The network of nitrifying and pathogenic prokaryotic interactions in a recirculating aquaculture system of a sole (Solea senegalensis) hatchery
- 1Instituto de Ciências Biomédicas Abel Salazar (ICBAS), University of Porto, Porto, Portugal
- 2Interdisciplinary Centre of Marine and Environmental Research (CIIMAR), University of Porto, Porto, Portugal
- 3Safiestela S.A., SEA EIGHT Group, Póvoa de Varzim, Portugal
- 4Faculty of Sciences, University of Porto (FCUP), Porto, Portugal
- 5School of Science, University of Waikato, Hamilton, New Zealand
Prokaryotic interactions in recirculating aquaculture systems (RAS) environmental communities may play a crucial role in driving their functional potential. However, these interactions are often neglected. The aim of this work is to detect prokaryotic interactions in a Solea senegalensis RAS, through the definition of relevant taxa and cluster identification using co-variance and co-occurrence tools. Amplicon sequence variants (ASV) were obtained from the water, tank biofilm, and biofilters of two systems, pre-ongrowing and weaning, and the study focuses on two microbial target groups, the potentially pathogenic and the nitrifying prokaryotes. No significant negative correlations were found with any target ASVs, indicating a mostly cooperative environment. As expected, ammonia oxidizing bacteria (Nitrosomonas) and archaea (Candidatus Nitrosopumilus) were found to be positively interacting with the nitrite oxidizing bacteria Nitrospira. However, no interactions were found between them, and results hint at a niche differentiation based on ammonia competition. Nitrospira also showed subcommunities with no ammonia oxidizing archaea or ammonia oxidizing bacteria correlations, hinting at a separate functional role of complete ammonia oxidation to nitrate for some Nitrospira ASVs. Two taxa commonly associated with pathogenic outbreaks, Tenacibaculum and Vibrio, had a significant positive correlation in one of the systems. With no outbreaks reported, this association may prove relevant in disease preventions and to improve outbreak predictions. Future studies may further elucidate this interaction, as cumulative evidence is still needed to better understand this correlation.
Introduction
Sole aquaculture, particularly of the species Solea senegalensis, has high potential for aquaculture diversification in southern Europe (Morais et al., 2016). With disease outbreaks identified as one of the main challenges in sole farms (Howell, 1997), the industry has turned to Recirculating Aquaculture Systems (RAS), that enable a tight control on the environmental parameters and eliminate contact with wild species. This type of system has the additional advantage of making intensive aquaculture compatible with environmental sustainability objectives, since it allows a reduction in water usage and an improvement in waste management and nutrient recycling (Piedrahita, 2003; Martins et al., 2010).
Monitoring the composition of bacterial communities established in aquaculture systems is of paramount importance to evaluate their potential to serve as reservoirs for pathogenic bacterial strains (Rud et al., 2017; Canada et al., 2020). This community influences the water quality and health of the fish being reared in the system (Blancheton et al., 2013) with a complex network of microbial roles (Martínez-Porchas and Vargas-Albores, 2017). Alterations to this structure, translated in changes in bacterial diversity, have been linked to animal performance and health (Infante-Villamil et al., 2020). One of the main outcomes, dysbiosis, has been defined as a shift in the structure of a commensal community that may result in perturbations in the immune system and mediated diseases (Petersen and Round, 2014). Among the diverse roles microbial communities play in RAS, the presence/absence of pathogens and active nitrogen cycling are crucial for fish health and well-being.
Despite technological improvements, disease outbreaks are still one of the great concerns in sole aquaculture management, particularly of the bacterial disease flexibacteriosis (Tenacibaculum maritimum) (Toranzo et al., 2005). A previous study (Wynne et al., 2020) reported high relative abundance of T. maritimum in healthy fish and suggested that this is a complex multifactorial disease, and the interactions with other taxa may play a key role in disease progression.
A vital feature of a RAS is the conversion of ammonia (a metabolite from protein catabolism) to nitrate in the nitrification process, performed by a microbial community incubated in the biofilter carriers (Ebeling et al., 2002). Nitrification is the naturally occurring pathway where ammonia oxidizing bacteria (AOB) oxidize ammonia to nitrite, and coupled to this reaction, nitrite oxidizing bacteria (NOB) oxidize nitrite to nitrate (Sharma and Ahlert, 1977), thus removing ammonia from the system. Some of the main genera that have been identified as performing the nitrification process in marine biofilters are the AOB Nitrosomonas (Paungfoo et al., 2007; Foesel et al., 2008) and Nitrosococcus (Foesel et al., 2008) and the NOB Nitrospira (Tal et al., 2003; Foesel et al., 2008). Previous studies (Brailo et al., 2019) have found, through Next Generation Sequencing (NGS) techniques, bacteria with the capacity to carry out ammonia and nitrite oxidation in a RAS nitrification biofilter. Active biofilters have also shown to possess a more diverse community when compared with water samples from the same systems (Ruan et al., 2015; Almeida et al., 2021). One of the challenges in managing a RAS microbial community is the competition between chemoautotrophic nitrifiers (such as AOB and NOB) and heterotrophs for oxygen, nutrients, and space (Michaud et al., 2006) in the biofilter. A disbalance could lead to an increase in ammonia and nitrite concentrations in detriment of the nitrifying populations (Blancheton et al., 2013). As a sector for optimal, but undifferentiated, bacterial growth, there is a risk that disruptions in the system may cause pathogenic outbreaks by opportunist bacteria (Blancheton et al., 2013).
In general, microbiomes are strongly influenced by the multiplicity of ecological processes that affect community assembly (Goldford et al., 2018), such as selective pressures and nutrient availability, generating cross-feeding networks. In these, microbes communicate and trade metabolites and services (Marx, 2009). RAS develop complex and dynamic microbial ecosystems, with differential composition between systems and matrices (Almeida et al., 2021). Co-variance network models are useful to infer the complex taxonomic architectures of these communities in their multitude of ecological processes (Goldford et al., 2018) by attributing to targeted taxa (nodes) the connections between them (edges) (Newman, 2003). Previous studies in freshwater RAS have used this method to conclude that interactions decrease in complexity during the biofilter start-up, after an initial peak (Jiang et al., 2019). Biofilters have been found to have more nodes but less interactions than biofloc reactors used in wastewater treatment (Deng et al., 2019). These, in turn, have been found to be more complex than water (Wei et al., 2020). Interestingly, a recent study revealed a competitive interaction between taxa responsible for ammonia removal and nitrate removal processes (Deng et al., 2021), justifiable by different niche requirement.
In this work, we targeted our networking analysis to two groups as important model microorganisms for RAS sustainability: potentially pathogenic and nitrifying prokaryotes. For this analysis, hub taxa are defined as highly connected with other taxa (Faust et al., 2012) and cosmopolitan taxa have a wide-spread occurrence across different environments, linked to a tendency to form positive connections (Faust et al., 2015).
