Wide-Ranging Temporal Variation in Transoceanic Movement and Population Mixing of Bluefin Tuna in the North Atlantic Ocean
- 1Department of Marine Biology, Texas A&M University, Galveston, TX, United States
- 2Department of Wildlife and Fisheries Sciences, Texas A&M University, College Station, TX, United States
- 3Marine Research Division, AZTI, Pasaia, Spain
- 4Regional Centre of Tangier, National Institute of Fisheries Research, Tangier, Morocco
- 5Department of Oceanography and Coastal Sciences, Louisiana State University, Baton Rouge, LA, United States
- 6National Research Institute of Far Seas Fisheries, Japan Fisheries Research and Education Agency, Shizuoka, Japan
- 7Instituto Español de Oceanografía, Centro Oceanográfico de Santander, Santander, Spain
Uncertainty regarding the movement and population exchange of Atlantic bluefin tuna (Thunnus thynnus) from the two primary spawning areas (Gulf of Mexico, Mediterranean Sea) is increasingly implicated as a major impediment for the conservation of this species. Here, two mixture methods were applied to natural chemical markers (δ18O and δ13C) in otoliths (ear stones) to comprehensively investigate the nature and degree of transoceanic movement and mixing of eastern and western populations in several areas of the North Atlantic Ocean that potentially represent mixing hotspots. Areas investigated occurred on both sides of the 45°W management boundary as well as waters off the coast of Africa (Morocco, Canary Islands) where both populations are known to occur. Projections of population composition (i.e., natal or nursery origin) from a multinomial logistic regression (MLR) classification method with different probability thresholds were generally in agreement with maximum likelihood estimates from the commonly used mixed-population program HISEA; however, predicted contributions for the less abundant population were occasionally higher for MLR estimates. Both MLR and HISEA clearly showed that mixing of Atlantic bluefin tuna in the Central North Atlantic Ocean was highly variable from year to year with expatriates of eastern or western origin commonly crossing into the other management area. Pronounced transoceanic movement and mixing of western migrants was also present off the coast of Africa, with the occurrence of western migrants in the Canary Islands and Morocco ranging from zero to the majority of the individuals assayed for the years examined. Results indicate highly variable rates of movement and population exchange for Atlantic bluefin tuna, highlighting the need for temporally resolved estimates of natal origin in mixing hotspots to improve population models used to evaluate the status of this threatened species.
Introduction
Highly migratory pelagic species such as billfishes and tunas are capable of transoceanic crossings that often traverse international borders or jurisdictions (Ortiz et al., 2003; Madigan et al., 2014; Rooker et al., 2016, 2019). Many of these apex predators require multiple ecosystems and/or ocean basins to complete their life cycle (Rooker et al., 2007), with individuals commonly venturing outside of their respective management area (Block et al., 2011; Wilson et al., 2015; Harrison et al., 2018). Although fisheries managers concede that knowledge of a species’ migratory history is fundamental to conserving and rebuilding populations (Kerr and Goethel, 2014), data on the movement and population structure of many large marine predators are insufficient to integrate into assessments. Unfortunately, these data gaps are common for several highly migratory species (e.g., Atlantic and Pacific bluefin tuna [Thunnus thynnus, T. orientalis], yellowfin tuna [T. albacares], blue marlin [Makaira nigricans]), limiting our ability to adequately parameterize population models and develop effective conservation plans (Collette et al., 2011).
Atlantic bluefin tuna is the prototypical highly migratory species with individuals commonly crossing the Atlantic Ocean but also returning to natal areas to spawn (Rooker et al., 2008b, 2014; Graves et al., 2015). It is widely recognized that an improved understanding of the movement and exchange of Atlantic bluefin tuna from the two primary production zones—Gulf of Mexico (western) and Mediterranean Sea (eastern)—is critically needed for management purposes (Porch et al., 2000; Kerr et al., 2017). Chemical markers in otoliths (δ18O and δ13C) have been used to investigate the natal origin of this species throughout the Atlantic Ocean and, previous research indicates that individuals from both production zones readily cross the 45°W management boundary and intermingle with the other population (Rooker et al., 2008a, 2014; Siskey et al., 2016); however, the degree and persistence of population mixing is unresolved in several parts of their range. The composition of Atlantic bluefin tuna on both sides of the management boundary in the Central North Atlantic Ocean (CNAO) is of particular interest to resource managers because of the strong asymmetrical production between the two populations (i.e., an order of magnitude higher in the east; Secor, 2015). Areas in the Eastern North Atlantic Ocean (ENAO) off the coast of Africa also have been identified as putative mixing areas of eastern and western populations using otolith δ18O and δ13C values as indicators of natal origin (Rooker et al., 2014; Fraile et al., 2015); nevertheless, the prevalence and regularity of western-migrants in this region is unresolved.
Even though it is widely recognized that population models for Atlantic bluefin tuna are highly sensitive to mixing of individuals from eastern and western production zones (Kerr et al., 2017), incomplete data on the origin of this species in presumed mixing areas of the CNAO and ENAO has delayed the integration of movement and connectivity data into population assessments. This is due in part to the lack of consensus on the primary locations and extent of population exchange, and also the absence of data regarding the temporal persistence of mixing within these zones. In addition, a variety of statistical approaches, namely mixture models and discriminant functions, have been applied to otolith chemistry data to estimate the contribution of different stocks or nurseries (e.g., Campana et al., 1999; Elsdon and Gillanders, 2003; White et al., 2008; Smith and Campana, 2010; Niklitschek and Darnaude, 2016), and the accuracy and robustness of commonly used approaches for otolith δ18O and δ13C data on Atlantic bluefin tuna have yet to be comprehensively investigated. The aim of the present study was to apply two different types of classification methods—multinomial logical regression (MLR) and conditional maximum likelihood estimation (HISEA)—to otolith chemistry data to assess the origin of Atlantic bluefin tuna in four areas of the CNAO and ENAO. MLR was introduced as an alternative classification method for mixed-stock analysis of Atlantic bluefin tuna based on otolith δ18O and δ13C because model assumptions are more flexible than HISEA. Mixture models were applied to collections made over 4–5 consecutive years in each area to comprehensively assess interannual variability in transatlantic movement and mixing of this species.
Materials and Methods
Sample Collections
Our baseline sample used to predict natal origin was comprised of yearling Atlantic bluefin tuna (approximately 12–18 months old) from eastern and western nurseries collected from 1998 to 2011 from the Mediterranean Sea/Bay of Biscay (eastern) and the Gulf of Mexico/U.S. Atlantic Ocean (western). The baseline sample was first reported in Rooker et al. (2014) and shown to be stable temporally for the primary marker (δ18O) used to determine natal origin. Eastern (Mediterranean Sea) and western (Gulf of Mexico) nurseries included areas proximal to each, including the Bay of Biscay in the east and the eastern seaboard (Mid Atlantic Bight) of the United States in the west. The baseline sample used here is comprehensive in geographic scope and includes yearling Atlantic bluefin tuna from multiple locations in both spawning/nursery areas. Moreover, samples were collected in both nurseries for the majority of years between 1998 and 2011, suggesting the baseline sample effectively accounts for temporal variability and indicates that the extended baseline does not degrade nor bias estimates of nursery origin because differences between eastern and western nurseries are retained even when samples were drawn from different years. Yearling Atlantic bluefin tuna were collected with a variety of fishing gears including baitboats, hook and line, lighted purse seines, and longlines.
The current assessment of population mixing is based on sub-adult and adult Atlantic bluefin tuna collected from 2010 to 2016 on both sides of the management boundary in the CNAO and two locations in the ENAO where Rooker et al. (2014) detected western migrants: Morocco and Canary Islands (Figure 1). All collections were made under the provision of the ICCAT Atlantic Wide Research Program for Bluefin Tuna, and samples of Atlantic bluefin tuna from all areas were comprised of medium and large size category fish (25–100 kg and >100 kg, respectively), with the majority of samples from each area and year comprised principally of individuals near or over 100 kg; hereafter both size categories are referred to collectively as adults. All areas included samples of Atlantic bluefin tuna from 4 to 5 consecutive years to evaluate interannual variability in population mixing. While the current study was based predominantly on new data for Atlantic bluefin tuna collected from 2012 to 2016, otolith chemistry data for individuals collected between 2010 and 2011 from a previous study (Rooker et al., 2014) were included in the analysis to increase the extent of our time series. Estimates of natal origin were determined by comparing δ18O and δ13C values in the otolith cores of adults (core material represents otolith material deposited during the yearling period) to δ18O and δ13C values from otoliths of yearlings (i.e., baseline sample).
