Divergence in metabolomic profile in clownfish and damselfish skin mucus
- 1Department of Computational Biology, Faculty of Biology and Medicine, University of Lausanne, Lausanne, Switzerland
- 2Metabolomics Unit, Faculty of Biology and Medicine, University of Lausanne, Lausanne, Switzerland
Introduction: The clownfish - sea anemone mutualism was suggested to have triggered the adaptive radiation of clownfishes, but the origin of clownfish resistance to stinging tentacles of host anemones remains unclear. The presence of specific compounds in the mucus of clownfishes conferring them the unique ability to prevent nematocyst discharge from their hosts has been the most supported hypothesis. Yet the mystery regarding the types of compounds found in clownfish skin mucus remains unsolved.
Methods: We analyzed the chemical composition of clownfish and damselfish mucus using an untargeted metabolomics (HILIC-HRMS) and lipidomics (RPLC-HRMS) approach.
Results and Discussion: The polar and lipid metabolome signatures were highly specific and allowed to discriminate between the clownfish and damselfish clades. The most discriminative part of the signature was the sphingolipid profile, displaying a broader diversity of ceramides present in significantly higher levels in clownfish mucus. Importantly, the inter-specific variability of metabolic signature was significantly higher in clownfishes, although their diversification is evolutionarily more recent, thus implying the impact of symbiosis on metabolic variability and adaptation. Furthermore, specialists and generalists clownfish species displayed distinctive metabolite signature. Two strict clownfish specialists, which are phylogenetically distant but share the same host species, clustered together based on their molecular signature, suggesting a link with their mutualistic nature. Overall, comparative analyses of metabolic signatures highlight differences in chemical composition of clownfish mucus and provide insight into biochemical pathways potentially implicated in clownfish adaptation to inhabit sea anemones and consequently diversify.
Introduction
Interactions between organisms constitute a fundamental component of ecosystems (Chapin III et al., 2011) and these interactions are usually mediated by chemically induced responses (Atema, 1995). Chemical communication plays a critical role in marine ecosystems, like coral reefs that harbor vast biodiversity, by shaping the population structure, community organization and ecosystem function (Hay, 2009). Marine species sense the environment via chemical signals that determine the selection of mates and habitat location, acquisition of food resources or host recognition by symbiont (Breithaupt and Thiel, 2010; Hagedorn et al., 2015). In the case of the sea cucumber Bohadschia vitiensis, for example, it was shown that the products of specialized metabolism, in particular triterpene glycosides saponins, have a double function: to repel predators (unpalatability effect) and to attract the symbiont harlequin crab Lissocarcinus orbicularis (Caulier et al., 2013), thereby fostering interactions between communities of different species. However, except in a few rare cases, the molecular mechanisms involved in chemical communication are still largely unknown. In this context, the use of marine chemical ecology can significantly contribute to our understanding of the evolution and organization of marine populations as well as the function of marine ecosystems (Pohnert et al., 2007).
Chemical cues and signals are particularly important in the case of mutualism, where organisms benefit from each other, because of the tight dependency that such an interaction entails (Biedermann and Kaltenpoth, 2014; Hojo et al., 2014). A classic example of mutualism in a marine environment involves the photosynthetic dinoflagellates inhabiting corals and clams (Knowlton and Rohwer, 2003; Stat et al., 2008). It was shown that the dinoflagellate Symbiodinium and the larvae of Fungia scutaria corals can establish symbiosis via chemical communication through the release of disaccharide trehalose and glycerol into the environment by Symbiodinium when in presence of coral tissue. These metabolites are detected by coral larvae possessing the trehalase enzyme to initiate a feeding behavior (Hagedorn et al., 2015). Such findings demonstrate the importance of chemical communication between organisms sharing the same ecological niche.
Coral reefs host another well-known case of symbiotic mutualism between clownfishes and sea anemones. It was shown that this mutualism could be responsible for the adaptive radiation of clownfishes (Litsios et al., 2012; Marcionetti et al., 2019). Although this system has been studied for a long time, the molecular signals allowing the close interactions between these two organisms remain poorly understood. Clownfishes are a common group of reef fishes composed of 28 known species and two hybrid species within the damselfish family (Teleostei; Perciformes; Pomacentridae; Quenouille et al., 2004). Spanning from the Indian to the Western Pacific Ocean, clownfishes are obligate mutualists with at least one species of sea anemone (Anthozoa: Actiniaria) and they are the only adult fishes capable of living within the venomous tentacles of sea anemones (Madio et al., 2019).
Clownfish species have been described as either generalists, for those able to establish a mutualistic interaction with several species of sea anemones (up to 10), or specialists, for those restricted to only one species of sea anemones (Elliott and Mariscal, 2001). Despite several studies on the evolution of this group of fish (Fautin, 1991; Mebs, 2009; Rolland et al., 2018), we still do not have a clear explanation about the mechanisms enabling clownfishes to get in close contact with sea anemones without being stung. The main hypothesis is that chemical signals within the mucus layer coating the skin of clownfishes could help to inhibit the discharge of nematocysts by sea anemones (Mebs, 2009). However, the chemical composition of clownfish mucus has not been characterized so far.
Fish skin mucus is a slimy layer acting as the first physical and chemical barrier against the external environment (Wang et al., 2011). The mucus is constantly produced by goblet cells located in fish epidermis (Shephard, 1994) and it is an important component of the innate immune system (Dash et al., 2018). The main molecules identified in this biological matrix are antimicrobial peptides, lectins, glycoproteins, mucins, saturated fatty acids, polyunsaturated fatty acids, as well as certain metabolites such as azelaic acid, N-acetylneuraminic acid and N-acetylglucosamine, and hydroxyisocaproic acid (Zaccone et al., 2001; Swain et al., 2007; Guardiola et al., 2014; Ekman et al., 2015). Major studies on fish mucus have focused on its immune function (Palaksha et al., 2008; Esteban, 2012), but whether or not it may play a role in chemical communication and recognition remains undescribed. Most of these studies utilize freshwater fishes or commercial fish species (flathead grey mullet/rainbow trout/salmon) and these aspects are therefore still to be explored in marine reef fishes. Further, the roles that different compounds could play in enabling the clownfish association with sea anemone remain unexplored. We thus investigate whether the mucus composition differs between clownfish species depicting varying levels of host specificity and between clownfishes and their closest relatives that are unable to interact with sea anemone.
In this study, we used metabolomics and lipidomics to investigate the small molecule composition of the mucus of clownfishes and several related species of damselfishes. We hypothesized that the unique ability of clownfishes to initiate mutualistic interactions with sea anemones, which is absent in non-mutualistic damselfishes, is due to differences in their mucus composition. We further expected to see differences in the mucus composition between clownfish species that differ in the level of specialization with their host sea anemone. However, metabolites are highly influenced by external cues and, in our study, we wanted to detect relevant differences coming from the species observed rather than effects from varying external environmental conditions. We thus estimated the profile of the mucus of different fish species in similar conditions and without the effect of their respective host anemone to observe if clownfishes possess innate metabolic features allowing them to establish their mutualistic association. We sampled mucus of acclimated fish species and used an untargeted approach combining four different analysis modes to maximize the polar and lipid metabolome coverage (Putri et al., 2013). Our results showed that clownfishes have distinct features in their mucus composition that can potentially be associated with their interactions with sea anemones. The identification of the molecules involved represents the first step forward towards new insights into the mechanisms that allowed the clownfishes to interact with their host.
Materials and methods
Sampling of clownfish species and their mucus
We selected six clownfish species spanning the full range of mutualistic behavior (see Litsios et al., 2012) from strict specialists (A. frenatus and A. biaculeatus), to specialists on a few anemone species (A. ocellaris and A. percula), to two generalists (A. clarkii and A. perideraion). We also sampled two damselfish species (Chromis viridis and Pomacentrus moluccensis) that are closely related to clownfishes but unable to interact within sea anemone. The number of individuals sampled ranged from 4 to 10 depending on the species. The adult fish were all imported from different areas of Indonesia (Supplementary Figure S1) and kept in sea water aquariums without sea anemones at Aqua-Home Aigle, Switzerland, for a quarantine of 10 days. The aquarium temperature was 25°C, salinity was 1.025, NH3/NH4 were kept at 0–0.2 ppm and nitrates were around 10 ppm. All fishes were under a 12 h day and 12 h night light environment.
We sampled the mucus on the skin surface of all individuals between September 2018 and May 2019. All fishes were anesthetized individually in a solution of sea water and Tricaine methanesulfonate (100 mg/l) for a few minutes (1–2 min). They were then removed from this solution and placed in a sterilized glass petri dish for mucus collection (3–4 scrapes per side) starting from the pectoral fin until the end of the tail with the help of a soft cell scraper (SARSTEDT, Nümbrecht Germany). Fishes were then put back into fresh sea water until they awakened and regained mobility. The mucus was washed off from the cell scraper using 500 μl of UHPLC water, transferred into an Eppendorf tube, snapped frozen on dry ice and stored at −80°C prior to metabolite extraction. All procedures were performed in accordance with protocols approved by the veterinary authority of the Canton de Vaud.
Metabolite extraction
We lyophilized the mucus samples and extracted the metabolites using a biphasic extraction with the following solvent mixture: 540 μl of MTBE, 200 μl of MeOH and 360 μl of H2O (Reverter et al., 2017). We vortexed the sample extracts before bath-sonication for 2 min to improve the metabolite extraction. We finally centrifuged the samples 15 min at 14,000 rpm at 4°C. The organic, upper phase (non-polar) was separated from the aqueous, lower (polar) phase and evaporated to dryness in a vacuum concentrator (LabConco, Missouri, US). The dry polar (aqueous phase) and lipid metabolite (organic phase) extracts were stored at −80°C prior to UHPLC-HRMS analysis. We normalized the sample amounts by measuring the total protein content in the protein pellet of each sample following metabolite extraction (see Supplementary Methods for Details).
