Time to death and its predictors among adult patients with COVID-19: A retrospective cohort study in Ethiopia
- 1School of Nursing, College of Health Science, Woldia University, Woldia, Ethiopia
- 2School of Nursing and Midwifery, College of Health Science, Addis Ababa University, Addis Ababa, Ethiopia
Background: Coronavirus (COVID-19) disease affected people throughout the globe and has become a severe threat to the health and wellbeing of the global community. Time to death and predictors of mortality vary across settings. So far, no or few related studies have been undertaken in Ethiopia. Studying the time to death from COVID-19 and its predictors is essential to understand the characteristics of the disease and thereby contribute to the identification of indicators for early detection and initiation of treatment. Therefore, this study aimed to estimate time to death and its predictors among adults with COVID-19 in Ethiopia.
Methods: A retrospective follow-up study was conducted among 602 adults with COVID-19 attending Eka Kotebe General Hospital, COVID-19 Treatment Center, between 13 March 2020 and 13 November 2020. The data were entered by Epi-data version 4.2 while the analysis was carried out using STATA version 16. A Kaplan–Meier survivor curve was computed to estimate the survival probabilities. A log-rank test was used to compare the difference in survival curves. Cox proportional hazard models were fitted to identify the predictors of time to death.
Results: The overall median time to death was 21 days. Older adults (aged ≥65 years) [adjusted hazard ratio (AHR) 2.22, 95% confidence interval (CI) 1.02–4.86], being men (AHR 3.04, 95% CI 1.61–5.74), shortness of breathing at admission (AHR 2.29, 95% CI 1.16–4.54), comorbidity (AHR 2.23, 95% CI 1.04–4.80), diabetes mellitus (AHR 2.31, 95% CI 1.30–4.08), altered cardiac function (AHR 2.07, 95% CI 1.21–3.43), and baseline white blood cell count of greater than 10 (103/µl) (AHR 2.62, 95% CI 1.55–4.44) were independent predictors of COVID-19 mortality.
Conclusion: Male sex, older adults, shortness of breathing at admission, patients with comorbidities, and higher blood cell count were significant predictors of time to death from COVID-19. Therefore, concerned stakeholders should focus on those predictors of mortality and design interventions accordingly to enhance the survival of patients with COVID-19.
Introduction
The human coronavirus (COVID-19) is a large group of coronaviruses infecting multiple organs, mainly the respiratory system, ranging from the common cold to bronchiolitis and pneumonia. The coronavirus is named after its crown-like shape (1). The COVID-19 is a respiratory tract disease caused by a newly occurring coronavirus species called SARS-CoV-2. It was first identified in China, in Wuhan, Hubei Province, in December 2019. On 7 January 2020, in Wuhan, Chinese researchers started isolating a new individual infected by a coronavirus (2). The WHO announced on 11 March 2020 that the emerging COVID-19 disease was a global pandemic (3). In Ethiopia, the first case of COVID-19 was detected on 13 March 2020, in a 48-year-old Japanese man with a travel history. Within a few days, another three cases of COVID-19 were identified, who had had contact with the first case (4). To date, the country has tested more than five million individuals with suspected COVID-19, of which 494,180 cases were confirmed positive and, of these, 7,572 died, and 472,171 recovered (5).
Globally, the distribution and seriousness of the virus have increased dramatically (6). According to the latest WHO weekly epidemiological report, the virus appeared in more than 218 countries and territories, and over 624 million confirmed cases and over 6.5 million deaths have been reported globally. Furthermore, the highest number of deaths were reported in the United States (>1.1 million), India (>0.5 million), and France (>150,000) (7).
The likelihood of COVID-19 mortality rises with age. Studies showed that men had risk of death 11.5% higher than women. Renal disease, chronic lung diseases, and diabetes mellitus increased the risk of death by 52.3%, 89.5%, and 41.3%, respectively (8). The rates of spread of COVID-19 are higher than influenza and the likelihood of mortality is also greater. While most patients are estimated to have a remarkable improvement, the prognosis of older adults with the underlying disease may be poor (9).
The survival time varies across different countries. In the Democratic Republic of the Congo (DRC), Africa, the median survival from the first day of hospitalization among all admitted patient with COVID-19 is 9 days. The overall probability of survival at 2, 3, 5, and 10 days was 90%, 78.7%, 73.0%, and 71.6%, respectively (10). In Brazil, the median survival time from the first day of hospitalization was 12 days. The overall survival rate was 79.21% at 5 days and 59.22% at 10 days of hospitalization (11). In Mexico, the survival rate at 3 days was 86.9% (12), and in another set of Brazil numbers, the probability of survival at 10 days was 95.1% (13). A prospective observational cohort study among adult patients (aged ≥18 years) in New York found that the overall median survival time from the date of hospital admission was 9 days in New York City (14). A multicenter observational study in Mexico among critically ill patients reported a median survival time of 25 day from the first day of admission to the intensive care unit (15). Furthermore, a study from China among severely and critically ill patients found a median survival time of 25 day from the first confirmed positive result of a reverse transcription (RT)-PCR test (16).
Older age (14), male sex (17, 18), shortness of breath on admission (19, 20), chronic pulmonary diseases (21), diabetes (21, 22), cardiovascular diseases (23, 24), hypertension (25), severe acute kidney injury (21, 24), asthma (26), hypoalbuminemia (27), and increase in white blood cells (WBC) (28) were factors that decreased the survival status of patients with COVID-19.
The time to death from COVID-19 varies across different settings. Studying the time to death from COVID-19 and its predictors is essential to understand the characteristics of the diseases and thereby contribute to the identification of indicators for the early detection and initiation of treatment. So far, no or few related studies have been undertaken in Ethiopia. Little is known about the time to death from COVID-19 and its predictors, and even the process of the disease is not clearly known. The reports of time to death in different studies are also inconclusive. Therefore, the aim of the present study was to investigate time to death from COVID-19 and its predictors among adult patients in Ethiopia.
Materials and methods
Study design and setting
A retrospective follow-up study was conducted among 602 adult patients with COVID-19 confirmed by RT-PCT admitted to Eka Kotebe General Hospital, COVID-19 Treatment Center. Eka Kotebe General Hospital is the first COVID-19 treatment center in Ethiopia. The center is located in Addis Ababa, the capital of Ethiopia, where the highest number of cases of COVID-19 was recorded among all towns in Ethiopia. For the first time in Ethiopia, on 13 March 2020, 450 infected and suspected patients entered this facility, and 73 patients were confirmed to have COVID-19. The Eka Kotebe COVID-19 Treatment Center was relatively the only well-quipped treatment center in terms of ventilator machine, availability of the COVID-19 diagnosis test, and healthcare providers. According to a study in Ethiopia, the median time from the onset of symptoms to admission to hospital (diagnosis) was 4 days (interquartile range 3–5 days) for all cases.
Study participants
The source population was adult patients (aged ≥18 years) with COVID-19 admitted to the Eka Kotebe General Hospital COVID-19 Treatment Center. The study population was adult patients with COVID-19 who attended the Eka Kotebe General Hospital COVID-19 Treatment Center between 13 March 2020 and 13 November 2020.
The inclusion criteria were as follows: (1) medical records of patients with confirmed COVID-19 at Eka Kotebe General Hospital COVID-19 Treatment Center; (2) patients aged 18 years and above; and (3) patients admitted during the study period.
The exclusion criteria were as follows: (1) incomplete patient charts (i.e., charts without the date of hospitalization and the date on which the outcome occurred, charts without final outcomes, such as death or censored); and (2) some independent variables.