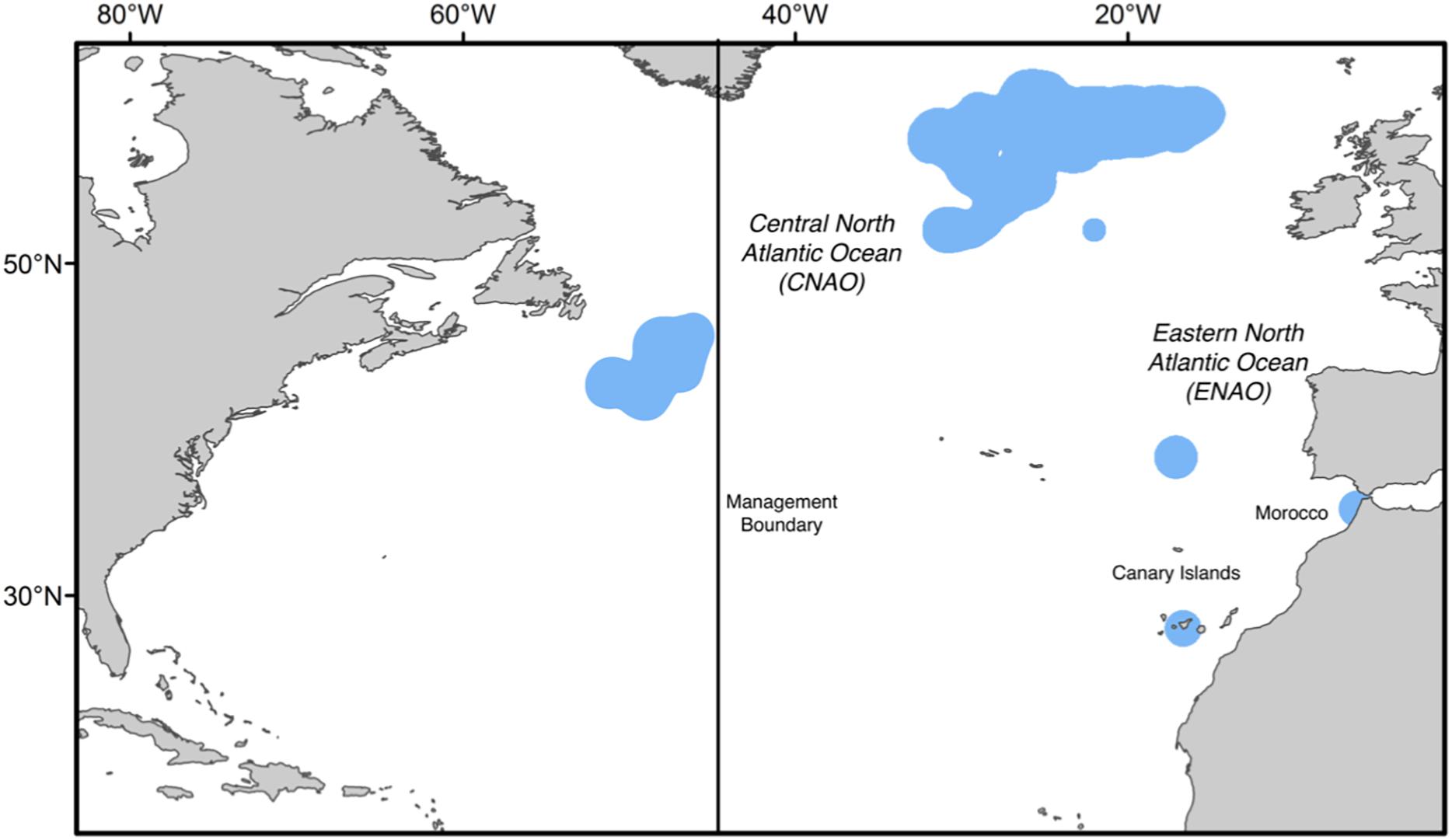
Figure 1. Summary of the four sampling areas (blue shading) for Atlantic bluefin tuna (Thunnus thynnus). Collections included adult Atlantic bluefin tuna from both sides of the management boundary (45°W longitude) in the Central North Atlantic Ocean (CNAO) and two locations in the Eastern North Atlantic Ocean (ENAO): Morocco and Canary Islands. Sampling conducted from 2010 to 2016.
Otolith Processing and Chemical Analysis
For chemical analysis, one sagittal otolith from each Atlantic bluefin tuna was cleaned and rinsed following Rooker et al. (2016). Otoliths were then embedded in Struers epoxy resin (Struers A/S, Ballerup, Denmark) and a 1.5 mm thick section was cut along a transverse plane using a Buehler IsoMet saw (Beuhler, Lake Bluff, Illinois). Sections were then attached to a sample plate using Crystalbond thermoplastic glue (SPI Supplies/Structure Probe Inc., West Chester, Pennsylvania), and the region of the otolith corresponding to the first year of life was isolated following Rooker et al. (2008a). Approximately 1.0–1.5 mg of powder was collected during the milling of each otolith and a fraction of the powder was loaded into sample trays for stable isotope analysis. Otolith δ18O and δ13C was determined using an automated carbonate preparation device (KIEL-III; Thermo Fisher Scientific, Inc., Waltham, Mass.) coupled to a gas chromatograph – isotope ratio mass spectrometer (Finnigan MAT 252; Thermo Fisher Scientific, Inc.) at the University of Arizona. Powdered otolith samples (ca. 0.5 μg) were reacted with dehydrated phosphoric acid under vacuum at 70°C. The isotope ratio measurement was calibrated based on repeated measurements of National Bureau of Standards (NBS), NBS-19 and NBS-18, with 6 standards ran for every 40 samples; precision was ± 0.08‰ (SD) and ± 0.11‰ (SD) for δ18O and δ13C, respectively. Otolith δ18O and δ13C values are reported relative to the Vienna Pee Dee Belemnite (VPDB) scale after comparison to an in-house laboratory standard calibrated to VPDB.
Classification Methods
The stock composition program HISEA is a commonly used statistical method for estimating the proportion of populations or stocks in a sample (Millar, 19901), and this approach has been used extensively for assignments of Atlantic bluefin tuna to eastern and western populations (e.g., Schloesser et al., 2010; Rooker et al., 2014; Fraile et al., 2015; Secor et al., 2015; Siskey et al., 2016). Direct maximum likelihood estimation of population composition in HISEA has two key assumptions. First, it assumes that individuals used to establish the baseline are randomly sampled and representative of each population in the fishery being investigated (Millar, 1987, 1990). Second, it assumes the probability of randomly selecting an individual from a mixed sample with measured characteristics (i.e., isotopic signature) is known from the baseline. As a result, the baseline sample must be representative of all individuals of each population within a mixed sample. For highly migratory species such as Atlantic bluefin tuna, baseline data are normally sampled opportunistically and/or intermittently from a variety of nursery locations, and mixing zones comprised of individuals of unknown origin are often far removed from spawning and/or natal sites. Therefore, individuals of unknown origin in a mixed sample may not be accurately estimated from the baseline, possibly affecting the efficacy of this method for delineating the composition of Atlantic bluefin tuna in areas where populations intermingle.
The MLR is a type of classification method that is increasingly used in machine learning, psychology, and economics (Hosmer et al., 2013). This modeling framework also shows promise for estimating contribution rates in mixed-population fisheries and this study is the first to apply this approach in this manner. This MLR framework allows for estimating the probability of occurrence of each category (i.e., population) as a function of explanatory variables (i.e., δ18O, δ13C) in question. While model parameters in MLR are also estimated using a maximum likelihood estimator, MLR is fundamentally different than HISEA in that it does not directly estimate the proportion of each population and does not assume a classification matrix where the probability of individuals being randomly selected from a specific population in the mixed sample is known from the baseline. In fact, MLR predicts the probability for individuals randomly sampled from the mixed sample and assigns them to a category and then estimates the proportions of each population. For each population in MLR, the response variable follows a multinomial distribution and the probability of each category of the dependent variable is predicted based on information from explanatory variables included in the model. MLR assumes (1) independence among the dependent variable choices, that is the choice of one category is not related to the choice of another category (i.e., the dependent variable), (2) non-perfect separation, that is the dependent variable cannot be perfectly predicted from the independent variables, and (3) multicollinearity of independent variables is relatively low. One potential advantage of MLR is that it can be applied to a mixed sample that may not include all potential sources or nurseries by establishing probability thresholds that assign individuals with lower predicted probabilities to an undetermined source that may not be represented in the baseline. Classification in MLR is based on a vector of conditional probability, and here we applied a series of probability thresholds for positive predictions with all modeling performed in R (R Core Team, 2018).
For all MLR and HISEA mixture models, the degree of uncertainty around estimated proportions was estimated with bootstrapping (500 simulations; error term equivalent to ± 1 SD around estimated proportion). MLR-based predictions were derived for four probability thresholds: 0.5, 0.6, 0.7, and 0.8. A probability threshold of 0.5—which was the only probability also used for HISEA—indicates that when an individual’s predicted probability for the western baseline sample is above 0.5, it will be classified to the western population. As the probability threshold increases above 0.5, some individuals do not have large enough predicted probabilities to be classified to either the eastern or western population. For such cases, they are assigned to an undetermined source or nursery, and the fraction of individuals failing to meet the probability threshold of either population will increase as the probability threshold increases (highest for 0.8).
Results
Otolith δ18O and δ13C of yearling Atlantic bluefin tuna from eastern and western nurseries from Rooker et al. (2014) were distinct for year classes investigated (MANOVA, p < 0.01) and indicated that these markers can be used to determine an individuals’ place of origin. Otolith δ18O of eastern yearlings were consistently heavier (less negative) than western yearlings and a demarcation at −0.9%, although some degree of overlap occurred for this marker from approximately −1.1 to −0.8 (Figure 2). Despite the overlap in otolith δ18O and a slight separation of otolith δ13C for eastern and western yearlings, cross-validated classification success remained relatively high (QDFA = 87%), supporting the use of otolith δ18O and δ13C as a baseline (reference signatures) for determining the origin of Atlantic bluefin tuna from presumed mixing zones in the CNAO and ENAO.