We reconstituted the dry polar metabolite extracts in 100 μl MeOH:H2O (4,1, v/v) and the lipid extracts in 100 μl Butanol:MeOH (1,1, v/v) spiked with the ISTD Splash Lipidomix mix (Avanti Polar Lipids, Birmingham, United States). Both reconstituted extracts were vortexed, sonicated for 30 s and centrifuged for 15 min at 14,000 rpm at 4°C to remove insoluble debris. We transferred the supernatants to LC vials for UHPLC-HRMS analysis.
Untargeted UHPLC-HRMS based metabolome and lipidome profiling
We analyzed the aqueous mucus extracts by hydrophilic interaction liquid chromatography coupled with high-resolution mass spectrometry using electrospray ionization source operating in positive mode (HILIC (+) ESI HRMS) and in negative mode (HILIC (−) ESI HRMS) as described by Gallart-Ayala et al. (2018). The instrument was set to acquire over a m/z range between 30 and 1,200 Da, with the MS acquisition rate of 2 spectra/s.
The organic phase of clownfish mucus extracts was analyzed by reversed phase liquid chromatography coupled to high-resolution mass spectrometry using electrospray ionization source operating in positive mode (RP (+) ESI HRMS) and in negative mode (RP (−) ESI HRMS).
RPLC-HRMS analytical conditions
Analyses were performed on a 6,550 iFunnel Q-TOF mass spectrometer interfaced with the 1,290 UHPLC system (Agilent Technologies). In both modes, we analyzed samples using a pre-column ZORBAX Eclipse Plus UHPLC Guard 3PK, 1.8 μm, 2.1 mm × 5 mm coupled to a ZORBAX Eclipse plus rapid resolution HD, 1.8 μm, 2.1 mm × 100 mm (Agilent Technologies). The mobile phases were composed of A = 0.1% formic acid in water and B = 0.1% formic acid in IPA/ACN (90:10). For both modes, the linear gradient elution started at 15% B, rising to 30% B (2 min) then to 48–72% B (2.50–8.50 min) reaching 99% B (11.50–12 min) and back at 15% B (12.10–15 min).
We applied a 5-min post-run to ensure the re-equilibration of the columns at the end of every injection. The flow rate was 600 μl/min, column temperature 60°C and sample injection volume 2 μl. We set the source conditions for ESI as follows: dry gas temperature of 200°C and flow of 14 l/min, fragmentor voltage of 175 V, sheath gas temperature of 300°C and flow of 11 l/min, nozzle voltage of 1,000 V, and capillary voltage of 3,500 V. The instrument was set to acquire over a m/z range between 30 and 1,700 Da, with the MS acquisition rate of 3 spectra/s.
MS/MS data acquisition
MS/MS data were acquired in data-dependent acquisition (DDA) mode on QC samples using iterative injections through PC-driven exclusion (Broadhurst et al., 2018). Parameters were set according to Agilent Technologies, Inc. Santa Clara, CA, United States (Koelmel et al., 2020). The additional MS/MS data were acquired in targeted mode on selected precursors with narrow isolation width (≈ 1.3 m/z), MS acquisition rate at 3 spectra/s and MS/MS acquisition at 3 spectra/s. The collision energy for polar metabolites was fixed at 25 eV, and for lipids at 20 and 40 eV.
Metabolomics data (pre) processing
We converted the raw UHPLC-HRMS metabolome data to mzXML files using ProteoWizard MS Convert. The mzXML files were uploaded to XCMS software for data processing including peak detection, retention time correction, profile alignment, putative identification and isotope annotation (Kuhl et al., 2012; Tautenhahn et al., 2012; Gowda et al., 2014). We processed the data as a multi-group experiment and the parameter settings were as follows: centWave algorithm for feature detection (Δm/z = 20 ppm, minimum peak width = 5 s and maximum peak width = 20 s, S/N threshold = 10, mzdiff = 0.01, integration method = 1, prefilter peaks = 3, prefilter intensity = 10,000, noise filter = 5,000); obiwarp settings for retention time correction (profStep = 1); and parameters for chromatogram alignment, including mzwid = 0.02, minfrac = 0.5 and = 5 (Libiseller et al., 2015).
Lipidomics data (pre) processing
We converted the raw UHPLC-HRMS lipidome data to ABF files using Reifycs Abf Converter. The ABF files were uploaded to MS-DIAL RIKEN software downloadable from the PRIMe website for data processing including peak detection, deconvolution, profile alignment, putative identification, and isotope annotation (Tsugawa et al., 2015). The following parameters were used: retention time begin: 1 min; retention time end: 13 min; mass range begin: 0 Da; mass range end: 1500 Da; MS1 (centroiding) tolerance: 0.01 Da; smoothing level: 6 scans; minimum peak height: 10,000 amplitude; mass slice width: 0.1 Da; accurate mass tolerance (MS1): 0.01 Da; accurate mass tolerance (MS2): 0.005 Da; retention time tolerance for alignment: 0.05 min; MS1 tolerance for alignment: 0.015 Da.
Signal intensity drift correction
We exported the metabolite feature table (containing peak areas of all detected polar and lipid metabolites across all samples) to the R software (Chambers, 2008) and used the batchCorr package1 for signal intensity drift correction and noise filtering (CV (QC features) > 30; Brunius et al., 2016).
Data quality assessment and statistical analyses
We analyzed pooled quality control (QC) samples (representative of all analyzed samples per species) at regular intervals (every 5–6 samples) across the entire run to correct for the signal intensity drift and to filter chemical and/or informatic noise. Data was curated by applying the following filters: CV (QC) < 30%, peak intensity >10′000 ion counts, visual inspection of peak shape.
Metabolite levels were log transformed prior to statistical analysis. Positive and negative acquisition modes were merged to represent the global polar and lipid metabolome of fish mucus. We reduced the dimensionality of the data to better visualize the distribution of samples based on the molecular signature of the mucus found in the curated metabolome and lipidome using T-distributed stochastic neighbor embedding (t-SNE) as implemented in the R package “tsne”2. We also used the UMAP algorithm on our data, but it led to the same pattern as with the t-SNE and we therefore do not show it. We also used PCA analyses to reduce the dimensionality of the data prior to hierarchical clustering based on Manhattan distances. The analyses were done using the R packages “FactoMineR”3 and “factoextra”4.
Finally, we selected the metabolite features whose levels varied between different groups (clownfishes vs. damselfishes and generalists vs. specialists) using pairwise t-tests. We did not correct for phylogenetic relationships because of the small number of species involved and the fact that the main comparison (clownfish vs. damselfish) is done between two monophyletic groups. We corrected the p-values obtained using an optimized False Discovery Rate (FDR) approach based on the Benjamini Hochberg procedure (Benjamini and Hochberg, 1995) and set the FDR threshold to 0.05. For the metabolites that were significant after FDR correction, we evaluated the difference in mucus profiles by calculating the log-fold change of each metabolite between the groups tested. We used the peak area of each metabolite feature as the measure to scale the data. Finally, we highlighted the metabolite features showing the highest log-fold change in a circular bar plot using a custom-made R script5.
Metabolite and lipid identification
Following data pre-processing (metabolite/lipid profile alignment) and statistical analysis, metabolite/lipid features were matched against Human Metabolome Database (HMDB) (Wishart et al., 2007), Metlin (Smith et al., 2005) and LipidMaps (Fahy et al., 2007) based on accurate mass to charge ratio (m/z) with Δppm = 20, using the online CEU mass Mediator tool6.
We validated the identifications of the metabolites by MS/MS data matching (Mylonas et al., 2009) against the in-house PCDL database as well as METLIN7 (Smith et al., 2005; Benton et al., 2008). The spectra from lipid profiles were matched against the LipidBlast database for lipid identification (Koelmel et al., 2020). The GNPS platform was used to perform feature-based molecular networking (Aron et al., 2020) to help us identify features that could not be recognized by other available databases.
Results
In the general lipid metabolome profile, we detected several lipid classes such as carnitines, sphingolipids, glycerolipids, glycerophospholipids, and fatty acids. The polar metabolome profile of the fish mucus comprised molecular species belonging to amino acids, alkaloids, amines, sugars, peptides, glycerophospholipids and carnitines (See Supplementary Figure S1).
Metabolomic and lipidomic signature of clownfish and damselfish mucus
Raw polar metabolome (HILIC-HRMS) and lipidome profiles (RPLC-HRMS) comprised 3,366 and 4,126 features in total, respectively. Following noise [using CV (QCs) < 30%] and redundancy filtering (by feature annotation and grouping), we reduced these complex datasets to 938 and 903 unique polar and lipid metabolite features, respectively. Within these comprehensive profiles, we were able to confirm the identity of 46 known polar metabolites and 232 lipid species.
The t-SNE visualization of polar metabolite and of lipid signatures (including unknown features) revealed two main clusters: clownfishes and damselfishes (Figures 1A,B). While both signatures allowed for the discrimination between the clownfishes and the damselfishes, the polar metabolome profile was also species-specific and allowed for narrower grouping of individuals of the same species. The exceptions were two samples of the species A. perideraion that clustered close to A. ocellaris and A. percula. Based on the lipid signature, two individuals of the strict specialist A. biaculeatus and three individuals of A. frenatus also remained isolated farther away from their main cluster in the center of the t-SNE (Figure 1A).
