Divergent Responses of Floral Traits of Lonicera nervosa to Altitudinal Gradients at the Eastern Margin of Hengduan Mountains
- 1Chengdu Institute of Biology, Chinese Academy of Sciences, Chengdu, China
- 2University of Chinese Academy of Sciences, Beijing, China
- 3College of Resources and Environment, Aba Teachers University, Aba, China
- 4Mangkang Ecological Station, Tibet Ecological Safety Monitor Network, Changdu, China
- 5International Centre for Integrated Mountain Development, Lalitpur, Nepal
- 6Xinjiang Institute of Ecology and Geography, Chinese Academy of Sciences, Ürümqi, China
- 7Chengdu Botanical Garden, Chengdu, China
Understanding phenotypic responses is crucial for predicting and managing the effects of environmental change on native species. Color and display size are typically used to evaluate the utilization value of ornamental plants, which are also important ornamental characters of Lonicera nervosa Maxim. (L. nervosa). However, there is limited documentation of its floral environmental adaptation. The environmental conditions for the development of an organism changes with altitudinal variation. The aim of this research was to find flower trait variability maintenance and the tradeoff among the organs in five different populations of L. nervosa growing at distinct altitudes. We investigated the distribution patterns of floral color, floral display, and biomass tradeoff along a 700-m altitude gradient from 2,950 to 3,650 m. One-way ANOVA analysis was performed to assess the variability of flower traits and floral color across different altitudes. Moreover, correlations and tradeoffs between flowers and vegetative organs were also observed at different altitude ranges. The results indicated that L. nervosa flowers had a strong adaptability along the elevation and divergent altitude-range-specific patterns, which was divided by an altitude breakpoint at around 3,300 m. Below 3,300 m, petal lightness (petal L) decreased, but total floral display area (TFDA), individual floral dry mass (IFDM), and total floral dry mass (TFDM) increased with an increase in altitude. Whereas, above 3,300 m no significant difference was observed in petal L, TFDA, IFDM, and TFDM decreased slightly with an increase in altitude. The responsibility for the selection on floral color at a lower altitude was stronger than that at a higher altitude, while the selection agents on floral biomass had significant effects within the entire altitude range. However, the effects on floral biomass were opposite on both sides of 3,300 m. Thus, floral trait and floral color can be useful indicators for the domestication of horticultural plants and help to evaluate and initiate management and conservation actions.
Introduction
Phenotype or trait is the morphological, anatomical, physiological, biochemical, and phenological characteristic of an organism resulting from both genetic and environmental influences (Kattge et al., 2020). Elevation is a multiple-gradient factor affecting atmospheric processes, such as precipitation, temperature, solar radiation, etc. (Körner, 2007), which can lead to geographic variation in traits. Plant species can adjust to these diverse conditions through natural selection or migrate to follow conditions to which they are adapted; these options are not mutually exclusive (Nicotra et al., 2010). The floral part is an important reproductive organ which is strongly influenced by the environment. It attracts wide attention for its ornamental value in gardening or landscaping. Adaptation to environmental change is an important and nearly universal aspect of the biotic response to climate change (Davis et al., 2005). The mountainous region has large altitudinal gradients and provides “natural laboratories” to study the evolutionary and adaptive response of plant species to changes in the environment (Körner, 2003).
Flower color shows both a strong genetic basis and a sharp geographic transition (Streisfeld and Kohn, 2005), and both biotic and abiotic agents of selection may affect floral traits (Rodríguez-Castañeda et al., 2020). However, the intensity of different biotic and abiotic interactions varies spatially, resulting in divergent selection along with maintenance of the variability of floral traits that influence these interactions (Mitchell-Olds et al., 2007; Ågren et al., 2013; Vaidya et al., 2018). Conventional wisdom is that a large proportion of these transitions reflect adaptation to novel pollinator regimes (Darwin, 1862) and is supported as one of the mainstream views until now (Fenster et al., 2004; Souto-Vilarós et al., 2018; Ramos and Schiestl, 2019). Evidence indicates that due consideration is given to the hypothesis that interactions with pollinators has driven the evolution of flower color in many (if not all) species (Rausher, 2008). The petal color could be a sign of reward for pollinators (McCall and Primack, 1992; Bauer et al., 2017; Deng et al., 2017), affecting the flower visitation behavior of pollinators (Campbell et al., 2010), and can reflect pigment deposition by calculating the petal color (He et al., 2011; Del Valle et al., 2015). However, recent research suggests that additional insights into the non-pollinator agents of selection should be addressed, which can act on the pleiotropic effects of flower color genes (Rausher, 2008; Koski et al., 2020). As altitude increases, ultraviolet radiations increase, and plants precipitate through the pigments to cope with ultraviolet stress (Koski et al., 2020; Peach et al., 2020). Meanwhile, the variability in floral color is also related to heat absorption, and obtaining and maintaining an optimal flower temperature is often imperative for successful plant reproduction (van der Kooi et al., 2019).
The local flower size adaptations of plants to elevational gradient may be affected by biotic factors, such as pollinator community (Kuriya et al., 2015) or simply by the abiotic selective pressure, regardless of changes in the pollinator community (Bode and Dufresne, 2020). Previous research has shown that some plant species tend to develop larger flowers at a higher altitude (Malo and Baonza, 2002; He et al., 2017; Bode and Dufresne, 2020), while some studies have shown that there is no common regularity in the correlation between floral size and altitude itself (Nagano et al., 2014). When both floral display size and flower color vary within a plant species, each has the potential to play an important role in pollinator attraction and subsequent seed set (Malerba and Nattero, 2011). Larger floral displays or inflorescences with more open flowers usually increase pollinator visitation, and greater visitation can augment pollen receipt and seedset (Cayenne Engel and Irwin, 2003; Karron and Mitchell, 2012; Bauer et al., 2017). In hermaphroditic plants, floral display size can serve as a proxy for pollinator resource availability, and this seems to hold true for both pollen and nectar rewards (Makino and Sakai, 2007; Brunet et al., 2015). In addition, floral shape and size can influence heat accumulation and retention within flowers to affect the flowery behavior of pollinators (van der Kooi et al., 2019), so the variation in abiotic factors along an elevational gradient, such as temperature and illumination intensity will also affect the floral display.
Despite the fact that trait-based approaches can make a significant contribution to understanding the effects of management and changes in the human environment on productivity and general plant performance (Gagliardi et al., 2015), relatively few studies have been applied to identify the floral performance of L. nervosa, not to mention integrating flower display, floral color, and leaf traits for a comprehensive assessment. Using multiple imaging and sensing modalities to evaluate many genetic lines repeatedly is of great value to plant breeding programs (Bai et al., 2018), and the most significant effect of plant color in landscaping is to attract and induce the sight, the contrast, and the difference in color which is the first thing perceived as a recognition characteristic by tourists (Li, 2010). Therefore, the study on floral color and floral display is of great significance to enhance the utilization of plant resources and in providing important guidance for the breeding of ornamental plants. From the lower ecotone to the higher boundary of sub-alpine, i.e., forest line, the belt of dense forests presented different habitats not only due to the abiotic factors but also by biological interactions, in particular, shading, allelopathic effect, or other competitions. Thus, in this study, we aim to raise some hypothesis as follows: (1) Floral traits would present divergent response to altitudinal gradient; the flowers of L. nervosa tend to be darker in color and larger in display with an increase in elevation; and (2) Variation in plant traits shows one break point at the dense forests.
Based on the above-mentioned scientific hypothesis, this paper studies the characteristics of flower color diversity and biomass distribution among organs aimed at exploring the adaptation of flowers and tradeoff mechanism of organs to altitudinal gradient. The study also provides a basis for predicting the adaptive changes in L. nervosa under different climate change scenarios, thus aiding in the identification of the best suitable area for ornamental performance and to provide more material and theoretical basis for resource utilization.
Materials and Methods
Study Sites
The study was conducted at the Bipenggou valley in Lixian County, Sichuan, China (31°13′47”–31°20′17” N, 102°52′19”–102°57′41” E), which belongs to Qionglai Mountains located at the eastern margin of Hengduan Mountains—Southeastern China, as the watershed of Minjiang River and Daduhe River. Bipenggou is part of the Miaro Nature Reserve, the eastern part of Bipenggou is adjacent to Wolong National Nature Reserve, and the southern part is adjacent to Siguniangshan Scenic Area in Xiaojin County, which is also part of the “World Heritage Site of Giant Panda Corridor.” Bipenggou has a complex topography, large relative elevation, and complex climate, which is mainly influenced by three air masses from the Pacific Ocean, Indian Ocean, and Qinghai–Tibet Plateau; the climate is rich in precipitation. The altitude in this region ranges from 2,015 to 5,922 m, with a steep vertical drop that provides several vertical zones over a small area, making it an ideal location to study plant adaptation to a changing environment.
The forest type is coniferous subalpine forest in the range of 2,200–3,600 m in this region. Studies show that in Bipenggou the dominant species between 2,900 and 3,200 m are Picea asperata and Picea likiangensis, under which are deciduous trees, such as Sorbus koehneana, Betula albosinensisi, and Acer laxiflorus, and the main shrubs are Sophora davidii, Berberis veitchii, Daphne odora, Viburnum betulifolium, and Cotoneaster multiflorus; in the range of 3,100–3,300 m, the dominant species are Betula albosinensisi, Abies recurvata, Picea asperata, and Cupressus chengiana; the main shrubs are Rhododendron violaceum, Prunus plusinensis, Rosaceae sp., Rhododendron setosum, Lonicera tangutica, and Sophora davidii; in the range of 3,400–3,600 m, the dominant species are Larix masteriana, Cupressus chengiana, Abies faxoniana, and Abies squamata; the main shrubs are Rhododendron violaceum, Sorbus koehneana, Prunus plusinensis, Berberis veitchii, and Rhododendron cephalanthum. In the range of 2,800–3,600 m, consistent with our study area, the soil is brown loam with pH 5.5–7.0, with high gravel content and shallow soil layer in the area (Li et al., 2010). The study site and overview of the sampling plots are shown in Figure 1.
