Riverscape Genomics Clarifies Neutral and Adaptive Evolution in an Amazonian Characin Fish (Triportheus albus)
- 1Molecular Ecology Laboratory, College of Science and Engineering, Flinders University, Adelaide, SA, Australia
- 2Molecular Ecology Lab, Department of Biological Sciences, Macquarie University, Sydney, NSW, Australia
- 3Bio-Amazonia Conservation International, Brookline, MA, United States
- 4National Museum of Marine Biology and Aquarium, Pintung, Taiwan
Understanding the role of natural selection in the evolution of wild populations is challenging due to the spatial complexity of natural systems. The richest diversity of freshwater fishes in the world is found in the Amazon Basin, a system where marked hydrochemical differences exist at the interface of major rivers with distinct “water colors” (i.e., black, white, and clear water). We hypothesize that divergent natural selection associated with these “aquatic ecotones” influences population-level adaptive divergence in the non-migratory Amazonian fish fauna. This hypothesis was tested using a landscape genomics framework to compare the relative contribution of environmental and spatial factors to the evolutionary divergence of the Amazonian characin fish Triportheus albus. The framework was based on spatial data, in situ hydrochemical measurements, and 15,251 filtered SNPs (single nucleotide polymorphisms) for T. albus sampled from three major Amazonian rivers. Gradient Forest, redundancy analysis (RDA) and BayPass analyses were used to test for signals of natural selection, and model-based and model-free approaches were used to evaluate neutral population differentiation. After controlling for a signal of neutral hierarchical structure which was consistent with the expectations for a dendritic system, variation in turbidity and pH were key factors contributing to adaptive divergence. Variation in genes involved in acid-sensitive ion transport pathways and light-sensitive photoreceptor pathways was strongly associated with pH and turbidity variability. This study improves our understanding of how natural selection and neutral evolution impact on the distribution of aquatic biodiversity from the understudied and ecologically complex Amazonia.
Introduction
Natural selection is a ground-breaking conceptual framework to our understanding of evolution (Darwin, 1859). However, challenges with empirically studying adaptive divergence in nature has ultimately led to a bias toward using spatial factors to explain evolutionary patterns (Wang and Bradburd, 2014). Selectively neutral models of population divergence include Isolation by Distance (IBD), where gene flow aligns with gradients of distance between populations (Wright, 1943; Sexton et al., 2014), and neutral hierarchical structure, where punctuated barriers restrict gene flow to particular geographic zones (Meirmans, 2012). When gene flow and differentiation better align with similar environments, an adaptive Isolation by Environment (IBE) model, where divergent natural selection acts on evolutionary advantageous phenotypes, might provide a more superior explanation for inferred patterns of population structure (Sexton et al., 2014; Wang and Bradburd, 2014).
The empirical challenge of clarifying patterns of adaptive divergence in wild populations can be addressed using “landscape genetics,” a multi-disciplinary study that relies on explicit statistical quantification of environmental and geographic variables to test for the relative influence of each on genetic variation across the landscape (Storfer et al., 2007). More recently, the arrival of “landscape genomics,” which utilizes genome-wide information to identify environmental features influencing genetic variation, has considerably increased the power to study local adaptation and adaptive divergence in wild populations—the initial outcomes of the process of divergent natural selection (Wang and Bradburd, 2014; Rellstab et al., 2015; Grummer et al., 2019). This includes assessment of a population’s ability to evolve and maintain fitness in response to environmental changes, or their “adaptive capacity,” an important issue in conservation management and applied evolution (Grummer et al., 2019). This is particularly timely as habitats across the globe are rapidly shifting in environmental properties, with many niches under notable threat (Thurman et al., 2020).
Limited research has explained how natural selection acts in aquatic environments (Kelley et al., 2016; Grummer et al., 2019). In rivers and streams, a constrained, unidirectional spatial flow of genes along well-defined migratory paths provides an ideal environment for the study of natural selection, as the impacts of geographic separation on genetic divergence can be controlled for (Hughes et al., 2009; Brauer et al., 2018; Attard et al., 2022). The Amazon, also known as Amazonia, holds the highest diversity of freshwater fishes in the world, yet little is understood about their evolution (Corlett and Primack, 2010; Beheregaray et al., 2014). Patterns of diversification in the Amazon have been mostly studied in the context of geographic isolation based on neutral genetic markers (Albert et al., 2011, 2020). Very few studies have assessed the role of natural selection in population divergence and evolution in the Amazon (reviewed in Beheregaray et al., 2014), an endeavor that requires information about adaptive genetic variation (Grummer et al., 2019).
The Amazon River network is composed of three distinctive river types with characteristic attributes resulting from the geological origin of water they contain, groundwater level, soil types, and vegetation (Val, 1995). Each water color shows a unique composition of pH, turbidity, water temperature and dissolved oxygen; thus “water color” can be used as an umbrella term to summarize differences in hydrochemical properties of water bodies. Variances in these hydrochemical parameters can drive constraints on the ecological communities inhabiting water bodies, and as such water color can be considered a major “aquatic ecotone” (Beheregaray et al., 2014). Water colors and their boundaries in the Amazon have been demonstrated to influence the distribution of forest floodplain avifauna (Laranjeiras et al., 2021) and the migratory paths of important fish species (McClain and Naiman, 2008).
From a landscape genetics perspective, several studies have investigated how genetic divergence in Amazonian fish populations might be influenced by water color (Cooke et al., 2012a,b,c, 2014; Beheregaray et al., 2014). This included the proposal of a framework capable of distinguishing the relative contributions of natural selection associated with aquatic ecotones vs. biogeographic history in the origin of fish population diversity (Beheregaray et al., 2014). These studies generally found support for water color as a selecting agent in Amazonian fish divergence. However, the limited number and the anonymous nature of the DNA markers used (amplified fragment length polymorphisms, AFLPs) offer little power to disentangle adaptive from neutral genetic variation (Luikart et al., 2003) and to gain insights about the functional relevance of putative adaptations (Grummer et al., 2019). Advancements in genome-wide screening technologies using single nucleotide polymorphisms (SNPs) has enabled a higher genotyping efficiency, data quality, analytical simplicity, and coverage across the genome (Luikart et al., 2003; Morin et al., 2004, 2009). This has allowed for greater power to differentiate adaptive from neutral genetic variation, enabling the identification of candidate loci influenced by natural selection (Vitalis et al., 2001; Morin et al., 2004, 2009; Seehausen et al., 2014).
This study focuses on the Amazonian benthopelagic fish Triportheus albus (Characiformes: Triporthidae) (Cope, 1871). Triportheus albus is a non-migratory species that has been recorded to grow up to 26 cm with a weight of up to 200 g (Giarrizzo et al., 2015). Little is known about the population dynamics and ecology of T. albus, a deficiency that applies to most other fish species in the Amazon (Prestes et al., 2010). This work aims to assess the relative contribution of spatial factors and environmental variation driving evolutionary divergence of T. albus, within the context of riverine “water color.” We hypothesize that, after controlling for spatial covariables, evolutionary divergence due to divergent selection should be stronger between populations of T. albus found in different ecotones than between populations from the same ecotone. This possibility derives from the strong physiological constraints that major hydrochemical and environmental gradients impose upon aquatic communities in Amazonia (Junk et al., 1983; Saint-Paul et al., 2000). To test this hypothesis, we generated a genome-wide dataset for T. albus populations that was integrated analytically with environmental and spatial datasets within a landscape genomics framework. We employed model-based and model-free approaches to assess neutral population differentiation and several Genotype-Environment Association (GEA) analyses to test how genetic variation might be associated with environmental predictors embodying different water colors. We also identify gene regions involved with adaptation to different water habitats and predict their role in driving natural selection. To the best of our knowledge, this is the first landscape genomics study for an Amazonian fish, providing valuable insights into how spatial and environmental factors simultaneously influence adaptation and population-level divergence within the ecologically rich Amazon Basin.
Materials and Methods
Sampling and Study Site
Our fieldwork explored over 1,100 km of total riverine distance in the Amazon Basin in February 2005 and in February 2008. Sampling was done at 10 sites representing five major river systems. These systems cover all three major hydrochemical environments of the Amazon Basin; the Amazon and the Madeira (white), the Negro (black), the Branco (seasonally black), and the Tapajós (clear) rivers (Figure 1). The white water Amazon River (known as Solimões upstream of Manaus) is central to the sampling transect and drains west to east, with the black water Negro, white water Madeira and clear water Tapajós rivers flowing into it. The black water portion of the Branco River that flows into the Negro was also included in the transect. Our sampling design consists of two ecological gradients, where black (Negro, Figure 1i) and clear (Tapajós, Figure 1ii) water meets white (Amazon) water, and two controls, where rivers of the same water color meet (Branco to Negro and Madeira to Amazon). Sampling was carried out in sandy and shallow beaches along the river banks and floodplains. This is the preferred habitat for T. albus (Figure 1iii), a species that has not been recorded in deeper river channels. Fish were caught with seine nets, euthanized, and muscle tissue preserved in 95% ethanol. Geographic coordinates of each site were obtained using a global positioning system (GPS). Measurements of pH, temperature (°C), turbidity (cm), dissolved oxygen (mg/L), and oxygen saturation (%) were collected in situ at each locality to assess environmental heterogeneity (Table 1).
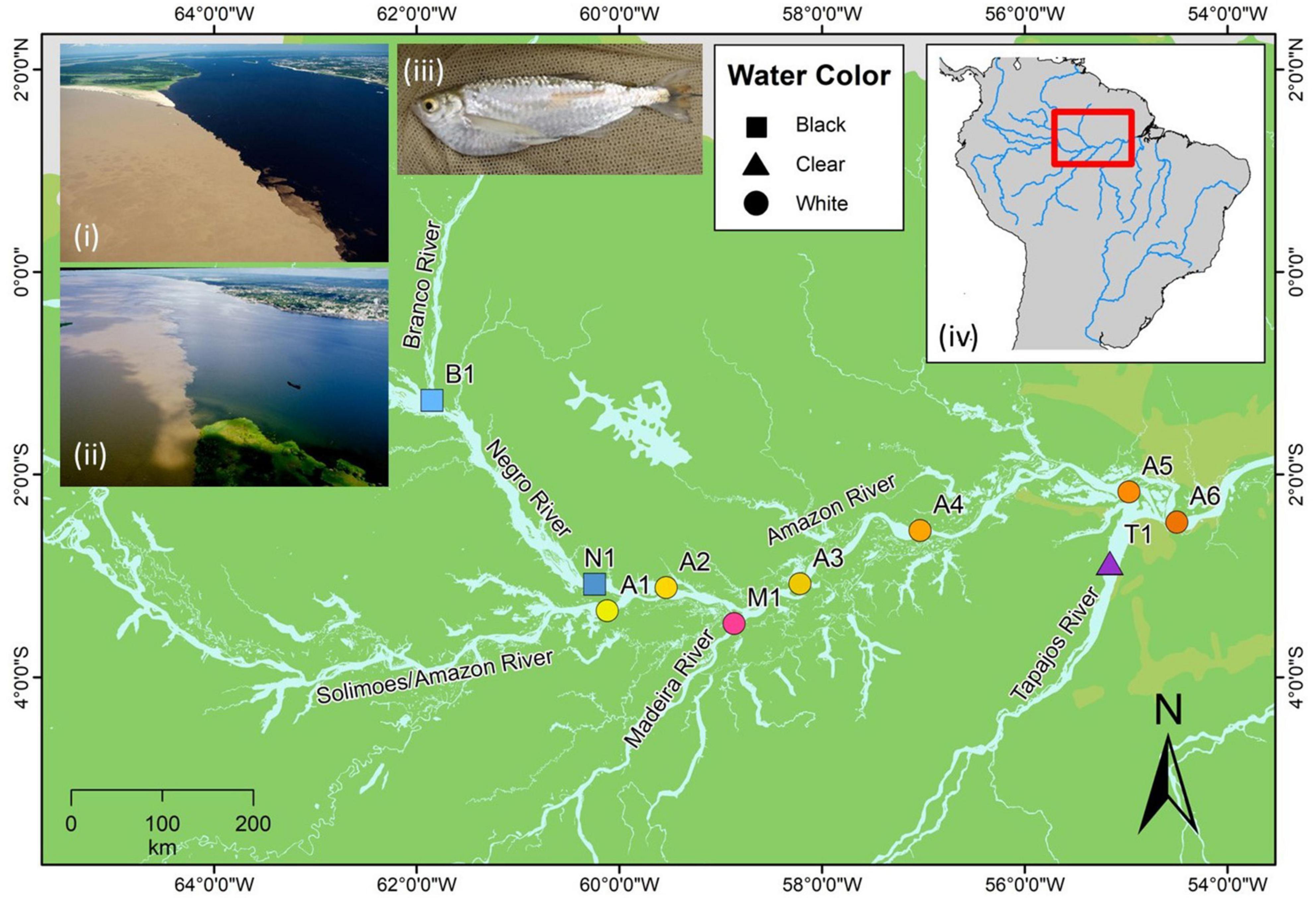
Figure 1. Sampling localities of Triportheus albus in the Amazon Basin. Shape of site points correspond to water color of the locality (square = black water, triangle = clear water, circle = white water). Black water sites are labeled in blue points (B1 = Branco, N1 = Negro), clear water in purple (T1 = Tapajós), the white water Amazon in a gradient of yellow to orange (A1–A6), and the white water Madeira in pink (M1). Inset (i) shows the confluence of the Amazon and Negro rivers, and (ii) shows the confluence of the Amazon and Tapajós rivers. Inset (iii) depicts the study species T. albus. Inset (iv) displays the study area in northern South America.