Variables
The dependent variable was time to death.
Independent variables were as follows: (1) sociodemographic factors, such as age and sex; (2) clinical characteristics on admission (cough, fever, shortness of breath, fatigue, myalgia, chest pain, sore throat, blood pressure, and oxygen saturation); (3) comorbidities [having an existing comorbidity, number of comorbidities, cardiac disease, congestive heart failure (CHF)], hypertension, diabetes mellitus, chronic lung diseases, HIV/AIDS, malignancy, chronic liver disease, renal diseases, and chronic neurological diseases); (4) treatment-related factors (azithromycin, dexamethasone, ceftriaxone, and vancomycin); and (5) laboratory findings (neutrophil, monocyte, lymphocyte, red blood count, hemoglobin hematocrit, platelet count, urea, creatinine, AST, ALT, random blood sugar (RBS), and blood group).
Operational definitions
Time to death is the time interval from the first day of hospitalization to the time when the death of a patient is confirmed. The event of interest in this study was the occurrence of death due to COVID-19 at the time of hospital stay between 13 March 2020 and 13 November 2020. The follow-up time is the time from the first day of hospitalization until an event occurred. Censored indicated patients who did not develop the outcome of interest (death) during the follow-up period. The baseline laboratory marker was the first laboratory marker after hospitalization.
The severity of COVID-19 was classified as follows: (1) Asymptomatic infection: individuals who test positive for SARS-CoV-2 using a virologic test or an antigen test) but who have no symptoms that are consistent with COVID-19; (2) mild illness: individuals who have any of the various signs and symptoms of COVID-19 (e.g., fever, cough, sore throat, malaise, headache, muscle pain, nausea, vomiting, diarrhea, loss of taste and smell) but who do not have shortness of breath, dyspnea, or abnormal chest imaging; (3) moderate illness: individuals who show evidence of lower respiratory disease during the clinical assessment or imaging and who have an oxygen saturation measured by pulse oximetry (SpO2) ≥94% on room air at sea level; and (4) severe illness: individuals who have an SpO2 <94% on room air at sea level, a ratio of arterial partial pressure of oxygen to fraction of inspired oxygen (PaO2/FiO2) <300 mmHg, a respiratory rate >30 breaths/min, or lung infiltrates >50% (29, 30).
Statistical analysis
Data entry was done by Epi-data version 4.2 and the analysis was carried out using STATA version 16. The incidence of death was calculated for the entire study period per 1000 person-days. A survival table was employed to estimate survival probabilities after diagnosis of COVID-19 at different time intervals. A Kaplan–Meier survivor curve was computed to estimate the survival time. A log-rank test was used to compare the difference in survival curves. A Cox proportional hazard regression model was fitted to identify significant predictors. The variance inflation factor (VIF) and tolerance were computed to check the existence of multicollinearity before running the multivariable Cox proportional hazard regression model. A VIF above 4 or tolerance below 0.25 indicated that multicollinearity might exist (31). In this study, the maximum VIF was 2.33 for comorbidity status with a mean VIF of 1.42 and the minimum tolerance value was 0.43. Thus, there was no multicollinearity between covariates (Supplementary File S1). Based on the global test result of Schoenfeld residuals, all the covariates fulfill the PH assumption (chi-square = 12.73and p-value = 0.6234). The crude hazard ratio (cHR) was computed in a bivariate analysis to see the strength association of a single variable with the outcome variable. Variables with a p-value of ≤0.25 in the bivariate analysis were fitted and included in the multivariable Cox proportional hazard regression model. In the multivariable analysis, an adjusted hazard ratio (AHR) with its 95% confidence interval (CI) and p-value ≤0.05 were used to determine the strength of the relationship and to identify statistically significant variables, respectively.
Results
A total of 614 charts of adult patients with COVID-19 were reviewed, of which 602 (98.04%) records met the enrollment criteria in the final analysis. The remaining 12 charts were incomplete because the date of hospitalization or the final outcome (death or censored) was not recorded. Out of the 602 adult COVID-19 charts followed for 8 months, 514 were censored and 87 died.
Sociodemographic characteristics of the study participants
Of the 602 study participants, 381 (63.29%) were men. Regarding the age distribution, the median age of the study participants was 41 years and the mean age was 44.8 ± 18.9 years.
Clinical features of the study participants on admission
The majority of study participants (n=518, 86.05%) had a history of one or more symptoms at admission. Most of the participants (n=473, 78.57%) had a cough on admission, followed by fever (n=240, 39.87%), shortness of breath (n=239, 39.70%), fatigue 134 (22.26%), myalgia 87 (14.45%), chest pain 80 (13.29%), and sore throat (n=77, 12.79%). The mean diastolic blood pressure on admission was higher among those who died than among those who were censored (84.6 vs. 76.8 mmHg). Regarding oxygen saturation, the mean SpO2 (%) among those who died was significantly lower than those who were censored (86.1% vs. 92.2%). Regarding the severity of COVID-19 illness on admission, 84 (13.9%) of the participants were asymptomatic, 304 (64.4%) were mild, 64 (10.6%) were moderate, and 150 (24.9%) were severe.
Baseline laboratory marker of the study participants
Concerning the baseline complete cell count, the mean WBC count of study participants was higher among those who died than those who were censored (13.7 vs. 7.33). The mean RBS of study participants who died and who were censored was 140 and 126.2 mg/dl, respectively. The majority of participants (n=252, 44.2%) were of the blood type “B,” followed by the blood group “A” (n=213, 37.3%) (Table 1).
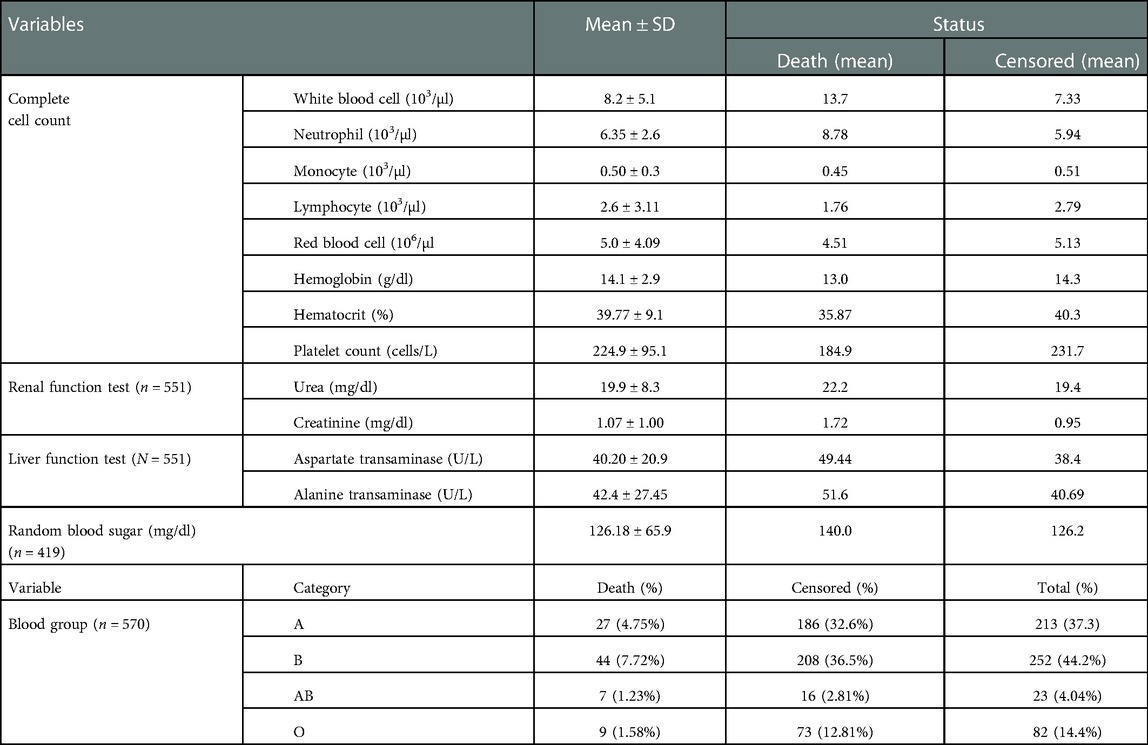
Table 1. Baseline laboratory marker of adult COVID-19 patients at Eka Kotebe General Hospital, COVID-19 Treatment Center, Addis Ababa, Ethiopia, 2021 (n = 602).