The biotic relationships between microorganisms present in these communities may play a crucial role in driving their functional potential. However, these interactions are often neglected in engineered environments. The aim of this work is to detect prokaryotic interactions among relevant taxa in RAS, using network correlations and cluster identification.
Methods
Sample collection and DNA extraction
This study was performed in partnership with an aquaculture production unit, a sole hatchery (Safiestela S.A.), located in Estela, Portugal. The analysis was performed using the dataset from Almeida et al. (2021), details about the equipment used for physical, chemical, and biological description of the collected samples are presented in the mentioned paper. Samples of water column, tank biofilm and biofilter carrier were collected from the two parallel recirculating systems: pre-ongrowing (PO) and weaning (WE) (Figure 1). Total DNA was isolated from the water column using the DNeasy PowerWater Sterivex DNA Isolation Kit (QIAGEN, Merck KGaA, Darmstadt, Germany), following the manufacturer instructions. In tank wall biofilm and biofilter carrier samples, the DNA was isolated with DNeasy Power Soil Kit (QIAGEN, Merck KGaA, Darmstadt, Germany) with some adaptations to the manufacturer protocol. For the biofilter carriers, to ensure maximum release of biofilm from the matrix, additional steps were performed before starting the extraction protocol: biofilter carriers were centrifuged inside 15 mL tubes for 15 min at maximum speed (4300 g), followed by a quick vortex and additional 5 min centrifugation. For both biofilter carriers and tank biofilm samples, additional beads were added to facilitate cell lysis.
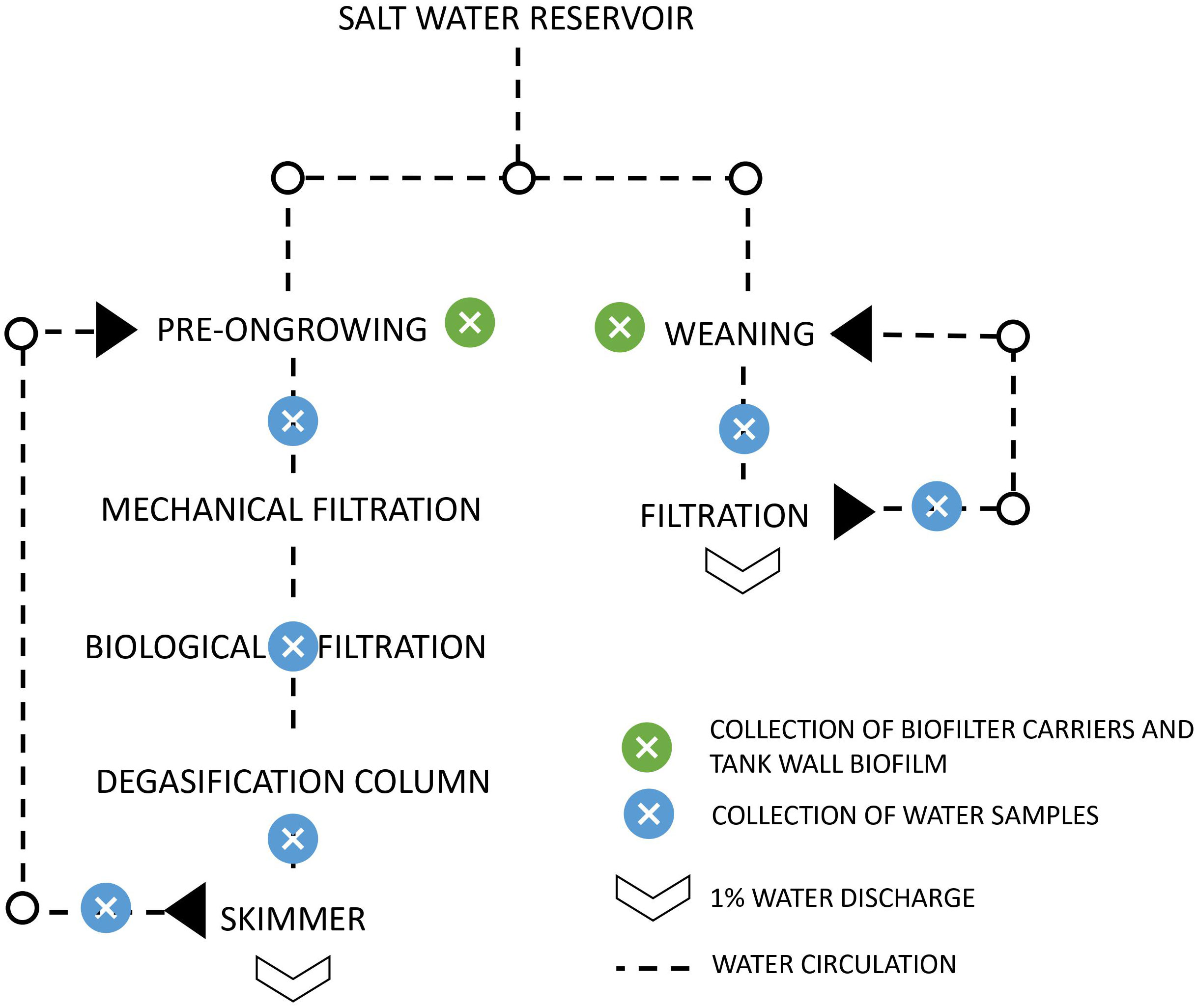
Figure 1 Representation of the aquaculture unit in study. The pre-ongrowing and weaning operate with water recirculation.
The V4-V5 region (515F/926R primers, amplicon size approximately 410 bp) of the 16S rRNA gene (Caporaso et al., 2011; Caporaso et al., 2012; Apprill et al., 2015; Parada et al., 2016) was used for the NGS Analysis. The PCR protocol involved a 3 min denaturation step at 95°C, followed by 25 cycles of 98°C for 20 s, 60°C for 30 s and 72°C for 30 s and a final extension at 72°C for 5 min. PCR products were purified and normalized using SequalPrep 96-well plate kit (ThermoFisher Scientific, Waltham, USA) (Comeau et al., 2017), pooled and pair-end sequenced in the Illumina MiSeq® sequencer at Genoinseq (Cantanhede, Portugal). Sequence data was pre-processed at Genoinseq (Cantanhede, Portugal), by exporting sequences in fastq format, quality filtering with PRINSEQ version 0.20.4 (Schmieder and Edwards, 2011) removing sequencing adapters and reads with less than 100 bases, and trimming bases with an average quality lower than Q25 in a window of 5 bases. Forward and reverse reads were merged by overlapping paired-end reads with AdapterRemoval version 2.1.5 using default parameters.