Laboratory Procedures
A modified salting-out protocol (Sunnucks and Hales, 1996) was used to extract DNA from muscle tissue samples. Double digest restriction-site associated DNA (ddRAD) libraries were developed using a modified protocol by Peterson et al. (2012), as detailed in Brauer et al. (2016). Libraries were sequenced at Novogene across two lanes of Illumina HiSeq4000 at 150 base-pair paired-end reads. Details of the DNA extraction method and the ddRAD library protocol are in Supplementary Appendix 1.
Bioinformatics and Single Nucleotide Polymorphism Filtering
Illumina HiSeq4000 sequencing produced a total of 902,555,657 sequence reads, each of around 150 bp. Quality control tests were initially performed using FASTQC 0.11.8 (Andrews, 2010). Sequences were demultiplexed and barcodes and adapters were removed using process_ragtags 2.4 from STACKS (Catchen et al., 2013). Low quality bases (Q < 20) and reads (more than 5% Ns, less than 40 bp, or < 30 average Q) were removed using TRIMMOMATIC 0.39 (Bolger et al., 2014), SNP variants were characterized using the DDOCENT 2.7.8 pipeline (Puritz et al., 2014), as detailed in Sandoval-Castillo et al. (2018). The SNPs were filtered in a pipeline of steps from VCFTOOLS (Danecek et al., 2011). A detailed summary of the filtering process is found in Supplementary Table 1.
Genetic Diversity and Population Structure
Percentage polymorphic loci, expected heterozygosity (He) and observed heterozygosity (Ho) were calculated across the filtered SNP dataset for each sampling locality using ARLEQUIN 3.5.2.2 (Excoffier and Lischer, 2010).
Population structure was assessed using model-based and model-free methods. Pairwise FST among sampling localities was estimated with the Jukes and Cantor (1969) method using 1,000 permutations. P-values were adjusted for a false discovery rate (FDR) of 5%, using the false discovery script.1 A principal component analysis (PCA) of the total SNP dataset was generated using the program ade4 (Dray and Dufour, 2007) in R. Population structure was also inferred using Admixture (Alexander et al., 2009). The number of ancestral populations (K) was assessed by comparing log-likelihood ratios for multiple independent runs of each K (K = 1–7) before using a cross-validation (CV) procedure with 10 replicates to identify the K-value that best explains the number of random mating populations (Alexander et al., 2009). Admixture coefficients were plotted using the ggplot2 package (Wickham, 2009) in R. Relationships among individual fish were then assessed based on the SNPs with a Neighbor-joining (NJ) tree in PAUP4 (Wilgenbusch and Swofford, 2003) and the GRT + G model (Tavaré, 1986), which best fitted our data according to the Bayesian information criterion implemented in ModelTest-NG (Darriba et al., 2020). The aim of this analysis was not to infer phylogenetic history in T. albus, but to instead obtain a visual representation of the relationships among individuals across localities that can be contrasted with results of other approaches used to infer population structure.
Controlling for Spatial Genetic Structure
Patterns of genetic structure are known to be influenced by the physical structure of river catchments, including the spatial separation between populations via IBD, and the hierarchical structure of the dendritic system (Excoffier et al., 2009; Fourcade et al., 2013; Brauer et al., 2018). Riverine distance between sampling localities was estimated using Google Earth Pro 7.3.3 (2020) to test if any inferred genetic differentiation is consistent with the IBD model. A global Mantel test based on 999 permutations was performed to assess correlation between riverine distance and linearized genetic distance between all localities (FST/1—FST) using the ade4 package in R (Dray and Dufour, 2007). A second Mantel test (999 permutations) exclusively assessed the relationship between riverine distance and linearized genetic distance of white water Amazon and Madeira River localities. Localities A5 and A6 were omitted from this analysis due to insufficient sample size. These relationships were visualized using regression plots. A pairwise matrix of riverine distances between localities was calculated and translated to a set of synthetic IBD coordinates for each locality in R using the isoMDS function in the MASS library (Venables and Ripley, 2002) for use as a control for IBD in subsequent GEA analyses.
Hierarchical structure can be identified through distinct breaks in allele frequencies between clusters of populations, which may coincide with geographical features such as river catchments (Meirmans, 2012). A matrix of population covariance across locality allele frequencies (Omega matrix) was calculated through the core model in the BayPass program [explained below under “Genotype-Environment Association (GEA) Analyses; Gautier, 2015]. This matrix infers gene flow resulting from elements of demographic history, including neutral models of hierarchical structure. This matrix was translated to a set of covariance variables using the MASS library (Venables and Ripley, 2002) in R. The Omega coordinates were used as a control for hierarchical structure in successive GEA analyses.
Genotype-Environment Association Analyses
Five hydrochemical variables sampled in situ were considered in the GEA analyses; pH, temperature (°C), turbidity (cm), dissolved oxygen (mg/L), and oxygen saturation (%) (Table 1). These variables have been suggested as possible sources of ecological selection in fishes between Amazonian water colors (Beheregaray et al., 2014; Borghezan et al., 2021). The association between genetic and environmental heterogeneity was assessed using two multivariate approaches, redundancy analysis (RDA) (Legendre and Legendre, 1998) and Gradient Forest (Ellis et al., 2012), as well as by the univariate approach in BayPass (Gautier, 2015).
BayPass is a program for Bayesian population association analyses (Gautier, 2015). This Bayesian hierarchical model was proposed by Coop et al. (2010) as an FST-based approach to evaluate associations between variation in ecological variables and genetic markers, and to identify candidate loci for adaptive divergence while controlling for neutral covariance of alleles across populations. BayPass was used to investigate the signal of neutral and adaptive variation through three models; the core model, the standard covariate model, and the auxiliary model (Günther and Coop, 2013). Explanations of these three models are in Supplementary Appendix 2. The auxiliary model was used to identify candidate adaptive loci significantly correlated with each environmental variable over a log10(BF) threshold of 30.
The RDA is an asymmetric ordination method used to explore the relationship between multivariate response data and a set of explanatory variables using multiple linear regression (Legendre and Legendre, 1998). The RDA has been shown as a robust method to identify polygenic adaptive divergence through a strong ability to detect minor changes in allele frequency over many covarying loci (Grummer et al., 2019), while providing an optimal balance of low false-positive and high true-positive rates (Forester et al., 2018). An RDA was carried out using the vegan package in R studio (Dixon, 2003) comparing the response allele frequencies for each SNP with the five environmental variables (Table 1). To avoid collinearity, highly correlated variables were excluded using a variance inflation factor ≥10 (Dyer et al., 2010). First, we performed a standard RDA comparing variance in allele frequencies with variance in environmental variables. Subsequently, two partial RDAs were performed accounting for different aspects of spatial neutral population structure. These included synthetic IBD coordinates as a conditional variable and the synthetic Omega coordinates (see “Controlling for spatial genetic structure” section above) to control for other elements of demographic history, including hierarchical structure. A hierarchical analysis of molecular variance (AMOVA) and marginal AMOVA were performed to determine the significance of the partial and non-partial RDA models under a 0.05 threshold at 1,000 permutations in the R package vegan (Dixon, 2003). The SNPs exhibiting high contribution to environmental associations in the RDA (p = 0.05) were identified as candidate loci for adaptive divergence.
Random Forest (Breiman, 2001) is a machine-learning regression tree technique that can effectively handle thousands of DNA markers simultaneously to identify regions of the genome accounting for complex polygenic traits (Brieuc et al., 2018; Grummer et al., 2019). The original randomForest package was developed by Liaw and Wiener (2002). This was later modified by Ellis et al. (2012) into the gradientForest package, which extends the random forest method to the community level. Gradient Forest can pinpoint where compositional turnover occurs along an environmental gradient, which allows identification of important environmental thresholds that correlate to distinct changes in allele frequencies (Ellis et al., 2012). Gradient Forest was run to determine the correlation of allele frequencies with the predictor variables of environmental variation, synthetic IBD coordinates, and synthetic Omega coordinates (ntree = 750, mtry = number of variables/3, corr. threshold = 0.5). The cumulative importance of each predictor variable in shaping genetic populations was also assessed, with threshold values of each predictor in determining allele frequency breaks identified. Candidate adaptive loci were identified by assessing the R2 weighted importance distribution of SNPs (Supplementary Figure 7). Loci above the upper elbow of this distribution curve (>0.86) were selected as candidates. As there is not a specific method implemented in random forest analyses to extract outlier SNPs (Goldstein et al., 2011; Laporte et al., 2016), the upper elbow approach used above has proven valuable in genome-wide association studies (Batley et al., 2019).
Functional Annotation
Candidate adaptive SNPs identified through RDA, Gradient Forest and BayPass methods were compared to select loci for functional analysis. The flanking sequence of each candidate SNP was aligned to the UniProt2 Actinopterygii protein database using BLASTX 2.11.0 + (Altschul et al., 1997) with e-value threshold set to 1 × 10––6. Gene ontology (GO) terms were assigned with a modified version of the python script GAWN.3 The functional roles of annotated candidate loci were investigated further by exploring the relevant literature.
Results
Sequencing Quality and Genetic Diversity
The SNP calling resulted in a total dataset of 743,123 raw SNP variants. After the filtering process (detailed in Supplementary Table 1), 15,251 high-quality SNPs were retained for analysis in 98 T. albus individuals (including four replicates). Genotyping error rates for the dataset were < 1%, with ∼0.05% missing data.
Adjacent Amazon River (Amazonas) localities with small sample sizes (A4, A5, and A6) were merged into a single sample to estimate genetic diversity. Genetic diversity was high and similar among localities, with average expected heterozygosity of 0.263 (He; 0.252–0.300), average observed heterozygosity of 0.240 (Ho; 0.221–0.275), and average polymorphic loci of 64.9% (54.4–76.1%) (Supplementary Table 2).
