Climatic Benefits From the 2006–2017 Avoided Deforestation in Amazonian Brazil
- 1Scion—New Zealand Forest Research Institute, Rotorua, New Zealand
- 2Center for Development Research (ZEF), University of Bonn, Bonn, Germany
- 3Institute for Food and Resource Economics (ILR), University of Bonn, Bonn, Germany
- 4National Institute for Research in Amazonia (INPA), Manaus, Brazil
Over three-fourths of Brazil's greenhouse gas (GHG) emissions originate from land-use/cover change (LUCC). Alarming rates of forest loss in the Amazon region gained international attention in the past, but deforestation declined substantially since 2004 (although the rate has trended upwards since 2012). This result conforms to the GHG emission reduction targets set by the National Climate Change Plan, originally divided into three phases: 2006–2009; 2010–2013; and 2014–2017. According to the plan, emission reductions are measured against a deforestation baseline, initially corresponding to the average forest loss observed from 1996 to 2005 (reference period). We used an artificial neural network (ANN) model to spatialize the plan's original three-phase baseline and compare (i) the simulated carbon emissions from the baseline scenario to (ii) the emissions from observed deforestation during 2006–2017. Baseline spatialization was performed at the state level and informed by the 2000–2004 deforestation patterns in each state, resulting in nine state-specific calibrated ANNs. Simulated (baseline) and observed deforestation were compared to five biomass-density maps in order to estimate Brazil's GHG emission reductions. Our results indicate that forest loss during the study period was 62,321 km2 lower than the baseline and associated with 1.5 ± 0.4 Pg of avoided CO2 emissions to the atmosphere. Virtually all emission reductions (93%) occurred in the states of Mato Grosso (74%) and Rondônia (19%). In contrast, Roraima, Amazonas, and Amapá states increased GHG emissions by 44.8, 36.7, and 14.7 Tg CO2, respectively. Lastly, we discuss the issue of attribution of deforestation reductions for results-based payments (REDD+) and the emission reduction certificates issued by the Amazon Fund.
Introduction
Sixty percent of the Amazon rainforest is located in Brazil. Besides holding high biodiversity levels, the forest plays a central role in water cycling and climate regulation (Nobre et al., 2016; Exbrayat et al., 2017; Khanna et al., 2017), and stores over 117 Pg of carbon (equivalent to 429 Pg of CO2) in aboveground woody vegetation alone across tropical South America (Baccini et al., 2012). Hence, conservation of the Amazon is of paramount importance to the success of global efforts to mitigate climate change like the Paris Agreement (Gullison et al., 2007; Rochedo et al., 2018). Given that 78% of the Brazilian greenhouse-gas (GHG) emissions originate from land-use/cover change (LUCC), of which two-thirds take place in the Amazon region (Brazil, 2014), protection of the rainforest has become the central component of Brazil's National Climate Change Plan announced in 2008 (Brazil, 2008) and revised in 2010 (Brazil, 2013a). In this study, we evaluate the plan's original three-phase GHG emission reduction targets for the 2006–2017 period by spatializing the plan's baseline deforestation and comparing it to the observed deforestation in Amazonia. We provide spatially-explicit estimates of the avoided forest loss and carbon emissions in each state by considering the changes in the deforestation patterns after 2004, when a major conservation reform was initiated in the country (Brazil, 2013b).
Historically, Brazil is among the tropical countries with the highest rates of forest loss, but annual deforestation declined substantially from 2004 to 2012 (Figure 1). Many factors were allegedly responsible for this outcome, e.g., shifts in commodity prices and currency exchange rates, as well as recent private and public conservation initiatives, in particular, the government's Action Plan for the Prevention and Control of Deforestation in the Legal Amazon (PPCDAm; Hargrave and Kis-Katos, 2013; Arima et al., 2014; Assunção et al., 2015; Börner et al., 2015). The PPCDAm, introduced in mid-2004, supported a series of integrated conservation activities divided into three thematic areas: (1) territorial planning and land tenure, including the creation of protected areas; (2) improved monitoring and law enforcement; and (3) incentives for the sustainable use of natural resources (Brazil, 2013b). The 2004–2007 period after the launch of the PPCDAm was characterized by declines in soy and beef prices and by the increase in the value of the Brazilian real against the US dollar by over 50%, making commodity exports much less profitable because export returns are received in dollars while all expenses are in Brazilian currency (Fearnside, 2017). Commodity prices recovered from 2008 onwards while deforestation rates continued to decline through 2012, showing the effects of the environmental governance reform more clearly during this period (Hargrave and Kis-Katos, 2013; Assunção et al., 2015).
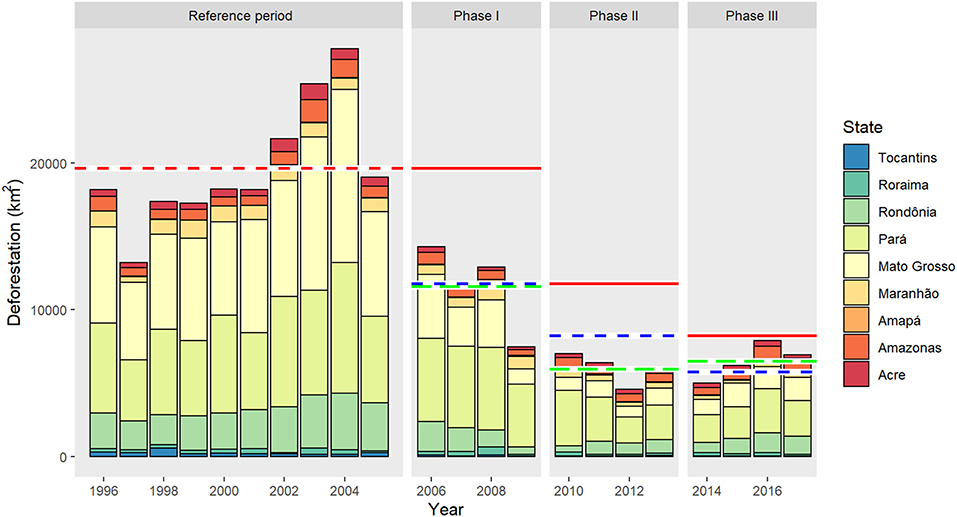
Figure 1. Brazil's original three-phase deforestation baseline set by the National Climate Change Plan (solid-red lines). Dashed-red line is the baseline reference period based on the average 1996–2005 deforestation. Dashed-blue lines represent deforestation reduction targets. Dashed-green lines are observed deforestation averages.
Among the most relevant activities promoted by the PPCDAm were: (1) the development of the DETER product (a satellite-based, early-warning system for detection of deforestation) by the National Institute for Space Research (INPE; Finer et al., 2018), which assisted law-enforcement actions on the ground (Arima et al., 2014); (2) the 2008 “blacklisting” of Amazonian municipalities with the highest levels of deforestation, which blocked access to public rural credit and legal deforestation permits in these municipalities (Cisneros et al., 2015); (3) the 2008 Resolution No. 3545 from the Brazilian Central Bank/National Monetary Council that blocked loans from public banks to landowners who were in nonconformance with environmental and rural regulations, and; (4) the 2008 Federal Decree No. 6514, which strengthened the 1997 Environmental Crimes Law and, consequently, the impacts of environmental fines and embargoes (Börner et al., 2015; Fearnside, 2017). Other important PPCDAm activities were the promotion of the Rural Environmental Registry (CAR) after 2012, leading to the construction of a national, spatially-explicit database of virtually all farms in the country, and the current Terra Legal program, which promotes land-tenure regularization in the Amazonian region (IPEA-GIZ-CEPAL, 2011; Duchelle et al., 2014; Azevedo et al., 2017). The PPCDAm also shaped the State-Level Plans for the Prevention and Control of Deforestation, the implementation of which started after 2008 (Brazil, 2013b). Still, since many of the reportedly most effective conservation measures only came into effect from 2008 onwards, the decline from 2004 through 2007 (≈70% of the total) cannot be attributed to these measures.