Pre-existing comorbidity status of study participants
Nearly half of the study participants (n=292, 48.5%) had at least one comorbidity; of them, 56 (18.86%) patients had three or more comorbidities. Among the comorbidities, hypertension was the most common (28.24%), followed by diabetes mellitus (23.26%), cardiac disease (9.63%), and renal diseases (6.81%) (Table 2).
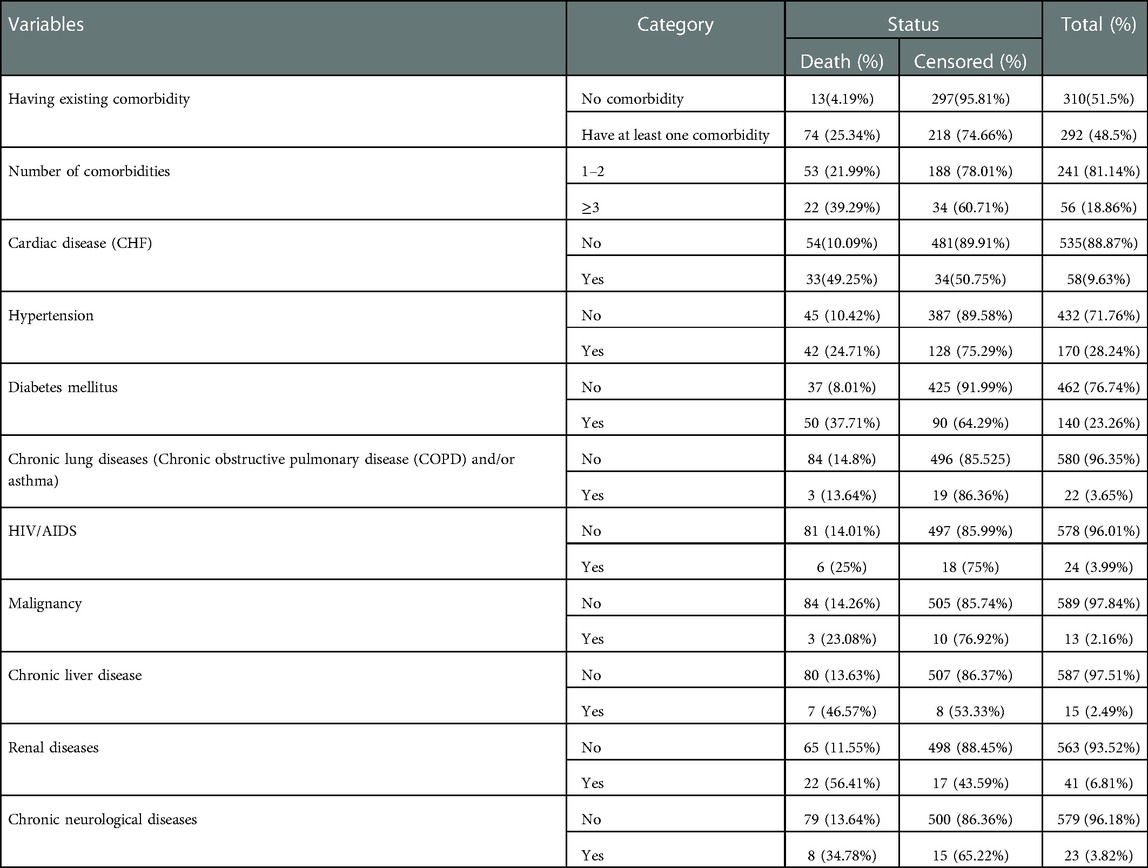
Table 2. Pre-existing comorbidity status of adult COVID-19 patients at Eka Kotebe General Hospital, COVID-19 Treatment Center, Addis Ababa, Ethiopia, 2021.
Treatment-related characteristics of study participants
Nearly half (n=297, 49.34%) the study participants received azithromycin and 189 (31.4%) were given dexamethasone. In total, 122 (20.27%) and 75 (12.46%) participants received ceftriaxone and vancomycin treatment. Only 12 (1.99%) of the participant were given chloroquine.
Survival status of patients with COVID-19
In the present study, 602 adult patients with COVID-19 were followed retrospectively. The median hospital stay was 13 days, with a minimum and maximum follow-up time of 2 and 58 days, respectively. In this study, 514 patients were censored and 87 died, resulting in a total cumulative incidence of death of 14.4% during the follow-up period. The total follow-up time was 8,109 person-days, with an incidence rate of 10.7 deaths per 1,000 person-day observations (95% CI 8.79–13.38).
Overall survival rate of patients with COVID-19
The overall Kaplan–Meier estimate showed that the probability of survival of patients with COVID-19 was high on the first day of admission and falls as the follow-up time increases. The overall median survival time of adult patients with COVID-19 was 21 days (Figure 1). The mean survival time of the study participants was 27.23 days (95% CI 23.82–30.63). The probability of survival for patients with COVID-19 at the start of follow-up was 100%. The probability of survival at 2, 3, 5, and 10 days was 99.8%, 99.5%, 97.6%, and 76.7%, respectively. The Kaplan–Meier curve with log-rank p-value shows differences in survival function between the categories of variables: age (Figure 2), shortness of breath at admission (Figure 3), dexamethasone treatment status (Figure 4), cardiac disease (Figure 5), hypertension (Figure 6), and diabetes (Figure 7).
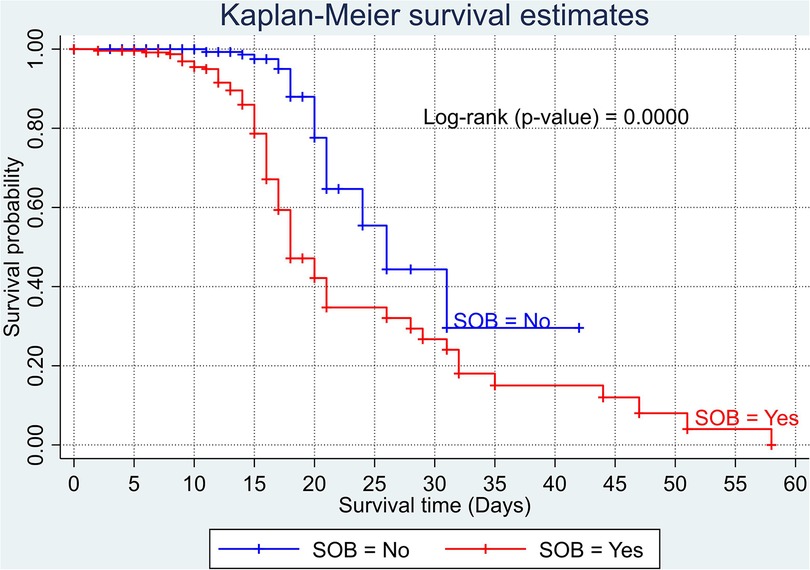
Figure 3. Kaplan–Meier survival curve based on the presence of shortness of breathing at admission among adult COVID-19 patients.