Bioinformatic and statistics
To obtain the amplicon sequence variant (ASV) table, the DADA2 pipeline was implemented on our filtered sequences dataset. This was done using R 3.6.1 (R Core Team, 2019) and the package dada2 (v1.12.1). For taxonomic attribution, the SILVA database version 132 was used (Quast et al., 2012). Taxa classified at the Kingdom level as Eukaryota, at the Order level as Chloroplast and at the Family level as Mitochondria were removed. The water column, tank biofilm and biofilter carrier overall prokaryotic diversity and composition analysis is described in Almeida et al. (2021).
The biotic relationship between microorganisms was inferred using eLSA software (Xia et al., 2011; Xia et al., 2013) and the Spearman correlation coefficient (SCC) between ASVs pairs (Spearman, 1987). To avoid misleading correlations, the ASV table was pre-filtered to remove low frequency ASVs (not present in at least three samples). Samples were split by system (pre-ongrowing and weaning) before the correlation analysis to avoid spurious autocorrelations. The correlations set obtained for each system was trimmed to include only significant interactions (SCC > |0.7|, p-value < 0.001). Network vertexes were selected based on a significant correlation with one (or more) of the ASVs belonging to the targeted pathogenic and nitrifying genera (taxa identification described below). Correlation networks were plotted in the R environment version 4.1.2 (R Core Team, 2019) using iGraph v. 1.2.7 (Csardi and Nepusz, 2006) and sub-communities were identified with the Louvain algorithm (Blondel et al., 2008). Vertexes with 0 significant correlations (degrees) were removed.
As mentioned in the introduction, this study was based on the definition of two target groups in a recirculating aquaculture system, the potentially pathogenic and nitrifying, the later subdivided into ammonia oxidizing bacteria (AOB), nitrite oxidizing bacteria (NOB) and ammonia oxidizing archaea (AOA). The selection was based on the taxonomic attribution. Potentially pathogenic bacteria are those belonging to either the Tenacibaculum or Vibrio genus. For the nitrification target group, ASVs were identified at different taxonomic levels to include unclassified sequences, as described by (Semedo et al., 2021): Thaumarchaeota, Nitrospinae, Nitrospirae, and Nitrospinota were selected at the phylum level; Nitrosomonadaceae and Nitrosococcaceae at the family level; and Nitrosococcus, Nitrospirae, Nitrobacter, Candidatus Nitrotoga, Nitrotoga, Nitrospina, Nitrococcus, Nitrolancea, Candidatus Nitromaritima, and Nitromaritima at the genus level. However, only members of the families Nitrosococcaceae, Nitrosomonadaceae and Nitrospiraceae. The Nitrososphaeria class were identified. To evaluate the effect of the system and matrix variables on the abundance response, a two-way ANOVA test was performed, also in the R environment.
Results
The dataset generated for the downstream analysis has a minimum read count per sample (after trimming) of 10,396, a mean per sample of 28,850 and a max sample read count of 70,487. The complete list of read counts per sample and observed ASVs, before and after filtering (for nitrifying and pathogenic genus), can be found in Table S1.
Nitrifying and potentially pathogenic genus distribution
The relative distribution of the two target groups is presented in Figure 2, mean values per system and matrix can be found in Table S2. The potentially pathogenic genus Tenacibaculum (that includes a total of 81 ASVs) is most predominant in the weaning samples across all matrices (p-value < 0.05), while Vibrio (13 ASVs) seems to be associated with the water matrix, regardless of the RAS system (p-value < 0.05). The AOB Nitrosomonas (24 ASVs) is more present in the biofilm samples (both in the biofilter carriers and tank wall; p-value < 0.05), as well as the NOB Nitrospira (28 ASVs, p-value p-value < 0.05) and the AOA Candidatus Nitrosopumilus (11 ASVs, p-value p-value < 0.05). In these matrices, the average relative abundance of putative AOB and NOB are 3 and 2 times higher in the pre-ongrowing than the weaning system, respectively, while AOAs are 30 times higher in the weaning (being almost absent in the pre-ongrowing). Computed two-way ANOVA test p-values can be found in Table S3. The matrix appears to influence taxa presence and abundance. The genera Vibrio, Nitrospira and Nitrosomonas show a cosmopolitan nature between systems, as they show a similar distribution between them. With the inverse behaviour, Tenacibaculum and Candidatus Nitrosopumilus are shaped by the systems.
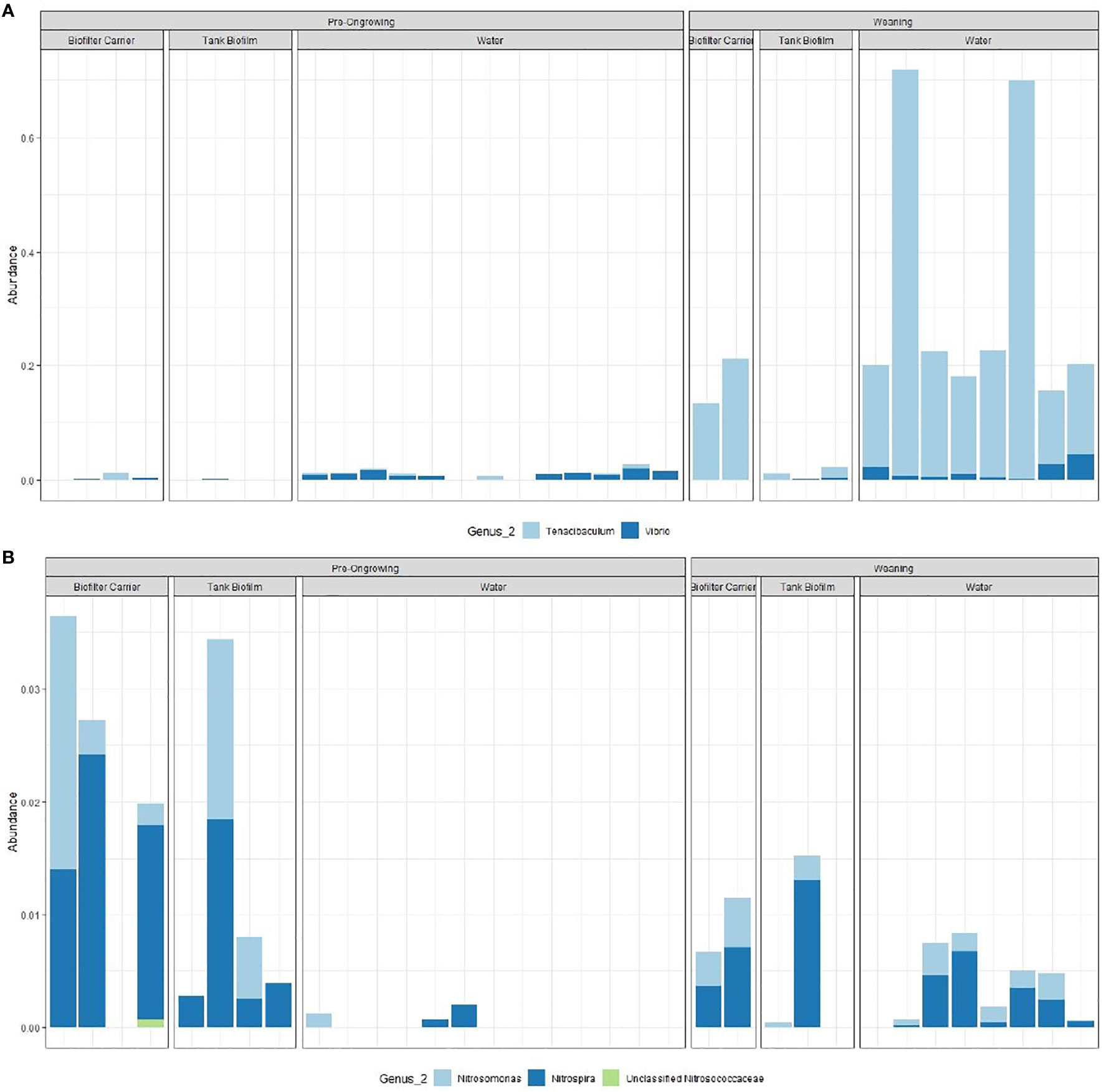
Figure 2 Relative distribution of the functional groups, genus associated with nitrification (A), the ammonia oxidizing bacteria (AOB, Nitrosomonas, Unclassified Nitrosococcaceae), nitrite oxidizing bacteria (NOB, Nitrospira) and ammonia oxidizing archaea (AOA, Candidatus Nitrosopumilus); and genus with potentially pathogenic activity (B, Vibrio and Tenacibaculum).
