A comparative study of 17 phenological models to predict the start of the growing season
- 1College of Water Sciences, Beijing Normal University, Beijing, China
- 2Jiangsu Provincial Key Laboratory of Geographic Information Science and Technology, International Institute for Earth System Science, Nanjing University, Nanjing, China
Vegetation phenological models play a major role in terrestrial ecosystem modeling. However, substantial uncertainties still occur in phenology models because the mechanisms underlying spring phenological events are unclear. Taking into account the asymmetric effects of daytime and nighttime temperature on spring phenology, we analyzed the performance of 17 spring phenological models by combining the effects of photoperiod and precipitation. The global inventory modeling and mapping study third-generation normalized difference vegetation index data (1982–2014) were used to extract the start of the growing season (SOS) in the North–South Transect of Northeast Asia. The satellite-derived SOS of deciduous needleleaf forest (DNF), mixed forest (MF), open shrublands (OSL), and woody savannas (WS) showed high correlation coefficients (r) with the model-predicted SOS, with most exceeding 0.7. For all vegetation types studied, the models that considered the effect of photoperiod and precipitation did not significantly improve the model performance. For temperature-based models, the model using the growing-degree-day temperature response had a lower root mean square error compared with the models using the sigmoid temperature response Importantly, we found that daily maximum temperature was most suitable for the spring phenology prediction of DNF, OSL, and WS; daily mean temperature for MF; and daily minimum temperature for grasslands. These findings indicate that future spring phenological models should consider the asymmetric effect between daytime and nighttime temperature across different vegetation types.
1. Introduction
Phenology is the study of repetitive life-cycle events, such as plant leaf expansion, flowering, and animal migration (Morisette et al., 2009). Vegetation phenology is the study of the relationship between climate and specific biological events, such as germination, flowering, and defoliation (de Beurs and Henebry, 2008). Phenological events have a strong control over the seasonal exchange of material and energy between the land surface and the atmosphere (Chuine et al., 2000). Extensive vegetation phenological changes (Julien and Sobrino, 2009; Brown et al., 2012; Oberbauer et al., 2013; Park et al., 2015; Liu et al., 2016; Zhou et al., 2020) caused by climate change (Anderegg and Diffenbaugh, 2016) may threaten the function of ecosystems (Thackeray et al., 2016). They can also have widespread consequences for agriculture, forestry, human health, and the global economy (Peñuelas and Filella, 2001).
Appropriate plant phenological timing can protect plants from abiotic stress, which is essential for plant growth (Basler, 2016; Delpierre et al., 2016). The dispersion of plant phenological timing allows different species in plant communities to coexist, and it also has an important impact on ecosystem processes such as nutrient acquisition (Cleland et al., 2007). Therefore, vegetation phenology plays an important role in species distribution models (Chuine and Beaubien, 2001) and dynamic global vegetation models (Krinner et al., 2005; Peano et al., 2019). Phenological models that simulate the start of the growing season (SOS) can effectively predict the response of vegetation to changes in the climate and energy balance (Chuine et al., 2000). The phenological processes in models that couple land surfaces to the climate system should be accurately represented, particularly when these models are used to predict future climates (Richardson et al., 2013). Therefore, accurate simulation of the phenological changes in coupled biosphere/atmosphere climate models, terrestrial biogeochemical cycling models, and vegetation dynamics models is important (Botta et al., 2000; Yun et al., 2017; Gauzere et al., 2019; Peano et al., 2021).
Bud dormancy consists of three phases, namely paradormancy, endodormancy, and ecodormancy (Lang et al., 1987). Plants need to accumulate chilling during the endodormancy phase and accumulate heat (forcing temperature) during the ecodormancy phase. Models that involve only the ecodormancy phase are called one-phase models, while models that involve both the endodormancy and ecodormancy phases are called two-phase models (Fu et al., 2020). The oldest one-phase model dates back to 1735 (Reaumur, 1735). That model considered the need for plants to accumulate heat in spring, and could be called the thermal time model (Basler, 2016). Since then, researchers have developed many thermal time models (Wang, 1960; Cannell and Smith, 1983; Hunter and Lechowicz, 1992; Chuine et al., 1999) by using the growing-degree-day as a unit of heat accumulation. The temperature response of the thermal time model is a linear function. Some studies have used the sigmoid function as a substitute to develop the sigmoid temperature response model (Hänninen, 1990; Kramer, 1994). Because the photoperiod affects heat accumulation, Masle et al. developed a photothermal-time model for crops (Masle et al., 1989). According to the relationship between chilling accumulation and heat accumulation, the two-phase models are divided into the sequential model, parallel model, alternating model, and unified model (Cannell and Smith, 1983; Murray et al., 1989; Hänninen, 1990; Kramer, 1994; Chuine, 2000). In addition, dormancy induction processes have been introduced into spring phenology models. The most complex of these is the DormPhot model (Caffarra et al., 2011), because it considers dormancy induction, chilling, forcing, and photoperiod, simultaneously. Unlike models based on dormant phases, promoter–inhibitor models do not distinguish between dormant phases and treat the release of dormancy as the result of the combined control of promoters and inhibitors, where promoters and inhibitors include hormones or enzymes that determine the physiological developmental state of the plant and its response to external drivers (Schaber and Badeck, 2003). Most spring phenology models do not include the effects of precipitation, although models that include precipitation have been developed for grasslands (e.g., grassland pollen model) (García-Mozo et al., 2009). Although models have been developed to simulate spring phenology for specific species, they do not include recent new insights into spring phenology, such as the asymmetric effects of temperature. In addition to average temperatures, previous studies have found that daytime and nighttime temperatures also affect vegetation greening (Shen et al., 2018; Meng et al., 2020). It is thus important to consider the effect of asymmetric warming on vegetation greening in spring phenological models.
High-latitude areas warm faster than low-latitude areas, and phenological changes may be more pronounced than those in other parts of the Earth (Parmesan, 2007; Pau et al., 2011), making them ideal to study phenological responses to climate change (Prevéy et al., 2017). Vegetation phenology varies with species and is influenced by environmental factors, such as air temperature, precipitation, soil temperature, soil moisture, and photoperiod (Fu et al., 2019; Meng et al., 2021), which depend on location and time (de Beurs and Henebry, 2008). Experimental studies (Henry and Molau, 1997; Price and Waser, 1998; Menzel and Fabian, 1999; Botta et al., 2000; Fu et al., 2015; Malyshev, 2020) have shown that air temperature is the main driver of phenological changes in northern temperate and high-latitude regions. In addition, precipitation has an impact on the spring phenology of grasslands (Shen et al., 2018; Castillioni et al., 2022). In the temperate grasslands of China, the effects of daytime and nighttime temperatures on spring phenology are asymmetric, with the monthly average maximum temperature having a greater effect in winter and the monthly average minimum temperature causing a greater effect in spring (Shen et al., 2018). On the Qinghai–Tibet Plateau, the daily minimum temperature has a stronger influence on the phenology during spring and summer (Shen et al., 2016). Previous studies have shown significant differences in the effects of daytime and nighttime temperatures on the leaf unfolding of European deciduous trees (Fu et al., 2016; Wang et al., 2021). In addition, daytime and nighttime warming had different or even opposite effects on spring phenology in the Appalachian Trail region of the eastern United States (Meng et al., 2020). Therefore, the characteristics of temperature effects on phenology should be considered in phenological models (Fu et al., 2016; Meng et al., 2020).
In this study, we used the global inventory modeling and mapping study (GIMMS) third-generation normalized difference vegetation index (NDVI3g) from 1982 to 2014 to extract the SOS in the North–South Transect of Northeast Asia (NSTNEA) region and then combined the ERA-Interim temperature data to test the asymmetric effect of temperature on 17 spring phenological models. The objectives of this study were as follows: (1) to analyze the influence of model structure and temperature response function on spring phenology prediction; (2) to find the optimal spring phenological model for each vegetation type; and (3) to test the effects of daily maximum temperature (Tmax), daily mean temperature (Tmean), and daily minimum temperature (Tmin) on the prediction performance of the spring phenological models.
2. Materials and methods
2.1. Study area and land cover datasets
To reduce the spatial heterogeneity of phenology (Suzuki et al., 2003), we chose the NSTNEA region as the study area because it covers a wide latitude range and a narrow longitude range. Transects are used to reflect the effects of major environmental factors on the structure, function, composition, and water cycle of terrestrial ecosystems. They comprise a series of research sites distributed over a large geographical area and are a good reference for studying terrestrial ecosystems with a large spatial extent, even at a global scale. The NSTNEA covers regions with coordinates ranging from 32 to 78°N and from 105 to 118°E, spanning China, Mongolia, and Russia. The center of the NSTNEA is located at Lake Baikal in Southern Siberia, Russia, and the south and north are bounded by the northern bank of the Yellow River in China and the shore of the Arctic Ocean, respectively (Wang et al., 2014).
