UAVs for improving seasonal vegetation assessment in arid environments
- 1Geography Department, College of Arts and Social Sciences, Sultan Qaboos University, Muscat, Oman
- 2Department of Ecology and Conservation Biology, Texas A&M University, College Station, Hawali, TX, United States
- 3Natural Environmental Systems and Technologies (NEST) Research Group, Ecolife Sciences Research and Consultation, Hawally, Kuwait
- 4Ecoresolve, San Francisco, CA, United States
- 5Applied Geography and GIS Program, Department of Humanities, College of Arts and Sciences, Qatar University, Doha, Qatar
- 6Department of Forest Analytics, Texas A&M Forest Service, Dallas, TX, United States
- 7Department of Geography, University of California—Berkeley, Berkeley, CA, United States
In the last few decades, revegetation strategies for ecosystem restoration have received great attention in dryland studies, especially those related to the restoration and revegetation of native desert plants to combat land degradation. Long-term monitoring and assessment are critical for the restoration programs to track the progress of the restoration program goals. The effectiveness and success of monitoring depend on the selected methods with respect to spatial and temporal scales. Traditional methods for vegetation monitoring are time-consuming, expensive, and require considerable labor efforts (manpower) in terms of field measurements, collecting samples, lab analysis, and the difficulty of accessing some study areas. Thus, satellite remote sensing images have been widely used to monitor land degradation and restoration programs using multispectral and hyperspectral sensors and indices such as NDVI, which is the most popular index for vegetation monitoring. However, such techniques showed many limitations when used in arid ecosystems, especially for seasonal vegetation assessments, which could mislead the monitoring and assessment of the restoration projects. This paper discusses lessons learned from previous research work, including the limitations of using satellite remote sensing in arid ecosystems and the use of UAV methods to overcome these issues and challenges to provide more accurate outcomes for seasonal assessment of vegetation in arid landscapes.
1 Introduction
Arid ecosystems support over 38% of the population worldwide and are critically important, especially in countries of the Middle East, as they support crop production, livestock grazing, and ecosystem services (Laliberte et al., 2007). These are complex ecosystems, where the interaction between plants, water, soil, nutrients, biodiversity, and climate play a significant role in the growth and distribution of native plants, and require ecosystem-specific methodologies to explore the relationships. In addition, the stability and functioning have been affected due to climate change impacts and anthropogenic influences, leading to desertification and loss of ecosystem services. Hence there is a need for accurate and comprehensive assessment of these ecosystems and to generate clear site-specific ecological descriptions in a timely manner to protect and restore such ecosystems. Also, the cultivation of native plants in urban areas is crucial, particularly in light of climate change and the rise in CO2 emissions, as well as the depletion of water resources in arid regions. Native plants are an excellent alternative as they act as a CO2 sink and do not require water resources, as they are already adapted to the environment.
The most common methodology for monitoring arid ecosystems has been the collection and interpretation of field data. However, this is time-consuming, cost-efficient, labor-intensive, and not suitable for large or inaccessible study areas. As a consequence, remote sensing (RS) data, especially satellite data, has been utilized in recent years for this ecosystem assessment. Some of the satellite remote sensing (SRS) based applications include monitoring of large-scale vegetation coverage, long-term assessments of changes in native plants, detecting land-cover changes after natural disasters, and managing natural resources (M. M. Abdullah et al., 2017a; Zhu et al., 2019). However, there are still some major concerns and challenges regarding SRS approaches in arid regions.
Due to differences in landscapes and ecosystem structure in arid regions compared to tropical and subtropical ecosystems, where most of the RS indices are built and tested, interpretations should be made with careful consideration. For instance, thresholds of indices such as normalized difference vegetation index (NDVI) for quantifying the health of vegetation can be challenging and even misleading at times if global values are extrapolated to arid environments. These discrepancies can be attributed to seasonal changes which affect what can be interpreted from satellite imagery. The accuracy of mapping native desert plants could vary based on the within and between year variation in temperature and rainfall. For example, plant coverage is almost zero during warm seasons or years with low rainfall, which is inaccurate since perennial plants are present in the field. These plants are difficult to be detected using vegetation indices due to their dormancy during warm seasons. Therefore, it is important to keep in mind these effects during the assessment and monitoring of native desert plants as they could mislead our understanding of the interconnection between plants and climate variations. Additionally, many restoration and management practices depend on the specific site situation and history. With open-source satellite platforms like Landsat, it is possible to acquire data for the past 30 years, but such low resolution is not suitable for delineating relevant information regarding the coverage and distribution of native arid plants. Similarly, issues with clouds, low density of vegetation, and frequency of available data might also provide challenges.
