Modeling Carbon Budgets and Acidification in the Mediterranean Sea Ecosystem Under Contemporary and Future Climate
- 1National Institute of Oceanography and Applied Geophysics – OGS, Trieste, Italy
- 2International Centre for Theoretical Physic ICTP, Trieste, Italy
- 3Plymouth Marine Laboratory, Plymouth, United Kingdom
- 4Centre National de Recherches Météorologiques (CNRM), Université de Toulouse, Météo-France, CNRS, Toulouse, France
We simulate and analyze the effects of a high CO2 emission scenario on the Mediterranean Sea biogeochemical state at the end of the XXI century, with a focus on carbon cycling, budgets and fluxes, within and between the Mediterranean sub-basins, and on ocean acidification. As a result of the overall warming of surface water and exchanges at the boundaries, the model results project an increment in both the plankton primary production and the system total respiration. However, productivity increases less than respiration, so these changes yield to a decreament in the concentrations of total living carbon, chlorophyll, particulate organic carbon and oxygen in the epipelagic layer, and to an increment in the DIC pool all over the basin. In terms of mass budgets, the large increment in the dissolution of atmospheric CO2 results in an increment of most carbon fluxes, including the horizontal exchanges between eastern and western sub-basins, in a reduction of the organic carbon component, and in an increament of the inorganic one. The eastern sub-basin accumulates more than 85% of the absorbed atmospheric CO2. A clear ocean acidification signal is observed all over the basin, quantitatively similar to those projected in most oceans, and well detectable also down to the mesopelagic and bathypelagic layers.
Introduction
The Mediterranean Sea (MS) is the largest semi-enclosed European sea, and one of the most valued seas in the world. While mainly oligotrophic, it is rich in biological diversity, one of the world biodiversity hotspots, and comprises a variety of coastal and marine ecosystems that deliver benefits to all its coastal countries (Myers et al., 2000; Cuttelod et al., 2009). Currently, anthropogenic pressures on MS ecosystem are increasing because of tourism (about a third of international tourist arrivals; UNWTO–World Tourism Organization, 2015) and population growth, economic growth of both European and North African countries, sea-based activities such as fisheries, aquaculture, and ship traffic (Micheli et al., 2013). Furthermore, the Mediterranean region has been recognized as a “climate change hotspot” (Giorgi, 2006; Darmaraki et al., 2019b), i.e., an area particularly affected by climate change. Therefore, it is important to assess to which extent climate change will affect the dynamics of Mediterranea marine ecosystems (Lejeusne et al., 2010), and—eventually—its capability to provide ecosystem services (Costanza et al., 1997).
Models projections suggest that climate change will cause an increase in MS surface water temperature (Adloff et al., 2015; Darmaraki et al., 2019a; Soto-Navarro et al., 2020) and an intensification of extreme events (Galli et al., 2017), as well as changes in Mediterranean circulation and in vertical mixing/stratification processes, with less dense water formation (at least in the Western basin), a weaker thermohaline circulation and a stronger vertical stratification (Thorpe and Bigg, 2000; Somot et al., 2006; Adloff et al., 2015; Soto-Navarro et al., 2020).
However, climate change impacts on the MS biogeochemical properties (i.e., nutrients concentration and plankton productivity) at the basin-scale are less explored in scientific literature. Lazzari et al. (2014) performed several simulations to assess the impact of a family of Special Report on Emissions Scenarios (SRES) A1B climate scenarios, in conjunction with different scenarios of land use and related nutrient loads in rivers nutrients, suggesting that in all scenarios the increment in temperature will cause an increase in both plankton productivity and respiration, with the net effect of reducing plankton biomass and the amount of biomass available to higher trophic level organisms (with possible consequences on fishery compartments) and of increasing the labile dissolved organic matter. In that study, however, biogeochemical simulations described only the first and last 10 years of the 21st century, without a continuous simulation of the whole period, potentially introducing a significant bias and unknown uncertainties in future projections. Macias et al. (2015) projected a small increment in primary productivity and significant changes in spatial distribution of plankton biomass under both RCP 4.5 and 8.5 warming scenarios, likely related to changes in the stratification regime of the basin. However, they used a model not validated against experimental observations, and did not discuss possible existence of biogeochemical biases and/or numerical drifts. Richon et al. (2019) explored the impact of SRES A2 scenarios with a model setup similar to the one used in the present study, suggesting that the unbalanced nitrogen-to-phosphorus input from riverine discharge and fluxes via the Strait of Gibraltar used in their scenario lead to an expansion of phosphorus-limited regions across the MS and a significant reduction of plankton net productivity. More recently, Pagès et al. (2020) produced a continuous RCP8.5 run, that assumed no effect of temperature on the kinetics of the biogeochemical processes and no changes in nutrient loads from rivers, and showed that the climate change related variations in (only) hydrodynamics suffice in causing changes in biogeochemistry (increased oligotrophication, reduced production, decreased phytoplankton abundance, nitrate decline in the surface layer) but mainly in the second half of the 21st century, and only in the western basin. Other projections refer to simulations performed within recent EU projects (Boero et al., 2016), and in a variety of downscaling studies at the regional (Holt et al., 2012; Herrmann et al., 2014), subregional (Lamon et al., 2014) or local (Cossarini et al., 2008; Salon et al., 2008; Canu et al., 2010) scales.
Yet, and as far as we know, no paper quantified the expected changes in ocean acidification in the MS, nor described the expected changes in carbon budgets and fluxes, rather than variables concentrations.
Therefore, here we performed a continuous 100 years simulation under a high greenhouse gases emission scenario, in order to assess the potential impact of climate changes related effects on carbonate and biogeochemical systems, with a particular focus on changes in carbon cycling, budgets, and ocean acidification. Furthermore, and notably, the impact of climate change has been assessed by contrasting the climate model simulation against a century-long baseline simulation, used as a control run to assess multidecadal trends not related to climate change, but potentially present in long simulations, and which need to be removed to isolate climate change effects and reduce the uncertainty in climate scenarios impact studies. The use of this methodology, widely recognized as appropriate (Sarmiento et al., 1998; Teutschbein and Seibert, 2012), but rarely implemented in biogeochemical studies, increases the robustness of our conclusion and represents an additional element of novelty in our study.
Materials and Methods
Model System
The simulations have been performed by using a state-of-the-art transport-biogeochemical model, purposely developed and validated for describing plankton productivity and carbon and nutrients biogeochemical cycles in the MS (Lazzari et al., 2012, 2016). The model is the product of a long-term research activity on MS ecosystem modeling (Crise et al., 1998; Crispi et al., 2001; Solidoro et al., 2003; Lazzari et al., 2012), it is now embedded in the European Copernicus Marine Environment Monitoring Service (CMEMS) infrastructure, and routinely used to produce short-term forecasts of the MS biogeochemistry (Salon et al., 2019; Cossarini et al., 2021). The capability of the model to reproduce (without any data assimilation) the main features of MS biogeochemistry has been assessed by contrasting model results against satellite estimates of surface chlorophyll (Lazzari et al., 2012), available information on primary productivity (Lazzari et al., 2012), nutrients (Lazzari et al., 2016), dissolved inorganic carbon and alkalinity data (Cossarini et al., 2015). It has been used in a number of applications, both at the basin wide scale (e.g., Lazzari et al., 2014; Melaku Canu et al., 2015; Galli et al., 2017) and for smaller subregions (Lamon et al., 2014; Fraysse et al., 2013).
The physical dynamics were pre-computed by the ocean general circulation model NEMOMED8 at 1/8° horizontal resolution (Sevault et al., 2009), then used in Beuvier et al. (2010), Herrmann et al. (2010), Albouy et al. (2013), Adloff et al. (2015), and Richon et al. (2019) for climate scenarios studies. NEMOMED8 supplied the temporal evolution at daily frequency of the 3D fields of horizontal and vertical current velocities, vertical eddy diffusivity, temperature, salinity, in addition to surface data for solar shortwave irradiance and wind stress. The meshgrid has 1/8° × 1/8°cos(latitude) horizontal resolution ranging from 9 to 12 km from the North to the South of the basin with square meshes and with a stretched and tilted grid at the Gibraltar Strait that increase locally the resolution up to 6 km (160 × 394 grid points in latitude and longitude directions), and 43 vertical z-levels (non-terrain following).
Details on the NEMOMED8 model and the protocol of the simulation scenario A2 can be found in Albouy et al. (2013, see their supplementary material) and Adloff et al. (2015). The same configuration for the SRES A2 scenario was adopted by Richon et al. (2019).
The biogeochemical dynamics are simulated using the Mediterranean implementation of the Biogeochemical Flux Model (BFM; Lazzari et al., 2012), where chlorophyll and carbon dynamics are based on the parameterization of chlorophyll synthesis proposed by Geider et al. (1997), and on a Plankton Functional Type (PTF, Anderson, 2005) representation of the planktonic food web that includes nine plankton pools, subdivided in photosynthetic producers (phytoplankton), consumers (zooplankton), and decomposers (bacteria). These broad functional groups are further subdivided into functional subgroups, so to have four phytoplankton groups (representative of diatoms and of not-diatoms of different size), heterotrophic bacteria, two microzooplankton groups preying on bacteria and smaller phytoplankton groups, and two mesozooplankton groups feeding of microzoo and larger phytoplankton groups. Each PFT is described in terms of its internal concentration of carbon, nitrogen, phosphorus and—for diatoms—silica, and contribute to the interlaced biogeochemical cycles of these elements. The model also includes the descriptions of the labile, semi-refractory, and refractory dissolved organic matter and of the particulate matter, as well as the dynamics of the microbial loop, which is known to play an important role in MS (Thingstad and Rassoulzadegan, 1995).
The carbonate system consists of two prognostic state variables: alkalinity (ALK) and dissolved inorganic carbon (DIC), from which pH is also computed (Cossarini et al., 2015). DIC exchange at the air-sea is resolved by computing the seawater pH, pCO2 and gas transfer formula (OCMIP I model, Orr, 1999; Melaku Canu et al., 2015).
The physical and biogeochemical models are coupled through a modified version of the OPA transport model (Foujols et al., 2000), named OGSTM-BFM (OGS Transport Model—Biogeochemical Flux Model; Lazzari et al., 2012), which was already used in many studies on MS biogeochemistry (Lazzari et al., 2012, 2014, 2016; Cossarini et al., 2015; Melaku Canu et al., 2015) and it is currently employed as a core component of the CMEMS forecast system for biogeochemical properties of the MS (Salon et al., 2019), integrated with a data assimilation scheme (Teruzzi et al., 2014, 2018, 2019).
The Scenario Numerical Experiment
The scenario simulation carried out covers the period between January 2000 to December 2099 over the MS domain (Figure 1), and assuming the atmosphere forcing are in agreement with a high CO2 scenario, representing a future socio-economic system in which economic activities and industrial processes continue to grow in line with the current situation. In particular, we adopted a scenario derived from the A2 emission scenario, therefore at the higher end of the SRES family related to a story line characterized by heterogeneity in the socio-economic system and a continuous population growth that reaches over 10 billion by 2050. According to Integrated Assessment Models this scenario has an expected CO2 concentration of about 575 and 870 ppm for the middle and end of the 21st century, respectively. Therefore, it is close and—for all of our purposes equivalent—to the RCP8.5 scenario, which assumes similar CO2 concentration regardless of the underpinning socio-economic dynamics (IPCC, 2014; Harrison et al., 2019). The time course of CO2 atmospheric concentration used in the simulation is illustrated in the plot within Figure 2.