The response of spring phenology to climate change varies by vegetation type and separate phenology models need to be constructed for each vegetation type. The vegetation type dataset used in this study was MODIS land cover from 2001 to 2012 with a spatial resolution of 5′ (0.083°) after aggregation (Friedl et al., 2010; Channan et al., 2014). To reduce the impact of changes in land cover (Wang et al., 2016), we only analyzed pixels with a stable vegetation coverage (Figure 1A). Considering the impact of human activities, we excluded croplands. Considering the effects of noise and non-vegetation signals, we excluded the “barren or sparsely vegetated” land cover type and selected pixels with NDVI greater than 0.1 for analysis. Finally, the following five vegetation types were selected for the analysis of the spring phenological models: deciduous needleleaf forest (DNF), mixed forest (MF), open shrublands (OSL), woody savannas (WS), and grasslands (GL). To match the temperature data resolution, we determined the main vegetation types at a resolution of 0.125° (Figure 1B). We overlaid the land cover data with the climate data. When the value of all land cover pixels covered by the climate data pixel was unique, we set the value of the corresponding pixel of the new land cover data to that unique value; otherwise, it was set to a null value. Therefore, the values of the mixed pixels at the edge of different land cover were set to null values to reduce the uncertainty in our research.
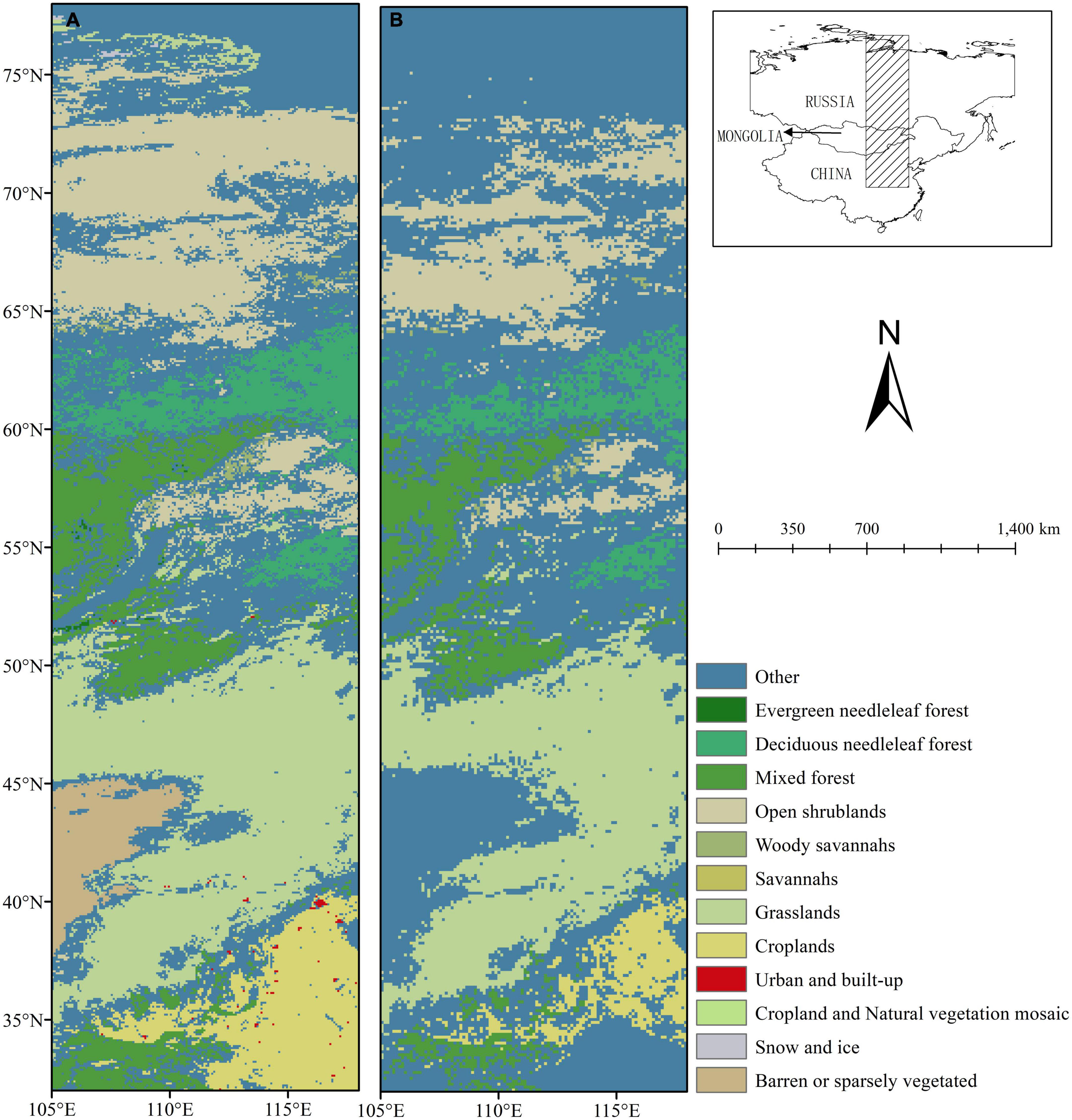
Figure 1. Land cover in the North–South Transect of Northeast Asia (NSTNEA) region: (A) original stable land cover, (B) redefined vegetation types.
2.2. Phenology and climate datasets
We used GIMMS NDVI3g to extract the SOS for the study area because it covered more than 30 years and it included the warming prior to the 1997/98 El Niño event as well as the subsequent warming hiatus (Meehl et al., 2011). Because the NDVI time-series data fluctuated significantly owing to various factors, a noise removal processing was required to reconstruct a smooth NDVI time-series curve. In the current study, this was achieved using the asymmetric gaussian model, double logistic method, and Savitzky–Golay filter (with a Savitzky–Golay filter window of 5). We adopted these methods because they are widely used for the extraction of vegetation phenological metrics (Zhong et al., 2019; Zeng et al., 2020). The NDVI curve fitting functions for the three methods are shown in Supplementary Table 1. The maximum rate of change in the fitted NDVI curve was used to determine the SOS. The flow chart for SOS extraction is shown in Supplementary Figure 1. Details of the methods can be found in previous studies (Jonsson and Eklundh, 2002; Jönsson and Eklundh, 2004; Piao et al., 2006). The present study used the TIMESAT software to construct a smooth NDVI time-series curve.1 A detailed comparison of the three extraction methods has been reported by Mo et al. (2019). We used the mean value of the three methods as the phenological modeling data. Table 1 shows the comprehensive results of the three methods.

Table 1. Statistics of spring phenology of five vegetation types in the study area (1982–2014) on the day of year (DOY): (a) deciduous needleleaf forest (DNF), (b) mixed forest (MF), (c) open shrublands (OSL), (d) woody savannas (WS), and (e) grasslands (GL).
The European Centre for Medium-Range Weather Forecasts is one of the world’s leading reanalysis centers. ERA-Interim is the global atmospheric numerical forecast reanalysis provided by the European Centre for Medium-Range Weather Forecasts to users worldwide. It is a newer product than EAR-40. Its purpose is to connect early products with a new generation of products, thus gradually replacing ERA-40. The ERA-Interim data cover reanalysis dates from 1979 and is updated in real-time (Berrisford et al., 2009, 2011). We used ERA-Interim as climate data because it is widely used and its spatial-temporal resolution met our needs. The ERA-Interim uses 4D-Var assimilation technology to improve the ERA-40 and other data, solving some of the key problems associated with ERA-40 and improving the accuracy of the forecast. ERA-Interim has a horizontal resolution of approximately 79 km and is interpolated globally to a variety of latitude and longitude grids ranging from 0.125 to 2.5° using interpolation techniques. We obtained daily temperature and precipitation data from 1982 to 2014 with a spatial resolution of 0.125°. The dataset contained atmospheric temperature data 2 m above the ground taken at four time intervals (6, 12, 18, and 24 h). The mean temperature at these intervals was taken as the average temperature of the day.
2.3. Spring phenological model
Because the study area is located in the middle and high latitudes, the SOS is primarily controlled by temperature and photoperiod. We selected 17 spring phenological models, including a linear model, 6 one-phase models, and 10 two-phase models for analysis (Table 2). The parameter common to most models was the start date; the differences were the environmental driver used and the response rate to the environmental driver controlled by one or more parameters. The formulas of the models used in this study can be found Supplementary Tables 2, 3.
2.4. Model calibration and evaluation
A part of dataset (1986-2010) was used to train the model and another part (1982-1985, 2011-2014) to evaluate its predictive performance. The PHENOR modeling framework (Hufkens et al., 2018) was used to train the different phenology models, using the generalized simulated annealing (GenSA) parameter optimization algorithm (Xiang et al., 2013). Considering the asymmetric effects of daytime and nighttime temperatures on SOS, we parameterized the model using Tmax, Tmean and Tmin as input data. The performance of the model was evaluated based on the results of Tmean. Model parameterization is an important process affecting the application of the model. As the sample size increases, the parameter optimization process becomes time-consuming. Therefore, the number of iterations was set to 5,000 in this study. Considering the definition of the phenological period and the scale effect, we did not validate the model with in situ spring phenological data.
