Distinctive metabolite profiles in migrating Amur ide (Leuciscus waleckii) reveal changes in osmotic pressure, gonadal development, and energy allocation strategies
- 1National and Local Joint Engineering Laboratory for Freshwater Fish Breeding, Heilongjiang Province’s Key Laboratory of Fish Stress Resistance Breeding and Germplasm Characteristics on Special Habitats, Heilongjiang River Fisheries Research Institute, Chinese Academy of Fishery Sciences, Harbin, Heilongjiang, China
- 2BGI Genomics, BGI-Shenzhen, Shenzhen, Guangdong, China
- 3College of Marine Life and Fisheries Sciences, Jiangsu Ocean University, Lianyungang, Jiangsu, China
- 4School of Fisheries, Aquaculture and Aquatic Sciences, Auburn University, Auburn, AL, United States
- 5College of Animal Science, Inner Mongolia Agriculture University, Huhhot, Inner Mongolia, China
- 6Higher Institution Center of Excellence (HICoE), Institute of Tropical Aquaculture and Fisheries, Universiti of Malaysia Terengganu, Kuala Nerus, Terengganu, Malaysia
Amur ide (Leuciscus waleckii) lives in alkali-saline water (pH = 9.6) in the Lake Dali and spawns in freshwater rivers after migration annually. During spawning migrations, Amur ide not only experience osmoregulation modification from alkali-saline water to freshwater but also deal with energy prioritization for basal metabolism and gonadal development. To achieve an optimal cost-benefit balance, a series of metabolism modifications are needed. This study investigated the changing metabolite profiles that contribute to maintaining a balance of osmotic pressure and energy allocation for gonadal maturation. We applied ultra-performance liquid chromatography together with quadrupole time-of-flight mass spectrometry (UPLC-QTOF-MS), combined with chemometrics, for identifying metabolic changes regarding spawning broodstocks of Amur ide during migration. According to findings, there were 11,333 metabolites in Amur ide serum and 3,159 metabolites were found to change significantly during migration. Differentially expressed metabolites mainly affected the steroid hormone biosynthesis, the arachidonic acid metabolism, the biosynthesis of phenylalanine, tyrosine, and tryptophan, pyruvate metabolism, citrate cycle, as well as glycerophospholipid metabolism. Based on the enrichment analysis regarding metabolic pathways, biosynthesis of steroid hormone and arachidonic acid metabolism are two representative pathways, which are crucial for osmoregulation and gonadal maturation. The perturbation of some metabolites during migration was highlighted, which involves sexual maturation and reproduction, nitrogenous waste excretion, and energy allocation. The study assists in understanding the physiological plasticity exhibited by Amur ide during migratory spawning from a new perspective, which is useful as a scientific basis for the artificial breeding of Amur ide.
1 Introduction
Amur ide (Leuciscus waleckii) is a cyprinid species commonly see in Northeast Asia (Xu et al., 2017). It can live well in both freshwater and alkali-saline water (Zhao et al., 2021). Specifically, Amur ide inhabits Lake Dali (116°25–116°45′E, 43°13″-43°23′N), Inner Mongolia, China, a typical soda lake where titratable alkalinity is more than 50 mmol/L at pH 9.6 and the salinity is 6‰ (Chi et al., 2010; Chang et al., 2013). Studies have investigated the geology of Lake Dali, finding that the origin of the lake is the ancient Amur River during the early Holocene (11,500–7,600 BP) with water area of about 1,600 km2. Due to serious drought in the late Holocene (∼3,450 BP), the area of Lake Dali reduced to less than 200 km2 and the water became alkaline (Geng and Zhang, 1988; Xiao et al., 2008). Amur ide gradually adapted to this highly alkali-saline water environment and gradually developed to be a dominant fish species in the lake in the last several thousand years (Chang et al., 2014; Xu et al., 2017).
With their adaptability, Amur ide serves as a model for studying the mechanisms related to alkaline tolerance, both physiologically and molecularly (Chen et al., 2019). (Chi, 2010) found that the alkali form of Amur ide was capable of tolerating higher bicarbonate alkalinity than the freshwater forms. This was supported by (Xu et al., 2013; Chang et al., 2014) finding the differential expression of many genes and encoding important modulators regarding stress tolerance and adaption by virtue of the comparative transcriptome analysis. These are involved in ionic and osmo-regulation (e.g., Na+-K+ ATPase, anion exchanger 1, solute carrier family 26 members 5, 6, and 11), ammonia transportation (e.g., Rh family C glycoproteins 1 and 2), and arachidonic acid synthesis (e.g., prostaglandin-endoperoxide synthase 1). Recently, through high throughput genomic resequencing analysis, genes adapting to alkaline environments have been identified, that affect the osmoregulation, inflammation, immune responses, and cardiorespiratory development (Xu et al., 2017; Wang et al., 2021); Subsequently, based on the expression of gill genes involved in Na+ and HCO3− transportation and performance of serum physiological indicators sampled in laboratory bicarbonate stress experiment, (Chang et al., 2021) explained why the alkali form of Amur ide can rapidly adapt to extreme alkaline water compared with its freshwater counterpart. Accordingly, Amur ide that inhabit Lake Dali experience substantial physiological and molecular changes that make it capable of handling the tough alkaline environments.
Although Amur ide in Lake Dali has evolved complex regulatory mechanisms to survive under extreme alkaline conditions, annual spawning can only be supported after migrating to freshwater waterway (Cui et al., 2015; Chen et al., 2019). As Figure 1 shown, Amur ide in Lake Dali migrate from a small Shali River to the dam of Lake Ganggeng for spawning in late April to early May each year, about 32 km from Lake Dali River mouth. During spawning migration, Amur ide encounter severe environmental fluctuations, the pH ranges from 9.6 to 7.8, alkalinity from 50 to 4 mmol/L, salinity from 6‰ to 0.4‰, temperature from 4°C to 15°C (Yu et al., 2008; Chen et al., 2019). To explore the molecular as well as physiological mechanisms regarding the spawning migration of Amur ide, (Cui et al., 2015) investigated transcriptomic profiles of liver between spawning migration population (SM) and colonized freshwater population (FW); Subsequently, (Chen et al., 2019) compared transcriptomic profiles of liver before and after spawning migration. In their study, there were abundant differentially expressed genes that impacted the oxidative phosphorylation, acid-base regulation, excretion of nitrogenous waste, sexual maturation and reproduction, as well as stress response, demonstrating that the plasticity of gene expression plays important roles in Amur ide spawning migration.
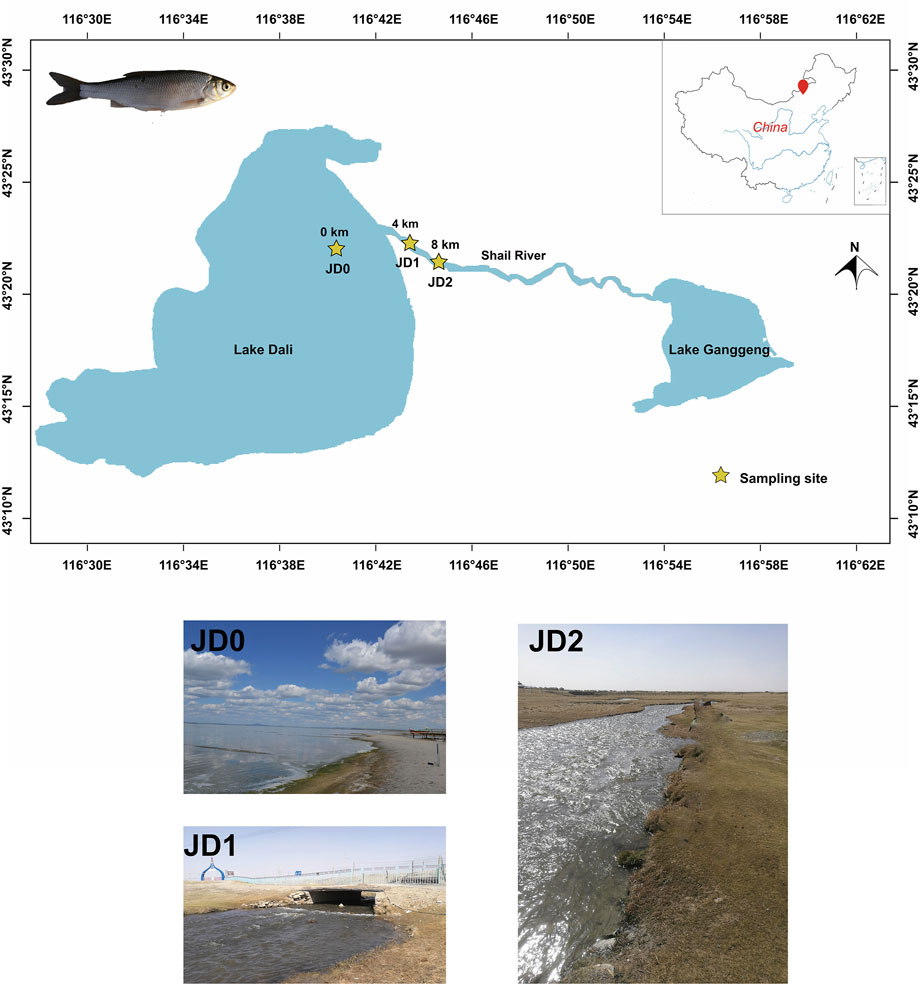
FIGURE 1. Geographic distribution of the collected samples along the migration route. The yellow asterisks represent the sampling sites. The number indicates the distance (river km) between the sampling site and Lake Dali.