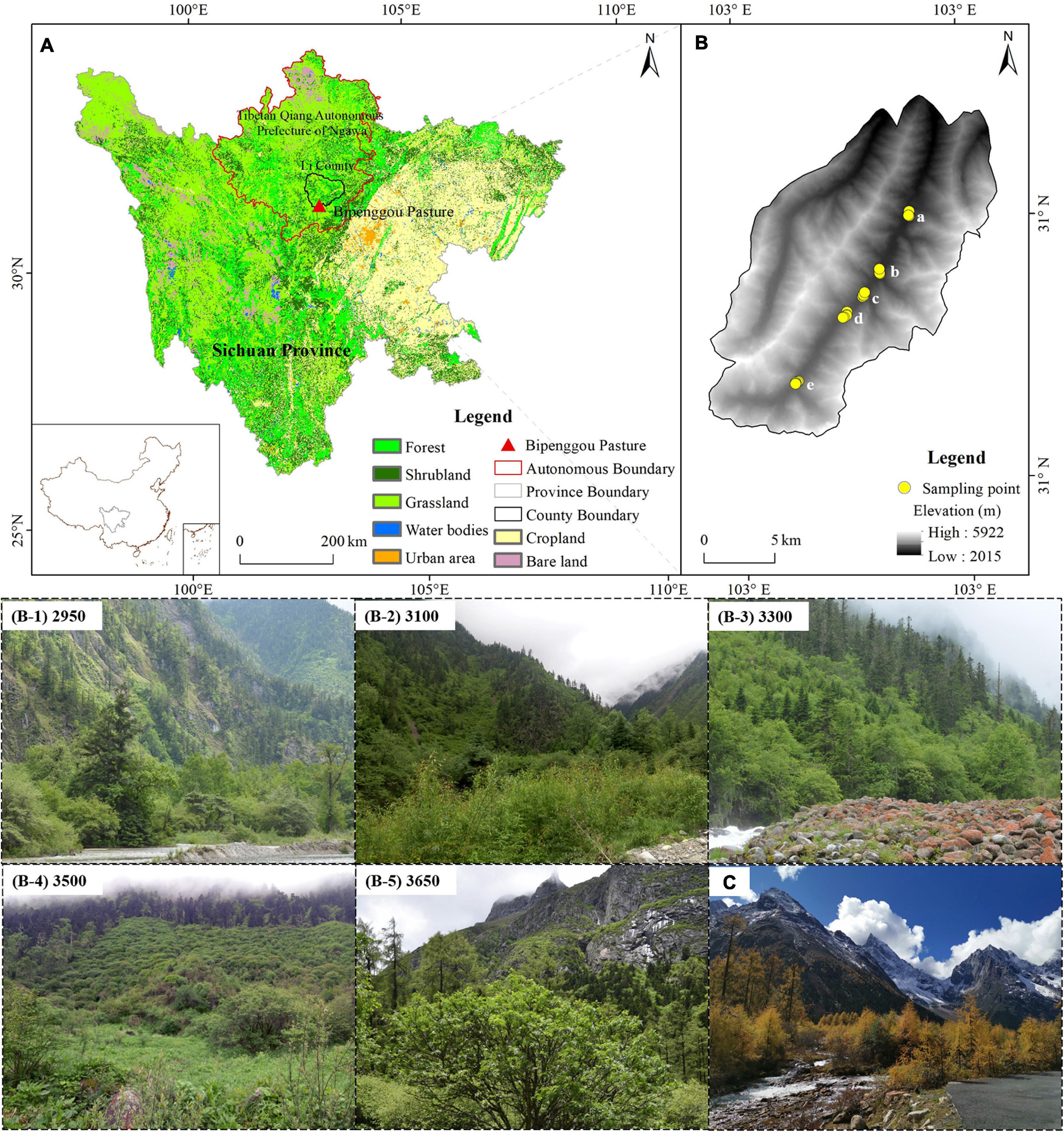
Figure 1. Location of the study site and sampling plots as well as the overview of Bipenggou valley and the biotope of each plot along the valley: (A) location of Bipenggou valley, (B) location and biotope of sampling plots sited at 2,950–3,650 m, and (C) overview of the study site.
Study Species
Lonicera L. is an important genus of the family Caprifoliaceae, which comprises about 180 species (Jachuła et al., 2019) and is a representative genus in the alpine region. Most species have labial crowns and are biflorous, with a pleasant color and a beautiful posture, making it an important genus of plants used for landscaping (Zhang et al., 2004; Liu et al., 2015), and some Lonicera species have been listed among valuable nectar- and pollen-yielding plants (Bożek, 2007). There are 99 species widespread in China (FRPS) which provide a good material for studying the diversity of the plant mating system for its rich flower color and morphological variation; however, most of the studies on Lonicera spp. are focused on the medicinal value of the genus (Shang et al., 2011; Ge et al., 2018). There are very limited studies conducted on the biological characteristics and utilization of the genus in landscaping. For this study, we selected L. nervosa (Figure 2), as it shows characteristics like bright color, luxuriant branches and leaves, beautiful shape, and strong adaptability. The corolla of L. nervosa is mauve or purplish red, and the color varies in different habitats at varying altitudes, which provide abundant material for resource utilization.
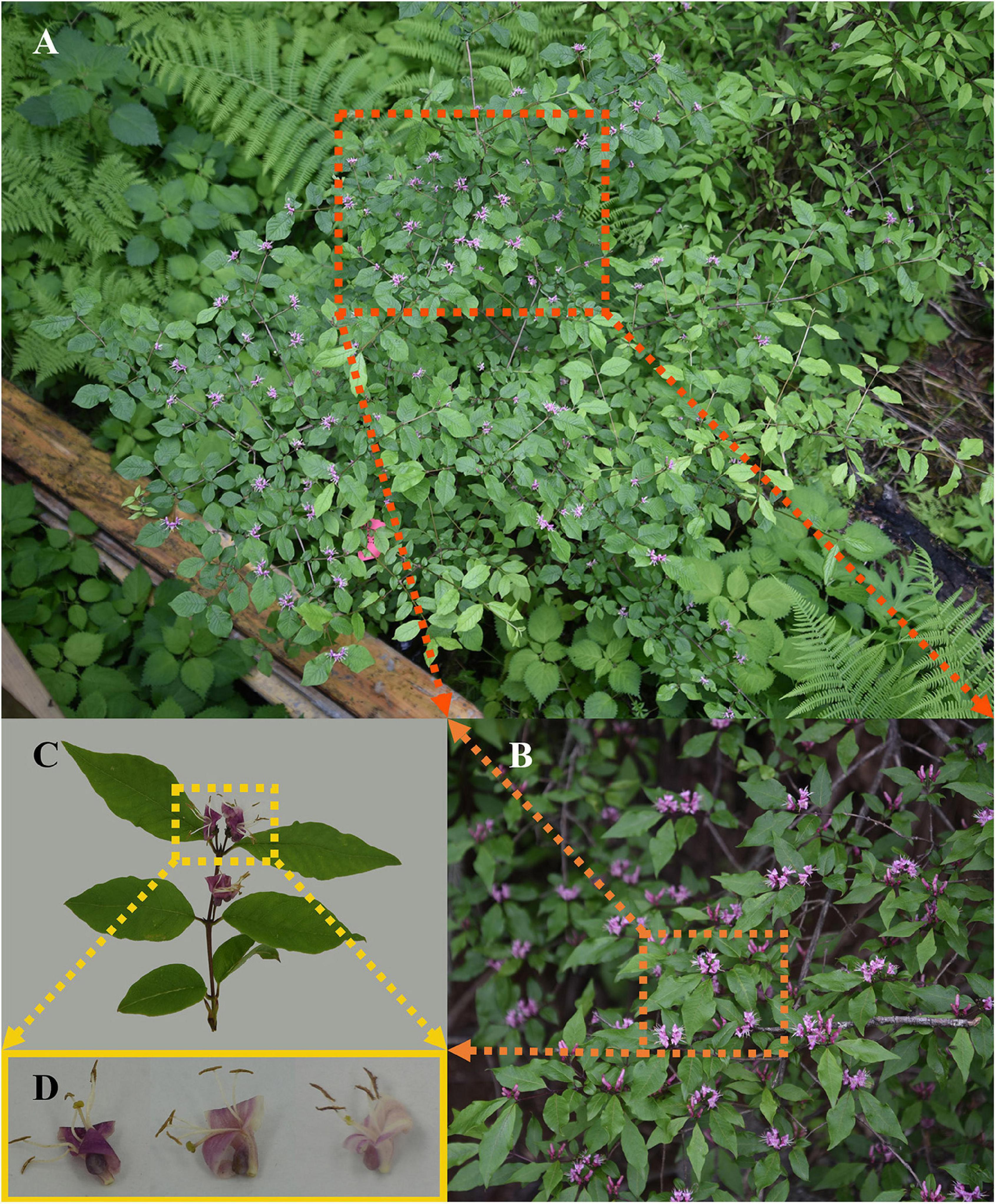
Figure 2. Appearance features of Lonicera nervosa: (A) overview of L. nervosa individual, (B) branches of L. nervosa, (C) details of a twig, and (D) details of flowers with different colors.
The reproduction pattern of L. nervosa is seed breeding (Wang et al., 2000), with limited preliminary cutting experiments on the breeding of L. nervosa (Feng et al., 2015); however, there is no study on the adaptability of L. nervosa. Thus, our study will be of great significance to fill the research gap on the environmental adaptability of L. nervosa and will be useful to the breeding and utilization of the species.
Sampling Methods
We set five sampling points at an interval of 200 m along the altitude from 2,950 m to around 3,650 m (2,945, 3,102, 3,326, 3,505, and 3,657 m). Depending on the distribution of L. nervosa at each point, three to five sampling sites were identified, where the Lonicera species bloom during May–June. During the late spring to the early summer of 2019, at each sampling site, we randomly selected three to five mature L. nervosa individuals at reproductive stages (June 18, 23, 25, and 29 and July 3), 10–20 individuals at each altitude point in total. From each individual, five twigs with flowers in full-bloom phase were randomly selected with no significant loss of leaves or flowers and no controlled variation in flower color or floral size within the twigs. After we cut the twigs from the branches, we quickly put them into Ziplock bags to keep them fresh for further indoor measurements, which included photography and trait measurements.