In addition, a controversial revision of the national Forest Code in 2012 granted amnesty to nearly 30 M ha illegally deforested prior to 2008 (Soares-Filho et al., 2014). According to INPE's data, from 2012 onwards deforestation trended upwards, reaching 7,900 km2 year−1 in 2018, 73% above the low point of 4,571 km2 year−1 in 2012, although still 72% below the high mark of 27,772 km2 year−1 in 2004. Moreover, Brazil's change of presidential administration in January 2019 has been accompanied by a significant weakening of environmental agencies and recent spikes in deforestation rates (Escobar, 2018; Tollefson, 2018; Abessa et al., 2019).
At the private level, two voluntary supply-chain initiatives to reduce deforestation were announced in response to pressure from non-governmental organizations and civil society, namely the Soy Moratorium of 2006 and the Beef Moratorium (or “Cattle Agreements”) of 2008 (Gibbs et al., 2015, 2016). Major soybean and beef traders who signed the moratoria committed not to purchase soybean or beef from recently deforested Amazonian areas. Nevertheless, rigorous impact evaluations suggest that the conservation outcomes associated with these interventions may have been much lower than initially conceived (Alix-Garcia and Gibbs, 2017; Klingler et al., 2018; Svahn and Brunner, 2018).
At the international level, 2005 marked the beginning of a new phase of negotiations on the role of avoided deforestation activities as recognized climate mitigation strategies at the 11th Conference of Parties (COP 11) to the United Nations Framework Convention on Climate Change (UNFCCC; Thompson et al., 2011). In 2007, REDD+ (Reducing Emissions from Deforestation and Forest Degradation in developing countries, and the role of conservation, sustainable forest management of forests, and enhancement of forest carbon stocks) activities were included in the Bali Action Plan (COP 13), in which parties were invited to voluntarily reduce emissions from deforestation and forest degradation (UN-REDD, 2015). The scope of REDD+ was defined in 2010 (COP 16) and procedures for countries to have GHG emission reductions from REDD+ activities recognized for results-based payments were set in 2013 (COP 19). Lastly, the 2015 Paris Agreement, formulated at COP 21, reinforced REDD+ as a key component of future global climate change mitigation efforts (UNFCCC, 2015).
Brazil announced its National Climate Change Plan in 2008, after most of the decline in deforestation rates had already occurred. This was a significant advance since Brazil's previous long resistance to any commitment to lower deforestation or emissions had been strongly influenced by the belief that the government would be incapable of controlling deforestation to fulfill any promises (Fearnside, 2012). The plan, aligned with other national conservation programs (in particular, the PPCDAm), included the country's commitment to achieving zero illegal deforestation in the Amazon by 2030 (Brazil, 2008)—note that the qualifier “illegal” leaves large tracts of land vulnerable to legal deforestation, for which authorization can more easily be obtained since the CAR implementation (Fearnside, 2017). To accomplish this goal, the plan set three consecutive deforestation reduction targets based on 4-year periods (Figure 1). The first target was to reduce the average rate of forest loss by 40% during 2006–2009 based on the 1996–2005 average (from 19,625 to 11,775 km2 year−1). An additional 30% reduction target was set for the two subsequent quadrennium, 2010–2013 (8,243 km2 year−1) and 2014–2017 (5,770 km2 year−1), based on their respective previous 4-year deforestation averages. In 2010, the government adjusted the initial deforestation reduction targets set by the plan (Federal Decree No. 7390). The new target aimed for 80% reduction in annual Amazonian deforestation, again in relation to the 1996–2005 average, by 2020 (equivalent to a reduction of 3,925 km2 year−1), as well as 40% reduction in annual clearing rates in the Cerrado (savanna) biome based on the 1999–2008 average (Brazil, 2013a).
Brazil's PPCDAm and the National Climate Change Plan were the basis for a series of REDD+-related measures announced by the country since 2008 (West, 2016). Among those are the establishment of the national Forest Reference Emission Level for Reducing Emissions from Deforestation in the Amazonia Biome for REDD+ Results-based Payments under the UNFCCC in 2014 (Brazil, 2014), the national REDD+ strategy, announced in 2016 and expected to launch in 2020 (Brazil, 2016), and the Amazon Fund, launched in 2008. The fund was designed to raise and manage donations for the promotion of actions aligned with the PPCDAm's goals to prevent, mitigate, and monitor deforestation, as well as to promote forest conservation and sustainable use of natural resources (Brazil, 2016). To date, the fund has raised over USD 1.2 billion in donations, mainly from the government of Norway, with smaller amounts from Germany and Petrobras (the Brazilian semi-public petroleum industry; Correa et al., 2019). In exchange for the donations, the Amazon Fund issues certificates of avoided carbon emissions from deforestation, which are nominal and non-transferable (Brazil, 2013b).
The combination of national conservation actions and international commitments has played a part in the declines of forest loss and consequential reductions in GHG emissions from LUCC, especially since 2008. Deforestation-control measures have also played a role in altering the spatial pattern of deforestation in the Amazon region (Arima et al., 2011; Rosa et al., 2012; Godar et al., 2014; Kalamandeen et al., 2018). Moreover, while most Amazonian states experienced a noticeable decline in forest loss between 2004 and 2012, deforestation trends are rising again in many states and in the region as a whole.
Methods
We used a spatially-explicit LUCC model, TerrSet v.18.2, to spatialize Brazil's 2006–2017 official deforestation baseline (Eastman, 2015; Figure 1). LUCC models generally simulate two complementary outcomes: (1) an expected amount of change over time; and (2) the spatial allocation of the change in the landscape. The first outcome is usually informed by observed changes in land use/cover between two or more points in time (i.e., historical trend). In this study, the amount of change was informed by the three-phase deforestation baseline set by the original National Climate Change Plan, based on the official statistics reported by INPE (solid red lines in Figure 1). Given the presence of state-level plans to control deforestation in the Amazon, we assume each state to share the same deforestation reduction targets (in percentages) as the Amazonian baseline.
The spatialization of the plan's deforestation baseline was based on state-level, likelihood-of-deforestation maps (≈1 km2 spatial resolution). The maps (one map for each state) were the output of the Multi-Layer Perceptron (MLP), an artificial neural network (ANN) algorithm in TerrSet (Sangermano et al., 2010). Observed LUCC patterns during the 2000–2004 calibration period obtained from the Global Forest Change dataset (Hansen et al., 2013)—before REDD+ gained international momentum and before the launch of Brazil's PPCDAm—were used to compute the likelihood of deforestation in each of the nine Amazonian states: Acre; Amapá; Amazonas; Maranhão; Mato Grosso; Pará; Rondônia; Roraima; and Tocantins (Figure 2). For each state, the MLP was informed by random samples of forest pixels that underwent LUCC or remained as forest throughout the calibration period. Based on ~10,000 interactions, the MLP established complex mathematical relationships following an ANN structure among 29 explanatory maps (Supplementary Table 1 and Supplementary Figure 1) to mimic the 2000–2004 spatial pattern of the Amazonian deforestation (Eastman, 2015). At each iteration, the MLP attempted to lessen the error of the ANN, making future responses more likely to be correct (Chan et al., 2001). This was done by testing the fitted ANN informed by half of the samples against the other half (i.e., calibration accuracy). Lastly, we used the required number of pixels with the highest computed deforestation risk in each state to spatialize the area of expected forest loss for the 2006–2017 period defined by the National Climate Change Plan's deforestation baseline. The spatialized 2006–2017 baseline scenario was then compared to the observed 2006–2017 deforestation in the region. The map comparison allowed quantification of (1) areas where deforestation was expected but did not occur, (2) areas where deforestation was expected and occurred, and (3) areas where deforestation was not expected but did occur.