The spawning migration of anadromous fish is often interpreted as a compensation-adaptive response, allowing for optimum conditions for the embryo and offspring development (Tamario et al., 2019). The trade-offs during migration involve osmo-regulation, gonadal development and energy expenditure. Recently, as different “omics” technologies have developed rapidly, above mentioned molecular as well as physiological mechanisms have received extensive attention. (Benskin et al., 2014) discovered metabolite changes in Skeena River sockeye salmon (Oncorhynchus nerka) in the migration process with a significant decrease in taurine, carnitine, and eicosapentaenoic and docosahexaenoic acid (two representative polyunsaturated fatty acids) for both sexes. (Zhu et al., 2020) found metabolic changes in female Chinese sturgeon (Acipenser sinensis) when ovary developed from stage II to IV with increasing amino acids and lipid, to meet the high energy requirement for the ovarian development. (Yin et al., 2020) explored metabolic profiles of Coilia nasus in response to food intake in the migration process with remarkably decreasing amino acid and linoleic acid metabolism, and increasing steroid hormone biosynthesis for feeding fish. These studies demonstrated that functional metabolites are involved in physiological plasticity, especially in osmo-regulation and gonadal development during fish reproductive migration.
Although transcriptomic profiles of liver in Amur ide during migration have been investigated (Cui et al., 2015; Chen et al., 2019), the physiological mechanisms behind spawning migration are still unclear. Compared to other tissues such as liver and muscle, blood is considered to be a window directly reflecting the individual’s physiological state as it circulates through the entire body (Aru et al., 2021). In addition, collecting blood samples can cause the smallest damage to protected and endangered species (Zhu et al., 2020). Thereby, serum metabolic profiles of Amur ide following the migration route using a non-targeted metabolomics platform were investigated. This study aimed at 1) identifying as well as characterizing the key metabolites and signal pathways that relate to osmoregulation, gonadal development, and energy demands during migration; and 2) depicting the hypothesized regulatory network models that allow Amur ide to spawn successfully during migration. The results provide new insights into the physiological features exhibited by Amur ide underline spawning migration, which will facilitate the artificial propagation of Amur ide as well as other migratory fish.
2 Materials and methods
2.1 Sample collection
During the Amur ide migration from 08:00 to 17:00 h on 02 May 2018–04 May 2018, a total of 11 individuals from Lake Dali (43°22ʹ43ʹʹ N, 116°39ʹ24ʹʹ E) (abbreviated as JD0), 12 individuals from the estuary of Lake Dali and Shali River (43°23ʹ53ʹʹ N, 116°43ʹ12ʹʹ E) (abbreviated as JD1), and 12 individuals from Shali River (43°21ʹ32ʹʹ N, 116°44ʹ56ʹʹ E) (abbreviated as JD2) (Figure 1) were collected. The average body weight was 107.23 ± 18.65 g and the average body length was 17.32 ± 2.57 cm. Despite the long migratory path of Amur ide, sampling site JD2 did not differ significantly in temperature, salinity, and alkalinity from the habitat of the remaining migratory path (Yu et al., 2008; Chang et al., 2013; Chen et al., 2019). Thus, no samples were collected from the remaining path for species conservation purposes. We collected blood sample from each individual’s caudal vein after the fishes underwent anesthetization by using a neutralized MS222 (Pharmaq Ltd., Hampshire, United Kingdom) at a concentration of 100 mg/L for about 30 s. The blood then underwent 10 min of centrifugation at 4,000 × g at 4°C to collect the serum. We placed the collected serum on dry ice and transported samples to the laboratory where they were stored at −80°C for subsequent analysis.
2.2 Sample preparation
Collected serum samples stayed at −20°C for half an hour, followed by the thawing treatment at 4°C until no ice existed in the tubes. A total of 40 μl of serum sample received 1 min of centrifugation in a new tube containing 120 μl ice-cold methanol and vortex-mixed; samples were then precipitated at room temperature for 10 min. Thereafter, we stored samples for one night in a refrigerator at −20°C for improving the protein precipitation. Before analysis, serum samples received 20 min of centrifugation at 4,000 × g at 4°C for precipitating the remaining proteins in the samples. After centrifugation, we transferred 25 μl protein-free supernatant to another centrifuge tube and used 50% methanol for ten times of dilution. This was used for UPLC-Q-TOF-MS. For evaluating the instrument performance, 40 μl of each test sample were completely mixed to prepare a quality control (QC) sample.
2.3 Non-targeted metabolic profiling
A 2777C UPLC system (Waters, Manchester, United Kingdom) with an ACQUITY UPLC BEH C18 column (100 mm × 2.1 mm, 1.7 μm, Waters,United Kingdom) served for the chromatographic separation. First, all samples were separated in the column at 50°C. We set the flow rate at 0.4 ml/min, and the mobile phase contained solvent A (water + 0.1% formic acid) and solvent B (acetonitrile + 0.1% formic acid). Gradient elution conditions included: 0–2 min, 100% phase A; 2–11 min, 0%–100% B; 11–13 min, 100% B; 13–15 min, 0%–100% A. Each sample had injection volume of 10 μl. Then, we detected the metabolites eluted from the column using Xevo G2-XS quadrupole time-of-flight mass spectrometer (Q-TOF-MS) (Waters, United Kingdom) with an electrospray ionization source (ESI) that operated under positive ion mode and negative ion mode. In terms of the positive ion mode, we respectively set the capillary and sampling cone voltages at 3.0 and 40.0 V. Specific to the negative ion mode, we respectively set the above two voltages at 2.0 kV and 40.0 V, and acquired the mass spectrometry data in Centroid MSE mode. The TOF mass was set in the range of 50–1,200 Da with scanning time intervals at 0.2 s. Specific to the MS/MS detection, all precursors received fragmentation treatment at 20–40 eV at 0.2 s. In the acquisition process, we acquired the LE signal each 3 s for calibrating the mass accuracy. Meanwhile, for evaluating the MS stability in the entire acquisition process, one QC sample (that pooled all test samples) contributed to a mean profile that represented all the analytes in the analysis. First, ten QC samples were injected when the analysis began, for ensuring system equilibrium, followed by every 10 test sample intervals with one QC sample injected for more deeply monitoring the analysis stability. Finally, we injected three QC samples at the end of analysis for calibrating the retention time drift of all analyses considering the matrix effect.
2.4 Data handling and statistical analyses
2.4.1 Raw data processing and multivariate analysis
Progenesis QI software (version 2.2) assisted in processing the raw data for filtering noise, finding peak area, removing isotope mass, aligning retention time (Rt) and mass (m/z), screening, and normalizing the total area. Normalizing the peak area involved changing the sum of ion intensities exhibited by each peak in each blood sample to 1, followed by calculating the proportion occupied by each number. The metaX software served for further processing the data processed in the Progenesis QI (Wen et al., 2017). Features that existed in <50% of the QC samples or <20% of the test samples would be removed and not be used for further analysis. Following filtering, the K-nearest neighbor method helped to impute the missing values. The QC-robust spline batch correction (QC-RSC) (Dunn et al., 2011) together with the combat normalization approach (Chen et al., 2011) assisted in correcting the signal drift and the batch variation. When the normalization was completed, we retained features that exhibited a relative standard deviation <30% from the QC samples. Prior to statistical analysis, the dataset underwent data cleaning algorithms. We also removed those with SNR < 1 (SNR = standard deviation sample/standard deviation QC) or relative difference in the mean intensity between QC samples and test samples 3 times larger than the standard deviation of the intensity of test samples. We carried out the dimensionality reduction and pattern recognition analysis by imputing pre-processed data to metaX (Wen et al., 2017). First, a principal component analysis (PCA) served for reducing data dimensionality and previewing the data. Then QC sample distribution in the PCA plot served for confirming the method stability. After, we adopted a partial least squares discriminant analysis (PLS-DA) for confirming the modeling that yielded the largest difference among groups (Barker and Rayens, 2003; Westerhuis et al., 2008). The permutation tests validated the PLS-DA model (200 times) for offering the R2/Q2 value distribution that could report the statistical significance.
For adjusting the significance of metabolites with high and low abundance to the same level, the data received preprocessing by converting the log2 and Pareto scaling before PLS-DA (Dunn et al., 2011). Variable importance in projection (VIP) values from the PLS-DA model were taken into account to screen features with significant differences. Specifically, we set the VIP cut-off value at 1.0 and considered VIP values > 1.0 as the major potential metabolites for differentiating the groups. These features were examined using a Wilcoxon rank-sum test (Degu et al., 2014). The Benjamin–Hochberg approach adjusted the p-value. Features exhibiting a VIP value > 1, fold change (FC) ≥1.2 or ≤ 0.8333, and a corrected p < 0.05 assisted in identifying differential metabolites between groups.