Inter-ASVs interactions in the pre-ongrowing system
The spearman correlation network in the pre-ongrowing system is composed by 343 ASVs (nodes/taxa) and 743 links (significant correlations) (Figure 3). Links were selected based on a significant correlation with one (or more) of the ASVs belonging to pathogenic or nitrifying genera. Nine subcommunities were identified with the multi-level modularity optimization algorithm. In this network, there is one genus associated with AOB, Nitrosomonas, with 2 ASVs and 86 correlations, and one genus associated with NOB, Nitrospira, with 7 ASVs and 629 correlations. The two genera associated with pathogenic outbreaks are also present in the pre-ongrowing network. Vibrio is present with 3 ASVs and 26 correlations, while Tenacibaculum has 2 ASVs with a total of 13 correlations. The remaining ASVs are classified between 110 genera in total. The complete summary of the pre-ongrowing community can be found in the Table S4-A, with the number of ASVs from the assigned ASVs, respective number of correlations and the mean number of correlations per node.
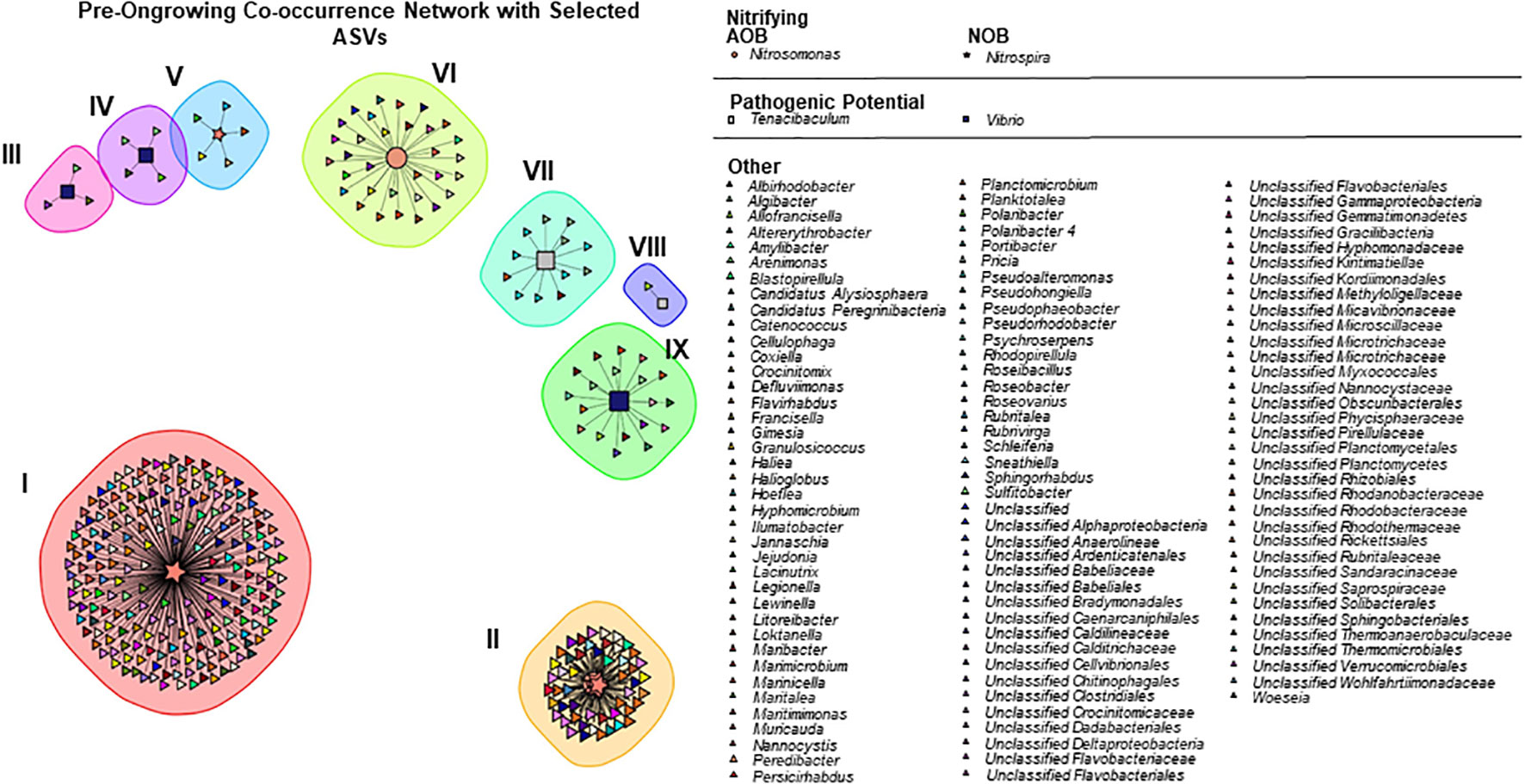
Figure 3 Correlations network in pre−ongrowing samples, coloured by Genus and shapes by function: pathogenic (squares), nitrifying oxidizing bacteria (stars), ammonia oxidizing bacteria (circles) and other (triangles).