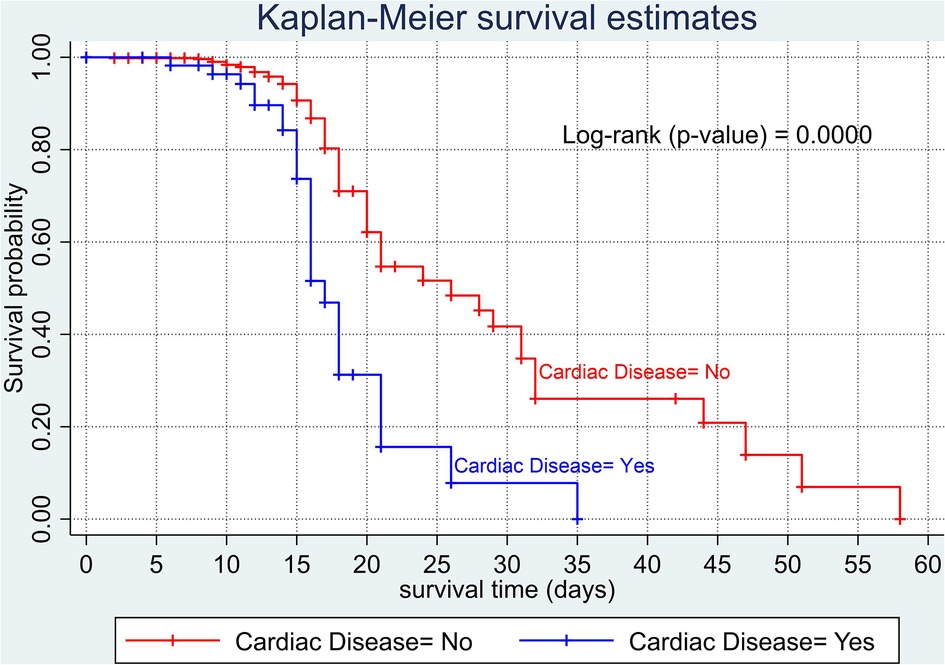
Figure 5. Kaplan–Meier survival curve based on the presence of cardiac disease (heart failure) among adult COVID-19 patients.
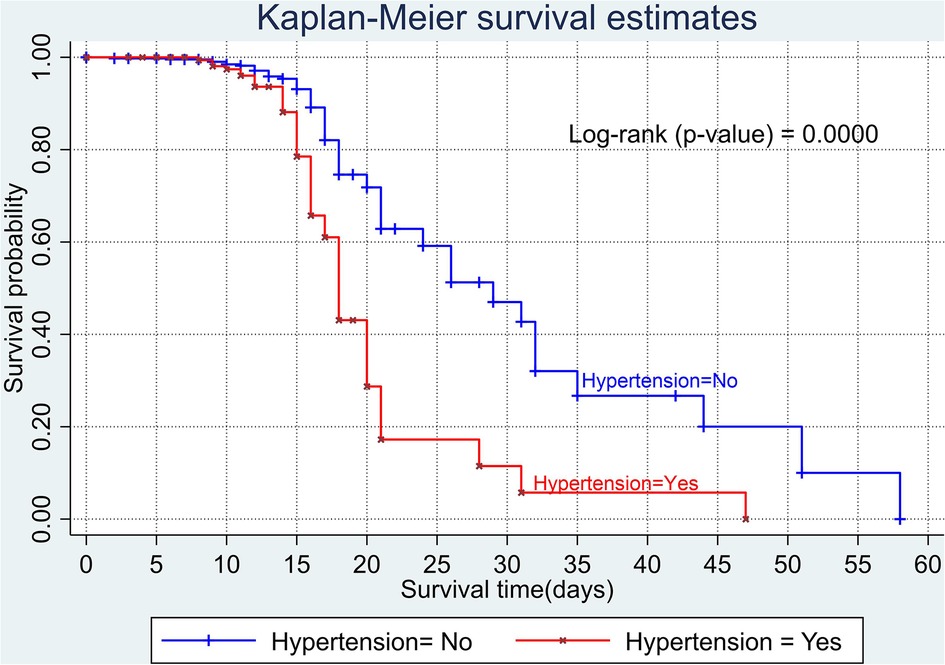
Figure 6. Kaplan–Meier survival curve based on the presence of hypertension among adult COVID-19 patients.
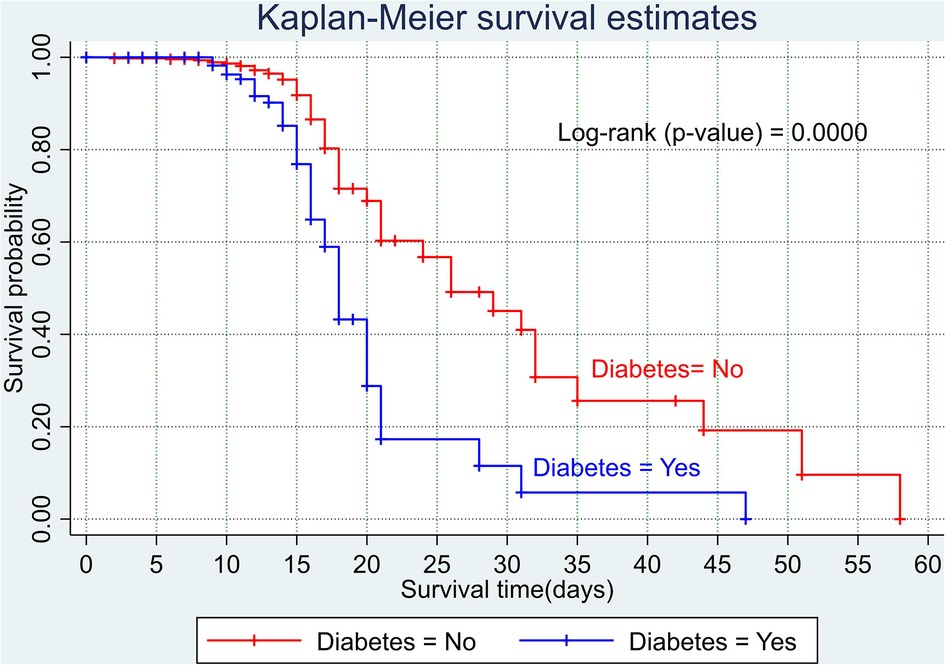
Figure 7. Kaplan–Meier survival curve based on the presence of diabetes among adult COVID-19 patients.
Predictors of COVID-19 mortality
In the multivariable Cox proportional hazards model, age, sex, shortness of breath, having existing comorbidities, diabetes mellitus, cardiac disease, and WBC (103/µl) were significant predictors of COVID-19 mortality with a p-value of <0.05.
