings they have received are similar. Therefore, a comprehensive study of the two can better reflect the overall risk preference, risk perception and willingness to participate in futures of the leading enterprises related to them.
Data were collected through a field survey using a well-structured questionnaire. We distributed questionnaires from April 2019 to August 2020 through training conferences for the cotton and jujube industries held by the Zhengzhou Commodity Exchange and the China Cotton Textile Forum. The people who participated in the survey are the heads or staff of the risk management departments, and they generally have a certain degree of understanding of the futures market. Based on the different requirements of each conference, data are collected through electronic questionnaires distributed on www.wjx.cn and paper questionnaires distributed on site. A total of 238 questionnaires are distributed to leading agricultural enterprises, and 219 are collected for a questionnaire recovery rate of 92%. Representatives from middle and high-level positions are responsible for filling out the questionnaires.
Variable selection
Willingness to participate in the futures market
The willingness of leading enterprises to participate in the futures market is an important indicator that reflects the effect of financial services on agriculture, rural areas, and farmers. In recent years, China has continued to promote tasks such as “financial services for agriculture, rural areas, and farmers” and the “expansion of the ‘insurance and futures’ pilot program”, and has considered financial services for the real economy to be the focus of agricultural and rural work. By investigating leading enterprises’ willingness to participate in the futures market, an objective understanding of the current awareness of the futures market in agricultural enterprises can be obtained to test the actual effect of the above work and provide a reference for the next steps. Therefore, the willingness of leading cotton and jujube enterprises to participate in the futures market is chosen as the binary dependent variable, with ‘enterprise willing to participate in the futures market’ set to 1 and ‘enterprise unwilling to participate in the futures market’ set to 0.
Risk preference
The degree of leading enterprises’ risk preference is also a key factor affecting their willingness to participate in the futures market. The enterprises participating in the study were asked to self-evaluate their risk preference based on this categorization: 1, Risk aversion (unwilling to take any risks); 2, Willingness to bear a small amount of risk (no more than 20% loss); 3, Willingness to bear a certain amount of risk (in the range of 20–50% loss); and 4, Willingness to bear a relatively large amount of risk (more than 50% loss).
Price risk perception
The risk of price fluctuations is an important factor affecting agricultural production and operational activities. The more attention leading enterprises pay to price risk, the stronger their demand for risk management and the higher their willingness to participate in the futures market. In this study, price risk perception is used as a key binary variable that affects willingness to participate in the futures market. It takes a value of 1 if the leading enterprise considers ‘price risk as the most important risk factor’ and 0 otherwise.
Type of enterprise
The type of enterprise reflects its basic characteristics and may have an impact on its willingness to participate in the futures market. If an enterprise is engaged in only one type of business, such as planting, processing, and trading, its sensitivity to price risk is obviously high. If an enterprise is more diversified, when a certain business is affected by price fluctuations and suffers losses, the profits of other businesses can compensate; thus, the price risk sensitivity of the enterprise could be accordingly lower. Therefore, this variable takes a value of 1 for enterprises with only one type of business and 0 for enterprises with multiple types of businesses.
Enterprise size
The size of an enterprise can also affect its willingness to participate in the futures market. Large enterprises usually have sufficient funds and risk management staff; therefore, they often have a great demand for risk management and a strong willingness to participate in the futures market. In this article, leading cotton and jujube enterprises are divided into three categories based on annual revenue: enterprises with annual revenue of less than 0.5 million yuan are assigned a value of 1, and enterprises with annual revenue of 0.5 million–5 million yuan are assigned a value of 2, and enterprises with annual revenue of more than 5 million yuan are assigned a value of 3.
Recognition of futures market
Generally, the higher the enterprises’ recognition of the futures market, the stronger their willingness to participate in it. Therefore, in this study, leading enterprises’ recognition of the futures market was surveyed. If leading enterprises believe that the futures market can effectively manage the risk, the variable takes a value of 1 and 0 otherwise.
Price information acquisition method
Different ways of obtaining price information may lead to changes in the estimated willingness of leading enterprises to participate in the futures market. With the advent of the Internet, information can be obtained more efficiently and quickly. For leading enterprises, it is efficient to obtain price information through online searches; the Internet is also conducive to quickly becoming familiar with online futures trading platforms, thereby increasing their willingness to participate in the futures market. Therefore, this variable takes a value of 1 for enterprises that obtain price information through the internet and 0 for those that do so through other means.