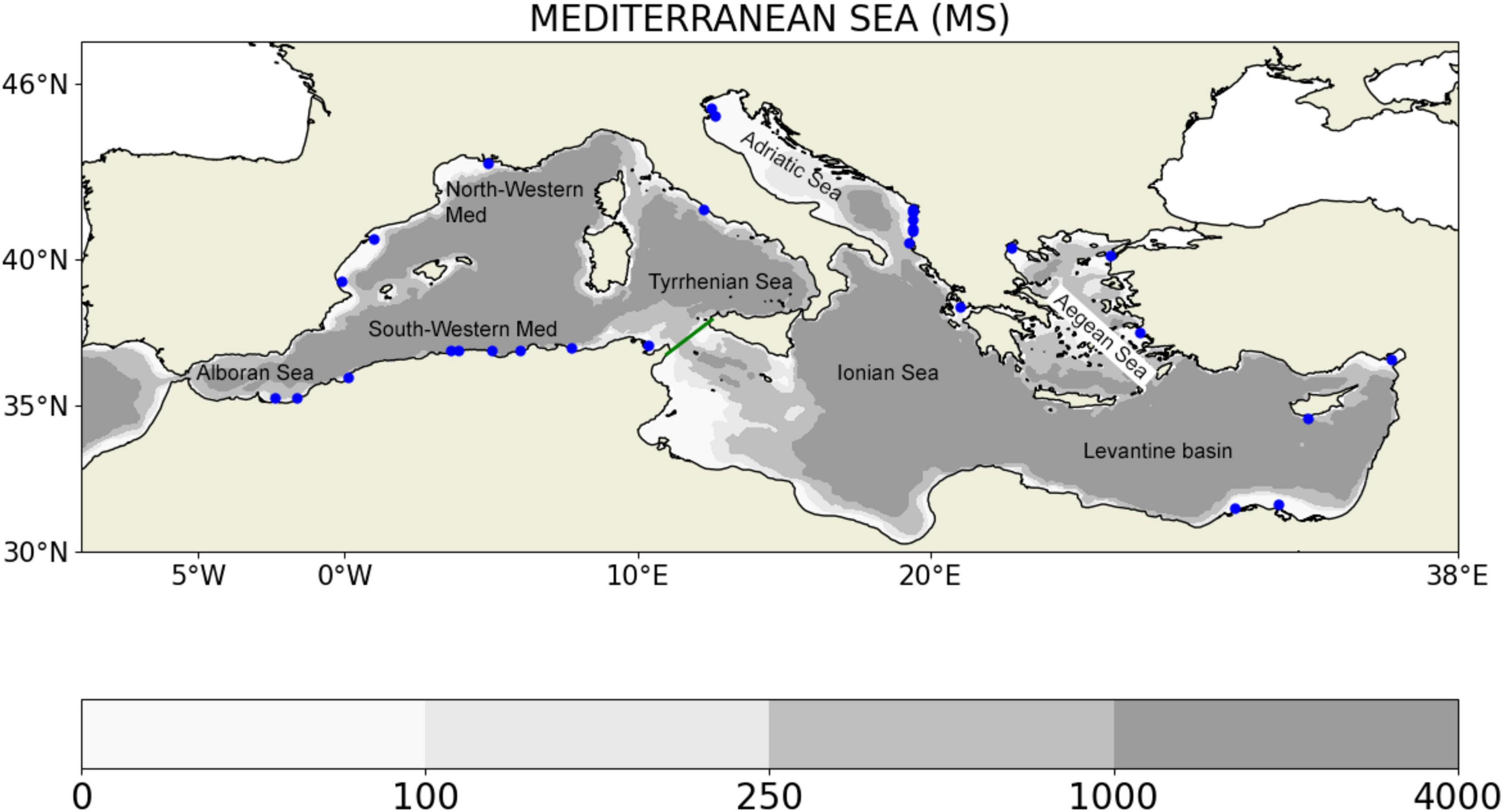
Figure 1. Mediterranean Sea (MS) simulation domain with bathymetry (m); The green line separated the West and East sub-basins. The blue dots indicate the position of major rivers considered in the model.
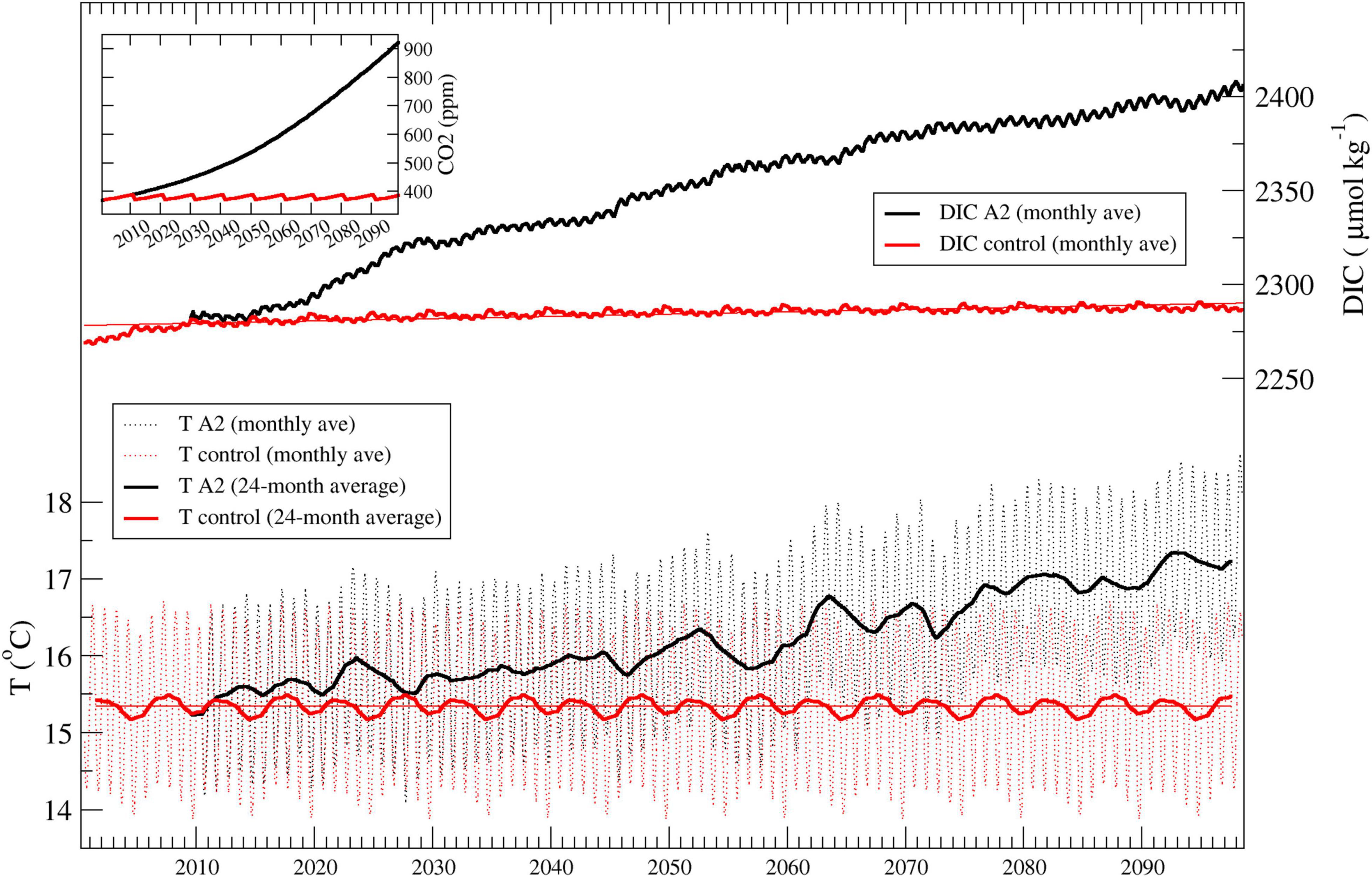
Figure 2. Time series 2000–2100 of basin-scale averaged temperature (T, monthly means and 24-month average) and dissolved inorganic carbon (DIC, 24-month average) in the surface layer, for the control run (red) and the SRES A2 scenario (black). The smaller plot depicts the time course of atmospheric CO2 concentration in the two simulations.
We chose to focus on this scenario because it might trigger signals large enough to be detected. At the same time, it is not unrealistic and, actually, analysis of historical data over the last decades indicates that it corresponds to the current trajectory (2005 to present) of emissions (Schwalm et al., 2020).
The boundary condition at Gibraltar and the biogeochemical initial conditions of the control run were set up in agreement to Lazzari et al. (2012), and therefore based on the MEDA-MEDATLAS database, while nutrient inputs from major rivers and Black Sea were based on Ludwig et al. (2009). Regarding the carbonate system variables (DIC and alkalinity), the initial conditions and inputs from rivers and Black Sea are as described in Cossarini et al. (2015), while the boundary condition at the Gibraltar were based on the CMIP5 global models. In the scenario simulation, rivers inputs were kept constant as in present-day condition, the Gibraltar boundary condition evolve consistently with the CMIP five global model output. The control run spin-up period was 50 years.
Conceptual Framework, Major Processes and Relationships Among Nutrients and Carbon Cycling
While the coupled model considers about 50 state variables and an even larger number of processes, it might be interesting to synthetize the major processes driving the carbon cycle in a conceptual scheme, useful also to highlight the relationships between the nutrients (N,P,Si) and carbon cycling and environmental forcing. The scheme is illustrated in Figure 3.