Photography and Image Analysis
With the advancement in camera technology, the sensor of a digital camera can record highly detailed (high pixel), pure (low ISO and low image noise), and more colorful (deep color depth) images that contain more optical and color information. Meanwhile, it is easy and convenient to operate and calibrate a camera. Thus, with proper use, digital cameras serve as useful and relatively inexpensive tools to capture images and quantify color and pattern (Stevens et al., 2007). In this study, we selected a Nikon D750 DSLR camera (Nikon Corporation, Tokyo, Japan) which had a 35.9 × 24-mm CMOS sensor (6,016 × 4,016 pixels) with 14-bit color depth, 100–25,600 ISO range. It showed full regulation of exposure and metering as recommended for unbiased data acquisition (Stevens et al., 2007; White et al., 2015; Del Valle et al., 2018).
The properties of color images are device dependent, and the display color of the object will vary when images are captured at different color temperatures and camera settings (Hong et al., 2001; Stevens et al., 2007), so we put the flowers in the miniature studio after sampling and captured them with the DSLR to ensure that the color temperature and other settings were consistent. The experimental data for the definition of typical sunlight are derived from the measured sunlight, and their relative spectral power distribution is similar to the measured sunlight. Compared with other standard lighting bodies, the chromaticity points of typical sunlight are more consistent with the actual sunlight. Therefore, the International Commission on Illumination recommended that the measured sunlight should be replaced by typical sunlight D55 (5,503 K), D65 (6,504 K), and D75 (7,504 K) and, as far as possible, typical sunlight D65 (Liang et al., 2013). In terms of the photographing conditions for this study, based on actual observation, we set the white balance of the camera to 5,500 K as the color at the color temperature of 5,500 K is closest to the natural situation. With all these pre-adjusted settings, we fixed the camera on the tripod and equipped it with an NIKKOR 24–85-mm autofocus lens. Then, we manually adjusted the focal length to 35 mm which was suitable to the miniature studio, and the aperture was set to f/8 and the integration time to 1/200 s. We deliberately underexposed all photographs by 0.3 f-stop to prevent color “clipping” or saturation (Stevens et al., 2007; Del Valle et al., 2018). The position between the camera and the platform to place the flowers was fixed. When capturing, we put a flannelette on the platform before we put the flowers on it to prevent shadow and excessive brightness (Kendal et al., 2013). All images were taken in RAW format, which contained unprocessed images that may be linearized using a specialized software. We used the ImageJ software (Schneider et al., 2012) for image processing. We selected the petal center as the region of interest in each image and extracted the mean value of red–green–blue (RGB) channels which was converted into hue–saturation–lightness (HSL) value afterward.
Color parameters can fully express pigment content so that measuring plant color parameters and computing by equation can provide a fast and accurate method to estimate pigment content (Qi et al., 2019). Digital cameras have become a common tool for studying plant colors. With the help of DSLR and image processing tools, Del Valle et al. (2018) built a fast, non-invasive method to estimate anthocyanin pigment concentration in reproductive and vegetative plant tissues, which provided reliable measurement results.
Color information detected by the DSLR was in RGB color space, which was not intuitive, and its process of distinguishing color aberrations was nonlinear (Chien and Tsai, 2014), so RGB color space was not suitable for color recognition. HSL color space, however, was more intuitive and consistent with human visual characteristics (Mizunuma et al., 2014; Lin et al., 2015; Qiao et al., 2016) and is typically used in color recognition applications. In the HSL color mode, H [hue, H∈ (0°, 360°)] was defined to characterize the type of color, and the value of hue could be used to represent the color warmth—the hue value looped from 0° to 360° and defined 0° and 180° as the y axis and 90° and 270° as the x axis; the chromophore was divided into four quadrants. When the hue value was in the first and fourth quadrants, the color was considered as warm color, where 0° (360°) was the warm pole, and when the hue value was in the second and third quadrants, the color was considered cold, where 180° was the cold pole. S [color saturation, S∈ (0, 1)] described the discrimination of color purity in the case of the same hue and lightness (Weeks et al., 1995). L [color lightness, L∈ (0, 1)] spanned the full range of the selected hue from black to white in the HSL color space, so HSL is suitable for observing the color lightness.
We converted the RGB channels into HSL value; the equations are listed below (Joblove and Greenberg, 1978):
Trait Measurements
After capturing the images for color measurement, we quickly measured the fresh mass, and then we scanned the floral display area and leaf area with a scanner. After this, the samples were put into the oven, set at a temperature of 75°C, to dry for 48 h for the dry mass measurement (LY/T 1211-1999). The leaf and flower traits were determined separately both in fresh mass and dry mass. The trait measurements are provided in Table 1—based on the TRY plant trait database (Kattge et al., 2020).
Statistical Analyses
Data was checked for the normality and homogeneity of variance before analyses. Data analyses were performed by using SPSS 18.0 (SPSS Inc., Chicago, IL, United States) and Canoco 5.0 (Ithaca, NY, United States). One-way ANOVA was performed to find the differences in floral traits along the altitudinal gradient, and Duncan’s multiple-range tests were employed to detect significance among means at p ≤ 0.05. Pearson’s correlation coefficients and redundancy analysis (RDA) were calculated to determine the relationship between floral color, floral display, leaf traits, and altitude. Floral water content (FWC) was a strong predictor to imply the variation of the ambient humidity (Roddy et al., 2019), and specific leaf area (SLA) was a common indicator for the intensity of illumination, so we substitute FWC and SLA for ambient humidity and light intensity as environmental factors in calculating the RDA. Simple linear regression was used to examine these relationships; however, sometimes the community species diversity or functional pattern change in response to environmental characteristics was not linear, and sudden changes in ecological processes may occur in the response. A stable response state was present to a certain environmental gradient but, after the environment stress reaches a threshold, another state of response may occur with severe fluctuations. Obviously, different ecological processes were reflected in different gradient ranges on both sides of the critical point of the environment. Thus, piecewise regression was performed to confirm the altitudinal trends of the traits. R studio (R Core Team, 2020) was used to perform the data visualization; we used the R package ggpubr (Kassambara, 2020) to plot boxplot figures and the packages Hmisc (Harrell and Dupont, 2021) and PerformanceAnalytics (Peterson and Peter, 2020) to plot the correlation matrix.
Results
Floral Color Performance
Petal H, petal L, and petal S vary significantly within the entire altitudinal range; however, at altitudes above 3,300 m, there was no significant difference for petal L and petal S (Table 2). The petal H was between 330° and 340° (Figure 1A), and regression analysis showed a linear relationship between petal H and elevation (Figure 3A). Altitude had a significant positive effect on petal S and a significant negative effect on petal L; piecewise regression showed that the gradient change of petal L and petal S had a clear breakpoint at around 3,300 m (Figures 3B,C). Below 3,300 m, petal S increased with an increase in altitude and reached its maximum value at 3,300 m, while a further increase in altitude had no significant effect on petal S. On the contrary, below 3,300 m, petal L showed a significant decreasing trend with an increase in altitude, while at above 3,300 m, no significant difference was observed. Moreover, the correlation analysis showed that both petal H and petal L had a significant negative correlation with altitude (Figures 4A–C)—specifically, the correlation between petal H and altitude was consistent within all altitudes, while the correlation between petal L and altitude was mainly found at a lower altitude (below 3,300 m). Within the entire altitudinal range, there was no significant correlation between petal S and altitude, but a positive significant correlation existed below 3,300 m.
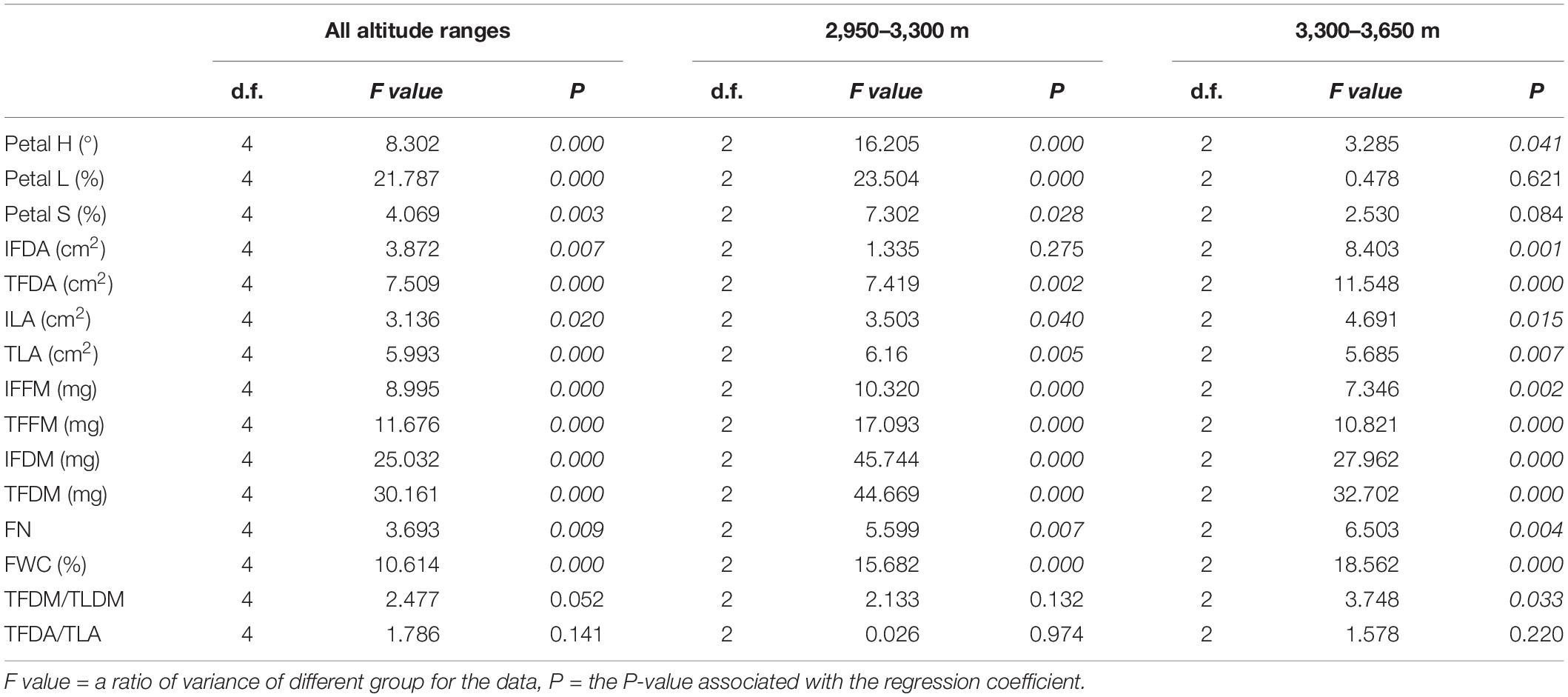
Table 2. Altitudinal effects on floral morphological traits and biomass at different altitude ranges.