Descriptive statistics
The questionnaire poses seven questions, and Figure 1 and Table 2 present the final survey results. First, regarding the type and size of leading cotton and jujube enterprises, most enterprises participating in this survey are engaged in only one type of business (i.e., planting, trading, or processing), accounting for more than 70%. Most are medium and large enterprises with annual revenue of more than 5 million yuan. Second, regarding the willingness of leading cotton and jujube enterprises to participate in the futures market, most enterprises are willing to participate. Because the corresponding futures products for both cotton and jujube are listed, the survey results indicate, to a certain extent, that the listed corresponding futures products can effectively promote the participation of relevant industrial enterprises in the futures market for risk management. Third, among the leading cotton and jujube enterprises that participated in this survey, only three are completely risk averse. These enterprises are completely unwilling to carry out risk management. Enterprises that can bear a certain amount of risk account for more than 50%, and those that can bear small and large amounts of risk account for 21.5 and 18.7%, respectively. Fourth, among the leading cotton and jujube enterprises, 74.8% are very concerned about price changes and believe that price risk is the most important risk factor in production and operation. The other enterprises believe that there are other risk factors that are more important. Fifth, among the leading cotton and jujube enterprises that participated in the survey, 70.8% believe that the futures market could effectively manage risks. That is, these enterprises have a high recognition of the futures market; the other enterprises have a relatively low recognition. Sixth, regarding ways to obtain price information, 82% of the leading cotton and jujube enterprises obtain information through the Internet, while only 17.8% obtain price information through other channels.
Empirical results
Using STATA15.0, a logit model is used to perform a regression analysis of the factors that affect the willingness of leading enterprises to participate in the futures market. The regression results are shown in Table 3. Regression model 1 includes price risk perception, risk preference, and other control variables; regression model 2 is formed by adding the binary interaction term of price risk perception and risk preference to regression model 1 and is used to test the existence of an interaction effect (moderating effect). The regression results for both are significant at the 1% level (Table 3). That is, the fit of the models is good. Therefore, we proceed to the next step of the analysis.
Factors influencing leading enterprises’ willingness to participate in the futures market
Regression model 1 shows the effects of all the original variables on the willingness of leading cotton and jujube enterprises to participate in the futures market. Detailed analyses of the regression results for each variable follow.
First, risk preference has a significant positive impact on the willingness of leading cotton and jujube enterprises to participate in the futures market, indicating that the more the leading enterprises are willing to spend time and energy on risk management and invest in the risk management market, the stronger their willingness to participate in the futures market. Therefore, we prove that the risk preference of leading enterprises has a significant impact on their willingness to participate in the futures market, as stated in Hypothesis 1. Further, our empirical results show that the impact is positive.
Second, the price risk perception of the leading cotton and jujube enterprises has a significant positive impact on their willingness to participate in the futures market, indicating that the leading agricultural enterprises that regard price fluctuations as the main source of risk generally have a stronger awareness of risk management and a better understanding of the risk management role of the futures market and are more willing to use financial instruments, such as futures, for risk management. Therefore, Hypothesis 2 is valid.
Third, recognition of the futures market and the information acquisition method have a significant positive impact on the willingness to participate in the futures market. The more a leading enterprise recognizes the hedging function of futures, the stronger its willingness to participate in the futures market, which is consistent with the basic logic of enterprises participating in the futures market. The information acquisition method has a significant positive influence on leading enterprises’ willingness to participate in the futures market. One possible explanation for this finding is that with the development of information technology, it is increasingly easy for enterprises to obtain commodity price information through the Internet, and futures orders also require the Internet for transmission; therefore, enterprises that often use the Internet to obtain commodity price information are more likely to participate in the futures market with the help of online instruments.
Fourth, the type and size of enterprises do not have a significant impact on the willingness of the sampled enterprises to participate in the futures market, indicating that for leading enterprises in China, the willingness to participate in the futures market is less correlated with their basic characteristics.
Moderating effect
Wen et al. (2005) showed that when both the moderating and independent variables are categorical variables and the two factors have an interaction effect (as indicated by the analysis of variance or ANOVA), the interaction effect is the moderating one. The data in Table 2 show that both the price risk perception and risk preference are categorical variables. Therefore, in regression model 2, the interaction term of price risk perception and risk preference is added to test whether the price risk perception plays a moderating role in risk preference and willingness to participate in the futures market.