We used the root mean square error (RMSE) to evaluate the spring phenological model. The RMSE reflects the deviation between the predicted value of the model and the observed value. The calculation formula of RMSE is shown in Equation 1:
Here, Oi is the observed value, Pi is the predicted value, n is the number of observed samples.
The Akaike information criterion (AIC) is often used to evaluate a set of models for a given data (Akaike, 1974). The AIC is based on the concept of information entropy and is used to evaluate the complexity of the model and measure the goodness of the model. For a certain dataset, the lower the AIC value, the better the model. The calculation formula of AIC is shown in Equation 2:
where k is the number of parameters to be fitted in the model.
In summary, we used correlation coefficients, RMSE and AIC to evaluate the predictive performance of the 17 models used in this study, which were calculated from the 8-year observed and predicted SOS.
3. Results
Figure 2 shows the scatter plot of the SOS predicted by the 17 models for DNF, MF, OSL, WS, and GL and the satellite-derived SOS. For DNF, MF, OSL, and WS, the SOS simulated by the models had a good consistency with the satellite-derived SOS, and most points were concentrated near the 1:1 line. For GL, the data points were more scattered. This characteristic was reflected in the correlation coefficient. From the perspective of vegetation types, overall, the satellite-derived SOS of DNF, MF, OSL, and WS had a high correlation with the model-predicted SOS, with most coefficients exceeding 0.7 and some even exceeding 0.8. In contrast, the corresponding correlation coefficient of GL did not exceed 0.5.
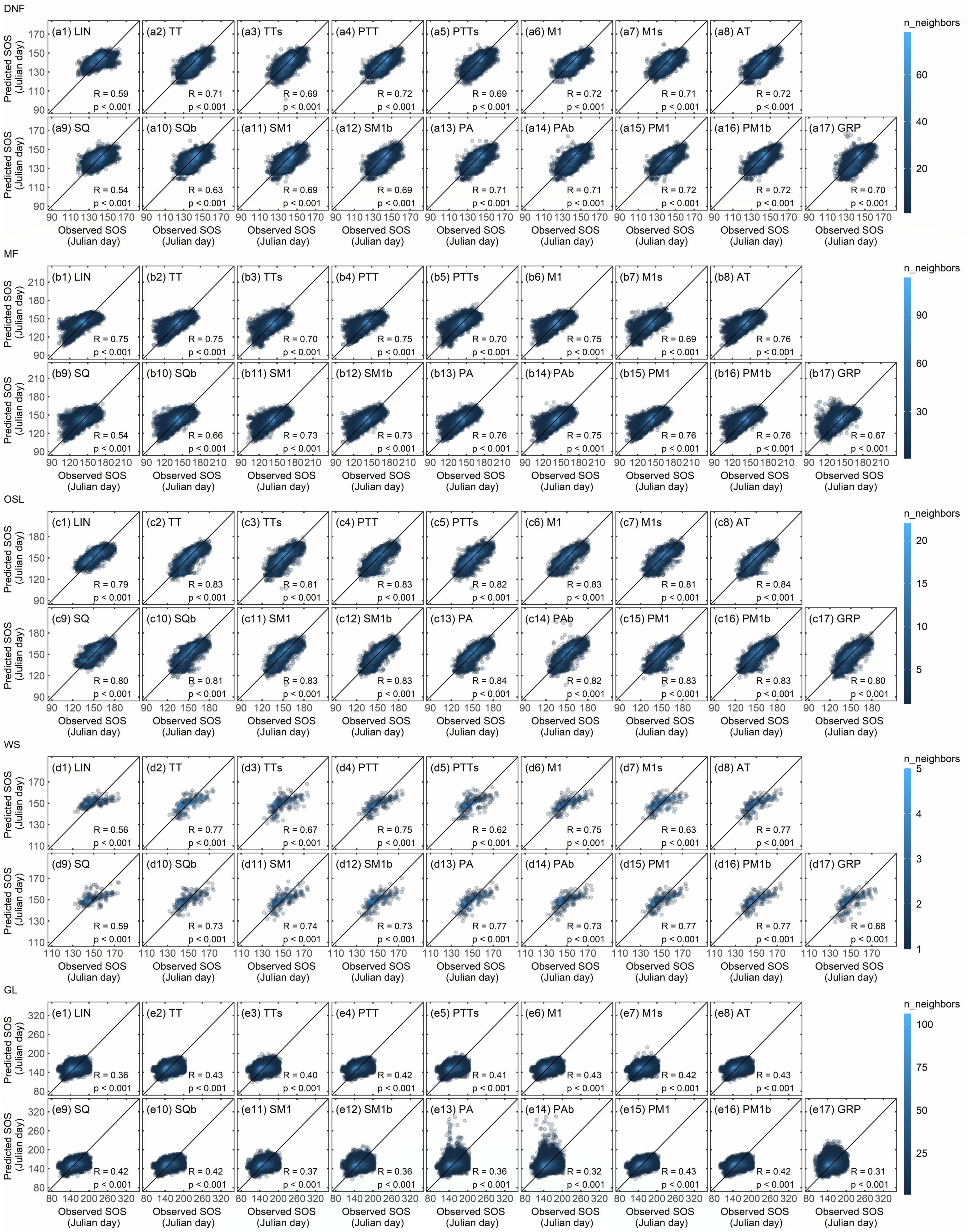
Figure 2. Scatter plot of the model-predicted start of the growing season (SOS) and satellite-derived SOS. The dots in each graph indicate all pixels for each vegetation type across the model training period. Figures a1–a17, b1–b17, c1–c17, d1–d17 and e1–e17 show the prediction results of the 17 models of deciduous needleleaf forest (DNF), mixed forest (MF), open shrublands (OSL), woody savannas (WS), and grasslands (GL), respectively. The solid lines in the figures are 1:1 lines. A point on the line indicates that the satellite-derived SOS is equal to the model-predicted SOS. n_neighbors indicates the number of points within the radius of each data point, which reflected the degree of aggregation of data points. It was drawn by geom_pointdensity in the ggpointdensity package (version 0.1.0) in R, with the radius adjustment parameter set to 0.1.
Figure 3 shows the box plot of RMSE between the SOS predicted by the 17 models and the SOS derived from the satellite. From Figures 3A, C, it can be seen that for DNF and OSL the difference in the prediction results of most spring phenological models was relatively small. For MF and WS, the temperature response functions and model structures had a significant impact on the model prediction results, while for GL, there was no significant difference in the prediction results of most models.
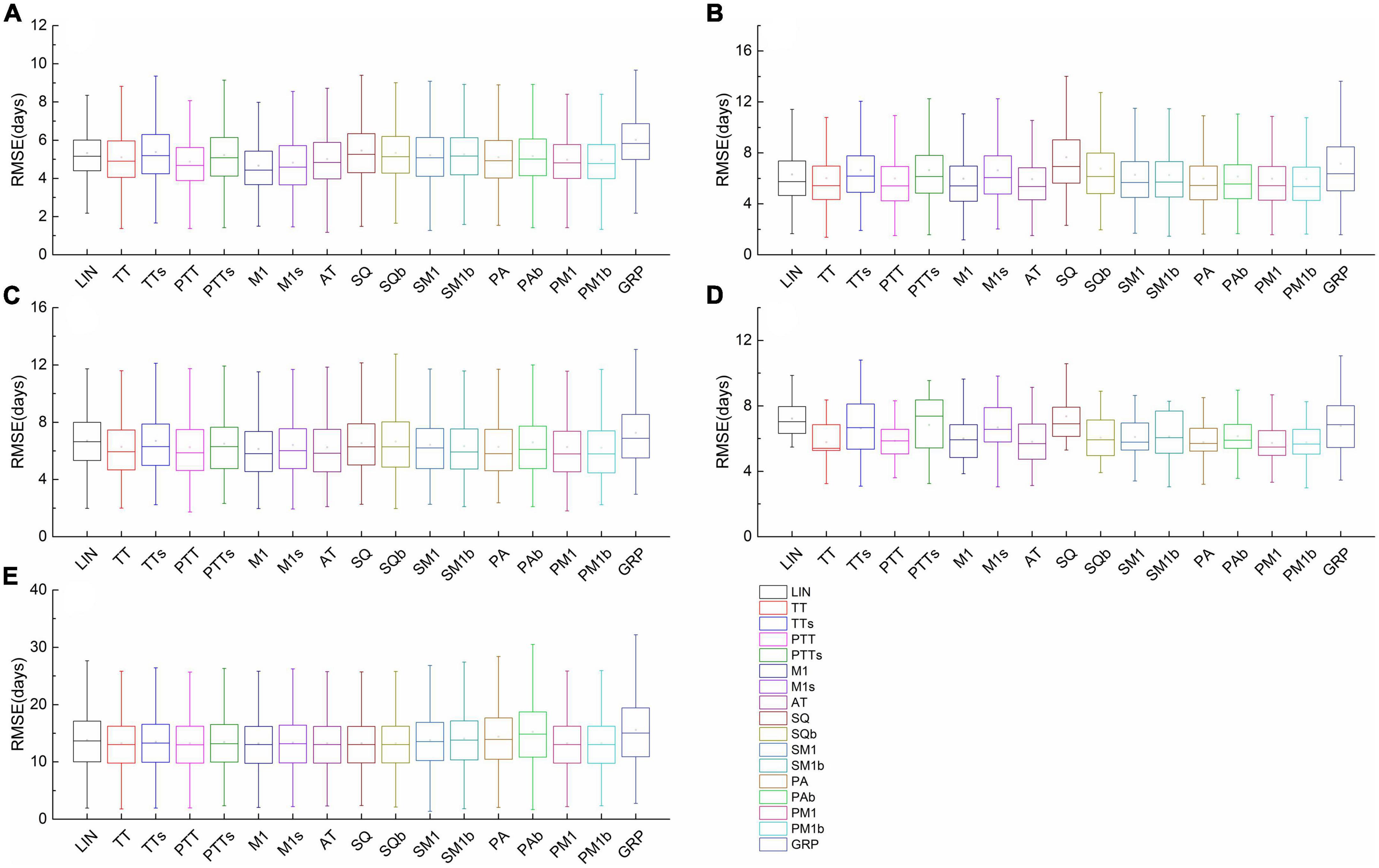
Figure 3. Comparison of the results of 17 spring phenological models for five vegetation types: (A) deciduous needleleaf forest (DNF), (B) mixed forest (MF), (C) open shrublands (OSL), (D) woody savannas (WS), and (E) grasslands (GL). The five panels show box plots of the root mean square error (RMSE) between the start of the growing season (SOS) predicted by the 17 models used in this study and the satellite-derived SOS. The RMSEs were calculated from the validation dataset.