Population Structure
Population structure was strong across the region sampled in the Amazon Basin. In general, genetic differentiation among localities was highest between white (A1–A6, M1) and black (B1, N1) localities, and between white and clear (T1) localities. Genetic differentiation was nil to low between localities within the white and black selective environment (Supplementary Table 4). Pairwise FST estimates were significant (p < 0.05) after 5% FDR correction between black water localities, between black and clear localities, and between black or clear and white water localities. Pairwise FST estimates between white water localities were not significant (p < 0.05) after 5% FDR correction (Supplementary Table 4).
For the PCA and subsequent GEA analyses, Amazon River localities A5 and A6 were merged to a single sample (n = 4), with A4 (n = 6) kept as a separate sample. The PCA plot (Figure 2i) supported the pattern of population differentiation estimated via FST, showing distinct clusters of individuals among white, black, and clear water. A stronger level of separation was evident between white water T. albus and individuals from black and clear water localities, as explained by PC1 (17.412%). Clustering was also observable among black and clear water localities, explained by PC2 (2.871%)(Figure 2i). Two putative hybrids between white and clear water clusters were observed, originating from sites T1 and A5/A6. Possible migrants were also detected between black and white water, and between the clear water Tapajós and white water cluster (Figure 2i).
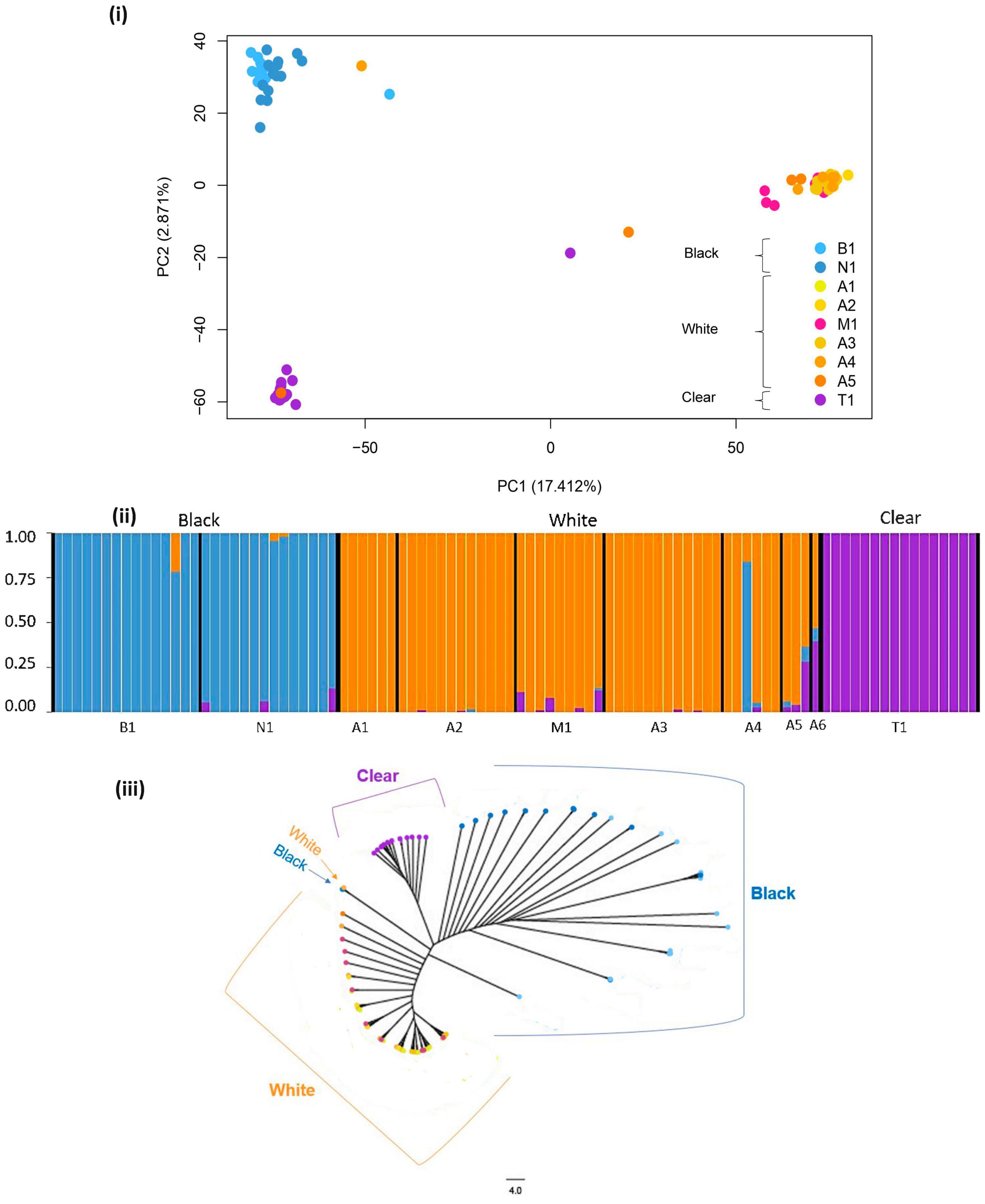
Figure 2. Population genomic structure of Triportheus albus based on 15,251 SNPs. Site labels are described as B1, Rio Branco (black water); N1, Rio Negro (black water); A1–A6, Rio Amazonas (white water); M1, Rio Madeira (white water); T1, Rio Tapajós (clear water). (i) PCA plot with each point indicating an individual and color a sampled site (shades of blue = black water sites, shades of orange = white water sites, purple = clear water site); (ii) admixture plot with each vertical bar depicting an individual and color representing ancestry from each water color catchment (blue = black water, orange = white water, purple = clear water). (iii) Neighbor-joining tree generated with PAUP4 based on the GRT + G substitution model. Catchment water color is labeled.
The Admixture analysis provided highest support for two genetic populations of T. albus, closely followed by three populations, as indicated by CV errors displayed in Supplementary Figure 1. The K = 2 plot combined the geographically distant black and the clear water samples into a single genetic population, with the second population represented by white water samples (Supplementary Figure 2). The K = 3 plot divided the black and clear catchments into two separate populations (Figure 2ii). An individual caught at A4 had ancestry to the black water population, supporting its migrant status suggested by the PCA. Two individuals from sites A5 and A6 shared ancestry between white and clear water populations, suggesting the possibility of an admixture zone (Figure 2ii).
The topology of the NJ tree was in agreement with results of population structure analyses (Figure 2iii). Individuals from the clear water Tapajós clustered as a single clade, whereas individuals from black and white water sites also comprised separate clades. Three individuals deviated from this pattern; one from white water A4, and one from each of the black water sites B1 and N1 (Figure 2iii). The outlier in site A4 corresponded to the potential migrant identified in the PCA and Admixture, supporting its suggested ancestry from the black water tributary. Potential hybrids from sites A5 and A6 identified in PCA and Admixture population structure analyses grouped closely to the clear water cluster in the NJ tree (Figure 2iii).
Genotype-Environment Associations and Controls for Models of Neutral Structure
The composition of environmental variables varied substantially between rivers of different water “color” (Table 1), and as such these are considered here as predictors of putative adaptive divergence within T. albus. The pH was considerably lower in the Negro River (5.2) than all other localities (6.7–7.2), including the seasonally black Branco River (6.9) (Table 1). Turbidity was substantially higher in the white water Amazon (14.5 cm) and Madeira (5.5 cm) sites compared to both black (79.7 cm) and clear (118 cm) water sites. Temperature remained relatively consistent across all systems. Dissolved oxygen was lowest at Amazon 3 (4.8 mg/L) and highest in the clear water Tapajós (7.1 mg/L). Oxygen saturation was greatest in the Tapajós (97.5%) and lowest at Amazon 5 (82.0%).
The Mantel test performed globally across white, black and clear water localities was not significant (p = 0.14), suggesting a relatively low effect of IBD at the broader regional scale. This was reflected in the global matrix regression plot (Supplementary Figure 3i) which shows no distinct pattern between riverine distance and FST among T. albus populations. The Mantel test among white water localities was also not significant (p = 0.09), although a trend of increasing genetic distance with geographic distance was observable in the regression plot (Supplementary Figure 3ii).
After controlling for highly correlated environmental predictors, pH and turbidity were included in the RDA model. The standard RDA separated individuals from white water localities from those in black and clear water sites (Figure 3i). This RDA model indicated that pH and turbidity accounted for 48.2% of total genetic variation and was globally significant (p = 0.010) (Supplementary Table 6). After controlling for IBD, which explained 37.7% of variation, pH and turbidity accounted for 32.0%, with the RDA model being globally significant (p = 0.036) (Supplementary Table 6 and Supplementary Figure 4). A clear pattern was less apparent in the RDA plot controlled by Omega (Figure 3ii). In that model, turbidity and pH explained 15.9% of the total genetic variation after accounting for demographic history (including hierarchical structure), which explained 53.9% of variation, with the RDA model not being significant (p = 0.384). From the RDA model conditional to Omega, we identified 1,749 candidate adaptive loci which associated highly with environmental predictors.
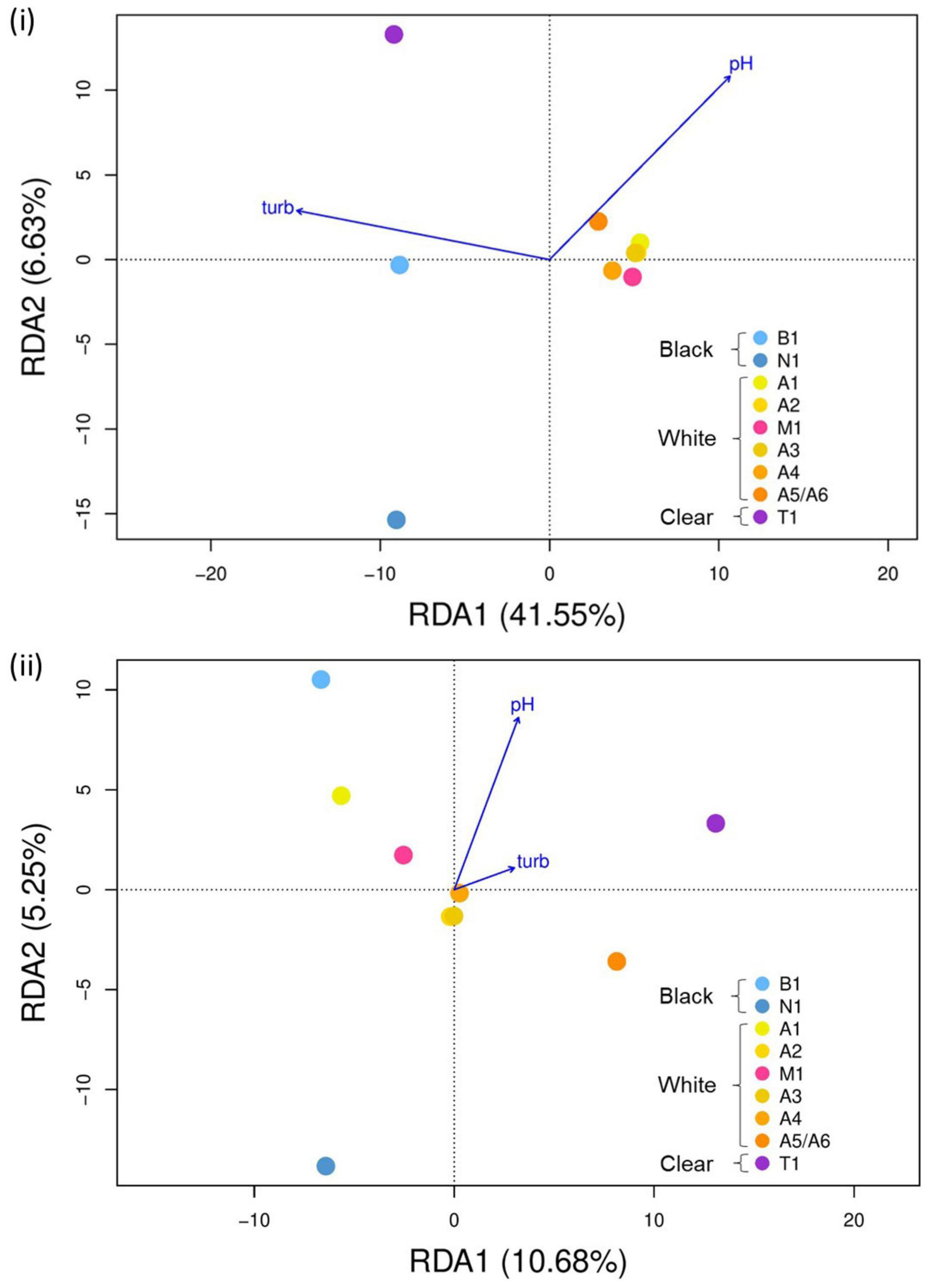
Figure 3. Genotype-environment associations of T. albus in the Amazon Basin based on RDA. The RDA shows how genetic differentiation between localities is explained by non-correlated environmental variables pH and turbidity (turb) collected in situ at each site. (i) Unconstrained, without controlling for spatial structure, and (ii) constrained to population covariance calculated with BayPass (Gautier, 2015) to control for neutral hierarchical genetic structure. Each colored point represents a sampling locality. Black water Branco River (B1) and Negro River (N1) localities = shades of blue, white water Amazon River localities = gradient of yellow to orange (A1–A5), white water Madeira River (M1) = pink, clear water Tapajós River (T1) = purple. Based on 15,251 filtered SNP loci.