No ASVs were found to be functionally exclusive, that is, correlated only with a specific target group, and a total of 15 different phyla correlated strongly with our target groups in the pre-ongrowing. Of these, Proteobacteria and Bacteroidetes had the highest number of correlations (respectively, 361 and 182). In unclassified genera, the families Rhodobacteraceae (85 correlations), Saprospiraceae (43 correlations) and Flavobacteriaceae (39 correlations) had the highest number of correlations, and of the classified genera, Sphingorhabdus (24 correlations), Litoreibacter (22 correlations) and Blastopirellula (17 correlations) had a higher number of correlations.
The sub-communities III to IX have an individualist nature between the functions studied, meaning that only one genus from the assigned groups is present. The reverse behaviour (where there is a cluster of positive correlations between target groups) can be found in Community I, composed of an interaction between two Nitrospira ASVs and in community II between four Nitrospira and one Nitrosomonas.
Although ASVs belonging to the target groups have different correlation patterns, this was not a simple consequence of different groupings by system or matrix (barplots can be consulted in Table S5).
Inter-ASVs interactions in the weaning system
The weaning system spearman correlation network, composed by 391 ASVs with 1164 correlations is presented in (Figure 4). Vertexes were selected with the same criteria as the previous system. In this system, there are three kinds of nitrifying ASVs: AOA, AOB, and NOB. The AOA Candidatus Nitrosopumilus, of the Nitrosopumilaceae family, has 6 ASVs with 351 total correlations. There is only one ASV belonging to the AOB genus Nitrosomonas with a total of 6 correlations. Nitrospira, the only NOB genus detected in this network, has 4 ASVs with 208 total correlations. As for the potentially pathogenic genus, Tenacibaculum is present with 56 ASVs and a total of 812 correlations and Vibrio with 5 ASVs, 38 total correlations. The complete summary of the community can be found in the Table S4-B.
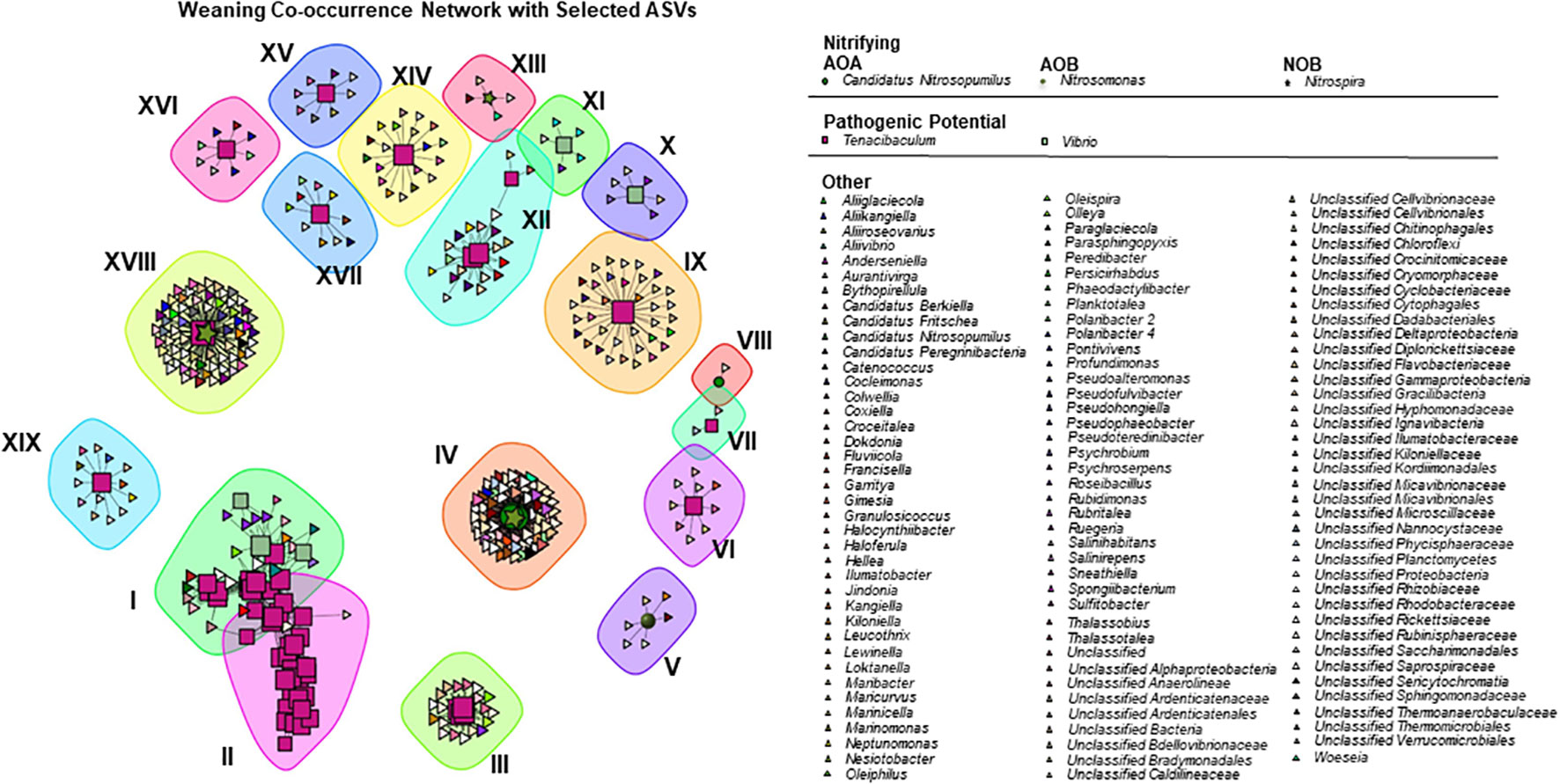
Figure 4 Correlations network in weaning samples, coloured by Genus and shapes by function: pathogenic (squares), nitrifying oxidizing bacteria (stars), ammonia oxidizing bacteria (circles), ammonia oxidizing archaea (spheres) and other (triangles).
No ASVs were found to be functionally exclusive, as before, correlating only with a specific target group, and a total of 12 different phyla correlated strongly with our target groups. Of these, Proteobacteria and Bacteroidetes had the highest number of correlations (respectively, 438 and 193), as seen in the pre-ongrowing system. In unclassified genera, the families Ardenticatenaceae (90 correlations), Rhodobacteraceae (63 correlations), Bradymonadales (40 correlations) and Flavobacteriaceae (40 correlations) had the highest number of correlations with the selected target groups. Of the classified genera, Polaribacter 2 (23 correlations), Pseudoalteromonas (17 correlations) and Thalassobius (17 correlations) had the highest number of correlations.