2.4.2 Differential metabolites identification and univariate analysis
Volcano plots served for filtering differential metabolites considering the log2-fold-change and −log10 (p-value) regarding metabolites. These metabolites underwent the Kyoto Encyclopedia of Genes and Genomes (KEGG) pathway enrichment analysis under the assistance of MBRole (Chagoyen and Pazos, 2011). In addition, the metabolic changes of some potential biomarkers among different groups were shown using box-whisker plots. Specific to each metabolite, we selected one or two spectrum without, or with only minimal, overlap for calculating the integral area that contributes to obtaining each metabolite’s relative concentration. By calculating the spectra peaks of corresponding metabolites, the relative concentration of metabolites also was acquired. The significance of the differentially expressed metabolites was determined using Mann-Whitney test in the R package (version 3.6.3) (Team, 2013), which were indicated as *p < 0.05 or **p < 0.01.
3 Results
3.1 Metabolic profiles analysis
We screened a total of 11,333 metabolite peaks (6,376 and 4,957 peaks in positive and negative ion mode, respectively) based on the final data set. We respectively constructed the PCA score plots in the two modes. As Figures 2A,B show, the QC samples were close to the center of PCA plots with a tight clustering, indicating that the detection instrument was stable and the obtained data was of higher quality. In addition, the data for each group clustered differently in the PCA plot, which show three obvious clusters that correspond to three sampling groups (JD0, JD1, and JD2) with different environments.
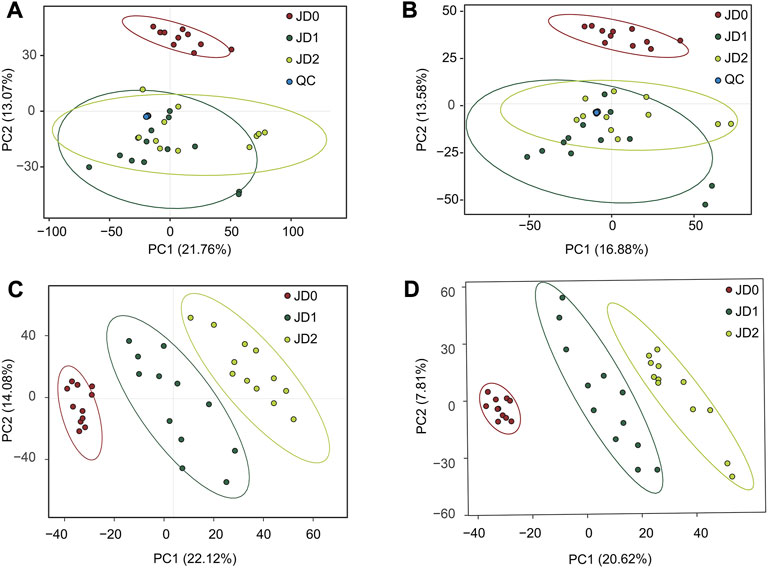
FIGURE 2. PCA score plot of JD0, JD1, and JD2 in the positive (A) and negative (B) ion modes. PLS-DA score plot of JD0, JD1, and JD2 in the positive (C) and negative (D) ion modes.
The PLS-DA was used to profile the metabolites. Figures 2C,D showed that all groups presented clear difference, and all samples in each group were in the 95% confidence ellipses. Permutation test showed that in the positive ion modes, R2 and Q2 were 0.970 and 0.896 between groups of JD0 vs. JD1, 0.974 and 0.860 between groups of JD0 vs. JD2, and 0.856 and 0.370 between groups of JD1 vs. JD2. In the negative modes, R2 and Q2 were 0.986 and 0.906 between groups of JD0 vs. JD1, 0.988 and 0.885 between groups of JD0 vs. JD2, and 0.908 and 0.611 between groups of JD1 vs. JD2 (Table 1), which confirmed the credibility of the PLS-DA model.
3.2 Identification of differential metabolites and functional analysis
Finally, VIP value > 1, fold change (FC) ≥1.2 or ≤ 0.8333, and a corrected p < 0.05 served for identifying differential metabolites between groups. Approximately 3,159 differential metabolites were detected. Specifically, 913 positive and 786 negative ions in JD0 vs. JD1, 114 and 184 in JD1 vs. JD2, and 636 and 526 in JD0 vs. JD2 (Table 2). According to the volcano plot, there were 199 and 262 upregulated and downregulated positive ions, respectively and 166 and 150 upregulated and downregulated negative ions, respectively in the pairwise comparison of JD0 vs. JD1 (Figures 3A,B); 19 positive ions were upregulated and 33 were downregulated, and 36 negative ions were upregulated and 23 were downregulated in pairwise of JD1 vs. JD2 (Figures 3C,D); and 19 negative ions were upregulated and 33 were downregulated and 133 negative ions were upregulated and 111 were downregulated in pairwise of JD0 vs. JD2 (Figures 3E,F).
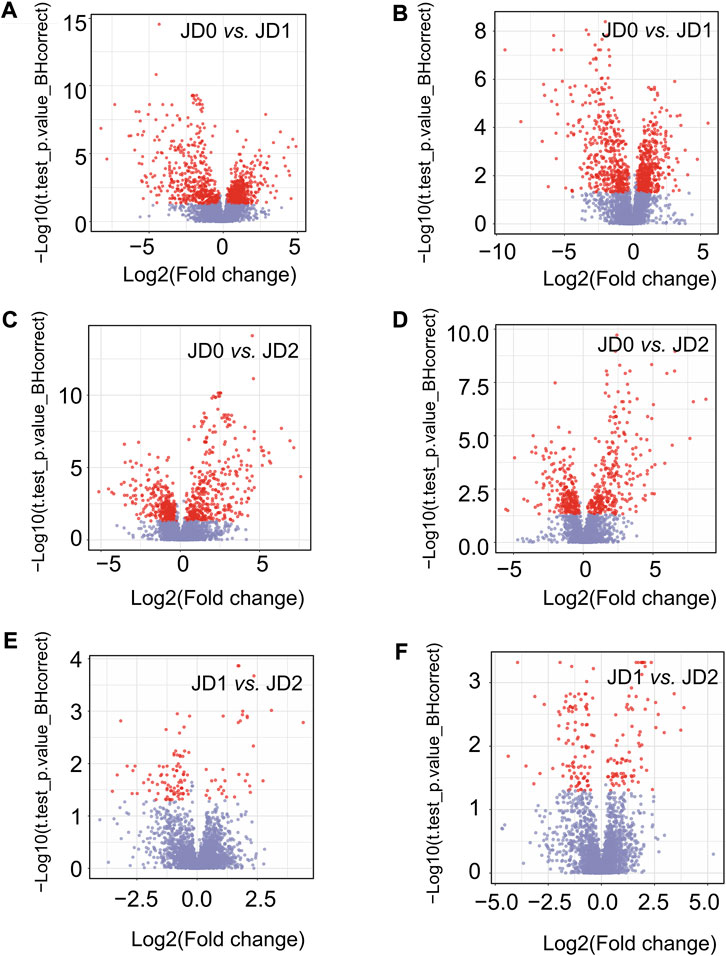
FIGURE 3. Volcano plot of differential metabolite of pairwise comparisons including JD0 vs. JD1 (A,B), JD0 vs. JD2 (C,D), and JD1 vs. JD2 (E,F) in positive and negative ion modes.
Metabolite pathway analysis based on significantly changed metabolites between different sampling sites were determined using MetaboAnalyst 5.0. In JD0 vs. JD1, we screened 53 pathways that involved at least one annotated metabolite as the potential target pathways. Of these, 11 metabolic pathways had lower p-values (p < 0.05) and higher pathway impact scores. Two representative pathways were the Arachidonic acid (ArA) metabolism and the steroid hormone biosynthesis. Other pathways included amino acid metabolism and biosynthesis (alanine, aspartate and glutamate metabolism; tyrosine metabolism; tyrosine metabolism; phenylalanine metabolism; beta-alanine metabolism; phenylalanine, tyrosine and tryptophan biosynthesis; arginine biosynthesis), glycan biosynthesis and metabolism (pentose and glucuronate interconversions; pentose phosphate pathway) as well as galactose metabolism (Figure 4A). In JD0 vs. JD2, a total of 55 potential pathways including 11 significantly expressed pathways were identified, with most of the key metabolic pathways overlapping in the JD0 vs. JD1 group (Figure 4B). In JD1 vs. JD2, approximately 21 potential pathways were detected (Figure 4C). Of which 5 key pathways are mainly involved in sex hormone biosynthesis (steroid hormone biosynthesis) and energy supply (valine, leucine, and isoleucine biosynthesis; arginine and proline metabolism; linoleic acid metabolism; the citrate cycle). Collectively, most of the key intermediate metabolites involved in these pathways were significantly altered in Amur ides during migratory spawning.