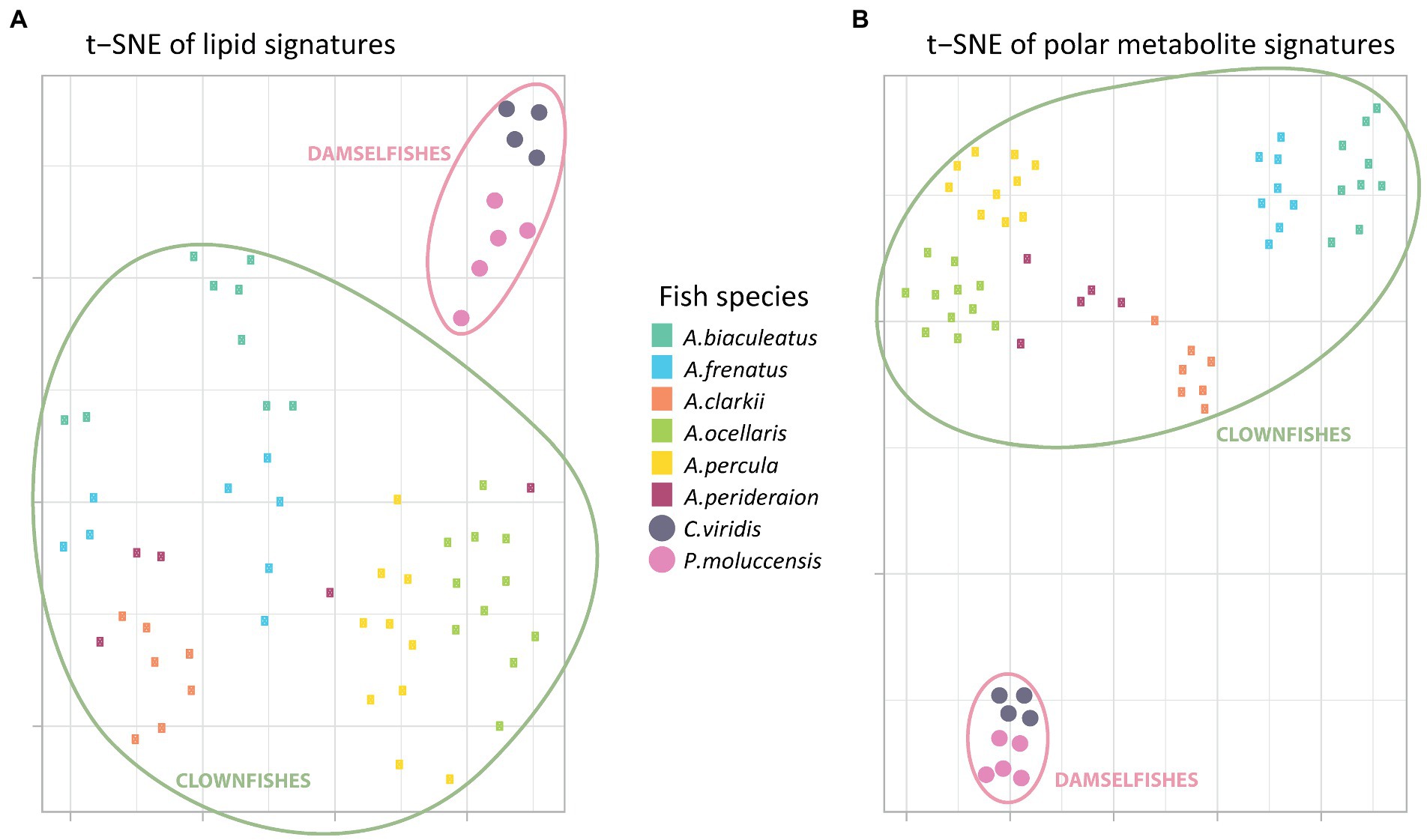
Figure 1. T-distributed stochastic neighbour embedding of the (A) global polar metabolome and (B) lipidome curated profiles (post data processing). Each little rectangle (clownfish) and circle (damselfish) represents one individual and each colour represents a fish species. The pink circles englobe the damselfishes and the green circles englobe the clownfishes.
Moreover, the t-SNE visualization of the polar metabolome signature (Figure 1B) showed three additional, well-defined, clusters. The first one included all samples from the most generalist species A. clarkii and A. perideraion. The second grouped the two strict specialists A. frenatus and A. biaculeatus, which share the same sea anemone host species. The third cluster represented the specialists and sister species A. ocellaris and A. percula with two individuals (the same as in Figure 1A) of the generalist A. perideraion.
The hierarchical clustering analyses done on the PCA loadings for both the metabolome and lipidome features corroborated the t-SNE clusters (Supplementary Figure S2). It further showed that the clownfish mucus composition was more variable than the damselfishes for both datasets. While clownfishes were again grouped in clear clusters based on species identity (except for the two individuals of A. perideraion and a few other exceptions; Supplementary Figure S2), they were distributed across the deeper splits of the hierarchical clustering. In contrast, the two damselfish species remained grouped in narrow clusters regardless of the data type.
We identified the features that were specific to clownfishes versus damselfishes and, within the clownfishes, generalists versus specialists. In the lipidome, the abundance patterns of 92 lipid features were significantly different {FDR < 0.05 and abs [log2(FC)] > 2} between clownfishes and damselfishes. Among them, 52 lipid species were more abundant in clownfish than in damselfish mucus, while 40 lipids showed the opposite pattern (Figure 2A). Most of the lipids present in higher levels in clownfish mucus belonged to the sphingolipid class of ceramides, whereas the more abundant species in damselfish mucus were mainly glycerophospholipids and glycerolipids (Figures 2B,C).
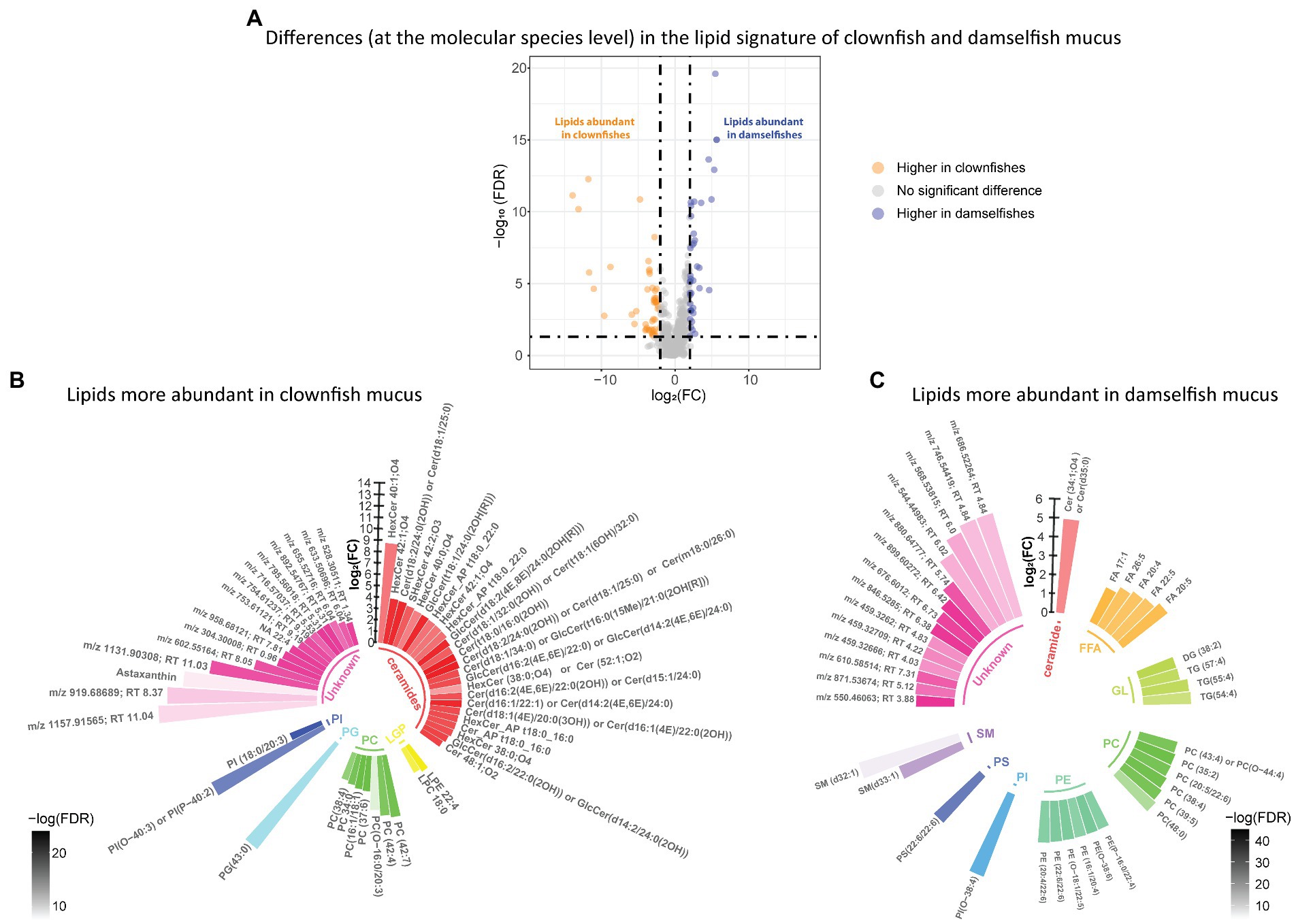
Figure 2. (A) Volcano plot of the global lipidome detected in the fish individuals. The log2(Fold change) limited at −2 and 2 highlights the lipid features higher in clownfishes (orange) and the lipid features higher in damselfishes (purple). These lipids are subsequently represented in circular bar plots (B) and (C) to highlight the identified lipids and lipid classes differing between these two fish groups. Each colour represents a lipid class and the different shades of one colour reflect the -log(FDR) of each lipid, calculated by performing a t-test.