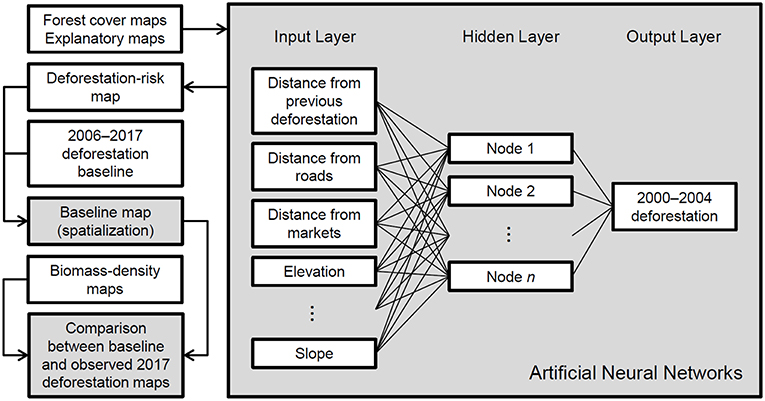
Figure 2. Flowchart of the deforestation baseline spatialization based on artificial neural networks (ANN). Relationships among variables associated with deforestation from the Input Layer (“neurons”) are established in the nodes of the Hidden Layer as an exercise to reproduce the observed pattern of deforestation in the Output Layer. The ANN output is a raster map with ranked likelihoods of deforestation at the pixel level. White boxes represent inputs or outputs, shaded boxes represent processes.
The spatialized, state-level baselines were also used to quantify the CO2 emission reductions in the Brazilian Amazon during the 2006–2017 period by comparing the areas of simulated and observed deforestation to five biomass-density maps available for the region (Baccini et al., 2012, 2017; Soares-Filho et al., 2014; Avitabile et al., 2016; Englund et al., 2017). We used the biomass-density maps to compute stock averages (and standard errors) at the pixel level. Stocks were conservatively estimated based exclusively on the living biomass (i.e., above—plus belowground). Belowground biomass estimates were based on a default root-to-shoot ratio of 0.235 (Mokany et al., 2006). Carbon emissions (in the form of Mg CO2) were based on a standard biomass-carbon fraction of 0.47 (IPCC, 2006) and the molecular weights of carbon dioxide/carbon (i.e., 44/12). Because carbon stocks are not zero after deforestation (e.g., crops and pastures also store some biomass), we adopted a post-deforestation land-use scenario with 28.5 Mg ha−1 of biomass at equilibrium (Fearnside, 1996) and a conservative carbon fraction of 0.5, resulting in a stock of 52.25 Mg CO2 ha−1.
Results
Most state-level models used for the baseline spatialization presented MLP calibration accuracy between 70 and 89% (Supplementary Table 2). These values suggest that the ANNs were successful at explaining the deforestation patterns observed from 2000 to 2004 in the Amazonian states. Accuracies were lower for the states of Maranhão (64%) and Tocantins (61%). The most important explanatory maps driving the accuracy the ANNs were distances from roads in Roraima and Pará, from previously deforested areas in Acre, Amapá, Amazonas, and Mato Grosso, and from sawmills in Maranhão, the ecological-economic zoning in Rondônia, and mining potential in Tocantins. Other important explanatory variables among the state ANN models were the categorical maps of ranked-conservation priority, soil quality, indigenous lands, settlements, and biomes, and the distance-based maps from timber transportation areas, slaughterhouses, urban centers, state capitals, highways, major rivers, and soybean processing plants (Supplementary Figure 1).
Deforestation Reductions
The three-phase deforestation baseline for the Brazilian Amazon assumed 158,572 km2 of forests to be lost from 2006 to 2017, whereas, according to Brazil's official statistics, the observed deforestation for that period was 96,251 km2 (nearly 40% of the baseline). Most of the 62,321 km2 of avoided forest loss (i.e., the difference between the baseline and the observed deforestation) from 2006 to 2017 took place in Mato Grosso state (−40,938 km2), followed by Rondônia (−9,898 km2) and Pará (−9,134 km2). For the same period, deforestation increased in the states of Amazonas (924 km2), Amapá (377 km2), and Roraima (691 km2) in comparison to the baseline. Proportional reductions in deforestation at the state level were also higher in Mato Grosso (−66%), followed by Tocantins (−64%), Rondônia (−44%), Acre (−33%), Maranhão (−19%), and Pará (−18%). Proportional increases in deforestation of 13, 293, and 36% were observed in Amazonas, Amapá, and Roraima, respectively. The latter states failed to meet reduction targets because of the recent deforestation spikes in Amazonas (>1,000 km2 in 2016 and 2017; above the historical 870 km2 average), the 2008 deforestation spike in Roraima (which was more than double the annual historical average), and potentially the challenges of accurately mapping the annual forest cover in Amapá due to constant cloud cover.
Deforestation reduction goals for the Amazon were met in two of the three-phase baseline periods (Figure 1). During the first baseline phase (2006–2009), 46,312 km2 of forest was lost (11,578 km2 year−1 on average), representing a reduction of 41% in comparison to the 1996–2005 historical average, i.e., the reference period for the construction of the baselines (19,625 km2 year−1 on average). The observed deforestation was 1% below the reduction target of 11,775 km2 year−1 on average for the 2006–2009 period. Only 23,880 km2 of the forest was lost during the second phase of the baseline (2010–2013; 5,970 km2 year−1 on average), roughly half of what was observed during the first period, which far surpassed the deforestation reduction target of 8,243 km2 year−1 for the second period. In contrast, during the third phase (2014–2017), 26,059 km2 of forest was lost (6,515 km2 year−1 on average), which still represented a reduction of 21% in comparison to the baseline for that period (8,243 km2 year−1 on average) but did not meet this phase's target of lowering the forest loss to 5,770 km2 year−1 on average.
Carbon Emission Reductions
Carbon-dioxide emissions were directly influenced by the spatial allocation of the deforestation baseline (Figures 3–5). The areas expected to experience forest loss in the baseline scenario (based on 2000–2004 LUCC patterns) presented an average carbon stock equivalent to 313 ± 69.8 Mg CO2 ha−1, whereas the areas that experienced deforestation during the study period averaged 362.5 ± 73.5 Mg CO2 ha−1 (Table 1). Amazon wide, the 62,321 km2 of saved forests from 2006 to 2017 were associated with the avoided emission of 1.5 ± 0.4 Pg CO2 to the atmosphere. The state of Mato Grosso alone contributed to 1.2 ± 0.2 Pg CO2 (74%) of the total emission reductions, followed by Rondônia (0.3 Pg CO2; 19%). At the state level, proportional emission reductions were the highest in Tocantins (−75%), followed by Mato Grosso (−67%), Rondônia (−39%), Acre (−21%), and Maranhão (−19%) when compared to their respective deforestation baselines. In contrast, our simulations suggest that the 50,337 km2 expected to be deforested in the baseline scenario for Pará state had an average carbon stock equivalent to 325.9 ± 78.2 Mg CO2 ha−1 (likely associated with degraded, secondary forest), which is nearly 20% lower than the average stock in the 41,403 km2 that experienced deforestation during 2006–2017 (394.8 ± 79.7 Mg CO2 ha−1; likely influenced by the presence of primary forests from less-accessible locations). As a result, the 9,134 km2 of reduced deforestation in Pará represented only an average proportional reduction of −0.8% in emissions when compared to the baseline. Among the states that increased their annual deforestation during 2006–2017 in comparison to the 1996–2005 reference average, Amapá had the largest proportional increase in emissions (1053%; but remained the lowest in absolute terms), followed by Roraima (69%) and Amazonas (11%).