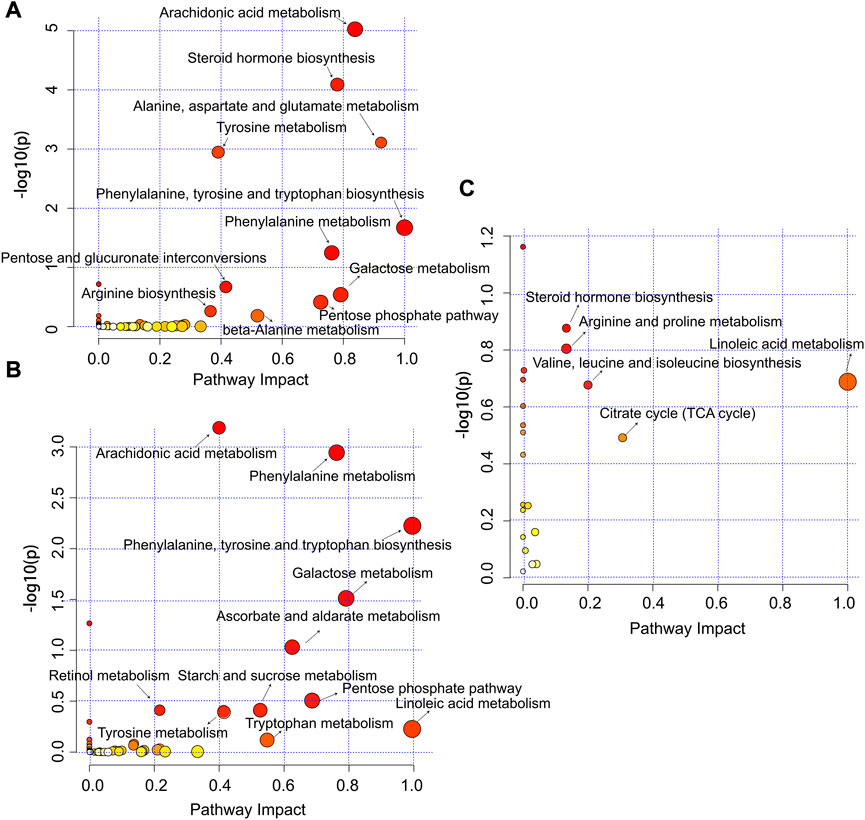
FIGURE 4. Summary of metabolic pathways of significantly changed metabolites of JD0 vs. JD1 (A), JD0 vs. JD2 (B), and JD1 vs. JD2 (C). Circles represent metabolism. The color gradient and circle size indicate the significance of the pathway ranked by p-value (yellow are higher p-values and red are lower p-values) and pathway impact score (the larger the circle, the higher the influence score).
3.3 Alterations of target metabolites during migration
According to metabolic pathway analysis results (Figure 4), most metabolites with differential expressions were involved in the biological processes of osmo-regulation, gonadal maturation, energy metabolism, and ammonia excretion. We considered the relative peak area intensity exhibited by metabolites to calculate the relative concentration of potential target metabolites among different groups using box-whisker plots. The content of cortisol associated with osmoregulation remained constant during the initial stage of the migration (JD0, JD1), but then decreased significantly at JD2 by 1.85-fold (Figure 5A). The level of ArA and its metabolites related to osmoregulation were found to increase at the beginning of the migration and then decreased significantly at a later stage (Figure 5B). As shown in Figures 5C,D, JD1 exhibited remarkably higher content of prostaglandin E2 (PGE2) and prostaglandin F2α (PGF2α) compared to JD0, 6.3 and 6.8-fold higher respectively, then decreased to the initial level of JD0 in JD2.
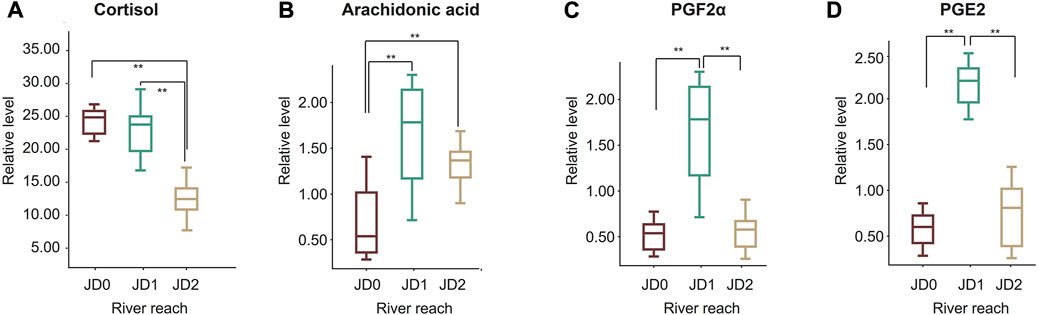
FIGURE 5. Box-whisker plots of some target metabolites associated with osmotic regulation. Cortisol (A); Arachidonic acid (B); Prostaglandin F2α, PGF2α (C); Prostaglandin E2, PGE2 (D). The red boxplots represent JD0, the green boxplots JD1, and the yellow boxplots JD2.
Among steroid hormones related to gonadal maturation, the levels of dehydroepiandrosterone (DHEA), testosterone, androsterone, and estradiol-17β increased during the migration (Figures 6A–D). Of these, the expression of DHEA, a precursor of sex hormones in JD1 and JD2, was expressed at an average of 26.13- and 33.37-fold higher than that of JD0, respectively. In addition, estradiol-17β, estrogen was expressed at 3.86- and 2.91-fold higher than that of JD0, respectively.
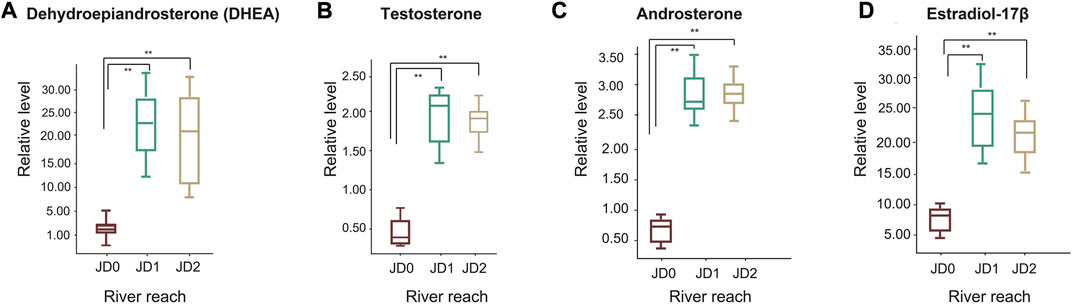
FIGURE 6. Box-whisker plots of some target metabolites associated with sex hormone. Dehydroepiandrosterone, DHEA (A); Testosterone (B); Androsterone (C); Estradiol-17β (D). The red boxplots represent JD0, the green boxplots JD1, and the yellow boxplots JD2.
In the energy allocation metabolism class, L-palmitoyl-carnitine was found to continuously increase (Figure 7A), whereas linoleic acid decreased during migration (Figure 7B). For amino acids, the contents of phenylalanine, aspartate, alanine, tyrosine, valine, and isoleucine decreased continuously and significantly throughout the migration (Figures 7C–H).
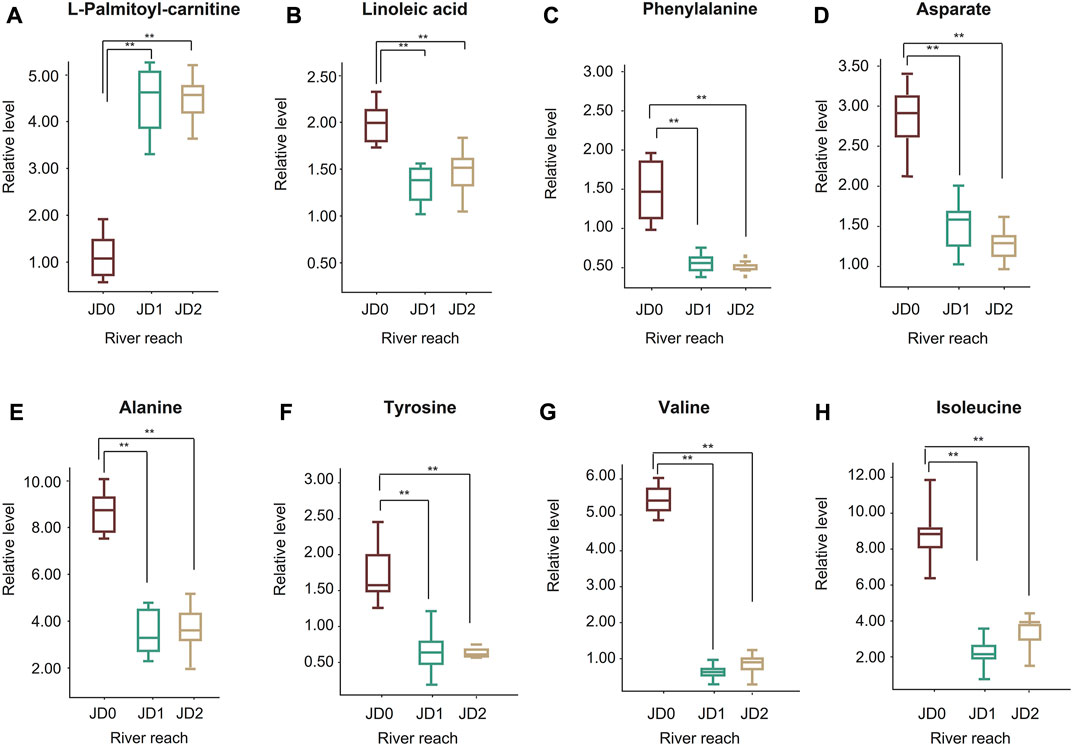
FIGURE 7. Box-whisker plots of some target metabolites associated with energy metabolism. L-palmitoyl-carnitine (A); Linoleic acid (B); Phenylalanine (C); Aspartate (D); Alanine (E); Tyrosine (F); Valine (G); Isoleucine (H). The red boxplots represent JD0, the green boxplots JD1, and the yellow boxplots JD2.