Distinct lipid signatures of clownfish generalists versus specialists mucus were characterized by 63 lipid features {FDR < 0.05 and abs [log2(FC)] > 2}, out of which 16 were more abundant in generalists and 47 in specialists (Figure 3A). Among the lipid features identified, generalist mucus was richer in glycerolipids whereas specialist mucus was richer in phosphatidylcholines (Figures 3B,C).
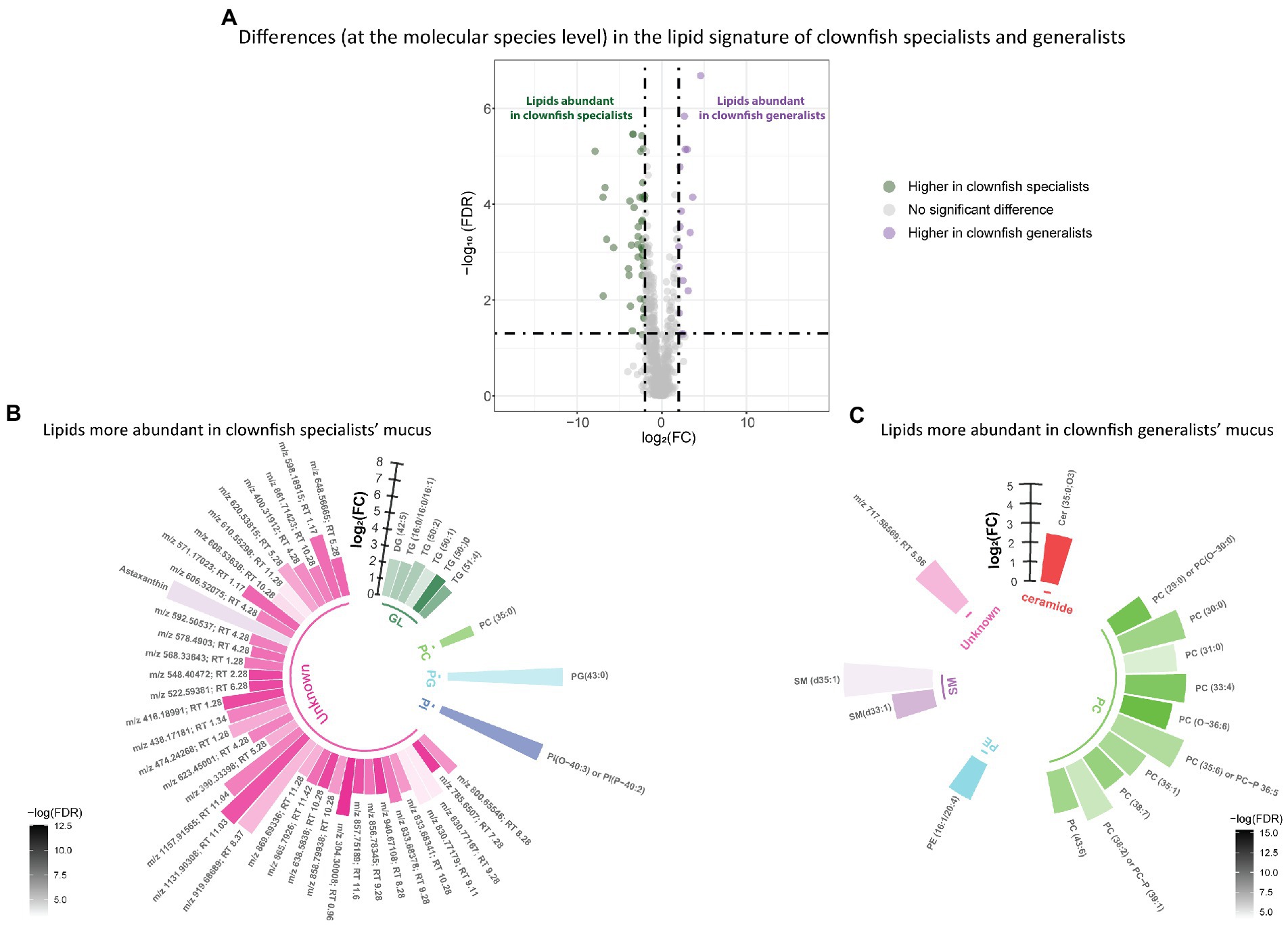
Figure 3. (A) Volcano plot of the global lipidome detected in the clownfish generalist and specialist individuals. The log2(Fold change) limit at −2 and 2 highlights the lipid features higher in clownfish specialists (dark green) and the lipids higher in clownfish generalists (purple). These lipids are subsequently represented in circular bar plots (B) and (C) to highlight the identified lipids and lipid classes differing between these two clownfish groups. Each colour represents a lipid class and the different shades of one colour reflect the -log(FDR) of each lipid, calculated performing a t-test.
Based on the polar metabolome signature, family specific clustering of clownfishes versus damselfishes was characterized by differential patterns of 224 metabolite features {FDR < 0.05 and abs [log2(FC)] > 2}: 175 were more abundant in clownfish mucus compared to damselfishes whereas 49 showed the reverse pattern (Figure 4A). The main class of polar features identified as more abundant in clownfish mucus were carnitines (polar lipids) followed by a few amino acids and derivatives, and peptides (Figure 4B). Different amino acids and peptides were, however, also present in higher levels in damselfish mucus compared to clownfish (Figure 4C). Among the unidentified polar features higher in damselfish mucus (i.e., “unknowns” that did not return any matches against databases), many eluted at 2 min, which suggested that these may be a distinct class of polar molecules specific to damselfish mucus (Figure 4C). Within clownfishes, 100 polar metabolite features significantly differed between clownfish generalists and specialists {FDR < 0.05 and abs [log2(FC)] > 2} with the majority present in higher levels in specialists (Figure 5). However, very few polar features differing amongst clownfish generalists and specialists could be identified (See Supplementary Table S1; Supplementary Table S2 for lipid and polar metabolite feature IDs in fish mucus respectively).
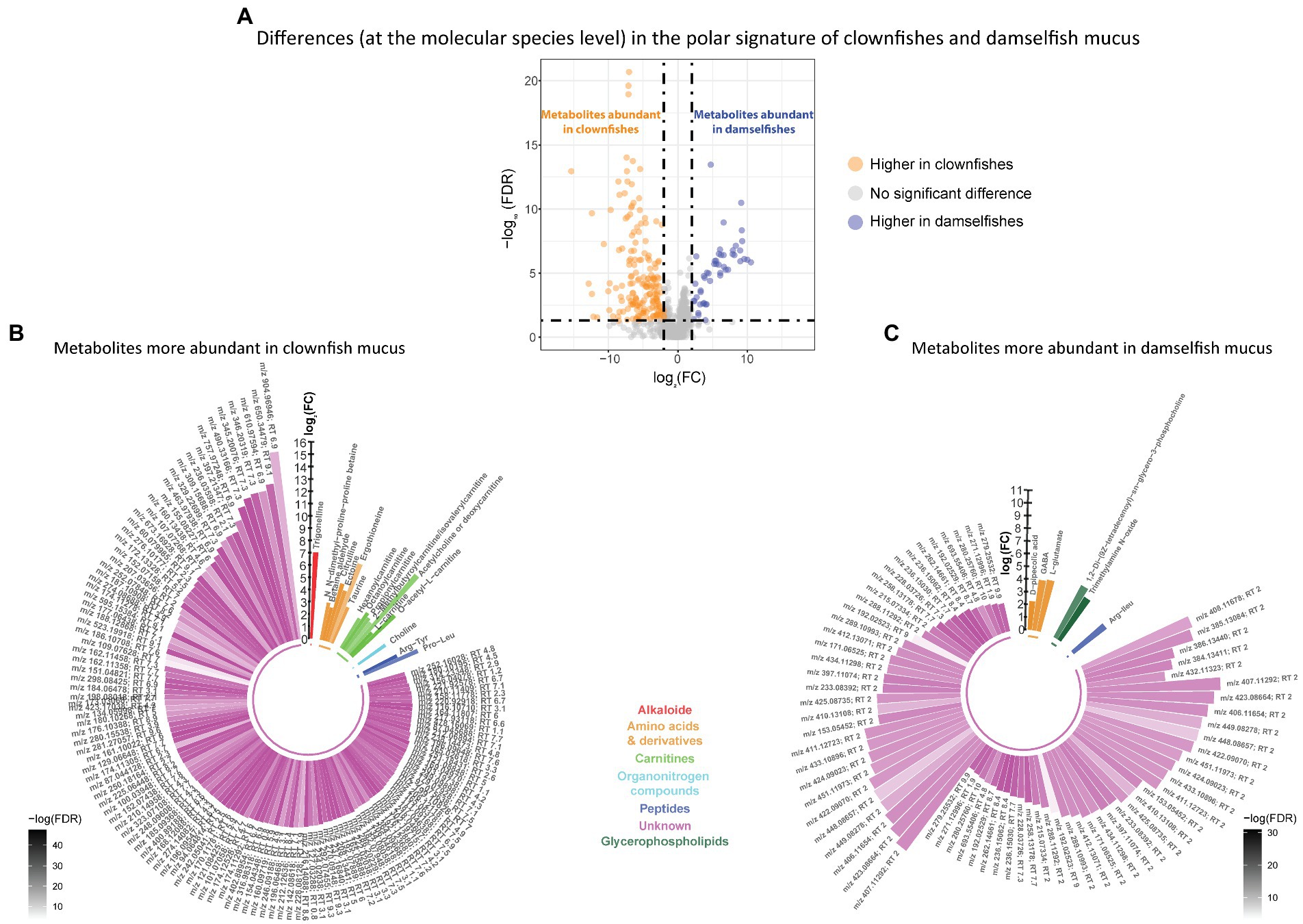
Figure 4. (A) Volcano plot of the global polar metabolome detected in the fish individuals. The log2(Fold change) limited at −2 and 2 highlights the polar metabolite features higher in clownfishes (orange) and the metabolite features higher in damselfishes (purple). These features are subsequently represented in circular bar plots (B) and (C) to highlight the identified metabolite features and classes differing between these two fish groups. Each colour represents a polar metabolite class and the different shades of one colour reflect the -log(FDR) of each lipid, calculated by performing a t-test.