The advancement of unmanned aerial vehicle (UAV) platforms offers new opportunities to address various technical challenges faced by SRS and aerial imagery such as insensitivity to non-photosynthetic vegetation (NPV), accuracy and radiometric factors of the target location, the high reflectance of background soil in arid ecosystems, and lack of data during periods of dense cloud cover (Toutin, 2004; Cui et al., 2013; Tahsin et al., 2017). Especially when dealing with vast landscapes, significant spatial variability within study plots that might not be captured in field data due to unintentionally biased sampling designs could be addressed with UAVs. The affordable prices of UAVs also make them an optimal choice for frequent and ad hoc data collection options when data continuity is required. In addition, this aspect is essential for seasonal studies as changes happen very rapidly and for a shorter period of time and may thus be difficult to detect from satellite imagery. Therefore, UAVs can be used as a way to bridge some gaps between the satellite imagery and field data in terms of spatial and temporal resolution.
Herein, we look into the current status of SRS in the arid environment of the Middle East to understand the specific loopholes and underscore things to keep in mind while pursuing studies related to seasonality. Finally, we provide UAV-based approaches that could resolve some of the technical problems and steps forward for further research.
2 Limitations of satellite remote sensing studies
While SRS can provide useful information for ecological applications, there are several limitations when used in arid environments. These include:
• Desert plants are characterized by small size, tiny leaves, scattered distribution, and lacking vigorous green color. This makes it difficult to detect the coverage and specific types of desert species because of the high reflectance of the soil background (Laliberte et al., 2007).
• Some commonly-used RS vegetation indices and methods are insensitive to NPV, including dry or dead shrubs, leafless drought-deciduous plants, and senescent plants (Cui et al., 2013). NPVs are considered a major component of the total desert surface cover and decrease the risk of soil loss in arid environments (Abdullah M. M. et al., 2017).
• The accuracy of the geographic location and radiometric factors of the target feature can limit the identification and mapping of arid ecosystems. Atmospheric scattering, for example, is influenced by the amount of radiation that reaches the sensors, which can reduce the accuracy of remotely sensed data, and geometric distortions can affect the accuracy of geographic locations (Toutin, 2004).
• In arid environments, vigor and green leaves occur significantly during the wet season. Thus, the desert phenology period is short and associated with sufficient water availability. Medium- and high-spatial-resolution satellites also suffer on days of dense cloud cover, which causes a lack of data, affecting the temporal resolution for long-term monitoring (Figure 1) (Tahsin et al., 2017).
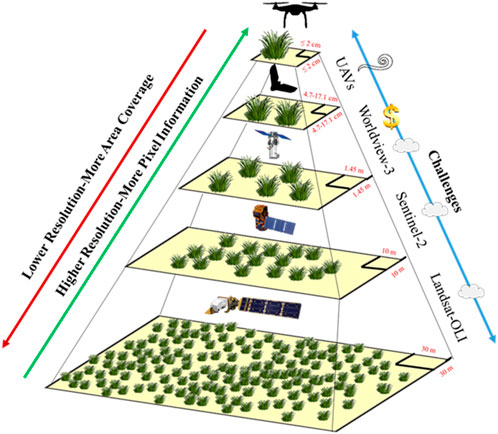
Figure 1. The main challenges of various remotely sensed data: spatial resolution vs. area coverage vs. information details.
3 Advantages of utilizing UAVs for vegetation assessment
UAVs, along with Light Detection and Ranging (LiDAR) sensors (including airborne and terrestrial LiDAR), and multispectral sensors have significant advantages over SRS platforms (Wang et al., 2018; Yao et al., 2019). These advantages include very high-resolution images, with pixel sizes ranging from meters to millimeters, that contribute to the identification of vegetation quickly and with minimal errors, which enables the discrimination between perennial shrubs and small annual grasses in arid ecosystems (Figure 2) (Baena et al., 2017; Gallacher, 2019; Zhang et al., 2019). UAVs can also produce high-resolution Digital Surface Models (DSM), Digital Terrain Models (DTM), and 3D models, which can support ecological studies by assessing aboveground biomass (AGB) and allometric parameters such as diameter at breast height (DBH), canopy cover (CC), crown area (CA), and canopy volume (CV) (Chen et al., 2004; Conti et al., 2013; Ali et al., 2015; Baena et al., 2017; Abdullah et al., 2021). Additionally, UAVs provide higher temporal resolution because the revisit time for image acquisition is on-demand, which plays a pivotal role in filling gaps in satellite data acquisition (Dash et al., 2018). Finally, UAVs can support continuous and long-term monitoring of ecosystem dynamics, such as the spread of invasive species and diseases (Müllerová et al., 2017).