The metabolites involved in ammonia detoxification were found to change significantly. The expression level of glutamine continued to decline to an average depletion of 3.2-fold lower relative to JD0 (Figure 8A). The expression level of 5-hydroxyheteroester hydrolase (HIUase) was found to be significantly higher at JD2 than at JD1 and JD0 (Figure 8B).
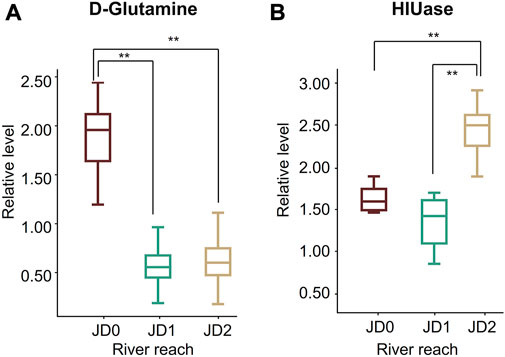
FIGURE 8. Box-whisker plots of some target metabolites associated with ammonia metabolism. D-glutamine (A); 5-hydroxyheteroester hydrolase, HIUase (B). The red boxplots represent JD0, the green boxplots JD1, and the yellow boxplots JD2.
4 Discussion
4.1 Metabolites in osmoregulation
The first challenge experienced by Amur ide during migration is the drastic change in external osmotic pressure from alkaline-saline water to freshwater environments. Metabolism plasticity is necessary to reach optimal conditions for successful adaptability and spawning. The metabolism plasticity involved is not limited to metabolite compensation and metabolic pathways, but also occurs at the molecular level with either upregulating or downregulating of associated genes. One of the metabolites discovered in this study was arachidonic acid (ArA), known to regulate the external ion channels present on the surface of various cell membranes, such as smooth muscle cells and cardiovascular cells, thereby maintaining intra-and-extracellular homeostasis of the cells (De Souza et al., 2016; Zeng et al., 2017; Yu et al., 2021). In principle, fatty acid composition in feed affects osmotic pressure regulation in fish and consumption of ArA-rich feeds improves stress resistance (Atalah et al., 2011). For example, ArA can remarkably assist in regulating Na+/K+-ATPase (NKA) gene expression as well as enzyme activity, which maintains cellular osmotic balance in the gilthead seabream (Sparus aurata) (Van Anholt et al., 2004) and the black sea bass (Centropristis striata) (Carrier et al., 2011). In addition, salinity is known to affect fatty acid composition in fish tissues. Atlantic salmon (Salmo salar) (Zheng et al., 2005), yellow-spotted bluefish (Siganus canaliculatus) (Li et al., 2008), and red sea bream (Pagrus major) (Sarker et al., 2011) exposed to low salinity conditions were found to have higher long-chain polyunsaturated fatty acid content (LC-PUFA). This suggests that when fish encounter different salinities, adjusting lipid metabolism to increase LC-PUFA synthesis in the cell membranes is necessary to regulate ionic permeability and to facilitate adaptation to the changing salinity environment. This is also consistent with our study in that the levels of ArA increased in samples from the first sampling site (JD1) and decreased in individuals from the second site (JD2) during migration, which demonstrated that ArA contributed an important role in osmotic regulation during the migration of Amur ide from alkali-saline water to freshwater.
Besides ArA, cortisol has long been known as an essential corticosteroid hormone, that affects ionic and osmo-regulation in teleosts (Evans et al., 2005). In many euryhaline teleosts, cortisol may regulate branchial NKA activity and its transcription and interacts with other transporters to facilitate saline water adaptation (McCormick, 2001; Sunny and Oommen, 2001). In this study, high cortisol levels were found in fish collected from JD0 with alkaline water, and JD1, a transition environment between alkaline water and freshwater. Low cortisol levels were found in fish from JD2 with freshwater environment. This is consistent with our recent study, which showed that serum cortisol levels as well as gill NKA activity and its mRNA expression in the alkali form of Amur ide increased with prolonged bicarbonate exposure (Chang et al., 2021). The upregulation of NKA expression and activities in association with cortisol levels was also reported previously in common carp (Cyprinus carpio) administrated with cortisol and forced to exercise till exhausted (Liew et al., 2015; Liew et al., 2020).
Moreover, we also observed that the levels of other metabolites, such as PGE2 and PGF2α, were expressed significantly higher at JD1 compared with JD0 and JD2. The upregulation of PGE2 and PGF2α found in the transfer of Amur ide from alkaline water to freshwater is believed to act as a secondary messenger to induce cortisol secretion to the hypothalamus-pituitary-interrenal cell (HPI) axis for signaling physiological adjustment in maintaining basal metabolism demands during migration (Martins et al., 2013; Zhang et al., 2017). Hence, higher concentration of cortisol found in Amur ide from JD0 and JD1 was conducive for biological reactions to handle the osmotic pressure change due to the transition from hypertonic to hypotonic environments, with osmoregulatory activation of ArA and associated metabolites involved in osmotic pressure and steroid hormone regulation (Figure 9).
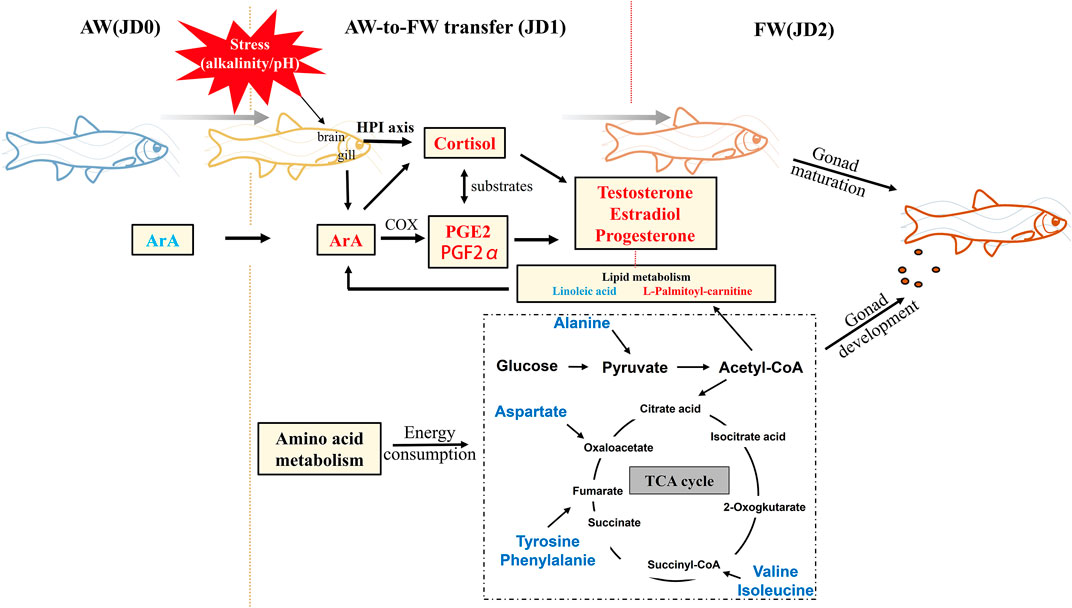
FIGURE 9. Hypothetical schematic of the changes in metabolites associated with osmotic pressure, gonadal development and energy allocation. Red text indicates metabolites whose expression are increased in the corresponding river reach and blue text indicates decreased.
4.2 Metabolites in gonadal maturation
Steroid hormones are the essential messenger in regulating the development and maturation of gonad in fish (Villamarín et al., 2016; Ma et al., 2019). In this study, steroid hormones of Amur ide significantly overexpressed during migration (Figure 7). Compared with JD0, the concentrations of dehydroepiandrosterone sulfate (DHEA) increased dramatically following the migration route at JD1 and JD2. This metabolite is known to be used in the conversion to more active sex hormones, such as androsterone and testosterone for gonad maturation (Boxer et al., 2010; Xu et al., 2016; Liu et al., 2017). In addition, as the most active estrogen, the expression of estradiol-17β was also upregulated throughout the spawning migration period. This implies that the sex steroid hormone biosynthesis pathways were activated by the dramatic changes of osmotic pressure to stimulate gonad maturation. Similarly, transcriptomic studies also confirmed that the genes related to sex steroid hormones are expressed in Amur ide following the transfer from alkali-saline water to freshwater for spawning (Chen et al., 2019).