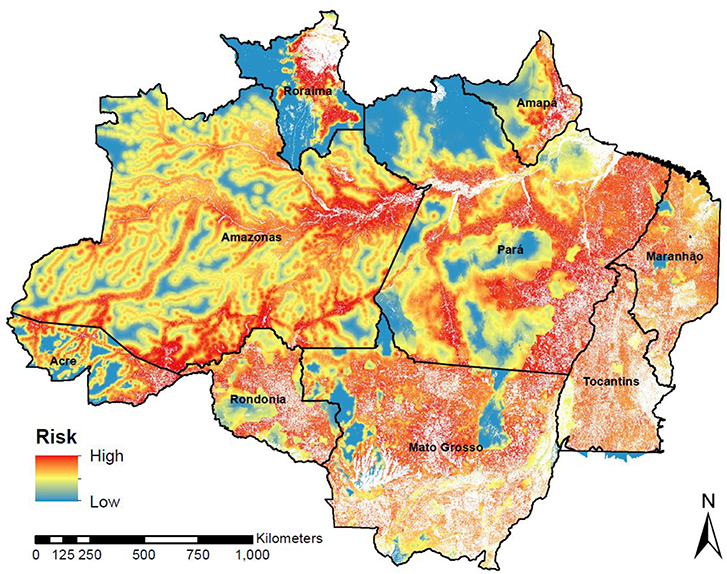
Figure 3. Likelihood of deforestation informed by the 2000–2004 spatial pattern of forest loss observed in the Brazilian Legal Amazon region.
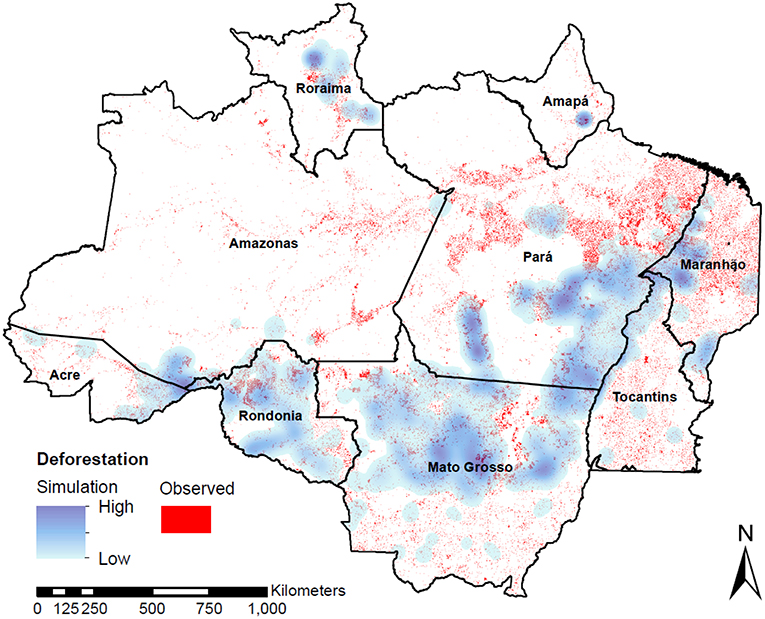
Figure 4. Comparison between the spatialized 2006–2017 deforestation baseline (simulation) and the observed 2006–2017 deforestation (red patches). Simulated baseline displayed as deforestation hotspots (Kernel density) for improved visualization.
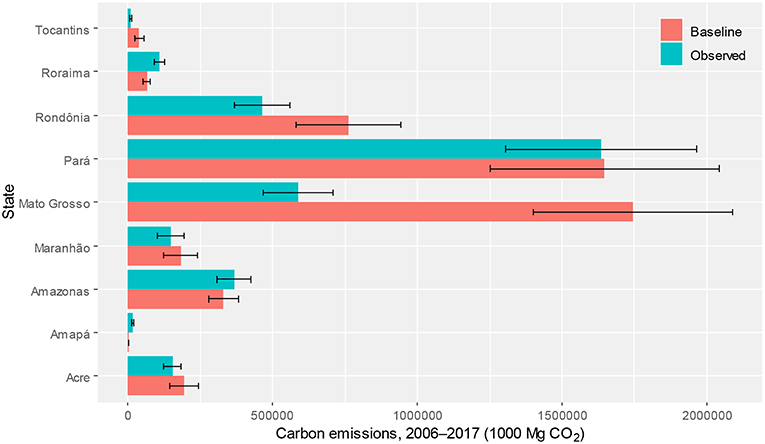
Figure 5. Cumulative baseline and observed carbon emissions from deforestation for the 2006–2017 period in the Amazonian states of Brazil. Error bars represent standard errors computed at the pixel level of the biomass-density maps.
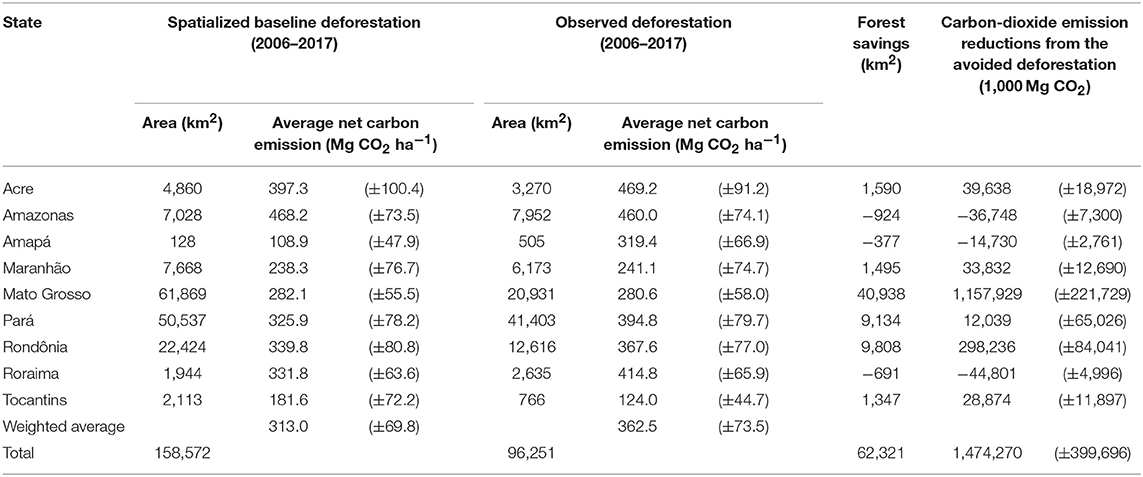
Table 1. Carbon-dioxide emission reductions based on the original deforestation baseline set by Brazil's National Climate Change Plan from 2006 to 2017 (standard errors reported in parenthesis).
