Malthusian Factors as Proximal Drivers of Human Population Crisis at Sub-Saharan Africa
- 1Population Dynamic Lab, Center of Applied Ecology and Sustainability, Pontificia Universidad Católica de Chile, Santiago, Chile
- 2Instituto de Ecología y Biodiversidad, Pontificia Universidad Católica de Chile, Santiago, Chile
There is a growing interest and concern for understanding the interaction among human population growth and the sustainability of natural resources. In fact, many agrarian societies experienced an increasing frequency of wars, famines and epidemics during the periods of resource depletion. People from Sub-Saharan Africa (SSA) have suffered the demographic consequences of famines, civil wars and political instabilities during the last 50 years. Almost half of the countries of SSA have undergone some form of demographic crisis over the past 50 years. Our analysis indicate that despite that environmental conditions were positively correlated with crop production across SSA, Malthusian factors correlated inversely with cultivation intensity, which in turn translated into a higher magnitude of depopulation suffered during the past 50 years. In this paper, we provide empirical evidence that population collapses in SSA during the last 50 years have been multifactorial, although more closely associated with “Malthusian” factors as proximal drivers. Other proximal drivers such as economic indicators, political stability and environmental determinants did not explain as much variance as Malthusian forces, suggesting that explanations of collapse magnitude in SSA are embedded in a complex multi-causal chain, in which demographic factors may play a modulating role yet to be explored in more depth.
Introduction
There is a growing interest and concern for understanding the interaction among human population growth and the sustainability of natural resources (Motesharrei et al., 2014). In fact, some authors have raised the possibility of future resource depletion and depopulation in modern societies (Butler, 2000, 2004), while others think that human societies can achieve sustainable development by balancing economic and population growth (Galor and Weill, 2000). Several recent studies have shown how different pre-industrial societies have collapsed during periods of climate change, resource scarcity, and population growth (Zhang et al., 2007, 2011; Turchin and Nefedov, 2009; Lima, 2014). In fact, many agrarian societies experienced an increasing frequency of wars, famines and epidemics during the periods of climatic change and resource depletion (McMichael, 2012).
Human population growth has experienced an unprecedented acceleration since the industrial revolution (Lima and Berryman, 2011). Despite improvements in health, hygiene and nutrition in Western industrial societies, population collapses events associated with famine and drought have continued to occur especially in the second half of the nineteenth century and the first half of the twentieth century (McMichael, 2012). For example, at global scale, despite of the positive trend in per capita growth rates, the rate change of growth rates revealed a strong slowdown rate of population growth during the period 1920–1940 (Lima and Berryman, 2011), which can be related to the great economic depression. However, the causes of population collapses occurred during the second half of the twentieth century are much less studied.
People from Sub-Saharan Africa (SSA) have suffered the demographic consequences of famines, civil wars and political instabilities during the last 50 years (Elbadawi and Sambanis, 2000, 2002). Deciphering the causes of these conflicts has been an important area of research in socio-economic and demographic sciences (Collier and Hoeffler, 1998, 2004; Gyimah-Brempong and Corley, 2005). The dominant view in the existing literature is that economic conditions are the main determinants of civil wars and conflicts in less developed countries (Collier and Hoeffler, 1998; Elbadawi and Sambanis, 2000, 2002; Goldstone et al., 2010). In addition, recent studies have explored the role of ethnic (Easterly and Levine, 1997; Elbadawi and Sambanis, 2002; Posner, 2004) and climatic (Miguel et al., 2004; Burke et al., 2009; O'Loughlin et al., 2012; Scheffran et al., 2012; Hsiang et al., 2013) variables as potential explanatory factors of the high incidence of civil wars in SSA. However, most of these studies have used the frequency/intensity of civil conflicts or wars as response variables. To date, no previous study has addressed this problem from a demographic perspective as an attempt to address the population consequences.