The Gradient Forest identified that Omega 2, pH, Omega 1, and turbidity all correlated significantly with allele frequency at a similar level to one another (Figure 4i). The synthetic IBD coordinates, dissolved oxygen, temperature, and oxygen saturation did not correlate highly with allele frequency (Figure 4i). Demographic history predictors Omega 1 and 2 were the strongest in influencing breaks in allele frequencies of T. albus across Amazonia (Supplementary Figure 5). This was closely followed by turbidity and pH, which both influenced compositional turnover of allele frequency to a high level (Supplementary Figure 5). Turbidity classified one distinct compositional turnover in allele frequency, defined by ∼42 cm (Figure 4ii), which separated white water localities from black and clear water localities (Table 1). The pH defined two allele frequency breaks occurring at threshold pH values of 6.2 and 7.0 (Figure 4iii), which generally corresponded to pH differences between each of the three water colors (Table 1). The Branco River (pH 6.9; Table 1) was an outlier to this pattern, grouping with the clear water population. Dissolved oxygen, oxygen saturation and temperature did not substantially define any turnover in allele frequency (Supplementary Figure 6). The Gradient Forest identified 53 candidate adaptive loci associated with environmental variables over threshold R2C value 0.86.
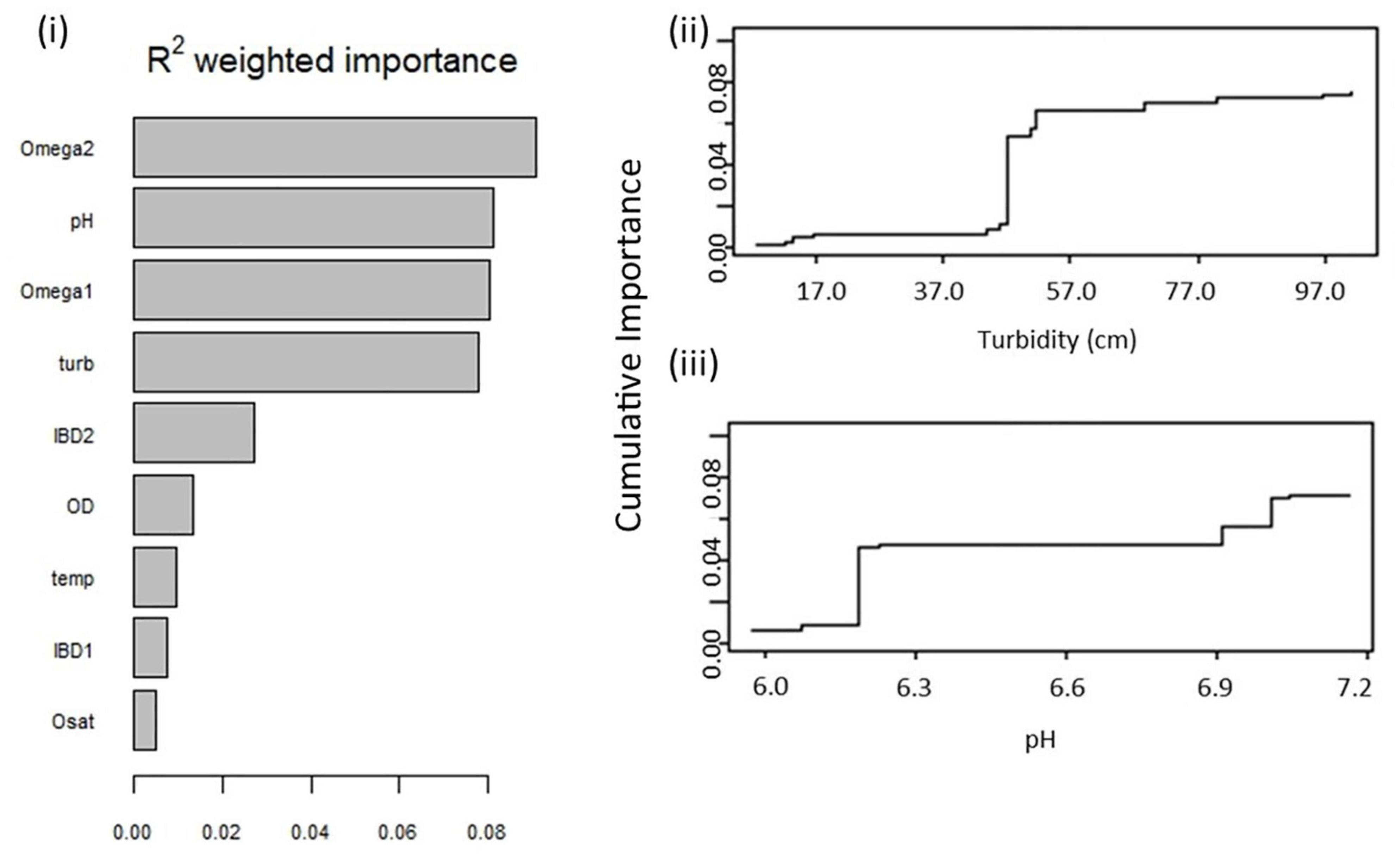
Figure 4. Gradient Forest (Ellis et al., 2012) results for associations between environmental variables and genetic diversity of Triportheus albus across the Amazon Basin. Based on 15,251 genome-wide SNPs. R2 weighted importance (i) clarifies the level of correlation between each predictor variable and allele frequency variation. Omega 1 and Omega 2 denote control variables of neutral hierarchical structure, measured through a matrix of population covariance translated to two synthetic coordinates. The IBD1 and IBD2 are controls of isolation by distance, measured using a matrix of geographic distance between localities. Turbidity (turb), pH, dissolved oxygen (OD), oxygen saturation (Osat), and temperature (temp) are hydrochemical variables measured in situ at each sampling locality of T. albus. Cumulative importance displays the allele frequency compositional turnover functions for important environmental predictor variables of Triportheus albus divergence across the Amazon Basin, turbidity (ii) and pH (iii).
The auxiliary model in BayPass (Gautier, 2015) identified 268 candidate adaptive loci, mostly associated with pH (67) (Supplementary Figure 8). Of the 1,749 candidate loci identified by RDA and 268 by BayPass, 53 loci were consistent between the two methods (Supplementary Table 1). The 53 candidate SNPs identified by Gradient Forest were not discovered by RDA or BayPass as outliers (Supplementary Table 1).
Functional Annotation
For the 53 unique candidate loci identified by both BayPass and RDA, and the 53 loci identified by Gradient Forest, 29 were annotated and assigned to 58 GO terms. Many genes annotated were components of cellular membranes, with the most common GO terms “integral component of the membrane” and “plasma membrane” (10 and four of 29 annotated genes, respectively). Twelve of these 14 genes had molecular functions associated with the binding of ions, nucleic acids, or ATP. The most common biological processes identified were related to signaling pathways (four of 29 annotated genes) (Supplementary Table 7). Most candidates were highly associated with pH (14 of 23) or turbidity (7 of 23) (Supplementary Table 7). Further information about GO Terms assigned to genes, environmental variables that genes were associated to, and regression coefficients of the correlation between genetic variation and variation in the associated environmental variables are reported in Supplementary Table 7.
Discussion
Disentangling adaptive from neutral genetic diversity in wild populations is a difficult task, but the ease and power to do so has improved substantially with the development of genome-wide screening techniques and approaches in landscape genomics (Yeaman et al., 2016; Grummer et al., 2019). Here, we were able to identify the contributions of spatial and environmental factors in shaping genomic divergence in populations of the characin tetra T. albus from central Amazonia. Using a robust approach that integrated multivariate RDA and Gradient Forest, and univariate BayPass approaches, we have demonstrated that in addition to strong neutral hierarchical structure, adaptive divergence is likely to be occurring in T. albus populations across black, white and clear water selective environments.
Evolution and Population Genomic Structure
Our study is among the first aimed at assessing population genomic structure of an Amazonian aquatic species using genome-wide approaches. To the best of our knowledge, a study by Torati et al. (2019) examining stock structure in the teleost Arapaima gigas between South American basins using 393 SNPs represents the only prior attempt to this. Landscape genetic methods have been used to identify population structure among Amazonian catchments for the characin T. albus (Cooke et al., 2012a), the silver croaker Plagioscion squamosissimus (Cooke et al., 2012b), the riverine puffer Colomesus asellus (Cooke et al., 2012c), and the barred knife fish Steatogenys elegans (Cooke et al., 2014). Genetic structure between Amazon and Madeira River populations has also been identified for the catfish Brachyplatystoma platynemum (Ochoa et al., 2015). None of these studies have employed datasets and statistical approaches capable of inferring genome-wide neutral and adaptive signal (Luikart et al., 2003; Grummer et al., 2019). As such, comparisons of genome-wide diversity with our T. albus dataset are hampered by the remarkably small number of aquatic studies (including for characiform tetras from other basins). For instance, for the least-concern (IUCN) characiform Astyanax mexicanus (blind cavefish) in Northeast Mexico (Bradic et al., 2013), population-level observed heterozygosity ranged from 0.05 to 0.19. For the abundant atheriniform Melanotaenia fluviatilis (Murray River rainbowfish) in Australia, heterozygosity values ranged from 0.111 to 0.317 (Brauer et al., 2018). For our study, genetic diversity was not only moderate to high but was markedly similar across all sampled sites (observed heterozygosity ranged from 0.221 to 0.275), suggesting high adaptive potential for the inferred populations in our study region.