The multivariable analysis (Table 3) revealed that adults with COVID-19, who were aged 65 years or older, had a more than twofold risk of death (AHR 2.22, 95% CI 1.02–4.86) than those adults in the age group of 18–40 years. The risk of death from COVID-19 was nearly three times higher (AHR 3.04, 95% CI 1.61–5.74) for men than for women. Patients with a shortness of breath at admission had more than twice (AHR 2.29, 95% CI 1.16–4.54) the risk of death than those patients without shortness of breath at admission. Patients with COVID-19 with at least one comorbidity had a more than twofold risk of death (AHR 2.23, 95% CI 1.04–4.80) than those with no comorbidities. The risk of death from COVID-19 was more than twofold (AHR 2.31, 95% CI 1.30–4.08) for patients with diabetes than those without diabetes. Patients with cardiac disease had a more than twofold risk of death (AHR 2.07, 95% CI 1.21–3.43) from COVID-19 than those without cardiac disease. Furthermore, patients with a baseline WBC count greater than 10 (103/µl) had a more than twofold risk of death (AHR 2.62, 95% CI 1.55–4.44) than those with a baseline WBC count within the normal range (≥4–≤10 [103/µl]).
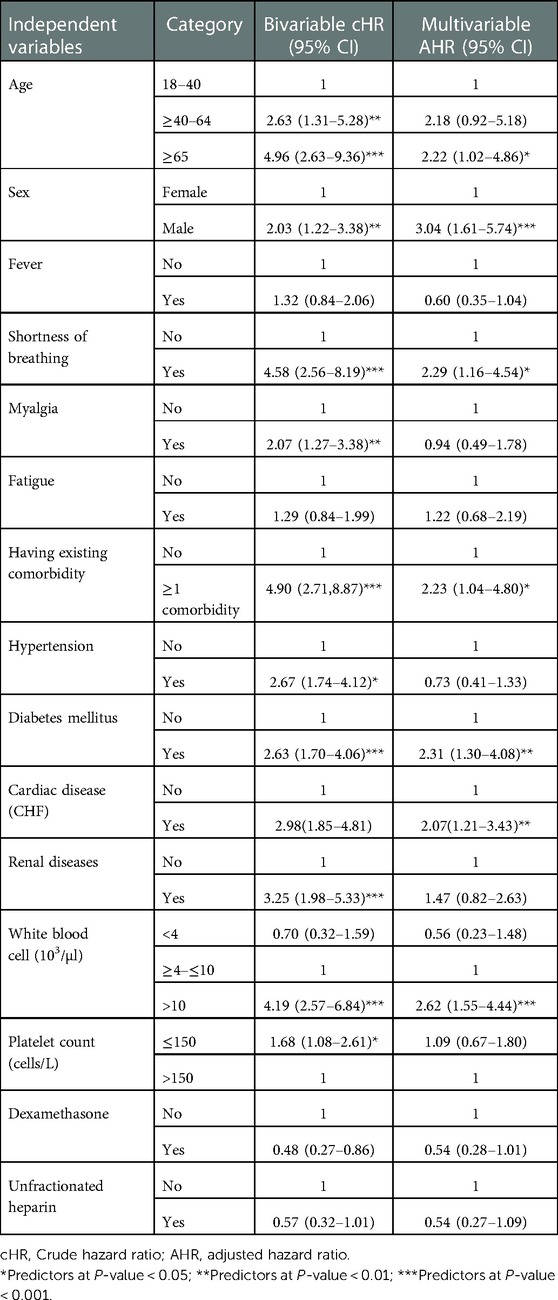
Table 3. Cox proportional hazard model analysis for predictors of time to death among adults with COVID-19 at Eka Kotebe General Hospital, COVID-19 Treatment Center, Addis Ababa, Ethiopia, 2021.
Discussion
The present study aimed to assess the survival status and predictors of mortality among adults with COVID-19 attending Eka Kotebe General Hospital, COVID-19 Treatment Center. This study shows that the overall cumulative mortality rate in an adult with COVID-19 during the study period was 14.4%. This finding is lower than the study carried out in DRC (29%) (32), China (28%) (33), New York City (43%) (34), and Brazil (46.25%) (35). The discrepancy of results that have been seen among studies might be due to the high prevalence of comorbidity recorded in studies from New York City (77%) and Brazil (70.9%) and the high number of older adults (aged ≥65 years) in the study from DRC compared to this study (31.9% vs. 19.93%, respectively) (36). Furthermore, this retrospective follow-up study includes patients with mild symptoms and a high number of younger adults than the above studies.
On the other hand, the overall cumulative mortality rate in the present study is higher than the studies carried out in Egypt (11.7%) (37) and Denmark (5.5%) (21). The difference is likely to be due to the fact that, later in our setup, health resources are restricted and admission becomes available for moderate and severe cases. This may increase the mortality rate in the hospital. The mortality rate in this study is consistent with the study in Italy (14.4%) (38).
The present study revealed that the probability of survival at 2, 3, 5, and 10 days was 99.8%, 99.5%, 97.6%, and 76.7%, respectively. The probability of survival in the present study was higher than in a study conducted in DRC (90%, 78.7%, 73.0%, and 71.6%, respectively) (39), in Mexico (86.9%) (40) at 3 days, and Brazil (79.21% and 59.22%) (41) at 5 and 10 days, respectively. In contrast, the findings of the present study were lower than the study conducted in another setting in Brazil, with a probability of survival of 95.1% at 10 days (42). This difference might be due to demographic characteristics. Furthermore, this discrepancy may be due to a lack of an early screening program and a higher proportion of severe COVID-19 at admission. It may also be due to variations in follow-up time, study period, and the clinical features of patients.
In this study, adults’ overall median survival time with COVID-19 was found to be 21 days. This finding is higher than the study carried out in DRC (9 days) (43), New York City (9 days) (14), Brazil (12 days) (26), and Fortaleza (Northeast Brazil) (19 days) (44). A possible explanation might be the high number of severely or critically ill patients. For example, the study conducted in DRC, at Kinshasa University Hospital, is in a tertiary care hospital. A high number of critically ill patients were referred from other hospitals. Therefore, patients may die within a short period of time.
In addition, a possible explanation for variations in the median survival time could be due to different follow-up times. In this study, there is a long follow-up time compared to the above studies. However, this finding was lower than that in the study carried out in Tongji Hospital, China (25 days) (45), and Germany (25 days) (26). This could be due to the difference in the quality of care in the cases of COVID-19 between low-income and high-income countries, such as a shortage of ventilator machines (46).
According to the results of this study, age was found to be a significant predictor of COVID-19 mortality. Older adults (aged ≥65 years) had an almost twofold risk of dying than young adults (aged 18–40 years). This finding points out that older people with early symptoms of COVID-19 should seek care immediately. This finding is supported by other previous studies conducted in African countries (47, 48), Denmark (49), China (50), and the United States and Mexico (42). A possible explanation might be that older adults were susceptible to multiple organ failure and comorbidities, increasing the likelihood of dying. Furthermore, a recent study revealed that older adults are at high risk of mortality with COVID-19 due to four main reasons: (1) the existence of asymptomatic systemic inflammation in the absence of significant disease; (2) a reduced immune system and type I interferon response due to long-lasting inflammation; (3) downregulation of ACE2 receptors; and (4) biological aging (26).
Sex is also found to be another critical predictor of COVID-19 mortality. Men have a nearly threefold higher risk of mortality compared to women. This finding is consistent with the studies carried out in China (44, 51, 52) and the United States (53–55). This may be due to recent emerged scientific evidence that COVID-19 in men can be worsened by androgen-enabled expression of ACE2 receptors, a permissive feature that engages the SARS-CoV-2 spike protein for infection (14). In addition, the staggeringly high mortality rate of COVID-19 in men may be partly explained by their pre-existing diseases (cardiac diseases, hypertension, and diabetes), higher risk behavioral patterns (cigarette smoking and alcohol consumption), and occupational exposure.