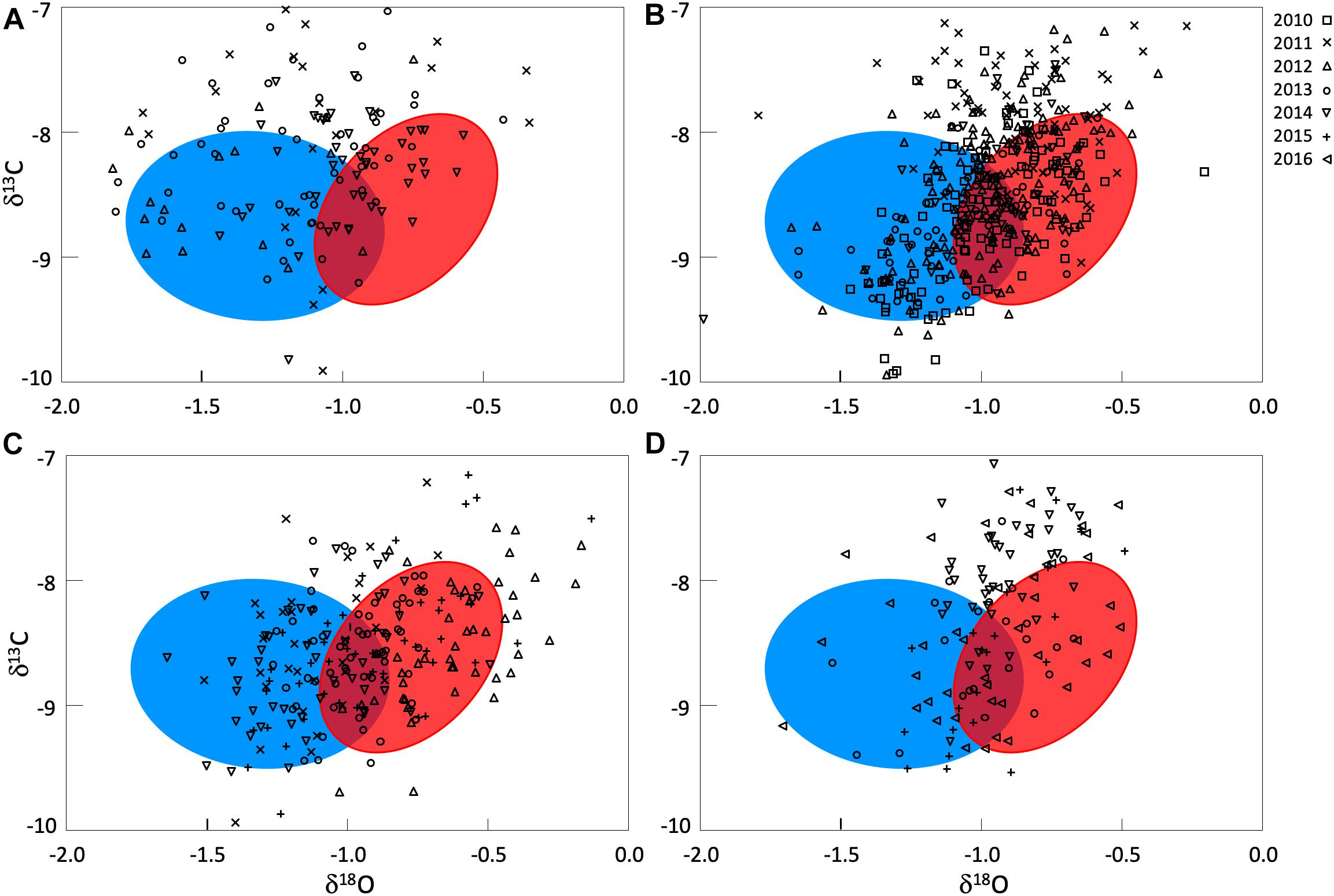
Figure 2. Otolith δ18O and δ13C values for Atlantic bluefin tuna of unknown origin collected from four locations from 2010 to 2016: (A) Central North Atlantic Ocean- West of 45°W, (B) Central North Atlantic Ocean- East of 45°W, (C) Eastern North Atlantic Ocean off Morocco, (D) Eastern North Atlantic Ocean off Canary Islands. Confidence ellipses shown are based on otolith δ18O and δ13C values of yearling Atlantic bluefin tuna (baseline) and represent 1 SD of the western (blue) and eastern (red) sample. Otolith δ18O and δ13C values of yearlings used to create confidence ellipses and for mixed-stock analysis from Rooker et al. (2014).
Central North Atlantic Ocean
Otolith core δ18O and δ13C values of adult Atlantic bluefin tuna run in HISEA and MLR modeling frameworks indicated that individuals collected in the CNAO readily crossed the 45°W management boundary with expatriates being relatively common both east and west of the boundary (Figure 2). For both HISEA and MLR (all probability thresholds) based assignments, the majority of adults collected west of 45°W from 2011 to 2014 were assigned to the western population, although contribution rates varied widely among years and mixture models (Figure 3). For HISEA, Atlantic bluefin tuna of western origin accounted for 13% to 96% of the samples collected west of 45°W over the four years investigated (Table 1). Although findings from MLR models were generally in agreement with HISEA, MLR-derived estimates of western contribution were noticeably reduced in 2012 across all probability thresholds. Conversely, the proportion of western migrants west of 45°W in the 2014 sample was greater for all MLR models relative to HISEA.
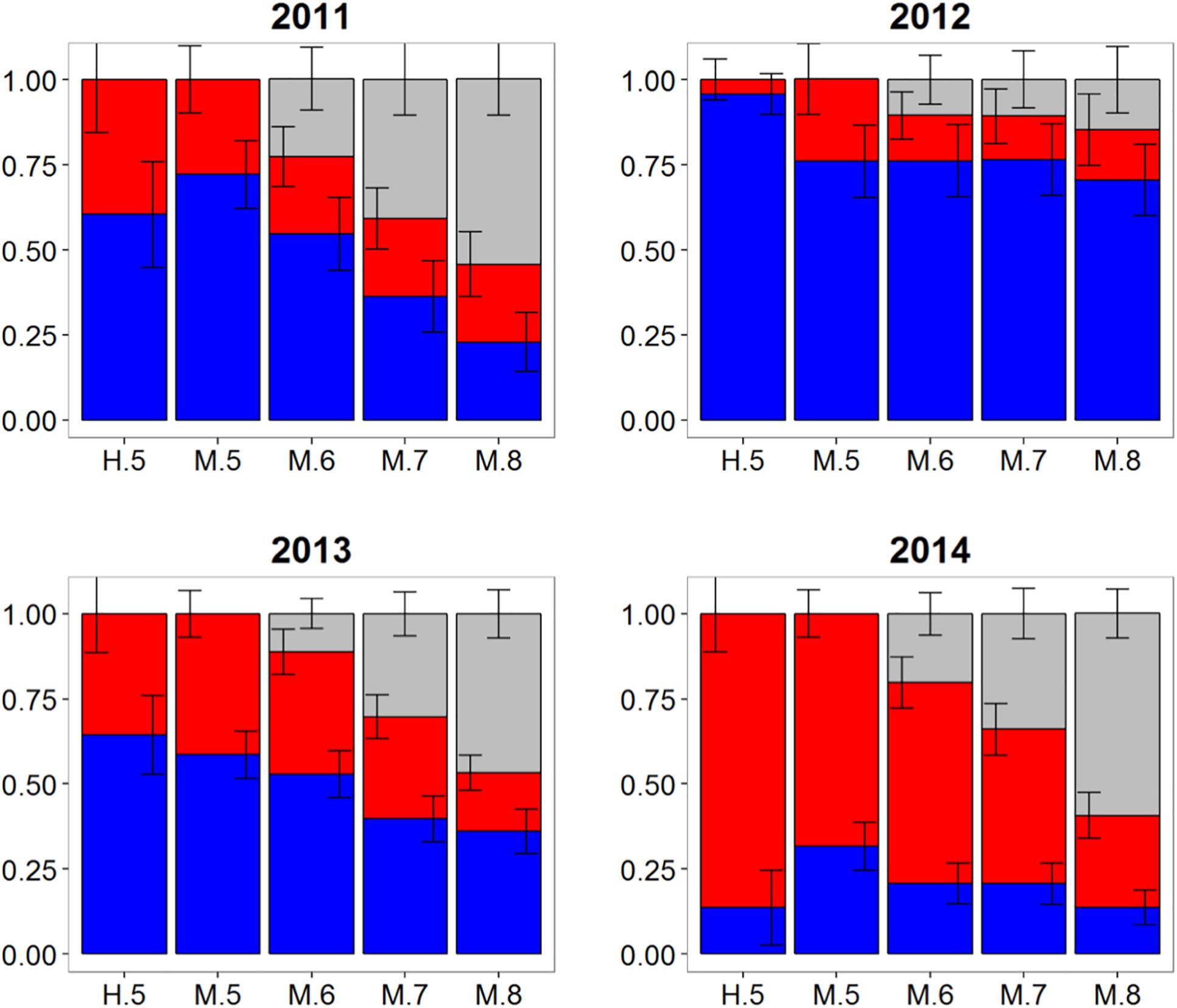
Figure 3. Estimated proportions of western (blue), eastern (red), and undetermined (gray) Atlantic bluefin tuna in samples collected from 2011 to 2014 in the Central North Atlantic Ocean—West of 45°W. Results are based on mixed-population analysis using HISEA and multinomial logistic regression (MLR) frameworks. H.5 denotes predictions from HISEA while MLR estimates are shown as M.5, M.6, M.7, M.8 to represent the four different probability thresholds (0.5–0.8). Uncertainty around predictions shown with error bars which represent 1 ± SD around estimated proportion.