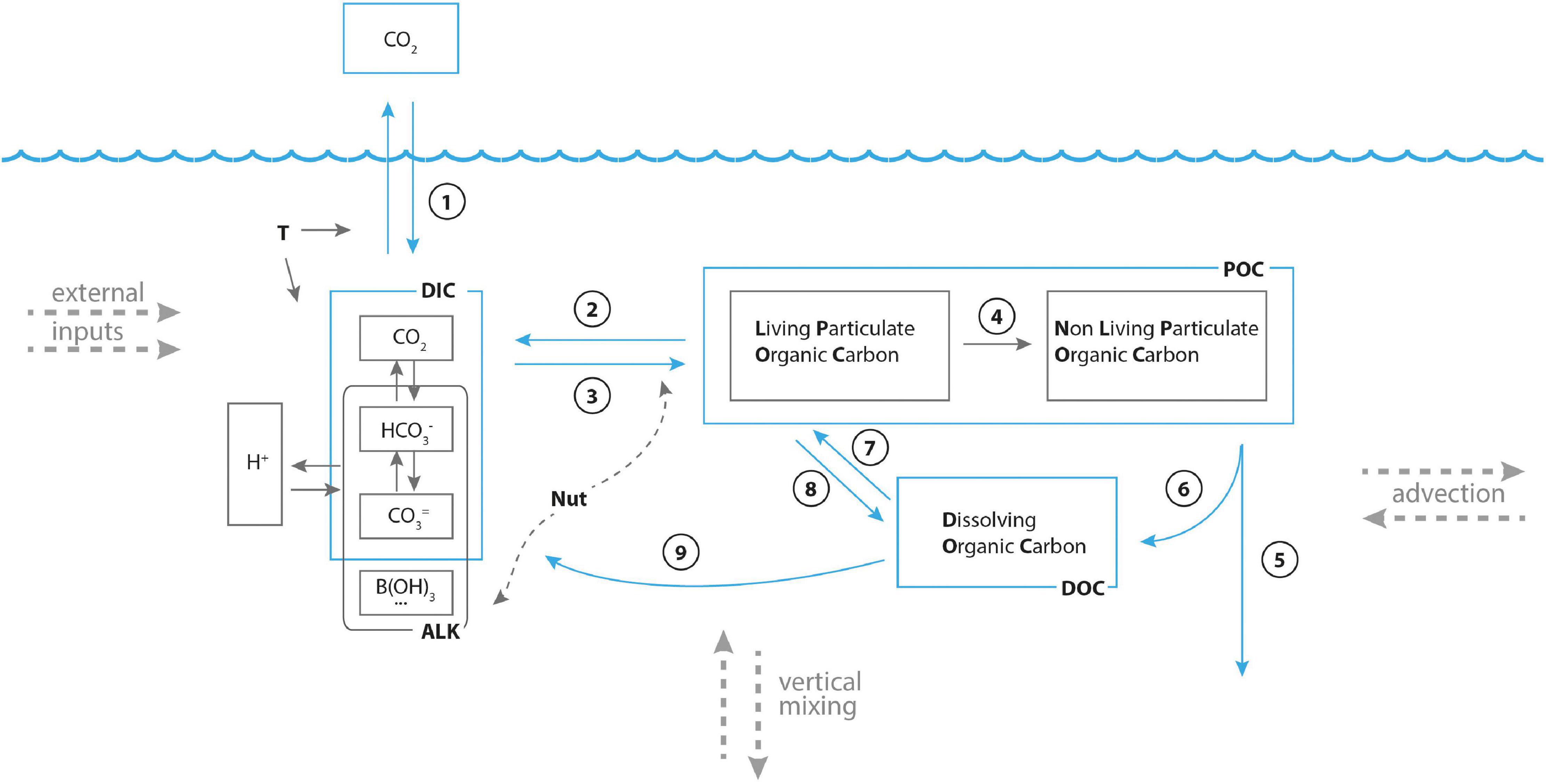
Figure 3. Conceptual framework for carbon cycle and major processes considered in the model. See text in section 2.2 for explanation.
Atmospheric CO2 dissolves in sea water through the air-sea exchange process (arrow #1 in figure), proportional to the difference between concentration of CO2 in the atmosphere and in the surface ocean, and occurring at a rate depending on sea temperature T and other factors affecting the interface, such as wind intensity. Since the major driver of the dissolution/degassing process is the CO2 gradient between atmosphere and surface sea, all factors able to change the CO2 sea surface concentration indirectly affect this process. The factors acting to reduce sea surface CO2 will favor CO2 atmospheric dissolution, those acting to increase it will favor CO2 degassing. Most of these factors depend on sea temperature, in turn a function of climate warming and ocean transport, but many are also influenced by biogeochemical processes.
Once dissolved in the sea, CO2 chemically reacts with water to form carbonic acid (H2CO3), which partially dissociates in bicarbonate () and bicarbonate () ions, while releasing hydrogen ions H+. The concentration of H+ defines the ocean acidity, measured by pH. Other substances present in the ocean that partially dissociate in hydrogen ions and weak chemical bases impacts on the carbonate system equilibria by bonding to (and therefore removing) some of the hydrogen ions. The final equilibrium concentrations of the carbon dioxide system (carbonate system) and of hydrogen ions (pH) therefore result from multiple equilibria, and depend on the total amount of dissolved inorganic carbon (DIC, the sum of CO2 hco3 and co3), the total alkalinity (excess of proton acceptors over proton donors), and temperature (Zeebe and Wolf-Gladrow, 2001; Middelburg et al., 2020).
The photosynthesis (arrow #2 in Figure 3) reduces DIC concentrations by transforming DIC in living particulate organic matter. Beside temperature, photosynthesis rate depends on light availability, in turn a function of water transparency and surface irradiance level. The opposite process is respiration (arrow #3 in figure), a process continuously performed by all living organisms and during which organic carbon is burned and transformed back to DIC. During photosynthesis, the photosynthetic organisms also need to assimilate nitrogen and phosphorus, that are uptaken from the sea. Therefore, a shortage of dissolved inorganic nutrients causes a limitation to photosynthetic activity, and hence in carbon cycling. The death of living organism implies a flux from living POC to non-living POC (arrow #4), which then sinks (arrow #5) and is dissolved and mineralized to DOC (arrow #6) and—thanks to bacterial activity- DIC (arrow #9). During mineralization, nutrients are released in their dissolved inorganic forms too. Finally, several biogeochemical processes (exudation, assimilation, microbial food web activity) drive the fluxes that support exchange of carbon from DOC to POC (arrows #7 and 8). Those processes also depend on N and P concentrations.
It is therefore evident that the cycle of carbon in sea, as well as the exchange of carbon dioxide though the sea-air interface, depends on many biogeochemical processes, and is deeply interlaced with the cycling of nitrogen and phosphorus.
Reducing Uncertainties in Long-Term Dynamic
Coupled transport-biogeochemical models are based on the numerical integration of a system of a large number of partial differential equations, subjected to prescribed initial and boundary conditions. Given the complexity of the system and the large number of uncertainty sources present in any code, it is only fair to assume that any model, when run over a period as long as 100 years, would show some multi-year trend in some of its state variables. These trends might be representative of a real dynamic of the system, moving from its initial conditions in agreement with boundary conditions, and/or the result of some numerical drifts, or the cumulative effect of the propagation of some uncertainties. While to reduce the uncertainty sources in a model simulation surely is a meritorious activity, it is true that any complex model will always present some degree of dysfunctionality, approximations and uncertainty sources (Oreskes et al., 1994; Stow et al., 2009; Hipsey et al., 2020), so that—while keeping on improving model structures and parameterizations—it is important to be able to extract the largest fraction of useful information from currently available, though still imperfect, simulations.
A viable way to detect the effect of a specific forcing is to run pairs of simulations, differing only for that specific forcing, so that one simulation can be used as a “control,” and any difference between the two simulations can be related to the single changed factor.
In our case, this implies to get a “control” run by performing a 100-years long simulation forced by present day atmospheric conditions (i.e., by repeating for 10 times the atmospheric conditions used for the period 2000–2010) in addition to the 100-years long “scenario” simulations, in which the atmospheric forcing changes in agreement with the climate scenarios, all the rest being equal.
In reality, in our application, as a preliminary study, we performed not a single run but a number of “control” simulations, in order to fine tune a few highly uncertain model parameters, and to adjust the long-term dynamic to a somehow reasonable state. The model set-up has then been frozen, but for atmospheric forcing which have been replaced by those corresponding to the future scenarios. Finally, we computed the multidecadal signal in the “control” simulation, removed it (if present) from the “climate” scenario simulations, and analyzed the corrected results.
Figure 2 exemplifies what above described: the time series of the basin-scale averaged surface temperature and dissolved inorganic carbon concentration (taken as examples) are shown for the control run and the scenario simulation. Changes in the control run (that cannot be ascribed to climate change) are then estimated as the difference between the decadal means 2090–2099 and 2000–2009 in the control run (SCTRLFUT and SCTRLPRES, respectively); this bias is then removed from the difference in the scenario simulation between the decadal means 2090–2099 and 2000–2009 (SCLIMFUT and SCLIMPRES, respectively) to highlight the climate change signal ΔS:
where < . > denotes the decadal means.
Results and Discussion
Model Corroboration and Dynamics During the Historical Period
To demonstrate the model capability to reproduce the most important properties of MS biogeochemistry, we compare the model results in the historical period 2000–2010 with a series of observational evidences.
The model correctly simulates the mean 3D structure of the chlorophyll field, in agreement with previous studies using models (e.g., Lazzari et al., 2012) and observations (e.g., Mignot et al., 2014; Lavigne et al., 2015). The mean surface concentration of the control run computed in the period 2001–2010 (Figure 4, top) clearly shows the east-west gradient and the highly productive areas in north-western MS and Alboran Sea. The model also properly reproduces the mean seasonal cycle of the averaged vertical chlorophyll profile in western and eastern sub-basins (Figure 4, bottom), featuring the winter bloom, mainly limited to the upper 100 m layer and with highest values in the western areas, and the summer stratification, characterized by the deep chlorophyll maximum (DCM) at depths increasing from 75 m in the western areas to 100 m in the eastern ones.