The results for regression model 2 (Table 3) indicate that the regression coefficient of the interaction term between the price risk perception and risk preference is positive and significant at the 5% level; that is, the interaction between the price risk perception and risk preference has a positive effect on the willingness of leading cotton and jujube enterprises to participate in the futures market. This finding indicates that the price risk perception has a positive moderating effect on the relationship between risk preference and the willingness of leading cotton and jujube enterprises to participate in the futures market. That is, when enterprises are more sensitive to price fluctuations, the willingness of risk-loving enterprises to participate in the futures market is stronger, while that of risk-averse enterprises is further weakened. This result confirms Hypothesis 3.
To further test the robustness of the interaction effect, ANOVA is used to investigate the interaction between price risk perception and risk preference. The ANOVA results for the original variables and the interaction term of price risk perception and risk preference (Table 4) suggest that when the control variables are considered, the interaction term is significant at the 5% level, indicating that the regression results of the interaction term in model 2 are robust. Additionally, the robustness of Hypothesis 3 is verified.
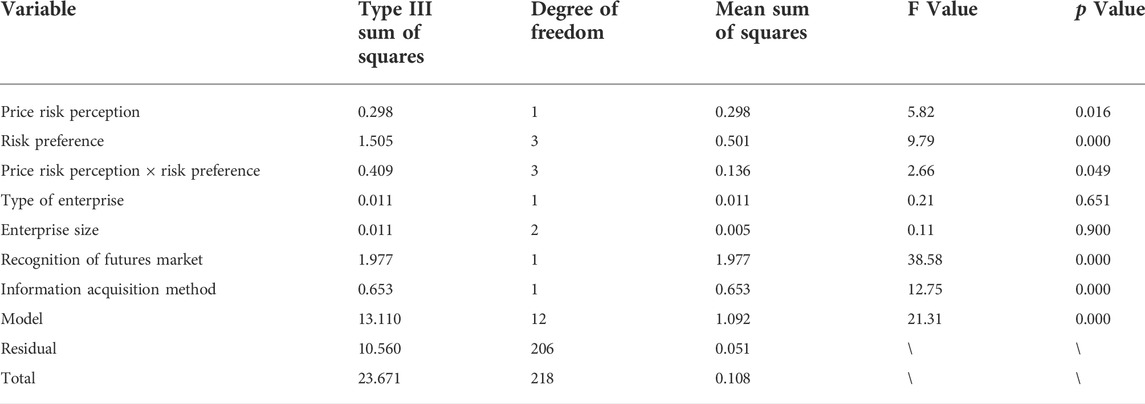
TABLE 4. ANOVA results for the moderating effect of price risk perception on risk preference and willingness to participate in futures.
Mediating effect
To investigate whether the risk preference of leading cotton and jujube enterprises can affect their willingness to participate in the futures market through the mediating role of price risk perception, stepwise regression is used; the results are shown in Table 5.
Table 5 shows that in the regression analysis of risk preference and price risk perception, and willingness to participate in the futures market, both regression coefficients for risk preference are significant at the 1% level. When risk preference and price risk perception are added to the same model for regression analysis, the regression coefficients are also significant at the 1% level, but the coefficient of risk preference after standardization decreases, indicating that price risk perception does have a mediating effect on risk preference and the willingness of leading enterprises to participate in the futures market. Therefore, Hypothesis 4 is valid. The proportion of the mediating effect in the total effect is calculated to be 35.1%, indicating that the higher the risk preference, the stronger the willingness of leading enterprises to directly participate in the futures market; accordingly, some risk-loving leading enterprises further enhance their willingness to participate in the futures market by improving their price risk perception. Thus, Hypothesis 4 is further verified.
Conclusion and policy implication
Conclusion
Based on a logit model, we analyze the impact of risk preference and price risk perception of leading cotton and jujube enterprises on their willingness to participate in the futures market and further explore the moderating effect of the price risk perception on the relationship between risk preference and willingness to participate in the futures market. Below are our main conclusions.
First, the risk preference and price risk perception of leading enterprises have a significant positive impact on their willingness to participate in the futures market. This willingness of leading enterprises increases with an increase in risk preference and price risk perception. That is, risk-averse enterprises have a relatively low willingness to participate in the futures market, while risk-loving enterprises are more willing. Additionally, enterprises that pay more attention to price risk are more willing to participate in the futures market, and those that do not pay sufficient attention to price risk are less willing.
Second, there is an interactive effect between the risk preference and price risk perception of leading enterprises; together, these factors have an impact on the willingness to participate in the futures market. The price risk perception of leading enterprises positively moderates the relationship between risk preference and willingness to participate in the futures market. Among leading enterprises that pay closer attention to price risk, risk-loving enterprises are more willing to participate in the futures market to hedge risks, while risk-averse enterprises are less inclined to do so.