Despite the overall reduction in deforestation at the Amazon level, as well as in six of the nine Amazonian states, our results suggest that only three of them (Mato Grosso, Rondônia, and Tocantins) achieved significant carbon emission reductions when compared to their respective baselines during the study period (Figure 5). In contrast, Amapá and Roraima presented significant increases in carbon emissions compared to their respective deforestation baselines. We note that these findings are subject to considerable uncertainty in biomass estimates at the pixel level, inherent to the biomass-density maps employed in our analysis (captured by the large standard-error bars in Figure 5).
Discussion
Biomass and Carbon-Stock Uncertainties
The spatial allocation of deforestation is a critical component of estimating GHG emissions based on biomass-density maps. In our case, standard model validation methods (i.e., comparison between simulated and observed maps; Visser and De Nijs, 2006) do not apply because our goal was to simulate a spatially-explicit counterfactual scenario for what could have happened without the policy-induced shifts in Amazonian deforestation patterns (Arima et al., 2011; Rosa et al., 2012; Godar et al., 2014; Kalamandeen et al., 2018). To assess the validity of our simulation, we instead rely on calibration accuracy assessments. Calibration accuracies of the ANN models were mostly high (61–89%; Supplementary Table 2) and comparable to others reported in the literature (85% in Sangermano et al., 2012; 74–85% Thies et al., 2014; 61% in Riccioli et al., 2016; and 71% in West et al., 2018a).
Another caveat relates to the accuracy of the biomass-density maps. While the maps used here are arguably among the state-of-the-art datasets available in the literature (Baccini et al., 2012, 2017; Soares-Filho et al., 2014; Avitabile et al., 2016; Englund et al., 2017), biomass estimates are inherently subject to large (and often unreported) uncertainty at the pixel level (Mitchard et al., 2014; Ometto et al., 2014; Fearnside, 2018). Limited ground measurements, inaccurate plot coordinates, and the use of different allometric models are some examples of uncertainty drivers behind biomass-density maps with large area coverage and mapping units (Mitchard et al., 2013; Saatchi et al., 2015; Englund et al., 2017). Other constraints that limit accurate estimations of changes in biomass stocks, and consequently carbon emissions, emerge from often incomplete information on liana, dead wood, belowground biomass, hollow stems, and soil carbon (Malhi et al., 2006; Nogueira et al., 2008; Chatterjee et al., 2018), as well as the fate of the harvested timber prior to forest clearing (Lippke et al., 2011). Englund et al. (2017) emphasize that, while biomass-density maps tend to focus on aboveground stocks, it is not always clear what biomass or carbon pools they consider. As a result, Avitabile et al. (2016) noted lower estimates in their dataset for central Amazonia when compared to the map produced by Baccini et al. (2012), which in turn, presented lower estimates than the Baccini et al. (2017) updated dataset. Given the unreported data constraints, we attempted to minimize such limitation by adopting the average stock from five biomass-density maps to estimate the carbon emissions reductions from the avoided deforestation in the Brazilian Amazon.
Carbon stock averages estimated in this study for the areas of observed and simulated deforestation in the Amazon (313 ± 69.8 and 362.5 ± 73.5 Mg CO2 ha−1, respectively) overlap with the previous value adopted by government to quantify the avoided emissions from the loss of “intact” forest in the region (i.e., 366.7 Mg CO2 ha−1; Brazil, 2009). Based on that value, Brazil's gross avoided emissions from deforestation during 2006–2010 were estimated at 2.7 Pg CO2 (Brazil, 2009), or 1.4 Pg CO2 in terms of net avoided emissions based on the assumptions adopted in this study. The per-hectare value was, however, subsequently revised upwards with the inclusion of belowground biomass and the biomass from palms, vines, and litter (resulting in a weighted average of 555.9 Mg CO2 ha−1 across the whole region) for the calculation of the climatic benefits from the avoided deforestation achieved by the country (Brazil, 2014; Fearnside, 2018).
External factors, such as shifts in commodity prices and currency exchange rates, are known to influence deforestation rates in the Amazon (Hargrave and Kis-Katos, 2013; Assunção et al., 2015). During the first years of the National Climate Change Plan's baseline period, both agricultural commodity prices and currency exchange rates were significantly lower than the historical averages (Fearnside, 2017). Considering an average price of USD 5 Mg (World Bank, 2018), and assuming that the reductions in annual deforestation rates were the result of improvements in Brazil's environmental governance rather than external factors, findings from this study suggest that the 2006–2017 carbon emission reductions from LUCC in the Brazilian Amazon, based on the original national baseline, are valued at USD 7.4 ± 2 billion. Alternatively, by assuming that only 56% of the decline in deforestation could be attributed to the government's efforts, as suggested in the literature (Assunção et al., 2015; Cunha et al., 2016), the value of the avoided deforestation would be USD 4.1 ± 1.1 billion. In both cases, these estimates far exceed the current amount of USD 1.2 billion donated to the Amazon Fund to support forest conservation and sustainable development activities in the country (Correa et al., 2019). We note that these values only refer to emissions from deforestation and do not necessarily capture the impacts of forest degradation from logging and associated facilitation of forest fires and biological invasions by lianas, bamboos, or pioneer tree species (e.g., Vidal et al., 2016; Tyukavina et al., 2017; Aragão et al., 2018). The Brazil's control measures have been much more effective against deforestation (clear-cutting) than against the (legal and illegal) degradation from logging (Souza et al., 2013; Brancalion et al., 2018).
Attribution of Deforestation Reductions
This study does not establish causal links between the government's efforts to mitigate deforestation and the estimated reductions in carbon emissions. Nevertheless, progress toward disentangling the effects of conservation policies on forest loss is particularly relevant because of the certificates of emission reductions from deforestation issued by Amazon Fund (Brazil, 2013b). Like in this study, the fund avoided the attribution challenge by assuming all reductions vis-à-vis a baseline to be eligible for “results-based” compensation.
Results-based compensations for avoided deforestation can be controversial and have both advantages and disadvantages (Pana and Gheyssens, 2016; Sills et al., 2017; West et al., 2018b). Results-based accounting gives credit or blame for changes in deforestation rates independent of the causes. This choice is advantageous because causal attribution can be difficult and uncertain, and giving any reward for environmental services is often rendered inviable by requiring the demonstration of “additionality,” i.e., showing that reductions in deforestation would only have occurred because of a mitigation effort (Fearnside, 2000). Results-based assessments from the Amazon Fund adopt a baseline corresponding to a 10-year deforestation average, updated every 5 years (the same baseline as used for the Green Carbon Fund of the UNFCCC). This approach has caused controversy because rewarded reductions in deforestation can be short-lived (i.e., raising the issue of “permanence;” West et al., 2019), and this is aggravated by delays between the reporting of the reductions in question and the granting of financial rewards (e.g., Sax, 2019). For example, Brazil's January 2019 proposal to the Green Climate Fund to receive payment for the 2014–2015 avoided deforestation, covered a period when deforestation was trending upward since Brazil's deforestation slowdown ended in 2012. Still, the justification for payment was based on the difference between the deforestation rate in those years and the average from 1996 to 2010, which is the Forest Reference Emission Level (FREL) for reducing emissions from deforestation in the Amazon for REDD+ results-based payments that Brazil submitted to the UNFCCC in November 2014 (Brazil, 2014).