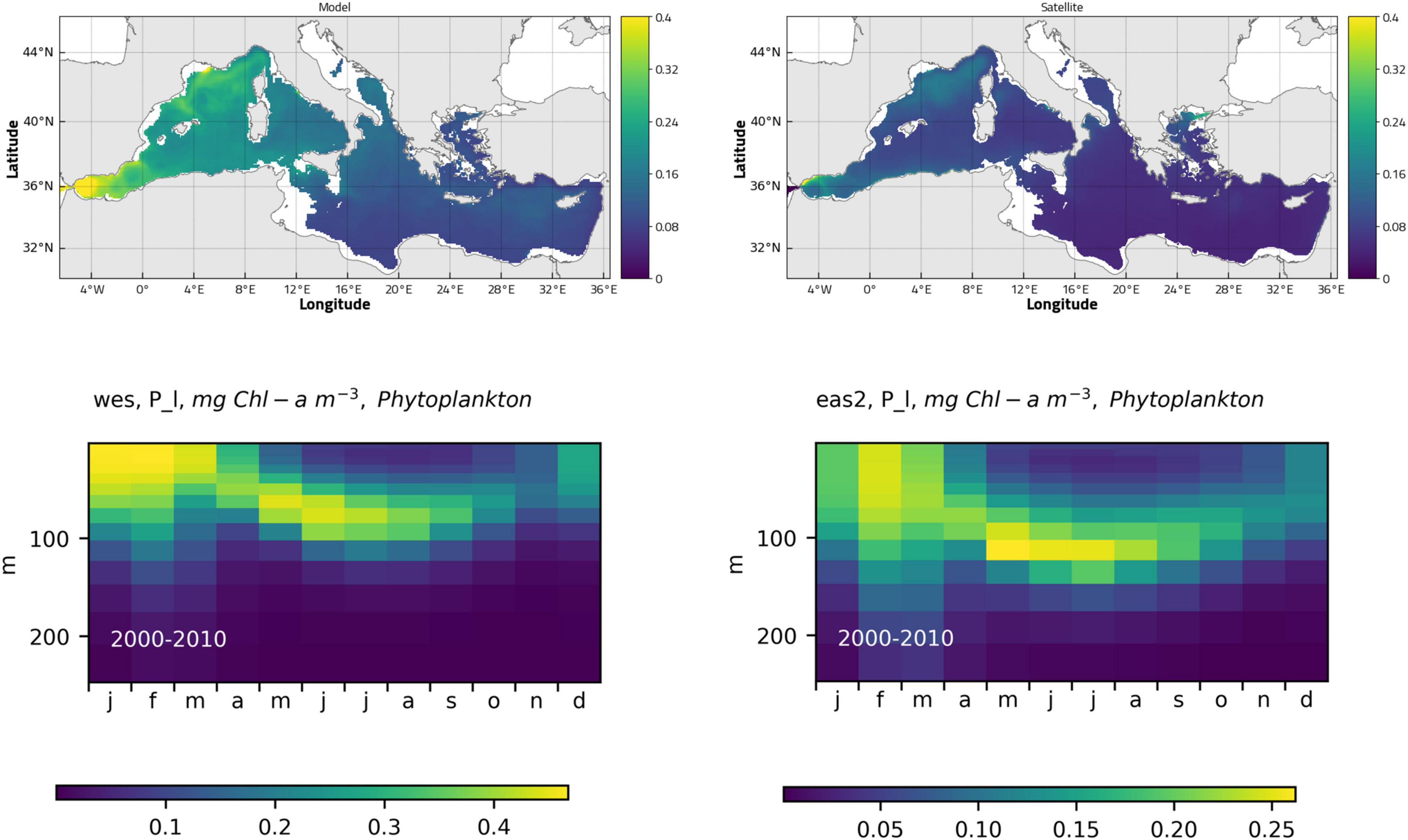
Figure 4. Mean surface chlorophyll concentration computed from control run (top, left) and CMEMS Ocean Color dataset (OCEANCOLOUR_MED_CHL_L3_REP_OBSERVATIONS_009_073) (top, right) for the historical period. Mean seasonal cycle of chlorophyll in the historical period: 0–250 m layer in the western (bottom, left) and eastern (bottom, right) sub-basins.
Model nutrients (phosphate and nitrate), dissolved oxygen, dissolved inorganic carbon, and alkalinity concentrations computed for the historical period are compared with a reference observational climatology derived from available moorings and vessels provided by the OGS National Oceanographic Data Center (NODC-OGS), spanning the period 2000–2011 (reported in Cossarini et al., 2015; Lazzari et al., 2016). Standard skill metrics (RMSD, bias, and correlation) are computed following a basin-aggregated approach for eight different vertical layers (Table 1) and compared to typical variability of the variables (i.e., standard deviation computed on spatial and temporal variability of the MS).
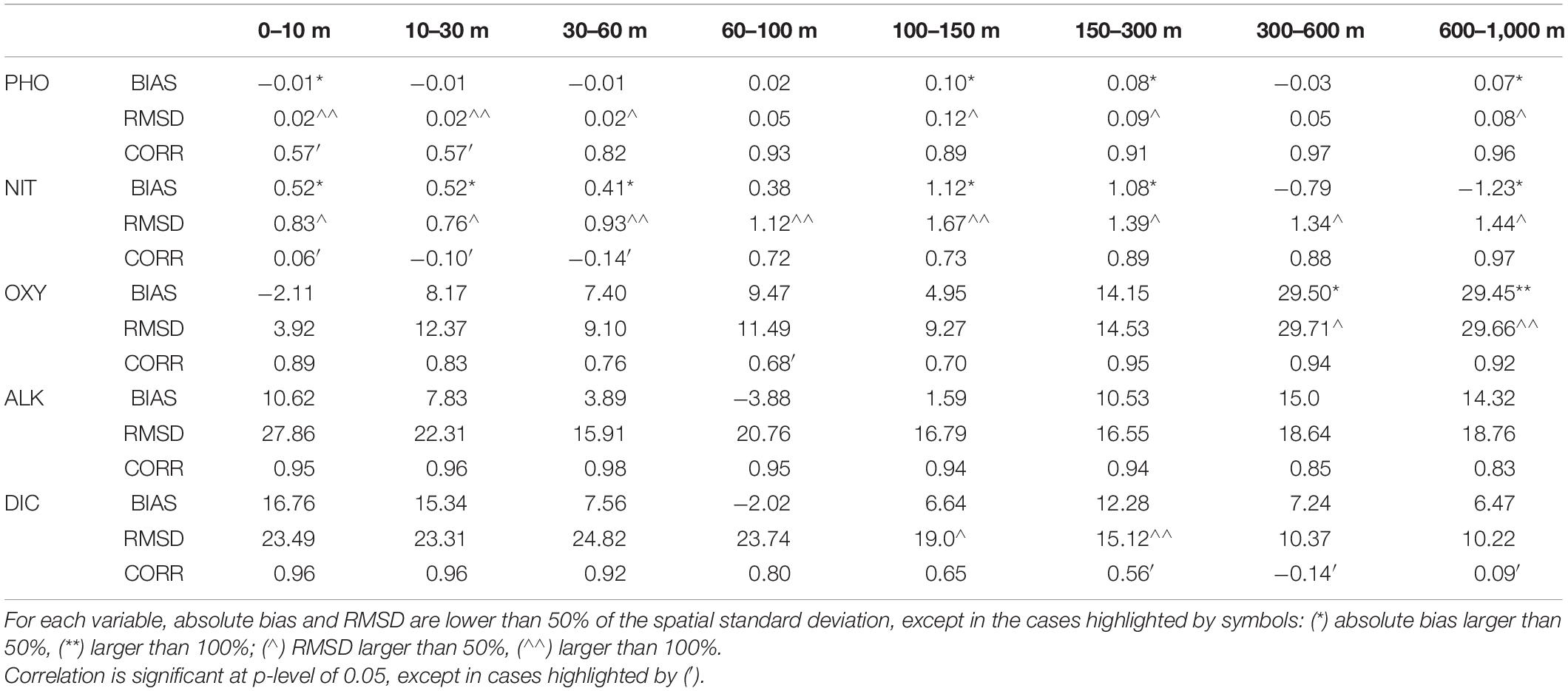
Table 1. Basin-scale averaged BIAS, RMSD and correlation over the period 2000–2010 between phosphate (PHO), nitrate (NIT), oxygen (OXY), alkalinity (ALK), and dissolved inorganic carbon (DIC) of the control run outputs and the climatological vertical profiles provided by the NODC-OGS dataset.
Carbonate system variables are characterized by a very high level of accuracy compared to their typical variability. Phosphate shows some inaccuracy in the surface layers (i.e., RMSD higher than the typical variability of the basin), however, it must be noted that in absolute terms the surface phosphate values (i.e., mean value is 0.02 ± 0.02 mmol/m3) and small absolute errors are amplified. The accuracy of oxygen, which is compared with its typical temporal variability, is very good in all layers except the deepest ones where the model overestimates of about 30 mmol/m3. Nitrate is the least accurate variable, with errors exceeding its typical variability in several layers. However, given the fact that phosphate is the limiting nutrient in the MS (Lazzari et al., 2016), it is reasonable to consider nitrate inaccuracy impacting less on the scenario reliability.
In general, correlation is larger than 0.7, confirming the ability of the model to correctly reproduce the basin-wide gradients along the water column, with the exception of dissolved inorganic carbon below 150 m. In this case, it is worth to remind that the spatial gradient of carbonate system variables is pretty low in the deep layers (Cossarini et al., 2015).
Long Term Dynamics
The long-term dynamics of the biogeochemical properties are assessed by analyzing the scenario simulations in 2090–2099 after removing the trend in the control simulation, if present (see Section 2). The analysis shows that at the end of the century, the concentration of nutrients (both nitrate and phosphate) increases in both epi- and mesopelagic layers, with larger increments in the surface layers and in the western part (around +17% with respect to the present conditions, and in partial agreement with Richon et al., 2019, specifically for nitrate), whereas in the bathypelagic layer we observe a very modest increase of nitrate in the western basin, a modest decrease in the eastern part, and no variation in phosphate (Table 2).
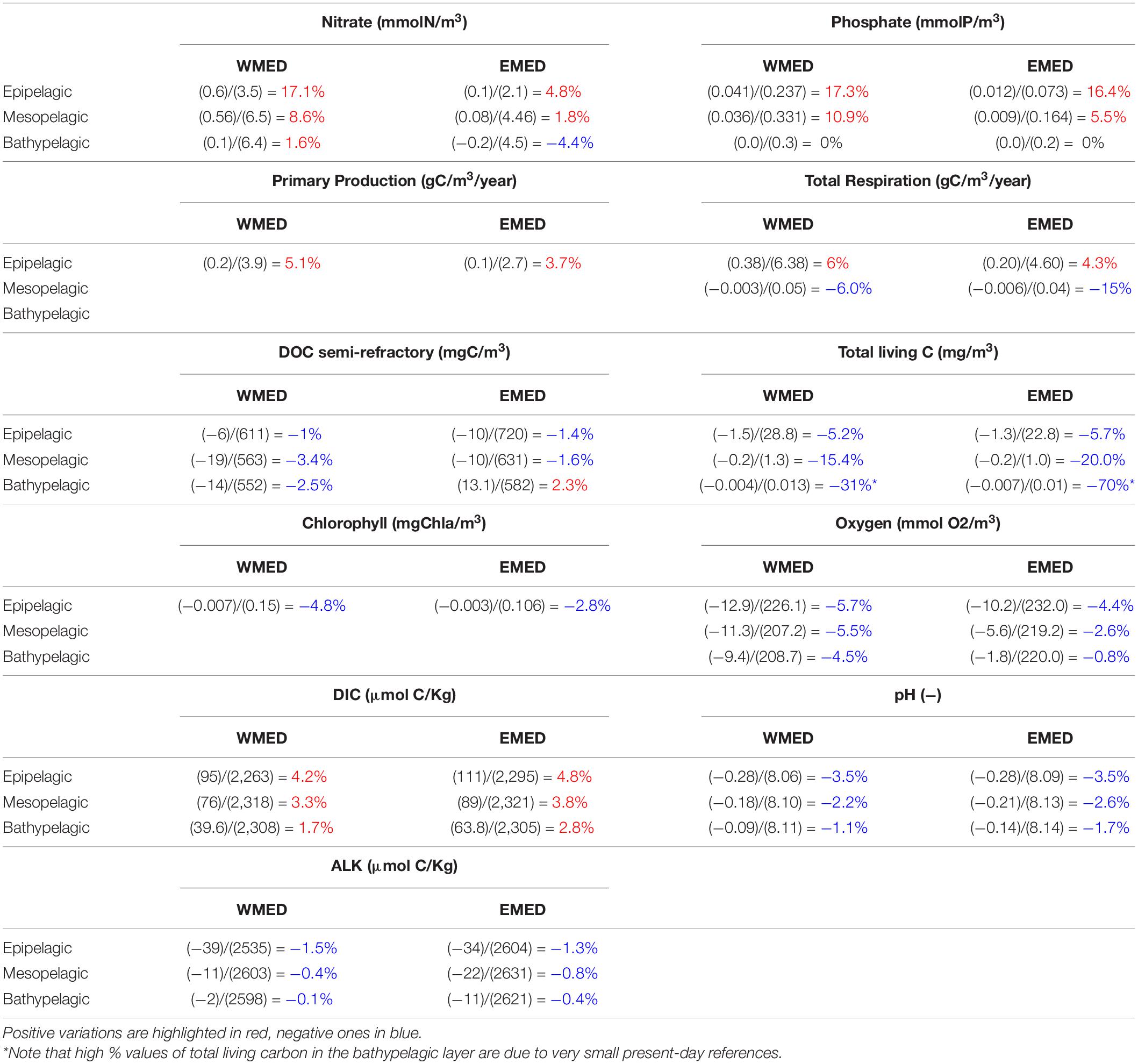
Table 2. Mean present-day values for western and eastern basins and variations at the end of the scenario simulation (as in Eq. 1), for the epipelagic, mesopelagic and bathypelagic layers, defined, respectively, as the average between 0 and 250, 250, and 1,000 m, 1,000 and 5,000 m. Each cell reports the percentage ratio between the net variation estimated at the end of the century (2090–2099, numerator), and the present-day reference (2000–2009, denominator).