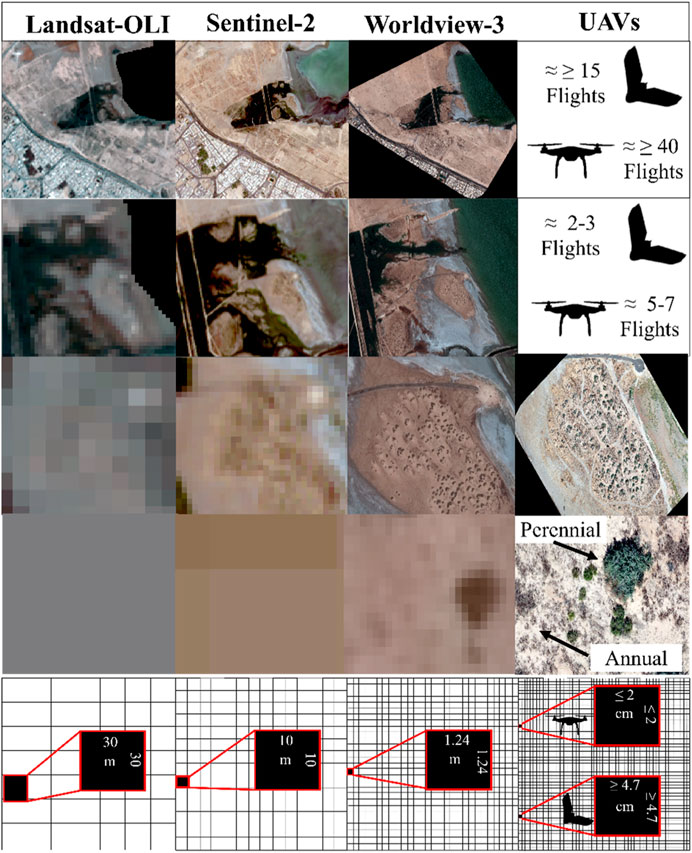
Figure 2. An example of a multi-spatial resolution of different satellite imageries (Landsat-OLI, Sentinel-2, and Worldview-3) and UAVs (Fixed-wing and Multirotor) at Jahra nature reserve in Kuwait (all images were captured in February 2019).
While the choice of remotely sensed data depends on the purpose of the study and the scale of the project, many SRS limitations have been resolved with the development of modern RS technologies, such as micro-UAVs (Figure 1; Figure 2). UAV Remote Sensing is more suitable for small-scale projects for providing details of vegetation information (e.g., species types, vegetation structure and health, and AGB) and for filling gaps in satellite data. The integration of field-based experiments and spatial analysis using UAVs can help in determining ecological indicators to determine suitable areas for the growth of native plants. It was found that annual plants could play a significant role in enhancing the soil by providing approximately 50% higher soil moisture, phosphorus (P), organic matter (OM), and carbon dioxide (CO2) sequestration (REF). Additionally, UAVs are suitable for hard-to-access areas, such as locations with high-density vegetation, wetlands and areas highly impacted by natural hazards (cyclones, floods, landslides and earthquakes). UAV imagery can also be used as the ground-truth or calibration of satellite imagery (Pla et al., 2017). However, satellite imagery is useful for monitoring vegetation coverage at a large scale with an acceptable resolution of 10 m (such as Sentinel-2) in areas that are difficult to cover using UAVs. In this context, UAVs cannot replace satellite imagery; they are considered complementary data and vice versa.
For a local assessment of native plants, transect lines and quadrats can be selected and assessed using very high-resolution techniques. The researcher can obtain a high-resolution image to evaluate the site and select hotspot locations for vegetation assessment, which is difficult to implement using traditional in situ methods. After determining the hotspots, transect lines and/or quadrats can be added to the image using Geographic Information Systems (GIS). The researcher can zoom into the transect line or quadrat to estimate the type of plants and implement all the measurements required for vegetation surveying. The choice of spatial resolution for the image depends on the type of ecosystem and the assessment purpose. For instance, arid ecosystems will require very high-resolution imagery (<2 cm) to define the type of species; however, researchers may face some difficulties in estimating some annual plants with tiny sizes. This approach will allow for the assessment of large landscapes with a shorter period, fewer field visits (which are conducted to capture the imagery), and less labor (manpower), as only a few experts are required to evaluate the quadrats and transect lines using very high-resolution imagery.