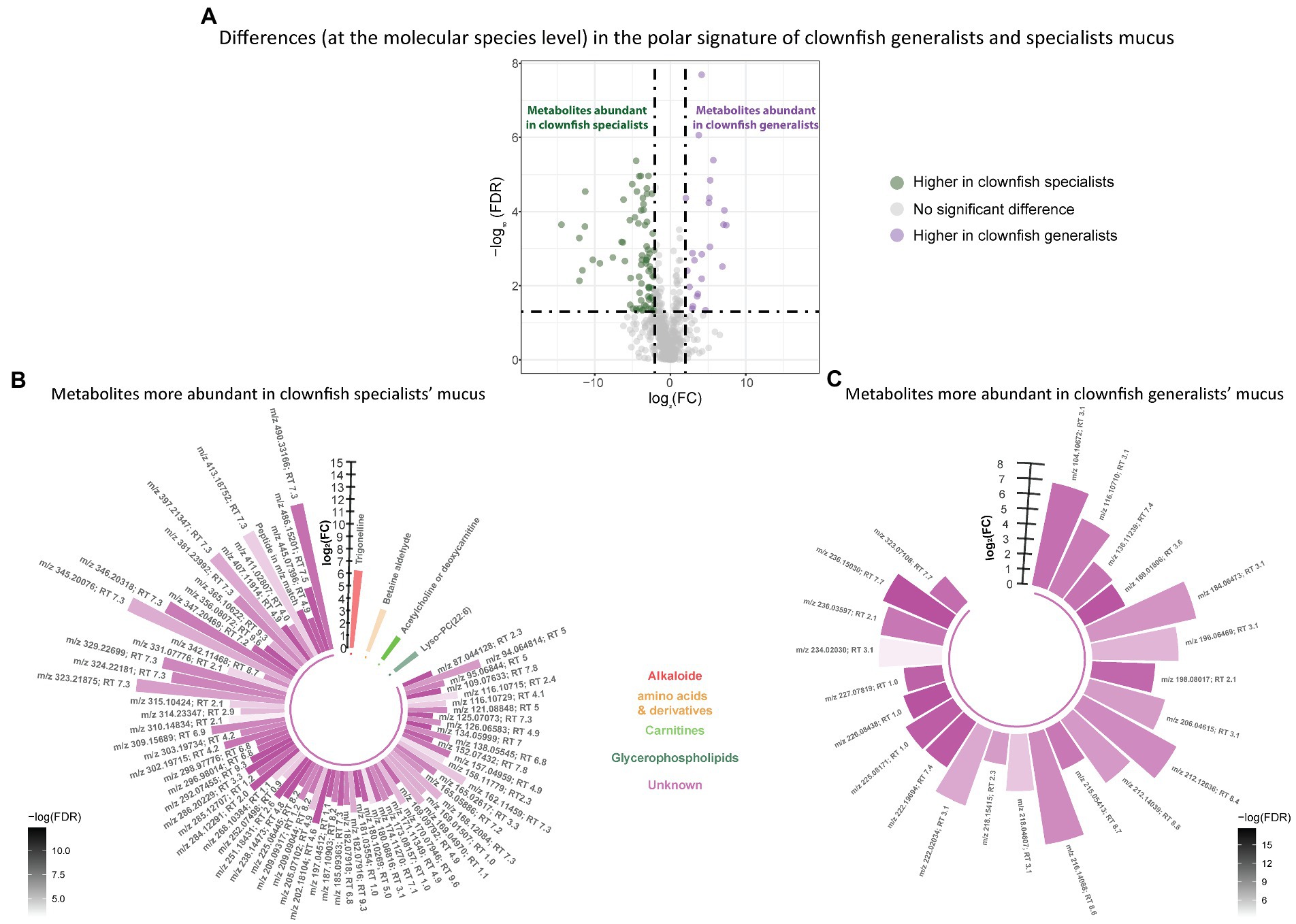
Figure 5. (A) Volcano plot of the global polar metabolome detected in the clownfish generalist and specialist individuals. The log2(Fold change) limit at −2 and 2 highlights the lipids higher in clownfish specialists (dark green) and the lipids higher in clownfish generalists (purple). These polar features are subsequently represented in circular bar plots (B) and (C) to highlight the identified features and polar metabolite classes differing between these two clownfish groups. Each colour represents a polar metabolite class and the different shades of one colour reflect the -log(FDR) of each feature, calculated by performing a t-test.
It should be noted that because of the nature of our samples, which come from non-model species, and incomplete metabolite/lipid databases available, there are still many lipids and polar metabolites significantly differing in our fish groups that could not be identified.
Discussion
The adaptation of clownfishes to their sea anemone hosts is still poorly understood, but it has been suggested that the surface skin mucus plays an important role in the interaction and specific recognition in this mutualism (Fautin, 1991; Mebs, 2009; Rolland et al., 2018). Fish mucus acts as a natural, semipermeable, physical and biochemical barrier that can dynamically change in chemical composition in response to environmental variations (Shephard, 1994; Reverter et al., 2018). The few studies that investigated the chemical composition of fish skin mucus mainly used freshwater fishes from aquacultures (Ekman et al., 2015; Ivanova et al., 2018) and we have little understanding of the mucus composition in marine fishes. Moreover, most of these studies investigated the polar metabolome signature and neglected the lipid composition of fish mucus.
Here, we tested the hypothesis that the adaptive radiation of clownfishes through the establishment of the mutualism with sea anemones led to a unique molecular footprint in their skin mucus to be recognized by their venomous host. We compared for the first time the polar and lipid metabolite composition of the mucus in eight marine fish species (acclimated to the same aquarium conditions) and showed that the mucus composition of clownfishes is significantly different from the mucus of the non-mutualistic damselfish relatives. Furthermore, the variability of the mucus signatures was greater among closely related clownfish species compared to distantly related damselfish species.
Metabolic signature variability between clownfish and damselfish clade
We detected several different classes of metabolite features in clownfish and damselfish skin mucus such as amino acids, peptides, carnitines, sphingolipids and glycerophospholipids (Supplementary Tables 1, 2; Supplementary Figure S1).
While the chemical characterization of polar and lipid metabolites in samples from non-model species remains challenging, we successfully characterized the presence of 46 polar metabolites and 232 lipid species in the global molecular profile of clownfish and damselfish mucus. Using pattern recognition approaches, we showed that the acquired metabolic signatures (including annotated and unknown features) are highly discriminative of the clownfish and damselfish skin mucus. These results suggest that the clownfishes have a specific chemical signature that could be associated with their adaptation to thrive within sea anemones. Although the current data do not allow us to fully understand the mechanisms involved and the metabolite function, we found that the signatures of the two damselfish species are very similar and far less variable compared to the signatures of closely related clownfish species. This was unexpected because the divergence time between the two damselfish species is much larger than between the clownfishes (50-million-year time span within the damselfish family whereas clownfishes diversified within the last 15 million years) (McCord et al., 2021). Instead, social structure and living behavior of damselfishes are more similar compared to those within the clownfish clade. It suggests that the host-specific association of clownfishes with sea anemones could lead to a larger variation in their mucus composition because of the interaction and adaptation to this new environment.
Among a multitude of differences in metabolic signatures, we revealed one major lipid class which was significantly more abundant in clownfish mucus. Clownfish mucus was characterized by the presence of higher (chemical) diversity and relative abundance of ceramides. Interestingly, most of the detected ceramides had an even number of carbons in their chains. Ceramides are precursors of most sphingolipids and one of the enzymes involved in their biosynthesis, serine palmitoyltransferase (SPT) (Merrill Jr, 2002), is highly conserved in both eukaryotes and bacteria (Yard et al., 2007). Eukaryotes, however, mainly synthesize even-chained sphingoid backbones and side chains, while bacterial sphingolipids consist mainly in odd-chain sphingoid backbone and fatty acyl chains (Zitomer et al., 2009). The roles of sphingolipids are many, ranging from plasma membrane constituents and inflammation regulators to important messengers in apoptosis and cell growth (Bikman and Summers, 2011; Olsen and Færgeman, 2017). The ceramides identified in clownfish mucus range from 32 to 50 carbon atoms and the majority has an even number of carbons in their acyl chains suggesting that they are synthesized by the clownfish itself. Ceramides could be responsible for the mediation of the interaction with sea anemones, and this particularly concerns species with a carbohydrate backbone containing glucose, galactose, N-acetylglucosamine, N-acetylgalactosamine, fucose, glucuronic acid or sialic acid (Miller-Podraza, 2000). This specific part of ceramides can be recognized by receptors of human and mouse immune cells thereby activating the production of several cytokines (Morales-Serna et al., 2007). The presence of sensory and chemoreceptors binding N-acetylated sugars triggers the nematocyst discharge (Ozacmak et al., 2021) although it has been shown that the co-presence of proline can counteract this effect (Watson and Roberts, 1995). The large number of ceramides found in the skin mucus of clownfishes compared to damselfish may act both as a physical and immune protective layer but also as the mediator of chemical communication between the fish and the host sea anemone. It further suggests that investigating the N-terminal sugars of glycolipids in clownfishes and damselfishes could shed light on the molecules triggering or inhibiting the discharge on these fish.