It is important that Brazil be conservative in its claims of avoided deforestation. The temptation must be avoided to allege that all the decline in deforestation after 2004 was due to governance efforts such as the PPCDAm. Such a claim puts at risk the entire effort to transform the environmental services of Amazonian forests into a viable basis for maintaining both the forests and the traditional human population that inhabits them (Fearnside, 1997, 2008).
Conclusions
In this study, we estimated the climatic benefits in terms of carbon emission reductions from the avoided Amazonian deforestation during 2006–2017 based the spatialization of the deforestation baseline set by Brazil's National Climate Change Plan (Brazil, 2008). Results from state-level simulations suggest that the accumulated 62,321 km2 difference between the baseline scenario and the observed Amazonian deforestation was associated with the avoided emission of 1.5 ± 0.4 Pg CO2 to the atmosphere. Most of the total emission reductions (74%) occurred in Mato Grosso, the state with the highest historical rates of LUCC in the region. Overall, the decline in deforestation rates from 2004 to 2012 resulted in a substantial reduction in emissions, and the net result was still highly positive by 2017 despite the upward trend in deforestation after 2012. Since 2018, Brazil's new presidency has continuously proposed to weaken forest conservation regulations and related institutions (Abessa et al., 2019). Such plans risk a reversal toward Brazil's inglorious past as the world leader in deforestation and the loss of the carbon emission reductions achieved so far.
Data Availability
Publicly available datasets were analyzed in this study. These data can be found here: https://earthenginepartners.appspot.com/, http://dados.gov.br/, http://www.imazongeo.org.br/, http://mapas.mma.gov.br/, http://earthexplorer.usgs.gov/.
Author Contributions
TW designed the study, processed the data, performed the simulations, and wrote the first draft of the article. JB and PF contributed to the writing, editing, and revisions.
Conflict of Interest Statement
The authors declare that the research was conducted in the absence of any commercial or financial relationships that could be construed as a potential conflict of interest.
Supplementary Material
The Supplementary Material for this article can be found online at: https://www.frontiersin.org/articles/10.3389/ffgc.2019.00052/full#supplementary-material
References
Abessa, D., Famá, A., and Buruaem, L. (2019). The systematic dismantling of Brazilian environmental laws risks losses on all fronts. Nat. Ecol. Evol. 3, 510–511. doi: 10.1038/s41559-019-0855-9
Alix-Garcia, J., and Gibbs, H. K. (2017). Forest conservation effects of Brazil's zero deforestation cattle agreements undermined by leakage. Glob. Environ. Chang. 47, 201–217. doi: 10.1016/j.gloenvcha.2017.08.009
Aragão, L. E. O. C., Anderson, L. O., Fonseca, M. G., Rosan, T. M., Vedovato, L. B., Wagner, F. H., et al. (2018). 21st Century drought-related fires counteract the decline of Amazon deforestation carbon emissions. Nat. Commun. 9:536. doi: 10.1038/s41467-017-02771-y
Arima, E. Y., Barreto, P., Araújo, E., and Soares-Filho, B. (2014). Public policies can reduce tropical deforestation: lessons and challenges from Brazil. Land Use Policy 41, 465–473. doi: 10.1016/j.landusepol.2014.06.026
Arima, E. Y., Richards, P., Walker, R., and Caldas, M. M. (2011). Statistical confirmation of indirect land use change in the Brazilian Amazon. Environ. Res. Lett. 6:024010. doi: 10.1088/1748-9326/6/2/024010
Assunção, J., Gandour, C., and Rocha, R. (2015). Deforestation slowdown in the Brazilian Amazon: prices or policies? Environ. Dev. Econ. 20, 697–722. doi: 10.1017/S1355770X15000078
Avitabile, V., Herold, M., Heuvelink, G. B. M., Lewis, S. L., Phillips, O. L., Asner, G. P., et al. (2016). An integrated pan-tropical biomass map using multiple reference datasets. Glob. Chang. Biol. 22, 1406–1420. doi: 10.1111/gcb.13139
Azevedo, A. A., Rajão, R., Costa, M. A., Stabile, M. C. C., Macedo, M. N., dos Reis, T. N. P., et al. (2017). Limits of Brazil's Forest Code as a means to end illegal deforestation. Proc. Natl. Acad. Sci. U.S.A. 114, 7653–7658. doi: 10.1073/pnas.1604768114
Baccini, A., Goetz, S. J., Walker, W. S., Laporte, N. T., Sun, M., Sulla-Menashe, D., et al. (2012). Estimated carbon dioxide emissions from tropical deforestation improved by carbon-density maps. Nat. Clim. Chang. 2, 182–185. doi: 10.1038/nclimate1354
Baccini, A., Walker, W., Carvalho, L., Farina, M., Sulla-Menashe, D., and Houghton, R. A. (2017). Tropical forests are a net carbon source based on aboveground measurements of gain and loss. Science 358, 230–234. doi: 10.1126/science.aam5962
Börner, J., Kis-Katos, K., Hargrave, J., and König, K. (2015). Post-crackdown effectiveness of field-based forest law enforcement in the Brazilian Amazon. PLoS ONE 10:e0121544. doi: 10.1371/journal.pone.0121544
Brancalion, P. H. S., de Almeida, D. R. A., Vidal, E., Molin, P. G., Sontag, V. E., Souza, S. E. X. F., et al. (2018). Fake legal logging in the Brazilian Amazon. Sci. Adv. 4:eaat1192. doi: 10.1126/sciadv.aat1192
Brazil (2013b). Plano de Ação para Prevenção e Controle do Desmatamento na Amazônia Legal (PPCDAm): 3a fase (2012-2015) pelo Uso Sustentável e Conservação da Floresta. Brasília.
Brazil (2014). Brazil's Submission of a Forest Reference Emission Level for Deforestation in the Amazonia Biome for Results-Based Payments for REDD+ Under the UNFCCC. Brasília.
Chan, J. C. W., Chan, J. C. W., Chan, K. P., Chan, K. P., Yeha, G. O., and Yeha, G. O. (2001). Detecting the nature of change in an urban environment: a comparison of machine learning algorithms. Photogramm. Eng. Remote Sensing 67, 213–225.
