Impact of population mobility on regional carbon emissions: empirical evidence from Australia
- Business School, University of Technology Sydney, Sydney, NSW, Australia
Understanding carbon emissions through the lens of population mobility is crucial for addressing sustainability challenges and fostering economic development. This study aims to investigate the influence of population mobility on carbon emissions in Australia, providing insights for targeted policy interventions. Utilizing panel datasets spanning from 2007 to 2020, encompassing both international and domestic migration in Australia, empirical analyses were conducted. The focus was on assessing the relationship between population mobility and regional carbon emissions. The study reveals distinct effects of domestic and international population mobility on carbon emissions. Domestic mobility demonstrates a negative correlation, whereas international mobility shows a positive association with carbon emissions. Moreover, significant regional heterogeneity in carbon emissions is observed, influenced by economic development and population size variations between eastern and western regions. These findings underscore the importance of tailored emission reduction strategies based on the nature of population mobility. By elucidating the intricate dynamics between mobility patterns and carbon emissions, this study contributes to a nuanced understanding of CO2 emission trends, informing policy decisions amidst the challenges posed by global climate change.
1 Introduction
In the face of the significant challenge posed by global climate change, the international community has undertaken ambitious agreements to mitigate carbon dioxide emissions, aiming to constrain the temperature rise to 2°C by the century’s end (Liang et al., 2020). The trajectory from the establishment of the United Nations Framework Convention on Climate Change (UNFCCC) to the enactment of the Kyoto Protocol and, subsequently, the ratification of the Paris Agreement underscores the collective determination of nations and regions to collaborate in addressing global climate change. Nonetheless, these agreements do not fully consider the shifts in population structure and distribution that could potentially alter the CO2 emission patterns within regions. With the ongoing globalization and regional economic integration, the frequency of interregional population mobility has been escalating. Interregional population mobility emerges as a pivotal demographic factor that could influence carbon emissions (de Sherbinin et al., 2008; Han and Deng, 2023), primarily due to its impact on the size and composition of populations both at the origin and destination. The repercussions of population mobility extend beyond mere demographic changes, as they entail shifts in energy consumption and lifestyle patterns, thereby affecting CO2 emissions (Feng and Hubacek, 2016).
However, scant attention has been paid to the ramifications of population mobility on carbon emissions within countries or regions. Population mobility, inherent to human civilization and globalization, has surged in recent decades, with increasingly diverse destinations. The multifaceted impacts of population mobility on host countries encompass political, economic, cultural, and welfare dimensions (Duncan and Waldorf, 2016; Young et al., 2018). Thus, population mobility assumes significance as a crucial component among demographic factors influencing carbon emissions. Nevertheless, extant research pertaining to the influence of demographic factors on carbon emissions predominantly centers on population size and structure (Dietz and Rosa, 1997; Lozano and Gutiérrez, 2008; Wei and Liu, 2022). Against the backdrop of global economic integration and accelerated population mobility, a more precise and comprehensive exploration of the nexus between population mobility and carbon emissions becomes imperative for the realization of national or regional emission reduction objectives. Consequently, the pivotal question arises: What is the impact of population mobility on carbon emissions within each country or region? This study endeavors to bridge this knowledge gap by empirically analyzing the influence of population mobility on carbon dioxide emissions in Australia, thereby addressing this query.
The investigation into factors influencing carbon emissions constitutes a pivotal facet in advancing the green and sustainable growth of the global economy and is an indispensable prerequisite for achieving global greenhouse gas emission reduction targets. Consequently, scholarly attention has long been devoted to unraveling the determinants of regional carbon emissions. In recent years, such endeavors have yielded two systematic strands of research.
Firstly, studies have delved into the impact of non-demographic factors on carbon emissions. On one hand, a burgeoning body of literature has scrutinized the effects of environmental policies and regulations on carbon emissions. Notably, at the provincial level, the carbon emissions trading pilot policy has yielded discernible results, substantially curtailing carbon emissions in pilot areas (Zhang et al., 2021). Likewise, at the municipal level, Zhang (2020) assessed the carbon emission reduction effects of pilot low-carbon city policies and pilot innovative city policies, underscoring the efficacy of environmental policies and regulations in abating urban carbon emissions. On the other hand, a plethora of studies have examined the nexus between economic growth (GDP) (Pao and Tsai, 2011; Wang et al., 2018), Foreign Direct Investment (FDI) (Zhu et al., 2016; Zhang and Zhang, 2018), International Trade (IT) (Khan et al., 2020), Urbanization (Cole and Neumayer, 2004; Fan et al., 2006; Liddle and Lung, 2010; Poumanyvong and Kaneko, 2010), per capita income (GDP per capita) (Martínez-Zarzoso and Maruotti, 2011), fixed asset investment (FCI) (Jin and Han, 2021), technological innovation (R&D) (Zhao et al., 2014; Jiao et al., 2018a; Jiao et al., 2018b), and carbon emissions. Additionally, some scholars have explored the impacts of factors such as trade openness (Shahbaz et al., 2017), industrial structure upgrading (Wu et al., 2021), and regional integration (Li and Lin, 2017) on carbon emissions and carbon emission intensity. In the context of carbon peaking and carbon neutrality, it is imperative to ascertain how non-demographic factors influence carbon emissions and whether they contribute to carbon emission mitigation. Diverging from prior literature, this paper empirically scrutinizes the direction and magnitude of the impact of non-population factors on carbon emissions and conceptualizes the mechanism underpinning the role of technological innovation, thereby elucidating the contribution of non-population factors to carbon emission reduction.
Secondly, research has focused on the influence of population factors on carbon emissions. In recent years, propelled by the relentless expansion of the global population, both direct and indirect energy consumption by the populace has surged, emerging as a pivotal driver of carbon emissions, surpassing even the industrial sector in several developed countries (Lu and Zhao, 2008). Consequently, the examination of the impact of demographic factors on carbon emissions has garnered considerable attention in academic circles. Presently, studies scrutinizing the impact of population factors on carbon emissions predominantly center on the influence of population size. Numerous studies have underscored that population growth constitutes the primary driver of GHG emissions in the past and projected future (Schelling, 1992; Chesnais et al., 2001). Nonetheless, the influence of demographic factors on GHG emissions transcends population size alone, with population mobility intricately linked to carbon emissions, potentially impacting climate change dynamics. While population size serves as a static indicator of regional demographic factors, regional population mobility remains dynamic and fails to fully capture population fluctuations. Typically, regions with higher levels of economic development experience greater inflows of migrants, whereas less developed regions witness outflows. Given the perpetual flux in regional population sizes, exploring the impact of regional population mobility on carbon emissions offers a more rational, precise, and comprehensive assessment of the influence of demographic factors on carbon emissions. Despite the prevailing focus on the impact of population size on carbon emissions within demographic literature, no literature specifically examines the systematic impact of population mobility on carbon emissions, particularly concerning international and domestic population mobility. This lacuna underscores the urgent need to devise effective strategies leveraging the incentivizing effect of population mobility on carbon emission reduction.