Nineteen subcommunities were identified with the multi-level modularity optimization algorithm. Fifteen subcommunities did not have correlations between target groups (V to XI, XIII-XVII and XIX), while eight subcommunities did (I to IV, XII and XVIII). The cluster of the subcommunities I and II are composed of three Vibrio ASVs, and 40 Tenacibaculum ASVs. There are two subcommunities composed of exclusively inter-Tenacibaculum ASVs (not counting ASVs with function “other”), III and XII, with four and three ASVs, respectively. In the subcommunity XVIII, two Nitrospira ASVs interact with one Tenacibaculum. The only exclusively nitrifying target group subcommunity is IV with five Candidatus Nitrosopumilus (AOA) ASVs and one Nitrospira (NOB).
Only three ASVs had significant strong interactions in both networks, all three of them had opposite natures between systems. Two Vibrio ASVs (126 and 221) had no correlation with other target groups in the pre-ongrowing system but had significant correlations in the weaning system (with Tenacibaculum ASVs). The only ASV attributed to Nitrosomonas in both networks (323) also had no correlations with other target groups in the weaning system but did have them in the pre-ongrowing system (with Nitrospira).
Discussion
A previous study from our research group has generally characterized these two parallel recirculating systems two systems (pre-ongrowing and weaning) (Almeida et al., 2021), reporting that the prokaryotic communities of the sole hatchery aquaculture unit were richer in the tank biofilm and in the biofilter carriers compared with the water. Also, that the weaning had a higher prokaryotic diversity than the pre-ongrowing and that parallel RAS systems develop unique communities. So, for NGS studies, we should consider that each RAS environment will have its specific prokaryotic community and speculate this to be true for other aquaculture environments as well. In this study, we found that predominancy alters between ASVs. The potentially pathogenic Tenacibaculum is most abundant in the weaning system and Vibrio is mostly associated with water samples. The nitrifying genera (Nitrosomonas, Nitrospira and Candidatus Nitrosopumilus) are more predominant in the biofilms (biofilter carriers and tank wall biofilm). Additionally, the AOA Candidatus Nitrosopumilus appears to dominate the ammonia-oxidizing community in the weaning system, while the Nitrosomonas AOB are more abundant in the pre-ongrowing system.
Overall, with the lack of negative correlations, the dynamic of these prokaryotic communities appear to be of a cooperative nature. Negative correlations are associated with competition between microbes, antibiotic interactions, and lack of cooperative secretions (Fiegna and Velicer, 2005), but no significant negative correlation was found in the present study. As a RAS system, the stability of the environmental factors most likely contributes to this dynamic and a cooperative network may be a desirable trait because it promotes overall metabolic efficiency. But it has been hypothesised that it may also have a destabilizing impact due to the coupling effect where if one ASV decreases, it will also destabilize the others (Coyte et al., 2015) and so it may be beneficial to add competitors or promote diversity.
Going forward, we also need to consider the potential biases in community network studies. These are usually associated with an unequal amount of abundance-yielding material (that can be sequencing depth or a varying number of samples, or both) per sample/condition that can lead to artificial correlations between ASVs with low-abundance with the ASVs that dominate the community (Faust and Raes, 2012). This bias results in misleading positive correlations, and it comes from a technical aspect of NGS studies, where more ASVs are detected in deeply sequenced samples, which causes a co-variation with sequencing depth (Faust et al., 2015). One way to detect this bias is by identifying cosmopolitan ASVs with a wide-spread occurrence across the samples (Faust et al., 2015), which is the case for some of the ASVs in our target groups: Vibrio, Nitrosomonas and Nitrospira.
The ASVs that had the highest number of correlations with our selected target groups in both networks (pre-ongrowing and weaning) were unclassified members of the order Bradymonadales and families Rhodobacteraceae, Saprospiraceae, Flavobacteriaceae, Ardenticatenaceae and. The diverse family Rhodobacteraceae includes both phototrophs and chemotrophs, with aerobic or facultative anaerobic metabolism (Garrity et al., 2015), mainly involved in sulphur and carbon biogeochemical cycling (Pujalte et al., 2014). Members of this family have been previously isolated from RAS biofilters (Foesel et al., 2011), which might indicate a role in sulphur and carbon nutrient recycling assigned to this family in our communities. The strictly heterotrophic and aerobic family Saprospiraceae, although without an obvious role in the community, has also been isolated from activated sludge from nutrient removal plants (Xia et al., 2008). The family Flavobacteriaceae is a diverse bacterial family (Bernardet et al., 2002) that includes the common Solea senegalensis pathogen Tenacibaculum maritimum (Avendaño-Herrera et al., 2006). The presence of unclassified members of this family could be explained by the variety of its members, including several non-pathogenic members of the genus Tenacibaculum. The family Ardenticatenaceae has only one thermophilic, chemoheterotrophic genus, Ardenticatena (Kawaichi et al., 2013) and, as with the family Rhodobacteraceae, likely also plays a role in sulphur and carbon nutrient recycling within the community of our RAS. Finally, Bradymonadales is a group of bacterial opportunistic predators in saline environments (Mu et al., 2020), which could be important in regulating global nutrient cycling in these networks as well as in creating ecological niches for interacting bacteria.
Aside from the families mentioned above, of the classified genera that dominated the correlations in our networks, Sphingorhabdus has previously been identified in saline bioreactors (Gao et al., 2020), but its functional role is not clear. Litoreibacter was isolated from seawater around an aquaculture site (Kanamuro et al., 2021) and has been positively correlated with Tenacibaculum (Liu et al., 2020), although in the present study it is only positively correlated with Nitrosomonas and Nitrospira (within the target groups selected). These genera are part of the Rhodobacteraceae family which, as mentioned before, have roles as phototrophs and chemotrophs. Blastopirellula has been detected in marine RAS biofilters (Hüpeden et al., 2020) as a carbon degrading heterotroph. Polaribacter is a dominating bacterium in marine RAS compartments and biofilters (Rud et al., 2017), and has in marine RAS, been identified as a fish pathogen in the Norwegian Fish Health Report of 2014 (Bornø and Lie, 2015). However, we did not find references associating it with sole diseases, so its presence is potentially innocuous in our communities. The genus Pseudoalteromonas has been identified as a probiotic in aquaculture with anti-Vibrio activity (Handayani et al., 2021), although our study found no negative correlations between them. Finally, Thalassobius has also been described in marine RAS communities (Michaud et al., 2009), with species characterized as aerobic chemoorganotrophic marine bacteria (Pujalte et al., 2018), justifying their role in this environment.