When assessing evolutionary patterns of freshwater biodiversity it is important to consider the history and timing of geological formation of river basins. The Characiformes originated over 100 million years ago (Ma) in Gondwana (Ortí and Meyer, 1997), and as such deep evolutionary divergence in the Neotropics should be assessed in relation to the uplift of the Andes and the initial development of the Amazon drainage basin (Rull, 2008; Hoorn et al., 2010a). Prior to the influence of the Andes, the Amazon was an undivided sedimentary basin dominated by the fluvial system of the cratonic shields of which the Negro and Tapajós rivers are derived from Figueiredo et al. (2009) and Hoorn et al. (2010b). Increased sedimentation, rising sea levels, and overfilling from the late Miocene (∼6.4 Ma) led to the establishment of west to east transcontinental flow and formation of the modern Andean-dominated Amazon River network by the late Pliocene (∼2.5 Ma) (Val, 1995; Campbell et al., 2006; Figueiredo et al., 2009).
Geomorphological evolution is thought to have impacted the phylogeography of T. albus. The work by Cooke et al. (2012a) based on 360 AFLP loci supported two historically distinct populations of T. albus in central Amazon; a white water Amazonas/Madeira population, and a black water Negro and clear water Tapajós population. Phylogenetic and phylogeographic analyses indicated a recent divergence between white water and black/clear water ecotypes of T. albus followed by demographic expansions dated to the late Pleistocene (Cooke et al., 2012a). These findings, paired with geomorphological and paleoecological evidence for the basin, indicate that the black/clear water ecotype is older, with the west to east transcontinental formation of the Amazon River promoting the colonization and demographic expansion of the white water T. albus ecotype (Lundberg et al., 1998; Campbell et al., 2006; Cooke et al., 2012a). This scenario is supported by comparative phylogeographic studies of co-distributed fishes; Colomesus asellus, Plagioscion squamosissimus, and Steatogenys elegans (Cooke et al., 2012b,c, 2014). Although assessing phylogeographic history of T. albus is outside the scope of this study, our results support the strong divergence between white and black/clear ecotypes, as well as the similarity between black and clear water populations detected by Cooke et al. (2012a). The latter was found despite the large contemporary riverine distance between the black water Negro and the clear water Tapajós (up to 1,100 km) and their different geological settings (Latrubesse and Franzinelli, 2005; Hoorn et al., 2010b), pointing to the strong influence of phylogeographic history (Cooke et al., 2012a) on contemporary patterns of population structure in T. albus.
In addition, the greater power offered by our genome-wide dataset revealed weaker, but biologically meaningful, genomic divergence between black and clear water populations. Only a low level of admixture was noted between black and white, and between clear and white populations, suggesting downstream migration following the direction of river flow; from tributaries into the main Amazon channel (Figure 2ii). This is consistent with the isolation with migration model results of Cooke et al. (2012a), which inferred a higher probability of unidirectional gene flow in T. albus from black and clear water into white water than of any other alternative scenario. Overall, population structure analyses indicated three contemporary genetic clusters for T. albus in central Amazonia that correspond to rivers of distinct water color. As genetic structure was not observed between the white water Amazon and Madeira rivers, divergence due to the confluence of a tributary alone remains an unsupported explanation. Similarly, a simple model of IBD seems insufficient to explain divergence patterns across the vast study region. Instead, our study results suggest that population divergence is also associated with the boundaries of catchments that harbor divergent hydrochemical conditions.
Neutral and Adaptive Contributions to Genomic Divergence
Species that inhabit contrasting selective environments provide a valuable opportunity to study divergent natural selection (Schluter, 2000). The dendritic arrangement of the Amazon Basin encompasses distinct ecotones of varying environmental conditions characterizing different water “colors,” which may theoretically promote divergent selection and IBE across populations of aquatic organisms. For instance, Pires et al. (2018) described divergence and reproductive isolation between Amazon and Negro River lineages of the sailfin tetra Crenuchus spilurus. A landscape genetics study by Cooke et al. (2012a) suggested a putative pattern of IBE for T. albus across Amazonia, as well as several co-inhabiting fishes (Cooke et al., 2012b,c, 2014; Beheregaray et al., 2014). In those studies, evidence for putative divergent selection lay in patterns of diversification that aligned best with catchments of similar water properties, irrespective of riverine distance. The genomic dataset used in this study not only provides comparatively higher resolution than the above genetic datasets to infer fine- and broad-scale population divergences (Luikart et al., 2003), but also contains sequence information around candidate adaptive regions that might prove useful to identify mechanisms influenced by divergent selection.
The IBD model is not uncommon in freshwater systems and has been observed for species with varying dispersal potential (e.g., Hrbek et al., 2005; Hubert et al., 2007; Zieritz et al., 2010; Crookes and Shaw, 2016). The GEA analyses strongly indicated that genetic divergence among T. albus populations cannot be explained by a simple IBD model. Broadly speaking, we found no clear relationship between genetic and riverine distance across the entire study area in central Amazon, which was also reflected in both the RDA and Gradient Forest results. A strong pattern of genetic structure was found between white water and black/clear water localities, but not between white water Amazon and Madeira Rivers. Similarly, very low differentiation and a low but non-significant signal of IBD was present along the Amazon River, despite the ∼750 km separating sites A1 and A6. Critically, the low levels of neutral differentiation and corresponding reduced genetic drift along white waters are not expected to impact on our ability to characterize putative adaptive divergence within that selective environment (Grummer et al., 2019).
The stream hierarchy model (Meffe and Vrijenhoek, 1988) should be routinely considered when assessing gene flow among populations in complex dendritic spatial environments (Brauer et al., 2018). Consistent with the broad inferences of population structure, the RDA analysis demonstrated hierarchical structure as the prevailing factor driving genetic divergence among populations (Figure 3ii). Although pH and turbidity explained a substantial proportion of the variance in all RDAs, their contributions were not statistically significant as model complexity increased following the addition of demographic and spatial factors, such as hierarchical structure (Supplementary Table 6). This is expected due to the complex links between spatial-demographic factors and environmental variables and the resulting difficulties in separating adaptive from neutral variation (Grummer et al., 2019). As such, analyses did not suitably disentangle whether divergence followed a neutral hierarchical pattern resulting exclusively from the arrangement of tributaries, or a combination of neutral and non-neutral influences due to the varying environmental conditions within each of the catchments.
The Gradient Forest analysis also revealed neutral hierarchical structure as the greatest driver of genetic differentiation, however, turbidity and pH were revealed to correlate with allele frequency at comparably high levels (Figure 4i). Heterogeneity in turbidity created two distinct genetic populations of T. albus, separating the white water localities from the black and clear sites (Figure 4ii). The white Amazonian headwaters are subject to copious erosion in the Andes, and are consequently rich in suspended sediments (Sioli, 1984; Val, 1995; McClain and Naiman, 2008). Associated with this heavy sediment load is an abundance of nutrients and organic matter which facilitate a higher productivity and diversity of species in white waters than adjoining black and clear tributaries (McClain and Naiman, 2008; Hoorn et al., 2010a). However, characiformes are generally visually-orientated, surface-dwelling diurnal fishes (Tejerina-Garro et al., 1998), and consequently may be offered an ecological advantage in the transparent, mineral-deficient black and clear water tributaries (Wallace, 1854; Furch, 1984; McClain and Naiman, 2008). A detailed proposal of how Amazonian water types may promote differentiation in the sensory mechanisms of fish has been outlined by Borghezan et al. (2021).
Variation in pH across the basin is associated with the split T. albus into three distinct genetic populations corresponding to each water color (Figure 4iii and Table 1). The dark color of the Negro River is attributed to staining from tannins and humic acids leached by decaying vegetation (Wallace, 1854; McClain and Naiman, 2008), and as a result exhibits lower pH than their pH-neutral white and clear counterparts. The acidic, nutrient-poor properties of black water systems forms a challenging environment for aquatic species (Val, 1995), however, research has suggested that phenotypic plasticity of mechanisms conferring acid-base regulation has enabled T. albus to survive in harsh Negro waters (Araújo et al., 2017). The Branco River has white headwaters of mountainous origin, however, sampling took place near its junction with the Negro, thus this site was heavily influenced by cratonic black water. Even so, geochemical analyses have revealed the Branco River to be chemically and sedimentologically intermediate between black and white water (Küchler et al., 2000; Evangelista and Tosi, 2015). The clear water Tapajós represents a second intermediate physiochemical condition to the Amazon and Negro rivers (Duncan and Fernandes, 2010), hence why the pH of the Branco and Tapajós tributaries are similar. However, the influence of environmental discontinuities on population divergence presented by the Tapajós should be cautiously interpreted given the lack of replication in this river system.
A key limitation of the study was that sample size was small in terms of individuals for some white water sites, and in terms of locality replication for the Tapajós tributary. However, simulations by Gaughran et al. (2018) demonstrated that accurate estimates of genetic differentiation in highly structured populations can be obtained using thousands of SNPs and only 2–5 individuals per locality. Thus, merging sites A5 and A6 for analyses, should be sufficient to provide a reasonable representation of their genetic patterns, and is justified by their genetic similarity identified in population structure analyses. A greater number of sampled sites in the Tapajós would provide an improved understanding of the structure of the clear water tributary as a whole. The study was also limited as a whole genome reference is not available for T. albus; the reference of a closely related species would help to better characterize regions under selection. In addition, the hypothesis would be better tested by performing a comparative analysis on multiple co-distributed species, as done by Beheregaray et al. (2014) using a landscape genetics framework.
The discovery of 106 candidate loci highly associated with variation in the environment provides further support for adaptive divergent selection of T. albus across the Amazon Basin. Although no Gradient Forest candidates were identified as outliers in the RDA or BayPass analyses, it is important to consider that methods of outlier detection all vary in assumptions and balance between low false positive and high true positive rates (Narum and Hess, 2011). The strong associations of pH and turbidity with allele frequency that correspond to environmental differences between each water color, and the discovery of candidate adaptive genes for environmental associations, are supportive that characteristics of water color can perhaps induce selection and promote adaptive divergence between populations.
Functions of Candidate Adaptive Genes
Establishing the function of candidate adaptive genes and their ecological importance should be done cautiously, as a large fraction of genes remains without annotation of ecological relevance (Pavey et al., 2012). The correlation of potentially adaptive genes with mechanisms linked to mediation of the impacts of environmental fluctuations do not necessarily imply causation, but nevertheless can be useful to understand how adaptive divergence may arise (Grummer et al., 2019). Most candidates identified here were annotated to genes associated with ion channel activity (Supplementary Table 7), which is consistent with up-regulated GO terms for T. albus reported by Araújo et al. (2017). As aquatic environments are composed of a wide range of salinities, ion compositions, and pH values, fish are required to cope with challenging osmotic and ionic gradients (Hwang and Lee, 2007). Ion channels can be sensitive to changes in extracellular pH (Holzer, 2003), and fluxes of ions play an important role in maintaining acid-base homeostasis in freshwater fish (Goss et al., 1992). The black water Rio Negro drains the nutrient-poor soils of the Amazon forest, and consequently contains low concentrations of nutrients such as Na+, Cl–, K+, Ca2+, and Mg2+ (Furch, 1984; Val, 1995). The gene coding for the K+ channel protein KCNK1 was identified in T. albus to be associated with environmental fluctuations across the study region, specifically in relation to pH in the RDA (Supplementary Table 7). The KCNK1 protein was found to be sensitive to low pH in zebrafish (Christensen et al., 2016), alluding that its function in T. albus may be sensitive to the strong fluctuations in pH across Amazonia.