As a results of changes in temperature and nutrients, gross primary production increases in both western and eastern surface waters (as already suggested in Lazzari et al., 2014), with higher absolute variation in the west, but its increase is more than compensated by an increase in total respiration (absolute values), so that the epipelagic layer concentration of total carbon associated to living component (plankton) decreases (around −5% at west, −6 % at east), similarly to chlorophyll (−5% at west, −3% at east) and oxygen concentration (−6% at west, −4% at east).
In the mesopelagic layer, where primary production is not occurring, the decrease in living carbon component is even higher, reaching 15 and 20% in the western and eastern sub-basin, respectively. Changes are up to 30 and 70% in the deep layer, tough there the absolute values are two order of magnitude lower than in the mesopelagic layer.
DIC concentration increases everywhere, as a result of increased respiration, but also because of the increase in atmospheric CO2 and of the changes in CO2 solubilization driven by changes in temperature and salinity (see also Richon et al., 2019), with higher changes in the epipelagic layer and variation in the eastern part larger than that in the western one.
Similarly, pH decreases everywhere, but acidity variations are more marked in the epipelagic layer (−3.5%) and in the eastern basin, where significant changes are projected also in the bathypelagic part of the basin. The basin-scale reduction in pH results of about 0.3 in a century, and therefore similar to what is projected in the global ocean (Bopp et al., 2013; Jiang et al., 2019; and recently reviewed by Kwiatkowski et al., 2020), even if the MS alkalinity is higher than in the ocean. In fact, the relevant processes acting to change alkalinity level (namely evaporation/dilution and -to some extent- rivers input) also cause a corresponding change in DIC concentration, and the two factors have opposite effects on pH. It is evident that those change are a significant hazard for the Mediterranean marine ecosystems (Zunino et al., 2019, 2021).
The analysis of the alkalinity time series (not shown) reveals the presence of a small negative trend in the upper layers (values of around 0.6 mmol/m3/y Table 2), which is induced by changes in the Gibraltar boundary condition derived by the global ocean model, possibly resulting from a freshening of the Northern Atlantic induced by Greenland and Artic ice melting, or from changes in the ocean general circulation pattern close to the area (also see Reale et al., 2020).
The differential behavior of eastern and western basin may be also related to the weakening of the thermohaline circulation in the MS (as already observed by Adloff et al., 2015, who analyzed the same physical forcing adopted in this work), with the eastern basin being more sensitive to the strength reduction of the circulation deep cell.
The analysis of spatial distribution of changes at the regional and sub-basins level is not the major focus of the present manuscript, but it might be worth highlighting a few major features in the surface layer (Figure 5).
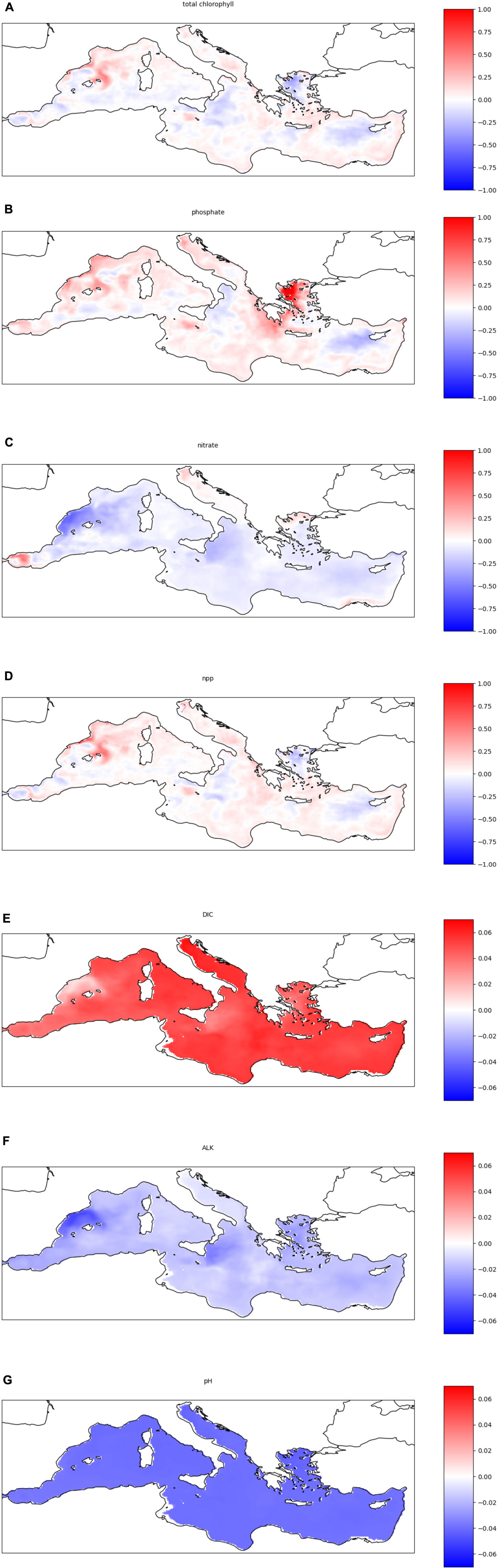
Figure 5. Anomaly maps of biogeochemical properties averaged over the first 25 m depth, obtained as a difference between the 2090–2099 decade minus the 2000–2009 decade normalized over the latter. Positive values indicate a future increase in the considered property. (A) Anomaly maps of biological variables and nutrients: (A) total chlorophyll (summed over the four planktonic groups), inorganic nutrients (B) phosphate and (C) nitrate; (D) plankton primary production; (E) dissolved inorganic carbon (DIC), (F) alkalinity (ALK) and (G) pH.
While the changes in pH are homogenous all over the basin, some heterogeneity is present in the alkalinity and DIC, which show variations of opposite sign and are generally spatially correlated. A larger degree of spatial variability is observed in nutrient concentrations, plankton primary production and chlorophyll density.
Indeed, from visual inspection of Figure 5 it is possible to recognize widespread positive anomaly regions in phosphate concentrations in the north-western part of the basin, the Adriatic Sea, large part of the Ionian sea, and the Aegean sea, which are contrasted by negative regions in the Levantine basin from the Rhodus gyre till Cyprus.
The primary production anomaly map is well correlated to the phosphate one, suggesting the presence of phosphorus limitation in plankton growth in most of the above-mentioned area, with the notable exception of the Aegean Sea, where plankton production decreases even if the phosphate concentration increases, possibly suggesting nitrogen limitation. The correlation between phosphate and primary production anomalies is observable also at smaller scales, such as the production hotspot in the Sicily channel or close to the Balearic islands.
The spatial distribution of total chlorophyll concentration resembles the primary production one, while nitrate concentration shows a general decrement, suggesting that nitrogen is not the limiting nutrient in most of the basin. Conversely, the pattern in the spatial distribution of nitrate anomaly might be co- shaped also by the uptake of nitrogen related to phosphorus-limited plankton growth.
It is clear, anyway, that different choices of future scenarios of land use and land changes might produce significant changes in the spatial pattern of nutrients, and as a consequence in plankton productivity ones. As an additional lateral comment, our model results (not shown here) point to the presence of different responses to climate scenarios from different plankton functional groups, with cascading consequences on different zooplankton groups. This result, however, requires a deeper analysis to be developed further and cannot be expanded in the present manuscript.
The effect of the increasing CO2 atmospheric concentration is well captured also in Figure 6, representing the depth distribution along a west-east transect of the DIC anomaly, obtained as the difference between future (2090–2099 decadal means) and present (2000–2009) DIC concentration. The increment in atmospheric concentration forces higher CO2 dissolution, resulting in higher concentration of surface DIC, which in turn drives significantly higher downward fluxes of DIC. The increment in DIC fluxes and concentrations is well recognizable down to 1,500 m, and more marked in the eastern sub-basin than in the eastern one. Carbon vertical fluxes can be related to the superposition and the cumulated effect of changes in solubility (solubility pump), vertical mixing and advection (physical pump), fluxes related to sinking of particulate organic carbon (biological pump), but also to the fluxes related to dense water formation and cascading along the continental shelves (continental shelf pump). Indeed, the strong increment all over the first 1,500 m between 20°E and 25°E is related to the spreading of the DIC rich dense water along the Adriatic and the Aegean shelves.