Academic investigations have delved into the research on factors influencing carbon emissions from various perspectives. Upon scrutinizing existing literature, we identify limitations in three aspects. Firstly, while prior studies have examined the mechanisms by which technological innovation and industrial structural upgrades affect carbon emissions, there is a paucity of literature elucidating the theoretical mechanisms underlying the impact of population mobility on carbon emissions. Secondly, although certain literature has initiated discussions on the influence of population factors on carbon emissions, it predominantly focuses on population size as a static indicator, overlooking a direct empirical examination of the relationship between population mobility and carbon emissions. Despite Chesnais et al. (2001) examination of the relationship between demographic factors and carbon emissions, their study is unfortunate in its exclusion of dynamic indicators of population mobility and carbon emissions within the same dimension for empirical analysis. Thirdly, existing literature lacks an exploration of the regional heterogeneity in the impact of population mobility on carbon emissions.
In contrast to existing studies, this paper scrutinizes the impact of population mobility on carbon emissions through theoretical elucidations and empirical analyses based on state-level data. By integrating domestic population mobility, international population mobility, and carbon emissions into the same analytical framework, we address the aforementioned gaps in the literature. Leveraging datasets encompassing carbon emissions, international and domestic population mobility, total population, urbanization rate, GDP, innovation index, and fixed asset investment across Australian states from 2007 to 2020, we use cointegration theory, heterogeneity analysis, endogeneity examination, and multiple regression modeling as analytical tools to investigate the relationship between population mobility and carbon emissions. Specifically, utilizing data provided by the Australian Bureau of Statistics (ABS), we use multiple regression models to explore the impacts of international and domestic population mobility on carbon emissions across the eight states of Australia. Through the introduction of an econometric model based on panel data, we unveil the relationship between population mobility and regional carbon emissions, thereby facilitating an understanding of the nexus between population dynamics changes and carbon emissions.
In summary, this paper not only elucidates the mechanisms through which population mobility affects carbon emissions theoretically but also assesses the intensity and direction of population mobility’s impact on carbon emissions. Furthermore, it examines the regional heterogeneity in carbon emissions attributable to population mobility. Thus, this paper furnishes empirical and theoretical evidence to aid Australia in formulating a rational population mobility policy to attain future carbon emission reduction targets. Additionally, it offers new research perspectives and policy focal points for relevant stakeholders in achieving global carbon emission reduction objectives.
Subsequently, the paper is structured as follows: Part II presents the relevant theoretical mechanisms and hypotheses. Part III delineates the detailed research methodology, data sources, etc. The fourth section presents the corresponding analysis and a detailed discussion of the primary findings. Finally, the concluding part summarizes the paper and presents corresponding policy recommendations.
2 Theoretical mechanisms and research hypotheses
In this paper, by reviewing existing relevant studies, we argue that the mechanisms underlying the impact of population mobility on carbon emissions can be delineated from two perspectives: the scale effect and the innovation effect.
Firstly, the scale effects are notable. Changes in population size resulting from population mobility primarily influence carbon emissions through international and domestic population movements. Initially, the impact of international population mobility on carbon emissions manifests in alterations in consumption patterns. Typically, international population mobility leads to an expansion of the population size in the destination country, thereby fostering increased local consumption demand. This surge in demand encompasses heightened requirements for natural resources (Pan et al., 2007; Oldekop et al., 2018), housing and land (Taylor et al., 2016), and food, thereby elevating carbon emissions (Radel et al., 2010). Secondly, domestic population movements often precipitate urbanization and urban sprawl. The economies of scale and intensified development associated with urbanization enhance energy utilization efficiency (Lv et al., 2020). For instance, larger structures, factories, and facilities can optimize energy usage and minimize wastage, consequently reducing carbon emissions. Furthermore, urbanization typically coincides with denser urban construction and improved transport networks, mitigating road traffic congestion and subsequent carbon emissions (Sun and Huang, 2020). Building upon these analyses, we propose the first research hypothesis of this paper.
Hypothesis 1:. Population mobility affects regional carbon emissions, wherein international population mobility increases carbon emissions within the region, while domestic population mobility decreases carbon emissions within the region.
Secondly, the innovation effect is noteworthy. Many studies overlook the influx of high-quality and skilled human resources facilitated by population mobility. Yet, the arrival of such talents invariably accelerates technological innovation within the region. Technological innovation plays a pivotal role in mitigating carbon emissions (Costa-Campi et al., 2015). Primarily, technological innovation influences carbon emissions by fostering the optimization and upgrading of industrial structures (You and Zhang, 2022). Widely employed in production and daily life, technological innovation facilitates cleaner production processes, enhances energy efficiency, promotes green energy consumption, and reduces resource consumption from both production and consumption perspectives. Moreover, it empowers the optimization and transformation of industrial structures toward low-carbon and eco-friendly industries, thereby curbing carbon emissions at their source. Secondly, technological innovation can also spur carbon emission reduction by reshaping the energy landscape (Shan et al., 2021). The application of technological innovation in the energy sector expedites the development of photovoltaic, wind power, and renewable energy sources, fostering the transition to a green, low-carbon, and clean energy consumption structure, thereby directly mitigating carbon dioxide emissions. However, it is imperative to note the predominant flow of innovative talent. High-end talents typically gravitate toward developed regions (Oliinyk et al., 2021) owing to the plethora of career opportunities, access to superior educational resources, convenient living conditions, vibrant entrepreneurial and innovative ecosystems, and extensive social networks available in such locales. The disparities arising from such population mobility may exacerbate regional discrepancies, thereby engendering heterogeneous impacts on carbon emissions. Building upon these insights, we propose the second research hypothesis of this paper.
Hypothesis 2:. There exists regional heterogeneity in the impact of population mobility on regional carbon emissions, wherein the effect of population mobility on regional carbon emissions varies across regions.
3 Models and data
3.1 The econometric model and variables
This paper proposes the adoption of an econometric model based on panel data to investigate the impact of population mobility on carbon emissions in Australia. It is evident from prior empirical research that employing such an econometric analytical model significantly enhances the reliability of estimation results (Azomahou et al., 2006; Baltagi, 2021). Moreover, this model endeavors to incorporate various control variables to mitigate potential inaccuracies arising from the omission of relevant influencing factors (Halicioglu, 2009; Al-mulali & Sheau-Ting, 2014). Furthermore, recognizing the issue of multicollinearity between domestic and international population mobility within the same econometric framework (Zhong et al., 2021), both variables are included in the empirical analyses within the econometric model. Hence, this paper formulates the following econometric model:
where
The influence of population mobility on carbon emissions in Australia is delineated by two variables: international and domestic population mobility. While both domestic and international population mobility can impact changes in population size, consumption structure, and scale across different states of Australia, consequently affecting changes in carbon emissions, international population mobility is more inclined to stimulate technological advancements in sustainable energy innovations and alter consumption structures at migration destinations compared to domestic population mobility. Given Australia’s status as a nation of immigrants, there has been a surge in international inflows over the past two decades, perpetuating the influence of the international population on population growth in each of Australia’s states. Conversely, domestic population mobility influence population growth in receiving states while simultaneously contributing to population decline in the states experiencing out-migration. Therefore, we use both international and domestic population movements to characterize the impact of population mobility on carbon emissions in Australia.