Chatterjee, N., Nair, P. K. R., Chakraborty, S., and Nair, V. D. (2018). Changes in soil carbon stocks across the Forest-Agroforest-Agriculture/Pasture continuum in various agroecological regions: a meta-analysis. Agric. Ecosyst. Environ. 266, 55–67. doi: 10.1016/j.agee.2018.07.014
Cisneros, E., Zhou, S. L., and Börner, J. (2015). Naming and shaming for conservation: evidence from the Brazilian Amazon. PLoS ONE 10:e0136402. doi: 10.1371/journal.pone.0136402
Correa, J., van der Hoff, R., and Rajão, R. (2019). Amazon Fund 10 years later: lessons from the world's largest REDD+ program. Forests 10:272. doi: 10.3390/f10030272
Cunha, F. A. F., de, S., Börner, J., Wunder, S., Cosenza, C. A. N., and Lucena, A. F. P. (2016). The implementation costs of forest conservation policies in Brazil. Ecol. Econ. 130, 209–220. doi: 10.1016/j.ecolecon.2016.07.007
Duchelle, A. E., Cromberg, M., Gebara, M. F., Guerra, R., Melo, T., Larson, A., et al. (2014). Linking forest tenure reform, environmental compliance, and incentives: lessons from redd+ initiatives in the brazilian amazon. World Dev. 55, 53–67. doi: 10.1016/j.worlddev.2013.01.014
Englund, O., Sparovek, G., Berndes, G., Freitas, F., Ometto, J. P., Oliveira, P. V. D. C. E., et al. (2017). A new high-resolution nationwide aboveground carbon map for Brazil. Geo Geogr. Environ. 4:e00045. doi: 10.1002/geo2.45
Escobar, H. (2018). Scientists, environmentalists brace for Brazil's right turn. Science. 362, 273–274. doi: 10.1126/science.362.6412.273
Exbrayat, J.-F., Liu, Y. Y., and Williams, M. (2017). Impact of deforestation and climate on the Amazon Basin's above-ground biomass during 1993–2012. Sci. Rep. 7:15615. doi: 10.1038/s41598-017-15788-6
Fearnside, P. M. (1996). Amazonian deforestation and global warming: carbon stocks in vegetation replacing Brazil's Amazon forest. For. Ecol. Manage. 80, 21–34. doi: 10.1016/0378-1127(95)03647-4
Fearnside, P. M. (1997). Environmental services as a strategy for sustainable development in rural Amazonia. Ecol. Econ. 20, 53–70. doi: 10.1016/S0921-8009(96)00066-3
Fearnside, P. M. (2000). Uncertainty in land-use change and forestry sector mitigation options for global warming: plantation silviculture versus avoided deforestation. Biomass Bioenergy 18, 457–468. doi: 10.1016/S0961-9534(00)00003-9
Fearnside, P. M. (2008). Amazon forest maintenance as a source of environmental services. An. Acad. Bras. Cienc. 80, 101–114. doi: 10.1590/S0001-37652008000100006
Fearnside, P. M. (2012). Brazil's Amazon forest in mitigating global warming: unresolved controversies. Clim. Policy 12, 70–81. doi: 10.1080/14693062.2011.581571
Fearnside, P. M. (2017). Deforestation of the Brazilian Amazon. Oxford Res. Encycl. Environ. Sci. 58, 1–52. doi: 10.1093/acrefore/9780199389414.013.102
Fearnside, P. M. (2018). Brazil's Amazonian forest carbon: the key to Southern Amazonia's significance for global climate. Reg. Environ. Chang. 18, 47–61. doi: 10.1007/s10113-016-1007-2
Finer, M., Novoa, S., Weisse, M. J., Petersen, R., Mascaro, J., Souto, T., et al. (2018). Combating deforestation: from satellite to intervention. Science. 360, 1303–5. doi: 10.1126/science.aat1203
Gibbs, H. K., Munger, J., L'Roe, J., Barreto, P., Pereira, R., Christie, M., et al. (2016). Did ranchers and slaughterhouses respond to zero-deforestation agreements in the Brazilian Amazon? Conserv. Lett. 9, 32–42. doi: 10.1111/conl.12175
Gibbs, H. K., Rausch, L., Munger, J., Schelly, I., Morton, D. C., Noojipady, P., et al. (2015). Brazil's soy moratorium. Science 347, 377–378. doi: 10.1126/science.aaa0181
Godar, J., Gardner, T. A., Tizado, E. J., and Pacheco, P. (2014). Actor-specific contributions to the deforestation slowdown in the Brazilian Amazon. Proc. Natl. Acad. Sci. U.S.A. 111, 15591–15596. doi: 10.1073/pnas.1322825111
Gullison, R. E., Frumhoff, P. C., Canadell, J. G., Field, C. B., Nepstad, D. C., Hayhoe, K., et al. (2007). Tropical forests and climate policy. Science 316, 1136163–1136986. doi: 10.1126/science.1136163
Hansen, M. C., Potapov, P. V., Moore, R., Hancher, M., Turubanova, S. A., Tyukavina, A., et al. (2013). High-resolution global maps of 21st-century forest cover change. Science 342, 850–853. doi: 10.1126/science.1244693
Hargrave, J., and Kis-Katos, K. (2013). Economic causes of deforestation in the Brazilian Amazon: a panel data analysis for the 2000s. Environ. Resour. Econ. 54, 471–494. doi: 10.1007/s10640-012-9610-2
IPCC (2006). “Forest land,” in 2006 IPCC Guidelines for National Greenhouse Gas Inventories, Vol 4, Agriculture, Forestry and Other Land Use, eds H. S. Eggleston, L. Buendia, K. Miwa, T. Ngara, and K. Tanabe (Hayama: Institute for Global Environmental Strategies (IGES.)), 4.1–4.83.
IPEA-GIZ-CEPAL (2011). Avaliação do plano de ação para prevenção e controle do desmatamento na Amazônia Legal: PPCDAm 2007-2010. Brasília.
Kalamandeen, M., Gloor, E., Mitchard, E., Quincey, D., Ziv, G., Spracklen, D., et al. (2018). Pervasive rise of small-scale deforestation in Amazonia. Sci. Rep. 8:1600. doi: 10.1038/s41598-018-19358-2
Khanna, J., Medvigy, D., Fueglistaler, S., and Walko, R. (2017). Regional dry-season climate changes due to three decades of Amazonian deforestation. Nat. Clim. Chang. 7, 200–204. doi: 10.1038/nclimate3226
Klingler, M., Richards, P. D., and Ossner, R. (2018). Cattle vaccination records question the impact of recent zero-deforestation agreements in the Amazon. Reg. Environ. Chang. 18, 33–46. doi: 10.1007/s10113-017-1234-1
Lippke, B., Oneil, E., Harrison, R., Skog, K., Gustavsson, L., and Sathre, R. (2011). Life cycle impacts of forest management and wood utilization on carbon mitigation: knowns and unknowns. Carbon Manag. 2, 303–333. doi: 10.4155/cmt.11.24
Malhi, Y., Wood, D., Baker, T. R., Wright, J., Phillips, O. L., Cochrane, T., et al. (2006). The regional variation of aboveground live biomass in old-growth Amazonian forests. Glob. Chang. Biol. 12, 1107–1138. doi: 10.1111/j.1365-2486.2006.01120.x
Mitchard, E. T. A., Feldpausch, T. R., Brienen, R. J. W., Lopez-Gonzalez, G., Monteagudo, A., Baker, T. R., et al. (2014). Markedly divergent estimates of Amazon forest carbon density from ground plots and satellites. Glob. Ecol. Biogeogr. 23, 935–946. doi: 10.1111/geb.12168
Mitchard, E. T. A., Saatchi, S. S., Baccini, A., Asner, G. P., Goetz, S. J., Harris, N. L., et al. (2013). Uncertainty in the spatial distribution of tropical forest biomass: a comparison of pan-tropical maps. Carbon Balance Manag. 8, 1–13. doi: 10.1186/1750-0680-8-10
Mokany, K., Raison, R. J., and Prokushkin, A. S. (2006). Critical analysis of root: shoot ratios in terrestrial biomes. Glob. Chang. Biol. 12, 84–96. doi: 10.1111/j.1365-2486.2005.001043.x