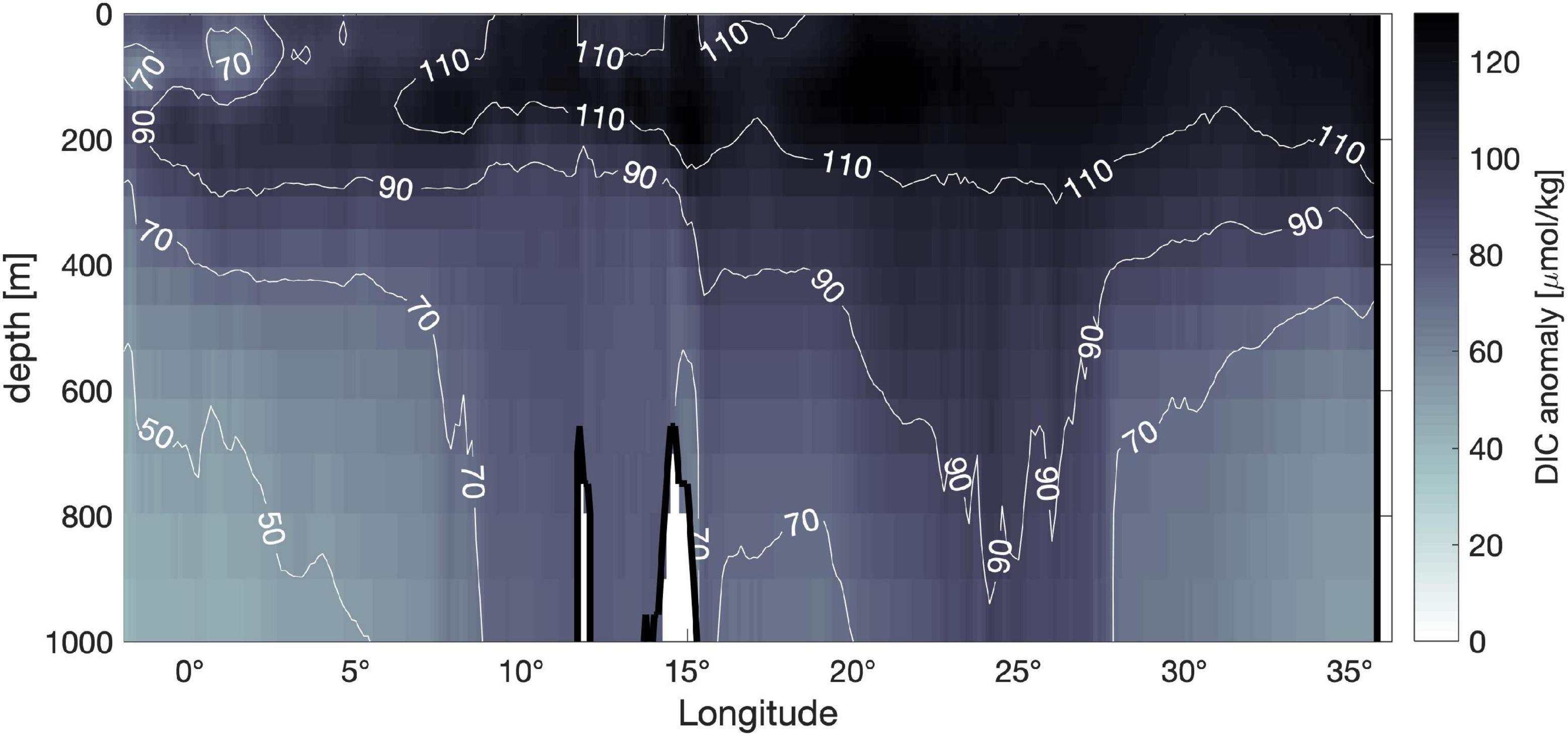
Figure 6. Depth distribution of DIC anomaly along a west-east transect, obtained as the difference between the 2090–2099 decade minus the 2000–2009 decade. Positive values indicate a future increase in DIC concentration.
Carbon Budgets
The analysis of our simulation also enables us to assess changes in the budgets of carbon stocks and fluxes for present and end of century conditions. Figure 7 summarizes the present situation (Figures 7A,B) and the main changes projected for the considered climate scenario (Figures 7C,D), both in terms of variable concentration and of variable total mass. In fact, while the budgets are assessed in terms of the distribution of the total mass of carbon through the different compartment, the physical and biogeochemical processes that support the mass transfer between compartments are often driven by differences in variable concentrations.
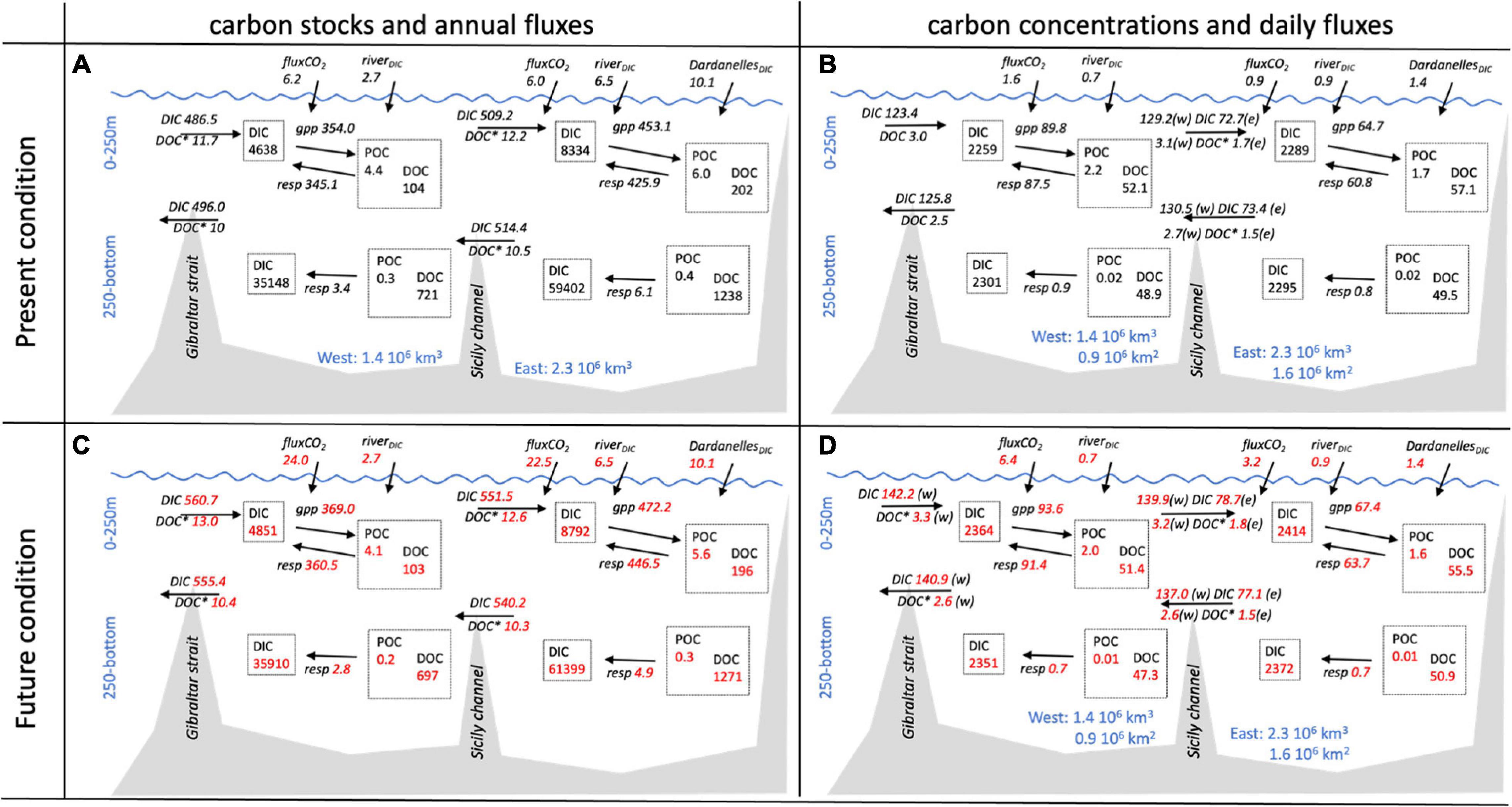
Figure 7. Carbon budgets (A,C) and carbon concentrations (B,D) in the MS subdivided into two layers (0–250 m and 250-bottom) and two sub-basins for the present day (A,B) and future (C,D) conditions. In (A,C) the stocks values (boxes) and fluxes (arrows) are expressed in TgC and FgC/y, respectively. In (C,D) the concentration values and fluxes (arrows) are expressed in mmol/m3 and mmol/m2/d where the normalization is performed on the volume and surface of the two sub-basins. Gross primary production (gpp) and respiration (resp) contributions are also included in the budget. The fluxes across the Sicily channel are normalized by the surface of both the western (w) and the eastern (e) sub-basin. (*) indicates that DOC is reported only for its semi-refractory component.
The simulation of the present day conditions shows the well-known east–west gradient in surface water POC concentration (Figure 7B), resulting from the superposition of the estuarine inverse circulation (defined by the inflow of surface water at Gibraltar Strait and a west to east advection driven flux, and the opposite east to west intermediate and deep fluxes) and the sinking of particulate organic carbon (the biological pump), which transfers carbon from the surface layer to the intermediate one, and while doing so reduces the entity of the eastward carbon flux (Crise et al., 1999; Crispi et al., 2001).
Model projections for future conditions indicate an increase in external inputs from Gibraltar and atmospheric fluxes (Figures 7C,D), which combined with changes in biological activity and internal circulations results in a reversal of the net global Atlantic-Mediterranean exchange, which changes from a slightly negative balance (export carbon to the Atlantic) to a slightly positive one (import carbon from the Atlantic). Globally, the system is slightly richer in carbon, with slightly higher horizontal exchanges among sub-basins, and higher total eastern carbon fluxes.
By contrast, the stock of organic particulate carbon, POC, and of surface dissolved organic carbon will decrease all over the basin (8%), as will the stock of bottom POC (Figures 7A,C), possibly because of the reduction in sinking from surface water and of the increase in respiration.
At bottom, the scenario projects an increase of the pool of dissolved organic carbon at east (1%) and a decrease at west (−3%) as a response of the thermohaline transport weakening, as indicated by the flux of DOC at the Sicily Strait.
The largest increment of the carbon pool is observed in the stock of dissolved inorganic carbon at bottom (+2,759 TgC) whereas the increase at surface is of 671 TgC (Figures 7A,C) even if the increase of the concentration is about 5% at surface and 2% at surface and bottom, respectively, as a result of the slow deepward invasion of absorbed atmospheric CO2 (+360 and 400% in the eastern and western sub-basins, respectively. The eastern sub-basin accumulated more than 85% of the newly assimilated carbon, because its volume is 60% greater and its rate of accumulation in the deeper layer per unit of surface is 50% higher than the western one (Figures 7B,D). The higher carbon accumulation efficiency in accumulation is a direct consequence of the lowering of the intensity of the thermohaline circulation.
The analysis of the metabolic fluxes (arrows in Figure 7) reveals that, in spite of the increase of atmospheric CO2 input and the increase in temperature which will fuel ecosystem metabolism, the increment in the respiration processes appear to be more relevant than the increment in photosynthesis, with the final results of smaller concentrations in POC and total organic matter, and higher DIC concentration and ocean acidity.
Conclusion
A set of Mediterranean Sea biogeochemical simulations forced by a high greenhouse gas emission scenario projects a basin-scale increment of the nutrient pools in the epipelagic and mesopelagic layers, an increment in surface water temperature, an increase in CO2 fluxes from the atmosphere, an increase of DIC inflow from Gibraltar Strait. As a result there is an increment in DIC all over the basin and an increase in gross primary production, which, however, is more than compensated by the increase in the system total respiration, so that globally there is a decrement in the concentrations of total living carbon, chlorophyll and oxygen in the epipelagic layer. The POC fraction also decreases, because of the increased respiration in the upper layer and of the reduced vertical transport in terms of sinking, as will -even if to a smaller extent- the DOC fraction. The decrease in the concentration of total living carbon concentration is even more significant in the mesopelagic layer.