Diurnal fishes such as the Characiformes depend highly on vision as a source of sensory information, and as a result have large, well-developed eyes (Guthrie, 1986; Tejerina-Garro et al., 1998). There is ample research demonstrating the effects of turbidity and light intensity on the feeding ability (Gardner, 1981; Rowe and Dean, 1998; Leahy et al., 2011), and antipredator behavior (Higham et al., 2015; Kimbell and Morrell, 2015) of fishes. Calcium ions and stores play an important role in the detection and transfer of light stimuli in photoreceptors (Krizaj and Copenhagen, 2002; Križaj, 2012). The ryanodine receptors (RYRs) are a family of Ca2+ release channels (Sutko and Airey, 1996) expressed in the photoreceptors of vertebrates (Križaj et al., 2004; Križaj, 2012). Photoreceptor pathways are sensitive to light (Križaj et al., 2011), and the RYRs have been linked to visual stimuli response in fish (Frank et al., 2019). The genes RYR2 and RYR3 were highly associated with environmental heterogeneity in T. albus, with RYR3 most highly correlated with variation in turbidity in the Gradient Forest analysis. Considering this, differences in light intensity produced by varying turbidity across Amazonia may be impacting the expression of the RYRs in T. albus, supporting sensory systems research directions suggested by Borghezan et al. (2021).
Fluctuations in dissolved oxygen is an issue in many aquatic systems including the Amazon (Junk et al., 1983) and can influence species distributions (Mandic et al., 2009) and cause negative impacts on freshwater fishes (Landman et al., 2005). Hypoxia is a condition in which cells suffer from oxygen deficiency (Hughes, 1973). Hypoxia has been found to affect the RYR2 gene in the large yellow croaker Larimichthys crocea (Mu et al., 2020). This gene was statistically associated to variation in oxygen saturation in T. albus (Supplementary Table 7). The connections of candidate genes to functions sensitive to environmental heterogeneity support the role of adaptive divergence in the evolution of T. albus across catchments of different water conditions.
Conclusion
By disentangling signal of adaptive and neutral divergence in T. albus across Amazonia using a novel landscape genomics framework, we have identified genetically distinct populations in black, white and clear water catchments of the Amazon Basin. The dominant driver of population divergence across the basin was hierarchical structure, which is expected in dendritic river systems (Brauer et al., 2018). However, pH and turbidity were identified as having a significant secondary influence on genetic patterns across the system, which was supported by the discovery of candidate adaptive genes with functions linked to mediating the biological impacts of hydrochemical fluctuations. Thus, pH and turbidity, which are of distinctive compositions in contrasting water colors, may be important agents of divergent natural selection. In spite of the known limitations of genome scans of selection (reviewed in Grummer et al., 2019), this study has improved our understanding of the evolutionary processes operating in a complex tropical system, which is vastly understudied in proportion to its immense biodiversity. The common IBD model appears as not being sufficient to explain broad patterns of genetic variation in this system. Importantly, the notable impact of IBE in freshwater fishes suggests that changing hydrological conditions of the Amazon Basin may have implications to their distribution and persistence. Understanding genomic vulnerability of Neotropical fishes amidst accelerated environmental and climatic change may be a valuable avenue for future research and would further help to inform on conservation management strategies for this diverse group of vertebrates.
Data Availability Statement
The datasets presented in this study can be found in online repositories. The names of the repository/repositories and accession number(s) can be found at: Figshare (https://figshare.com/articles/dataset/_/17109146).
Ethics Statement
The animal study was reviewed and approved by the Macquarie University Animal Welfare Committee 2007/033.
Author Contributions
AH carried out the molecular laboratory work, performed the data analyses, and wrote the first manuscript draft. JS-C provided technical and analytical advice, supervised the laboratory work and data analyses, and made suggestions for the manuscript. GC carried out fieldwork and laboratory work. NC carried out fieldwork and provided guidance about project design. LB conceptualized and designed the project, obtained funding, carried out fieldwork, provided technical and analytical advice, critically reviewed, and edited the manuscript. All authors contributed to the article and approved the submitted version.
Funding
This project was funded by the Discovery Program of the Australian Research Council (ARC DP0556496 to LB). Logistics and local arrangements were also supported in part through the Brazilian National Council of Research and Technology CNPq- SEAP 408782/2006-4 via NC (formerly at the Universidade Federal do Amazonas), and by Macquarie University through a postgraduate travel grant and research award to GC. Collection permit is under IBAMA No. 1920550, and ethical approval was received from Macquarie University (2007/033).
Conflict of Interest
The authors declare that the research was conducted in the absence of any commercial or financial relationships that could be construed as a potential conflict of interest.
Publisher’s Note
All claims expressed in this article are solely those of the authors and do not necessarily represent those of their affiliated organizations, or those of the publisher, the editors and the reviewers. Any product that may be evaluated in this article, or claim that may be made by its manufacturer, is not guaranteed or endorsed by the publisher.
Supplementary Material
The Supplementary Material for this article can be found online at: https://www.frontiersin.org/articles/10.3389/fevo.2022.825406/full#supplementary-material
Footnotes
- ^ https://github.com/carbocation/falsediscovery
- ^ https://www.uniprot.org/uniprot/
- ^ https://github.com/enormandeau/gawn
References
Albert, J. S., Carvalho, T. P., Petry, P., Holder, M. A., Maxime, E. L., Espino, J., et al. (2011). Aquatic biodiversity in the amazon: habitat specialization and geographic isolation promote species richness. Animals 1, 205–241. doi: 10.3390/ani1020205
Albert, J. S., Tagliacollo, V. A., and Dagosta, F. (2020). Diversification of neotropical freshwater fishes. Annu. Rev. Ecol. Evol. Syst. 51, 27–53. doi: 10.1146/annurev-ecolsys-011620-031032
Alexander, D. H., Novembre, J., and Lange, K. (2009). Fast model-based estimation of ancestry in unrelated individuals. Genome Res. 19, 1655–1664. doi: 10.1101/gr.094052.109
Altschul, S. F., Madden, T. L., Schäffer, A. A., Zhang, J., Zhang, Z., Miller, W., et al. (1997). Gapped BLAST and PSI-BLAST: a new generation of protein database search programs. Nucleic Acids Res. 25, 3389–3402. doi: 10.1093/nar/25.17.3389
Andrews, S. (2010). FastQC: a Quality Control Tool for High Throughput Sequence Data. Cambridge: Babraham Institute.
Araújo, J. D., Ghelfi, A., and Val, A. L. (2017). Triportheus albus (Cope, 1872) in the blackwater, clearwater, and whitewater of the Amazon: a case of phenotypic plasticity? Front. Genet. 8:114. doi: 10.3389/fgene.2017.00114
Attard, C. R. M., Sandoval-Castillo, J., Brauer, C. J., Unmack, P. J., Schmarr, D., Bernatchez, L., et al. (2022). Fish out of water: genomic insights into persistence of rainbowfish populations in the desert. Evolution 76, 171–183. doi: 10.1111/evo.14399
Batley, K. C., Sandoval-Castillo, J., Kemper, C. M., Attard, C. R., Zanardo, N., Tomo, I., et al. (2019). Genome-wide association study of an unusual dolphin mortality event reveals candidate genes for susceptibility and resistance to cetacean morbillivirus. Evol. Appl. 1, 718–732. doi: 10.1111/eva.12747
Beheregaray, L. B., Cooke, G. M., Chao, N. L., and Landguth, E. L. (2014). Ecological speciation in the tropics: insights from comparative genetic studies in Amazonia. Front. Genet. 5:477. doi: 10.3389/fgene.2014.00477
Bolger, A. M., Lohse, M., and Usadel, B. (2014). Trimmomatic: a flexible trimmer for Illumina sequence data. Bioinformatics 30, 2114–2120. doi: 10.1093/bioinformatics/btu170
Borghezan, E. D. A., da Silva Pires, T. H., Ikeda, T., Zuanon, J., and Kohshima, S. (2021). A review on fish sensory systems and amazon water types with implications to biodiversity. Front. Ecol. Evol. 8:589760. doi: 10.3389/fevo.2020.589760
Bradic, M., Teotónio, H., and Borowsky, R. L. (2013). The population genomics of repeated evolution in the blind cavefish Astyanax mexicanus. Mol. Biol. Evol. 30, 2383–2400. doi: 10.1093/molbev/mst136
Brauer, C. J., Hammer, M. P., and Beheregaray, L. B. (2016). Riverscape genomics of a threatened fish across a hydroclimatically heterogeneous river basin. Mol. Ecol. 25, 5093–5113. doi: 10.1111/mec.13830
Brauer, C. J., Unmack, P. J., Smith, S., Bernatchez, L., and Beheregaray, L. B. (2018). On the roles of landscape heterogeneity and environmental variation in determining population genomic structure in a dendritic system. Mol. Ecol. 27, 3484–3497. doi: 10.1111/mec.14808
Brieuc, M. S., Waters, C. D., Drinan, D. P., and Naish, K. A. (2018). A practical introduction to random forest for genetic association studies in ecology and evolution. Mol. Ecol. Res. 18, 755–766. doi: 10.1111/1755-0998.12773
Campbell, K. E. Jr., Frailey, C. D., and Romero-Pittman, L. (2006). The pan-Amazonian ucayali peneplain, late neogene sedimentation in Amazonia, and the birth of the modern Amazon river system. Palaeogeogr. Palaeoclim. Palaeoecol. 239, 166–219. doi: 10.1016/j.palaeo.2006.01.020
Catchen, J., Hohenlohe, P. A., Bassham, S., Amores, A., and Cresko, W. A. (2013). Stacks: an analysis tool set for population genomics. Mol. Ecol. 22, 3124–3140. doi: 10.1111/mec.12354
Christensen, A. H., Chatelain, F. C., Huttner, I. G., Olesen, M. S., Soka, M., Feliciangeli, S., et al. (2016). The two-pore domain potassium channel, TWIK-1, has a role in the regulation of heart rate and atrial size. J. Mol. Cell. Cardiol. 97, 24–35. doi: 10.1016/j.yjmcc.2016.04.006
Cooke, G. M., Chao, N. L., and Beheregaray, L. B. (2012a). Divergent natural selection with gene flow along major environmental gradients in Amazonia: insights from genome scans, population genetics and phylogeography of the characin fish Triportheus albus. Mol. Ecol. 21, 2410–2427. doi: 10.1111/j.1365-294X.2012.05540.x
Cooke, G. M., Chao, N. L., and Beheregaray, L. B. (2012b). Marine incursions, cryptic species and ecological diversification in Amazonia: the biogeographic history of the croaker genus Plagioscion (Sciaenidae). J. Biogeogr. 39, 724–738. doi: 10.1111/j.1365-2699.2011.02635.x
Cooke, G. M., Chao, N. L., and Beheregaray, L. B. (2012c). Natural selection in the water: freshwater invasion and adaptation by water colour in the Amazonian pufferfish. J. Evol. Biol. 25, 1305–1320. doi: 10.1111/j.1420-9101.2012.02514.x
Cooke, G. M., Landguth, E. L., and Beheregaray, L. B. (2014). Riverscape genetics identifies replicated ecological divergence across an Amazonian ecotone. Evolution 68, 1947–1960. doi: 10.1111/evo.12410
Coop, G., Witonsky, D., Di Rienzo, A., and Pritchard, J. K. (2010). Using environmental correlations to identify loci underlying local adaptation. Genetics 185, 1411–1423. doi: 10.1534/genetics.110.114819
Corlett, R., and Primack, R. B. (2010). Tropical Rainforests: An Ecological and Biogeographical Comparison. Malden, MA: Wiley-Blackwell.
Crookes, S., and Shaw, P. (2016). Isolation by distance and non-identical patterns of gene flow within two river populations of the freshwater fish Rutilus rutilus (L. 1758). Conserv. Genet. 17, 861–874. doi: 10.1007/s10592-016-0828-3
Danecek, P., Auton, A., Abecasis, G., Albers, C. A., Banks, E., DePristo, M. A., et al. (2011). The variant call format and VCFtools. Bioinformatics 27, 2156–2158. doi: 10.1093/bioinformatics/btr330
Darriba, D., Posada, D., Kozlov, A. M., Stamatakis, A., Morel, B., and Flouri, T. (2020). ModelTest-NG: a new and scalable tool for the selection of DNA and protein evolutionary models. Mol. Biol. Evol. 37, 291–294. doi: 10.1093/molbev/msz189
Darwin, C. (1859). On the Origin of Species by Means of Natural Selection, or Preservation of Favoured Races in the Struggle for Life. London: John Murray.