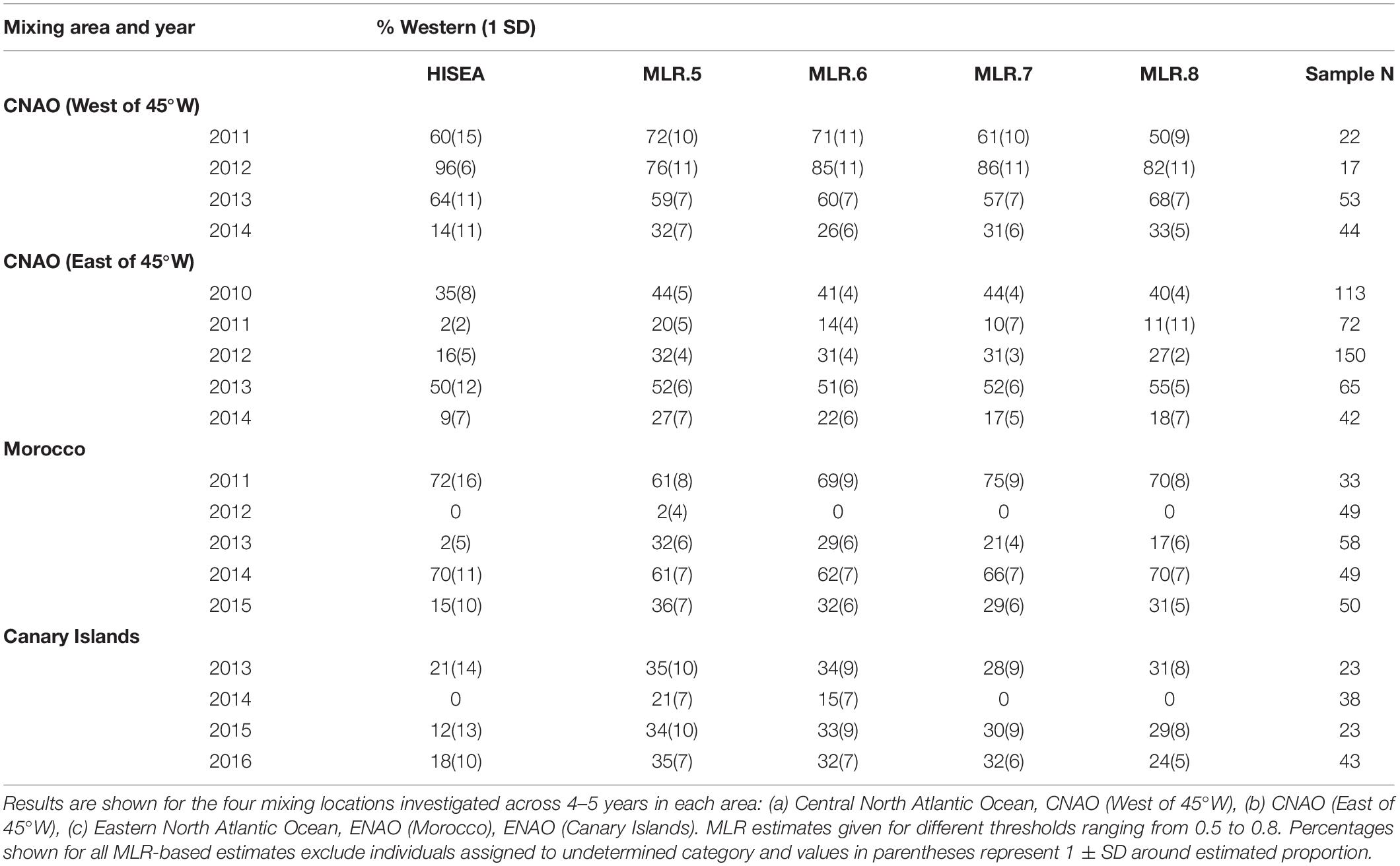
Table 1. Estimated proportion of “western migrants” detected in samples of Atlantic bluefin tuna from HISEA and multinomial logistic regression (MLR) models.
Wide-ranging interannual variability in otolith core δ18O and δ13C values was observed for Atlantic bluefin tuna collected east of 45°W in the CNAO (Figure 2), with contributions of western migrants variable across the years investigated. The presence of western migrants in this mixing zone was generally higher for MLR (all probability thresholds) relative to HISEA (Figure 4). For samples collected from 2011 to 2015, HISEA indicated that Atlantic bluefin tuna of western origin were consistently in the minority east of 45°W, often accounting for less than 10% of the sample in certain years (2011, 2014) (Table 1); however, nearly half of the sample from 2013 was determined to be of western origin, suggesting that large numbers of western migrants may occasionally enter the eastern management zone. MLR models showed less year-to-year variability than HISEA in the population composition of Atlantic bluefin tuna but also highlight the potential for meaningful temporal shifts in the population structure within this mixing zone. MLR (0.5 probability) showed the lowest amount of variability among the 5 years investigated with western contribution ranging from 21 to 52% (mean: 35%). Increasing MLR probability led to a greater proportion of samples assigned to the undetermined category (Figure 4) and generally resulted in minor reductions in western contribution rates (ca. < 5%).
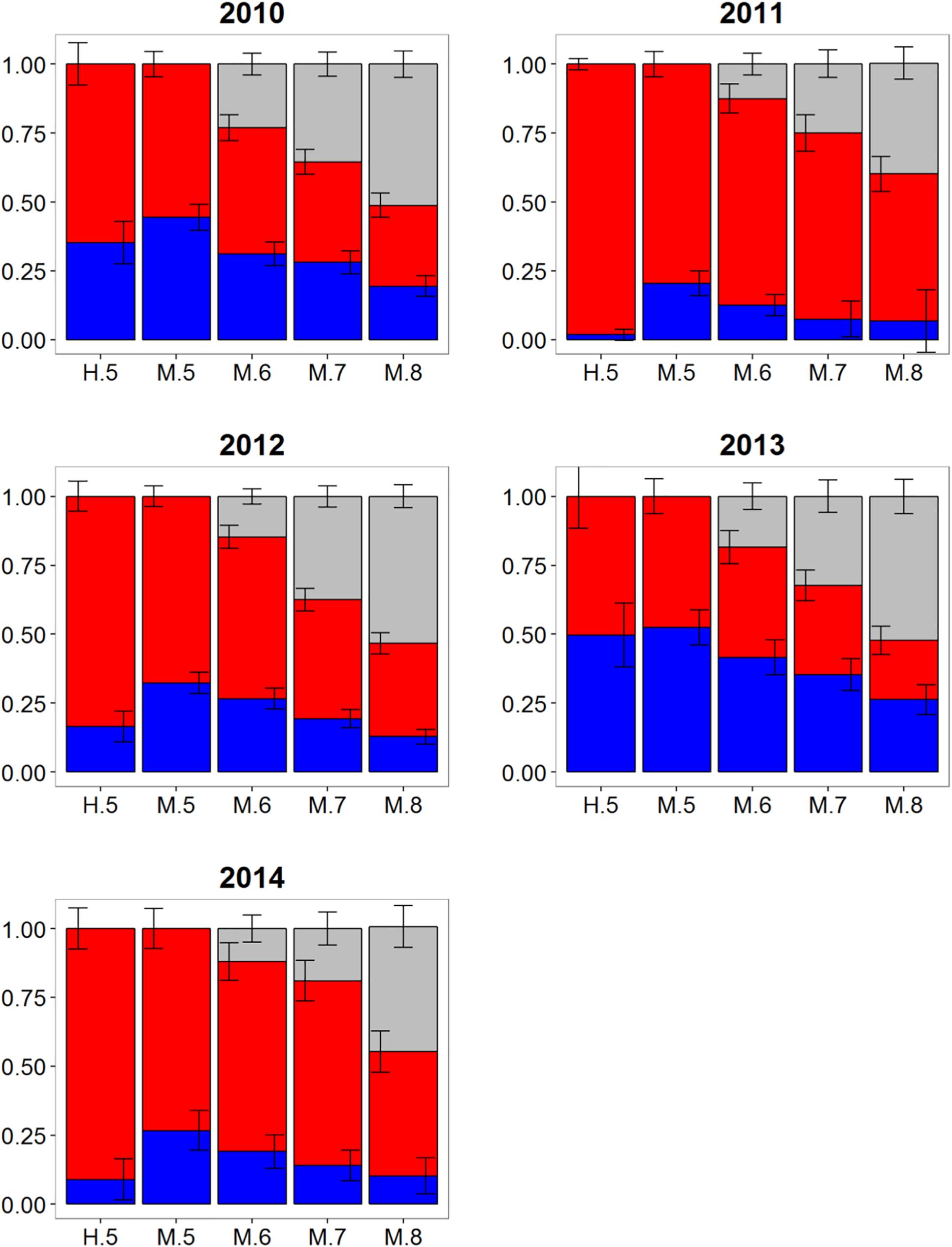
Figure 4. Estimated proportions of western (blue), eastern (red), and undetermined (gray) Atlantic bluefin tuna in samples collected from 2010 to 2014 in the Central North Atlantic Ocean—East of 45°W. Results are based on mixed-population analysis using HISEA and multinomial logistic regression (MLR) frameworks. H.5 denotes predictions from HISEA while MLR estimates are shown as M.5, M.6, M.7, M.8 to represent the four different probability thresholds (0.5–0.8). Uncertainty around predictions shown with error bars which represent 1 ± SD around estimated proportion.
Eastern North Atlantic Ocean
Atlantic bluefin tuna of western origin were clearly evident in the ENAO, and these transatlantic migrants were present in multiple years in the Moroccan trap fishery, possibly indicating that these waters occur within a common migratory pathway of the western population (Figure 2). Of particular note was highly variable mixing of western Atlantic bluefin tuna in Morocco, with all mixture models showing fluctuating rates and the strong contribution of western migrants was only observed in two of the 5 years examined (Figure 5). In both 2011 and 2014, approximately 60–75% of the sample from Morocco was predicted to be of western origin, and this finding was consistent between HISEA and among all probability thresholds in MLR models (Table 1). Interestingly, the presence of western migrants in the Moroccan sample for the other 3 years investigated was negligible (0 to 2%; 2012, 2013) or minor (15%; 2015) in HISEA. Population contributions from MLR models were similar to HISEA for the 2012 sample; however, the proportion of western migrants was consistently higher (ca. 15–30% higher in MLR models for 2013 and 2015 samples), signifying that MLR may elevate the contribution of the less abundant, western population in this mixing zone.