To enhance the reliability of the study’s findings, this paper selects control variables (Z) that accurately reflect the status of each region and may impact regional carbon emissions. Specifically, these include 1) urbanization rate (urban). Urbanization rate serves as a crucial indicator of regional development level, and numerous studies have illustrated a positive correlation between urbanization and carbon emissions, both globally and across countries or regions with varying income levels (Cole and Neumayer, 2004; Fan et al., 2006). However, the drivers behind increased energy consumption and carbon emissions due to population urbanization vary among countries or regions with differing income levels. Notably, population migration from rural to urban areas influences regional carbon emissions by altering economic production and consumption patterns (Zhao et al., 2012; Alola, 2019; Qi and Li, 2020). In this paper, we utilize the urban population as a percentage of the total population in the region to characterize the urbanization rate.
2) The size of the population (
3) The level of regional economic development (
4) Investment in fixed assets (
5) Environmental regulation (
6) Technological innovation (
3.2 Data
For the assessment of population mobility and regional carbon emissions, data on international and domestic population mobility, total regional population, urbanization rate, gross state product (GSP), and fixed asset investment are primarily obtained from the Australian Bureau of Statistics (ABS). The ABS provides the most comprehensive statistical data on the Australian economy, population, industry, labor, health, and environment for the period 2007–2020. Moreover, for the pertinent control variables in the econometric regressions—namely, urbanization rate, total state population, GSP, GSP per capita, innovation index, and fixed asset investment—these data are also sourced from the Australian Bureau of Statistics. State-level CO2 and SO2 emissions data are retrieved from the Department of Industry, Science, Energy, and Resources (DISER) database. Additionally, to eliminate the influence of price factors on economic data, all economic figures in this study are denoted in Australian dollars. Descriptive statistics for these variables are presented in Table 1 within the Table directory.
4 Results and discussions
The first step in the econometric analysis was to test the variables’ stationarity. This was achieved by applying the Panel unit root test. The panel unit root test became popular because of its high power. This study utilized the panel unit root tests namely, Levin, Lin and Chu (LLC) (Levin et al., 2002). The results revealed that some of the variables are non-stationary at the level thus the null hypothesis of a panel unit root cannot be rejected. On the other hand, the variables are significant at first difference which rejects the null hypothesis. Therefore, the variables are stationary at the first difference. The stationarity of the variables for each state was also tested by using the Augmented Dickey-Fuller (ADF) and Phillips–Perron (PP) tests and it was found that all the variables for each state were stationary but at different levels.”
4.1 Basic regression results
Using tests such as the F-value (p = 0.00) and LSDV, this paper finds that the above constructed econometric regression model [i.e., Eqs 2, 3)] has individual effects and thus determines that confluent regression should not be used. Further, the Hausman test and the over-identification test were also used to find that the model rejects random effects and that a fixed effects model should be used. On this basis, the results of the baseline econometric regression in this paper are as follows.
The regression results for the effects of international migration, domestic migration, and population size on carbon emissions in Australia are presented in (1)–(3) in Table 2 of Table directory. It can be seen that the estimated coefficients for international migration in Australia are all positive, while the estimated coefficients for domestic migration are all negative, conditional on the inclusion of control variables. There is a positive correlation between international migration and carbon emissions, with an increasing number of international population mobility leading to greater carbon emissions. This phenomenon may be explained by the fact that, on the one hand, since international migration is usually from relatively poorer to richer regions, international migrants usually live in more developed economies with significant changes in lifestyle (Zhao et al., 2012; Qi and Li, 2020). Their increased consumption of necessities (e.g., food and clothing), housing, infrastructure, healthcare, and education leads to higher CO2 emissions (Liebert and Ameringer, 2013; Teixeira, 2013; Larrotta, 2017).
On the other hand, population mobility has an impact on carbon emissions, mainly because it affects the size and structure of the population at the source and destination. Not only does population growth due to mobility translate into higher energy consumption, but the process of population mobility brings about changes in lifestyles, which affect consumption patterns and thus CO2 emissions (Feng and Hubacek, 2016). There is a negative correlation between domestic population mobility and carbon emissions, i.e., domestic population mobility leads to reduced carbon emissions. The reason for this phenomenon may be caused by the urbanisation process (Ma and Hofmann, 2019). Domestic population mobility tends to promote the transfer of labour from the agricultural sector to the non-agricultural sector, and the structure of urban employment and industry changes, triggering an industrial structure transformation effect. This means that after a certain degree of economic development, the proportion of primary and secondary industries in the economy will decrease accordingly, and the tertiary industry represented by high value-added services will become the centre of economic development (Tian et al., 2014). According to Wu et al. (2012), the decrease in the proportion of the secondary industry will lead to a decrease in per capita carbon emissions. Meanwhile, Guo (2012) found that there is a negative correlation between the proportion of primary industry and carbon dioxide emissions when studying the impact of industrial structure on carbon emissions.
As expected, urbanisation has a positive impact on carbon emissions. Specifically, an increase in the size of the urban population leads to a rise in carbon emissions, which is related to factors such as energy demand and traffic congestion that may increase during urbanisation. However, population size does not statistically significantly affect carbon emissions, possibly because the effect of population size on carbon emissions may vary by region. In some regions, an increase in population size may be associated with higher carbon emissions, while in other regions it may not be significant. We will verify this in the subsequent heterogeneity analysis. State GDP positively affects carbon emissions, with increased state GDP likely associated with higher levels of industrial production and energy consumption. Meanwhile, state GDP per capita also has a positive effect on carbon emissions, which may imply a non-linear relationship between the effect of state GDP per capita on carbon emissions, i.e., as productivity increases, carbon emissions rise at an accelerated rate. On the other hand, investment in fixed assets has a negative effect on carbon emissions, suggesting that increased investment in fixed assets may help to reduce carbon emissions, possibly due to technological innovations and more efficient resource utilisation. However, sulphur dioxide emissions do not statistically significantly affect carbon emissions, which may indicate that sulphur dioxide is not influential enough to statistically significantly affect carbon emissions in this model. Finally, the lack of significant impact of R&D expenditures on carbon emissions in the current model may be due to the fact that there may be a time lag in the impact of R&D expenditures, i.e., the impact of R&D expenditures on carbon emissions that occurs after a certain period of time. This lagged effect may not be captured if the time horizon is short. It may also be because there may be differences in the industrial structure and R&D priorities of different regions, leading to a significant impact of R&D expenditure on carbon emissions in some regions but not in others. We will also verify this in the subsequent heterogeneity analysis.
4.2 Endogenous treatment
Where endogeneity is overlooked, estimates may suffer from bias and inconsistency (Klette and Griliches, 1996). Endogeneity, in the context of this paper, primarily manifests as reverse causality, omitted variables, and measurement error (Roberts and Whited, 2013). Firstly, population mobility may exert both growth and inhibitory effects on regional carbon emissions. Conversely, regional carbon emissions may also exert a considerable impact on population mobility. Regions with high energy consumption and carbon emissions may be less susceptible to the influence of minor population mobility factors. For instance, a significant influx of population may lead to an increase in carbon emissions in recipient areas to a certain extent, while regions with high carbon emissions may experience population outflows due to deteriorating living conditions and reduced quality of life. Secondly, due to the impossibility of exhaustively listing all explanatory variables in the econometric model, omitted variables may arise. The inclusion of omitted variables in the error term, especially when they are correlated with other explanatory variables, can lead to endogeneity issues. For instance, the quality of incoming population can influence changes in carbon emissions. This quality is often linked to various factors such as the political, cultural, economic, and educational levels of the originating country, making it challenging to incorporate all relevant variables and potentially resulting in variable omissions that could impact regression outcomes. Finally, measurement errors in variables may also contribute to endogeneity problems.