Several studies have proposed that population pressure (Malthus, 1798) could be an important factor for determining some of the population crisis observed in SSA for the past 50 years (Homer-Dixon, 1994; Andre and Platteau, 1998; Butler, 2000, 2004; Diamond, 2005; Brückner, 2010). In fact, land-related conflicts in SSA appear to be an important factor triggering civil violence and political instability (Andre and Platteau, 1998; Deininger and Castagnini, 2006). In this paper, we provide empirical evidence that the magnitude of the population collapses in SSA during the last 50 years have been closely associated with Malthusian factors. Moreover, we used a well-established theoretical framework derived from population dynamic theory (Royama, 1992; Berryman, 1999; Turchin, 2003; Lima and Berryman, 2011) for analyzing human population collapses in SSA. In sum, we aim at reconcile competing hypotheses that have been proposed as explanations of demographic collapses organizing several indicator variables of socio-political, economic, environmental and land use drivers into a unified causal framework by means of latent variable path modeling.
Materials and Methods
Data
Data from demographic, economic, land use, social, and political variables from 41 countries of SSA were selected using the World Bank data base, 20 of which showed signatures of collapse according to criteria described in Section Demographic Collapses. For each variable we estimated the median values for the period 2000–2010 in order to avoid long term trends in these data. All the variables used in this study are shown in detail in Table 1. Further documentation and research is available at www.worldbank.org/wbi/governance. Other variables and derived quantities were also modeled and are summarized in Table 1.
Demographic Collapses
The per capita growth rate can be easily estimated from consecutive census of population data as: Rt = loge (Nt/Nt−d), where N is population size and d is the time period between censuses (10 years). The plot of annual per capita growth rates against years for each SSA country was used to determine the periods with sudden population decreases [Supporting Information (SI) Figure 1]. The demographic magnitude of these events (hereafter collapses or crisis) can be estimated as the difference between the R-values at the beginning and the end of the collapse (SI Figure 1). In addition, we also estimated the rate change of per capita population growth (second derivative). This measure could be important to detect positive/negative accelerations in the population growth rate (Ginzburg and Colyvan, 2004). The rate change of per capita population growth can be easily estimated from data as:
The presence of a population collapse was determined when the value of RGt is negative over a period of 10 years, but preceded and followed by positive values. The extent of collapse can be estimated by the value of the negative RGt observed over a period of 10 years. The absolute value of this quantity was modeled to account for the intensity or magnitude of observed demographic collapses in SSA.
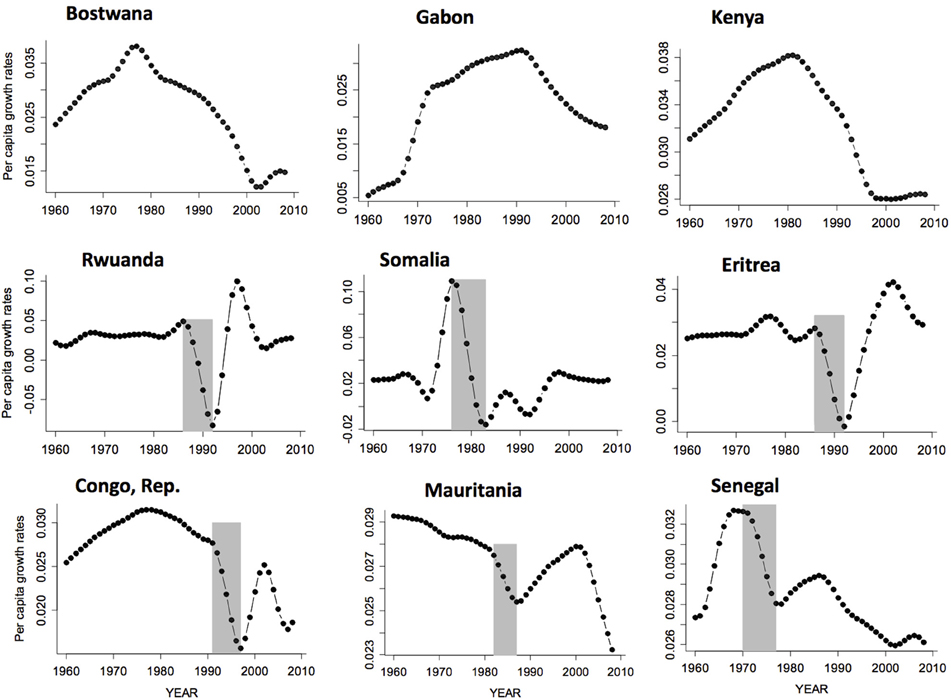
Figure 1. Annual estimates of per capita growth rates [R] of the African human population during the period 1960–2010. First row: examples of Sub-Saharan Africa (SSA) countries showing no population consequences of civil wars; second row, SSA countries exhibiting strong population effects; third row, SSA countries showing mild population effects of civil warfare. Gray stripes represent phases with sudden decreases in per capita growth rates: the magnitude of which is estimated by the difference between the initial and final value of these periods.