4 Next steps and concluding remarks
In order to support decision-makers in better managing scarce resources in arid regions, future research should focus on developing integrated and complex models. This will require:
• Integrating and implementing nexus approaches in the field of ecosystem management and services;
• Integrating field-based experiments and spatial analyses such as RS and GIS to help develop a quantitative characterization of natural ecosystems (soil, water, plant, and atmosphere interaction) and quantitative relationship models of ecological processes within natural systems in arid regions;
• Developing an interdisciplinary research program that involves several researchers in diverse specialized fields, and;
• Encouraging more persuasive communication between the academic community, governmental organizations, and non-governmental organizations.
Our current understanding of the complexity of arid ecosystems does not allow for a clear assessment of interactions among ecosystem elements and the impact of uncertainties, such as climate change, on ecosystems in arid regions. Furthermore, traditional techniques cannot provide a robust assessment, especially at national and regional scales. In order to overcome such challenges and develop a clear understanding of the complexity of ecosystems and the impact of future climate change, it is essential to develop a method for the quantitative characterization of processes of vegetation, soil, water, and atmosphere interactions. The development of RS techniques can help to answer critical ecological questions, as well as develop an exceptional understanding of the complexity of the system at national, regional, and international levels.
Data availability statement
The data analyzed in this study is subject to the following licenses/restrictions: The data that support the findings of this study are available from the corresponding authors, Zahraa Al-Ali, upon reasonable request. Requests to access these datasets should be directed to Zahraa Al Ali, zahraamma84@gmail.com.
Author contributions
MA: Conceptualization, Methodology, Supervision, Visualization, Writing–original draft. ZA-A: Conceptualization, Methodology, Visualization, Writing–original draft. AB: Methodology, Visualization, Writing–review and editing. YC: Writing–review and editing. AA: Writing–review and editing. TA-A: Writing–review and editing. SS: Writing–review and editing. EF: Writing–review and editing. MM: Visualization, Writing–review and editing.
Funding
The author(s) declare financial support was received for the research, authorship, and/or publication of this article. This publication was funded by the Internal Grant Award No. IG/ART/GEOG/23/01 from Sultan Qaboos University of Oman, entitled “Managing Ecosystem Services and Natural Resources for Water and Food Resilience in a Changing Climate”. High Impact Grant (HIG) award (211) from Qatar University.
Conflict of interest
Authors AB and MM were employed by Ecoresolve.
The remaining authors declare that the research was conducted in the absence of any commercial or financial relationships that could be construed as a potential conflict of interest.
Publisher’s note
All claims expressed in this article are solely those of the authors and do not necessarily represent those of their affiliated organizations, or those of the publisher, the editors and the reviewers. Any product that may be evaluated in this article, or claim that may be made by its manufacturer, is not guaranteed or endorsed by the publisher.
References
Abdullah, M., Feagin, R., and Musawi, L. (2017a). The use of spatial empirical models to estimate soil erosion in arid ecosystems. Environ. Monit. Assess. 189, 78–17. doi:10.1007/s10661-017-5784-y
Abdullah, M. M., Addae-Wireko, L., and Tena-Gonzalez, G. A. (2017b). Assessing native desert vegetation recovery in a war-affected area using multispectral and hyperspectral imagery: a case study of the Sabah Al-Ahmad Nature Reserve, Kuwait. Restor. Ecol. 25 (6), 982–993. doi:10.1111/rec.12527
Abdullah, M. M., Al-Ali, Z. M., Abdullah, M. M., Srinivasan, S., Assi, A. T., and Al Atiqi, S. (2021). Investigating the applicability of UAVs in characterizing desert shrub biomass and developing biological indicators for the selection of suitable revegetation sites. J. Environ. Manag. 288, 112416. doi:10.1016/j.jenvman.2021.112416
Ali, A., Xu, M.-S., Zhao, Y.-T., Zhang, Q.-Q., Zhou, L.-L., Yang, X.-D., et al. (2015). Allometric biomass equations for shrub and small tree species in subtropical China. Silva Fenn. 49 (4). doi:10.14214/sf.1275
Baena, S., Moat, J., Whaley, O., and Boyd, D. S. (2017). Identifying species from the air: UAVs and the very high resolution challenge for plant conservation. PloS one 12 (11), e0188714. doi:10.1371/journal.pone.0188714
Chen, X., Vierling, L., Rowell, E., and DeFelice, T. (2004). Using lidar and effective LAI data to evaluate IKONOS and Landsat 7 ETM+ vegetation cover estimates in a ponderosa pine forest. Remote Sens. Environ. 91 (1), 14–26. doi:10.1016/j.rse.2003.11.003
Conti, G., Enrico, L., Casanoves, F., and Díaz, S. (2013). Shrub biomass estimation in the semiarid Chaco forest: a contribution to the quantification of an underrated carbon stock. Ann. For. Sci. 70 (5), 515–524. doi:10.1007/s13595-013-0285-9
Cui, X., Gibbes, C., Southworth, J., and Waylen, P. (2013). Using remote sensing to quantify vegetation change and ecological resilience in a semi-arid system. Land 2 (2), 108–130. doi:10.3390/land2020108
Dash, J. P., Pearse, G. D., and Watt, M. S. (2018). UAV multispectral imagery can complement satellite data for monitoring forest health. Remote Sens. 10 (8), 1216. doi:10.3390/rs10081216
Gallacher, D. (2019). Drone-based vegetation assessment in arid ecosystems, 91–98. Sabkha Ecosystems: Volume VI: Asia/Pacific.