To find the optimal spring phenological model for each vegetation type, we listed the RMSE, correlation coefficient, and AIC of the SOS predicted by the 17 models and the SOS derived from the satellite (Table 3). For DNF, the M1 model was the best model for predicting spring phenology, because it had the lowest RMSE and AIC and the highest correlation coefficient. Similarly, the M1 model was the best model for predicting the spring phenology of OSL and GL. For MF, the AT model achieved the lowest RMSE and AIC and the highest correlation coefficient, making it the optimal model for predicting spring phenology. The PM1 model was the best model for predicting the spring phenology of WS.
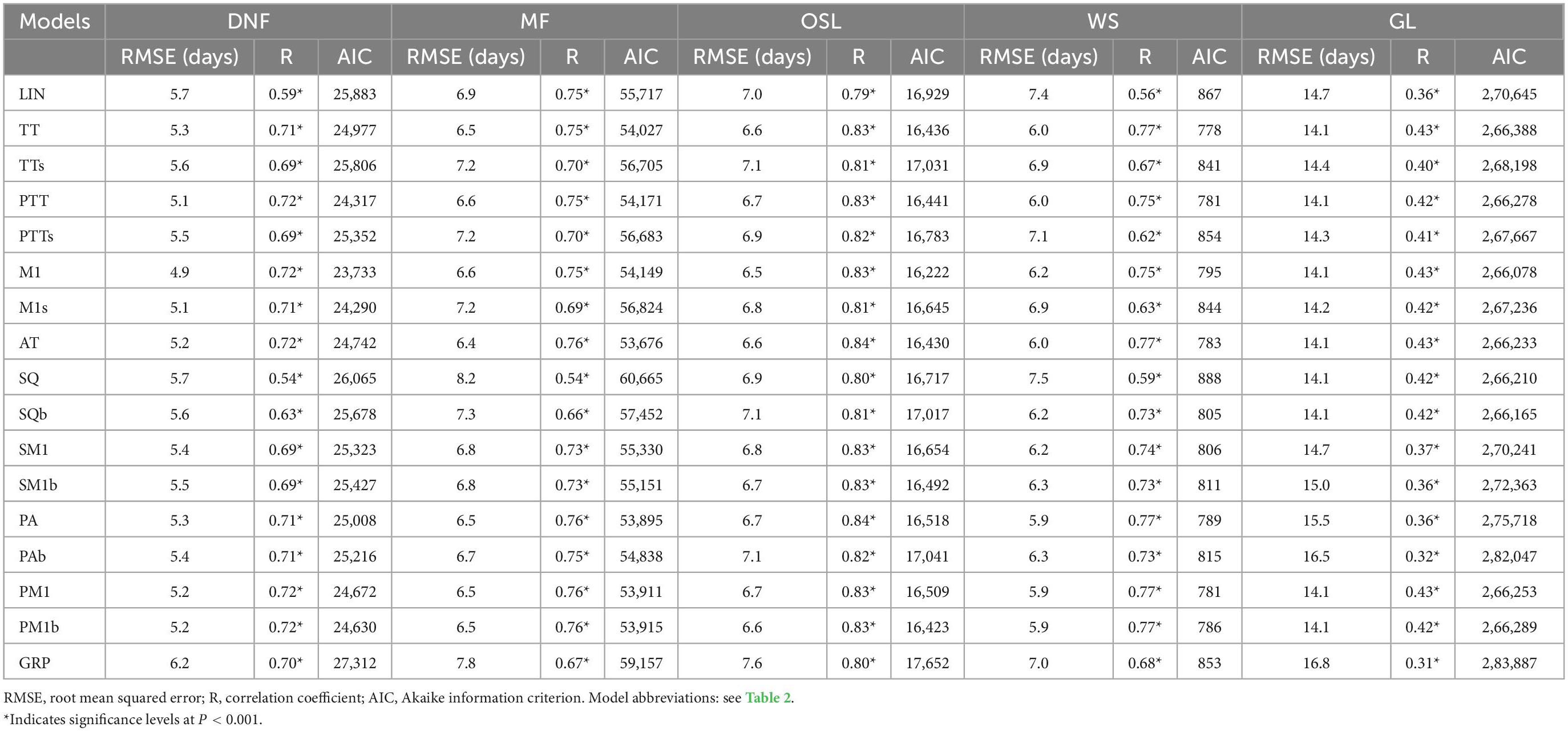
Table 3. Summary of the satellite-derived start of the growing season (SOS) versus the simulated SOS (in days) for the 17 models for five vegetation types [deciduous needleleaf forest (DNF), mixed forest (MF), open shrublands (OSL), woody savannas (WS), and grasslands (GL)].
We calculated the average results of the TT, PTT, and M1 models and the average results of the TTs, PTTs, and M1s models to compare the difference between growing-degree-day temperature response and sigmoid temperature response. We also calculated the average results of the SQ, PA, SM1, and PM1 models and the average results of the SQb, PAb, SM1b, and PM1b models to compare the difference between the triangular chilling response and the bell-shaped chilling response. Finally, we calculated the average results of the one-phase models and of the two-phase models to analyze the influence of the model structure (Figure 4).
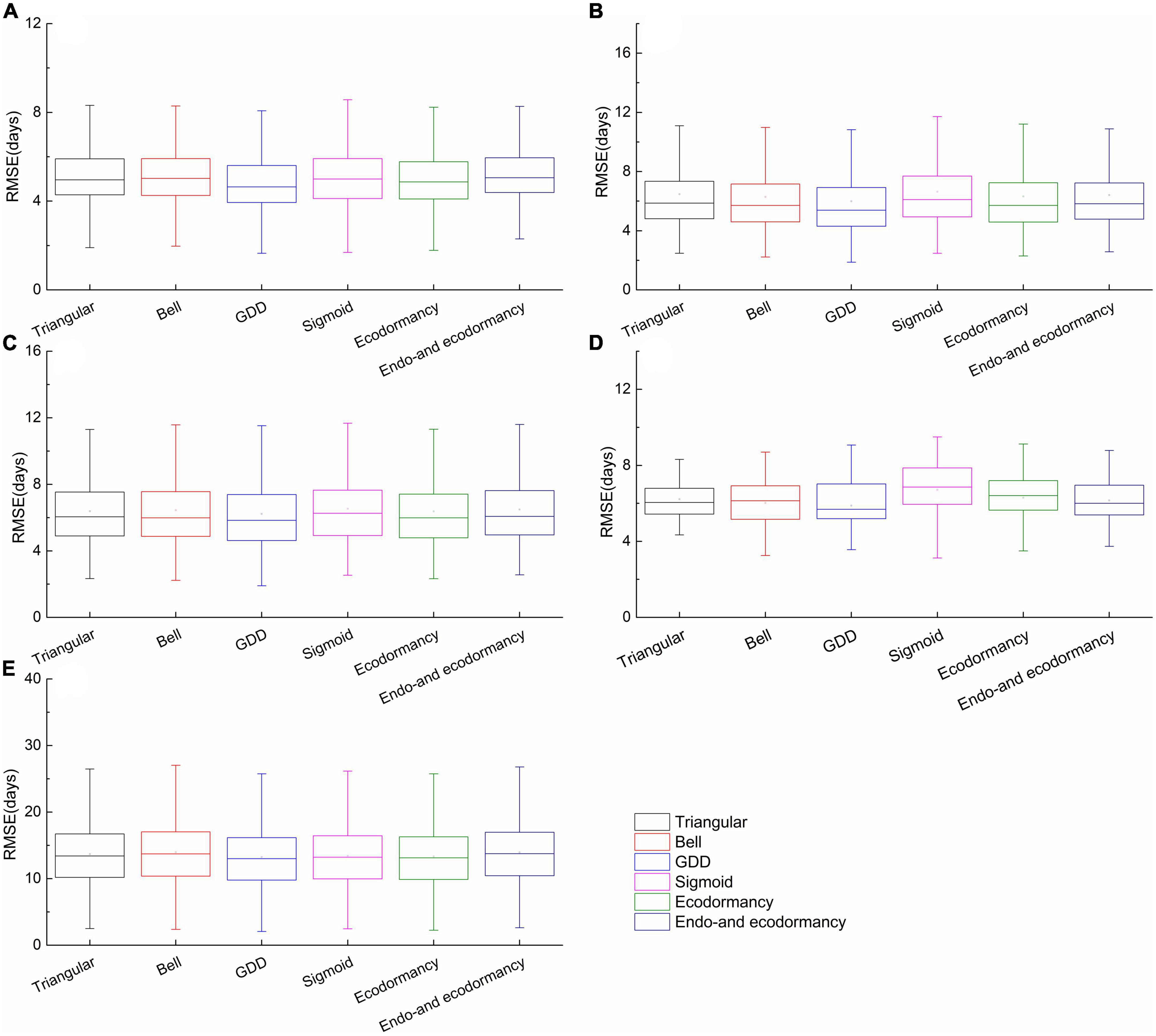
Figure 4. RMSE predicted by spring phenological models with different temperature response functions and model structures: (A) deciduous needleleaf forest (DNF), (B) mixed forest (MF), (C) open shrublands (OSL), (D) woody savannas (WS), and (E) grasslands (GL). Triangular and Bell are the average results of the model using triangular temperature response (SQ, PA, SM1, and PM1) and bell-shape temperature response (SQb, PAb, SM1b, and PM1b) during the endodormancy phase, respectively. GDD and Sigmoid are the average results of the model using growing-degree-day temperature response (TT, PTT, and M1) and sigmoid temperature response (TTs, PTTs, and M1s) during the ecodormancy phase, respectively. Ecodormancy, and endo- and ecodormancy, refer to the average results of the one-phase model and the two-phase model, respectively.