The risk of death among patients who presented with shortness of breath at admission was 2.29 times higher than those with no such symptom. Such a finding implies that the early identification of a patient with shortness of breath on admission may save patients' lives. This finding is supported by the study carried out in Ethiopia (14). Having a shortness of breath is one of the expressions of lung problems, ranging from reduced lung function up to a life-threatening condition. An insistent shortness of breath in patients might indicate structural and/or functional problems in the lung. This may increase the patients’ vulnerability to diseases such as COVID-19 and decrease the likelihood of survival with the stress leading to poor outcomes.
Patients with COVID-19 with at least one comorbid condition had twice the risk of death compared to patients without comorbidities. This finding is supported by other studies (17, 56). Patients with diabetes as a pre-existing comorbidity are twice as likely to die than those who do not have diabetes. This finding is pretty close to the studies conducted in Iran (57) and China (18). It is possible that since being diabetic is directly associated with low immune function, increasing the risk of bacterial and viral infection, including COVID-19, results in a poor outcome. This finding highlights the importance of timely monitoring and greater conservative management for those individuals with comorbidities. Furthermore, these findings point out that those vulnerable individuals should be in the front line in receiving the COVID-19 vaccine.
Likewise, several studies were conducted in different parts of the world: Brazil (24), China (58), Iran (59), and New York City (60). In this study, having heart disease is also another predictor of COVID-19 mortality. The presence of heart disease increases the risk of dying twofold. This might be because one of the most common immediate causes of death in patients with COVID-19 is respiratory failure (46). Having existing comorbidities such as cardiac disease might increase the incidence of respiratory failure and consequently lead to a poor prognosis. This finding implies that in the care of cardiac patients with COVID-19, special care should be incorporated to prevent further injury to the vital organs, thus improving their chance of survival.
Having a baseline WBC count of more than 10 (103/µl) had a risk of death 2.62 times higher compared to the normal range (≥4 to ≤10). This implies that an early increase in WBC count might be considered an indicator of a poor outcome. This finding argues that early screening of the WBC count on admission is essential to identify and provide special care for those patients, thereby reducing the likelihood of dying with COVID-19. This finding is in line with studies done in Hungary (61), China (62, 63), and Iran (19). The possible reason might be that an increase in the baseline WBC count is an early indicator of serious infection, increasing the risk of dying. However, the exact mechanism of how patients with a higher WBC level were at a high risk of death is unclear.
Since the data were derived retrospectively from medical reports of the patients, some critical variables such as laboratory markers (C-reactive protein level, albumin, cardiac markers dimer, IL-6, lactate dehydrogenase, and serum ferritin), which were strong predictors of COVID-19 mortality in other studies, were not or inadequately recorded and not included in the analysis. This led to difficulty in knowing the role of those predictors on survival time and was underestimated in predicting hospital death. The Eka Kotebe General Hospital COVID-19 Treatment Center is the first in Ethiopia and has better equipment and care. Patients were transferred late in their illness from other hospitals, and through these circumstances, the mortality rate might be overestimated.
Conclusion
Great efforts have been made to overcome the seriousness of COVID-19 diseases. However, the mortality rate does not show a significant decline. The probability of survival of patients with COVID-19 in this study was relatively high. Male sex, older adult (aged ≥65 years), having shortness of breath at admission, having at least one comorbidity, diabetes mellitus, cardiac disease, and baseline WBC count were found to be independent predictors of COVID-19 mortality. To the best of our knowledge, this is the first all-inclusive study in Ethiopia that gives insight into the survival status of patients with COVID-19. We believe it provides the best locally available evidence toward the different characteristics or nature of COVID-19 disease, thereby contributing to the initial identification of patients with a poor outcome.
Data availability statement
The raw data supporting the conclusions of this article will be made available by the authors, without undue reservation.
Ethics statement
The study protocol was reviewed by the Institutional Review Board (IRB) at Addis Ababa University, College of Health Science (protocol no. 06/21/SNM). Information obtained from the record was kept anonymous and confidential.
Author contributions
TA conceived the idea, developed the proposal, acquired the data, carried out the analysis and interpretation of the data, and drafted the article. AC and WA participated equally in the design, data management and analyses, interpretation, and manuscript writing. All authors contributed to the article and approved the submitted version.
Acknowledgments
We would like to acknowledge and thank Eka Kotebe General Hospital, COVID-19 Treatment Center, and the administrative officials for their valuable information and cooperation.
Conflict of interest
The authors declare that the research was conducted in the absence of any commercial or financial relationships that could be construed as a potential conflict of interest.
Publisher's note
All claims expressed in this article are solely those of the authors and do not necessarily represent those of their affiliated organizations, or those of the publisher, the editors and the reviewers. Any product that may be evaluated in this article, or claim that may be made by its manufacturer, is not guaranteed or endorsed by the publisher.
Supplementary material
The Supplementary Material for this article can be found online at: https://www.frontiersin.org/articles/10.3389/fepid.2022.1065184/full#supplementary-material.
References
1. Pene F, Merlat A, Vabret A, Rozenberg F, Buzyn A, Dreyfus F, et al. Coronavirus 229E-related pneumonia in immunocompromised patients. Clin Infect Dis. (2003) 37(7):929–32. doi: 10.1086/377612
2. Wang C, Horby PW, Hayden FG, Gao GF. A novel coronavirus outbreak of global health concern. Lancet. (2020) 395(10223):470–3. doi: 10.1016/S0140-6736(20)30185-9
3. Centers for Disease Control and Prevention. Human coronavirus types. Available at: https://www.cdc.gov/coronavirus/types.html (Accessed January 10, 2020).