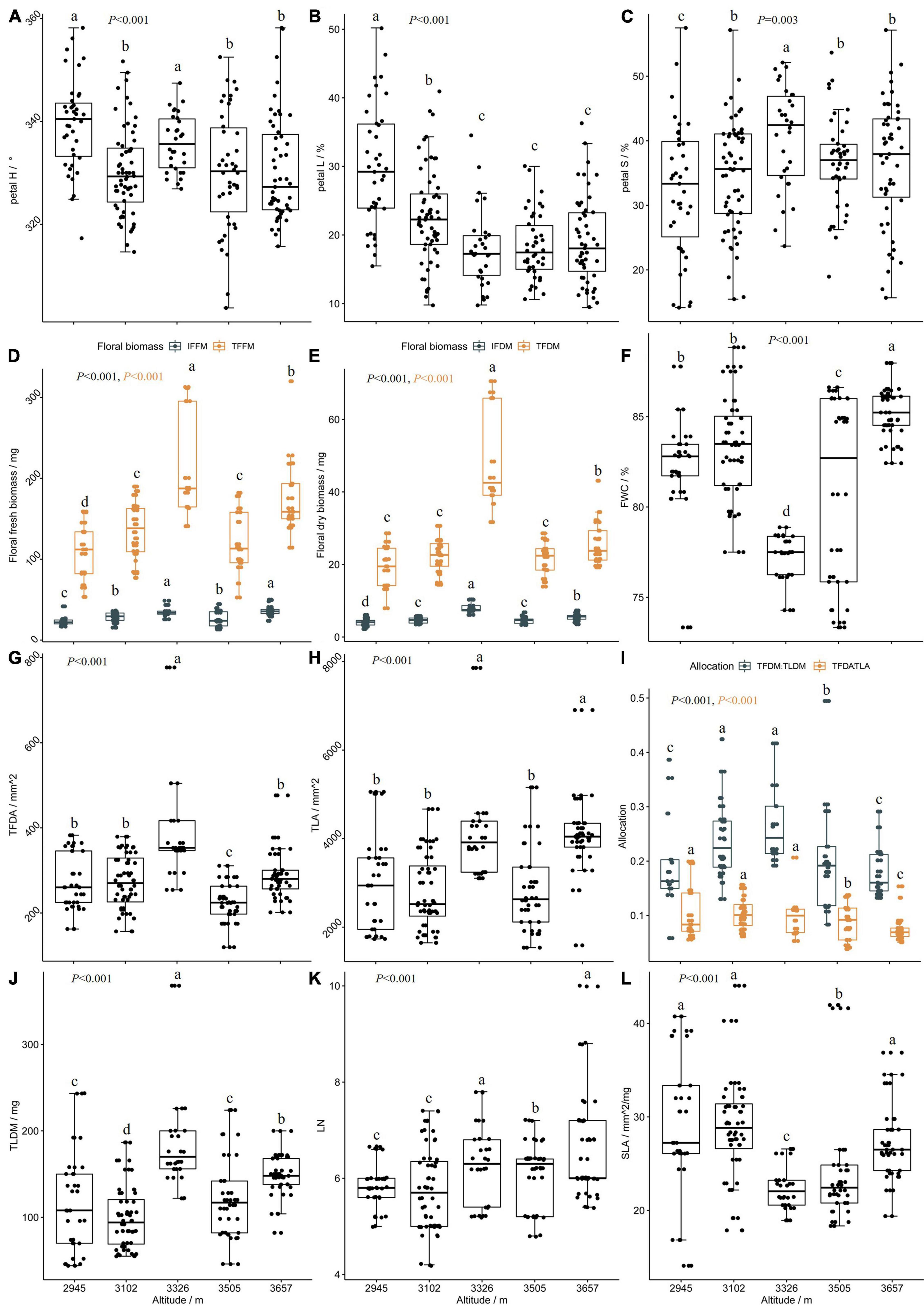
Figure 3. (A–L) The altitude has respective effects on floral color, floral display, leaf traits, and biomass allocation.
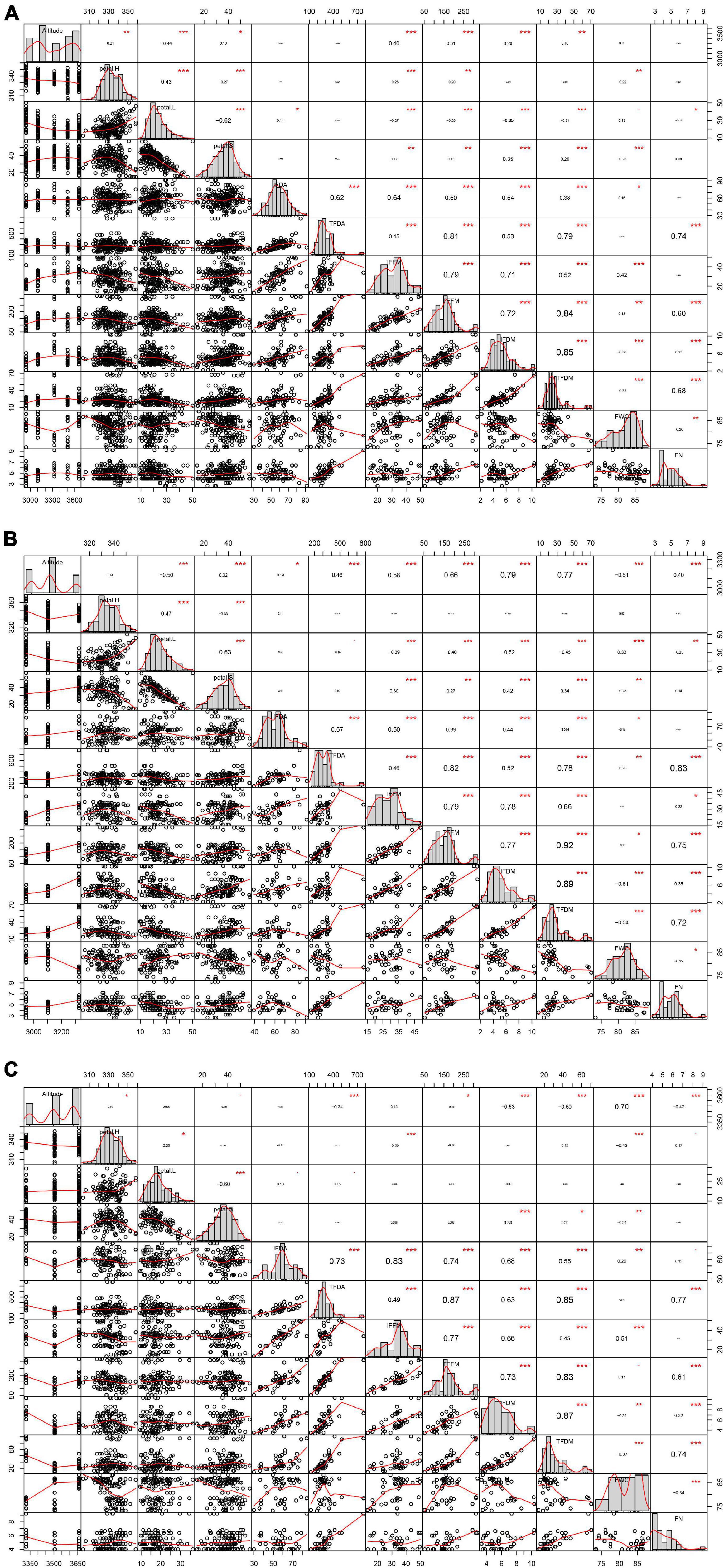
Figure 4. Correlation among floral traits and color within different altitude ranges: (A) 2,950–3,650 m, (B) 2,950–3,300 m, and (C) 3,300–3,650 m.
Floral Display
Altitude had a significant effect on individual floral display area (IFDA), total floral display area (TFDA), individual Leaf area (ILA), total leaf area (TLA), individual floral fresh mass (IFFM), total floral fresh mass (TFFM), individual floral dry mass (IFDM), total floral dry mass (TFDM), floral number per twig (FN), and FWC (Table 2). Within the altitudinal gradient, no significant correlation was observed between altitude and FN, but at 3,300 m, FN was significantly higher than at other altitudinal gradients (Figure 4). As for the floral biomass, both the IFFM and the IFDM had a significant correlation with altitude (Figures 4A,B). However, the variation trend by segment was different for IFFM and IFDM; the breakpoint was at around 3,300 m, below which the IFFM and the IFDM both had a significant positive correlation with altitude. The difference occurred when altitude was above 3,300 m; the IFFM no longer changed significantly after 3,300 m, but the IFDM showed a descending trend (Figures 3D,E). The gradient variation of TFFM was similar to that of IFFM. It showed a significant correlation with altitude, and below the breakpoint 3,300 m, the TFFM was increasing with an increase in altitude. Afterward, the TFFM showed a slight drop compared to that at 3,300 m (Figure 3D). The TFDM had a similar gradient variation tendency with IFDM, but no significance was observed (Figure 3E).