Third, the price risk perception of leading enterprises has a mediating effect on their risk preference and willingness to participate in the futures market. In other words, in addition to directly affecting their willingness to participate in the futures market, the risk preference of leading enterprises can also indirectly affect this willingness through the price risk perception. In terms of degree, the proportion of the direct influence of risk preference on the willingness to participate in the futures market is approximately twice that of the indirect influence, indicating that risk-loving leading enterprises can further enhance their willingness to participate in the futures market by improving their price risk perception.
This study empirically confirms that risk preference and risk perception are critical factors in agricultural business decision-making, which is consistent with Menapace et al. (2015), Akhtar et al. (2018), and Hellerstein et al. (2013). However, there are several limitations to our study. First, this study focuses on leading agricultural enterprises. Still, the new agricultural entities include not only the leading agricultural enterprises but also different types of agricultural cooperatives and family farms in China. Second, the scope of this study is limited to two agricultural commodities, cotton and jujube, but does not consider other varieties offered in the agricultural futures marketplace. To further strengthen the robustness of the conclusions provided by this study, a broader category of future varieties should be detailed in future research. Future studies are also recommended to investigate whether other agricultural business entities, such as agricultural cooperatives and family farms, have similar risk perceptions, risk preferences, and risk decision-making relationships.
Policy implication
First, the risk preference and risk perception of leading enterprises are heterogeneous, and policy should target and select risk management instruments for training and promotion. The results of this study indicate that the willingness of different leading enterprises to participate in the futures market is significantly different based on risk preference and price risk perception. Therefore, risk management instruments suitable for various types of leading enterprises should be selected for training. For example, for risk-averse enterprises, the focus should be on promoting and providing training related to agricultural insurance-related knowledge; for risk-loving enterprises, training should focus on futures- and derivative-related knowledge. Such an approach will help effectively improve the overall risk management awareness and capabilities of leading enterprises through the development of differentiated training strategies and targeted promotions and training.
Second, to enhance the price risk perception of leading enterprises, the futures association needs to further refine the training courses and optimize the publicity and training effect of the futures market. With the continuous development and progress of China’s modern agricultural economy and policy reform in recent years (Han et al., 2022), many leading agricultural enterprises continue to emerge, which greatly expands the types of agricultural business entities in China and creates many new sources of demand for price risk management. Futures market-related publicity and training cannot be adapted to the new situation. In the future, training courses should be accurately customized in addition to following the existing norms. For example, training should be conducted at different levels based on themes such as hedging, basis trading, warehouse receipt registration, warehousing financing, and internal control system design to optimize the overall training effectiveness and further enhance the price risk perception of leading enterprises.
Data availability statement
The raw data supporting the conclusion of this article will be made available by the authors, without undue reservation.
Author contributions
AC contributed analysis and figures and wrote, edited and revised the text, created and edited tables. XH edited and revised the text, edited tables. MA edited and revised the manuscript. All authors contributed to the tables, wrote portions of the text, and edited the manuscript.
Funding
This research was funded by 2021 Philosophy and Social Science Research General Projects at Colleges and Universities in Jiangsu Province (2021SJA1219) and the Agricultural Science and Technology Innovation Program of the Chinese Academy of Agricultural Sciences (10-IAED-08-2022, 10-IAED-RC-04-2022).
Conflict of interest
The authors declare that the research was conducted in the absence of any commercial or financial relationships that could be construed as a potential conflict of interest.
Publisher’s note
All claims expressed in this article are solely those of the authors and do not necessarily represent those of their affiliated organizations, or those of the publisher, the editors and the reviewers. Any product that may be evaluated in this article, or claim that may be made by its manufacturer, is not guaranteed or endorsed by the publisher.
References
Abid, M., Scheffran, J., Schneider, U. A., and Elahi, E. (2018). Farmer perceptions of climate change, observed trends and adaptation of agriculture in Pakistan. Environ. Manag. 63, 110–123. doi:10.1007/s00267-018-1113-7
Ahmad, M., Majeed, A., Khan, M. A., Sohaib, M., and Shehzad, K. (2021a). Digital financial inclusion and economic growth: Provincial data analysis of China. China Econ. J. 14, 291–310. doi:10.1080/17538963.2021.1882064
Ahmad, M., Shabir, M., Naheed, R., and Shehzad, K. (2021b). How do environmental innovations and energy productivity affect the environment? Analyzing the role of economic globalization. Int. J. Environ. Sci. Technol. (Tehran). 19, 7527–7538. doi:10.1007/s13762-021-03620-8
Akhtar, S., Li, G.-C., Ullah, R., Nazir, A., Iqbal, M. A., Raza, M. H., et al. (2018). Factors influencing hybrid maize farmers' risk attitudes and their perceptions in Punjab Province, Pakistan. J. Integr. Agric. 17, 1454–1462. doi:10.1016/s2095-3119(17)61796-9
An, Y. (2018). The innovation of China’s futures agriculture. Beijing, China: Economic Science Press.