Statistical Analyses
Data was analyzed using the Structural Equation Modeling framework. Structural Equation Models (SEM) are a confirmatory scheme designed to estimate causal effects between two or more variables arranged in direct and indirect relationships according to a predefined theoretical model (Kline, 2010). The idea behind SEM is that complexity inside a system can be studied by means of a causality network among latent concepts, known as Latent Variables (LV), which are measured in turn using observed indicators, known as Manifest or Indicator Variables (MV). A LV represents a concept that is hard to capture using a single observable, either because there are no instrumental means for that purpose, or because the concept itself is complex and multidimensional, needing a combination of MV to describe it empirically.
PLS Path Modeling (PLSPM) is a component-based estimation method that defines an inner (or structural) model of regressions among LV, and an outer model for relating the MV and LV (Tenenhaus et al., 2005). The contribution of each MV to its corresponding LV is achieved through the linear combination of loadings obtained as the outcome of a multivariate ordination procedure. Therefore, changes in one MV imply changes in the other MV forming the LV. PLSPM is an iterative algorithm that first solves the outer model and then estimates the path coefficients in the inner model. This technique is considered a soft modeling approach because no strong assumptions are made regarding distribution of variables, sample size and the measurement scale used. These properties make PLSPM an interesting methodology in situations where those assumptions are not tenable or difficult to meet.
Latent Variables
Variables described in Table 1 were considered as reflective indicator variables of latent constructs. This framework allows reducing a set of explanatory variables into a smaller set of composite variables by exploiting their correlation (Kline, 2010; Grace et al., 2012). This dimension-reduction procedure is achieved though linear combination in a manner comparable to principal component analysis. The advantage of providing a reduced set of predictors relies in that more attention can be paid to structural relations that are known to be important, but cannot be fully accounted by observable variables in isolation. In addition, statistical problems where multiple predictors are available, but sampling size is small, can be approached using the so-called soft-modeling approach by combining LV with confirmatory factor analysis (Wold, 1982; Russo, 2009; Kline, 2010; Zhang et al., 2011). During data analysis, several diagnostics such as cross-loading allow to assess whether or not a given indicator variable perform better in its current latent, or if global fit improves after relocating the indicator in another candidate latent (Tenenhaus et al., 2005). Therefore, it must be noted that LV defined in Table 1 are in fact the final result of an iterative process that started with a theoretically meaningful grouping of manifest variables that were subsequently altered using outer model checking diagnostics.
Partial Least Squared Path Modeling
Reducing the raw database to a smaller set of highly correlated indices in the form of latent constructs allow us to focus attention in the structural model (a.k.a. inner model) without sacrificing information by excluding informative variables in a model selection process through isolated pairwise regression analysis.
LV were arranged in a confirmatory path aimed at reconcile the effects of environmental, economic, political, and demographic drivers as proximal causes of collapse. Distal effects of other drivers were also included. Despite the confirmatory nature of the model, some re-wiring of causal paths was required to achieve better goodness of fit of the global model as well as the individual paths. In tuning the outer and inner model, care was taken to maintain the informative value of the constructs while improving fitting performance. The final model and the main hypotheses assessed are listed in Table 2. Inner and outer model diagnostics included uni-dimensionality metrics (i.e., composite reliability, Cronbach alpha, see Supplementary Table S1), cross-loadings, path coefficients of determination, and global goodness of fit (Wold, 1982; Tenenhaus et al., 2005). Bootstrap was used to test the statistical significance of beta coefficients of the inner (structural) model, as well as loadings of the outer (measurement) model. Analyses were done using SmartPLS (Hair et al., 2014). Before analysis, data was z-standardized.