Besides sex steroid hormones, PGF2α and PGE2 in ArA metabolism are also important in regulating reproductive hormone secretion, thereby altering sexual behavior in teleost fish (Mercure and Van Der Kraak, 1996). For example, female goldfish (Carassius auratus) lay their eggs using postovulatory PGF as a hormone for synchronizing the sexual behaviors with the occurrence of eggs ovulated inside ovary. This stimulates males to make responses to the released PGFs meanwhile initiating metabolites to complete the mating process (Sorensen et al., 2018). Moreover, cortisol appears to stimulate prostaglandin secretion, thereby enhancing progesterone and estradiol-17β secretion to improve fertilization and embryo development (Cobbetti and Zerani, 1992; Sirois, 1994; Sirois and Doré, 1997; Mikuni et al., 1998; Duffy and Stouffer, 2001; Duong et al., 2011). Combined with osmoregulation data, the changes of PGF2α and PGE2 stimulated by the transition from alkali-saline water (JD0) to brackish water (JD1) were found to significantly increase the synthesis of sex hormone-related precursors, which ultimately promote the gonadal maturation of Amur ide. This also indicated that the ArA pathway and the steroid synthesis pathway were involved synergistically in the process of osmoregulation and gonadal maturation during spawning migration of Amur ide (Figure 9).
4.3 Metabolites in energy supply
During long-distance spawning migrations, fish spend energy from feed intake and body reserves for locomotion, osmoregulation, and gonadal maturation (Yin et al., 2020). In this study, the pathway-based integration analysis identified that essential metabolites were expressed differentially to support different energy mobilization needs, like degradation of fatty acid, glycolysis, the TCA cycle, oxidative phosphorylation, biosynthesis of steroid hormone, as well as linoleic acid metabolism. According to relative expression levels, we found that fatty acid-degradation-related metabolites, such as L-Palmitoyl-carnitine, were upregulated to imply the synthesis of ATP from fatty acid β-oxidation. This also suggests that the oxidation of fatty acids generates more energy to support basic metabolism, e.g., swimming, as well as gonadal development. Furthermore, linoleic acid as a non-esterified fatty acid was found to be downregulated as it is utilized during the energy production through a β-oxidation with the ArA production (Wang et al., 2020).
During the spawning migration, the content of non-essential amino acids, like phenylalanine and tyrosine, exhibited an obvious reduction. (Chang et al., 2016) found that the content of non-essential amino acids in the plasma of Amur ide presented an increase with higher alkalinity exposure, which is speculated to be used as an energy supply that helps the fish cope with hyperosmotic pressure in the alkaline environment. The decreased levels of amino acids in the freshwater river revealed that the consumption rate was greater than the synthesis involved with the activation of the TCA cycle that generates energy for spawning (Figure 9).
4.4 Metabolites in ammonia metabolism
Exposure of fish to high pH or alkaline conditions is known to lead to ammonia excretion disturbances that might result in ammonia intoxication (Wicks and Randall, 2002). Most alkali-tolerant fishes can avoid ammonia accumulation by either inducing active ammonia excretion or making endogenous ammonia less toxic, such as free amino acids, glutamine, or urea (Wang et al., 2003; Sachs et al., 2006). The excessive ammonia is converted to glutamate through glutamate dehydrogenase (GDH), followed by converting to glutamine through glutamine synthetase (Gsase), hence the fish could temporarily store ammonia in a neutral form until the fish returns to a more favorable environment for excretion (Wicks and Randall, 2002). In this study, glutamine levels of Amur ide from JD0 were significantly higher than JD1 and JD2. Thus, the higher level of glutamine in Amur ide from JD0 indicated that the ammonia excretion system was impeded under the extreme alkali-saline environment with elevated pH. In contrast; the lower levels of glutamine in JD1 and JD2 suggested that ammonia excretion was gradually recovered and energy expense in converting ammonia to glutamine was reduced. Furthermore, as an alternative mechanism for ammonia excretion, the level of 5-hydroxyisourate hydrolase (HIUase) was lower in JD0 and JD1 compared with JD2. HIUase is an important hydrolase of urea conversion to ammonia and carbon dioxide (Forconi et al., 2014), which highlights an improvement of the purine metabolic pathway (uricolysis) in Amur ide under freshwater conditions. In short, the above results showed that ammonia excretion was hindered in Lake Dali and the estuary. When Amur ide re-acclimate to the freshwater environment, ammonia excretion recovers (He et al., 2016). This provides sufficient energy for the synthesis of sex hormone-related precursors and speeds up the gonadal pro-maturation process.
5 Conclusion
This study revealed that the dynamic changes of serum metabolome, caused by different osmoregulation responses to different environments along a migration route, as well as gonadogenesis, were crucial for Amur ide to maximize annual spawning success. Among important metabolomes associated with migratory swimming performance, pathways included ArA metabolism, biosynthesis of steroid hormone, amino acid synthesis, and metabolism, pyruvate metabolism, TCA cycle pathways, and glycerophospholipid metabolism. ArA and its derivatives, and sex steroid hormones, were responsible for osmotic pressure regulation and gonadal maturation to improve spawning performance. The study assists in understanding the physiological mechanisms underlying Amur ide underline spawning migration. Identified target metabolites related to osmoregulation, gonad maturation and ammonia metabolism will be useful for breeding programs of alkaline-tolerant fish.
Data availability statement
The raw data supporting the conclusion of this article will be made available by the authors, without undue reservation.
Ethics statement
The animal study was reviewed and approved by the Animal Care and Use Committee of Heilongjiang River Fisheries Research Institute of Chinese Academy of Fishery Sciences. Written informed consent was obtained from the owners for the participation of their animals in this study.
Author contributions
YC, LL, BaS designed and supervised the project. YC, SW wrote the manuscript. HL provided inputs and guided writing with constructive suggestions. SW and JH analyzed the data. YZ and LZ assisted samples collection. BoS assisted in experiments.
Funding
National Key R & D Program of China (2019YFD0900405); National Natural Science Foundation of China (32273120). Central Public-interest Scientific Institution Basal Research Fund, CAFS (2017HY-ZD0404).
Conflict of interest
Author SW was employed by the company BGI Genomics.
The remaining authors declare that the research was conducted in the absence of any commercial or financial relationships that could be construed as a potential conflict of interest.
Publisher’s note
All claims expressed in this article are solely those of the authors and do not necessarily represent those of their affiliated organizations, or those of the publisher, the editors and the reviewers. Any product that may be evaluated in this article, or claim that may be made by its manufacturer, is not guaranteed or endorsed by the publisher.
References
Aru, V., Khakimov, B., Sørensen, K. M., Chikwati, E. M., Kortner, T. M., Midtlyng, P., et al. (2021). The plasma metabolome of atlantic salmon as studied by 1H NMR spectroscopy using standard operating procedures: Effect of aquaculture location and growth stage. Metabolomics 17, 50. doi:10.1007/s11306-021-01797-0
Atalah, E., Hernández Cruz, C. M., Ganuza, E., Benítez Santana, T., Ganga, R., Roo, J., et al. (2011). Importance of dietary arachidonic acid for the growth, survival and stress resistance of larval European sea bass (Dicentrarchus labrax) fed high dietary docosahexaenoic and eicosapentaenoic acids. Aquac. Res. 42, 1261–1268. doi:10.1111/j.1365-2109.2010.02714.x
Barker, M., and Rayens, W. (2003). Partial least squares for discrimination. J. Chemom. 17, 166–173. doi:10.1002/cem.785
Benskin, J. P., Ikonomou, M. G., Liu, J., Veldhoen, N., Dubetz, C., Helbing, C. C., et al. (2014). Distinctive metabolite profiles in in-migrating Sockeye salmon suggest sex-linked endocrine perturbation. Environ. Sci. Technol. 48, 11670–11678. doi:10.1021/es503266x
Boxer, R. S., Kleppinger, A., Brindisi, J., Feinn, R., Burleson, J. A., and Kenny, A. M. (2010). Effects of dehydroepiandrosterone (DHEA) on cardiovascular risk factors in older women with frailty characteristics. Age Ageing 39, 451–458. doi:10.1093/ageing/afq043
Carrier, J. K., Watanabe, W. O., Harel, M., Rezek, T. C., Seaton, P. J., and Shafer, T. H. (2011). Effects of dietary arachidonic acid on larval performance, fatty acid profiles, stress resistance, and expression of Na +/K + ATPase mRNA in black sea bass Centropristis striata. Aquaculture 319, 111–121. doi:10.1016/j.aquaculture.2011.06.027
Chagoyen, M., and Pazos, F. (2011). MBRole: Enrichment analysis of metabolomic data. Bioinformatics 27, 730–731. doi:10.1093/bioinformatics/btr001
Chang, Y. M., He, Q., Sun, Y. C., Liang, L. Q., and Sun, X. W. (2016). Changes in plasma free amino acid levels in Leuciscus waleckii exposed to different environmental alkalinity levels (in Chinese). J. Fish. Sci. China 23, 117–124. doi:10.3724/SP.J.1118.2016.15080
Chang, Y. M., Tang, R., Dou, X. J., Tao, R., Sun, X. W., and Liang, L. Q. (2014). Transcriptome and expression profiling analysis of Leuciscus waleckii: An exploration of the alkali-adapted mechanisms of a freshwater teleost. Mol. Biosyst. 10, 491–504. doi:10.1039/c3mb70318e
Chang, Y. M., Tang, R., Sun, X. W., Liang, L. Q., Chen, J. P., Huang, J. F., et al. (2013). Genetic analysis of population differentiation and adaptation in Leuciscus waleckii. Genetica 141, 417–429. doi:10.1007/s10709-013-9741-6
Chang, Y. M., Zhao, X. F., Liew, H. J., Sun, B., Wang, S. Y., Luo, L., et al. (2021). Effects of bicarbonate stress on serum ions and gill transporters in alkali and freshwater forms of Amur ide (Leuciscus waleckii). Front. Physiol. 12, 676096. doi:10.3389/fphys.2021.676096
Chen, B. H., Xu, J., Cui, J., Pu, F., Peng, W. Z., Chen, L., et al. (2019). Transcriptional differences provide insight into environmental acclimatization in wild amur ide (Leuciscus waleckii) during spawning migration from alkalized lake to freshwater river. Genomics 111, 267–276. doi:10.1016/j.ygeno.2018.11.007
Chen, C., Grennan, K., Badner, J., Zhang, D., Gershon, E., Jin, L., et al. (2011). Removing batch effects in analysis of expression microarray data: An evaluation of six batch adjustment methods. PLoS One 6, e17238. doi:10.1371/journal.pone.0017238
Chi, B. J., Chang, Y. M., Yan, X. C., Cao, D. C., Gao, Y. K., Liu, Y. H., et al. (2010). Genetic variability and genetic structure of Leuciscus waleckii dybowski in wusuli river and Dali lake (in Chinese). J. Fish. Sci. China 17, 228–235.