Laliberte, A. S., Fredrickson, E. L., and Rango, A. (2007). Combining decision trees with hierarchical object-oriented image analysis for mapping arid rangelands. Photogrammetric Eng. Remote Sens. 73 (2), 197–207. doi:10.14358/pers.73.2.197
Müllerová, J., Brůna, J., Bartaloš, T., Dvořák, P., Vítková, M., and Pyšek, P. (2017). Timing is important: unmanned aircraft vs. satellite imagery in plant invasion monitoring. Front. Plant Sci. 8, 887. doi:10.3389/fpls.2017.00887
Pla, M., Duane, A., and Brotons, L. (2017). Potencial de las imágenes UAV como datos de verdad terreno para la clasificación de la severidad de quema de imágenes Landsat: aproximaciones a un producto útil para la gestión post incendio. Rev. teledeteccion (49), 91–102. doi:10.4995/raet.2017.7140
Tahsin, S., Medeiros, S. C., Hooshyar, M., and Singh, A. (2017). Optical cloud pixel recovery via machine learning. Remote Sens. 9 (6), 527. doi:10.3390/rs9060527
Toutin, T. (2004). Review article: geometric processing of remote sensing images: models, algorithms and methods. Int. J. remote Sens. 25 (10), 1893–1924. doi:10.1080/0143116031000101611
Wang, X., Duan, Z., Brydegaard, M., Svanberg, S., and Zhao, G. (2018). Drone-based area scanning of vegetation fluorescence height profiles using a miniaturized hyperspectral lidar system. Appl. Phys. B 124, 1–5. doi:10.1007/s00340-018-7078-7
Yao, H., Qin, R., and Chen, X. (2019). Unmanned aerial vehicle for remote sensing applications—a review. Remote Sens. 11 (12), 1443. doi:10.3390/rs11121443
Zhang, X., Zhang, F., Qi, Y., Deng, L., Wang, X., and Yang, S. (2019). New research methods for vegetation information extraction based on visible light remote sensing images from an unmanned aerial vehicle (UAV). Int. J. Appl. Earth Observation Geoinformation 78, 215–226. doi:10.1016/j.jag.2019.01.001
Keywords: unmanned aerial vehicles, multispectral sensors, arid ecosystems, ecological monitoring and assessment, revegetation and restoration
Citation: Abdullah MM, Al-Ali ZM, Blanton A, Charabi Y, Abulibdeh A, Al-Awadhi T, Srinivasan S, Fadda E and Mohan M (2024) UAVs for improving seasonal vegetation assessment in arid environments. Front. Environ. Sci. 12:1366712. doi: 10.3389/fenvs.2024.1366712
Received: 09 January 2024; Accepted: 22 March 2024;
Published: 10 April 2024.
Edited by:
Alejandro Valdecantos, Mediterranean Center for Environmental Studies, SpainReviewed by:
Deirdre Dragovich, The University of Sydney, AustraliaCopyright © 2024 Abdullah, Al-Ali, Blanton, Charabi, Abulibdeh, Al-Awadhi, Srinivasan, Fadda and Mohan. This is an open-access article distributed under the terms of the Creative Commons Attribution License (CC BY). The use, distribution or reproduction in other forums is permitted, provided the original author(s) and the copyright owner(s) are credited and that the original publication in this journal is cited, in accordance with accepted academic practice. No use, distribution or reproduction is permitted which does not comply with these terms.
*Correspondence: Meshal M. Abdullah, m.abdullah1@squ.edu.om; Austin Blanton, austin.blanton@ecoresolve.eco; Midhun Mohan, mikey@ecoresolve.eco