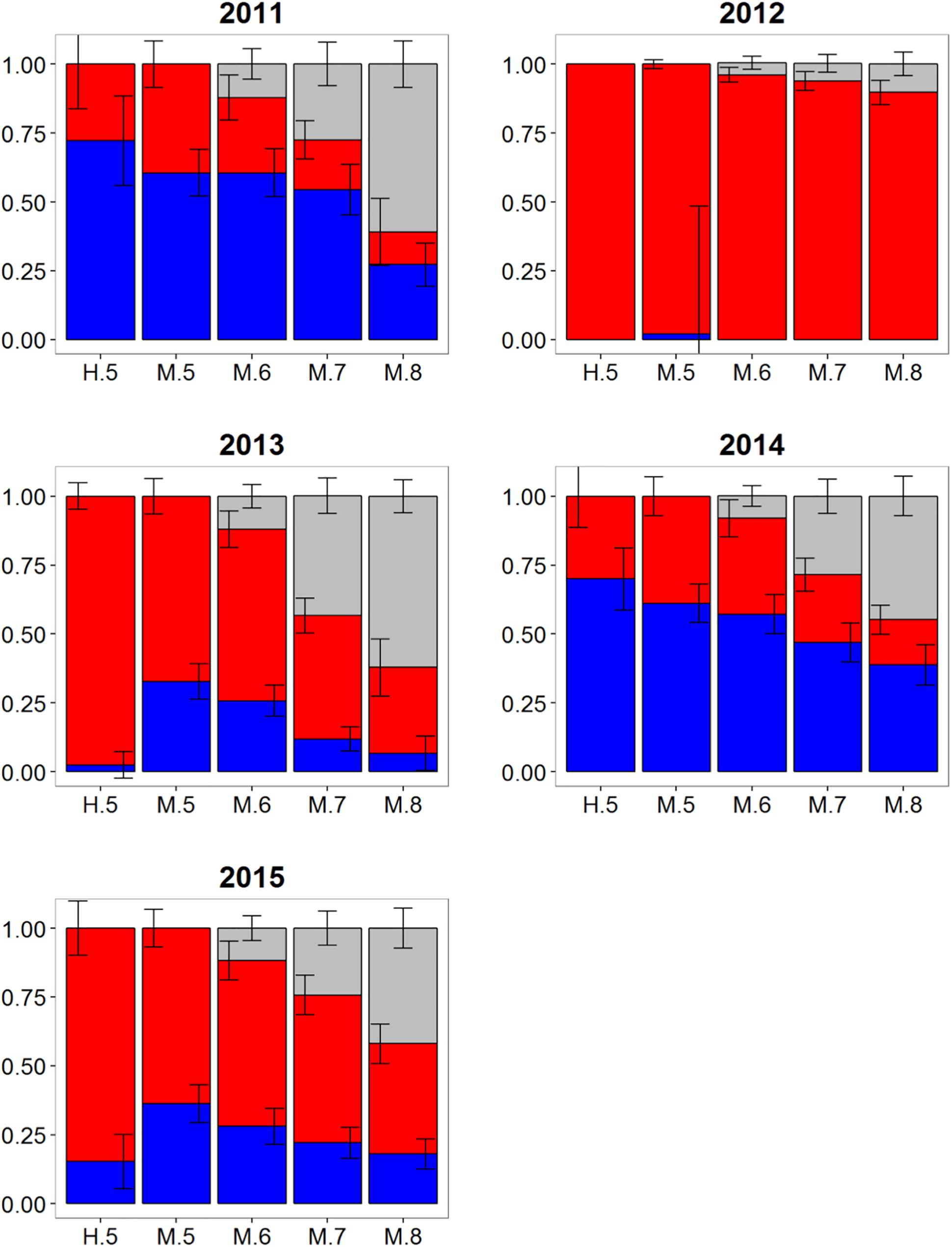
Figure 5. Estimated proportions of western (blue), eastern (red), and undetermined (gray) Atlantic bluefin tuna in samples collected from 2011 to 2015 off Morocco. Results are based on mixed-population analysis using HISEA and multinomial logistic regression (MLR) frameworks. H.5 denotes predictions from HISEA while MLR estimates are shown as M.5, M.6, M.7, M.8 to represent the four different probability thresholds (0.5–0.8). Uncertainty around predictions shown with error bars which represent 1 ± SD around estimated proportion.
Population mixing of Atlantic bluefin tuna in waters off the Canary Islands also varied temporally with consistent but low contributions of the western population to this fishery in three of the 4 years investigated (Figure 6). In 2013, 2015, and 2016 samples, the western contribution from HISEA ranged from 12 to 21%. Again, MLR estimates for the same years at most probability thresholds were consistently 10–20% higher than HISEA, often with approximately a third of the sample attributed to the western nursery (Table 1). No western migrants were detected in the 2014 sample from the Canary Islands for HISEA or for MLR at probability thresholds of 0.7 and 0.8, although western fish contributed marginally at probability thresholds of 0.5 and 0.6.
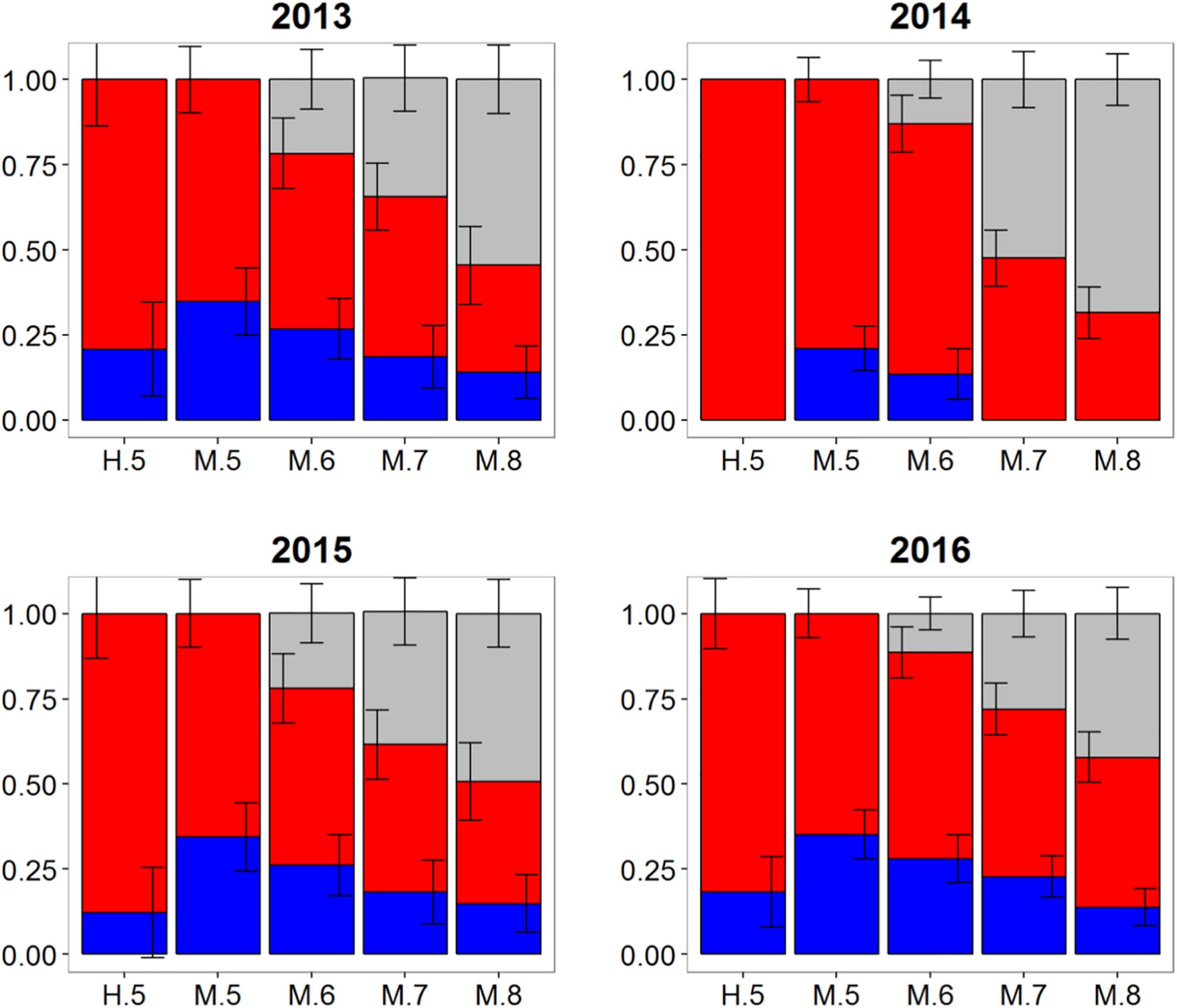
Figure 6. Estimated proportions of western (blue), eastern (red), and undetermined (gray) Atlantic bluefin tuna in samples collected from 2013 to 2016 in the Canary Islands. Results are based on mixed-population analysis using HISEA and multinomial logistic regression (MLR) frameworks. H.5 denotes predictions from HISEA while MLR estimates are shown as M.5, M.6, M.7, M.8 to represent the four different probability thresholds (0.5–0.8). Uncertainty around predictions shown with error bars which represent 1 ± SD around estimated proportion.
Discussion
The current study applied well-established chemical markers in otoliths to significantly expand our understanding of the movement and mixing of Atlantic bluefin tuna in areas identified as potentially important mixing hotspots. As documented in previous studies, otolith δ18O was primarily responsible for discriminating individuals from eastern and western nurseries (Rooker et al., 2008a, 2008b). Nursery-specific differences in chemical signatures of yearlings followed expected dissimilarities in seawater δ18O between eastern (Mediterranean Sea) and western (Gulf of Mexico/U.S. Atlantic) nurseries (LeGrande and Schmidt, 2006), with yearlings from the Mediterranean Sea displaying heavier otolith δ18O values than their western counterparts (Rooker et al., 2014).
Regardless of the modeling framework applied to δ18O and δ13C values derived from the milled otolith cores of adult Atlantic bluefin tuna, results from this study clearly showed that the 45°W management boundary that divides the two populations is readily crossed and high levels of mixing occur on both sides of this longitudinal demarcation. Our findings are in accord with earlier research using otolith δ18O and δ13C to demonstrate that both eastern and western migrants commonly ‘cross the line’ (Rooker et al., 2008b, 2014), and further corroborate an earlier finding that aggregations targeted by high seas longline fleets in the CNAO are consistently comprised of both populations on each side of the management boundary (Rooker et al., 2014). In response, there does not appear to be a biological basis for the construct of the current boundary used to delineate eastern and western populations, and persistent, high levels of mixing observed in both management zones in the CNAO further emphasize the importance of east-west exchange.