4. Zhu N, Zhang D, Wang W. China novel coronavirus investigating and research team. A novel coronavirus from patients with pneumonia in China, 2019. N Engl J Med. (2020) 382:727–733. doi: 10.1056/NEJMoa2001017
5. Cucinotta D, Vanelli M. WHO declares COVID-19 a pandemic. Acta Biomed. (2020) 91(1):157–60. doi: 10.23750/abm.v91i1.9397
6. Guan WJ, Ni ZY, Hu Y, Liang WH, Ou CQ, He JX, et al. Clinical characteristics of coronavirus disease 2019 in China. N Engl J Med. (2020) 382(18):1708–20. doi: 10.1056/NEJMoa2002032
7. Huang C, Wang Y, Li X, Ren L, Zhao J, Hu Y, et al. Clinical features of patients infected with 2019 novel coronavirus in Wuhan, China. Lancet. (2020) 395(10223):497–506. doi: 10.1016/S0140-6736(20)30183-5
8. Morawska L, Milton DK. It is time to address airborne transmission of coronavirus disease 2019 (COVID-19). Clin Infect Dis. (2020) 71(9):2311–3. doi: 10.1093/cid/ciaa939
9. Wang D, Hu B, Hu C, Zhu F, Liu X, Zhang J, et al. Clinical characteristics of 138 hospitalized patients with 2019 novel coronavirus–infected pneumonia in Wuhan, China. JAMA. (2020) 323(11):1061–9. doi: 10.1001/jama.2020.1585
10. Yang X, Yu Y, Xu J, Shu H, Xia J, Liu H, et al. Clinical course and outcomes of critically ill patients with SARS-CoV-2 pneumonia in Wuhan, China: a single-centered, retrospective, observational study. Lancet Respir Med. (2020) 8(5):475–81. doi: 10.1016/S2213-2600(20)30079-5
11. To KK, Hung IF, Ip JD, Chu AW, Chan WM, Tam AR, et al. COVID-19 re-infection by a phylogenetically distinct SARS-coronavirus-2 strain confirmed by whole genome sequencing. Clin Infect Dis. (2020) 73:e2946–e2951. doi: 10.1093/cid/ciaa1275
12. Van Elslande J, Vermeersch P, Vandervoort K, Wawina-Bokalanga T, Vanmechelen B, Wollants E, et al. Symptomatic SARS-CoV-2 reinfection by a phylogenetically distinct strain. Clin Infect Dis. (2020) 73:354–356. doi: 10.1093/cid/ciaa1330
13. Tillett RL, Sevinsky JR, Hartley PD, Kerwin H, Crawford N, Gorzalski A, et al. Genomic evidence for reinfection with SARS-CoV-2: a case study. Lancet Infect Dis. (2021) 21(1):52–8. doi: 10.1016/S1473-3099(20)30764-7
14. Bepouka BI, Mandina M, Makulo JR, Longokolo M, Odio O, Mayasi N, et al. Predictors of mortality in COVID-19 patients at Kinshasa University Hospital, Democratic Republic of the Congo from March to June 2020. Pan Afr Med J. (2020) 37(105):105. doi: 10.11604/pamj.2020.37.105.25279
15. Zhou F, Yu T, Du R, Fan G, Liu Y, Liu Z, et al. Clinical course and risk factors for mortality of adult inpatients with COVID-19 in Wuhan, China: a retrospective cohort study. Lancet. (2020) 395(10229):1054–62. doi: 10.1016/S0140-6736(20)30566-3
16. World Health Organization. First case of covid-19 confirmed in Ethiopia (2020). Available at: https://www.afro.who.int/news/first-case-covid-19-confirmed-ethiopia (Accessed April 19, 2020)
17. El Aidaoui K, Haoudar A, Khalis M, Kantri A, Ziati J, El Ghanmi A, et al. Predictors of severity in COVID-19 patients in Casablanca, Morocco. Cureus. (2020) 12(9):e10716. doi: 10.7759/cureus.10716
18. Du RH, Liang LR, Yang CQ, Wang W, Cao TZ, Li M, et al. Predictors of mortality for patients with COVID-19 pneumonia caused by SARS-CoV-2: a prospective cohort study. Eur Respir J. (2020) 55(5):3–6. doi: 10.1183/13993003.00524-2020
19. Wang L, He W, Yu X, Hu D, Bao M, Liu H, et al. Coronavirus disease 2019 in elderly patients: characteristics and prognostic factors based on 4-week follow-up. J Infect. (2020) 80(6):639–45. doi: 10.1016/j.jinf.2020.03.019
20. Xie J, Covassin N, Fan Z, Singh P, Gao W, Li G, et al. editors Association between hypoxemia and mortality in patients with COVID-19 2020. Union Hospital, Wuhan, China: Elsevier (2020).
21. Eshrati B, Baradaran HR, Erfanpoor S, Mohazzab A, Moradi Y. Investigating the factors affecting the survival rate in patients with COVID-19: a retrospective cohort study. Med J Islam Repub Iran. (2020) 34(1):88. doi: 10.34171/mjiri.34.88
22. Shang J, Wang Q, Zhang H, Wang X, Wan J, Yan Y, et al. The relationship between diabetes mellitus and COVID-19 prognosis: a retrospective cohort study in Wuhan, China. Am J Med. (2020) 134:e6–e14. doi: 10.1016/j.amjmed.2020.05.033
23. Saleh SK, Oraii A, Soleimani A, Hadadi A, Shajari Z, Montazeri M, et al. The association between cardiac injury and outcomes in hospitalized patients with COVID-19. Intern Emerg Med. (2020) 15:1–10. doi: 10.1007/s11739-020-02466-1
24. Li X, Xu S, Yu M, Wang K, Tao Y, Zhou Y, et al. Risk factors for severity and mortality in adult COVID-19 inpatients in Wuhan. J Allergy Clin Immunol. (2020) 146(1):110–8. doi: 10.1016/j.jaci.2020.04.006
25. Emami A, Javanmardi F, Akbari A, Kojuri J, Bakhtiari H, Rezaei T, et al. Survival rate in hypertensive patients with COVID-19. Clin Exp Hypertens. (2021) 43(1):77–80. doi: 10.1080/10641963.2020.1812624
26. Santos M, Lucena EES, Lima KC, Brito AAC, Bay MB, Bonfada D. Survival and predictors of deaths of patients hospitalized due to COVID-19 from a retrospective and multicenter cohort study in Brazil. medRxiv. (2020).
27. Violi F, Cangemi R, Romiti GF, Ceccarelli G, Oliva A, Alessandri F, et al. Is albumin predictor of mortality in COVID-19? Antioxid Redox Signal. (2020) 35:139–142. doi: 10.1089/ars.2020.8142
28. Moradi EV, Teimouri A, Rezaee R, Morovatdar N, Foroughian M, Layegh P, et al. Increased age, neutrophil-to-lymphocyte ratio (NLR) and white blood cells count are associated with higher COVID-19 mortality. Am J Emerg Med. (2021) 40:11–4. doi: 10.1016/j.ajem.2020.12.003
29. World Health Organization (2020). Clinical management of severe acute respiratory infection (SARI) when COVID-19 disease is suspected: interim guidance, 13 March 2020. World Health Organization. https://apps.who.int/iris/handle/10665/331446. License: CC BY-NC-SA 3.0 IGO
30. National Institutes of Health. COVID-19 treatment guidelines panel. Coronavirus disease (COVID-19) treatment guidelines. Available at: https://www.covid19treatmentguidelines.nih.gov/ (Accessed September 26, 2022).
31. Elsayed AMM. Using different methods to overcome modeling problems. iosrjournals. (2021) 18:28–43. doi: 10.9790/5728-1702022843
32. Worldometers. Report coronavirus cases (2020). Available at: https://www.worldometers.info/coronavirus/? (Accessed May 23, 2020)
33. World Health Organization. Director-General’s remarks at the media briefing on 2019-nCoV on 11 February 2020. Available at: https://www.who.int/dg/speeches/detail/who-director-general-s-remarks-at-the-media-briefing-on-2019-ncov-on-11-february-2020 (Accessed November 28, 2020).
34. World Health Organization. “Weekly Epidemiological Update - 29 December 2020.” Www.who.int, 29 Dec. 2020, www.who.int/publications/m/item/weekly-epidemiological-update---29-december-2020 (Accessed 5 January 2021).
35. Tadic M, Cuspidi C, Mancia G, Dell’Oro R, Grassi G. COVID-19, hypertension and cardiovascular diseases: should we change the therapy? Pharmacol Res. (2020) 158:104906. doi: 10.1016/j.phrs.2020.104906
36. Yang J, Chen X, Deng X, Chen Z, Gong H, Yan H, et al. Disease burden and clinical severity of the first pandemic wave of COVID-19 in Wuhan, China. Nat Commun. (2020) 11(1):1–10. doi: 10.1038/s41467-019-13993-7
37. Verity R, Okell LC, Dorigatti I, Winskill P, Whittaker C, Imai N, et al. Estimates of the severity of coronavirus disease 2019: a model-based analysis. Lancet Infect Dis. (2020) 20(6):669–77. doi: 10.1016/S1473-3099(20)30243-7
38. Wu C, Chen X, Cai Y, Xia J, Zhou X, Xu S, et al. Risk factors associated with acute respiratory distress syndrome and death in patients with coronavirus disease 2019 pneumonia in Wuhan, China. JAMA Intern Med. (2020) 180(7):934–43. doi: 10.1001/jamainternmed.2020.0994
39. Cots JM, Alós J, Bárcena M, Boleda X. COVID-19 in Brazil: “so what?”. Lancet. (2020) 395(1461):1095–3. doi: 10.1016/S0140-6736(20)31095-3
40. Bakker CM D, Anema JR, Huirne JAF, Twisk J, Bonjer HJ, Schaafsma FG. Predicting return to work among patients with colorectal cancer. Br J Surg. (2020) 107(1):140. doi: 10.1002/bjs.11313
41. Yihun B, Tiruneh G, Tebekaw Y, Bekele B, Emway D, Fesseha N, et al. Immediate impacts of COVID-19 pandemic on essential MNCH services in selected health facilities in Ethiopia. Addis Ababa, Ethiopia: JSI Research & Training Institute (2020).