Dixon, P. (2003). VEGAN, a package of R functions for community ecology. J. Veg. Sci. 14, 927–930. doi: 10.1111/j.1654-1103.2003.tb02228.x
Dray, S., and Dufour, A.-B. (2007). The ade4 package: implementing the duality diagram for ecologists. J. Stat. Softw. 22, 1–20. doi: 10.18637/jss.v022.i04
Duncan, W. P., and Fernandes, M. N. (2010). Physicochemical characterization of the white, black, and clearwater rivers of the Amazon Basin and its implications on the distribution of freshwater stingrays (Chondrichthyes, Potamotrygonidae). Panam. J. Aquat. Sci. 5, 454–464.
Dyer, R. J., Nason, J. D., and Garrick, R. C. (2010). Landscape modelling of gene flow: improved power using conditional genetic distance derived from the topology of population networks. Mol. Ecol. 19, 3746–3759. doi: 10.1111/j.1365-294X.2010.04748.x
Ellis, N., Smith, S. J., and Pitcher, C. R. (2012). Gradient forests: calculating importance gradients on physical predictors. Ecology 93, 156–168. doi: 10.1890/11-0252.1
Evangelista, E., and Tosi, C. (2015). First giant otter distribution survey in the southeast of Roraima, Brazil, with notes on the OSG guidelines for a standardization of survey methods. Lat. Am. J. Aquat. Mamm. 10, 143–146. doi: 10.5597/lajam00206
Excoffier, L., Hofer, T., and Foll, M. (2009). Detecting loci under selection in a hierarchically structured population. Heredity 103, 285–298. doi: 10.1038/hdy.2009.74
Excoffier, L., and Lischer, H. E. (2010). Arlequin suite ver 3.5: a new series of programs to perform population genetics analyses under Linux and windows. Mol. Ecol. Res. 10, 564–567. doi: 10.1111/j.1755-0998.2010.02847.x
Figueiredo, J., Hoorn, C., Van der Ven, P., and Soares, E. (2009). Late Miocene onset of the Amazon river and the Amazon deep-sea fan: evidence from the Foz do Amazonas basin. Geology 37, 619–622. doi: 10.1130/G25567A.1
Forester, B. R., Lasky, J. R., Wagner, H. H., and Urban, D. L. (2018). Comparing methods for detecting multilocus adaptation with multivariate genotype-environment associations. Mol. Ecol. 27, 2215–2233. doi: 10.1111/mec.14584
Fourcade, Y., Chaput-Bardy, A., Secondi, J., Fleurant, C., and Lemaire, C. (2013). Is local selection so widespread in river organisms? Fractal geometry of river networks leads to high bias in outlier detection. Mol. Ecol. 22, 2065–2073. doi: 10.1111/mec.12158
Frank, D. F., Brander, S. M., Hasenbein, S., Harvey, D. J., Lein, P. J., Geist, J., et al. (2019). Developmental exposure to environmentally relevant concentrations of bifenthrin alters transcription of mTOR and ryanodine receptor-dependent signaling molecules and impairs predator avoidance behavior across early life stages in inland silversides (Menidia beryllina). Aquat. Toxicol. 206, 1–13. doi: 10.1016/j.aquatox.2018.10.014
Furch, K. (1984). “Water chemistry of the Amazon basin: the distribution of chemical elements among freshwaters,” in The Amazon. Monographiae Biologicae, Vol. 56, ed. H. Sioli (Dordrecht: Springer), 167–199. doi: 10.1007/978-94-009-6542-3_6
Gardner, M. B. (1981). Effects of turbidity on feeding rates and selectivity of bluegills. Trans. Am. Fish. Soc. 110, 446–450. doi: 10.1577/1548-8659(1981)110<446:eotofr>2.0.co;2
Gaughran, S. J., Quinzin, M. C., Miller, J. M., Garrick, R. C., Edwards, D. L., Russello, M. A., et al. (2018). Theory, practice, and conservation in the age of genomics: the Galápagos giant tortoise as a case study. Evol. Appl. 11, 1084–1093. doi: 10.1111/eva.12551
Gautier, M. (2015). BayPass genome-wide scan for adaptive differentiation and association analysis with population-specific covariables. Genetics 201, 1555–1579. doi: 10.1534/genetics.115.181453
Giarrizzo, T., de Sena Oliveira, R., Costa Andrade, M., Pedrosa Gonçalves, A., Barbosa, T., Martins, A., et al. (2015). Length–weight and length–length relationships for 135 fish species from the Xingu River (Amazon Basin, Brazil). J. Appl. Ichthyol. 31, 415–424. doi: 10.1111/jai.12677
Goldstein, B. A., Polley, E. C., and Briggs, F. B. (2011). Random forests for genetic association studies. Stat. Appl. Genet. Mol. Biol. 10:32. doi: 10.2202/1544-6115.1691
Goss, G. G., Perry, S., Wood, C., and Laurent, P. (1992). Mechanisms of ion and acid-base regulation at the gills of freshwater fish. J. Exp. Zool. 263, 143–159. doi: 10.1002/jez.1402630205
Grummer, J. A., Beheregaray, L. B., Bernatchez, L., Hand, B. K., Luikart, G., Narum, S. R., et al. (2019). Aquatic landscape genomics and environmental effects on genetic variation. Trends Ecol. Evol. 34, 641–654. doi: 10.1016/j.tree.2019.02.013
Günther, T., and Coop, G. (2013). Robust identification of local adaptation from allele frequencies. Genetics 195, 205–220. doi: 10.1534/genetics.113.152462
Guthrie, D. (1986). “Role of vision in fish behaviour,” in The Behaviour of Teleost Fishes, ed. T. J. Pitcher (Boston, MA: Springer), 75–113. doi: 10.1007/978-1-4684-8261-4_4
Higham, T. E., Stewart, W. J., and Wainwright, P. C. (2015). Turbulence, temperature, and turbidity: the ecomechanics of predator–prey interactions in fishes. Integr. Comp. Biol. 55, 6–20. doi: 10.1093/icb/icv052
Holzer, P. (2003). Acid-sensitive ion channels in gastrointestinal function. Curr. Opin. Pharmacol. 3, 618–625. doi: 10.1016/j.coph.2003.06.008
Hoorn, C., Roddaz, M., Dino, R., Soares, E., Uba, C., Ochoa-Lozano, D., et al. (2010a). “The Amazonian craton and its influence on past fluvial systems (Mesozoic-Cenozoic, Amazonia),” in Amazonia: Landscape and Species Evolution: a Look into the Past, eds C. Hoorn and F. P. Wesselingh (Hoboken, NJ: Wiley), 103–122. doi: 10.1002/9781444306408.ch7
Hoorn, C., Wesselingh, F., Ter Steege, H., Bermudez, M., Mora, A., Sevink, J., et al. (2010b). Amazonia through time: andean uplift, climate change, landscape evolution, and biodiversity. Science 330, 927–931. doi: 10.1126/science.1194585
Hrbek, T., Farias, I. P., Crossa, M., Sampaio, I., Porto, J. I., and Meyer, A. (2005). Population genetic analysis of Arapaima gigas, one of the largest freshwater fishes of the Amazon basin: implications for its conservation. Anim. Conserv. 8, 297–308. doi: 10.1017/S1367943005002210
Hubert, N., Duponchelle, F., Nunez, J., Rivera, R., Bonhomme, F., and Renno, J. F. (2007). Isolation by distance and pleistocene expansion of the lowland populations of the white piranha Serrasalmus rhombeus. Mol. Ecol. 16, 2488–2503. doi: 10.1111/j.1365-294X.2007.03338.x
Hughes, G. M. (1973). Respiratory responses to hypoxia in fish. Am. Zool. 13, 475–489. doi: 10.1093/icb/13.2.475
Hughes, J. M., Schmidt, D. J., and Finn, D. S. (2009). Genes in streams: using DNA to understand the movement of freshwater fauna and their riverine habitat. Bioscience 59, 573–583. doi: 10.1525/bio.2009.59.7.8
Hwang, P.-P., and Lee, T.-H. (2007). New insights into fish ion regulation and mitochondrion-rich cells. Comp. Biochem. Phys. A Mol. Integr. Physiol. 148, 479–497. doi: 10.1016/j.cbpa.2007.06.416
Jukes, T. H., and Cantor, C. R. (1969). “Evolution of protein molecules,” in Mammalian Protein Metabolism, Vol. 3, ed. H. N. Munro (New York, NY: Academic Press), 21–132. doi: 10.1016/b978-1-4832-3211-9.50009-7
Junk, W. J., Soares, G. M., and Carvalho, F. M. (1983). Distribution of fish species in a lake of the Amazon river floodplain near Manaus (lago Camaleão), with special reference to extreme oxygen conditions. Amazoniana 7, 397–431.
Kelley, J. L., Brown, A. P., Therkildsen, N. O., and Foote, A. D. (2016). The life aquatic: advances in marine vertebrate genomics. Nat. Rev. Genet. 17, 523–534. doi: 10.1038/nrg.2016.66
Kimbell, H. S., and Morrell, L. J. (2015). Turbidity influences individual and group level responses to predation in guppies, Poecilia reticulata. Anim. Behav. 103, 179–185. doi: 10.1016/j.anbehav.2015.02.027
Križaj, D. (2012). Calcium stores in vertebrate photoreceptors. Adv. Exp. Med. Biol. 740, 873–889. doi: 10.1007/978-94-007-2888-2_39
Krizaj, D., and Copenhagen, D. R. (2002). Calcium regulation in photoreceptors. Front. Biosci. 7, d2023–d2044. doi: 10.2741/A896
Križaj, D., Liu, X., and Copenhagen, D. R. (2004). Expression of calcium transporters in the retina of the tiger salamander (Ambystoma tigrinum). J. Comp. Neurol. 475, 463–480. doi: 10.1002/cne.20170
Križaj, D., Mercer, A. J., Thoreson, W. B., and Barabas, P. (2011). Intracellular pH modulates inner segment calcium homeostasis in vertebrate photoreceptors. Am. J. Physiol. Cell Physiol. 300, 187–197. doi: 10.1152/ajpcell.00264.2010
Küchler, I. L., Miekeley, N., and Forsberg, B. R. (2000). A contribution to the chemical characterization of rivers in the rio negro basin, Brazil. J. Braz. Chem. Soc. 11, 286–292. doi: 10.1590/S0103-50532000000300015
Landman, M. J., Van Den Heuvel, M. R., and Ling, N. (2005). Relative sensitivities of common freshwater fish and invertebrates to acute hypoxia. N. Z. J. Mar. Freshwater Res. 39, 1061–1067. doi: 10.1080/00288330.2005.9517375
Laporte, M., Pavey, S. A., Rougeux, C., Pierron, F., Lauzent, M., Budzinski, H., et al. (2016). RAD sequencing reveals within-generation polygenic selection in response to anthropogenic organic and metal contamination in North Atlantic Eels. Mol. Ecol. 25, 219–237. doi: 10.1111/mec.13466
Laranjeiras, T. O., Naka, L. N., Leite, G. A., and Cohn-Haft, M. (2021). Effects of a major Amazonian river confluence on the distribution of floodplain forest avifauna. J. Biogeogr. 48, 847–859. doi: 10.1111/jbi.14042
Latrubesse, E. M., and Franzinelli, E. (2005). The late quaternary evolution of the negro river, Amazon, Brazil: implications for island and floodplain formation in large anabranching tropical systems. Geomorphology 70, 372–397. doi: 10.1016/j.geomorph.2005.02.014
Leahy, S. M., McCormick, M. I., Mitchell, M. D., and Ferrari, M. C. (2011). To fear or to feed: the effects of turbidity on perception of risk by a marine fish. Biol. Lett. 7, 811–813. doi: 10.1098/rsbl.2011.0645
Legendre, P., and Legendre, L. (1998). Numerical Ecology: Developments in Environmental Modelling, 2nd Edn. Amsterdam: Elsevier.