The IFDA had no significant correlation with altitude (Figure 4A), while altitude had opposite effects on TFDA at both sides of 3,300 m (Figures 4B,C)—below 3,300 m, TFDA increased with an increase in altitude. However, at altitudes above 3,300 m, the TFDA decreased with an increase in altitude (Figure 3G).
Correlation Within Floral Traits and Color Performance
The piecewise regression showed a breakpoint in the gradient change of FWC at around 3,300 m (Figure 3F). Below 3,300 m, the altitude had a significant negative effect on FWC, while the reverse was observed at above 3,300 m. The relationship between FWC and color performance could be determined in separated altitude ranges, with a breakpoint at around 3,300 m. The FWC had a significant positive effect on petal L and a significant negative effect on petal S up to 3,300 m, with no significant effect on petal H (Figure 4B). However, at above 3,300 m, the FWC showed a significant negative effect on petal H but no significant effect on either petal L or petal S (Figure 4C). As for the floral biomass, FWC had a significant negative correlation with IFFM and TFFM (Figures 4A–C). Otherwise, the floral biomass also had a separate correlation with color performance at different altitude ranges, and the floral biomass had a significant negative correlation with petal L while it had a positive correlation with petal S; both correlations were highly significant below 3,300 m (Figures 4A–C).
From the RDA analysis, within the whole range of altitude (2,950–3,650 m), the eigenvalues of each axis were 0.1620, 0.0466, 0.0134, and 0.4258, and the explained variations of each axis were 16.20, 20.86, 22.20, and 64.78%; at 2,950–3,300 m, the eigenvalues of each axis were 0.3964, 0.0364, 0.0153, and 0.3061, and the explained variations of each axis were 39.64, 43.28, 44.80, and 75.41%; at 3,300–3,650 m, the eigenvalues of each axis were 0.1405, 0.0762, 0.0337, and 0.4475, and the explained variations of each axis were 14.05, 21.67, 25.03, and 69.78%, respectively (Figure 5).
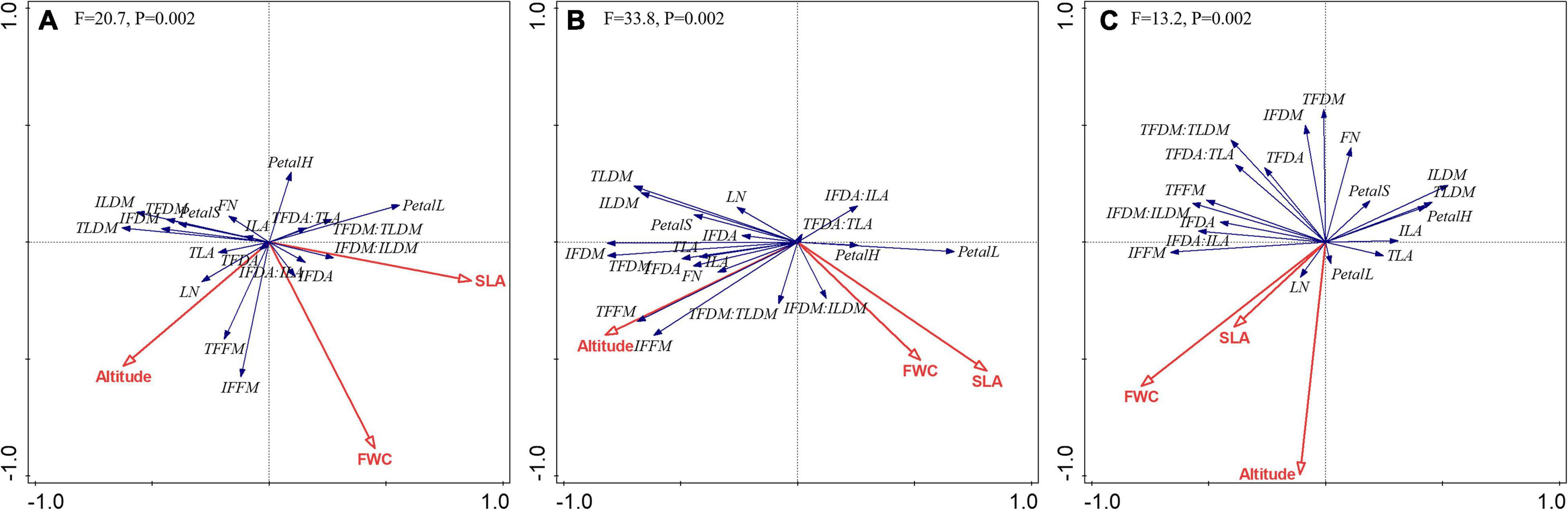
Figure 5. Redundancy analysis of the relationship among floral color, floral traits, leaf traits, and environmental factors under different altitude ranges: (A) 2,950–3,650 m, (B) 2,950–3,300 m, and (C) 3,300–3,650 m.
Discussion
As expected, the study shows that altitude has a significant effect on flower color and size, and one breakpoint occurred at around 3,300 m; however, our results also indicate that the altitudinal variation tendency of the flower color and size was different but complementary. The flower color darkened as the altitude increased till a range of below 3,300 m, while the flower size was expanding; however, both flower color and size remained stable at above 3,300 m (Figure 6).
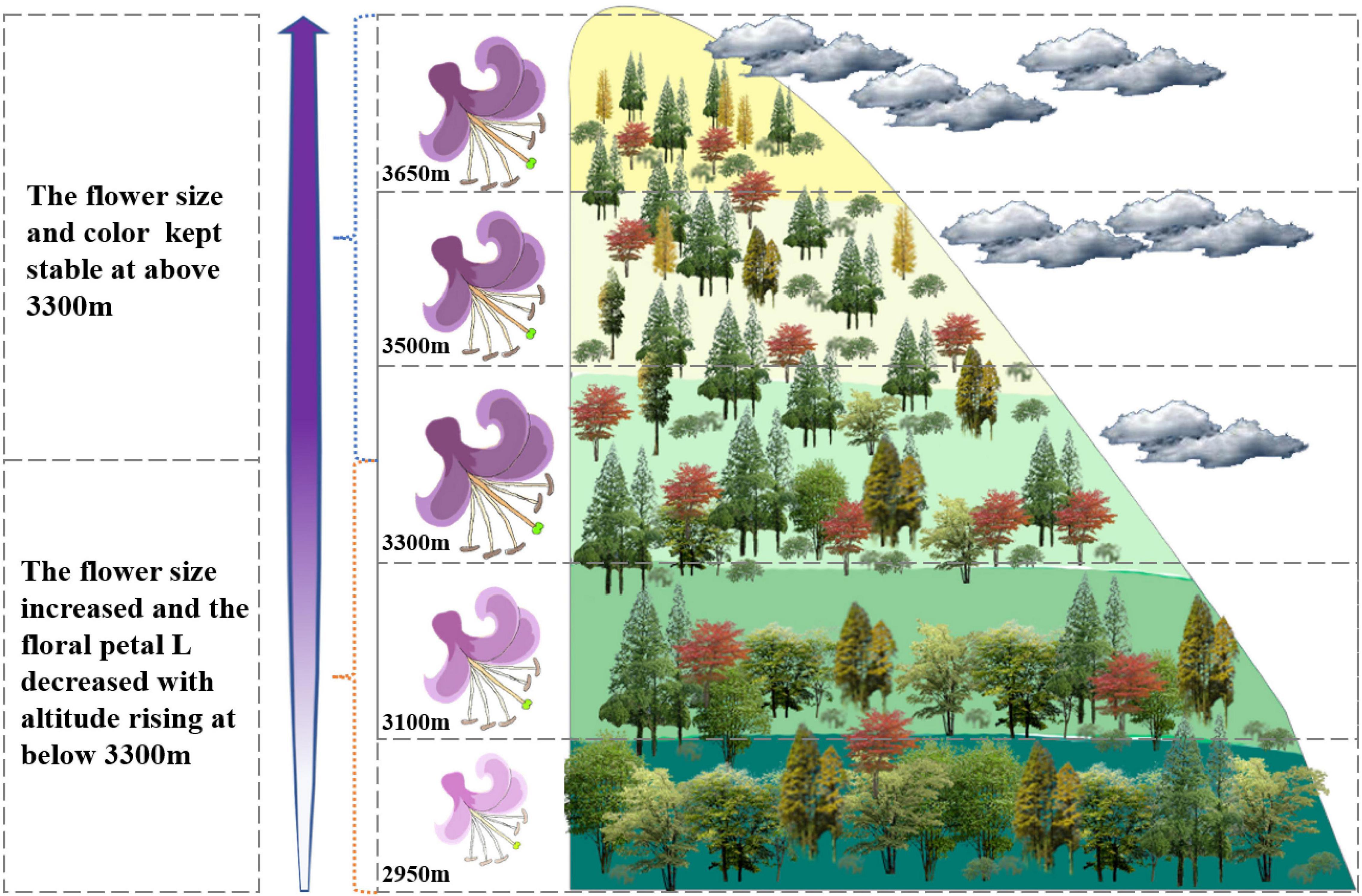
Figure 6. Diagrammatic sketch of the transformation on floral size and color of Lonicera nervosa. The flowers of L. nervosa tended to be larger and darker along the altitude; however, the floral display appeared to be largest at an altitude of around 3,300 m, and the petal color lightness was kept stable at above 3,300 m.
The temporal and spatial combinations of different pigments and their contents ultimately determine the floral color since the red, blue, or lavender pigmentation is mainly determined by anthocyanins (Liu et al., 2020). Experiments show that the color lightness of plants had a negative linear correlation with anthocyanin (Qi et al., 2019). For L. nervosa, the flowers were pink to purple, and the result of petal H also led to purplish red, which implied that the crucial pigment of L. nervosa was anthocyanin. The results of floral color performance can be explained in two aspects: abiotic and biotic, respectively. The floral color lightness could be determined by the deposition of pigment, which was strongly affected by abiotic factors, such as solar UV and temperature and biotic factors like pollinators.