To address potential endogeneity issues in econometric models, this paper employs the following strategies. Firstly, to mitigate the impact of endogeneity, a lagged period instrumental variable strategy is adopted, utilizing lagged CO2 emissions as instrumental variables for the current period’s values. This approach effectively addresses the aforementioned issues. Additionally, considering the inherent inertia of economic factor changes, a dynamic model lag term is introduced to better control for lagging factors. Furthermore, to minimize estimation bias due to the choice of econometric model, this paper introduces the systematic Generalized Method of Moments (GMM) approach. The systematic GMM approach offers several advantages: it eliminates bias in the presence of non-time-varying omitted variables, provides consistent estimates of coefficients in the presence of endogenous variables on the right-hand side of the model, and yields consistent estimates even in the presence of measurement error (Bond et al., 2001). Thus, estimation is conducted using systematic GMM in dynamic panels, effectively addressing measurement error, non-time-varying omitted variables, and endogeneity of explanatory variables (Caselli et al., 1996).
Table 3 in the Appendix presents the results of econometric regressions accounting for endogeneity. The regression outcomes concerning the effects of international population mobility, domestic population mobility, and population size on carbon emissions in Australia, when endogeneity is taken into account, indicate positive estimated coefficients for international population mobility and negative estimated coefficients for domestic population mobility, with control variables included. These findings suggest that both the positive impact of international population mobility on regional carbon emissions and the negative impact of domestic population mobility on inter-regional carbon emissions remain robust, unaffected by potential endogeneity risks.
Moreover, significant positive effects of international population mobility, and significant negative effects of domestic population mobility are observed in both types of econometric regressions (Tables 2; 3 in the Appendix), reinforcing the reliability of estimated results for core explanatory variables presented earlier.
4.3 Heterogeneity analysis
With the advancement of economic globalization and the evolution of human civilization, the phenomenon of population mobility, driven by meritocratic principles, has influenced the allocation of global resources across various regions and countries. This dynamic has resulted in the migration of people to different destinations, ultimately shaping patterns of population movement that are reflective of varying levels of economic development. Numerous studies have demonstrated that regions or countries with more developed economies often offer attractive employment prospects and living standards, thereby drawing highly skilled individuals, while those with lower to middle-income status struggle to attract such talent. This raises the question: does population mobility exert a varying impact on carbon emissions in regions or countries with differing levels of development? To investigate this, the following section presents an empirical analysis.
The findings are detailed in Tables 4, 5 in the Appendix. Notably, the effect of domestic migration on shifts in regional carbon emissions is consistently negative across both Eastern and Western regions. This suggests that the role of domestic population mobility in reducing carbon emissions remains robust across diverse geographical areas. As human civilization progresses and economic globalization continues to unfold, regional shifts in carbon emissions will undoubtedly influence the distribution of responsibility for emission reduction among different regions. Therefore, it is imperative to encourage the involvement of regions with varying degrees of population mobility in energy conservation and emission reduction efforts. This regression outcome substantiates Hypothesis 2 of this paper, which posits regional heterogeneity in the impact of population mobility on regional carbon emissions. Furthermore, most control variables exhibit directional and significant consistency with previous findings, further bolstering the reliability of prior empirical results.
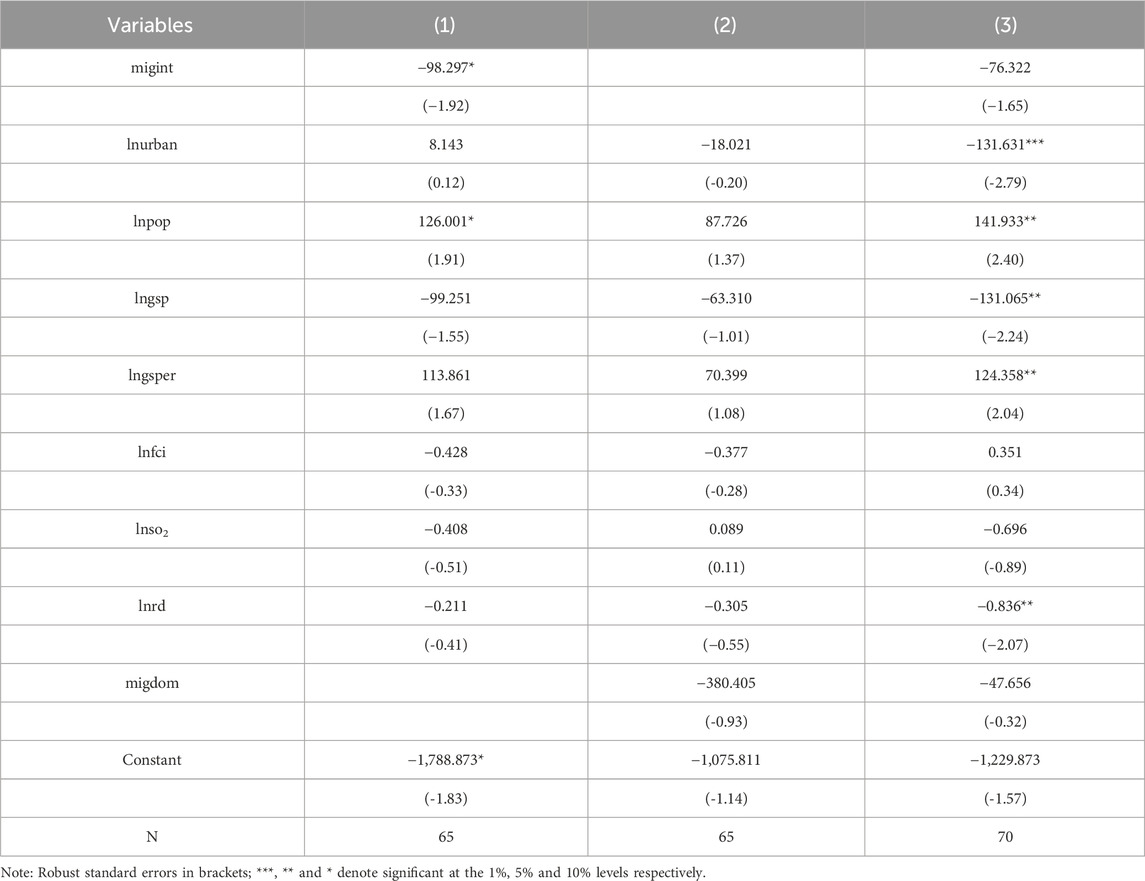
Table 4. Heterogeneity test for the effect of population mobility on carbon emissions in the eastern region.