Compared to clownfish mucus, the lipidome part of the damselfish mucus is richer in phospholipid content. We identified several abundant phosphatidylcholine (PC) and phosphatidylethanolamine (PE) species containing polyunsaturated long chain fatty acids, such as C20:4 arachidonic acid and C22:6 docosahexaenoic acid. The glycerophospholipids detected in the damselfish mucus could either come from the fish itself or from the damselfish-specific skin microbiome (LIPID MAPS® Lipidomics Gateway, n.d.).
Among polar lipid-like metabolites, we identified several acylcarnitines that were more abundant in clownfish mucus compared to damselfishes. In mammals, and probably in fish too, carnitines play an important role in fatty acid oxidation and constitute the markers of mitochondrial function (Vissing et al., 2019). Unfortunately, there are, to our knowledge, no studies discussing the role of carnitines in fish skin mucus. It therefore remains challenging to explain this difference observed between the two groups. Certain polar features such as ectoine and ergothioneine found at higher abundance in clownfish mucus are usually produced by bacteria, which could therefore mean that certain features detected may be coming from the microbiome present on fish skin surface. Ectoine has a role in protecting against osmotic stress and in skin health (Kocherovets, 2019). Ergothioneine is an antioxidant produced by bacteria and fungi (Kitsanayanyong et al., 2021). This compound may be produced at higher levels after contact with sea anemones by the bacterial flora on clownfish skin, but this will have to be tested and cannot be shown with our data. Trigonelline was also found to be significantly higher in clownfish mucus. This compound is a toxin that has already been detected in sea urchins and cnidarians (Arumugam et al., 2013) and was defined as a fear inducing cue in mud crabs (Poulin, 2017). The role of this compound in fish mucus is not known, but it may keep certain predators away from sea anemone locations if the chemical cue is released by clownfishes.
In damselfishes, the amino acids GABA and L-glutamate were found to be present at higher levels compared to clownfish mucus, but no specific role of these amino acids at the skin surface has been described. Amongst the unknown polar features higher in damselfish mucus, there are numerous molecules eluting at 2 min with respect to the applied gradient. This group accounted for most of the difference between clownfish and damselfish mucus and may therefore be an important portion of the moderately polar signature of damselfish mucus to be further explored.
Metabolic signature variability between specialists and generalists
The acquired metabolic signatures of mucus also allowed for the discrimination between generalists and specialists within the clownfish clade. The main lipid classes contributing to the differentiation between generalists and specialists mucus were sphingomyelins, phosphatidylcholines and some glycerolipids. Sphingomyelins are sphingolipids, considered as inactive precursors of ceramides, which suggest again their implication in the mediation of the mutualistic interaction with sea anemones.
The acquired metabolic signatures are highly species-specific. This was the case even for some species (e.g., the specialist A. biaculeatus and the generalist A. perideraion) whose individuals were sampled from different geographical locations in Indonesia. Metabolomic patterns are often strongly influenced by external environmental cues (Cachet et al., 2015), but the strong species-specific patterns that we detected could be due to either genetically induced responses (Ivanišević et al., 2011) or to the specific interaction with the species of host sea anemones from which they were reared in their natural habitat. Unfortunately, the latter hypothesis cannot be confirmed because we lack this information; further experiments should be done to test host influence on mucus molecular patterns.
The two strict specialists A. frenatus and A. biaculeatus which associate only with E. quadricolor in their natural environment and we observed that they clustered in proximity on the t-SNEs, suggesting that host preference may impact the metabolites present in their mucus (Lindon et al., 2007). It has already been shown that, in plant - pathogen and plant - fungi interactions, a metabolic shift occurred in the plant after penetration of the pathogen or after association with fungal endophytes (Jones et al., 2011; Nieva et al., 2021). This topic is scarcely investigated in marine non-model organisms, but suggests that for clownfishes, the external environmental factor weighing the most in mucus chemical modification could be the physical interaction with the host anemone. Since the study of clownfish and anemone microbiomes showed that after several days of symbiotic mutualism the clownfish microbiome shifts towards a similar composition as the anemone (Roux et al., 2019), it is plausible that this shift also occurs at a metabolite level. The metabolites present in anemone mucus should also be characterized to test this hypothesis since little is known about the anemone mucus chemical composition.
Considering the phylogenetic distance between the clownfish species in our study (Litsios et al., 2012), the mucus of A. frenatus, a strict specialist, clusters near one of the other specialists of this study. In addition, although the two generalist species are not very close phylogenetically, they also had a very similar chemical composition of their mucus. These results suggest that the chemical signature of fish mucus is species-specific, as a product of species-specific gene activity; there may also be an imprinted signature reflecting the choice of habitat such as the species of host sea anemone in natural environments.
Conclusion
The small molecule composition of clownfish mucus differs significantly from the one of damselfish. This difference could weigh on the ability of clownfishes to establish a symbiotic mutualism with sea anemones: clownfish generalists and specialists also showed distinct molecular profiles of their mucus. The sphingolipid class of ceramides is a lipid fingerprint specific to clownfish mucus when compared to damselfish mucus. Because of their biochemical properties, these molecules could play a crucial role in the clownfish-sea anemone interaction, although this needs to be further investigated by exposing the clownfishes to a host anemone and monitoring the ceramide content in their mucus. Moreover, carnitines and several amino acid derivatives may also be important elements in this marine mutualism. The symbiotic relationship between clownfishes and sea anemones remains a complex interaction that needs to be further investigated to fully understand the mechanisms involved. Our study provides new results supporting the hypothesis whereby fish skin mucus does play an important role in the symbiotic mutualism between clownfishes and sea anemones. Clownfishes could have evolved upregulating or down regulating certain metabolic pathways that would provide the necessary chemical language favoring their recognition to their anemone hosts.
Data availability statement
The datasets presented in this study can be found in online repositories. The names of the repository/repositories and accession number(s) can be found at: https://zenodo.org/record/6420973#.Yu0UtxxBy4R.
Ethics statement
Ethical review and approval was not required for the animal study because Non invasive sampling. All procedures were performed in accordance with protocols approved by the veterinary authority of the Canton de Vaud.
Author contributions
SH conducted the experiments, analyzed the data and wrote the article. TT and HG-A helped with the metabolomics and lipidomics data and methods of the manuscript. The research was supervised by JI and NS who also helped writing this article. All authors contributed to the article and approved the submitted version.
Funding
The work was funded by a Swiss National Science Foundation grant to NS (grant 310030_185223) as well as by funds from the University of Lausanne.
Acknowledgments
We would like to thank Linda Dib and Théo Cavinato for their help and discussion during the analyses of the metabolomic data. Many thanks to Fabio Caravaglio and AQUA-Home in Aigle, Switzerland for the maintenance of the fish individuals and aquariums. The bioinformatic analyses were done on the Computing Infrastructure of DCSR of the University of Lausanne while the metabolomic data were obtained in the Metabolomics Unit of the University of Lausanne.
Conflict of interest
The authors declare that the research was conducted in the absence of any commercial or financial relationships that could be construed as a potential conflict of interest.
Publisher’s note
All claims expressed in this article are solely those of the authors and do not necessarily represent those of their affiliated organizations, or those of the publisher, the editors and the reviewers. Any product that may be evaluated in this article, or claim that may be made by its manufacturer, is not guaranteed or endorsed by the publisher.
Supplementary material
The Supplementary material for this article can be found online at: https://www.frontiersin.org/articles/10.3389/fevo.2023.1050083/full#supplementary-material
Footnotes
1. ^https://gitlab.com/CarlBrunius/batchCorr
2. ^github.com/jdonaldson/rtsne/
4. ^www.sthda.com/english/rpkgs/factoextra
5. ^https://github.com/phylolab/saraheim.git
References
Aron, A. T., Gentry, E. C., McPhail, K. L., Nothias, L. F., Nothias-Esposito, M., Bouslimani, A., et al. (2020). Reproducible molecular networking of untargeted mass spectrometry data using GNPS. Nat. Protoc. 15, 1954–1991. doi: 10.1038/s41596-020-0317-5
Arumugam, M., Balasubramanian, T., and Kim, S. -K. (2013). “Marine toxins for natural products drug discovery” in Marine Biomaterials: Characterization, Isolation and Applications (Boca Raton: CRC Press), 89.