The presence of western migrants off Morocco and the Canary Islands in the ENAO indicates that movement extends beyond the CNAO, with individuals completing oceanic crossings and entering waters off the coast of Africa. Transoceanic migrations by Atlantic bluefin tuna have been previously reported using chemical markers in otoliths (Rooker et al., 2008b, 2014; Secor et al., 2015; Siskey et al., 2016), organochlorine tracers (Dickhut et al., 2009; Graves et al., 2015), and electronic tags (Block et al., 2005; Kurota et al., 2009; Galuardi et al., 2010). However, crossing of western migrants into the ENAO is generally assumed to be very low and expatriates from the west are even more rare inside the Mediterranean Sea. Rooker et al. (2014) evaluated the origin of Atlantic bluefin tuna from the ENAO and detected no western migrants from collections west of the Strait of Gibraltar in waters off Portugal; however, a small fraction of individuals from a trap fishery off Morocco was reported to be comprised of western migrants (6%). Similarly, Rodríguez-Ezpeleta et al. (in press) documented western migrants in fisheries from several areas of the ENAO including Morocco and the Canary Islands using single nucleotide polymorphism (SNP) markers. Still, western contribution estimates from otolith δ18O and δ13C were generally higher than genetic assignments, particularly for MLR-based estimates, possibly leading to overestimates of western contribution. In addition, Fraile et al. (2015) examined samples collected over 3 years from the bait boat fleet in the Bay of Biscay, and western migrants were only detected in a single year and at relatively low levels for juveniles and adults (2–5%). Here, we advance our understanding of transoceanic movements of Atlantic bluefin tuna by demonstrating that western migrants commonly enter waters off the coast of Africa year after year and mix with the eastern population. Both HISEA and MLR based estimates indicated that significant numbers of western migrants enter waters off Morocco (e.g., 2011 and 2014) and to a lesser degree the Canary Islands; nevertheless, it is important to note that observed contribution estimates may not represent the precise population composition in either fishery because samples sizes were limited in certain years and occasionally from abbreviated collection periods. In addition, early egress out of the Mediterranean Sea and into the North Atlantic Ocean by age-0 Atlantic bluefin tuna may produce chemical signatures that bear some resemblance to the western baseline (e.g., more depleted otolith δ18O), potentially overstating the contribution of western migrants in samples from Morocco and the Canary Islands.
It is also important to note that western migrants were detected in all 3 years with samples available from both Morocco and the Canary Islands regardless of the modeling framework used. Given the general proximity of both locations in the ENAO, it is possible that western migrants detected in these two locations were the result of shared migration pathway(s) across the Atlantic Ocean. Common transoceanic or inter-oceanic migration routes have been reported for other highly migratory species including southern bluefin (T. maccoyii), Pacific bluefin tuna (T. orientalis), and several other large marine vertebrates (Boustany et al., 2010; Block et al., 2011; Hobday et al., 2015). Shared population-specific migration routes across ocean basins are often correlated with dynamic ocean features, particularly biological and/or physicochemical fronts such as the Transition Zone Chlorophyll Front (TZCF). This feature is assumed to define the migration routes of highly migratory species such as tunas, sea turtles, and squid that are known to forage along this basin-wide frontal feature in the Pacific Ocean (Polovina et al., 2017) and similar dynamic features in the Atlantic Ocean (e.g., subtropical gyre front) may represent ecological bridges (sensu Briscoe et al., 2017), leading to common migration routes for Atlantic bluefin tuna to waters off Morocco and the Canary Islands.
Spatial shifts in the distribution of marine fishes occurs at multiple spatial scales ranging from small-scale habitat shifts (Moulton et al., 2017; Landry et al., 2018; Rooker et al., 2018) to large-scale journeys across ocean basins (Galuardi et al., 2010; Block et al., 2011; Kraus et al., 2011). Migratory patterns of pelagic fishes and other marine vertebrates at the basin scale (i.e., transoceanic) are often influenced by both intrinsic and external factors, including spatial shifts in prey quantity/quality, thermal tolerance/sea surface temperatures, and other incentives related to reproduction and/or energetics (Secor, 2015). In response, it is plausible to assume that movements across boundaries or oceans reported here for Atlantic bluefin tuna are physiologically and/or ecologically motivated behaviors to locate favorable physicochemical conditions and optimize foraging. Even though oceanic conditions or features are inherently dynamic in nature and known to alter the timing and extent of movements of pelagic fishes (Block et al., 2011; Sequeira et al., 2018), the common occurrence of western migrants in the mixing zones investigated suggests that this species may exploit certain areas of the CNAO and ENAO in fairly predictable ways and share common drivers that initiate these movements.
Significant temporal variation in the proportion of eastern and western migrants in the mixing zones investigated indicates that basin-scale movements of Atlantic bluefin tuna are highly variable from year to year, and our findings are in accord with earlier studies that revealed the potential for interannual changes in large-scale movements of this species. Sibert et al. (2006) contrasted movement parameters of Atlantic bluefin tuna with satellite tags and reported strong interannual variation in movement parameters of this species, albeit their assessment was based on a limited number of specimens and tagging years. Mather (1980) also reported shifts in transoceanic migration behaviors for different size classes of Atlantic bluefin tuna and hypothesized that changes in migration patterns were linked to ocean-scale events. Temporal shifts in the distribution and migration of pelagic fishes have been related to large-scale oceanic phenomena such as the Atlantic Multidecadal Oscillation (AMO) and El Niño–Southern Oscillation (ENSO), which alter the physical environment (e.g., sea surface temperatures, upwelling, circulation) and primary/secondary productivity, often times leading to changes in prey availability, the distribution of predators, and recruitment success (Otterson et al., 2001; Stenseth et al., 2002; Harford et al., 2017). While AMO is generally considered to fluctuate at the decadal scale, interannual variability is also associated with this phenomenon (Enfield et al., 2001). Alheit et al. (2014) observed that the migration and population dynamics of small pelagic fishes (i.e., forage fish of tunas) varied in synchrony with different AMO phases, and recently Faillettaz et al. (2019) demonstrated that AMO phases also influence the basin-scale distribution of bluefin tuna in the North Atlantic Ocean. Therefore, interannual changes in the AMO index during the years investigated (2011–20162) or other large-scale oceanic/weather phenomena that alter environmental conditions may have contributed in part to changes in movement and population mixing of Atlantic bluefin tuna observed in the CNAO and ENAO.
It is also possible that year to year variation in the movement and exchange of Atlantic bluefin tuna was due in part to random exploratory and/or physiologically motivated movements to optimize feeding (e.g., dynamic optimal foraging theory) or energetics, which may or may not be linked to ocean-scale events described above (Giuggioli and Bartumeus, 2010; Hays et al., 2016; Thygesen et al., 2016). Optimal foraging theory predicts that movements by this species should occur in a manner to most efficiently use resources (i.e., prey) that are inherently spatially heterogeneous throughout the North Atlantic Ocean. Mariani et al. (2016) demonstrated the link to foraging for Atlantic bluefin tuna using a game theoretical approach and reported that migrants benefit from highly productive environments with individuals often visiting a series of foraging hotspots connected by a series of migratory pathways. Similarly, energy intake and the spatial distribution of the forage base was shown to influence migration patterns for the Pacific congener T. orientalis; however, movements of this species were not always consistent with maximizing energy and physiological constraints linked to water temperature were also important drivers of movement—both in its timing and degree (Whitlock et al., 2015).
The development and application of numerical approaches to improve our understanding of the movement and exchange of eastern and western populations of Atlantic bluefin tuna is critical to the management of this species. To date, a variety of mixture models have been applied to genetic and chemical markers to predict the contribution of eastern and western recruits to different fisheries in the Atlantic Ocean (e.g., Rooker et al., 2014; Fraile et al., 2015; Puncher et al., 2018). Here, we show that a commonly applied mixture model (HISEA) provides comparable estimates to a new MLR-based approach; however, MLR-derived proportions of western migrants east of the management boundary and into the ENAO were occasionally higher than estimates from HISEA, particularly at the 0.5 probability threshold. This suggests that MLR identified more of the less dominant population in these mixing zones, which may lead to under- (HISEA) or over-estimates (MLR) of western contribution depending upon the modeling framework being adopted. Hanke et al. (2016) applied linear discriminant, quadratic discriminant (equivalent to direct MLE in HISEA), and randomForest classifiers to assess mixed samples of this species in U.S. Atlantic fisheries, and the authors noted that predictions of natal origin were comparable between the latter two classifiers, with both classifying fewer fish from the less abundant population—in this case eastern Atlantic bluefin tuna —in a mixed sample. While results from Hanke et al. (2016) indicated that conditional MLE (i.e., HISEA) and randomForest classifiers produced comparable estimates, it again raises the question of whether mixed-population approaches commonly used accurately represent the proportion of less abundant population(s) in a mixed sample. Here, the contribution of western migrants or the less dominant population in a mixed sample was often higher for MLR-based classifications for samples from Morocco and the Canary Islands. Moreover, this result did not change meaningfully for MLR models with higher probability thresholds (i.e., less uncertainty in eastern or western assignment), indicating that HISEA may underestimate the contribution of the western population in mixed samples in the ENAO. Increasing probability thresholds in MLR also led to another interesting observation; namely, a significant fraction of the sample was characterized as undetermined and not assigned to either the eastern or western population. While this may be a function of natural overlap in otolith δ18O and δ13C values for yearlings from eastern and western nurseries, it may also indicate that individuals from outside sources or nurseries may be present in the mixed samples examined.
An improved understanding of the migration and mixing of western migrants into the eastern management zone has important implications because of the depressed abundance and recruitment of the western population (Standing Committee on Research and Statistics [SCRS], 2017). The sustained presence of Atlantic bluefin tuna from the smaller, western population in waters east of the management boundary intensifies the fishing pressure on this vulnerable population, and therefore highlights the need for spatially and temporally resolved estimates of population composition throughout their range. Natural chemical markers in otoliths were used here to elucidate the nature and extent of population exchange in mixing hotspots in the Atlantic Ocean, and extensive and persistent east-west exchange observed here emphasizes the need to further refine and incorporate mixing into future assessments. We also provide contribution estimates from two useful mixed-population frameworks (HISEA and MLR) with a series of probability thresholds for the latter to better understand the range of conceivable mixing scenarios. In turn, this can then be used in virtual population analysis and simulation modeling to explore sensitivity of yields and population dynamics against different mixing scenarios (Kerr et al., 2017; Morse et al., 2018). However, it is important to note that an improved understanding of population mixing and the environmental drivers responsible for trans-Atlantic movement will require more comprehensive time-space coverage of collections that accurately represent the population composition in a given area. Perceptions of stock mixing from otolith chemistry and other natural markers (genetics) are almost certainly biased to some degree by sampling limitations (e.g., collections restricted in time and space from a region) that only provide a “snapshot” of the stock composition, which may not accurately reflect east-west exchange.