Antoniou, A., and Holmes, P. (1995). Futures trading, information and spot price volatility: Evidence for the FTSE-100 stock index futures contract using GARCH. J. Bank. Finance 19, 117–129. doi:10.1016/0378-4266(94)00059-c
Aragó, V., Barreda-Tarrazona, I., Breaban, A., Matallín, J. C., and Salvador, E. (2022). Market risk aversion under volatility shifts: An experimental study. Int. Rev. Econ. Finance 80, 552–568. doi:10.1016/j.iref.2022.02.022
Bauer, R. A. (1960). “Consumer behavior as risk taking,” in Proceedings of the 43rd National Conference of the American Marketing Assocation, Chicago, Illinois, June 15, 16, 17 (1960: American Marketing Association).
Brandt, L., Van Biesebroeck, J., Wang, L., and Zhang, Y. (2017). WTO accession and performance of Chinese manufacturing firms. Am. Econ. Rev. 107, 2784–2820. doi:10.1257/aer.20121266
Carter, C. A., Zhong, F., and Zhu, J. (2009). Development of Chinese agriculture since WTO accession. EuroChoices 8, 10–16. doi:10.1111/j.1746-692x.2009.00126.x
Chen, X. (2020). The core of China’s rural revitalization: Exerting the functions of rural area. China Agric. Econ. Rev. 12, 1–13. doi:10.1108/caer-02-2019-0025
Chen, X., and Wei, Y. (2019). Risk Percption, risk preference and the adoption of poor household’s agricultural risk management strategies. J. South China Agric. Univ. Soc. Sci. Ed. 18, 74–85.
Cheng, A., Chen, T., Jiang, G., and Han, X. (2021). Can major public health emergencies affect changes in international oil prices? Int. J. Environ. Res. Public Health 18, 12955. doi:10.3390/ijerph182412955
Cohn, A., Engelmann, J., Fehr, E., and Maréchal, M. (2015). Evidence for countercyclical risk aversion: An experiment with financial professionals. Am. Econ. Rev. 105 (2), 860–885. doi:10.1257/aer.20131314
Duan, K., Zhang, C., Li, J., Zhang, R., and Zhang, Y. (2020). Boundary-spanning search for knowledge, knowledge reconstruction and the sustainable innovation ability of agricultural enterprises: A Chinese perspective. Agriculture 10, 39. doi:10.3390/agriculture10020039
Elahi, E., Abid, M., Zhang, H., Cui, W., and Hasson, S. U. (2018a). Domestic water buffaloes: Access to surface water, disease prevalence and associated economic losses. Prev. veterinary Med. 154, 102–112. doi:10.1016/j.prevetmed.2018.03.021
Elahi, E., Abid, M., Zhang, L., Ul Haq, S., and Sahito, J. G. M. (2018b). Agricultural advisory and financial services; farm level access, outreach and impact in a mixed cropping district of Punjab, Pakistan. Land use policy 71, 249–260. doi:10.1016/j.landusepol.2017.12.006
Elahi, E., Khalid, Z., Tauni, M. Z., Zhang, H., and Lirong, X. (2021a). Extreme weather events risk to crop-production and the adaptation of innovative management strategies to mitigate the risk: A retrospective survey of rural Punjab, Pakistan. Technovation 102, 102255. doi:10.1016/j.technovation.2021.102255
Elahi, E., Khalid, Z., Weijun, C., and Zhang, H. (2020). The public policy of agricultural land allotment to agrarians and its impact on crop productivity in Punjab province of Pakistan. Land Use Policy 90, 104324. doi:10.1016/j.landusepol.2019.104324
Elahi, E., Khalid, Z., and Zhang, Z. (2022a). Understanding farmers’ intention and willingness to install renewable energy technology: A solution to reduce the environmental emissions of agriculture. Appl. Energy 309, 118459. doi:10.1016/j.apenergy.2021.118459
Elahi, E., Weijun, C., Jha, S. K., and Zhang, H. (2019a). Estimation of realistic renewable and non-renewable energy use targets for livestock production systems utilising an artificial neural network method: A step towards livestock sustainability. Energy 183, 191–204. doi:10.1016/j.energy.2019.06.084