Results
The dynamics of the SSA population during the last 50 years appears to be characterized by sudden changes in the per capita growth rates in 20 of the 41 countries analyzed (Figure S1), with some extreme examples of population collapses, such as in Rwanda and Somalia (Figure 1). From the 41 countries analyzed in SSA, only 20 of them have experienced demographic collapses. The magnitude of the reduction in per capita growth rates estimated by the rate of change of per capita population growth rate showed a median value of −0.091, with some countries having the steepest decreases, such as Somalia (−0.27) and Rwanda (−0.21).
The global goodness of fit of structural model shown in Figure 2 was 0.56, a notably high value given the complexity of the model and small sample size. Four out of nine regression paths explained a significant amount of variability, forming a connected chain of correlation from environmental exogenous determinant, trough cropland drivers, to Malthusian forces and finally the magnitude of population collapse. Proximal hypothesis of collapse favored Malthusian factors as the only significant latent predictor above environmental, agricultural, political and economic drivers (Table 2).
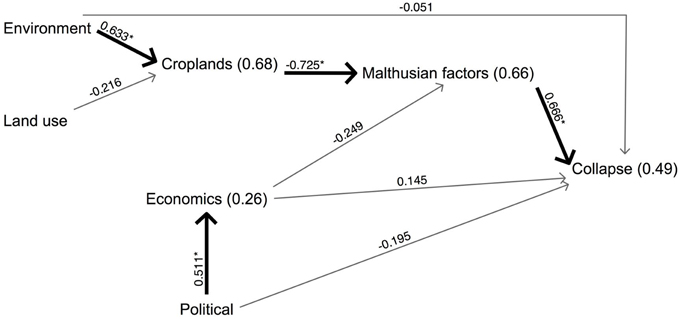
Figure 2. Structural model path diagram. Beta coefficients are shown above arrows with significant paths marked with * symbols. Numbers in parenthesis are coefficients of determination.
Discussion
Malthusian forces appear to be the key factor for determining the magnitude of population crisis suffered in the SSA region during the past 50 years. For example, countries like Burundi, Rwanda and Somalia characterized by high population densities (total or rural) have suffered the largest population collapses. While some authors have noted this causal link before (Homer-Dixon, 1994; Andre and Platteau, 1998; Butler, 2000, 2004; Diamond, 2005; Brückner, 2010; O'Loughlin et al., 2012), the role of Malthusian factors in determining the magnitude of population crisis in the region have been questioned (Urdal, 2005). Our findings on the effects of proximal variables on the magnitude of population crisis in Africa, reveal that environmental, economic, and political factors contribute very little in determining the severity or magnitude of the population collapses in SSA. Some studies have established that high population densities and an economy based on agrarian products heavily dependent on climatic variability seem to be associated with a high incidence of civil wars at SSA region (Miguel et al., 2004; Burke et al., 2009). However, at the cross-country level, the magnitude of population collapses seem to be more correlated with Malthusian drivers, which is consistent with previous studies that have proposed that high population density is an essential factor to trigger violent conflict and political instability in SSA (Andre and Platteau, 1998; Deininger and Castagnini, 2006).