Chi, B. J. (2010). Genetic variability of Amur ide (Dybowski) and construction of the cDNA substractive library of saline-alkaline tolerance. Shanghai: Shanghai Ocean University. doi:10.7666/d.y1821851
Cobbetti, A., and Zerani, M. (1992). PGF2 alpha, PGE2, and sex steroids from the abdominal gland of the male crested newt Triturus carnifex (Laur.). Prostaglandins 43, 101–109. doi:10.1016/0090-6980(92)90079-9
Cui, J., Xu, J., Zhang, S. H., Wang, K., Jiang, Y. L., Mahboob, S., et al. (2015). Transcriptional profiling reveals differential gene expression of amur ide (Leuciscus waleckii) during spawning migration. Int. J. Mol. Sci. 16, 13959–13972. doi:10.3390/ijms160613959
De Souza, E. O., Lowery, R. P., Wilson, J. M., Sharp, M. H., Mobley, C. B., Fox, C. D., et al. (2016). Effects of arachidonic acid supplementation on acute anabolic signaling and chronic functional performance and body composition adaptations. PLoS One 11, e0155153. doi:10.1371/journal.pone.0155153
Degu, A., Hochberg, U., Sikron, N., Venturini, L., Buson, G., Ghan, R., et al. (2014). Metabolite and transcript profiling of berry skin during fruit development elucidates differential regulation between Cabernet Sauvignon and Shiraz cultivars at branching points in the polyphenol pathway. BMC Plant Biol. 14, 188–220. doi:10.1186/s12870-014-0188-4
Duffy, D. M., and Stouffer, R. L. (2001). The ovulatory gonadotrophin surge stimulates cyclooxygenase expression and prostaglandin production by the monkey follicle. Mol. Hum. Reprod. 7, 731–739. doi:10.1093/molehr/7.8.731
Dunn, W. B., Broadhurst, D., Begley, P., Zelena, E., Francis-McIntyre, S., Anderson, N., et al. (2011). Procedures for large-scale metabolic profiling of serum and plasma using gas chromatography and liquid chromatography coupled to mass spectrometry. Nat. Protoc. 6, 1060–1083. doi:10.1038/nprot.2011.335
Duong, H. T., Piotrowska-Tomala, K. K., Acosta, T. J., Bah, M. M., Sinderewicz, E., Majewska, M., et al. (2011). Effects of cortisol on pregnancy rate and corpus luteum function in heifers: An in vivo study. J. Reprod. Dev. 58, 223–230. doi:10.1262/jrd.11-122t
Evans, D. H., Piermarini, P. M., and Choe, K. P. (2005). The multifunctional fish gill: Dominant site of gas exchange, osmoregulation, acid-base regulation, and excretion of nitrogenous waste. Physiol. Rev. 85, 97–177. doi:10.1152/physrev.00050.2003
Forconi, M., Biscotti, M. A., Barucca, M., Buonocore, F., De Moro, G., Fausto, A. M., et al. (2014). Characterization of purine catabolic pathway genes in coelacanths. J. Exp. Zool. 322, 334–341. doi:10.1002/jez.b.22515
Geng, K., and Zhang, Z. C. (1988). Eomorphologic features and evolution of the Holocene lakes in Dali nor area (in Chinese). J. Beijing Normal Univ. Nat. Sci., 25, 94–101.
He, Q., Chang, Y. M., Su, B. F., Sun, B., Sun, X., and Liang, L. Q. (2016). Effects of carbonate alkalinities on oxygen consumption, ammonia excretion and ammonia excretion gene expression in Leuciscus waleckii Dybowski (in Chinese). J. Shanghai Ocean Univ. 25, 551–558. doi:10.3969/j.issn.2095-1736.2016.06.048
Li, Y. Y., Hu, C. B., Zheng, Y. J., Xia, X. A., Xu, W. J., Wang, S. Q., et al. (2008). The effects of dietary fatty acids on liver fatty acid composition and Δ6-desaturase expression differ with ambient salinities in Siganus canaliculatus. Comp. Biochem. Physiology Part B Biochem. Mol. Biol. 151, 183–190. doi:10.1016/j.cbpb.2008.06.013
Liew, H. J., Fazio, A., Faggio, C., Blust, R., and De Boeck, G. (2015). Cortisol affects metabolic and ionoregulatory responses to a different extent depending on feeding ration in common carp, Cyprinus carpio. Comp. Biochem. physiology part A Mol. Integr. physiology 189, 45–57. doi:10.1016/j.cbpa.2015.07.011
Liew, H. J., Pelle, A., Chiarella, D., Faggio, C., Tang, C., Blust, R., et al. (2020). Common carp, Cyprinus carpio, prefer branchial ionoregulation at high feeding rates and kidney ionoregulation when food supply is limited: Additional effects of cortisol and exercise. Fish. Physiol. Biochem. 46, 451–469. doi:10.1007/s10695-019-00736-0
Liu, J. L., Zhang, W. Q., Zhao, M., and Huang, M. Y. (2017). Integration of transcriptomic and metabolomic data reveals enhanced steroid hormone biosynthesis in mouse uterus during decidualization. Proteomics 17, 1700059. doi:10.1002/pmic.201700059
Ma, F. J., Yang, Y. P., Jiang, M., Yin, D. H., and Liu, K. (2019). Digestive enzyme activity of the Japanese grenadier anchovy Coilia nasus during spawning migration: Influence of the migration distance and the water temperature. J. Fish. Biol. 95, 1311–1319. doi:10.1111/jfb.14136
Martins, D. A., Rocha, F., Castanheira, F., Mendes, A., Pousão-Ferreira, P., Bandarra, N., et al. (2013). Effects of dietary arachidonic acid on cortisol production and gene expression in stress response in Senegalese sole (Solea senegalensis) post-larvae. Fish. Physiol. Biochem. 39, 1223–1238. doi:10.1007/s10695-013-9778-6
McCormick, S. D. (2001). Endocrine control of osmoregulation in teleost fish. Am. Zool. 41, 781–794. doi:10.1093/icb/41.4.781
Mercure, F., and Van Der Kraak, G. (1996). Mechanisms of action of free arachidonic acid on ovarian steroid production in the goldfish. Gen. Comp. Endocrinol. 102, 130–140. doi:10.1006/gcen.1996.0054
Mikuni, M., Pall, M., Peterson, C. M., Peterson, C. A., Hellberg, P., Brännström, M., et al. (1998). The selective prostaglandin endoperoxide synthase-2 inhibitor, NS-398, reduces prostaglandin production and ovulation in vivo and in vitro in the Rat1. Biol. Reprod. 59, 1077–1083. doi:10.1095/biolreprod59.5.1077
Sachs, G., Kraut, J. A., Wen, Y., Feng, J., and Scott, D. R. (2006). Urea transport in bacteria: Acid acclimation by gastric Helicobacter spp. J. Membr. Biol. 212, 71–82. doi:10.1007/s00232-006-0867-7
Sarker, M. A., Yamamoto, Y., Haga, Y., Sarker, M. S. A., Miwa, M., Yoshizaki, G., et al. (2011). Influences of low salinity and dietary fatty acids on fatty acid composition and fatty acid desaturase and elongase expression in red sea bream Pagrus major. Fish. Sci. 77, 385–396. doi:10.1007/s12562-011-0342-y
Sirois, J., and Doré, M. (1997). The late induction of prostaglandin G/H synthase-2 in equine preovulatory follicles supports its role as a determinant of the ovulatory Process1. Endocrinology 138, 4427–4434. doi:10.1210/endo.138.10.5462
Sirois, J. (1994). Induction of prostaglandin endoperoxide synthase-2 by human chorionic gonadotropin in bovine preovulatory follicles in vivo. Endocrinology 135, 841–848. doi:10.1210/endo.135.3.8070377
Sorensen, P. W., Appelt, C., Stacey, N. E., Goetz, F. W., and Brash, A. R. (2018). High levels of circulating prostaglandin F2α associated with ovulation stimulate female sexual receptivity and spawning behavior in the goldfish (Carassius auratus). Gen. Comp. Endocrinol. 267, 128–136. doi:10.1016/j.ygcen.2018.06.014
Sunny, F., and Oommen, O. V. (2001). Rapid action of glucocorticoids on branchial ATPase activity in Oreochromis mossambicus: An in vivo and in vitro study. Comp. Biochem. Physiology Part B Biochem. Mol. Biol. 130, 323–330. doi:10.1016/s1096-4959(01)00438-9