Firstly, from an abiotic perspective, plants adapt to UV and low temperature stress by pigment deposition (Koski et al., 2020). They usually adopt darker colors in high-ultraviolet and low-temperature environments (Arista et al., 2013). Darker pigmentation was under a particularly strong selection by UV-B irradiance (Roulin, 2014); an increase in UV may drive an increase in UV-absorbing floral pigmentation (Koski et al., 2020), and anthocyanins have been thought to play a major role in acting as a UV screen that protects the DNA of a plant from sunlight damage (Steyn et al., 2002; He et al., 2011). In habitats with high solar UV, the floral anthocyanin concentration was usually found to be the highest (Peach et al., 2020). The amount of UV radiation in China is mainly dependent on its geographical latitude, altitude, cloud, and aerosols (Hu et al., 2007; Zhou and Chen, 2008), although it is commonly contributing a higher fraction at any given incoming irradiance at high altitude compared with low altitude (for clear-sky conditions) (Blumthaler, 2007), i.e., the UV radiation increased by 0.202–0.090 W/m2 with altitude increasing per 100 m, respectively, during the dry and wet seasons on Yunnan–Kweichow Plateau (Zhou and Chen, 2008); more frequent clouds and fogs can negate or even reverse this effect (Caldwell, 1968; Körner, 2003; Hu et al., 2007). In our study, a breakpoint occurred at around 3,300 m when the floral color was changing along the altitude—but why? Generally, as light intensity decreases, the leaf area and SLA of plants increase to improve their ability to capture light energy (Saldaña-Acosta et al., 2009; Xiao et al., 2015; Wang et al., 2021). We could consider SLA as an indicator for the intensity of illumination. SLA decreased with an increase in altitude till 3,300 m and reversed at above 3,300 m (Figure 3L). Meanwhile, SLA had a significant positive correlation with petal L (Supplementary Figure 1), so we could conclude that light intensity had a strong effect on floral color and the light intensity decreased with an increase in altitude above 3,300 m in our study region. Our results also showed that the correlation between FWC and floral color performance was different at both sides of the 3,300-m altitudinal range. Below 3,300 m, FWC had a significant correlation with petal L, while there was no correlation observed beyond 3,300 m (Figure 4). FWC can be an indicator for the variation of ambient humidity. Compared to leaves, flowers had a high saturated water content, which was a strong predictor of hydraulic capacitance in both leaves and flowers (Roddy et al., 2019). The vulnerability of plants to freezing temperatures gradually increased prior to and just after flushing in spring, when freezing events may still occur (Lenz et al., 2013). With decreasing temperature along the altitude, the phenological period of L. nervosa was delayed by 18 days at a higher altitude (according to our sampling date), so there was a shorter photoperiod at a low altitudinal range when flowers were blooming, which may imply a signal of a higher possibility of risk in cold spell in spring for the plants (Körner, 2003; Augspurger, 2008; Fu et al., 2019), and a lower water content could reduce the damage of cold (Körner, 2003). With the phenological period occurring later at high altitudes, the longer photoperiod may imply a lower risk of cold spell, so the FWC decreased with elevation, but things changed when the altitude rose above 3,300 m, suggesting that the moist environment could reverse the elevation effect on FWC. Combining the result of SLA with FWC, we determine that, at an altitude above 3,300 m, there were more frequent clouds during the period when the L. nervosa flower was blooming, but instead of turning brighter, the petal L of L. nervosa tended to remain stable above 3,300 m. We infer that it may occur as a combined effect of pollinators and floral temperature. Secondly, from a biotic perspective, anthocyanins are also thought to play a role in attracting insects for the purpose of pollination (He et al., 2011). Hymenoptera carried the highest proportion of pollinators (Zhao et al., 2018). Among them, bees and bumblebees had the highest pollination efficiency and the largest proportion (Zou and Huang, 2014). According to previous research and field observation, bumblebees are the main pollinator of L. nervosa, which were typical of alpine bees, mainly distributed in subalpine coniferous forest belt and alpine shrub meadow belt, with bumblebees showing a stronger preference for brighter flowers (Deng et al., 2017).
For the results of floral size, we could also take sights into the abiotic and biotic aspects. Obtaining an optimal flower temperature was crucial for plant reproduction because temperature mediated flower growth and development and pollen and ovule viability, and it influenced pollinator visitation (van der Kooi et al., 2019). Both the plant and anthophilous insects can benefit from a flower temperature that differs from ambient conditions; dark color may lead to a higher intra-floral temperature because they absorb more energy than light and reflective flowers (Jewell et al., 1994; van der Kooi et al., 2019), and a larger flower may expand the surface area that can absorb radiation (van der Kooi et al., 2019). Zhao et al. (2016) showed that pollinator diversity tended to be higher in communities at lower elevations, and plant species with large floral displays and high flower abundance were more selective in their exploitation of pollinators. However, the selection of a flower size from a plant species by pollinators could vary with changing habitats due to differences in pollinator communities (Bode and Tong, 2018). Meanwhile, the floral biomass was a ternary parameter, while floral display area was a binary parameter; therefore, the response of floral display area to the investment in floral biomass was not so efficient as a linear response, for example, the response in pigmentation, so the slope of IFDM was sharper than petal L (Figures 3B,E). Although the investment on leaf was increasing as the altitude increased (Figures 3H,J,K), the drivers for this change were different below 3,300 m. L. nervosa developed larger leaves to achieve more reproductive inputs; with a cloudier environment at above 3,300 m, photosynthesis was inhibited and assimilation efficiency was reduced. L. nervosa invested more biomass into vegetative growth to sustain, so TFDM/TLDM decreased in this altitude range (Figure 3I). We can conclude that L. nervosa adopts a more radical strategy on reproduction below 3,300 m and a conservative strategy at above 3,300 m. The results show that, when the environmental stress brought by altitude was moderate, L. nervosa adopted a more radical reproductive investment strategy, and the weight of different selection agents varied within different altitudinal ranges.
Conclusion
With the increase in environmental stress due to increasing elevation, the reproductive investment strategy of L. nervosa tended to be more conservative. Under the combined effect of temperature, UV radiation, clouds, and pollinator selection, a single flower of L. nervosa appeared to be darker and larger at a higher altitude. However, the darkening effect had a limitation, and the total investment in floral biomass had a negative correlation with petal L. The darkening of floral color and the expansion of floral display tend to be complementary patterns, which can provide guidance for the introduction and breeding of wild L. nervosa.
Natural creation is like a natural artist. The adaptability of the plants provides a factor to evaluate the utilization potential. When introducing and utilizing the plants for landscaping, people can choose the population suitable to their own needs. Therefore, for plant landscape utilization, the diversity of the characteristics of L. nervosa calls for more attention. However, the phenotypic molecular mechanism of the plasticity of color and flower display of L. nervosa requires further study.
Data Availability Statement
The raw data supporting the conclusions of this article will be made available by the authors, without undue reservation.
Author Contributions
WC, JW, and YW raised scientific questions and designed the experiments. WC, LW, SX, and XL conducted the experiments. WC, LW, JW, and YL analyzed the experimental data. WC, JW, SR, AT, and YW wrote the manuscript. All authors contributed substantially to this research work, discussed the results, and approved the final manuscript.
Funding
This research was supported by the National Key Research and Development Program of China (2017YFC0505005-1 and 2020YFE0203200) and the Program of Jiuzhaigou Administration Bureau (5132202020000046). The authors would like to acknowledge the funding support provided by National Natural Science Foundation of China (31971436 and 41661144045).
Conflict of Interest
The authors declare that the research was conducted in the absence of any commercial or financial relationships that could be construed as a potential conflict of interest.
The reviewer DL declared a shared affiliation, though no other collaboration, with several of the authors, WC, LW, JW, SX, AT, YL, and YW to the handling editor.
Publisher’s Note
All claims expressed in this article are solely those of the authors and do not necessarily represent those of their affiliated organizations, or those of the publisher, the editors and the reviewers. Any product that may be evaluated in this article, or claim that may be made by its manufacturer, is not guaranteed or endorsed by the publisher.
Acknowledgments
We would like to thank Jiagui Wang, Kun Yu, Qiang Wang, Fei Wang, Yinlin Shi, and Tianyang Zhou for their assistance during plant observation and sampling collection. We are grateful to the associate editor and one referee for providing valuable comments.
Supplementary Material
The Supplementary Material for this article can be found online at: https://www.frontiersin.org/articles/10.3389/fevo.2021.719838/full#supplementary-material
Supplementary Figure 1 | Specific leaf area had a significant correlation with the hue–saturation–lightness color space (petal L and petal S) of Lonicera nervosa.
Supplementary Figure 2 | The visitation of a bumblebee to the flowers of Lonicera nervosa.
Supplementary Figure 3 | Piecewise regression of the traits at different altitudes.