In general, the Malthusian factors have been rarely mentioned when analyzing the causes of civil wars and population collapses experienced by many countries in SSA in the last 50 years (Andre and Platteau, 1998; Butler, 2004; Diamond, 2005). Several previous studies have established the role of economic (Collier and Hoeffler, 1998; Elbadawi and Sambanis, 2000, 2002; Goldstone et al., 2010), socio-political and ethnic (Easterly and Levine, 1997; Anyanwu, 2002), and even climatic factors (Miguel et al., 2004; Burke et al., 2009; O'Loughlin et al., 2012; Scheffran et al., 2012; Hsiang et al., 2013) as potential explanatory variables for the high incidence of civil wars in SSA. However, no previous study has addressed the demographic effects of such conflicts as a response variable. Here, the role of Malthusian forces is deduced by using an explicit demographic variable representing the magnitude of a population collapse. In fact, our SEM results suggest a rather complex web of “cascading effects” of environmental drivers to crop production to malthus factors influencing indirectly the magnitude of population crisis. In fact, SSA countries with the highest rural density population appear to be in higher risk of suffering a population collapse associated with civil conflicts. The classical example is Rwanda, with 645 rural population per sq. km of arable land, and other examples experiencing strong demographic collapses are Burundi (660 per sq. km of arable land), Eritrea (569 per sq. km of arable land), and Somalia (474 per sq. km of arable land). Despite that most of the countries of this region have undergone civil armed conflicts during the last 50 years, only those countries characterized by a complex intertwining of environmental, crop and Malthusian drivers have been severely depopulated by massive genocides and migrations.
The population dynamic pattern of SSA during the second half of twentieth century was characterized by a positive relationship between per capita growth rates and population size until the mid-eighties, when it peaked; since then population growth rates have decreased with population size (Lima and Berryman, 2011). The accelerated phase of population growth rates is a human demographic signature of the industrial revolution: modern developed countries peaked during the second half of the nineteenth century [Europe, USA], Japan during 1920s, Latin America during 1950s and China during 1960s (Lima and Berryman, 2011). In the poorest regions of the world, such as SSA, this demographic pattern of population growth appears to be caused by the delayed global consequences of the industrial revolution, and not due to the improvements in local economy and socio-political development (Botha, 2010). This decoupling between demography and economic development at SSA could explain the high frequency of civil warfare and population collapses observed during the past decades.
Conclusions
During the past 50 years a great number of countries in SSA have suffered the tragic consequences of armed conflicts and civil wars, which seem to have a complex multi-causal origin (Butzer, 2012). However, only in some of these countries such conflicts have generated severe depopulation due to genocide and mass migration. In this study, it is suggested that population collapses appears to be trigger by the direct (proximal) effects of Malthusian factors associated with indirect (distal) drivers such as environmental and cropland production. The results from SSA n countries support the idea that the dramatic population collapses observed in this region during the last decades appear to have a proximal Malthusian cause above economic, socio-political or environmental drivers.
Conflict of Interest Statement
The authors declare that the research was conducted in the absence of any commercial or financial relationships that could be construed as a potential conflict of interest.
Acknowledgments
We thank F. Jaksic and A. Farias for discussions and comments on human population dynamics. ML acknowledges financial support from Fondo Basal-CONICYT grant FB-0002 and is grateful to LINCGlobal (Laboratorio Internacional en Cambio Global) for their support. SA is funded by ICM-MINECON, P05-002 IEB.
Supplementary Material
The Supplementary Material for this article can be found online at: https://www.frontiersin.org/article/10.3389/fevo.2015.00130
References
Andre, C., and Platteau, J. (1998). Land relations under unbearable stress: rwanda caught in the Malthusian trap. J. Econ. Behav. Org. 34, 1–47. doi: 10.1016/S0167-2681(97)00045-0
Anyanwu, J. C. (2002). Economic and political causes of civil wars in africa: some econometric results. Afr. Dev. Bank. Econ. Res. Pap. 73, 1–16.