Atema, J. (1995). Chemical signals in the marine environment: dispersal, detection, and temporal signal analysis. Proc. Natl. Acad. Sci. U. S. A. 92, 62–66. doi: 10.1073/pnas.92.1.62
Benjamini, Y., and Hochberg, Y. (1995). Controlling the false discovery rate: a practical and powerful approach to multiple testing. J. R. Stat. Soc. 57, 289–300. doi: 10.1111/j.2517-6161.1995.tb02031.x
Benton, H. P., Wong, D. M., Trauger, S. A., and Siuzdak, G. (2008). XCMS2: processing tandem mass spectrometry data for metabolite identification and structural characterization. Anal. Chem. 80, 6382–6389. doi: 10.1021/ac800795f
Biedermann, P. H. W., and Kaltenpoth, M. (2014). New synthesis: the chemistry of partner choice in insect-microbe mutualisms. J. Chem. Ecol. 40:99. doi: 10.1007/s10886-014-0382-8
Bikman, B. T., and Summers, S. A. (2011). Ceramides as modulators of cellular and whole-body metabolism. J. Clin. Invest. 121, 4222–4230. doi: 10.1172/JCI57144
Breithaupt, T., and Thiel, M. (2010). Chemical Communication in Crustaceans. Berlin: Springer Science & Business Media.
Broadhurst, D., Goodacre, R., Reinke, S. N., Kuligowski, J., Wilson, I. D., Lewis, M. R., et al. (2018). Guidelines and considerations for the use of system suitability and quality control samples in mass spectrometry assays applied in untargeted clinical metabolomic studies. Metabolomics 14:72. doi: 10.1007/s11306-018-1367-3
Brunius, C., Shi, L., and Landberg, R. (2016). Large-scale untargeted LC-MS metabolomics data correction using between-batch feature alignment and cluster-based within-batch signal intensity drift correction. Metabolomics 12:173. doi: 10.1007/s11306-016-1124-4
Cachet, N., Genta-Jouve, G., Ivanisevic, J., Chevaldonné, P., Sinniger, F., Culioli, G., et al. (2015). Metabolomic profiling reveals deep chemical divergence between two morphotypes of the zoanthid Parazoanthus axinellae. Sci. Rep. 5:8282. doi: 10.1038/srep08282
Caulier, G., Flammang, P., Gerbaux, P., and Eeckhaut, I. (2013). When a repellent becomes an attractant: harmful saponins are kairomones attracting the symbiotic harlequin crab. Sci. Rep. 3:2639. doi: 10.1038/srep02639
Chapin III, F. S., Matson, P. A., and Vitousek, P. M. (2011). Principles of Terrestrial Ecosystem Ecology. Springer, New York.
Dash, S., Das, S. K., Samal, J., and Thatoi, H. N. (2018). Epidermal mucus, a major determinant in fish health: a review. Iran. J. Vet. Res. 19, 72–81.
Ekman, D. R., Skelton, D. M., Davis, J. M., Villeneuve, D. L., Cavallin, J. E., Schroeder, A., et al. (2015). Metabolite profiling of fish skin mucus: a novel approach for minimally-invasive environmental exposure monitoring and surveillance. Environ. Sci. Technol. 49, 3091–3100. doi: 10.1021/es505054f
Elliott, J. K., and Mariscal, R. N. (2001). Coexistence of nine anemonefish species: differential host and habitat utilization, size and recruitment. Mar. Biol. 138, 23–36. doi: 10.1007/s002270000441
Esteban, M. A. (2012). An overview of the immunological defenses in fish skin. ISRN Immunol. 2012:853470. doi: 10.5402/2012/853470
Fahy, E., Sud, M., Cotter, D., and Subramaniam, S. (2007). LIPID MAPS online tools for lipid research. Nucleic Acids Res. 35, W606–W612. doi: 10.1093/nar/gkm324
Fautin, D.G. (1991). The Anemonefish Symbiosis: What is known and what is not Symbiosis. Philadelphia, PA: Balaban Publishers. Available at: https://dalspace.library.dal.ca/bitstream/handle/10222/77098/VOLUME%2010-NUMBERS%201,2,3-1991-PAGE%2023.pdf?sequence=1.
Gallart-Ayala, H., Konz, I., Mehl, F., Teav, T., Oikonomidi, A., Peyratout, G., et al. (2018). A global HILIC-MS approach to measure polar human cerebrospinal fluid metabolome: Exploring gender-associated variation in a cohort of elderly cognitively healthy subjects. Analytica Chimica Acta 1037, 327–337.
Gowda, H., Ivanisevic, J., Johnson, C. H., Kurczy, M. E., Benton, H. P., Rinehart, D., et al. (2014). Interactive XCMS online: simplifying advanced metabolomic data processing and subsequent statistical analyses. Anal. Chem. 86, 6931–6939. doi: 10.1021/ac500734c
Guardiola, F. A., Cuesta, A., Abellán, E., Meseguer, J., and Esteban, M. A. (2014). Comparative analysis of the humoral immunity of skin mucus from several marine teleost fish. Fish Shellfish Immunol. 40, 24–31. doi: 10.1016/j.fsi.2014.06.018
Hagedorn, M., Carter, V., Zuchowicz, N., Phillips, M., Penfield, C., Shamenek, B., et al. (2015). Trehalose is a chemical attractant in the establishment of coral symbiosis. PLoS One 10:e0117087. doi: 10.1371/journal.pone.0117087
Hay, M. E. (2009). Marine chemical ecology: chemical signals and cues structure marine populations, communities, and ecosystems. Annu. Rev. Mar. Sci. 1, 193–212. doi: 10.1146/annurev.marine.010908.163708
Hojo, M. K., Yamamoto, A., Akino, T., Tsuji, K., and Yamaoka, R. (2014). Ants use partner specific odors to learn to recognize a mutualistic partner. PLoS One 9:e86054. doi: 10.1371/journal.pone.0086054
Ivanišević, J., Thomas, O. P., Lejeusne, C., Chevaldonné, P., and Pérez, T. (2011). Metabolic fingerprinting as an indicator of biodiversity: towards understanding inter-specific relationships among Homoscleromorpha sponges. Metabolomics 7. Springer Science and Business Media LLC, 289–304. doi: 10.1007/s11306-010-0239-2
Ivanova, L., Tartor, H., Grove, S., Kristoffersen, A. B., and Uhlig, S. (2018). Workflow for the targeted and untargeted detection of small metabolites in fish skin mucus. Fishes of Sahul. J. Aust. N. G. Fishes Assoc. 3:21. doi: 10.3390/fishes3020021
Jones, O. A. H., Maguire, M. L., Griffin, J. L., Jung, Y. H., Shibato, J., Rakwal, R., et al. (2011). Using metabolic profiling to assess plant-pathogen interactions: an example using rice (Oryza sativa) and the blast pathogen Magnaporthe grisea. Eur. J. Plant Pathol. 129, 539–554. doi: 10.1007/s10658-010-9718-6
Kitsanayanyong, L., Pahila, J., Ishikawa, Y., Koyama, T., Kiron, V., and Ohshima, T. (2021). Functional identification of ergothioneine transporter in rainbow trout (Oncorhynchus mykiss). Comp. Biochem. Physiol. B Biochem. Mol. Biol. 256:110631. doi: 10.1016/j.cbpb.2021.110631
Knowlton, N., and Rohwer, F. (2003). Multispecies microbial mutualisms on coral reefs: the host as a habitat. Am. Nat. 162, S51–S62. doi: 10.1086/378684
Kocherovets, V. I. (2019). Ectoine a microbial metabolite with unique biotherapeutic properties. Pediatric 1, 108–115. doi: 10.26442/26586630.2019.1.190314
Koelmel, J. P., Li, X., Stow, S. M., Sartain, M. J., Murali, A., Kemperman, R., et al. (2020). Lipid annotator: towards accurate annotation in non-targeted liquid chromatography high-resolution tandem mass spectrometry (LC-HRMS/MS) Lipidomics using a rapid and user-friendly software. Metabolites 10:101. doi: 10.3390/metabo10030101
Kuhl, C., Tautenhahn, R., Böttcher, C., Larson, T. R., and Neumann, S. (2012). CAMERA: an integrated strategy for compound spectra extraction and annotation of liquid chromatography/mass spectrometry data sets. Anal. Chem. 84, 283–289. doi: 10.1021/ac202450g
Libiseller, G., Dvorzak, M., Kleb, U., Gander, E., Eisenberg, T., Madeo, F., et al. (2015). IPO: a tool for automated optimization of XCMS parameters. BMC Bioinformatics 16:118. doi: 10.1186/s12859-015-0562-8
Lindon, J. C., Nicholson, J. K., and Holmes, E. (2007). “Applications of metabonomics within environmental toxicology” in The Handbook of Metabonomics and Metabolomics. eds. J. C. Lindon, J. K. Nicholson, and E. Holmes (Amsterdam, Netherlands: Elsevier), 517–532.
LIPID MAPS® Lipidomics Gateway. (n.d.). Available at: http://www.lipidmaps.org/resources/lipidweb/index.php?page=lipids/simple/lipopep/index.htm (Accessed February 16, 2022).