For all vegetation types in this study, the difference in chilling temperature response had no significant effect on the model predictions. The difference in forcing temperature response had a significant impact on the prediction results of the spring phenological model. Overall, compared with the model using the sigmoid temperature response, the model using the growing-degree-day temperature response had a lower RMSE.
Figure 5 shows the RMSE of the satellite-derived SOS versus the simulated SOS for the spring phenological models using Tmax, Tmean, and Tmin. As shown in the figure, the performance of the models varied by vegetation type. In general, for DNF, OSL, and WS, the lowest RMSE was obtained when the model used the maximum daily temperature to predict the spring phenology. For MF, compared with the maximum daily temperature, a lower or similar RMSE was obtained when the model used the mean daily temperature. For GL, the lowest RMSE was achieved when the model used the minimum daily temperature, while for DNF, MF, OSL, and WS, the highest RMSE was achieved when the model used the minimum daily temperature.
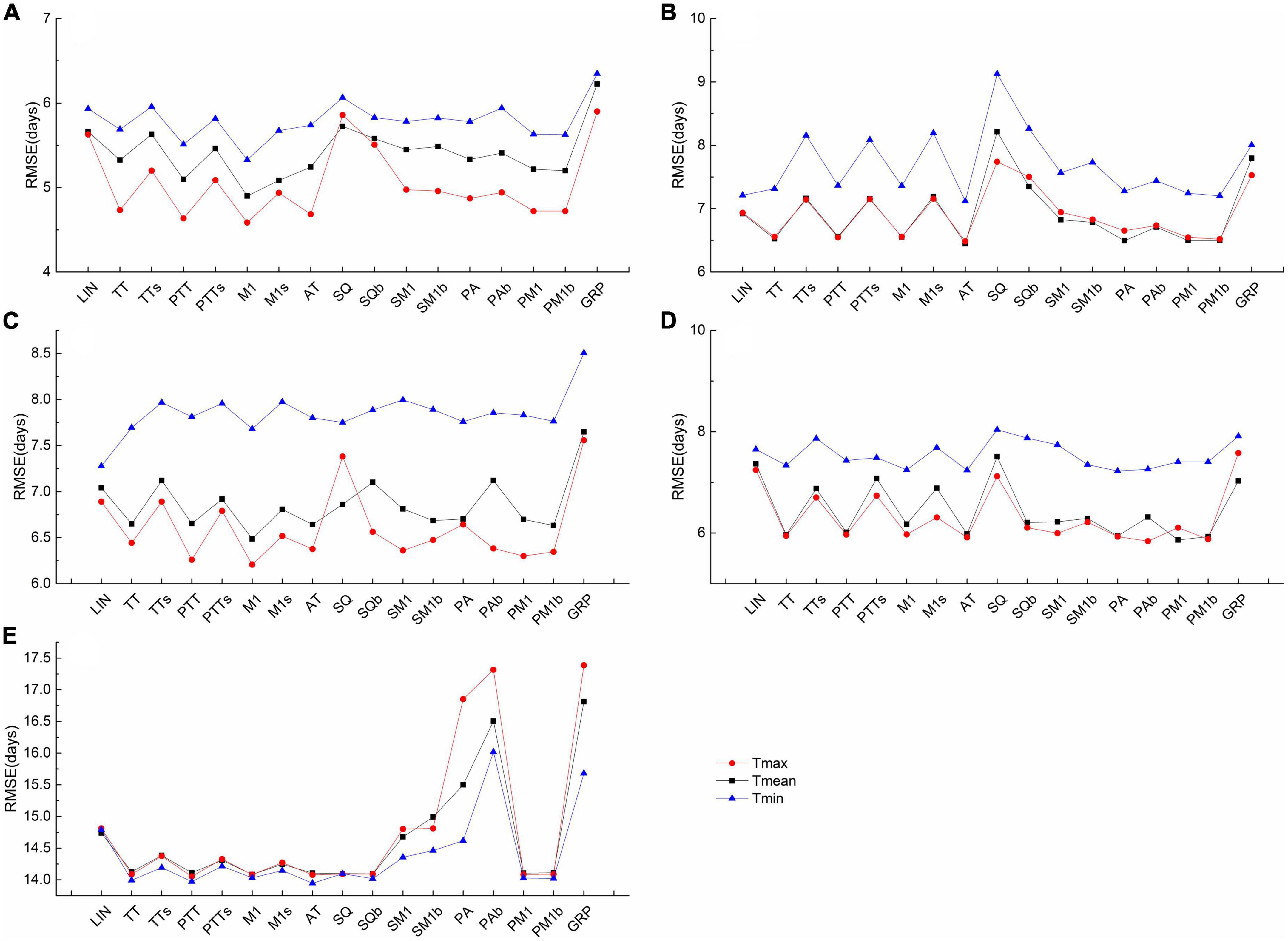
Figure 5. RMSE of the satellite-derived start of the growing season (SOS) versus the simulated SOS for the 17 models parameterized using Tmax, Tmean, and Tmin for five vegetation types: (A) deciduous needleleaf forest (DNF), (B) mixed forest (MF), (C) open shrublands (OSL), (D) woody savannas (WS), and (E) grasslands (GL). Model abbreviations: see Table 2. The RMSEs were estimated from the SOSs of the validation period.
4. Discussion and conclusion
The SOS of five vegetation types extracted using the NDVI dataset for the period 1982–2014 was used to parameterize and evaluate the performance of 17 spring phenological models. The effects of different chilling temperature responses, forcing temperature responses, and model structure on the prediction performance of the model were compared. The effects of Tmean, Tmin, and Tmax on the performance of the models were also tested.
For the five vegetation types studied, the models that explained only the ecodormancy release gave RMSE and correlation coefficients similar to the models that explained the releases of both endo- and ecodormancy, consistent with previous studies (Hänninen and Kramer, 2007; Linkosalo et al., 2008; Granhus et al., 2009; Basler, 2016). This may be because the spring temperature accumulation process that explains the release of ecodormancy common to all models dominates the occurrence of spring phenology (although the temperature response function is different), while other factors, such as the chilling response function, play only a modulating role. In the models that explain only the ecodormancy release, it is assumed that the chilling response is satisfied. This assumption is often valid under northern climatic conditions (such as the study area) but may lead to incorrect results in the future as the climate warms. The environmental adaptation strategies of different vegetation types tend to vary considerably, and differences in the best models were generally the result of a combination of model structure and environmental drivers. For DNF and OSL, SOS was mainly driven by spring forcing and photoperiod; for MF, SOS was mainly controlled jointly by chilling and forcing; and for WS, SOS was driven by a combination of chilling, forcing, and photoperiod.