Berryman, A. A. (1999). Principles of Population Dynamics and Their Application. London: Stanley Thornes. doi: 10.1038/21666
Botha, I. (2010). A comparative analysis of the synchronization of business cycles for developed and developing economies with the world business cycle. S. Afr. J. Econ. 78, 192–207. doi: 10.1111/j.1813-6982.2010.01241.x
Brückner, M. (2010). Population size and civil conflict risk: is there a causal link? Econ. J. 120, 535–550. doi: 10.1111/j.1468-0297.2010.02352.x
Burke, M. B., Miguel, E., Satyanath, S., Dykema, J. A., and Lobell, D. B. (2009). Warming increases the risk of civil war in Africa. Proc. Natl. Acad. Sci. U.S.A. 106, 20670–20674. doi: 10.1073/pnas.0907998106
Butler, C. D. (2000). Entrapment: global ecological and/or local demographic? Reflections upon reading the BMJ's “six billion day” special issue. Ecosys. Health 6, 171–180. doi: 10.1046/j.1526-0992.2000.006003171.x
Butler, C. D. (2004). Human carrying capacity and human health. PLoS Med. 1:e55. doi: 10.1371/journal.pmed.0010055
Butzer, K. W. (2012). Collapse, environment, and society. Proc. Natl. Acad. Sci. U.S.A. 109, 3632–3639. doi: 10.1073/pnas.1114845109
Collier, P., and Hoeffler, A. (1998). On economic causes of civil war. Oxf. Econ. Pap. 50, 563–573. doi: 10.1093/oep/50.4.563
Collier, P., and Hoeffler, A. (2004). Greed and Grievance in Civil War. Oxford, UK: Oxford University Press. doi: 10.1093/oep/gpf064
Deininger, K., and Castagnini, R. (2006). Incidence and impact of land conflict in Uganda. J. Econ. Behav. Org. 60, 321–345 doi: 10.1016/j.jebo.2004.04.008
Easterly, W., and Levine, R. (1997). Africa's growth tragedy: policies and ethnic divisions. Q. J. Econ. 112, 1203–1250. doi: 10.1162/003355300555466
Elbadawi, I., and Sambanis, N. (2000). Why are there so many civil wars in Africa? understanding and preventing violent conflict. J. Afr. Econ. 3, 244–269. doi: 10.1093/jae/9.3.244
Elbadawi, I., and Sambanis, N. (2002). How much war will we see? explaining the prevalence of civil war. J. Confl. Res. 46, 307–344. doi: 10.1177/0022002702046003001
Galor, O., and Weill, D. N. (2000). Population, technology, and growth: from Malthusian stagnation to the demographic transition and beyond. Pop. Tech. Growth 90, 806–828. doi: 10.1257/aer.90.4.806
Ginzburg, L. R., and Colyvan, M. (2004). Ecological Orbits: How Planets Move and Populations Grow. New York, NY: Oxford University Press.
Goldstone, J. A., Bates, R. H., Epstein, D. L., Gurr, T. R., Lustik, M. B., Marshall, M. G., et al. (2010). A global model for forecasting political instability. Am. J. Pol. Sci. 54, 190–208. doi: 10.1111/j.1540-5907.2009.00426.x
Grace, J. B., Schoolmaster, D. R., Guntensperger, G. R., Little, A. M., Mitchell, B. R., Miller, K. M., et al. (2012). Guidelines for a graph-theoretic implementation of structural equation modeling. Ecosphere 3, 73. doi: 10.1890/ES12-00048.1
Gyimah-Brempong, K., and Corley, M. E. (2005). Civil wars and economic growth in Sub-Saharan Africa. J. Afr. Econ. 14, 270–311. doi: 10.1093/jae/eji004
Hair, J. F., Hult, G. T. M., Ringle, C. M., and Sarstedt, M. (2014). A Primer on Partial Least Squares Structural Equation Modeling. Thousand Oaks, CA: Sage.
Homer-Dixon, T. (1994). Environmental scarcities and violence conflict: evidence from cases. Inter. Sec. 19, 5–40. doi: 10.2307/2539147
Hsiang, S. M., Burke, M., and Miguel, E. (2013). Quantifying the influence of climate on human conflict. Science 341:123567. doi: 10.1126/science.1235367
Kline, R. B. (2010). Principles and Practice of Structural Equation Modeling, 3rd Edn. New York, NY: Guilford Press.