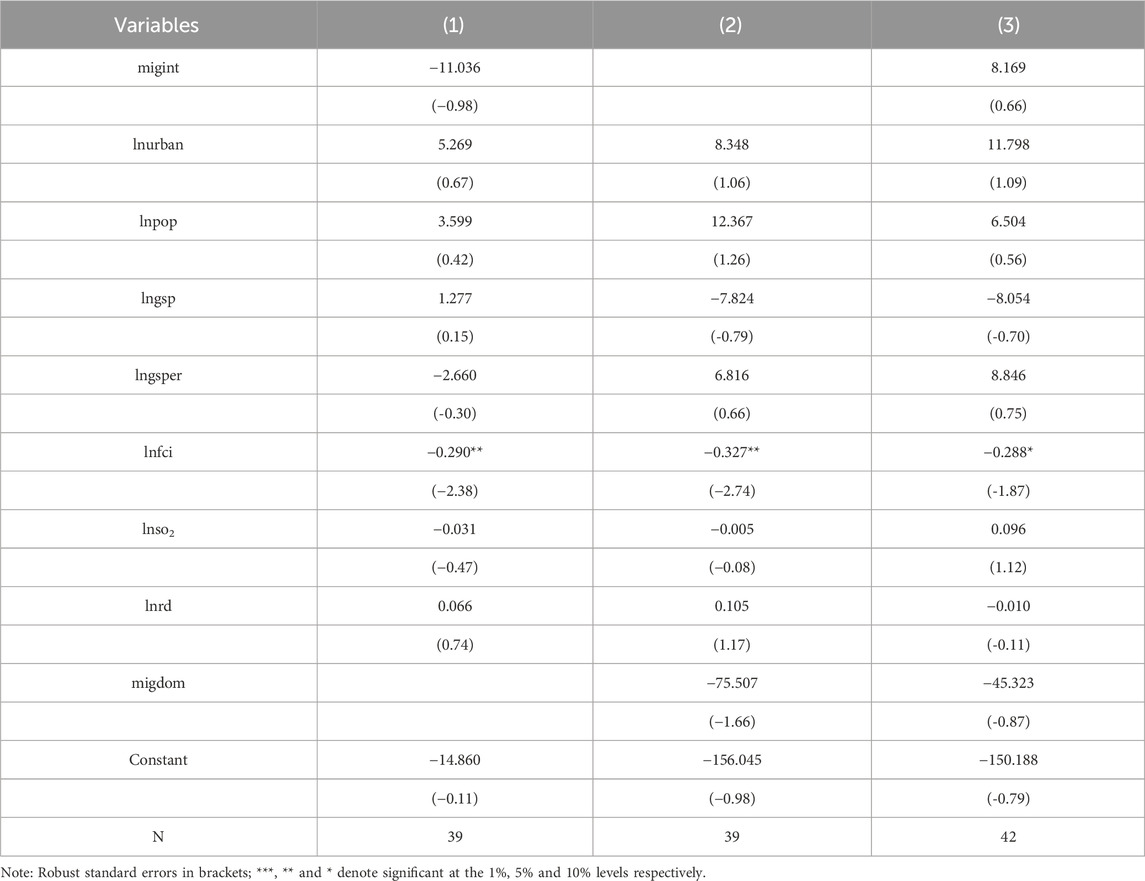
Table 5. Heterogeneity test for the effect of population mobility on carbon emissions in the western region.
Drawing insights from the estimated results of control variables in both developed Eastern and less developed Western regions, several conclusions can be derived. Firstly, the positive impact of population size is highly significant in both regions. Secondly, the estimated coefficient for the level of economic development in the Eastern region surpasses that of the Western region. Thirdly, notable disparities are observed in the effects of international population mobility on regional carbon emissions. In the developed Eastern region, international population mobility significantly inhibits carbon emissions, whereas in the Western region, international population mobility exhibits distinct regional variations in its impact on carbon emissions. Fourthly, the positive effect of per capita income is more pronounced. Lastly, the impact of GSP per capita is negative for the Western region but positive for the Eastern region.
4.4 Discussions
Firstly, regarding domestic population mobility, the empirical findings of this study reveal a significantly negative regression coefficient for domestic population mobility at the 10 percent level. This finding aligns with Hypothesis 1 outlined in the Theoretical Mechanisms section, which posits that domestic population mobility decreases carbon emissions within the region. Domestic population mobility primarily influences carbon emissions through structural shifts. It often facilitates the transition of labor from the agricultural sector to the non-agricultural sector, prompting changes in employment and industrial structures within urban areas, thus catalyzing industrial transformation. The relocation of labor to urban centers fosters the growth of secondary and tertiary sectors and incentivizes the transition from traditional agriculture to modern methods, consequently reducing the proportion of the primary sector. Guo (2012) observed that a 1 percent reduction in the share of energy-intensive industries within the primary sector could potentially decrease carbon dioxide emissions by 220–290 million tons, which corroborates our regression findings. As a developed nation, Australia’s economy has advanced to a certain degree, leading to a decline in the proportion of primary and secondary industries, with the tertiary sector, epitomized by high value-added services, assuming centrality in economic development. Wu et al. (2012) demonstrated that higher carbon emissions correlate with a larger share of the secondary industry, yet a decrease in the secondary sector’s proportion, under constant per capita GDP, results in reduced per capita carbon emissions. This suggests that the transition of Australia’s domestic workforce from the secondary to the tertiary sector is conducive to carbon emission reduction, consistent with our econometric regression results.
Secondly, concerning international population mobility, the regression outcomes indicate a positive elasticity of the impact of international population mobility on carbon emissions. These findings validate the hypothesis proposed in Hypothesis 1, suggesting that international population movements increase carbon emissions within the region. International mobility influences carbon emissions primarily through the scale effect and technological innovation facilitated by the mobility of skilled individuals. The scale effect induced by international mobility significantly influences population growth, with population size exhibiting a notable positive effect on carbon emissions growth, consistent with both our empirical findings and those of prior researchers.
Moreover, it is noteworthy that the impact of population growth on carbon emissions manifests in two primary ways: firstly, population growth directly precipitates a sharp rise in energy consumption and carbon emissions, and secondly, population growth fosters low-carbon technological innovation, thereby mitigating carbon emissions. The positive elasticity coefficient of the impact of international population mobility on carbon emissions suggests that the promotional effect of international population mobility on low-carbon technological progress and research and development (R&D) is not substantial enough to offset the scale effect’s influence on carbon emissions growth. Consequently, the positive elasticity coefficient of the impact of international population mobility on carbon emissions persists.
5 Conclusion and policy implications
This paper aimed to offer a more comprehensive understanding of the determinants of CO2 emission changes from the perspective of population mobility and empirically examine the impacts of population mobility on CO2 emissions. Building on this foundation, we derived relevant policy conclusions concerning population mobility and carbon emissions. Our primary conclusions and policy implications are outlined below.
Firstly, in the baseline regression analysis, domestic and international population mobility exert different impacts on carbon emissions. A negative correlation typically exists between domestic population mobility and carbon emissions. This phenomenon arises because domestic population mobility often accompanies urbanization, leading to more efficient energy usage and reduced carbon emissions. For instance, urban migration tends to increase population density, thereby enhancing transport efficiency and promoting public transport utilization, consequently mitigating carbon emissions from transportation. Generally, as domestic population mobility rises, carbon emissions tend to decline. Conversely, there exists a positive correlation between international population mobility and carbon emissions. International migration can alter consumption patterns, thereby influencing carbon emissions. For example, an influx of international migrants may drive increased consumption of material goods, consequently raising carbon emissions from logistics. Furthermore, international migration may escalate logistics transportation, further exacerbating carbon emissions. Overall, as international migration increases, carbon emissions tend to rise.