Piao et al. (2015) showed that leaf onset was triggered by Tmax rather than Tmin or Tmean, and this finding was supported by the analysis of satellite-derived spring vegetation phenology in the northern hemisphere (>30°N). The results of the current study indicated that Tmax had the highest impact on DNF, OSL and WS, and Tmean had the highest impact on MF. As for GL, most models parameterized by Tmin gave the lowest RMSE, indicating that the spring phenology of grassland was mainly affected by Tmin. Shen et al. (2018) showed that for the temperate grasslands of China, Tmin had a greater effect in spring, consistent with the results of this study.
Shen et al. (2018) demonstrated that for temperate grasslands in China, precipitation significantly affected grassland SOS. On average, an increase of 10 mm in precipitation during spring will advance the SOS by 1.7 days. Liu et al. suggested that factors such as precipitation should be considered when simulating the spring phenology of grasslands in the northern hemisphere (Liu et al., 2018). The GRP model used in this study considered the impact of precipitation, but the simulation results did not improve. This may be a limitation of the model structure, because the interactions between the drivers are still largely unknown. In addition, soil moisture may also be an important factor affecting the spring phenology of grassland (Liu et al., 2013). Because of the unsatisfactory results of spring phenology predicted by existing models for GL, the effects of temperature, photoperiod, and precipitation need to be further investigated. In addition, soil moisture can be integrated into future spring phenological models (Liu et al., 2013; Tao et al., 2020).
It should be noted that although Tmax and Tmin showed asymmetric effects on the performance of most models, their effects may be different for one-phase and two-phase models. One-phase models only consider cumulative forcing temperature, which may be influenced mainly by Tmax, whereas two-phase models consider both cumulative chilling temperature and cumulative forcing temperature, where the chilling temperature accumulation process may be more influenced by Tmin. For two-phase models, especially those with concurrent cumulative chilling and cumulative forcing temperatures, the effects of Tmax and Tmin need to be further investigated.
Model structure is a decisive factor affecting model performance; thus, understanding the interaction of the environment with plant growth is critical. In this study, the effects of chilling, forcing, photoperiod, and precipitation on spring phenology were considered, but the synergistic relationship of each factor still needs to be further clarified. Manipulating experiments is a useful means of understanding the impact of environmental factors on plant growth and can help improve the predictive performance of models (Piao et al., 2020; Prevéy et al., 2021). In addition, phenological models are usually built at the species level, and the phenological information extracted in this study may be an integrated result from different species. Studies have shown that different tree species have different temperature and photoperiod sensitivities (Körner and Basler, 2010; Zohner et al., 2016; Fu et al., 2019). With the development of remote sensing technology, high-resolution data are increasingly available. High-resolution phenological data can be used to build models to reduce uncertainty in modeling datasets.
Our study shows that the satellite-derived SOS of DNF, MF, OSL, and WS had a higher correlation coefficient with the model-predicted SOS, with most exceeding 0.7, while the corresponding correlation coefficient of GL did not exceed 0.5. For all the five vegetation types studied, the difference in chilling temperature response had no significant effect on the model predictions, while the difference in forcing temperature response had a significant impact on the prediction results of the spring phenological model. Overall, compared with the model using a sigmoid temperature response, the model using the growing-degree-day temperature response had a lower RMSE. The models explaining only the ecodormancy release had RMSEs and correlation coefficients similar to those of models explaining the releases of both endo- and ecodormancy. The most suitable temperature predictors for spring phenology were Tmax for DNF, OSL, and WS; Tmean for MF; and Tmin for GL. Because phenology models based on temperature, photoperiod, and precipitation are not always satisfactory, their impact needs further study. Furthermore, asymmetric effects between daytime and nighttime temperatures in different vegetation types should be incorporated into future spring phenology models.
Data availability statement
The original contributions presented in this study are included in the article/Supplementary material, further inquiries can be directed to the corresponding author.
Author contributions
YF designed the research and drafted the manuscript. YM performed the analysis. All authors contributed to the interpretation of the results and to the text and approved the submitted version.
Funding
This study was funded by the National Science Fund for Distinguished Young Scholars (Grant No. 42025101), the National Key Research and Development Program of China (Grant No. 2017YFA06036001), the National Natural Science Foundation of China (Grant No. 31770516), the 111 Project (Grant No. B18006), the Program B for Outstanding Ph.D. candidate of Nanjing University, and the fundamental research project of MOST (2005DKA32306).
Acknowledgments
We thank the NASA GIMMS team for providing the AVHRR GIMMS NDVI3g.v1 dataset, the Global Land Cover Facility for providing the MODIS land cover, and the European Centre for Medium-Range Weather Forecasts for providing the ERA-Interim dataset. We also thank Leonie Seabrook, Ph.D. Liwen Bianji (Edanz) (www.liwenbianji.cn), for editing the language of a draft of this manuscript.
Conflict of interest
The authors declare that the research was conducted in the absence of any commercial or financial relationships that could be construed as a potential conflict of interest.
Publisher’s note
All claims expressed in this article are solely those of the authors and do not necessarily represent those of their affiliated organizations, or those of the publisher, the editors and the reviewers. Any product that may be evaluated in this article, or claim that may be made by its manufacturer, is not guaranteed or endorsed by the publisher.
Supplementary material
The Supplementary Material for this article can be found online at: https://www.frontiersin.org/articles/10.3389/ffgc.2022.1032066/full#supplementary-material
Footnotes
References
Akaike, H. (1974). A new look at the statistical model identification. IEEE Trans. Automat. Contr. 19, 716–723. doi: 10.1109/TAC.1974.1100705
Anderegg, W. R., and Diffenbaugh, N. S. (2016). Observed and projected climate trends and hotspots across the national ecological observatory network regions. Front. Ecol. Environ. 13:547–552. doi: 10.1890/150159
Basler, D. (2016). Evaluating phenological models for the prediction of leaf-out dates in six temperate tree species across central Europe. Agric. For. Meteorol. 217, 10–21. doi: 10.1016/j.agrformet.2015.11.007
Berrisford, P., Dee, D. P., Fielding, K., Fuentes, M., Kållberg, P. W., Kobayashi, S., et al. (2009). The ERA-interim archive. Era Report. Technical Report. Reading: European Centre for Medium-Range Weather Forecasts.
Berrisford, P., Dee, D., Poli, P., Brugge, R., Fielding, K., Fuentes, M., et al. (2011). The ERA-interim archive: Version 2.0. Nihon Seirigaku Zasshi 31, 1–23.
Blümel, K., and Chmielewski, F.-M. (2012). Shortcomings of classical phenological forcing models and a way to overcome them. Agric. For. Meteorol. 164, 10–19. doi: 10.1016/j.agrformet.2012.05.001
Botta, A., Viovy, N., Ciais, P., Friedlingstein, P., and Monfray, P. (2000). A global prognostic scheme of leaf onset using satellite data. Glob. Chang. Biol. 6, 709–725. doi: 10.1046/j.1365-2486.2000.00362.x
Brown, M., De Beurs, K., and Marshall, M. (2012). Global phenological response to climate change in crop areas using satellite remote sensing of vegetation, humidity and temperature over 26 years. Remote Sens. Environ. 126, 174–183. doi: 10.1016/j.rse.2012.08.009
Caffarra, A., Donnelly, A., and Chuine, I. (2011). Modelling the timing of Betula pubescens budburst. II. Integrating complex effects of photoperiod into process-based models. Clim. Res. 46, 159–170. doi: 10.3354/cr00983
Cannell, M., and Smith, R. (1983). Thermal time, chill days and prediction of budburst in Picea sitchensis. J. Appl. Ecol. 20, 951–963. doi: 10.2307/2403139
Castillioni, K., Newman, G. S., Souza, L., and Iler, A. M. (2022). Effects of drought on grassland phenology depend on functional types. New Phytol. 236, 1558–1571. doi: 10.1111/nph.18462
Channan, S., Collins, K., and Emanuel, W. (2014). Global mosaics of the standard MODIS land cover type data. College Park, MD: University of Maryland and the Pacific Northwest National Laboratory.