Nutrients concentration, primary production and respiration all increase more in the western sub-basin than in the eastern one, but the plankton primary production is large enough to deplete the nutrient stocks in the surface layers, giving rise (in this land use scenario) to phosphorus limitation to plankton growth in most areas. sub-basins. Because of the interplay between primary production and respiration, the decrease in the concentration of total living carbon will decrease everywhere, but more in the eastern sub-basin than in the western one. The increment in surface DIC related to the increasing CO2 atmospheric concentration drives a downward flux of DIC supported by the solubility, physical and biological pumps, but also by the effects of the dense water formation and of the continental shelf pumps acting along the Adriatic and the Agean seas.
In terms of mass budgets, the increase in the DIC compartment at the bottom constitutes the largest contribute in the MS carbon budget at the end of the century, with the eastern sub-basin accumulating more than 85% of the absorbed atmospheric CO2, while the major changes in the exchanges through the boundaries occur at the air-water surface, where the increase in atmospheric CO2 forces a large increase in CO2 dissolution in surface water. The horizontal carbon exchange between the two sub-basins also increases.
Finally, even if alkalinity in the MS is higher than in the ocean, a clear ocean acidification signal is observed all over the basin. In fact, the acidification rate is similar to those observed in most oceans, the major processes acting to change alkalinity level also cause a corresponding change in DIC concentration, and the two processes have opposing effects on pH, while CO2 invasion affects only DIC. The acidification signal is well detectable down to the mesopelagic and bathypelagic layers, and potentially able to impact Mediterranean surface and deep-sea ecosystems.
Data Availability Statement
The raw data supporting the conclusion of this article will be made available by the authors, without undue reservation.
Author Contributions
CS and SSa contributed to the conception and design of the study and wrote the first draft of the manuscript with the contributions from all authors. PL, SSo, GC, GG, and GB organized the database and performed the simulation. All authors contributed to manuscript revision, read, and approved the submitted version.
Funding
This study has been co-sponsored by the MEDSEA EU project, the COCONET EU project, and the grant “Cambiamenti Climatici in Friuli Venezia Giulia” of the Regione Friuli Venezia Giulia (Italy).
Conflict of Interest
The authors declare that the research was conducted in the absence of any commercial or financial relationships that could be construed as a potential conflict of interest.
Publisher’s Note
All claims expressed in this article are solely those of the authors and do not necessarily represent those of their affiliated organizations, or those of the publisher, the editors and the reviewers. Any product that may be evaluated in this article, or claim that may be made by its manufacturer, is not guaranteed or endorsed by the publisher.
Acknowledgments
GG was supported by OGS and CINECA under HPC-TRES program award number 2015-05. This study has been conducted using data from the OGS National Oceanographic Data Center (NODC-OGS) and information from the E. U. Copernicus Marine Service. The physical NEMOMED8 simulation has been run on the Meteo-France supercomputer center (Toulouse, France) as a contribution to the Med-CORDEX initiative (www.medcordex.eu). We would like to thank F. Sevault for running the NEMOMED8 simulation and sharing the forcing dataset.
References
Adloff, F., Somot, S., Sevault, F., Jordà, G., Aznar, R., Déqué, M., et al. (2015). Mediterranean Sea response to climate change in an ensemble of twenty first century scenarios. Clim. Dyn. 45, 2775–2802.
Albouy, C., Guilhaumon, F., Leprieur, F., Lasram, F. B. R., Somot, S., Aznar, R., et al. (2013). Projected climate change and the changing biogeography of coastal Mediterranean fishes. J. Biogeogr. 40, 534–547. doi: 10.1111/jbi.12013
Anderson, T. R. (2005). Plankton functional type modelling: running before we can walk? J. Plankton Res. 27, 1073–1081. doi: 10.1093/plankt/fbi076
Beuvier, J., Sevault, F., Herrmann, M., Kontoyiannis, H., Ludwig, W., Rixen, M., et al. (2010). Modelling the Mediterranean Sea interannual variability over the last 40 years: focus on the EMT. JGR Ocean 115:C08017. doi: 10.1029/2009JC005950
Boero, N., Foglini, F., Fraschetti, S., Goriup, P., Macpherson, E., Planes, S., et al. (2016). CoCoNet: towards coast to coast networks of marine protected areas (from the shore to the high and deep sea), coupled with sea-based wind energy potential Scires-it. SCIRES-IT 6, 1–95.
Bopp, L., Resplandy, L., Orr, J. C., Doney, S. C., Dunne, J. P., Gehlen, M., et al. (2013). Multiple stressors of ocean ecosystems in the 21st century: projections with CMIP5 models. Biogeosciences 10, 6225–6245. doi: 10.5194/bg-10-6225-2013
Canu, D. M., Solidoro, C., Cossarini, G., and Giorgi, F. (2010). Effect of global change on bivalve rearing activity and the need for adaptive management. Clim. Res. 42, 13–26. doi: 10.3354/cr00859
Cossarini, G., Feudale, L., Teruzzi, A., Bolzon, G., Coidessa, G., Solidoro, C., et al. (2021). High-resolution reanalysis of the Mediterranean Sea biogeochemistry (1999-2019). Front. Mar. Sci. 8:741486. doi: 10.3389/fmars.2021.741486
Cossarini, G., Libralato, S., Salon, S., Gao, X., Giorgi, F., and Solidoro, C. (2008). Downscaling experiment for the Venice lagoon. II. Effects of changes in precipitation on biogeochemical properties. Clim. Res. 38, 43–59.
Cossarini, G., Querin, S., and Solidoro, C. (2015). The continental shelf carbon pump in the northern Adriatic Sea (Mediterranean Sea): influence of wintertime variability. Ecol. Modell. 314, 118–134. doi: 10.1016/j.ecolmodel.2015.07.024
Costanza, R., d’Arge, R., De Groot, R., Farber, S., Grasso, M., Hannon, B., et al. (1997). The value of the world’s ecosystem services and natural capital. Nature 387, 253–260.
Crise, A., Allen, J. I., Baretta, J., Crispi, G., Mosetti, R., and Solidoro, C. (1999). The Mediterranean pelagic ecosystem response to physical forcing. Prog. Oceanogr. 44, 219–243. doi: 10.1016/S0079-6611(99)00027-0
Crise, A., Crispi, G., and Mauri, E. (1998). A seasonal three-dimensional study of the nitrogen cycle in the Mediterranean Sea: part I. Model implementation and numerical results. J. Mar. Syst. 18, 287–312. doi: 10.1016/s0924-7963(98)00016-5
Crispi, G., Mosetti, R., Solidoro, C., and Crise, A. (2001). Nutrients cycling in Mediterranean basins: the role of the biological pump in the trophic regime. Ecol. Modell. 138, 101–114. doi: 10.1016/s0304-3800(00)00396-3
Cuttelod, A., García, N., Malak, D. A., Temple, H. J., and Katariya, V. (2009). The Mediterranean: A Biodiversity Hotspot Under Threat. Wildlife in a Changing World–an analysis of the 2008 IUCN Red List of Threatened Species. Gland: IUCN, 89.
Darmaraki, S., Somot, S., Sevault, F., Nabat, P., Cabos, W., Cavicchia, L., et al. (2019a). Future evolution of marine heat waves in the Mediterranean Sea. Clim. Dyn. 53, 1371–1392. doi: 10.1007/s00382-019-04661-z
Darmaraki, S., Somot, S., Sevault, F., and Nabat, P. (2019b). Past variability of Mediterranean Sea marine heatwaves. Geophys. Res. Lett. 46, 9813–9823. Special Section: The CNRM Climate and Earth System Models for CMIP6, doi: 10.1029/2019GL082933
Foujols, M.-A., Lévy, M., Aumont, O., and Madec, G. (2000). OPA 8.1 Tracer Model Reference Manual (Note du Pole de Modélisation) France. Guyancourt: Institut Pierre-Simon Laplace (IPSL), 45.
Fraysse, M., Pinazo, C., Faure, V. M., Fuchs, R., Lazzari, P., Raimbault, P., et al. (2013). Development of a 3D coupled physical-biogeochemical model for the Marseille coastal area (NW Mediterranean Sea): what complexity is required in the coastal zone? PLoS One 8:e80012. doi: 10.1371/journal.pone.0080012
Galli, G., Solidoro, C., and Lovato, T. (2017). Marine heat waves hazard 3D maps and the risk for low motility organisms in a warming Mediterranean Sea. Front. Mar. Sci. 4:136. doi: 10.3389/fmars.2017.00136
Geider, R. J., MacIntyre, H. L., and Kana, T. M. (1997). Dynamic model of phytoplankton growth and acclimation: responses of the bal-anced growth rate and the chlorophyll a: carbon ratio to light, nutrient-limitation and temperature. Mar. Ecol. Prog. Ser. 148, 187–200. doi: 10.3354/meps148187
Harrison, P. A., Harmáčková, Z. V., Karabulut, A. A., Brotons, L., Cantele, M., Claudet, J., et al. (2019). Synthesizing plausible futures for biodiversity and ecosystem services in Europe and Central Asia using scenario archetypes. Ecol. Soc. 24:27.
Herrmann, M., Estournel, C., Adloff, F., and Diaz, F. (2014). Impact of climate change on the northwestern Mediterranean Sea pelagic planktonic ecosystem and associated carbon cycle. J. Geophys. Res. Oceans 119, 5815–5836. doi: 10.1002/2014jc010016
Herrmann, M., Sevault, F., Beuvier, J., and Somot, S. (2010). What induced the exceptional 2005 convection event in the Northwestern Mediterranean basin? Answers from a modeling study. J. Geophys. Res. Oceans 115:C12051. doi: 10.1029/2010JC006162
Hipsey, M. R., Gal, G., Arhonditsis, G. B., Carey, C. C., Elliott, J. A., Frassl, M. A., et al. (2020). A system of metrics for the assessment and improvement of aquatic ecosystem models. Environ. Modell. Softw. 128:104697. doi: 10.1016/j.envsoft.2020.104697
Holt, J., Butenschön, M., Wakelin, S. L., Artioli, Y., and Allen, J. I. (2012). Oceanic controls on the primary production of the northwest European continental shelf: model experiments under recent past conditions and a potential future scenario. Biogeosciences 9, 97–117. doi: 10.5194/bg-9-97-2012
IPCC (2014). Climate Change 2014: Synthesis Report. Contribution of Working Groups I, II and III to the Fifth Assessment Report of the Intergovernmental Panel on Climate Change, eds Core Writing TeamR. K. Pachauri and L. A. Meyer Geneva: IPCC, 151.
Jiang, L., Carter, B. R., Feely, R. A., Lauvset, S. K., and Olsen, A. (2019). Surface ocean pH and buffer capacity: past, present and future. Sci. Rep. 9:18624. doi: 10.1038/s41598-019-55039-4
Kwiatkowski, L., Torres, O., Bopp, L., Aumont, O., Chamberlain, M., Christian, J. R., et al. (2020). Twenty-first century ocean warming, acidification, deoxygenation, and upper-ocean nutrient and primary production decline from CMIP6 model projections. Biogeosciences 17, 3439–3470.