References
Ågren, J., Hellström, F., Toräng, P., Toräng, P., and Ehrlén, J. (2013). Mutualists and antagonists drive among-population variation in selection and evolution of floral display in a perennial herb. Proc. Natl. Acad. Sci. U.S.A. 110, 18202–18207. doi: 10.1073/pnas.1301421110
Arista, M., Talavera, M., Berjano, R., Ortiz, P. L., and Whitney, K. (2013). Abiotic factors may explain the geographical distribution of flower colour morphs and the maintenance of colour polymorphism in the scarlet pimpernel. J. Ecol. 101, 1613–1622. doi: 10.1111/1365-2745.12151
Augspurger, C. K. (2008). Early spring leaf out enhances growth and survival of saplings in a temperate deciduous forest. Oecologia 156, 281–286. doi: 10.1007/s00442-008-1000-7
Bai, G., Jenkins, S., Yuan, W., Graef, G. L., and Ge, Y. (2018). Field-based scoring of soybean iron deficiency chlorosis using rgb imaging and statistical learning. Front. Plant Sci. 9:1002. doi: 10.3389/fpls.2018.01002
Bauer, A. A., Clayton, M. K., and Brunet, J. (2017). Floral traits influencing plant attractiveness to three bee species: consequences for plant reproductive success. Am. J. Bot. 104, 772–781. doi: 10.3732/ajb.1600405
Blumthaler, M. (2007). “Factors, trends and scenarios of uv radiation in arctic-alpine environments,” in Arctic Alpine Ecosystems and People in a Changing Environment, eds J. B. Ørbæk, R. Kallenborn, I. Tombre, and E. N. Hegseth (Berlin: Springer Berlin Heidelberg), 181–193. doi: 10.1007/978-3-540-48514-8_11
Bode, R. F., and Dufresne, C. (2020). Natural selection on flower size in invasive Cytisus scoparius along an elevation gradient. J. Plant Ecol. 13, 165–170. doi: 10.1093/jpe/rtz058
Bode, R. F., and Tong, R. (2018). Pollinators exert positive selection on flower size on urban, but not on rural scotch broom (Cytisus scoparius l. Link). J Plant Ecol 11, 493–501. doi: 10.1093/jpe/rtx024
Bożek, M. (2007). Pollen productivity and morphology of pollen grains in two cultivars of honeyberry (Lonicera kamtschatica (sevast.) pojark.). Acta Agrobot. 60, 73–77. doi: 10.5586/aa.2007.008
Brunet, J., Thairu, M. W., Henss, J. M., Link, R. I., and Kluever, J. A. (2015). The effects of flower, floral display, and reward sizes on bumblebee foraging behavior when pollen is the reward and plants are dichogamous. Int. J. Plant Sci. 176, 811–819. doi: 10.1086/683339
Caldwell, M. M. (1968). Solar ultraviolet radiation as an ecological factor for alpine plants. Ecol. Monogr. 38, 243–268. doi: 10.2307/1942430
Campbell, D. R., Bischoff, M., Lord, J. M., and Robertson, A. W. (2010). Flower color influences insect visitation in alpine New Zealand. Ecology 91, 2638–2649. doi: 10.1890/09-0941.1
Cayenne Engel, E., and Irwin, R. E. (2003). Linking pollinator visitation rate and pollen receipt. Am. J. Bot. 90, 1612–1618. doi: 10.3732/ajb.90.11.1612
Chien, C. L., and Tsai, W. H. (2014). Image fusion with no gamut problem by improved nonlinear ihs transforms for remote sensing. IEEE Trans. Geosci. Remote Sens. 52, 651–663. doi: 10.1109/tgrs.2013.2243157
Darwin, C. (1862). On the various contrivances by which british and foreign orchids are fertilized by insects; and on the good effects of intercrossing. Br. Foreign Med. Chir. Rev. 30, 312–318.
Davis, M. B., Shaw, R. G., and Etterson, J. R. (2005). Evolutionary responses to changing climate. Ecology 86, 1704–1714. doi: 10.1890/03-0788
Del Valle, J. C., Buide, M. L., Casimiro-Soriguer, I., Whittall, J. B., and Narbona, E. (2015). On flavonoid accumulation in different plant parts: variation patterns among individuals and populations in the shore campion (Silene littorea). Front. Plant Sci. 6:939. doi: 10.3389/fpls.2015.00939
Del Valle, J. C., Gallardo-López, A., Buide, M. L., Whittall, J. B., and Narbona, E. (2018). Digital photography provides a fast, reliable, and noninvasive method to estimate anthocyanin pigment concentration in reproductive and vegetative plant tissues. Ecol. Evol. 8, 3064–3076. doi: 10.1002/ece3.3804
Deng, H., Xiang, G. J., Guo, Y. H., and Yang, C. F. (2017). Study on the breeding system and floral color change of four lonicera species in the Qinling mountains. Plant Sci. J. 35, 1–12.
Feng, X. R., Wang, X. W., Jing, Y. C., Mao, L. Q., Lu, C. H., and Cui, J. L. (2015). A study of introduction and cutting propagation of wild plants. Ningxia J. Agric. For. Sci. Tech. 56, 12–14.
Fenster, C. B., Armbruster, W. S., Wilson, P., Dudash, M. R., and Thomson, J. D. (2004). Pollination syndromes and floral specialization. Annu. Rev. Ecol. Evol. Syst. 35, 375–403.
Fu, Y. H., Piao, S., Zhou, X., Geng, X., Hao, F., Vitasse, Y., et al. (2019). Short photoperiod reduces the temperature sensitivity of leaf-out in saplings of Fagus sylvatica but not in horse chestnut. Glob. Chang. Biol. 25, 1696–1703. doi: 10.1111/gcb.14599
Gagliardi, S., Martin, A. R., Filho, E. d. M. V, Rapidel, B., and Isaac, M. E. (2015). Intraspecific leaf economic trait variation partially explains coffee performance across agroforestry management regimes. Agric. Ecosyst. Environ. 200, 151–160. doi: 10.1016/j.agee.2014.11.014
Ge, L., Li, J., Wan, H., Zhang, K., Wu, W., Zou, X., et al. (2018). Novel flavonoids from lonicera japonica flower buds and validation of their anti-hepatoma and hepatoprotective activity in vitro studies. Ind. Crops Prod. 125, 114–122. doi: 10.1016/j.indcrop.2018.08.073
Harrell, F. E. Jr., and Dupont, C., et al. (2021). Hmisc: Harrell Miscellaneous. R package version 4.5-0.
He, J., Dong, T., Huang, K., Yang, Y., Li, D., Xu, X., et al. (2017). Sex-specific floral morphology, biomass, and phytohormones associated with altitude in dioecious Populus cathayana populations. Ecol. Evol. 7, 3976–3986. doi: 10.1002/ece3.2808
He, Q., Shen, Y., Wang, M., Huang, M., Yang, R., Zhu, S., et al. (2011). Natural variation in petal color in Lycoris longituba revealed by anthocyanin components. PLoS One 6:e22098. doi: 10.1371/journal.pone.0022098
Hong, G., Luo, M. R., and Rhodes, P. A. (2001). A study of digital camera colorimetric characterization based on polynomial modeling. Color Res. Appl. 26, 76–84. doi: 10.1002/1520-6378(200102)26:1<76::aid-col8>3.0.co;2-3
Hu, B., Wang, Y., and Liu, G. (2007). Ultraviolet radiation spatio-temporal characteristics derived from the ground-based measurements taken in china. Atmos. Environ. 41, 5707–5718. doi: 10.1016/j.atmosenv.2007.02.044
Jachuła, J., Denisow, B., and Strzałkowska-Abramek, M. (2019). Floral reward and insect visitors in six ornamental lonicera species–plants suitable for urban bee-friendly gardens. Urban For. Urban Green. 44:126390. doi: 10.1016/j.ufug.2019.126390
Jewell, J., McKee, J., and Richards, A. J. (1994). The keel colour polymorphism in Lotus corniculatus l.: differences in internal flower temperatures. New Phytol. 128, 363–368. doi: 10.1111/j.1469-8137.1994.tb04020.x
Joblove, G. H., and Greenberg, D. (1978). Color spaces for computer graphics. Comput. Graph. 12, 20–25. doi: 10.1145/965139.807362
Karron, J. D., and Mitchell, R. J. (2012). Effects of floral display size on male and female reproductive success in mimulus ringens. Ann. Bot. 109, 563–570. doi: 10.1093/aob/mcr193
Kattge, J., Bonisch, G., Diaz, S., Lavorel, S., Prentice, I. C., Leadley, P., et al. (2020). Try plant trait database–enhanced coverage and open access. Glob. Chang. Biol. 26, 119–188.
Kendal, D., Hauser, C. E., Garrard, G. E., Jellinek, S., Giljohann, K. M., and Moore, J. L. (2013). Quantifying plant colour and colour difference as perceived by humans using digital images. PLoS One 8:e72296. doi: 10.1371/journal.pone.0072296
Körner, C. (2003). Alpine Plant Life-Functional Plant Ecology of High Mountain Ecosystems. Berlin: Springer-Verlag Berlin Heidelberg.
Körner, C. (2007). The use of ‘altitude’ in ecological research. Trends Ecol. Evol. 22, 569–574. doi: 10.1016/j.tree.2007.09.006
Koski, M. H., MacQueen, D., and Ashman, T. L. (2020). Floral pigmentation has responded rapidly to global change in ozone and temperature. Curr. Biol. 30, 4425–4431.e3.
Kuriya, S., Hattori, M., Nagano, Y., and Itino, T. (2015). Altitudinal flower size variation correlates with local pollinator size in a bumblebee-pollinated herb, Prunella vulgaris l. (lamiaceae). J. Evol. Biol. 28, 1761–1769. doi: 10.1111/jeb.12693
Lenz, A., Hoch, G., Vitasse, Y., and Korner, C. (2013). European deciduous trees exhibit similar safety margins against damage by spring freeze events along elevational gradients. New Phytol. 200, 1166–1175. doi: 10.1111/nph.12452
Li, G. H. (2010). Study on Plant Landscaping Art from the Perspective of Plant Landscape Evaluation. Beijing: Beijing Forestry University.
Li, M., Yang, W. Q., Xiao, Y., and Wu, F. Z. (2010). Effects of disturbance on soil physical characteristics in bipengou ecotourism area. Chin. Agric. Sci. Bull. 26, 303–307.
Liang, S. Y., Yang, C. Y., Zhang, Q. W., and Weng, J. (2013). Experimental analysisi of color temperature and color rendering properties of daylight. J. Civil Archit. Environ. Eng. 35, 112–117.
Lin, T., Liao, B., Hsu, S., and Wang, J. (2015). “Experimental investigation of hsl color model in error diffusion,” in Proceedings International Conference on Ubi-Media Computing (UMEDIA), (Colombo: IEEE), 268–272.
Liu, A. C., Yu, Q., Wang, F. W., Bai, G. Q., Wang, Q., Li, S. J., et al. (2020). Changes of floral color and pigment content during flowering in several species of Lonicera. J. Southwest Univ. 42, 22–29.
Liu, A. C., Yu, Q., Wang, Q., Wang, L. S., and Pang, C. M. (2015). Changes in composition and content of anthocyanins during flowering process of Lonicera. J. Northwest A F Univ. (Nat. Sci. Ed.) 43, 169–174.