Elahi, E., Weijun, C., Zhang, H., and Abid, M. (2019b). Use of artificial neural networks to rescue agrochemical-based health hazards: A resource optimisation method for cleaner crop production. J. Clean. Prod. 238, 117900. doi:10.1016/j.jclepro.2019.117900
Elahi, E., Weijun, C., Zhang, H., and Nazeer, M. (2019c). Agricultural intensification and damages to human health in relation to agrochemicals: Application of artificial intelligence. Land use policy 83, 461–474. doi:10.1016/j.landusepol.2019.02.023
Elahi, E., Zhang, H., Lirong, X., Khalid, Z., and Xu, H. (2021b). Understanding cognitive and socio-psychological factors determining farmers’ intentions to use improved grassland: Implications of land use policy for sustainable pasture production. Land Use Policy 102, 105250. doi:10.1016/j.landusepol.2020.105250
Elahi, E., Zhixin, Z., Khalid, Z., and Xu, H. (2022b). Application of an artificial neural network to optimise energy inputs: An energy-and cost-saving strategy for commercial poultry farms. Energy 244, 123169. doi:10.1016/j.energy.2022.123169
Fang, R., An, Y., and Liu, W. (2019). Can the pilot project of “insurance + futures” improve farmers’ enthusiasm to grow grain? An analysis from the perspectives of mediating effect of farmers’ participation willingness and moderating effect of their satisfaction with government subsidies. China Rural. Econ. 6, 113–126.
Guiso, L., Sapienza, P., and Zingales, L. (2018). Time varying risk aversion. J. Financial Econ. 128, 403–421. doi:10.1016/j.jfineco.2018.02.007
Han, J. (2020). Prioritizing agricultural, rural development and implementing the rural revitalization strategy. China Agric. Econ. Rev. 12, 14–19. doi:10.1108/caer-02-2019-0026
Han J, J., Song, J., and Qi, T. (2019). Impact of sino-US trade friction on import and export trade pattern of soybean in heilongjiang. Asian Agric. Res. 11, 1–10. doi:10.22004/ag.econ.290286
Han, X., Chen, Y., and Wang, X. (2021). Impact of grain subsidy reform on the land use of smallholder farms: Evidence from huang-huai-hai plain in China. Land 10, 929. doi:10.3390/land10090929
Han, X., Xue, P., and Zhang, N. (2022). Impacts of China’s bioethanol policy on the global maize market: A partial equilibrium analysis to 2030. Food Secur. 14, 147–163. doi:10.1007/s12571-021-01212-5
Han X, X., Yang, S., Chen, Y., and Wang, Y. (2019). Urban segregation and food consumption: The impacts of China’s household registration system. China Agric. Econ. Rev. 11, 583–599. doi:10.1108/caer-07-2018-0153
Hao, A. (2010). Uncertainty, risk aversion and risk management in agriculture. Agric. Agric. Sci. procedia 1, 152–156. doi:10.1016/j.aaspro.2010.09.018
Harrison, G. W., Lau, M. I., and Rutström, E. E. (2007). Estimating risk attitudes in Denmark: A field experiment. Scand. J. Econ. 109, 341–368. doi:10.1111/j.1467-9442.2007.00496.x
He, Z., Hu, L., and Lu, Q. (2018). Influence of farmer's risk preference and risk perception on water-saving irrigation technology adoption. Resour. Sci. 40, 797–808. doi:10.18402/resci.2018.04.13
Hellerstein, D., Higgins, N., and Horowitz, J. (2013). The predictive power of risk preference measures for farming decisions. Eur. Rev. Agric. Econ. 40, 807–833. doi:10.1093/erae/jbs043
Huang, J., Rozelle, S., and Chang, M. (2004). Tracking distortions in agriculture: China and its accession to the World trade organization. World Bank Econ. Rev. 18, 59–84. doi:10.1093/wber/lhh033
James, L. R., and Brett, J. M. (1984). Mediators, moderators, and tests for mediation. J. Appl. Psychol. 69 (2), 307–321. doi:10.1037/0021-9010.69.2.307
R. E. Just, and R. D. Pope (Editors) (2002). A comprehensive assessment of the role of risk in U.S. Agriculture, (New York, NY: Springer Science + Business Media, LLC).