Secondly, concerning heterogeneity, significant disparities in carbon emissions exist between Australia’s eastern and western regions. The eastern region, characterized by a more developed economy, exhibits greater dependence on industrial and urban development, resulting in higher carbon emissions. For instance, major cities like Sydney and Brisbane, being economic hubs, also register high carbon emissions owing to industrial and urban development. Conversely, the western region boasts a less developed economy reliant on agriculture and resource development, thus recording lower carbon emissions. For example, Western Australia’s economy is predominantly based on agriculture and mining, resulting in comparatively lower emissions.
The empirical findings of this paper suggest that with global economic integration and the advancement of human civilization, population mobility can contribute to carbon emissions reduction in Australia.
Firstly, in terms of scale effects, population mobility alters Australia’s population size and composition, consequently affecting consumption patterns and environmental dynamics, thereby influencing changes in carbon emissions. Given Australia’s status as a major migrant destination, this trend in population mobility is likely to persist. Policymakers can implement various measures on both the supply and demand sides to mitigate carbon dioxide emissions associated with population mobility. Destination countries can optimize consumption behaviors and provide incentives for technological advancements, such as imposing carbon taxes on goods and services. Additionally, measures like taxes, support, and subsidies can be employed to reduce the energy intensity of productive sectors, thus offsetting migration-induced greenhouse gas emissions.
Secondly, with regard to the influx of innovative talent, as previously discussed, the migration of highly skilled individuals resulting from population mobility is poised to accelerate technological innovation in Australia, thereby playing a pivotal role in addressing climate change challenges and fostering sustainable development. Consequently, Australian authorities should prioritize technology development, innovation, and research and development by strategically increasing the target for skilled migration in clean energy within migration policy frameworks. However, it is essential to acknowledge the heterogeneous impact of acquiring innovative talent on regional carbon emissions.
While this paper enriches the literature on the relationship between population mobility and the environment, it is not without limitations. Future research could address these limitations by examining the relationship between population mobility and the environment in other countries and regions outside Australia, employing different econometric and statistical methodologies. Furthermore, future studies may utilize alternative indicators such as greenhouse gases, air quality indices, ecological footprints, and deforestation rates to gauge environmental quality. Such endeavors will deepen our understanding of the relationship between population mobility and the environment, guiding the formulation of sustainable development policies.
Data availability statement
The original contributions presented in the study are included in the article/supplementary material, further inquiries can be directed to the corresponding author.
Author contributions
HG: Conceptualization, Data curation, Formal Analysis, Investigation, Methodology, Project administration, Resources, Software, Validation, Visualization, Writing–original draft, Writing–review and editing.
Funding
The author(s) declare that no financial support was received for the research, authorship, and/or publication of this article.
Conflict of interest
The author declares that the research was conducted in the absence of any commercial or financial relationships that could be construed as a potential conflict of interest.
Publisher’s note
All claims expressed in this article are solely those of the authors and do not necessarily represent those of their affiliated organizations, or those of the publisher, the editors and the reviewers. Any product that may be evaluated in this article, or claim that may be made by its manufacturer, is not guaranteed or endorsed by the publisher.
Footnotes
1Net CO2 emissions are the amount of carbon dioxide after all emissions released by human activities have been offset by removing carbon from the atmosphere. Net CO2 emissions consist of two main components. Firstly, human-caused CO2 emissions (e.g., from fossil-fuelled cars and factories). Second, carbon removal, which is usually done through natural methods such as absorption by forests, land, and oceans, or through technologies such as direct air capture and storage (DACS), which removes carbon directly from the atmosphere.
2Population mobility in this paper refers to the net inflow of population as a proportion of the total local population, i.e., population inflow minus population outflow divided by the total local population.
References
Al-mulali, U., and Sheau-Ting, L. (2014). Econometric analysis of trade, exports, imports, energy consumption and CO2 emission in six regions. Renew. Sustain. Energy Rev. 33, 484–498. doi:10.1016/j.rser.2014.02.010
Alola, A. A. (2019). Carbon emissions and the trilemma of trade policy, migration policy and health care in the US. Carbon Manag. 10 (2), 209–218. doi:10.1080/17583004.2019.1577180
Aslam, B., Hu, J., Shahab, S., Ahmad, A., Saleem, M., Shah, S. S. A., et al. (2021). The nexus of industrialization, GDP per capita and CO2 emission in China. Environ. Technol. Innovation 23, 101674. doi:10.1016/j.eti.2021.101674
Azomahou, T., Laisney, F., and Nguyen Van, P. (2006). Economic development and CO2 emissions: a nonparametric panel approach. J. Public Econ. 90 (6-7), 1347–1363. doi:10.1016/j.jpubeco.2005.09.005
Baltagi, B. H. (2021). “Econometric analysis of panel data,” in Springer texts in business and economics (Springer International Publishing). doi:10.1007/978-3-030-53953-5
Birdsall, N. (1992). “Another look at population and global warming,” in Policy Research working papers WPS 1020 (Washington, D.C.: World Bank Group). http://documents.worldbank.org/curated/en/985961468766195689/Another-look-at-population-and-global-warming.
Bond, S. R., Hoeffler, A., and Temple, J. R. W. (2001). GMM estimation of empirical growth models (november 2001). Economics papers 2001-W21, economics group. Available at: https://ssrn.com/abstract=290522.
Caselli, F., Esquivel, G., and Lefort, F. (1996). Reopening the convergence debate: a new look at cross-country growth empirics. J. Econ. Growth 1 (3), 363–389. doi:10.1007/bf00141044
Chen, W., and Lei, Y. (2018). The impacts of renewable energy and technological innovation on environment-energy-growth nexus: new evidence from a panel quantile regression. Renew. Energy 123, 1–14. doi:10.1016/j.renene.2018.02.026
Chesnais, J.-C., Birdsall, N., Kelley, A. C., and Sinding, S. W. (2001). Population matters. Demographic change, economic growth and poverty in the developing world. Popul. Fr. Ed. 56 (6), 1075. doi:10.2307/1534753
Cole, M. A., and Neumayer, E. (2004). Examining the impact of demographic factors on air pollution. Popul. Environ. 26 (1), 5–21. doi:10.1023/b:poen.0000039950.85422.eb
Costa-Campi, M. T., García-Quevedo, J., and Trujillo-Baute, E. (2015). Challenges for R&D and innovation in energy. Energy Policy 83, 193–196. doi:10.1016/j.enpol.2015.04.012
deHaan, E. (2020). Practical guidance on using and interpreting fixed effects models. SSRN Electron. J. 2020, 3699777. doi:10.2139/ssrn.3699777
de Sherbinin, A., VanWey, L. K., McSweeney, K., Aggarwal, R., Barbieri, A., Henry, S., et al. (2008). Rural household demographics, livelihoods and the environment. Glob. Environ. Change 18 (1), 38–53. doi:10.1016/j.gloenvcha.2007.05.005
Dietz, T., and Rosa, E. A. (1997). Effects of population and affluence on CO2 emissions. Proc. Natl. Acad. Sci. 94 (1), 175–179. doi:10.1073/pnas.94.1.175
Dobbie, B., and Green, D. (2015). Australians are not equally protected from industrial air pollution. Environ. Res. Lett. 10 (5), 055001. doi:10.1088/1748-9326/10/5/055001
Duncan, N. T., and Waldorf, B. S. (2016). Immigrant selectivity, immigrant performance and the macro-economic context. Regional Sci. Policy and Pract. 8 (3), 127–143. doi:10.1111/rsp3.12078
Fan, Y., Liu, L.-C., Wu, G., and Wei, Y.-M. (2006). Analyzing impact factors of CO2 emissions using the STIRPAT model. Environ. Impact Assess. Rev. 26 (4), 377–395. doi:10.1016/j.eiar.2005.11.007
Feng, K., and Hubacek, K. (2016). Carbon implications of China’s urbanization. Energy, Ecol. Environ. 1 (1), 39–44. doi:10.1007/s40974-016-0015-x
Guo, C. (2012). Effect of industrial structure change on carbon emission in China. China Popul. Resour. Environ. 2012 (7), 15–20.