Makino, T. T., and Sakai, S. (2007). Experience changes pollinator responses to floral display size: from size-based to reward-based foraging. Funct. Ecol. 21, 854–863. doi: 10.1111/j.1365-2435.2007.01293.x
Malerba, R., and Nattero, J. (2011). Pollinator response to flower color polymorphism and floral display in a plant with a single-locus floral color polymorphism: consequences for plant reproduction. Ecol. Res. 27, 377–385. doi: 10.1007/s11284-011-0908-2
Malo, J. E., and Baonza, J. (2002). Are there predictable clines in plant–pollinator interactions along altitudinal gradients? The example of Cytisus scoparius (l.) link in the sierra de guadarrama (central spain). Divers. Distribut. 8, 365–371. doi: 10.1046/j.1472-4642.2002.00161.x
McCall, C., and Primack, R. B. (1992). Influence of flower characteristics, weather, time of day, and season on insect visitation rates in three plant communities. Am. J. Bot. 79, 434–442. doi: 10.1002/j.1537-2197.1992.tb14571.x
Mitchell-Olds, T., Willis, J. H., and Goldstein, D. B. (2007). Which evolutionary processes influence natural genetic variation for phenotypic traits? Nat. Rev. Genet. 8, 845–856. doi: 10.1038/nrg2207
Mizunuma, T., Mencuccini, M., Wingate, L., Ogée, J., Nichol, C., Grace, J., et al. (2014). Sensitivity of colour indices for discriminating leaf colours from digital photographs. Methods Ecol. Evol. 5, 1078–1085. doi: 10.1111/2041-210x.12260
Nagano, Y., Abe, K., Kitazawa, T., Hattori, M., Hirao, A. S., and Itino, T. (2014). Changes in pollinator fauna affect altitudinal variation of floral size in a bumblebee-pollinated herb. Ecol. Evol. 4, 3395–3407. doi: 10.1002/ece3.1191
Nicotra, A. B., Atkin, O. K., Bonser, S. P., Davidson, A. M., Finnegan, E. J., Mathesius, U., et al. (2010). Plant phenotypic plasticity in a changing climate. Trends Plant Sci. 15, 684–692. doi: 10.1016/j.tplants.2010.09.008
Peach, K., Liu, J. W., and Mazer, S. J. (2020). Climate predicts uv floral pattern size, anthocyanin concentration, and pollen performance in Clarkia unguiculata. Front. Plant Sci. 11:847. doi: 10.3389/fpls.2020.00847
Peterson, B. G., and Peter, C. (2020). PerformanceAnalytics: Econometric Tools for Performance and Risk Analysis. R package version 2.0.4.
Qi, R., Li, X. H., Shi, B. Y., Wang, Y., Tian, G., Bai, X., et al. (2019). Analysis on the relationship of leaf color parameters and pigments contents in photinia × fraseri dress with veraison stage. J. Henan Agric. Sci. 48, 93–101.
Qiao, P., Liu, H., Yan, X., Jia, Z., and Pi, X. (2016). A smart capsule system for automated detection of intestinal bleeding using hsl color recognition. PLoS One 11:e0166488. doi: 10.1371/journal.pone.0166488
R Core Team (2020). R: A Language and Environment for Statistical Computing. Vienna: R Foundation for Statistical Computing.
Ramos, S. E., and Schiestl, F. P. (2019). Rapid plant evolution driven by the interaction of pollination and herbivory. Science 364:193. doi: 10.1126/science.aav6962
Rausher, M. D. (2008). Evolutionary transitions in floral color. Int. J. Plant Sci. 169, 7–21. doi: 10.1086/523358
Roddy, A. B., Jiang, G. F., Cao, K., Simonin, K. A., and Brodersen, C. R. (2019). Hydraulic traits are more diverse in flowers than in leaves. New Phytol. 223, 193–203. doi: 10.1111/nph.15749
Rodríguez-Castañeda, N. L., Ortiz, P. L., Arista, M., Narbona, E., and Buide, M. L. (2020). Indirect selection on flower color in Silene littorea. Front. Plant Sci. 11:588383. doi: 10.3389/fpls.2020.588383
Roulin, A. (2014). Melanin-based colour polymorphism responding to climate change. Glob. Chang. Biol. 20, 3344–3350. doi: 10.1111/gcb.12594
Saldaña-Acosta, A., Meave, J. A., and Sánchez-Velásquez, L. R. (2009). Seedling biomass allocation and vital rates of cloud forest tree species: responses to light in shade house conditions. For. Ecol. Manag. 258, 1650–1659. doi: 10.1016/j.foreco.2009.07.027
Schneider, C. A., Rasband, W. S., and Eliceiri, K. W. (2012). Nih image to imagej: 25 years of image analysis. Nat. Methods 9, 671–675. doi: 10.1038/nmeth.2089
Shang, X., Pan, H., Li, M., Miao, X., and Ding, H. (2011). Lonicera japonica thunb.: ethnopharmacology, phytochemistry and pharmacology of an important traditional Chinese medicine. J. Ethnopharmacol. 138, 1–21. doi: 10.1016/j.jep.2011.08.016
Souto-Vilarós, D., Vuleta, A., Jovanović, S. M., Budečević, S., Wang, H., Sapir, Y., et al. (2018). Are pollinators the agents of selection on flower colour and size in irises? Oikos 127, 834–846. doi: 10.1111/oik.04501
Stevens, M., PÁRraga, C. A., Cuthill, I. C., Partridge, J. C., and Troscianko, T. S. (2007). Using digital photography to study animal coloration. Biol. J. Linn. Soc. 90, 211–237. doi: 10.1111/j.1095-8312.2007.00725.x
Steyn, W. J., Wand, S. J. E., Holcroft, D. M., and Jacobs, G. (2002). Anthocyanins in vegetative tissues: a proposed unified function in photoprotection. New Phytol. 155, 349–361. doi: 10.1046/j.1469-8137.2002.00482.x
Streisfeld, M. A., and Kohn, J. R. (2005). Contrasting patterns of floral and molecular variation across a cline in Mimulus aurantiacus. Evolution 59, 2548–2559. doi: 10.1554/05-514.1
Vaidya, P., McDurmon, A., Mattoon, E., Keefe, M., Carley, L., Lee, C. R., et al. (2018). Ecological causes and consequences of flower color polymorphism in a self-pollinating plant (Boechera stricta). New Phytol. 218, 380–392. doi: 10.1111/nph.14998
van der Kooi, C. J., Kevan, P. G., and Koski, M. H. (2019). The thermal ecology of flowers. Ann. Bot. 124, 343–353.
Wang, D., Huang, X., Chen, J., Li, L., Cheng, J., Wang, S., et al. (2021). Plasticity of leaf traits of Juglans regia l. F. Luodianense liu et xu seedlings under different light conditions in karst habitats. Forests 12:81. doi: 10.3390/f12010081
Wang, Z. L., Wang, H., Zheng, S. X., and Geng, S. L. (2000). Main wild ornamental plants of honeysuckle genera Lonicera, in qin hai plateau area. Qinghai Sci. Technol. 7, 37–38.
Weeks, A., Felix, C., and Myler, H. (1995). Edge detection of color images using the hsl color space: SPIE. Int. Soc. Opt. Eng. 2424, 291–301.
White, T. E., Dalrymple, R. L., Noble, D. W. A., O’Hanlon, J. C., Zurek, D. B., and Umbers, K. D. L. (2015). Reproducible research in the study of biological coloration. Anim. Behav. 106, 51–57. doi: 10.1016/j.anbehav.2015.05.007
Xiao, H., Wang, C., Liu, J., Wang, L., and Du, D. (2015). Insights into the differences in leaf functional traits of heterophyllous Syringa oblata under different light intensities. J. For. Res. 26, 613–621. doi: 10.1007/s11676-015-0100-6
Zhang, J. Z., Liang, S. J., and Shi, L. (2004). The study on the cultivation and application of climbing honeysuckle resource. Chin. Landsc. Archit. 20, 53–56.
Zhao, Y. H., Lázaro, A., Ren, Z. X., Zhou, W., Li, H. D., Tao, Z. B., et al. (2018). The topological differences between visitation and pollen transport networks: a comparison in species rich communities of the Himalaya–Hengduan mountains. Oikos 128, 551–562. doi: 10.1111/oik.05262
Zhao, Y. H., Ren, Z. X., Lazaro, A., Wang, H., Bernhardt, P., Li, H. D., et al. (2016). Floral traits influence pollen vectors’ choices in higher elevation communities in the Himalaya-Hengduan mountains. BMC Ecol. 16:26. doi: 10.1186/s12898-016-0080-1
Zhou, P., and Chen, Z. Y. (2008). Analysis of the spatio-temporal characteristics of uv-b strength change over the yunnan plateau. J. Nat. Resour. 23, 477–493.
Keywords: environmental adaptation, floral color, floral display, HSL, Lonicera nervosa, ornamental utilization
Citation: Chen W, Wang L, Wang J, Joshi S, Xiang S, Tariq A, Liu X, Liao Y and Wu Y (2021) Divergent Responses of Floral Traits of Lonicera nervosa to Altitudinal Gradients at the Eastern Margin of Hengduan Mountains. Front. Ecol. Evol. 9:719838. doi: 10.3389/fevo.2021.719838
Received: 03 June 2021; Accepted: 20 July 2021;
Published: 10 August 2021.
Edited by:
Jian Sun, Institute of Tibetan Plateau Research (CAS), ChinaReviewed by:
Dongliang Luo, Northwest Institute of Eco-Environment and Resources, Chinese Academy of Sciences, ChinaGang Fu, Institute of Geographic Sciences and Natural Resources Research, Chinese Academy of Sciences, China
Copyright © 2021 Chen, Wang, Wang, Joshi, Xiang, Tariq, Liu, Liao and Wu. This is an open-access article distributed under the terms of the Creative Commons Attribution License (CC BY). The use, distribution or reproduction in other forums is permitted, provided the original author(s) and the copyright owner(s) are credited and that the original publication in this journal is cited, in accordance with accepted academic practice. No use, distribution or reproduction is permitted which does not comply with these terms.
*Correspondence: Jinniu Wang, wangjn@cib.ac.cn; Yan Wu, wuyan@cib.ac.cn