Nobre, C. A., Sampaio, G., Borma, L. S., Castilla-Rubio, J. C., Silva, J. S., and Cardoso, M. (2016). Land-use and climate change risks in the Amazon and the need of a novel sustainable development paradigm. Proc. Natl. Acad. Sci. U.S.A. 113, 10759–10768. doi: 10.1073/pnas.1605516113
Nogueira, E. M., Fearnside, P. M., Nelson, B. W., Barbosa, R. I., and Keizer, E. W. H. (2008). Estimates of forest biomass in the Brazilian Amazon: new allometric equations and adjustments to biomass from wood-volume inventories. For. Ecol. Manage. 256, 1853–1867. doi: 10.1016/j.foreco.2008.07.022
Ometto, J. P., Aguiar, A. P., Assis, T., Soler, L., Valle, P., Tejada, G., et al. (2014). Amazon forest biomass density maps: tackling the uncertainty in carbon emission estimates. Clim. Change 124, 545–560. doi: 10.1007/s10584-014-1058-7
Pana, A. C., and Gheyssens, J. (2016). Baseline choice and performance implications for REDD. J. Environ. Econ. Policy 5, 79–124. doi: 10.1080/21606544.2015.1028465
Riccioli, F., El Asmar, T., El Asmar, J.-P., Fagarazzi, C., and Casini, L. (2016). Artificial neural network for multifunctional areas. Environ. Monit. Assess. 188:67. doi: 10.1007/s10661-015-5072-7
Rochedo, P. R. R., Soares-Filho, B., Schaeffer, R., Viola, E., Szklo, A., Lucena, A. F. P., et al. (2018). The threat of political bargaining to climate mitigation in Brazil. Nat. Clim. Chang. 8, 695–698. doi: 10.1038/s41558-018-0213-y
Rosa, I. M. D., Souza, C., and Ewers, R. M. (2012). Changes in size of deforested patches in the Brazilian Amazon. Conserv. Biol. 26, 932–937. doi: 10.1111/j.1523-1739.2012.01901.x
Saatchi, S., Mascaro, J., Xu, L., Keller, M., Yang, Y., Duffy, P., et al. (2015). Seeing the forest beyond the trees. Glob. Ecol. Biogeogr. 24, 606–610. doi: 10.1111/geb.12256
Sangermano, F., Eastman, J. R., and Zhu, H. (2010). Similarity weighted instance-based learning for the generation of transition potentials in land use change modeling. Trans. GIS 14, 569–580. doi: 10.1111/j.1467-9671.2010.01226.x
Sangermano, F., Toledano, J., and Eastman, R. (2012). Land cover change in the Bolivian Amazon and its implications for REDD+ and endemic biodiversity. Landsc. Ecol. 27, 571–584. doi: 10.1007/s10980-012-9710-y
Sax, S. (2019). Fears of a Dire Precedent as Brazil Seeks Results-Based REDD+ Payment. Mongabay Series Global Forest. Available online at: https://news.mongabay.com/2019/02/fears-of-a-dire-precedent-as-brazil-seeks-results-based-redd-payment/ (accessed April 23, 2019).
Sills, E. O., de Sassi, C., Jagger, P., Lawlor, K., Miteva, D. A., Pattanayak, S. K., et al. (2017). Building the evidence base for REDD+: study design and methods for evaluating the impacts of conservation interventions on local well-being. Glob. Environ. Chang. 43, 148–160. doi: 10.1016/j.gloenvcha.2017.02.002
Soares-Filho, B., Rajao, R., Macedo, M., Carneiro, A., Costa, W., Coe, M., et al. (2014). Cracking Brazil's forest code. Science 344, 363–364. doi: 10.1126/science.1246663
Souza, C. M., Siqueira, J. V., Sales, M. H., Fonseca, A. V., Ribeiro, J. G., Numata, I., et al. (2013). Ten-year landsat classification of deforestation and forest degradation in the Brazilian Amazon. Remote Sens. 5, 5493–5513. doi: 10.3390/rs5115493
Svahn, J., and Brunner, D. (2018). Did the Soy Moratorium reduce deforestation in the Brazilian Amazon? A counterfactual analysis of the impact of the Soy Moratorium on deforestation in the Amazon biome (Master Thesis). Norwegian School of Economics. Bergen, Norway.
Thies, B., Meyer, H., Nauss, T., and Bendix, J. (2014). Projecting land-use and land-cover changes in a tropical mountain forest of Southern Ecuador. J. Land Use Sci. 9, 1–33. doi: 10.1080/1747423X.2012.718378
Thompson, M. C., Baruah, M., and Carr, E. R. (2011). Seeing REDD+ as a project of environmental governance. Environ. Sci. Policy 14, 100–110. doi: 10.1016/j.envsci.2010.11.006
Tollefson, J. (2018). Brazil's lawmakers renew push to weaken environmental rules. Nature 557, 17–17. doi: 10.1038/d41586-018-05022-2
Tyukavina, A., Hansen, M. C., Potapov, P. V., Stehman, S. V., Smith-Rodriguez, K., Okpa, C., et al. (2017). Types and rates of forest disturbance in Brazilian Legal Amazon, 2000–2013. Sci. Adv. 3:e1601047. doi: 10.1126/sciadv.1601047
UN-REDD (2015). UN-REDD Programme Strategic Framework 2016-20. Washington, DC: The United Nations Collaborative Programme on Reducing Emissions from Deforestation and Forest Degradation in Developing Countries.
Vidal, E., West, T. A. P., and Putz, F. E. (2016). Recovery of biomass and merchantable timber volumes twenty years after conventional and reduced-impact logging in Amazonian Brazil. For. Ecol. Manage. 376, 1–8. doi: 10.1016/j.foreco.2016.06.003
Visser, H., and De Nijs, T. (2006). The map comparison kit. Environ. Model. Softw. 21, 346–358. doi: 10.1016/j.envsoft.2004.11.013
West, T. A. P. (2016). Indigenous community benefits from a de-centralized approach to REDD+ in Brazil. Clim. Policy 16, 924–939. doi: 10.1080/14693062.2015.1058238
West, T. A. P., Grogan, K. A., Swisher, M. E., Caviglia-Harris, J. L., Sills, E., Harris, D., et al. (2018a). A hybrid optimization-agent-based model of REDD+ payments to households on an old deforestation frontier in the Brazilian Amazon. Environ. Model. Softw. 100, 159–174. doi: 10.1016/j.envsoft.2017.11.007
West, T. A. P., Grogan, K. A., Swisher, M. E., Caviglia-Harris, J. L., Sills, E. O., Roberts, D. A., et al. (2018b). Impacts of REDD+ payments on a coupled human-natural system in Amazonia. Ecosyst. Serv. 33, 68–76. doi: 10.1016/j.ecoser.2018.08.008
West, T. A. P., Wilson, C., Vrachioli, M., and Grogan, K. A. (2019). Carbon payments for extended rotations in forest plantations: conflicting insights from a theoretical model. Ecol. Econ. 163, 70–76. doi: 10.1016/j.ecolecon.2019.05.010
Keywords: REDD+, greenhouse gas emission, land-use/cover change, carbon offset, artificial neural network
Citation: West TAP, Börner J and Fearnside PM (2019) Climatic Benefits From the 2006–2017 Avoided Deforestation in Amazonian Brazil. Front. For. Glob. Change 2:52. doi: 10.3389/ffgc.2019.00052
Received: 14 May 2019; Accepted: 21 August 2019;
Published: 18 September 2019.
Edited by:
David Burslem, University of Aberdeen, United KingdomReviewed by:
Giuliano Maselli Locosselli, University of São Paulo, BrazilAdriane Esquivel-Muelbert, University of Birmingham, United Kingdom
Copyright © 2019 West, Börner and Fearnside. This is an open-access article distributed under the terms of the Creative Commons Attribution License (CC BY). The use, distribution or reproduction in other forums is permitted, provided the original author(s) and the copyright owner(s) are credited and that the original publication in this journal is cited, in accordance with accepted academic practice. No use, distribution or reproduction is permitted which does not comply with these terms.
*Correspondence: Thales A. P. West, thales.west@scionresearch.com