Litsios, G., Sims, C. A., Wüest, R. O., Pearman, P. B., Zimmermann, N. E., and Salamin, N. (2012). Mutualism with sea anemones triggered the adaptive radiation of clownfishes. BMC Evol. Biol. 12:212. doi: 10.1186/1471-2148-12-212
Madio, B., King, G. F., and Undheim, E. A. B. (2019). Sea anemone toxins: a structural overview. Mar. Drugs 17, 17:325. doi: 10.3390/md17060325
Marcionetti, A., Rossier, V., Roux, N., Salis, P., Laudet, V., and Salamin, N. (2019). Insights into the genomics of clownfish adaptive radiation: genetic basis of the mutualism with sea anemones. Genome Biol. Evol. 11, 869–882. doi: 10.1093/gbe/evz042
McCord, C. L., Nash, C. M., Cooper, W. J., and Westneat, M. W. (2021). Phylogeny of the damselfishes (Pomacentridae) and patterns of asymmetrical diversification in body size and feeding ecology. PLoS One 16:e0258889. doi: 10.1371/journal.pone.0258889
Mebs, D. (2009). Chemical biology of the mutualistic relationships of sea anemones with fish and crustaceans. Toxicon 54, 1071–1074. doi: 10.1016/j.toxicon.2009.02.027
Merrill, A. H. Jr. (2002). De novo sphingolipid biosynthesis: a necessary, but dangerous, pathway. J. Biol. Chem. 277, 25843–25846. doi: 10.1074/jbc.R200009200
Miller-Podraza, H. (2000). Polyglycosylceramides, poly-N-acetyllactosamine-containing Glycosphingolipids: methods of analysis, structure, and presumable biological functions. Chem. Rev. 100, 4663–4682. doi: 10.1021/cr990347o
Morales-Serna, J. A., Boutureira, O., Díaz, Y., Matheu, M. I., and Castillón, S. (2007). Recent advances in the glycosylation of sphingosines and ceramides. Carbohydr. Res. 342, 1595–1612. doi: 10.1016/j.carres.2007.03.028
Mylonas, R., Mauron, Y., Masselot, A., Binz, P. A., Budin, N., Fathi, M., et al. (2009). X-rank: a robust algorithm for small molecule identification using tandem mass spectrometry. Anal. Chem. 81, 7604–7610. doi: 10.1021/ac900954d
Nieva, A. S., Romero, F. M., Erban, A., Carrasco, P., Ruiz, O. A., and Kopka, J. (2021). Metabolic profiling and metabolite correlation network analysis reveal that Fusarium solani induces differential metabolic responses in Lotus japonicus and Lotus tenuis against severe phosphate starvation. J. Fungi (Basel) 7:765. doi: 10.3390/jof7090765
Olsen, A. S. B., and Færgeman, N. J. (2017). Sphingolipids: membrane microdomains in brain development, function and neurological diseases. Open Biol. 7, 7:170069. doi: 10.1098/rsob.170069
Ozacmak, V. H., Arrieta, A. R., Thorington, G. U., and Hessinger, D. A. (2021). N-acetyl Neuraminic acid (NANA) activates L-type calcium channels on isolated tentacle supporting cells of the sea anemone (Aiptasia pallida). Biol. Bull. 241, 196–207. doi: 10.1086/715844
Palaksha, K. J., Shin, G.-W., Kim, Y.-R., and Jung, T. S. (2008). Evaluation of non-specific immune components from the skin mucus of olive flounder (Paralichthys olivaceus). Fish Shellfish Immunol. 24, 479–488. doi: 10.1016/j.fsi.2008.01.005
Pohnert, G., Steinke, M., and Tollrian, R. (2007). Chemical cues, defence metabolites and the shaping of pelagic interspecific interactions. Trends Ecol. Evol. 22, 198–204. doi: 10.1016/j.tree.2007.01.005
Poulin, R. X. (2017). Waves of Communication: Metabolomics Describe the Nature and Role of Waterborne Cues in the Marine Environment. Atlanta, GA: Georgia Institute of Technology.
Putri, S. P., Yamamoto, S., Tsugawa, H., and Fukusaki, E. (2013). Current metabolomics: technological advances. J. Biosci. Bioeng. 116, 9–16. doi: 10.1016/j.jbiosc.2013.01.004
Quenouille, B., Bermingham, E., and Planes, S. (2004). Molecular systematics of the damselfishes (Teleostei: Pomacentridae): Bayesian phylogenetic analyses of mitochondrial and nuclear DNA sequences. Mol. Phylogenet. Evol. 31, 66–88. doi: 10.1016/S1055-7903(03)00278-1
Reverter, M., Sasal, P., Tapissier-Bontemps, N., Lecchini, D., and Suzuki, M. (2017). Characterisation of the gill mucosal bacterial communities of four butterflyfish species: a reservoir of bacterial diversity in coral reef ecosystems. FEMS Microbiol. Ecol. 93:fix051. doi: 10.1093/femsec/fix051
Reverter, M., Tapissier-Bontemps, N., Lecchini, D., Banaigs, B., and Sasal, P. (2018). Biological and ecological roles of external fish mucus: a review. Fishes 3:41. doi: 10.3390/fishes3040041
Rolland, J., Silvestro, D., Litsios, G., Faye, L., and Salamin, N. (2018). Clownfishes evolution below and above the species level. Proc. Biol. Sci. R. Soc. 285, 285:20171796. doi: 10.1098/rspb.2017.1796
Roux, N., Lami, R., Salis, P., Magré, K., Romans, P., Masanet, P., et al. (2019). Sea anemone and clownfish microbiota diversity and variation during the initial steps of symbiosis. Sci. Rep. 9:19491. doi: 10.1038/s41598-019-55756-w
Shephard, K. L. (1994). Functions for fish mucus. Rev. Fish Biol. Fish. 4, 401–429. doi: 10.1007/BF00042888
Smith, C. A., O’Maille, G., Want, E. J., Qin, C., Trauger, S. A., Brandon, T. R., et al. (2005). METLIN: a metabolite mass spectral database. Ther. Drug Monit. 27, 747–751. doi: 10.1097/01.ftd.0000179845.53213.39
Stat, M., Morris, E., and Gates, R. D. (2008). Functional diversity in coral–dinoflagellate symbiosis. Proc. Natl. Acad. Sci. U. S. A. 105, 9256–9261. doi: 10.1073/pnas.0801328105
Swain, P., Dash, S., Sahoo, P. K., Routray, P., Sahoo, S. K., Gupta, S. D., et al. (2007). Non-specific immune parameters of brood Indian major carp Labeo rohita and their seasonal variations. Fish Shellfish Immunol. 22, 38–43. doi: 10.1016/j.fsi.2006.03.010
Tautenhahn, R., Cho, K., Uritboonthai, W., Zhu, Z., Patti, G. J., and Siuzdak, G. (2012). An accelerated workflow for untargeted metabolomics using the METLIN database. Nat. Biotechnol. 30, 826–828. doi: 10.1038/nbt.2348
Tsugawa, H., Cajka, T., Kind, T., Ma, Y., Higgins, B., Ikeda, K., et al. (2015). MS-DIAL: data-independent MS/MS deconvolution for comprehensive metabolome analysis. Nat. Methods 12, 523–526. doi: 10.1038/nmeth.3393
Vissing, C. R., Dunø, M., Wibrand, F., Christensen, M., and Vissing, J. (2019). Hydroxylated long-chain Acylcarnitines are biomarkers of mitochondrial myopathy. J. Clin. Endocrinol. Metab. 104, 5968–5976. doi: 10.1210/jc.2019-00721
Wang, S., Wang, Y., Ma, J., Ding, Y., and Zhang, S. (2011). Phosvitin plays a critical role in the immunity of zebrafish embryos via acting as a pattern recognition receptor and an antimicrobial effector. J. Biol. Chem. 286, 22653–22664. doi: 10.1074/jbc.M111.247635
Watson, G. M., and Roberts, J. (1995). Chemoreceptor‐mediated polymerization and depolymerization of actin in hair bundles of sea anemones. Cell Moti. Cytoskeleton 30, 208–220.
Wishart, D. S., Tzur, D., Knox, C., Eisner, R., Guo, A. C., Young, N., et al. (2007). HMDB: the human Metabolome database. Nucleic Acids Res. 35, D521–D526. doi: 10.1093/nar/gkl923
Yard, B. A., Carter, L. G., Johnson, K. A., Overton, I. M., Dorward, M., Liu, H., et al. (2007). The structure of serine palmitoyltransferase; gateway to sphingolipid biosynthesis. J. Mol. Biol. 370, 870–886. doi: 10.1016/j.jmb.2007.04.086
Zaccone, G., Kapoor, B. G., and Fasulo, S. (2001). Structural, histochemical and functional aspects of the epidermis of fishes. Adv. Mar. 40, 253–276. doi: 10.1016/S0065-2881(01)40004-6
Zitomer, N. C., Mitchell, T., Voss, K. A., Pruett, S. T., Garnier-Amblard, E. C., Liebeskind, L. S., et al. (2009). Ceramide synthase inhibition by fumonisin B1 causes accumulation of 1-deoxysphinganine: a novel category of bioactive 1-deoxysphingoid bases and 1 deoxydihydroceramides biosynthesized by mammalian cell lines and animals. J. Biol. 284, 4786–4795. doi: 10.1074/jbc.M808798200
Keywords: clownfish, damselfish, metabolomics, lipidomics, molecular profiling, chemical signature
Citation: Heim S, Teav T, Gallart-Ayala H, Ivanisevic J and Salamin N (2023) Divergence in metabolomic profile in clownfish and damselfish skin mucus. Front. Ecol. Evol. 11:1050083. doi: 10.3389/fevo.2023.1050083
Edited by:
Ernesto Mollo, National Research Council (CNR), ItalyReviewed by:
Benjamin Marie, Délégation Ile-de-France Sud (CNRS), FranceAntoni Ibarz, University of Barcelona, Spain
Copyright © 2023 Heim, Teav, Gallart-Ayala, Ivanisevic and Salamin. This is an open-access article distributed under the terms of the Creative Commons Attribution License (CC BY). The use, distribution or reproduction in other forums is permitted, provided the original author(s) and the copyright owner(s) are credited and that the original publication in this journal is cited, in accordance with accepted academic practice. No use, distribution or reproduction is permitted which does not comply with these terms.
*Correspondence: Sara Heim, ✉ sara.heim@unil.ch; Julijana Ivanisevic, ✉ julijana.ivanisevic@unil.ch; Nicolas Salamin, ✉ nicolas.salamin@unil.ch