Chuine, I. (2000). A unified model for budburst of trees. J. Theor. Biol. 207, 337–347. doi: 10.1006/jtbi.2000.2178
Chuine, I., and Beaubien, E. G. (2001). Phenology is a major determinant of tree species range. Ecol. Lett. 4, 500–510. doi: 10.1046/j.1461-0248.2001.00261.x
Chuine, I., Cambon, G., and Comtois, P. (2000). Scaling phenology from the local to the regional level: Advances from species-specific phenological models. Glob. Chang. Biol. 6, 943–952. doi: 10.1046/j.1365-2486.2000.00368.x
Chuine, I., Cour, P., and Rousseau, D. (1999). Selecting models to predict the timing of flowering of temperate trees: Implications for tree phenology modelling. Plant Cell Environ. 22, 1–13. doi: 10.1046/j.1365-3040.1999.00395.x
Cleland, E. E., Isabelle, C., Annette, M., Mooney, H. A., and Schwartz, M. D. (2007). Shifting plant phenology in response to global change. Trends Ecol. Evol. 22, 357–365. doi: 10.1016/j.tree.2007.04.003
Ćrepinšek, Z., Kajfež-Bogataj, L., and Bergant, K. (2006). Modelling of weather variability effect on fitophenology. Ecol. Modell. 194, 256–265. doi: 10.1016/j.ecolmodel.2005.10.020
de Beurs, K. M., and Henebry, G. M. (2008). Northern annular mode effects on the land surface phenologies of Northern Eurasia. J. Clim. 21, 4257–4279. doi: 10.1175/2008JCLI2074.1
Delpierre, N., Vitasse, Y., Chuine, I., Guillemot, J., Bazot, S., and Rathgeber, C. B. (2016). Temperate and boreal forest tree phenology: From organ-scale processes to terrestrial ecosystem models. Ann. For. Sci. 73, 5–25. doi: 10.1007/s13595-015-0477-6
Friedl, M. A., Sullamenashe, D., Tan, B., Schneider, A., Ramankutty, N., Sibley, A., et al. (2010). MODIS collection 5 global land cover: Algorithm refinements and characterization of new datasets. Remote Sens. Environ. 114, 168–182. doi: 10.1016/j.rse.2009.08.016
Fu, Y. H., Liu, Y., De Boeck, H. J., Menzel, A., Nijs, I., Peaucelle, M., et al. (2016). Three times greater weight of daytime than of night-time temperature on leaf unfolding phenology in temperate trees. New Phytol. 212, 590–597. doi: 10.1111/nph.14073
Fu, Y. H., Piao, S., Zhou, X., Geng, X., Hao, F., Vitasse, Y., et al. (2019). Short photoperiod reduces the temperature sensitivity of leaf-out in saplings of Fagus sylvatica but not in horse chestnut. Glob. Chang. Biol. 25, 1696–1703. doi: 10.1111/gcb.14599
Fu, Y. H., Zhao, H., Piao, S., Peaucelle, M., Peng, S., Zhou, G., et al. (2015). Declining global warming effects on the phenology of spring leaf unfolding. Nature 526, 104–107. doi: 10.1038/nature15402
Fu, Y., Li, X., Zhou, X., Geng, X., Guo, Y., and Zhang, Y. (2020). Progress in plant phenology modeling under global climate change. Sci. China Earth Sci. 63, 1237–1247. doi: 10.1007/s11430-019-9622-2
García-Mozo, H., Galán, C., Belmonte, J., Bermejo, D., Candau, P., de la Guardia, C. D., et al. (2009).. Predicting the start and peak dates of the Poaceae pollen season in Spain using process-based models. Agric. For. Meteorol. 149, 256–262. doi: 10.1016/j.agrformet.2008.08.013
Gauzere, J., Lucas, C., Ronce, O., Davi, H., and Chuine, I. (2019). Sensitivity analysis of tree phenology models reveals increasing sensitivity of their predictions to winter chilling temperature and photoperiod with warming climate. Ecol. Model. 411:108805. doi: 10.1016/j.ecolmodel.2019.108805
Granhus, A., Fløistad, I. S., and Søgaard, G. (2009). Bud burst timing in Picea abies seedlings as affected by temperature during dormancy induction and mild spells during chilling. Tree Physiol. 29, 497–503. doi: 10.1093/treephys/tpn039
Hänninen, H. (1990). Modelling bud dormancy release in trees from cool and temperate regions. Acta For. Fenn. 213, 1–47. doi: 10.14214/aff.7660
Hänninen, H., and Kramer, K. (2007). A framework for modelling the annual cycle of trees in boreal and temperate regions. Silva Fenn. 41, 167–205. doi: 10.14214/sf.313
Henry, G., and Molau, U. (1997). Tundra plants and climate change: The international Tundra experiment (ITEX). Glob. Chang. Biol. 3, 1–9. doi: 10.1111/j.1365-2486.1997.gcb132.x
Hufkens, K., Basler, D., Milliman, T., Melaas, E. K., and Richardson, A. D. (2018). An integrated phenology modelling framework in R. Methods Ecol. Evol. 9, 1276–1285. doi: 10.1111/2041-210X.12970
Hunter, A. F., and Lechowicz, M. J. (1992). Predicting the timing of budburst in temperate trees. J. Appl. Ecol. 29, 597–604. doi: 10.2307/2404467
Jonsson, P., and Eklundh, L. (2002). Seasonality extraction by function fitting to time-series of satellite sensor data. IEEE Trans. Geosci. Remote Sens. 40, 1824–1832. doi: 10.1109/TGRS.2002.802519
Jönsson, P., and Eklundh, L. (2004). TIMESAT–a program for analyzing time-series of satellite sensor data. Comput. Geosci. 30, 833–845. doi: 10.1016/j.cageo.2004.05.006
Julien, Y., and Sobrino, J. A. (2009). Global land surface phenology trends from GIMMS database. Int. J. Remote Sens. 30, 3495–3513. doi: 10.1080/01431160802562255
Körner, C., and Basler, D. (2010). Phenology under global warming. Science 327, 1461–1462. doi: 10.1126/science.1186473
Kramer, K. (1994). Selecting a model to predict the onset of growth of Fagus sylvatica. J. Appl. Ecol. 31, 172–181. doi: 10.2307/2404609
Krinner, G., Viovy, N., de Noblet-Ducoudré, N., Ogée, J., Polcher, J., Friedlingstein, P., et al. (2005). A dynamic global vegetation model for studies of the coupled atmosphere-biosphere system. Glob. Biogeochem. Cycles 19, 1–44. doi: 10.1029/2003GB002199
Landsberg, J. (1974). Apple fruit bud development and growth; analysis and an empirical model. Ann. Bot. 38, 1013–1023. doi: 10.1093/oxfordjournals.aob.a084891
Lang, G., Early, J. D., Martin, G., and Darnell, R. (1987). Endo-, para-, and ecodormancy: Physiological terminology and classification for dormancy research. HortScience 22, 371–377. doi: 10.21273/HORTSCI.22.3.371
Linkosalo, T., Lappalainen, H. K., and Hari, P. (2008). A comparison of phenological models of leaf bud burst and flowering of boreal trees using independent observations. Tree Physiol. 28, 1873–1882. doi: 10.1093/treephys/28.12.1873
Liu, H., Tian, F., Hu, H., Hu, H., and Sivapalan, M. (2013). Soil moisture controls on patterns of grass green-up in Inner Mongolia: An index based approach. Hydrol. Earth Syst. Sci. 17, 805–815. doi: 10.5194/hess-17-805-2013
Liu, Q., Fu, Y. H., Liu, Y., Janssens, I. A., and Piao, S. (2018). Simulating the onset of spring vegetation growth across the Northern Hemisphere. Glob. Chang. Biol. 24, 1342–1356. doi: 10.1111/gcb.13954
Liu, Q., Fu, Y. H., Zeng, Z., Huang, M., Li, X., and Piao, S. (2016). Temperature, precipitation, and insolation effects on autumn vegetation phenology in temperate China. Glob. Chang. Biol. 22, 644–655. doi: 10.1111/gcb.13081
Malyshev, A. V. (2020). Warming events advance or delay spring phenology by affecting bud dormancy depth in trees. Front. Plant Sci. 11:856. doi: 10.3389/fpls.2020.00856
Masle, J., Doussinault, G., Farquhar, G., and Sun, B. (1989). Foliar stage in wheat correlates better to photothermal time than to thermal time. Plant Cell Environ. 12, 235–247. doi: 10.1111/j.1365-3040.1989.tb01938.x
Meehl, G. A., Arblaster, J. M., Fasullo, J. T., Hu, A., and Trenberth, K. E. (2011). Model-based evidence of deep-ocean heat uptake during surface-temperature hiatus periods. Nat. Clim. Chang. 1, 360–364. doi: 10.1038/nclimate1229
Meng, L., Zhou, Y., Gu, L., Richardson, A. D., Peñuelas, J., Fu, Y., et al. (2021). Photoperiod decelerates the advance of spring phenology of six deciduous tree species under climate warming. Glob. Chang. Biol. 27, 2914–2927. doi: 10.1111/gcb.15575
Meng, L., Zhou, Y., Li, X., Asrar, G. R., Mao, J., and Wanamaker, A. D. Jr., et al. (2020). Divergent responses of spring phenology to daytime and nighttime warming. Agric. For. Meteorol. 281:107832. doi: 10.1016/j.agrformet.2019.107832
Menzel, A., and Fabian, P. (1999). Growing season extended in Europe. Nature 397:659. doi: 10.1038/17709
Mo, Y., Chen, S., Jin, J., Lu, X., and Jiang, H. (2019). Temporal and spatial dynamics of phenology along the North–South Transect of Northeast Asia. Int. J. Remote Sens. 40, 7922–7940. doi: 10.1080/01431161.2019.1608390