Data Availability
Data supporting the conclusions of this manuscript will be made available by the authors, without undue reservation, to any qualified researcher.
Author Contributions
JR, IF, NA, TI, AK, YT, ER-M, and HA contributed to the design and collection of the biological samples. JR, HL, IF, and MD processed and analyzed the data, and performed the mixed-stock analyses. JR wrote the manuscript.
Funding
This study was funded in part by the European Community (Grant SI2/542789), Canada, Croatia, Japan, Norway, Turkey, United States (NMFS NA11NMF4720107), Chinese Taipei, and the ICCAT Secretariat. The study was also supported in part by the McDaniel Charitable Foundation and by grant CTM2011-27505 from the Spanish Ministry of Economy and Competitiveness (MINECO).
Conflict of Interest Statement
The authors declare that the research was conducted in the absence of any commercial or financial relationships that could be construed as a potential conflict of interest.
Acknowledgments
This work was carried out under the provision of the ICCAT Atlantic Wide Research Program for Bluefin Tuna (GBYP). The contents of this manuscript do not necessarily reflect the point of view of ICCAT or of the other funders.
Footnotes
- ^ https://www.stat.auckland.ac.nz/~millar/mixedstock/code.html
- ^ https://www.esrl.noaa.gov/psd/data/correlation/amon.us.data
References
Alheit, J., Licandro, P., Coombs, S., Garcia, A., Giráldez, A., Garcia Santamaría, M. T., et al. (2014). Atlantic Multidecadal Oscillation (AMO) modulates dynamics of small pelagic fishes and ecosystem regime shifts in the eastern North and Central Atlantic. J. Mar. Sys. 131, 21–35. doi: 10.1016/j.jmarsys.2013.11.002
Block, B. A., Jonsen, I. D., Jorgensen, S. J., Winship, A. J., Shaffer, S. A., Bograd, S. J., et al. (2011). Tracking apex marine predator movements in a dynamic ocean. Nature 475, 86–90. doi: 10.1038/nature10082
Block, B. A., Teo, S. L. H., Walli, A., Boustany, A., Stokesbury, M. J. W., Farwell, C. J., et al. (2005). Electronic tagging and population structure of Atlantic bluefin tuna. Nature 434, 1121–1127. doi: 10.1038/nature03463
Boustany, A., Matteson, M. R., Castleton, M., Farwell, C., and Block, B. A. (2010). Movements of Pacific bluefin tuna (Thunnus orientalis) in the Eastern North Pacific revealed with archival tags. Prog. Oceanogr. 86, 94–104. doi: 10.1016/j.pocean.2010.04.015
Briscoe, D. K., Hobday, A. J., Carlisle, A., Scales, K., Eveson, J. P., Arrizabalaga, H., et al. (2017). Ecological bridges and barriers in pelagic ecosystems. Deep Sea Res. Part II 140, 182–192. doi: 10.1016/j.dsr2.2016.11.004
Campana, S. E., Chouinard, G. A., Hanson, J. M., and Fréchet, A. (1999). Mixing and migration of overwintering cod stocks near the mouth of the gulf of St. Lawrence. Can. J. Fish. Aquat. Sci. 56, 1873–1881. doi: 10.1139/cjfas-56-10-1873
Collette, B. B., Carpenter, K. E., Polidoro, B. A., Juan-Jorda, M. J., Boustany, A., Die, D. J., et al. (2011). Conservation: high value and long life—double jeopardy for tunas and billfishes. Science 333, 291–292. doi: 10.1126/science.1208730
Dickhut, R. M., Deshpande, A. D., Cincinelli, A., Cochran, M. A., Corsolini, S., Brill, R. W., et al. (2009). Atlantic bluefin tuna (Thunnus thynnus) population dynamics delineated by organochlorine tracers. Environ. Sci. Tech. 43, 8522–8527. doi: 10.1021/es901810e
Elsdon, T. S., and Gillanders, B. M. (2003). Reconstructing migratory patterns of fish based on environmental influences on otolith chemistry. Rev. Fish Biol. Fish. 13, 217–235. doi: 10.1023/b:rfbf.0000033071.73952.40
Enfield, D. B., Mestas-Nuñez, A. M., and Trimble, P. J. (2001). The Atlantic multidecadal oscillation and its relation to rainfall and river flows in the continental U.S. Geophys. Res. Lett. 28, 2077–2080. doi: 10.1029/2000GL012745
Faillettaz, R., Beaugrand, G., Goberville, E., and Kirby, R. R. (2019). Atlantic multidecadal oscillations drive the basin-scale distribution of Atlantic bluefin tuna. Sci. Adv. 5:eaar6993. doi: 10.1126/sciadv.aar6993
Fraile, I., Arrizabalaga, H., and Rooker, J. R. (2015). Origin of bluefin tuna (Thunnus thynnus) in the bay of biscay. ICES J. Mar. Sci. 72, 625–634. doi: 10.1093/icesjms/fsu156
Galuardi, B., Royer, R., Golet, W., Logan, J., Neilson, J., and Lutcavage, M. (2010). Complex migration routes of Atlantic bluefin tuna question current population structure paradigm. Can. J. Fish. Aquat. Sci. 67, 966–976. doi: 10.1139/f10-033
Giuggioli, L., and Bartumeus, F. (2010). Animal movement, search strategies and behavioural ecology: a cross-disciplinary way forward. J. Animal Ecol. 79, 906–909.
Graves, J. E., Wozniak, A. S., Dickhut, R. M., Cochran, M. A., MacDonald, E. H., Bush, E., et al. (2015). Transatlantic movements of juvenile Atlantic bluefin tuna inferred from analyses of organochlorine tracers. Can. J. Fish. Aquat. Sci. 72, 1–9.
Hanke, H., Busawon, D., Rooker, J. R., and Secor, D. H. (2016). Estimates of stock origin for bluefin tuna caught in western Atlantic fisheries from 1975-2013. ICCAT Col. Vol. Sci. Pap. 72, 1376–1393.
Harford, W. J., Karnauskas, M., Walter, J. F., and Liu, H. (2017). Non-parametric modeling reveals environmental effects on bluefin tuna recruitment in Atlantic, Pacific, and Southern Oceans. Fish. Oceanogr. 26, 396–412. doi: 10.1111/fog.12205
Harrison, A. L., Costa, D. P., Winship, A. J., Benson, S. R., Bograd, S. J., Antolos, M., et al. (2018). The political biogeography of migratory marine predators. Nat. Ecol. Evol. 2, 1571–1578. doi: 10.1038/s41559-018-0646-8
Hays, G. C., Ferreira, L. C., Sequeira, A. M. M., Meekan, M. G., Duarte, C. M., Bailey, H., et al. (2016). Key questions in marine megafauna movement ecology. Trends Ecol. Evol. 31, 463–475. doi: 10.1016/j.tree.2016.02.015
Hobday, A. J., Evans, K., Eveson, J. P., Farley, J. H., Hartog, J. R., Basson, M., et al. (2015). “Distribution and migration—southern bluefin tuna,” in Biology and Ecology of Bluefin, eds T. Kitagawa and S. Kimura (Boca Raton: CRC Press).
Hosmer, D. W. Jr., Lemeshow, S., and Sturdivant, R. X. (2013). Applied Logistic Regression, 3rd Edn. Hoboken, NJ: John Wiley & Sons.
Kerr, L. A., Cadrin, S. X., Secor, D. H., and Taylor, N. G. (2017). Modeling the implications of stock mixing and life history uncertainty of Atlantic bluefin tuna. Can. J. Fish. Aquat. Sci. 74, 1990–2004. doi: 10.1139/cjfas-2016-0067
Kerr, L. A., and Goethel, D. R. (2014). “Simulation modeling as a tool for synthesis of stock identification information,” in Stock Identification Methods, 2nd Edn, eds S. Cadrin, L. Kerr, and S. Mariani (Amsterdam: Elsevier).
Kraus, R. T., Wells, R. J. D., and Rooker, J. R. (2011). Horizontal movement of Atlantic blue marlin (Makaira nigricans) in the Gulf of Mexico. Mar. Biol. 158, 699–713. doi: 10.1007/s00227-010-1593-3
Kurota, H., McAllister, M. K., Lawson, G. L., Nogueira, J. I., Teo, S. L. H., and Block, B. A. (2009). A sequential Bayesian methodology to estimate movement and exploitation rates using electronic and conventional tag data: application to Atlantic bluefin tuna (Thunnus thynnus). Can. J. Fish. Aquat. Sci. 66, 321–342. doi: 10.1139/f08-197
Landry, J. J., Kessel, S. T., McLean, M. F., Ivanova, S. V., Hussey, N. E., O’Neill, C., et al. (2018). Movement types of an Arctic benthic fish, shorthorn sculpin (Myoxocephalus scorpius), during open water periods in response to biotic and abiotic factors. Can. J. Fish. Aquat. Sci. 76, 1041–1051.
LeGrande, A. N., and Schmidt, G. A. (2006). Global gridded data set of the oxygen isotopic composition in seawater. Geophys. Res. Lett. 33:L12604.