Lima, M. (2014). Climate change and the population collapse during the “Great Famine” in pre-industrial Europe. Ecol. Evol. 4, 284–291. doi: 10.1002/ece3.936
Lima, M., and Berryman, A. A. (2011). Positive and negative feedbacks in human population dynamics: future equilibrium or collapse? Oikos 120, 1301–1131. doi: 10.1111/j.1600-0706.2010.19112.x
McMichael, A. J. (2012). Insights from past millennia into climatic impacts on human health and survival. Proc. Natl. Acad. Sci. U.S.A. 109, 4730–4737. doi: 10.1073/pnas.1120177109
Miguel, E., Satyanath, S., and Sergenti, E. (2004). Economic shocks and civil conflict: an instrumental variables approach. J. Pol. Econ. 112, 725–753. doi: 10.1086/421174
Motesharrei, S., Rivas, J., and Kalnay, E. (2014). Human and nature dynamics (HANDY): modeling inequality and use of resources in the collapse or sustainability of societies. Ecol. Econ. 101, 90–102 doi: 10.1016/j.ecolecon.2014.02.014
O'Loughlin, J., Witmer, F. D. W., Linke, A. M., Laing, A., Gettelman, A., and Dudhia, J. (2012). Climate variability and conflict risk in East Africa, 1990-2009. Proc. Natl. Acad. Sci. U.S.A. 109, 18344–18349. doi: 10.1073/pnas.1205130109
Posner, D. N. (2004). Measuring ethnic fractionalization in Africa. Am. J. Pol. Sci. 48, 849–863. doi: 10.1111/j.0092-5853.2004.00105.x
Royama, T. (1992). Analytical Population Dynamics. London: Chapman and Hall. doi: 10.1007/978-94-011-2916-9
Russo, F. (2009). Causality and Causal Modelling in the Social Sciences: Measuring Variations. Dordrecht: Springer. doi: 10.1007/978-1-4020-8817-9
Scheffran, J., Brzoska, M., Kominek, J., Link, M., and Schilling, J. (2012). Climate change and violent conflict. Science 336, 869–871. doi: 10.1126/science.1221339
Tenenhaus, M., Esposito Vinzi, V., Chatelin, Y. M., and Lauro, C. (2005). PLS path modeling. Comput. Stat. Data Anal. 48, 159–205. doi: 10.1016/j.csda.2004.03.005
Turchin, P. (2003). Complex Population Dynamics: a Theoretical/Empirical Synthesis. Princeton, NJ: Princeton University Press. doi: 10.1126/science.1235367
Turchin, P., and Nefedov, S. (2009). Secular Cycles. Princeton, NJ: Princeton University Press. doi: 10.1515/9781400830688
Urdal, H. (2005). People vs. Malthus: population pressures, environmental degradation and armed conflict revisited. J. Peace Res. 42, 417–434. doi: 10.1177/0022343305054089
Wold, H. (1982). “Soft modeling: the basic design and some extensions,” in Systems Under Indirect Observations: Part II, eds K. G. Jöreskog and H. Wold (Amsterdam: North-Hollands), 1–54.
Zhang, D. D., Brecke, P., Lee, H. F., He, Y.-Q., and Zhang, J. (2007). Global climate change, war, and population decline in recent human history. Proc. Natl. Acad. Sci. U.S.A. 104, 19214–19219. doi: 10.1073/pnas.0703073104
Keywords: ecology, economy, demography, human collapses, Malthus, Africa, causal model, partial least squares path modeling
Citation: Lima M and Abades S (2015) Malthusian Factors as Proximal Drivers of Human Population Crisis at Sub-Saharan Africa. Front. Ecol. Evol. 3:130. doi: 10.3389/fevo.2015.00130
Received: 02 February 2015; Accepted: 04 November 2015;
Published: 20 November 2015.
Edited by:
Nikolaos Papadopoulos, University of Thessaly, GreeceReviewed by:
Meritxell Genovart, Institut Mediterrani Estudis Avançats (CSIC-UIB), SpainPablo Almaraz, Universidad de Cadiz, Spain
Copyright © 2015 Lima and Abades. This is an open-access article distributed under the terms of the Creative Commons Attribution License (CC BY). The use, distribution or reproduction in other forums is permitted, provided the original author(s) or licensor are credited and that the original publication in this journal is cited, in accordance with accepted academic practice. No use, distribution or reproduction is permitted which does not comply with these terms.
*Correspondence: Mauricio Lima, mlima@bio.puc.cl