Morisette, J. T., Richardson, A. D., Knapp, A. K., Fisher, J. I., Graham, E. A., Abatzoglou, J., et al. (2009). Tracking the rhythm of the seasons in the face of global change: Phenological research in the 21st century. Front. Ecol. Environ. 7:253–260. doi: 10.1890/070217
Murray, M., Cannell, M., and Smith, R. (1989). Date of budburst of fifteen tree species in Britain following climatic warming. J. Appl. Ecol. 26, 693–700. doi: 10.2307/2404093
Oberbauer, S. F., Elmendorf, S. C., Troxler, T. G., Hollister, R. D., Rocha, A. V., Bret-Harte, M. S., et al. (2013). Phenological response of tundra plants to background climate variation tested using the International Tundra experiment. Philos. Trans. R. Soc. Lond. B Biol. Sci. 368:20120481. doi: 10.1098/rstb.2012.0481
Park, H., Jeong, S.-J., Ho, C.-H., Kim, J., Brown, M. E., and Schaepman, M. E. (2015). Nonlinear response of vegetation green-up to local temperature variations in temperate and boreal forests in the Northern Hemisphere. Remote Sens. Environ. 165, 100–108. doi: 10.1016/j.rse.2015.04.030
Parmesan, C. (2007). Influences of species, latitudes and methodologies on estimates of phenological response to global warming. Glob. Chang. Biol. 13, 1860–1872. doi: 10.1111/j.1365-2486.2007.01404.x
Pau, S., Wolkovich, E. M., Cook, B. I., Davies, T. J., Kraft, N. J. B., Bolmgren, K., et al. (2011). Predicting phenology by integrating ecology, evolution and climate science. Glob. Chang. Biol. 17, 3633–3643. doi: 10.1111/j.1365-2486.2011.02515.x
Peano, D., Hemming, D., Materia, S., Delire, C., Fan, Y., Joetzjer, E., et al. (2021). Plant phenology evaluation of CRESCENDO land surface models–Part 1: Start and end of the growing season. Biogeosciences 18, 2405–2428. doi: 10.5194/bg-18-2405-2021
Peano, D., Materia, S., Collalti, A., Alessandri, A., Anav, A., Bombelli, A., et al. (2019). Global variability of simulated and observed vegetation growing season. J. Geophys. Res. Biogeosci. 124, 3569–3587. doi: 10.1029/2018JG004881
Peñuelas, J., and Filella, I. (2001). Responses to a warming world. Science 294, 793–795. doi: 10.1126/science.1066860
Piao, S., Fang, J., Zhou, L., Ciais, P., and Zhu, B. (2006). Variations in satellite-derived phenology in China’s temperate vegetation. Glob. Chang. Biol. 12, 672–685. doi: 10.1111/j.1365-2486.2006.01123.x
Piao, S., Tan, J., Chen, A., Fu, Y. H., Ciais, P., Liu, Q., et al. (2015). Leaf onset in the northern hemisphere triggered by daytime temperature. Nat. Commun. 6:6911. doi: 10.1038/ncomms7911
Piao, S., Wang, X., Park, T., Chen, C., Lian, X., He, Y., et al. (2020). Characteristics, drivers and feedbacks of global greening. Nat. Rev. Earth Environ. 1, 14–27. doi: 10.1038/s43017-019-0001-x
Prevéy, J. S., Vitasse, Y., and Fu, Y. (2021). Experimental manipulations to predict future plant phenology. Front. Plant Sci. 11:2183. doi: 10.3389/fpls.2020.637156
Prevéy, J., Vellend, M., Rüger, N., Hollister, R. D., Bjorkman, A. D., Myers-Smith, I. H., et al. (2017). Greater temperature sensitivity of plant phenology at colder sites: Implications for convergence across northern latitudes. Glob. Chang. Biol. 23, 2660–2671. doi: 10.1111/gcb.13619
Price, M. V., and Waser, N. M. (1998). Effects of experimental warming on plant reproductive phenology in a subalpine meadow. Ecology 79, 1261–1271. doi: 10.1890/0012-9658(1998)079[1261:EOEWOP]2.0.CO;2
Reaumur, R. D. (1735). Observations du thermomètre faites à Paris pendant l’année 1735, comparées avec celles qui ont été faites sous la ligne, à l’Isle de France, à Alger et quelques unes de nos iles de l’Amérique. Mémoires Acad. R. Sci. 1735, 545–576.
Richardson, A. D., Keenan, T. F., Migliavacca, M., Ryu, Y., Sonnentag, O., and Toomey, M. (2013). Climate change, phenology, and phenological control of vegetation feedbacks to the climate system. Agric. For. Meteorol. 169, 156–173. doi: 10.1016/j.agrformet.2012.09.012
Schaber, J., and Badeck, F.-W. (2003). Physiology-based phenology models for forest tree species in Germany. Int. J. Biometeorol. 47, 193–201. doi: 10.1007/s00484-003-0171-5
Shen, M., Piao, S., Chen, X., An, S., Fu, Y. H., Wang, S., et al. (2016). Strong impacts of daily minimum temperature on the green-up date and summer greenness of the Tibetan Plateau. Glob. Chang. Biol. 22, 3057–3066. doi: 10.1111/gcb.13301
Shen, X., Liu, B., Henderson, M., Wang, L., Wu, Z., Wu, H., et al. (2018). Asymmetric effects of daytime and nighttime warming on spring phenology in the temperate grasslands of China. Agric. For. Meteorol. 259, 240–249. doi: 10.1016/j.agrformet.2018.05.006
Suzuki, R., Nomaki, T., and Yasunari, T. (2003). West–east contrast of phenology and climate in northern Asia revealed using a remotely sensed vegetation index. Int. J. Biometeorol. 47, 126–138. doi: 10.1007/s00484-003-0164-4
Tao, Z., Huang, W., and Wang, H. (2020). Soil moisture outweighs temperature for triggering the green-up date in temperate grasslands. Theor. Appl. Climatol. 140, 1093–1105. doi: 10.1007/s00704-020-03145-z
Thackeray, S. J., Henrys, P. A., Hemming, D., Bell, J. R., Botham, M. S., Burthe, S., et al. (2016). Phenological sensitivity to climate across taxa and trophic levels. Nature 535:241. doi: 10.1038/nature18608
Wang, J. Y. (1960). A critique of the heat unit approach to plant response studies. Ecology 41, 785–790. doi: 10.2307/1931815
Wang, J., Ping, S. U., and Elena, A. (2016). Land cover change characteristics of North-South Transect in Northeast Asia from 2001 to 2012. J. Resour. Ecol. 7, 36–43. doi: 10.5814/j.issn.1674-764x.2016.01.005
Wang, J., Xi, Z., He, X., Chen, S., Rossi, S., Smith, N. G., et al. (2021). Contrasting temporal variations in responses of leaf unfolding to daytime and nighttime warming. Glob. Chang. Biol. 27, 5084–5093. doi: 10.1111/gcb.15777
Wang, J., Zhou, Y., Zhu, L., Gao, M., and Li, Y. (2014). Cultivated land information extraction and gradient analysis for a North-South Transect in Northeast Asia between 2000 and 2010. Remote Sens. 6, 11708–11730. doi: 10.3390/rs61211708
Xiang, Y., Gubian, S., Suomela, B., and Hoeng, J. (2013). Generalized Simulated annealing for global optimization: The GenSA package. R J. 5:13. doi: 10.32614/RJ-2013-002
Yun, K., Hsiao, J., Jung, M.-P., Choi, I.-T., Glenn, D. M., Shim, K.-M., et al. (2017). Can a multi-model ensemble improve phenology predictions for climate change studies? Ecol. Model. 362, 54–64. doi: 10.1016/j.ecolmodel.2017.08.003
Zeng, L., Wardlow, B. D., Xiang, D., Hu, S., and Li, D. (2020). A review of vegetation phenological metrics extraction using time-series, multispectral satellite data. Remote Sens. Environ. 237:111511. doi: 10.1016/j.rse.2019.111511
Zhong, L., Hu, L., and Zhou, H. (2019). Deep learning based multi-temporal crop classification. Remote Sens. Environ. 221, 430–443. doi: 10.1016/j.rse.2018.11.032
Zhou, X., Geng, X., Yin, G., Hänninen, H., Hao, F., Zhang, X., et al. (2020). Legacy effect of spring phenology on vegetation growth in temperate China. Agric. For. Meteorol. 281:107845. doi: 10.1016/j.agrformet.2019.107845
Keywords: spring phenological model, remote sensing, chilling, temperature, photoperiod
Citation: Mo Y, Zhang J, Jiang H and Fu YH (2023) A comparative study of 17 phenological models to predict the start of the growing season. Front. For. Glob. Change 5:1032066. doi: 10.3389/ffgc.2022.1032066
Received: 30 August 2022; Accepted: 14 December 2022;
Published: 05 January 2023.
Edited by:
Nikolaos M. Fyllas, University of the Aegean, GreeceReviewed by:
Shuai An, Beijing Union University, ChinaNan Cong, Institute of Geographic Sciences and Natural Resources Research (CAS), China
Copyright © 2023 Mo, Zhang, Jiang and Fu. This is an open-access article distributed under the terms of the Creative Commons Attribution License (CC BY). The use, distribution or reproduction in other forums is permitted, provided the original author(s) and the copyright owner(s) are credited and that the original publication in this journal is cited, in accordance with accepted academic practice. No use, distribution or reproduction is permitted which does not comply with these terms.
*Correspondence: Yongshuo H. Fu, ✉ yfu@bnu.edu.cn