Halicioglu, F. (2009). An econometric study of CO2 emissions, energy consumption, income and foreign trade in Turkey. Energy Policy 37 (3), 1156–1164. doi:10.1016/j.enpol.2008.11.012
Han, Z., and Deng, X. (2023). Impacts of cross-regional population migration and agglomeration on carbon emissions in China. Appl. Geogr. 159, 103088. doi:10.1016/j.apgeog.2023.103088
Jiao, J., Jiang, G., and Yang, R. (2018a). Impact of R&D technology spillovers on carbon emissions between China’s regions. Struct. Change Econ. Dyn. 47, 35–45. doi:10.1016/j.strueco.2018.07.002
Jiao, J., Yang, Y., and Bai, Y. (2018b). The impact of inter-industry R&D technology spillover on carbon emission in China. Nat. Hazards 91 (3), 913–929. doi:10.1007/s11069-017-3161-3
Jin, B., and Han, Y. (2021). Influencing factors and decoupling analysis of carbon emissions in China’s manufacturing industry. Environ. Sci. Pollut. Res. 28, 64719–64738. doi:10.1007/s11356-021-15548-0
Khan, Z., Ali, S., Umar, M., Kirikkaleli, D., and Jiao, Z. (2020). Consumption-based carbon emissions and International trade in G7 countries: the role of Environmental innovation and Renewable energy. Sci. Total Environ. 730, 138945. doi:10.1016/j.scitotenv.2020.138945
Klette, T. J., and Griliches, Z. (1996). The inconsistency of common scale estimators when output prices are unobserved and endogenous. J. Appl. Econ. 11 (4), 343–361. doi:10.1002/(sici)1099-1255(199607)11:4<343::aid-jae404>3.0.co;2-4
Knapp, T., and Mookerjee, R. (1996). Population growth and global CO2 emissions. Energy Policy 24 (1), 31–37. doi:10.1016/0301-4215(95)00130-1
Larrotta, C. (2017). Immigrants to the United States and adult education services. New Dir. Adult Continuing Educ. 2017 (155), 61–69. doi:10.1002/ace.20241
Levin, A., Lin, C.-F., and James Chu, C.-S. (2002). Unit root tests in panel data: asymptotic and finite-sample properties. J. Econ. 108 (1), 1–24. doi:10.1016/s0304-4076(01)00098-7
Li, J., and Lin, B. (2017). Does energy and CO2 emissions performance of China benefit from regional integration? Energy Policy 101, 366–378. doi:10.1016/j.enpol.2016.10.036
Liang, S., Yang, X., Qi, J., Wang, Y., Xie, W., Muttarak, R., et al. (2020). CO2 emissions embodied in international migration from 1995 to 2015. Environ. Sci. Technol. 54 (19), 12530–12538. doi:10.1021/acs.est.0c04600
Liddle, B., and Lung, S. (2010). Age-structure, urbanization, and climate change in developed countries: revisiting STIRPAT for disaggregated population and consumption-related environmental impacts. Popul. Environ. 31 (5), 317–343. doi:10.1007/s11111-010-0101-5
Liebert, S., and Ameringer, C. F. (2013). The health care safety net and the affordable care act: implications for hispanic immigrants. Public Adm. Rev. 73 (6), 810–820. doi:10.1111/puar.12147
Lozano, S., and Gutiérrez, E. (2008). Non-parametric frontier approach to modelling the relationships among population, GDP, energy consumption and CO2 emissions. Ecol. Econ. 66 (4), 687–699. doi:10.1016/j.ecolecon.2007.11.003
Lu, Y., and Zhao, X. (2008). Review of researches on household energy consumption. Int. J. Hydroelectr. Energy 26 (1), 187–191.
Lv, Y., Chen, W., and Cheng, J. (2020). Effects of urbanization on energy efficiency in China: new evidence from short run and long run efficiency models. Energy Policy 147, 111858. doi:10.1016/j.enpol.2020.111858
Ma, G., and Hofmann, E. T. (2019). Population, immigration, and air quality in the USA: a spatial panel study. Popul. Environ. 40 (3), 283–302. doi:10.1007/s11111-018-0311-9
Martínez-Zarzoso, I., and Maruotti, A. (2011). The impact of urbanization on CO2 emissions: evidence from developing countries. Ecol. Econ. 70 (7), 1344–1353. doi:10.1016/j.ecolecon.2011.02.009
Mensah, C. N., Long, X., Boamah, K. B., Bediako, I. A., Dauda, L., and Salman, M. (2021). Correction to: the effect of innovation on CO2 emissions across OECD countries from 1990 to 2014. Environ. Sci. Pollut. Res. 28 (15), 19535. doi:10.1007/s11356-021-13136-w
Mensah, C. N., Long, X., Dauda, L., Boamah, K. B., and Salman, M. (2019). Innovation and CO2 emissions: the complimentary role of eco-patent and trademark in the OECD economies. Environ. Sci. Pollut. Res. 26 (22), 22878–22891. doi:10.1007/s11356-019-05558-4
Oldekop, J. A., Sims, K. R. E., Whittingham, M. J., and Agrawal, A. (2018). An upside to globalization: international outmigration drives reforestation in Nepal. Glob. Environ. Change 52, 66–74. doi:10.1016/j.gloenvcha.2018.06.004
Oliinyk, O., Bilan, Y., Mishchuk, H., Akimov, O., and Vasa, L. (2021). The impact of migration of highly skilled workers on the country’s competitiveness and economic growth. Montenegrin J. Econ. 17 (3), 7–19. doi:10.14254/1800-5845/2021.17-3.1
Pan, W., Carr, D., Barbieri, A., Bilsborrow, R., and Suchindran, C. (2007). Forest clearing in the Ecuadorian amazon: a study of patterns over space and time. Popul. Res. Policy Rev. 26 (5-6), 635–659. doi:10.1007/s11113-007-9045-6
Pao, H.-T., and Tsai, C.-M. (2011). Multivariate Granger causality between CO2 emissions, energy consumption, FDI (foreign direct investment) and GDP (gross domestic product): evidence from a panel of BRIC (Brazil, Russian Federation, India, and China) countries. Energy 36 (1), 685–693. doi:10.1016/j.energy.2010.09.041
Poumanyvong, P., and Kaneko, S. (2010). Does urbanization lead to less energy use and lower CO2 emissions? A cross-country analysis. Ecol. Econ. 70 (2), 434–444. doi:10.1016/j.ecolecon.2010.09.029
Qi, W., and Li, G. (2020). Residential carbon emission embedded in China’s inter-provincial population migration. Energy Policy 136, 111065. doi:10.1016/j.enpol.2019.111065
Qin, M., Fan, L., Li, J., and Li, Y. (2021). The income distribution effects of environmental regulation in China: the case of binding SO2 reduction targets. J. Asian Econ. 73, 101272. doi:10.1016/j.asieco.2021.101272