Kahneman, D., and Tversky, A. (1979). Prospect theory: An analysis of decision under risk. Econometrica 47, 263–292. doi:10.2307/1914185
Kandasamy, N., Hardy, B., Page, L., Schaffner, M., Graggaber, J., Powlson, A., et al. (2014). Cortisol shifts financial risk preferences. Proc. Natl. Acad. Sci. U. S. A. 111 (9), 3608–3613. doi:10.1073/pnas.1317908111
König-Kersting, C., and Trautmann, S. T. (2018). Countercyclical risk aversion: Beyond financial professionals. J. Behav. Exp. Finance 18, 94–101. doi:10.1016/j.jbef.2018.03.001
Kuo, Y.-F., Lin, Y.-M., and Chien, H.-F. (2021). Corporate social responsibility, enterprise risk management, and real earnings management: Evidence from managerial confidence. Finance Res. Lett. 41, 101805. doi:10.1016/j.frl.2020.101805
Leiserowitz, A. A. (2005). American risk perceptions: Is climate change dangerous? Risk Anal. 25, 1433–1442. doi:10.1111/j.1540-6261.2005.00690.x
Li, M., Balistreri, E. J., and Zhang, W. (2020a). The U.S.–China trade war: Tariff data and general equilibrium analysis. J. Asian Econ. 69, 101216. doi:10.1016/j.asieco.2020.101216
Li, M., Gan, C., Ma, W., and Jiang, W. (2020b). Impact of cash crop cultivation on household income and migration decisions: Evidence from low-income regions in China. J. Integr. Agric. 19 (10), 2571–2581. doi:10.1016/s2095-3119(20)63161-6
Liu, T., Kou, F., Liu, X., and Elahi, E. (2022). Cluster commercial credit and total factor productivity of the manufacturing sector. Sustainability 14, 3601. doi:10.3390/su14063601
Liu, W., and He, X. (2018). Current situation and countermeasure of modern agriculture development in northeast China. OALib 5, 1–12. doi:10.4236/oalib.1104922
Lv, W. (2014). Executive risk appetite, risk perception and the willingness to purchase science and technology insurance. China Soft Sci. 7, 128–138.
Martin, W. (2001). Implications of reform and WTO accession for China’agricultural policies. Econ. Transition 9, 717–742. doi:10.1111/1468-0351.00097
Menapace, L., Colson, G., and Raffaelli, R. (2015). A comparison of hypothetical risk attitude elicitation instruments for explaining farmer crop insurance purchases. Eur. Rev. Agric. Econ. 43, 113–135. doi:10.1093/erae/jbv013
Palich, L. E., and Bagby, D. R. (1995). Using cognitive theory to explain entrepreneurial risk-taking: Challenging conventional wisdom. J. Bus. Ventur. 10, 425–438. doi:10.1016/0883-9026(95)00082-j
Peng, B., Chen, H., Elahi, E., and Wei, G. (2020a). Study on the spatial differentiation of environmental governance performance of Yangtze river urban agglomeration in Jiangsu province of China. Land Use Policy 99, 105063. doi:10.1016/j.landusepol.2020.105063
Peng, B., Chen, S., Elahi, E., and Wan, A. (2021a). Can corporate environmental responsibility improve environmental performance? An inter-temporal analysis of Chinese chemical companies. Environ. Sci. Pollut. Res. 28, 12190–12201. doi:10.1007/s11356-020-11636-9
Peng, B., Wang, Y., Zahid, S., Wei, G., and Elahi, E. (2020b). Platform ecological circle for cold chain logistics enterprises: The value co-creation analysis. Industrial Manag. Data Syst. 120, 675–691. doi:10.1108/IMDS-10-2019-0531
Peng, R., Zhao, Y., Elahi, E., and Peng, B. (2021b). Does disaster shocks affect farmers’ willingness for insurance? Mediating effect of risk perception and survey data from risk-prone areas in east China. Nat. Hazards (Dordr). 106, 2883–2899. doi:10.1007/s11069-021-04569-0
Rizwan, M., Ping, Q., Saboor, A., Ahmed, U. I., Zhang, D., Deyi, Z., et al. (2020). Measuring rice farmers’ risk perceptions and attitude: Evidence from Pakistan. Hum. Ecol. Risk Assess. Int. J. 26 (7), 1832–1847. doi:10.1080/10807039.2019.1602753
Shang, Y., Xiong, T., and Li, C. (2020). Risk perception, risk attitude and farmers’ willingness to adopt risk management strategies: A case study of crop insurance and ‘insurance + futures. China Rural. Survey 41, 52–72.