42. EPHI. COVID-19 pandemic preparedness and response in Ethiopia weekly bulletin (2020). Available at: https://www.ephi.gov.et/index.php/public-health-emergency/novel-corona-virus-update (Accessed November 20, 2020).
43. World Health Organization. WHO coronavirus disease (COVID-19) dashboard (2020). Available at: https://covid19.who.int/table (Accessed November 30, 2020).
44. Sousa GJB, Garces TS, Cestari VRF, Florencio RS, Moreira TMM, Pereira MLD. Mortality and survival of COVID-19. Epidemiol Infect. (2020) 148:e123. doi: 10.1017/S0950268820001405
45. Chilimuri S, Sun H, Alemam A, Mantri N, Shehi E, Tejada J, et al. Predictors of mortality in adults admitted with COVID-19: retrospective cohort study from New York city. West J Emerg Med. (2020) 21(4):779–84. doi: 10.5811/westjem.2020.6.47919
46. Reilev M, Kristensen KB, Pottegaard A, Lund LC, Hallas J, Ernst MT, et al. Characteristics and predictors of hospitalization and death in the first 9,519 cases with a positive RT-PCR test for SARS-CoV-2 in Denmark: a nationwide cohort. medRxiv. (2020).
47. Yanez ND, Weiss NS, Romand JA, Treggiari MM. COVID-19 mortality risk for older men and women. BMC Public Health. (2020) 20(1):1742. doi: 10.1186/s12889-020-09826-8
48. Li LQ, Huang T, Wang YQ, Wang ZP, Liang Y, Huang TB, et al. COVID-19 patients’ clinical characteristics, discharge rate, and fatality rate of meta-analysis. J Med Virol. (2020) 92(6):577–83. doi: 10.1002/jmv.25757
49. Promislow DEL. A geroscience perspective on COVID-19 mortality. J Gerontol A Biol Sci Med Sci. (2020) 75(9):e30–3. doi: 10.1093/gerona/glaa094
50. Africa CDC. Coronavirus disease 2019 (COVID-19) dashboard (2020). Available at: https://africacdc.org/covid-19/ (Accessed November 30, 2020).
51. Murillo-Zamora E, Hernandez-Suarez CM. Survival in adult inpatients with COVID-19. Public Health. (2021) 190:1–3. doi: 10.1016/j.puhe.2020.10.029
52. Cummings MJ, Baldwin MR, Abrams D, Jacobson SD, Meyer BJ, Balough EM, et al. Epidemiology, clinical course, and outcomes of critically ill adults with COVID-19 in New York city: a prospective cohort study. Lancet. (2020) 395(10239):1763–70. doi: 10.1016/S0140-6736(20)31189-2
53. Wang Y, Lu X, Li Y, Chen H, Chen T, Su N, et al. Clinical course and outcomes of 344 intensive care patients with COVID-19. Am J Respir Crit Care Med. (2020) 201(11):1430–4. doi: 10.1164/rccm.202003-0736LE
54. Ñamendys-Silva SA, Alvarado-Ávila PE, Domínguez-Cherit G, Rivero-Sigarroa E, Sánchez-Hurtado LA, Gutiérrez-Villaseñor A, et al. Outcomes of patients with COVID-19 in the intensive care unit in Mexico: a multicenter observational study. Heart Lung. (2021) 50(1):28–32. doi: 10.1016/j.hrtlng.2020.10.013
55. Barman MP, Rahman T, Bora K, Borgohain C. COVID-19 pandemic and its recovery time of patients in India: a pilot study. Diabetes Metab Syndr. (2020) 14(5):1205–11. doi: 10.1016/j.dsx.2020.07.004
56. Zhang S, Guo M, Duan L, Wu F, Hu G, Wang Z, et al. Development and validation of a risk factor-based system to predict short-term survival in adult hospitalized patients with COVID-19: a multicenter, retrospective, cohort study. Crit Care. (2020) 24(1):438. doi: 10.1186/s13054-020-03123-x
57. Chen R, Liang W, Jiang M, Guan W, Zhan C, Wang T, et al. Risk factors of fatal outcome in hospitalized subjects with coronavirus disease 2019 from a nationwide analysis in China. Chest. (2020) 158:97–105. doi: 10.1016/j.chest.2020.04.010
58. Tian W, Jiang W, Yao J, Nicholson CJ, Li RH, Sigurslid HH, et al. Predictors of mortality in hospitalized COVID-19 patients: a systematic review and meta-analysis. J Med Virol. (2020) 92(10):1875–83. doi: 10.1002/jmv.26050
59. Meng Y, Wu P, Lu W, Liu K, Ma K, Huang L, et al. Sex-specific clinical characteristics and prognosis of coronavirus disease-19 infection in Wuhan, China: a retrospective study of 168 severe patients. PLoS Pathog (2020) 16(4):e1008520. doi: 10.1371/journal.ppat.1008520
60. Woolcott OO, Bergman RN. Mortality attributed to COVID-19 in high-altitude populations. High Alt Med Biol. (2020) 21(4):409–16. doi: 10.1089/ham.2020.0098
61. Geospatial distribution and predictors of mortality in hospitalized patients with COVID-19: A cohort study. New York: Oxford University Press (2020).
62. Palaiodimos L, Kokkinidis DG, Li W, Karamanis D, Ognibene J, Arora S, et al. Severe obesity, increasing age and male sex are independently associated with worse in-hospital outcomes, and higher in-hospital mortality, in a cohort of patients with COVID-19 in the Bronx, New York. Metab Clin Exp. (2020) 108:154262. doi: 10.1016/j.metabol.2020.154262
Keywords: adult patients, COVID-19, Ethiopia, time to death, predictors
Citation: Atamenta T, Cherie A and Alemu W (2023) Time to death and its predictors among adult patients with COVID-19: A retrospective cohort study in Ethiopia. Front. Epidemiol. 2:1065184. doi: 10.3389/fepid.2022.1065184
Received: 9 October 2022; Accepted: 19 December 2022;
Published: 17 January 2023.
Edited by:
Shailendra Saxena, King George's Medical University, IndiaReviewed by:
Wildo Navegantes Araujo, University of Brasilia, BrazilHenry Maia Peixoto, University of Brasilia, Brazil
© 2023 Atamenta, Cherie and Alemu. This is an open-access article distributed under the terms of the Creative Commons Attribution License (CC BY). The use, distribution or reproduction in other forums is permitted, provided the original author(s) and the copyright owner(s) are credited and that the original publication in this journal is cited, in accordance with accepted academic practice. No use, distribution or reproduction is permitted which does not comply with these terms.
*Correspondence: Tegene Atamenta tegene2013@gmail.com
Specialty Section: This article was submitted to Infectious Disease Epidemiology, a section of the journal Frontiers in Epidemiology