Nitrifying prokaryotes
Regarding the nitrifying ASVs, overall, these were more abundant in biofilm samples (biofilter carriers and tank wall biofilm), where, in the pre-ongrowing system, there is one subcommunity of four Nitrospira ASVs interacting with one Nitrosomonas. Since Nitrospira are commonly associated with nitrite oxidation (Daims et al., 2015) and Nitrosomonas with ammonia oxidation (Head et al., 1993), the result is to be expected. Ecological and metabolic cooperation between AOB and NOB is widely recognized. However, three cases of Nitrospira ASVs with no correlations with other target groups were found, two in the pre-ongrowing and one in the weaning system. This genus has also been reported as able to perform the complete nitrification of ammonia to nitrate (Daims et al., 2015) and this could be an indication of some ASVs that perform the complete nitrification process (comammox) in both systems studied in the present work. In the pre-ongrowing system, no interactions were found with pathogenic species, but it might be due to their relative low abundance. Further nitrifying ASVs with no correlations with other target groups are present in the weaning system, one Candidatus Nitrosopumilus (AOA) and one Nitrosomonas (AOB), and considering an eventual competition for ammonia, it is interesting to find no negative correlations between them (although the bias mentioned earlier should be considered). Previous studies demonstrated that AOA and AOB differentiate by niche based on ammonia concentration, where AOA outcompete AOB at relatively low concentrations (Hatzenpichler, 2012). Although the current system is overall characterized by low ammonia concentrations, the pre-ongrowing system has a higher fish density and may experience more frequent peaks of ammonia (Almeida et al., 2021). Therefore, we hypothesise that this might be the reason why we only found positive correlations between AOA and NOB in the weaning system, and only found correlations between AOB and NOB in the pre-ongrowing system where no AOA were detected.
A non-conventional interaction was found between Nitrospira and Tenacibaculum in the weaning system. Nevertheless, Tenacibaculum is the most abundant type of node in the network (56 total) with the most correlations (812) and Nitrospira, although not high in abundance, is rich in interactions (208, third highest) and so an interaction between these two groups is most likely inevitable and may not translate in a direct biological meaning. Although an argument could be made if, as a biofilm promoting genus (Romero et al., 2010), Tenacibaculum may promote the establishment of the autotroph and slow growing Nitrospira. Confirming this speculation, however, would require more empirical data and further analysis to test a causal relationship. However, interactions between heterotrophic and autotrophic bacteria may become relevant in the future, as the use of microbiome products that promote heterotrophic assimilation of nitrogen, commonly used in pond‐based aquaculture systems, have been suggested for RAS as well (Dittmann et al., 2017).
Only one nitrifying ASV (classified as Nitrosomonas) had significant strong interactions in both networks of the two systems, with opposite natures in each. That is, Nitrosomonas had no correlations with other target groups in the weaning system and but did so in the pre-ongrowing system with Nitrospira. This could be justified by the increased abundance of Nitrospira in the pre-ongrowing system. A manifestation of the bias mentioned earlier.
Pathogenic potential
Two ASVs, commonly associated with pathogenic outbreaks, had a significant positive interaction in the weaning system: Tenacibaculum and Vibrio. Previously (Wynne et al., 2020), a similar association was observed between them in fish displaying clinical signs of yellow mouth syndrome. Keeping in mind the bias already mentioned, and the particularly high abundance of Tenacibaculum in this dataset, we speculate an association between these two genera in the surrounding environment of healthy fish as well. The specific interactions between these two genera may prove relevant in disease preventions and are deserving of further studies, particularly with experimental data.
These genera are also present in individual clusters. There are five Vibrio clusters (three in the pre-ongrowing and two in weaning system), with no correlation with other target groups, and 12 Tenacibaculum clusters (two in the pre-ongrowing and ten in the weaning). Their distribution is mainly in the weaning system samples where Tenacibaculum shows a higher prevalence than in pre-ongrowing system, which might be another illustration of the positive bias of network NGS studies. Only two Vibrio ASVs had significant strong interactions simultaneously in the networks of the two systems, and with opposite natures. Vibrio had no correlations with other target groups in the pre-ongrowing system but did so in the weaning system with Tenacibaculum; as previously described (Almeida et al., 2021), this is most likely justified by the increased abundance and diversity of Tenacibaculum in the weaning system. Overall, this work provides an initial descriptive analysis of the interactions and adds to previous evidence of a correlation between two potentially pathogenic genera, Tenacibaculum and Vibrio. However, the nature of NGS studies, with the significant bias for positive interactions makes it necessary for future studies to focus on empirical data to confirm this interaction.
Conclusion
Some ASVs in our target groups showed a cosmopolitan nature, with wide distribution in the dataset (Vibrio, Nitrosomonas and Nitrospira), while others showed a behaviour shaped by the system variable (Tenacibaculum and Candidatus Nitrosopumilus). All the significant interactions found were positive. Although studies like ours are characterized by positive interaction biases, it is expected that RAS have a cooperative prokaryotic network, considering the environment stability associated. Even if this environment promotes functional optimization, the coupling effect should also be considered as an event of one ASV destabilization may also destabilize others. Ammonia oxidizing bacteria and archaea were found to be positively interacting with nitrite oxidizing bacteria, as anticipated. Two ASVs commonly associated with pathogenic outbreaks had a significant positive interaction in one of the systems, even with no outbreaks reported. Future studies should focus on this interaction as it may prove relevant in disease preventions.
Data availability statement
The datasets presented in this study can be found in online repositories. The names of the repository/repositories and accession number(s) can be found below: https://www.ebi.ac.uk/ena, PRJEB39542.
Author contributions
DBA, MS, CM, IB, and AM all had substantial contributions in the conception and design of this work, DBA and IB were responsible for the acquisition of the samples, DBA and MS for the analysis, and DBA, MS, CM, and AM in the interpretation of the data. DBA drafted the first manuscript and MS, CM, IB, and AM revised it critically with MS, CM, and AM providing approval for publication of the content. All authors agree to be accountable for all aspects of the work in ensuring that questions related to the accuracy or integrity of any part of the work are appropriately investigated and resolved. All authors contributed to the article and approved the submitted version.
Funding
This work was funded by the project Ocean3R (NORTE-01-0145-FEDER-000064), supported by the North Portugal Regional Operational Program (NORTE2020), under the PORTUGAL 2020 Partnership Agreement and through the European Regional Development Fund (ERDF). DBA was supported by the Ph.D. grant with the reference PD/BDE/135542/2018, and Safiestela Sustainable Aquafarming Investments, S.A. (part of the SEA EIGHT group). MS was supported by the project 39948_FeedMi, supported by Portugal and the European Union through FEDER/ERDF, CRESC Algarve 2020 and NORTE 2020, in the framework of Portugal 2020. AM and CM were supported by the Strategic Funding UIDB/04423/2020 and UIDP/04423/2020 through national funds provided by FCT and ERDF.