Radel, C., Schmook, B., and McCandless, S. (2010). Environment, transnational labor migration, and gender: case studies from southern Yucatán, Mexico and Vermont, USA. Popul. Environ. 32 (2-3), 177–197. doi:10.1007/s11111-010-0124-y
Roberts, M. R., and Whited, T. M. (2013). Endogeneity in empirical corporate Finance1. Handb. Econ. Finance 2, 493–572. doi:10.1016/b978-0-44-453594-8.00007-0
Shahbaz, M., Nasreen, S., Ahmed, K., and Hammoudeh, S. (2017). Trade openness–carbon emissions nexus: the importance of turning points of trade openness for country panels. Energy Econ. 61, 221–232. doi:10.1016/j.eneco.2016.11.008
Shan, S., Genç, S. Y., Kamran, H. W., and Dinca, G. (2021). Role of green technology innovation and renewable energy in carbon neutrality: a sustainable investigation from Turkey. J. Environ. Manag. 294, 113004. doi:10.1016/j.jenvman.2021.113004
Solarin, S. A., and Bello, M. O. (2020). Energy innovations and environmental sustainability in the U.S.: the roles of immigration and economic expansion using a maximum likelihood method. Sci. Total Environ. 712, 135594. doi:10.1016/j.scitotenv.2019.135594
Sun, W., and Huang, C. (2020). How does urbanization affect carbon emission efficiency? Evidence from China. J. Clean. Prod. 272, 122828. doi:10.1016/j.jclepro.2020.122828
Taylor, M. J., Aguilar-Støen, M., Castellanos, E., Moran-Taylor, M. J., and Gerkin, K. (2016). International migration, land use change and the environment in Ixcán, Guatemala. Land Use Policy 54, 290–301. doi:10.1016/j.landusepol.2016.02.024
Teixeira, C. (2013). Living on the “edge of the suburbs” of Vancouver: a case study of the housing experiences and coping strategies of recent immigrants in Surrey and Richmond. Can. Geogr./ Le Géogr. Can. 58 (2), 168–187. doi:10.1111/j.1541-0064.2013.12055.x
Tian, X., Chang, M., Shi, F., and Tanikawa, H. (2014). How does industrial structure change impact carbon dioxide emissions? A comparative analysis focusing on nine provincial regions in China. Environ. Sci. Policy 37, 243–254. doi:10.1016/j.envsci.2013.10.001
Wang, S., Li, G., and Fang, C. (2018). Urbanization, economic growth, energy consumption, and CO2 emissions: empirical evidence from countries with different income levels. Renew. Sustain. Energy Rev. 81, 2144–2159. doi:10.1016/j.rser.2017.06.025
Wei, L., and Liu, Z. (2022). Spatial heterogeneity of demographic structure effects on urban carbon emissions. Environ. Impact Assess. Rev. 95, 106790. doi:10.1016/j.eiar.2022.106790
Wu, L., Sun, L., Qi, P., Ren, X., and Sun, X. (2021). Energy endowment, industrial structure upgrading, and CO2 emissions in China: revisiting resource curse in the context of carbon emissions. Resour. Policy 74, 102329. doi:10.1016/j.resourpol.2021.102329
Wu, Z., Xie, X., and Wang, S. (2012). The influence of economic development and industrial structure to carbon emission based on China’s provincial panel data. Chin. J. Manag. Sci. 2012 (3), 161–166.
You, J., and Zhang, W. (2022). How heterogeneous technological progress promotes industrial structure upgrading and industrial carbon efficiency? Evidence from China’s industries. Energy 247, 123386. doi:10.1016/j.energy.2022.123386
Young, Y., Loebach, P., and Korinek, K. (2018). Building walls or opening borders? Global immigration policy attitudes across economic, cultural and human security contexts. Soc. Sci. Res. 75, 83–95. doi:10.1016/j.ssresearch.2018.06.006
Zhang, C., Li, Z., and Zhou, Y. (2021). Can carbon emissions trading pilot policy promote regional 1152 emissions reductions? Soft Sci. 10, 93–99. doi:10.13956/j.ss.1001-8409.2021.10.15
Zhang, H. (2020). Can low-carbon city construction reduce carbon emissions? Evidence from a quasi-natural experiment. Bus. Manag. J. 42 (6), 25–41. doi:10.19616/j.cnki.bmj.2020.06.002
Zhang, Y., and Zhang, S. (2018). The impacts of GDP, trade structure, exchange rate and FDI inflows on China’s carbon emissions. Energy Policy 120, 347–353. doi:10.1016/j.enpol.2018.05.056
Zhao, X., Li, N., and Ma, C. (2012). Residential energy consumption in urban China: a decomposition analysis. Energy Policy 41, 644–653. doi:10.1016/j.enpol.2011.11.027
Zhao, Y., Zhang, Z., Wang, S., and Wang, S. (2014). CO2Emissions embodied in China’s foreign trade: an investigation from the perspective of global vertical specialization. China and World Econ. 22 (4), 102–120. doi:10.1111/j.1749-124x.2014.12077.x
Zhong, Z., Guo, Z., and Zhang, J. (2021). Does the participation in global value chains promote interregional carbon emissions transferring via trade? Evidence from 39 major economies. Technol. Forecast. Soc. Change 169, 120806. doi:10.1016/j.techfore.2021.120806
Keywords: population mobility, carbon emissions, Australia, heterogeneity, technological innovation
Citation: Guo H (2024) Impact of population mobility on regional carbon emissions: empirical evidence from Australia. Front. Environ. Sci. 12:1392267. doi: 10.3389/fenvs.2024.1392267
Received: 27 February 2024; Accepted: 02 April 2024;
Published: 01 May 2024.
Edited by:
Zhangqi Zhong, Guangdong University of Foreign Studies, ChinaReviewed by:
Lu Yang, Guangdong University of Foreign Studies, ChinaXuanxuan Jin, Zhejiang University of Finance and Economics, China
Copyright © 2024 Guo. This is an open-access article distributed under the terms of the Creative Commons Attribution License (CC BY). The use, distribution or reproduction in other forums is permitted, provided the original author(s) and the copyright owner(s) are credited and that the original publication in this journal is cited, in accordance with accepted academic practice. No use, distribution or reproduction is permitted which does not comply with these terms.
*Correspondence: Hongbo Guo, guohongboau@126.com