Van Winsen, F., De Mey, Y., Lauwers, L., Van Passel, S., Vancauteren, M., and Wauters, E. (2016). Determinants of risk behaviour: Effects of perceived risks and risk attitude on farmer’s adoption of risk management strategies. J. Risk Res. 19, 56–78. doi:10.1080/13669877.2014.940597
Veeck, G., Veeck, A., and Yu, H. (2020). Challenges of agriculture and food systems issues in China and the United States. Geogr. Sustain. 1, 109–117. doi:10.1016/j.geosus.2020.05.002
Weber, M., Weber, E., and Nosic, A. (2013). Who takes risks when and why: Determinants of changes in investor risk taking. Rev. Finance 17, 847–883. doi:10.1093/rof/rfs024
Wen, Z., Hau, K.-T., and Chang, L. (2005). A comparison of moderator and mediator and their applications. Acta Psychol. sin. 37 (2), 268–274.
Xue, P., Han, X., Elahi, E., Zhao, Y., and Wang, X. (2021). Internet access and nutritional intake: Evidence from rural China. Nutrients 13, 2015. doi:10.3390/nu13062015
Zhang, H., Veltri, A., Calvo-Amodio, J., and Haapala, K. R. (2021). Making the business case for sustainable manufacturing in small and medium-sized manufacturing enterprises: A systems decision making approach. J. Clean. Prod. 287, 125038. doi:10.1016/j.jclepro.2020.125038
Zhang, Y., Diao, X., Chen, K. Z., Robinson, S., and Fan, S. (2020). Impact of COVID-19 on China's macroeconomy and agri-food system – An economy-wide multiplier model analysis. China Agric. Econ. Rev. 12, 387–407. doi:10.1108/caer-04-2020-0063
Zhang, Z., Li, Y., Elahi, E., and Wang, Y. (2022a). Comprehensive evaluation of agricultural modernization levels. Sustainability 14, 5069. doi:10.3390/su14095069
Zhang, Z., Meng, X., and Elahi, E. (2022b). Protection of cultivated land resources and grain supply security in main grain-producing areas of China. Sustainability 14, 2808. doi:10.3390/su14052808
Zhao, H., Zhao, X., Elahi, E., and Wang, F. (2022). Policy evaluation of drama-related intangible cultural heritage tourism for boosting green industry: An empirical analysis based on quasi-natural experiment. Sustainability 14, 5380. doi:10.3390/su14095380
Zhao, X., Peng, B., Elahi, E., Zheng, C., and Wan, A. (2020). Optimization of Chinese coal-fired power plants for cleaner production using Bayesian network. J. Clean. Prod. 273, 122837. doi:10.1016/j.jclepro.2020.122837
Zhao, Y., Peng, B., Elahi, E., and Wan, A. (2021). Does the extended producer responsibility system promote the green technological innovation of enterprises? An empirical study based on the difference-in-differences model. J. Clean. Prod. 319, 128631. doi:10.1016/j.jclepro.2021.128631
Zhong, Z., Peng, B., and Elahi, E. (2021). Spatial and temporal pattern evolution and influencing factors of energy–environmental efficiency: A case study of yangtze river urban agglomeration in China. Energy & Environ. 32, 242–261. doi:10.1177/0958305x20923114
Keywords: risk preference, futures market, cotton, jujube, mediating effect
Citation: Cheng A, Han X and Ahmad M (2022) Risk preference and willingness to participate in the futures market: Evidence from cotton and jujube enterprises of China. Front. Environ. Sci. 10:978557. doi: 10.3389/fenvs.2022.978557
Received: 26 June 2022; Accepted: 29 July 2022;
Published: 29 August 2022.
Edited by:
Rita Yi Man Li, Hong Kong Shue Yan University, Hong Kong SAR, ChinaReviewed by:
Yuan Chai, University of Minnesota Twin Cities, United StatesZheng Shen, Zhejiang Agriculture and Forestry University, China
Steven Li, RMIT University, Australia
Copyright © 2022 Cheng, Han and Ahmad. This is an open-access article distributed under the terms of the Creative Commons Attribution License (CC BY). The use, distribution or reproduction in other forums is permitted, provided the original author(s) and the copyright owner(s) are credited and that the original publication in this journal is cited, in accordance with accepted academic practice. No use, distribution or reproduction is permitted which does not comply with these terms.
*Correspondence: Xinru Han, hanxinru@caas.cn