Madigan, D. J., Baumann, Z., Carlisle, A. B., Hoen, D. K., Popp, B. N., Dewar, H., et al. (2014). Reconstructing trans-oceanic migration patterns of Pacific bluefin tuna using a chemical tracer toolbox. Ecology 95, 1674–1683. doi: 10.1890/13-1467.1
Mariani, P., Krivan, V., MacKenzie, B. R., and Mullon, C. (2016). The migration game in habitat network: the case for tuna. Theor. Ecol. 9, 219–232. doi: 10.1007/s12080-015-0290-8
Mather, F. J. (1980). A preliminary note on migratory tendencies and distributional patterns of Atlantic bluefin tuna based on recently acquired and cumulative tagging results. ICCAT Col. Vol. Sci. Pap. 9, 478–491.
Millar, R. B. (1987). Maximum likelihood estimation of mixed-stock fishery composition. Can. J. Fish. Aquat. Sci. 44, 583–590. doi: 10.1139/f87-071
Millar, R. B. (1990). Comparison of methods for estimating mixed stock fishery composition. Can. J. Fish. Aquat. Sci. 47, 2235–2241. doi: 10.1139/f90-248
Morse, M., Cadrin, S., Kerr, L., Secor, D., Siskey, M., Arrizabalaga, H., et al. (2018). An updated analysis of bluefin tuna stock mixing. ICCAT Col. Vol. Sci. Pap. 74, 3486–3509.
Moulton, D. L., Dance, M. A., Williams, J. A., Sluis, M. Z., Stunz, G. W., and Rooker, J. R. (2017). Habitat partitioning and movement of red drum and spotted seatrout. Estuar. Coast. 40, 905–916. doi: 10.1007/s12237-016-0189-7
Niklitschek, E. J., and Darnaude, A. M. (2016). Performance of finite mixture distribution models to estimate nursery habitat contributions to fish stocks. Peer J. 4:e2022v1.
Ortiz, M., Prince, E. D., Serafy, J. E., Holts, D. B., Davy, K. B., Pepperell, J. G., et al. (2003). Global overview of the major constituent-based billfish tagging programs and their results since 1954. Mar. Freshw. Res. 54, 489–507.
Otterson, G., Planque, B., Belgrano, A., Post, E., Reid, P. C., and Stenseth, N. C. (2001). Ecological effects of the North Atlantic Oscillation. Oecologia 128, 1–14. doi: 10.1007/s004420100655
Polovina, J. J., Howell, E. A., Kobayashi, D. R., and Seki, M. P. (2017). The transition zone chlorophyll front updated: advances from a decade of research. Prog. Oceanogr. 150, 79–85. doi: 10.1016/j.pocean.2015.01.006
Porch, C. E., Turner, S. C., and Powers, J. E. (2000). Virtual population analyses of Atlantic bluefin tuna with alternative models of transatlantic migration: 1970-1997. ICCAT Col. Vol. Sci. Pap. 52, 1022–1045.
Puncher, G. N., Cariani, A., Maes, G. E., Van Houdt, J., Herten, K., Cannas, R., et al. (2018). Spatial dynamics and mixing of bluefin tuna in the Atlantic Ocean and Mediterranean Sea revealed using next generation sequencing. Mol. Ecol. Res. 18, 620–638. doi: 10.1111/1755-0998.12764
R Core Team (2018). R: A Language and Environment for Statistical Computing. Vienna: R Foundation for Statistical Computing. Available at: https://www.rproject.org
Rodríguez-Ezpeleta, N., Díaz-Arce, N., Walter, J. F., Richardson, D. E., Rooker, J. R., Nøttestad, L., et al. (in press). Improved Atlantic bluefin tuna management through genetic assignment of natal origin. Front. Ecol. Environ.
Rooker, J. R., Alvarado Bremer, J. R., Block, B. A., Dewar, H., DeMetrio, G., Kraus, R. T., et al. (2007). Life history and stock structure of Atlantic bluefin tuna (Thunnus thynnus). Rev. Fish. Sci. 15, 265–310. doi: 10.1073/pnas.1525636113
Rooker, J. R., Arrizabalaga, H., Fraile, I., Secor, D. H., Dettman, D. L., Abid, N., et al. (2014). Crossing the line: migratory and homing behaviors of Atlantic bluefin tuna. Mar. Ecol. Prog. Ser. 504, 265–276. doi: 10.3354/meps10781
Rooker, J. R., Dance, M. A., Wells, R. J. D., Ajemian, M. J., Block, B. A., Castleton, M. R., et al. (2019). Population connectivity of pelagic megafauna in the Cuba-Mexico-United States triangle. Sci. Rep. 9:e1663. doi: 10.1038/s41598-018-38144-8
Rooker, J. R., Dance, M. A., Wells, R. J. D., Quigg, A., Hill, R. L., Appeldorrn, R. S., et al. (2018). Seascape connectivity and the influence of predation risk on the movement of fishes inhabiting a back-reef ecosystem. Ecosphere 9:e02200. doi: 10.1002/ecs2.2200
Rooker, J. R., Secor, D. H., DeMetrio, G., Kaufman, J. A., Belmonte Rios, A., and Ticina, A. (2008a). Evidence of trans-Atlantic mixing and natal homing of bluefin tuna. Mar. Ecol. Prog. Ser. 368, 231–239. doi: 10.3354/meps07602
Rooker, J. R., Secor, D. H., DeMetrio, G., Schloesser, R., Block, B. A., and Neilson, J. D. (2008b). Natal homing and connectivity in Atlantic bluefin tuna populations. Science 322, 742–744. doi: 10.1126/science.1161473
Rooker, J. R., Wells, R. J. D., Itano, D. G., Thorrold, S. R., and Lee, J. M. (2016). Natal origin and population connectivity of bigeye and yellowfin tuna in the Pacific Ocean. Fish. Oceanogr. 25, 277–291. doi: 10.1111/fog.12154
Schloesser, R. W., Neilson, J. D., Secor, D. H., and Rooker, J. R. (2010). Natal origin of Atlantic bluefin tuna (Thunnus thynnus) from the Gulf of St. Lawrence based on δ13C and δ18O in otoliths. Can. J. Fish. Aquat. Sci. 67, 563–569. doi: 10.1139/f10-005
Secor, D. H., Rooker, J. R., Gahagan, B., Siskey, M., and Wingate, R. (2015). Depressed resilience of bluefin tuna in the western Atlantic Ocean associated with age truncation. Conserv. Biol. 29, 400–408. doi: 10.1111/cobi.12392
Sequeira, A. M. M., Rodríguez, J. P., Eguíluz, V. M., Harcourt, R., Hindell, M., Sims, D. W., et al. (2018). Convergence of marine megafauna movement patterns in coastal and open oceans. Proc. Nat. Acad. Sci. U.S.A. 115, 3072–3077. doi: 10.1073/pnas.1716137115
Sibert, J. R., Lutcavage, M. E., Nielsen, A., Brill, R. W., and Wilson, S. G. (2006). Interannual variation in large-scale movement of Atlantic bluefin tuna (Thunnus thynnus) determined from pop-up satellite archival tags. Can. J. Fish. Aquat. Sci. 63, 2154–2166. doi: 10.1139/f06-114
Siskey, M. R., Wilberg, M. J., Allman, R. J., Barnett, B. K., and Secor, D. H. (2016). Forty years of fishing: changes in the age structure and stock mixing in northwestern Atlantic bluefin tuna (Thunnus thynnus) associated with size selective and long-term exploitation. ICES J. Mar. Sci. 73, 2518–2528. doi: 10.1093/icesjms/fsw115
Smith, S. J., and Campana, S. E. (2010). Integrated stock mixture analysis for continuous and categorical data, with application to genetic–otolith combinations. Can. J. Fish. Aquat. Sci. 67, 1533–1567.
Standing Committee on Research and Statistics [SCRS] (2017). Report of The 2017 ICCAT Atlantic Bluefin Tuna Stock Assessment Meeting. Madrid: SCRS.
Stenseth, N. C., Mysterud, A., Ottersen, G., Hurrell, J. W., Chan, K. S., and Lima, M. (2002). Ecological effects of climate change. Science 297, 1292–1296.
Thygesen, U. H., Sommer, L., Evans, K., and Patterson, T. A. (2016). Dynamic optimal foraging theory explains vertical migration of bigeye tuna. Ecology 97, 1852–1861. doi: 10.1890/15-1130.1
White, J. W., Standish, J. D., Thorrold, S. R., and Warner, R. R. (2008). Markov chain Monte Carlo methods for assigning larvae to natal sites using natural geochemical tags. Ecol. Appl. 18, 1901–1913. doi: 10.1890/07-1792.1
Whitlock, R. E., Hazen, E. L., Walli, A., Farwell, C., Bograd, S. J., Foley, D. G., et al. (2015). Direct quantification of energy intake in an apex marine predator suggests physiology is a key driver of migrations. Sci. Adv. 1:e1400270. doi: 10.1126/sciadv.1400270
Keywords: migration, natal origin, stock mixing, Mediterranean Sea, otolith chemistry, stable isotopes
Citation: Rooker JR, Fraile I, Liu H, Abid N, Dance MA, Itoh T, Kimoto A, Tsukahara Y, Rodriguez-Marin E and Arrizabalaga H (2019) Wide-Ranging Temporal Variation in Transoceanic Movement and Population Mixing of Bluefin Tuna in the North Atlantic Ocean. Front. Mar. Sci. 6:398. doi: 10.3389/fmars.2019.00398
Received: 26 February 2019; Accepted: 27 June 2019;
Published: 11 July 2019.
Edited by:
Michael Paul Jensen, Southwest Fisheries Science Center (NOAA), United StatesReviewed by:
Brian R. MacKenzie, Technical University of Denmark, DenmarkSteven Campana, University of Iceland, Iceland
Copyright © 2019 Rooker, Fraile, Liu, Abid, Dance, Itoh, Kimoto, Tsukahara, Rodriguez-Marin and Arrizabalaga. This is an open-access article distributed under the terms of the Creative Commons Attribution License (CC BY). The use, distribution or reproduction in other forums is permitted, provided the original author(s) and the copyright owner(s) are credited and that the original publication in this journal is cited, in accordance with accepted academic practice. No use, distribution or reproduction is permitted which does not comply with these terms.
*Correspondence: Jay R. Rooker, rookerj@tamug.edu