Liaw, A., and Wiener, M. (2002). Classification and Regression by randomForest. R News 2, 18–22. Available online at: https://CRAN.R-project.org/doc/Rnews/
Luikart, G., England, P. R., Tallmon, D., Jordan, S., and Taberlet, P. (2003). The power and promise of population genomics: from genotyping to genome typing. Nat. Rev. Genet. 4, 981–994. doi: 10.1038/nrg1226
Lundberg, J. G., Marshall, L. G., Guerrero, J., Horton, B. M., and Wesselingh, F. (1998). The stage for neotropical fish diversification: a history of tropical South American rivers. Phylogeny Class. Neotrop. Fish. 27, 13–48.
Mandic, M., Todgham, A. E., and Richards, J. G. (2009). Mechanisms and evolution of hypoxia tolerance in fish. Proc. R. Soc B Biol. Sci. 276, 735–744. doi: 10.1098/rspb.2008.1235
McClain, M. E., and Naiman, R. J. (2008). Andean influences on the biogeochemistry and ecology of the Amazon river. Bioscience 58, 325–338. doi: 10.1641/B580408
Meffe, G. K., and Vrijenhoek, R. C. (1988). Conservation genetics in the management of desert fishes. Conserv. Biol. 2, 157–169. doi: 10.1111/j.1523-1739.1988.tb00167.x
Meirmans, P. G. (2012). The trouble with isolation by distance. Mol. Ecol. 21, 2839–2846. doi: 10.1111/j.1365-294X.2012.05578.x
Morin, P. A., Luikart, G., and Wayne, R. K. (2004). SNPs in ecology, evolution and conservation. Trends Ecol. Evol. 19, 208–216. doi: 10.1016/j.tree.2004.01.009
Morin, P. A., Martien, K. K., and Taylor, B. L. (2009). Assessing statistical power of SNPs for population structure and conservation studies. Mol. Ecol. Res. 9, 66–73. doi: 10.1111/j.1755-0998.2008.02392.x
Mu, Y., Li, W., Wei, Z., He, L., Zhang, W., and Chen, X. (2020). Transcriptome analysis reveals molecular strategies in gills and heart of large yellow croaker (Larimichthys crocea) under hypoxia stress. Fish Shellfish Immunol. 104, 304–313. doi: 10.1016/j.fsi.2020.06.028
Narum, S. R., and Hess, J. E. (2011). Comparison of FST outlier tests for SNP loci under selection. Mol. Ecol. Res. 11, 184–194. doi: 10.1111/j.1755-0998.2011.02987.x
Ochoa, L. E., Pereira, L. H. G., Costa-Silva, G. J., Roxo, F. F., Batista, J. S., Formiga, K., et al. (2015). Genetic structure and historical diversification of catfish Brachyplatystoma platynemum (Siluriformes: Pimelodidae) in the Amazon basin with implications for its conservation. Ecol. Evol. 5, 2005–2020. doi: 10.1002/ece3.1486
Ortí, G., and Meyer, A. (1997). The radiation of characiform fishes and the limits of resolution of mitochondrial ribosomal DNA sequences. Syst. Biol. 46, 75–100. doi: 10.1093/sysbio/46.1.75
Pavey, S. A., Bernatchez, L., Aubin-Horth, N., and Landry, C. R. (2012). What is needed for next-generation ecological and evolutionary genomics? Trends Ecol. Evol. 27, 673–678. doi: 10.1016/j.tree.2012.07.014
Peterson, B. K., Weber, J. N., Kay, E. H., Fisher, H. S., and Hoekstra, H. E. (2012). Double digest RADseq: an inexpensive method for de novo SNP discovery and genotyping in model and non-model species. PLoS One 7:e37135. doi: 10.1371/journal.pone.0037135
Pires, T. H., Borghezan, E. A., Machado, V. N., Powell, D. L., Röpke, C. P., Oliveira, C., et al. (2018). Testing Wallace’s intuition: water type, reproductive isolation and divergence in an Amazonian fish. J. Evol. Biol. 31, 882–892. doi: 10.1111/jeb.13272
Prestes, L., Soares, M. G. M., Silva, F. R., and Bittencourt, M. M. (2010). Dynamic population from Triportheus albus, T. angulatus and T. auritus (Characiformes: Characidae) in Amazonian central lakes. Biota Neotrop. 10, 177–181. doi: 10.1590/S1676-06032010000300020
Puritz, J. B., Hollenbeck, C. M., and Gold, J. R. (2014). dDocent: a RADseq, variant-calling pipeline designed for population genomics of non-model organisms. PeerJ. 2:e431. doi: 10.7717/peerj.431
Rellstab, C., Gugerli, F., Eckert, A. J., Hancock, A. M., and Holderegger, R. (2015). A practical guide to environmental association analysis in landscape genomics. Mol. Ecol. 24, 4348–4370. doi: 10.1111/mec.13322
Rowe, D. K., and Dean, T. L. (1998). Effects of turbidity on the feeding ability of the juvenile migrant stage of six New Zealand freshwater fish species. N. Z. J. Mar. Freshwater Res. 32, 21–29. doi: 10.1080/00288330.1998.9516803
Rull, V. (2008). Speciation timing and neotropical biodiversity: the Tertiary–Quaternary debate in the light of molecular phylogenetic evidence. Mol. Ecol. 17, 2722–2729. doi: 10.1111/j.1365-294X.2008.03789.x
Saint-Paul, U., Zuanon, J., Correa, M. A. V., García, M., Fabré, N. N., Berger, U., et al. (2000). Fish communities in central Amazonian white- and blackwater floodplains. Environ. Biol. Fishes 57, 235–250. doi: 10.1023/A:1007699130333
Sandoval-Castillo, J., Robinson, N. A., Hart, A. M., Strain, L. W., and Beheregaray, L. B. (2018). Seascape genomics reveals adaptive divergence in a connected and commercially important mollusc, the greenlip abalone (Haliotis laevigata), along a longitudinal environmental gradient. Mol. Ecol. 27, 1603–1620. doi: 10.1111/mec.14526
Seehausen, O., Butlin, R. K., Keller, I., Wagner, C. E., Boughman, J. W., Hohenlohe, P. A., et al. (2014). Genomics and the origin of species. Nat. Rev. Genet. 15, 176–192.
Sexton, J. P., Hangartner, S. B., and Hoffmann, A. A. (2014). Genetic isolation by environment or distance: which pattern of gene flow is most common? Evolution 68, 1–15. doi: 10.1111/evo.12258
Sioli, H. (1984). The Amazon: Limnology and Landscape Ecology of a Mighty Tropical River and its Basin, Vol. 56. Berlin: Springer Science+Business Media.
Storfer, A., Murphy, M. A., Evans, J. S., Goldberg, C. S., Robinson, S., Spear, S. F., et al. (2007). Putting the ‘landscape’ in landscape genetics. Heredity 98, 128–142. doi: 10.1038/sj.hdy.6800917
Sunnucks, P., and Hales, D. F. (1996). Numerous transposed sequences of mitochondrial cytochrome oxidase I-II in aphids of the genus Sitobion (Hemiptera: Aphididae). Mol. Biol. Evol. 13, 510–524. doi: 10.1093/oxfordjournals.molbev.a025612
Sutko, J. L., and Airey, J. A. (1996). Ryanodine receptor Ca2+ release channels: does diversity in form equal diversity in function? Physiol. Rev. 76, 1027–1071. doi: 10.1152/physrev.1996.76.4.1027
Tavaré, S. (1986). Some probabilistic and statistical problems in the analysis of DNA sequences. Lect. Math. Life Sci. 17, 57–86.
Tejerina-Garro, F. L., Fortin, R., and Rodríguez, M. A. (1998). Fish community structure in relation to environmental variation in floodplain lakes of the Araguaia River, Amazon Basin. Environ. Biol. Fishes 51, 399–410. doi: 10.1023/A:1007401714671
Thurman, L. L., Stein, B. A., Beever, E. A., Foden, W., Geange, S. R., Green, N., et al. (2020). Persist in place or shift in space? Evaluating the adaptive capacity of species to climate change. Front. Ecol. Environ. 18, 520–528. doi: 10.1002/fee.2253
Torati, L. S., Taggart, J. B., Varela, E. S., Araripe, J., Wehner, S., and Migaud, H. (2019). Genetic diversity and structure in Arapaima gigas populations from Amazon and Araguaia-Tocantins river basins. BMC Genet. 20:13. doi: 10.1186/s12863-018-0711-y
Val, A. L. (1995). Fishes of the Amazon and Their Environment Physiological and Biochemical Aspects, 1st Edn. Berlin: Springer.
Vitalis, R., Dawson, K., and Boursot, P. (2001). Interpretation of variation across marker loci as evidence of selection. Genetics 158, 1811–1823. doi: 10.1093/genetics/158.4.1811
Wallace, A. R. (1854). “A narrative of travels on the Amazon and Rio Negro, with an account of the native tribes and observations on the climate, geology, and natural history of the amazon valley,” in Annals and Magazine of Natural History, Vol. 13, ed. A. R. Wallace (London: Reeve & Co), 57–58.
Wang, I. J., and Bradburd, G. S. (2014). Isolation by environment. Mol. Ecol. 23, 5649–5662. doi: 10.1111/mec.12938
Wilgenbusch, J. C., and Swofford, D. (2003). Inferring evolutionary trees with PAUP. Curr. Protoc. Bioinformatics 6, 6.4.1–6.4.28. doi: 10.1002/0471250953.bi0604s00
Yeaman, S., Hodgins, K. A., Lotterhos, K. E., Suren, H., Nadeau, S., Degner, J. C., et al. (2016). Convergent local adaptation to climate in distantly related conifers. Science 353, 1431–1433. doi: 10.1126/science.aaf7812
Keywords: evolutionary ecology, adaptation, ecological genomics, Amazonia, tropical diversification, landscape genomics, ddRAD, teleost
Citation: Hay AC, Sandoval-Castillo J, Cooke GM, Chao NL and Beheregaray LB (2022) Riverscape Genomics Clarifies Neutral and Adaptive Evolution in an Amazonian Characin Fish (Triportheus albus). Front. Ecol. Evol. 10:825406. doi: 10.3389/fevo.2022.825406
Received: 30 November 2021; Accepted: 28 January 2022;
Published: 04 March 2022.
Edited by:
Neil Rosser, Harvard University, United StatesReviewed by:
Robert Thomson, University of Hawaii, United StatesDaniel Cardoso Carvalho, Pontifícia Universidade Católica de Minas Gerais, Brazil
Copyright © 2022 Hay, Sandoval-Castillo, Cooke, Chao and Beheregaray. This is an open-access article distributed under the terms of the Creative Commons Attribution License (CC BY). The use, distribution or reproduction in other forums is permitted, provided the original author(s) and the copyright owner(s) are credited and that the original publication in this journal is cited, in accordance with accepted academic practice. No use, distribution or reproduction is permitted which does not comply with these terms.
*Correspondence: Luciano B. Beheregaray, luciano.beheregaray@flinders.edu.au