Lamon, L., Rizzi, J., Bonaduce, A., Dubois, C., Lazzari, P., Ghenim, L., et al. (2014). An ensemble of models for identifying climate change scenarios in the Gulf of Gabes, Tunisia. Reg. Environ. Change 14, 31–40. doi: 10.1007/s10113-013-0430-x
Lavigne, H., D’ortenzio, F., d’Alcalà, M. R., Claustre, H., Sauzède, R., and Gacic, M. (2015). On the vertical distribution of the chlorophyll a concentration in the Mediterranean Sea: a basin-scale and seasonal approach. Biogeosciences 12, 5021–5039. doi: 10.5194/bg-12-5021-2015
Lazzari, P., Mattia, G., Solidoro, C., Salon, S., Crise, A., Zavatarelli, M., et al. (2014). The impacts of climate change and environmental management policies on the trophic regimes in the Mediterranean Sea: scenario analyses. J. Mar. Syst. 135, 137–149. doi: 10.1016/j.jmarsys.2013.06.005
Lazzari, P., Solidoro, C., Ibello, V., Salon, S., Teruzzi, A., Béranger, K., et al. (2012). Seasonal and inter-annual variability of plankton chlorophyll and primary production in the Mediterranean Sea: a modelling approach. Biogeosciences 9, 217–233. doi: 10.5194/bg-9-217-2012
Lazzari, P., Solidoro, C., Salon, S., and Bolzon, G. (2016). Spatial variability of phosphate and nitrate in the Mediterranean Sea: a modeling approach. Deep Sea Res. Part I Oceanogr. Res. Pap. 108, 39–52. doi: 10.1016/j.dsr.2015.12.006
Lejeusne, C., Chevaldonné, P., Pergent-Martini, C., Boudouresque, C. F., and Pérez, T. (2010). Climate change effects on a miniature ocean: the highly diverse, highly impacted Mediterranean Sea. Trends Ecol. Evol. 25, 250–260. doi: 10.1016/j.tree.2009.10.009
Ludwig, W., Dumont, E., Meybeck, M., and Heussner, S. (2009). River discharges of water and nutrients to the Mediterranean and BlackSea: major drivers for ecosystem changes during past and future decades? Progr. Oceanogr. 80, 199–217.
Macias, D. M., Garcia-Gorriz, E., and Stips, A. (2015). Productivity changes in the Mediterranean Sea for the twenty-first century in response to changes in the regional atmospheric forcing. Front. Mar. Sci. 2:79. doi: 10.3389/fmars.2015.00079
Melaku Canu, D., Ghermandi, A., Nunes, P. A., Lazzari, P., Cossarini, G., and Solidoro, C. (2015). Estimating the value of carbon sequestration ecosystem services in the Mediterranean Sea: an ecological economics approach. Glob. Environ. Change 32, 87–95.
Micheli, F., Halpern, B. S., Walbridge, S., Ciriaco, S., Ferretti, F., Fraschetti, S., et al. (2013). Cumulative human impacts on Mediterranean and Black Sea marine ecosystems: assessing current pressures and opportunities. PLoS One 8:e79889. doi: 10.1371/journal.pone.0079889
Middelburg, J. J., Soetaert, K., and Hagens, M. (2020). Ocean alkalinity, buffering and biogeochemical processes. Rev. Geophys. 58:e2019RG000681. doi: 10.1029/2019RG000681
Mignot, A., Claustre, H., Uitz, J., Poteau, A., D’Ortenzio, F., and Xing, X. (2014). Understanding the seasonal dynamics of phytoplankton biomass and the deep chlorophyll maximum in oligotrophic environments: a Bio-Argo float investigation. Glob. Biogeochem. Cycles 28, 856–876.
Myers, N., Mittermeier, R. A., Mittermeier, C. G., Da Fonseca, G. A., and Kent, J. (2000). Biodiversity hotspots for conservation priorities. Nature 403, 853–858. doi: 10.1038/35002501
Oreskes, N., Shrader-Frechette, K., and Belitz, K. (1994). Verification, validation, and confirmation of numerical models in the earth sciences. Science 263, 641–646. doi: 10.1126/science.263.5147.641
Orr, J. C. (1999). Ocean Carbon-Cycle Model Intercomparison Project (OCMIP): Phase I (1995–1997). GAIM Report, 7. Durham, NH: IGBP/GAIM office, EOS University of New Hampshire.
Pagès, R., Baklouti, M., Barrier, N., Ayache, M., Sevault, F., Somot, S., et al. (2020). Projected effects of climate-induced changes in hydrodynamics on the biogeochemistry of the Mediterranean Sea under the RCP 8.5 regional climate scenario. Front. Mar. Sci. 7:563615. doi: 10.3389/fmars.2020.563615
Reale, M., Salon, S., Somot, S., Solidoro, C., Giorgi, F., Crise, A., et al. (2020). Influence of large-scale atmospheric circulation patterns on nutrient dynamics in the Mediterranean Sea in the extended winter season (October-March) 1961-1999. Clim. Res. 82, 117–136.
Richon, C., Dutay, J.-C., Bopp, L., Le Vu, B., Orr, J. C., Somot, S., et al. (2019). Biogeochemical response of the Mediterranean Sea to the transient SRES-A2 climate change scenario. Biogeosciences 16, 135–165. doi: 10.5194/bg-16-135-2019
Salon, S., Cossarini, G., Bolzon, G., Feudale, L., Lazzari, P., Teruzzi, A., et al. (2019). Novel metrics based on Biogeochemical Argo data to improve the model uncertainty evaluation of the CMEMS Mediterranean marine ecosystem forecasts. Ocean Sci. 15, 997–1022.
Salon, S., Cossarini, G., Libralato, S., Gao, X., Solidoro, C., and Giorgi, F. (2008). Downscaling experiment for the Venice lagoon. I. Validation of the present-day precipitation climatology. Clim. Res. 38, 31–41.
Sarmiento, J., Hughes, T., Stouffer, R. S., and Manabe, S. (1998). Simulated response of the ocean carbon cycle to anthropogenic climate warming. Nature 393, 245–249.
Schwalm, C. R., Glendon, S., and Duffy, P. B. (2020). RCP8.5 tracks cumulative CO2 emissions. Proc. Natl. Acad. Sci. U.S.A. 117, 19656–19657. doi: 10.1073/pnas.2007117117
Sevault, F., Somot, S., and Beuvier, J. (2009). A Regional Version of the NEMO Ocean Engine on the Mediterranean Sea: NEMOMED8 User’s Guide. Note de Centre n°107. Groupe de Météorologie de Grande Echelle et Climat. Mai 2009. Toulouse: CNRM.
Solidoro, C., Crise, A., Crispi, G., and Pastres, R. (2003). An a priori approach to assimilation of ecological data in marine ecosystem models. J. Mar. Syst. 40, 79–97. doi: 10.1016/s0924-7963(03)00014-9
Somot, S., Sevault, F., and Déqué, M. (2006). Transient climate change scenario simulation of the Mediterranean Sea for the 21st century using a high-resolution ocean circulation model. Clim. Dyn. 27, 851–879. doi: 10.1007/s00382-006-0167-z
Soto-Navarro, J. Jordá, G., Amores, A., Cabos, W., Somot, S., Sevault, F., et al. (2020). Evolution of Mediterranean Sea water properties under climate change scenarios in the Med-CORDEX ensemble. Clim. Dyn. 54, 2135–2165. doi: 10.1007/s00382-019-05105-4
Stow, C. A., Jolliff, J., McGillicuddy, D. J. Jr., Doney, S. C., Allen, J. I., Friedrichs, M. A., et al. (2009). Skill assessment for coupled biological/physical models of marine systems. J. Mar. Syst. 76, 4–15. doi: 10.1016/j.jmarsys.2008.03.011
Teruzzi, A., Bolzon, G., Salon, S., Lazzari, P., Solidoro, C., and Cossarini, G. (2018). Assimilation of coastal and open sea biogeochemical data to improve phytoplankton modelling in the Mediterranean Sea. Ocean Model. 132, 46–60.
Teruzzi, A., Di Cerbo, P., Cossarini, G., Pascolo, E., and Salon, S. (2019). Parallel implementation of a data assimilation scheme for operational oceanography: the case of the OGSTM-BFM model system. Comput. Geosci. 124, 103–114. doi: 10.1016/j.cageo.2019.01.003
Teruzzi, A., Dobricic, S., Solidoro, C., and Cossarini, G. (2014). A 3-D variational assimilation scheme in coupled transport biogeochemical models: forecast of Mediterranean biogeochemical properties. J. Geophys. Res. Oceans 119, 200–217. doi: 10.1002/2013JC009277
Teutschbein, J., and Seibert, C. (2012). Bias correction of regional climate model simulations for hydrological climate-change impact studies: review and evaluation of different methods. J. Hydrol. 45, 12–29.
Thingstad, T. F., and Rassoulzadegan, F. (1995). Nutrient limitations, microbial food webs, and “biological C-pumps”: suggested inter-actions in a P-limited Mediterranean. Mar. Ecol. Prog. Ser. 117, 299–306.
Thorpe, R. B., and Bigg, G. R. (2000). Modelling the sensitivity of Mediterranean outflow to anthropogenically forced climate change. Clim. Dyn. 16, 355–368. doi: 10.1007/s003820050333
UNWTO–World Tourism Organization (2015). Tourism in the Mediterranean, 2015 Edn. Madrid: 12. Available online at: https://www.e-unwto.org/doi/pdf/10.18111/9789284416929 (accessed December 15, 2021).
Zeebe, R. E. Wolf-Gladrow, D. (2001). CO2 in Seawater: Equilibrium, Kinetics, Isotopes (No. 65). Bremerhaven: Gulf Professional Publishing.
Zunino, S., Canu, D. M., Zupo, V., and Solidoro, C. (2019). Direct and indirect impacts of marine acidification on the ecosystem services provided by Coralligenous reefs and Seagrass systems. Glob. Ecol. Conserv. 18:e00625.
Keywords: acidification, carbon cycling, Mediterranean Sea, carbon budgets, model uncertainty, high CO2 scenario
Citation: Solidoro C, Cossarini G, Lazzari P, Galli G, Bolzon G, Somot S and Salon S (2022) Modeling Carbon Budgets and Acidification in the Mediterranean Sea Ecosystem Under Contemporary and Future Climate. Front. Mar. Sci. 8:781522. doi: 10.3389/fmars.2021.781522
Received: 22 September 2021; Accepted: 21 December 2021;
Published: 12 January 2022.
Edited by:
Susana Agusti, King Abdullah University of Science and Technology, Saudi ArabiaReviewed by:
George Triantafyllou, Hellenic Centre for Marine Research (HCMR), GreeceThibaut Wagener, Aix-Marseille Université, France
Marta Álvarez, Spanish Institute of Oceanography (IEO), Spain
Copyright © 2022 Solidoro, Cossarini, Lazzari, Galli, Bolzon, Somot and Salon. This is an open-access article distributed under the terms of the Creative Commons Attribution License (CC BY). The use, distribution or reproduction in other forums is permitted, provided the original author(s) and the copyright owner(s) are credited and that the original publication in this journal is cited, in accordance with accepted academic practice. No use, distribution or reproduction is permitted which does not comply with these terms.
*Correspondence: Cosimo Solidoro, csolidoro@inogs.it