Tamario, C., Sunde, J., Petersson, E., Tibblin, P., and Forsman, A. (2019). Ecological and evolutionary consequences of environmental change and management actions for migrating fish. Front. Ecol. Evol. 7, 271. doi:10.3389/fevo.2019.00271
Team, R. C. (2013). R: A language and environment for statistical computing. Vienna: R Foundation for Statistical Computing. Available at: https://www.R-project.org/
Van Anholt, R. D., Koven, W. M., Lutzky, S., and Bonga, S. W. (2004). Dietary supplementation with arachidonic acid alters the stress response of gilthead seabream (Sparus aurata) larvae. Aquaculture 238, 369–383. doi:10.1016/j.aquaculture.2004.06.001
Villamarín, F., Magnusson, W. E., Jardine, T. D., Valdez, D., Woods, R., and Bunn, S. E. (2016). Temporal uncoupling between energy acquisition and allocation to reproduction in a herbivorous-detritivorous fish. PLoS One 11, e0150082. doi:10.1371/journal.pone.0150082
Wang, M. Y., Xu, P., and Zhu, Z. X. (2020). Regulation of signal transduction in Coilia nasus during migration. Genomics 112, 55–64. doi:10.1016/j.ygeno.2019.07.021
Wang, S. Y., Kuang, Y. Y., Liang, L. Q., Sun, B., Zhao, X., Zhang, L., et al. (2021). Resequencing and SNP discovery of Amur ide (Leuciscus waleckii) provides insights into local adaptations to extreme environments. Sci. Rep. 11, 5064–5114. doi:10.1038/s41598-021-84652-5
Wang, Y. S., Gonzalez, R. J., Patrick, M. L., Grosell, M., Zhang, C., Feng, Q., et al. (2003). Unusual physiology of scale-less carp, Gymnocypris przewalskii, in Lake qinghai: A high altitude alkaline saline lake. Comp. Biochem. Physiology Part A Mol. Integr. Physiology 134, 409–421. doi:10.1016/s1095-6433(02)00317-3
Wen, B., Mei, Z. L., Zeng, C. W., and Liu, S. (2017). metaX: a flexible and comprehensive software for processing metabolomics data. BMC Bioinforma. 18, 183–214. doi:10.1186/s12859-017-1579-y
Westerhuis, J. A., Hoefsloot, H. C., Smit, S., Vis, D. J., Smilde, A. K., van Velzen, E. J., et al. (2008). Assessment of PLSDA cross validation. Metabolomics 4, 81–89. doi:10.1007/s11306-007-0099-6
Wicks, B. J., and Randall, D. J. (2002). The effect of sub-lethal ammonia exposure on fed and unfed rainbow trout: The role of glutamine in regulation of ammonia. Comp. Biochem. Physiology Part A Mol. Integr. Physiology 132, 275–285. doi:10.1016/s1095-6433(02)00034-x
Xiao, J. L., Si, B., Zhai, D. Y., Itoh, S., and Lomtatidze, Z. (2008). Hydrology of Dali lake in central-eastern inner Mongolia and Holocene east asian monsoon variability. J. Paleolimnol. 40, 519–528. doi:10.1007/s10933-007-9179-x
Xu, G. C., Du, F. K., Li, Y., Nie, Z. J., and Xu, P. (2016). Integrated application of transcriptomics and metabolomics yields insights into population-asynchronous ovary development in Coilia nasus. Sci. Rep. 6, 31835–31911. doi:10.1038/srep31835
Xu, J., Ji, P. F., Wang, B. S., Zhao, L., Wang, J., Zhao, Z., et al. (2013). Transcriptome sequencing and analysis of wild Amur Ide (Leuciscus waleckii) inhabiting an extreme alkaline-saline lake reveals insights into stress adaptation. PLoS One 8, e59703. doi:10.1371/journal.pone.0059703
Xu, J., Li, J. T., Jiang, Y. L., Peng, W. Z., Yao, Z. L., Chen, B. H., et al. (2017). Genomic basis of adaptive evolution: The survival of amur ide (Leuciscus waleckii) in an extremely alkaline environment. Mol. Biol. Evol. 34, 145–159. doi:10.1093/molbev/msw230
Yin, D. H., Lin, D. Q., Ying, C. P., Ma, F. J., Yang, Y. P., Wang, Y. P., et al. (2020). Metabolic mechanisms of Coilia nasus in the natural food intake state during migration. Genomics 112, 3294–3305. doi:10.1016/j.ygeno.2020.05.027
Yu, J., Wen, X., You, C., Wang, S., Chen, C., Tocher, D. R., et al. (2021). Comparison of the growth performance and long-chain polyunsaturated fatty acids (LC-PUFA) biosynthetic ability of red tilapia (Oreochromis mossambicus♀× O. niloticus♂) fed fish oil or vegetable oil diet at different salinities. Aquaculture 542, 736899. doi:10.1016/j.aquaculture.2021.736899
Yu, Z., Hongbin, W., Jiang, H., Haitao, L., Cunliang, Z., Zhixin, W., et al. (2008). Investigation of water quality in the dalinor lake in inner Mongolia. Fish. Sci. 27, 1–11. doi:10.16378/j.cnki.1003-1111.2008.12.002
Zeng, C. W., Wen, B., Hou, G. X., Lei, L., Mei, Z. L., Jia, X. K., et al. (2017). Lipidomics profiling reveals the role of glycerophospholipid metabolism in psoriasis. Gigascience 6, 1–11. doi:10.1093/gigascience/gix087
Zhang, H., Liu, Y., Weng, J., Usuda, K., Fujii, K., Watanabe, G., et al. (2017). Decrease of lactogenic hormones induce epithelial-mesenchymal transition via TGFβ1 and arachidonic acid during mammary gland involution. J. Reprod. Dev. 63, 325–332. doi:10.1262/jrd.2016-157
Zhao, X. F., Liang, L. Q., Liew, H. J., Chang, Y. M., Sun, B., Wang, S. Y., et al. (2021). Identification and analysis of long non-coding RNAs in Leuciscus waleckii adapted to highly alkaline conditions. Front. Physiol. 785, 665268. doi:10.3389/fphys.2021.665268
Zheng, X. Z., Torstensen, B. E., Tocher, D. R., Dick, J. R., Henderson, R. J., and Bell, J. G. (2005). Environmental and dietary influences on highly unsaturated fatty acid biosynthesis and expression of fatty acyl desaturase and elongase genes in liver of Atlantic salmon (Salmo salar). Biochimica Biophysica Acta - Mol. Cell Biol. Lipids 1734, 13–24. doi:10.1016/j.bbalip.2005.01.006
Zhu, Y. H., Wu, J. M., Leng, X. Q., Du, H., Wu, J. P., He, S., et al. (2020). Metabolomics and gene expressions revealed the metabolic changes of lipid and amino acids and the related energetic mechanism in response to ovary development of Chinese sturgeon (Acipenser sinensis). PLoS One 15, e0235043. doi:10.1371/journal.pone.0235043
Keywords: Amur ide (Leuciscus waleckii), spawning migration, metabolome, osmoregulation, gonadal maturation
Citation: Wang S, Huang J, Liang L, Su B, Zhang Y, Liew HJ, Sun B, Zhang L and Chang Y (2022) Distinctive metabolite profiles in migrating Amur ide (Leuciscus waleckii) reveal changes in osmotic pressure, gonadal development, and energy allocation strategies. Front. Environ. Sci. 10:997827. doi: 10.3389/fenvs.2022.997827
Received: 19 July 2022; Accepted: 28 September 2022;
Published: 12 October 2022.
Edited by:
Benjamin Cook, FRC Environmental, AustraliaReviewed by:
Pronob Das, Central Inland Fisheries Research Institute (ICAR), IndiaChuanju Dong, Henan Normal University, China
Copyright © 2022 Wang, Huang, Liang, Su, Zhang, Liew, Sun, Zhang and Chang. This is an open-access article distributed under the terms of the Creative Commons Attribution License (CC BY). The use, distribution or reproduction in other forums is permitted, provided the original author(s) and the copyright owner(s) are credited and that the original publication in this journal is cited, in accordance with accepted academic practice. No use, distribution or reproduction is permitted which does not comply with these terms.
*Correspondence: Yumei Chang, changyumei@hrfri.ac.cn