Conflict of interest
Author IB was employed by Safiestela S.A., SEA EIGHT Group.
The remaining authors declare that the research was conducted in the absence of any commercial or financial relationships that could be construed as a potential conflict of interest.
This study received funding from Safiestela S.A., SEA EIGHT Group through the Ph.D. grant with the reference PD/BDE/135542/2018. The funder had the following involvement with the study: design, data collection and analysis, decision to publish.
Publisher’s note
All claims expressed in this article are solely those of the authors and do not necessarily represent those of their affiliated organizations, or those of the publisher, the editors and the reviewers. Any product that may be evaluated in this article, or claim that may be made by its manufacturer, is not guaranteed or endorsed by the publisher.
Supplementary material
The Supplementary Material for this article can be found online at: https://www.frontiersin.org/articles/10.3389/fmars.2022.1038196/full#supplementary-material
References
Almeida D. B., Magalhães C., Sousa Z., Borges M. T., Silva E., Blanquet I., et al. (2021). Microbial community dynamics in a hatchery recirculating aquaculture system (RAS) of sole (Solea senegalensis). Aquaculture 539, 736592. doi: 10.1016/j.aquaculture.2021.736592
Apprill A., McNally S., Parsons R., Weber L. (2015). Minor revision to V4 region SSU rRNA 806R gene primer greatly increases detection of SAR11 bacterioplankton. Aquat. Microbial. Ecol. 75, 129–137. doi: 10.3354/ame01753
Avendaño-Herrera R., Toranzo A. E., Magariños B. (2006). Tenacibaculosis infection in marine fish caused by Tenacibaculum maritimum: a review. Dis. Aquat. Organisms. 71, 255–266. doi: 10.3354/dao071255
Bernardet J.-F., Nakagawa Y., Holmes B. (2002). Proposed minimal standards for describing new taxa of the family flavobacteriaceae and emended description of the family. Int. J. Syst, Evol. Microbiol. 52, 1049–1070. doi: 10.1099/00207713-52-3-1049
Blancheton J. P., Attramadal K. J. K., Michaud L., d’Orbcastel E. R., Vadstein O. (2013). Insight into bacterial population in aquaculture systems and its implication. Aquacult. Eng. 53, 30–39. doi: 10.1016/j.aquaeng.2012.11.009
Blondel V. D., Guillaume J.-L., Lambiotte R., Lefebvre E. (2008). Fast unfolding of communities in large networks. J. Stat. Mechanics.: Theory Experiment. 2008, P10008. doi: 10.1088/1742-5468/2008/10/P10008
Brailo M., Schreier H. J., McDonald R., Maršić-Lučić J., Gavrilović A., Pećarević M., et al (2019). Bacterial community analysis of marine recirculating aquaculture system bioreactors for complete nitrogen removal established from a commercial inoculum. Aquaculture 503, 198–206.
Canada P., Pereira A., Nogueira N., Png-Gonzalez L., Andrade C., Xavier R. (2020). Analysis of bacterial microbiome associated with nylon and copper nets in an aquaculture context. Aquaculture 516, 734540. doi: 10.1016/j.aquaculture.2019.734540
Caporaso J. G., Lauber C. L., Walters W. A., Berg-Lyons D., Huntley J., Fierer N., et al. (2012). Ultra-high-throughput microbial community analysis on the illumina HiSeq and MiSeq platforms. ISME J. 6, 1621. doi: 10.1038/ismej.2012.8
Caporaso J. G., Lauber C. L., Walters W. A., Berg-Lyons D., Lozupone C. A., Turnbaugh P. J., et al. (2011). Global patterns of 16S rRNA diversity at a depth of millions of sequences per sample. Proc. Natl. Acad. Sci. 108, 4516–4522. doi: 10.1073/pnas.1000080107
Comeau A. M., Douglas G. M., Langille M. G. (2017). Microbiome helper: a custom and streamlined workflow for microbiome research. MSystems 2, e00127–e00116. doi: 10.1128/mSystems.00127-16
Coyte K. Z., Schluter J., Foster K. R. (2015). The ecology of the microbiome: networks, competition, and stability. Science 350, 663–666. doi: 10.1126/science.aad2602
Csardi G., Nepusz T. (2006). The igraph software package for complex network research. InterJ. Complex Syst. 1695, 1–9.
Daims H., Lebedeva E. V., Pjevac P., Han P., Herbold C., Albertsen M., et al. (2015). Complete nitrification by Nitrospira bacteria. Nature 528, 504–509. doi: 10.1038/nature16461
Deng Y., Ruan Y., Ma B., Timmons M. B., Lu H., Xu X., et al. (2019). Multi-omics analysis reveals niche and fitness differences in typical denitrification microbial aggregations. Environ. Int. 132, 105085. doi: 10.1016/j.envint.2019.105085
Deng Y., Ruan Y., Taherzadeh M. J., Chen J., Qi W., Kong D., et al. (2021). Carbon availability shifts the nitrogen removal pathway and microbial community in biofilm airlift reactor. Bioresour. Technol. 323, 124568. doi: 10.1016/j.biortech.2020.124568
Dittmann K. K., Rasmussen B. B., Castex M., Gram L., Bentzon-Tilia M. (2017). The aquaculture microbiome at the centre of business creation. Microbial. Biotechnol. 106, 1279. doi: 10.1111/1751-7915.12877
Ebeling J. M., Timmons M. B., Wheaton J. M., Summerfelt S. T., Vinci B. J. (2002). Recirculating aquaculture. 4th ed (Ithaca, NY and Springer, Berlin: Ithaca Publishing Company).
Faust K., Lima-Mendez G., Lerat J.-S., Sathirapongsasuti J. F., Knight R., Huttenhower C., et al. (2015). Cross-biome comparison of microbial association networks. Front. Microbiol. 6, 1200. doi: 10.3389/fmicb.2015.01200
Faust K., Raes J. (2012). Microbial interactions: from networks to models. Nat. Rev. Microbiol. 10, 538–550. doi: 10.1038/nrmicro2832
Faust K., Sathirapongsasuti J. F., Izard J., Segata N., Gevers D., Raes J., et al. (2012). Microbial co-occurrence relationships in the human microbiome. PloS Comput. Biol. 8, e1002606. doi: 10.1371/journal.pcbi.1002606
Fiegna F., Velicer G. J. (2005). Exploitative and hierarchical antagonism in a cooperative bacterium. PloS Biol. 3, e370. doi: 10.1371/journal.pbio.0030370
Foesel B. U., Drake H. L., Schramm A. (2011). Defluviimonas denitrificans gen. nov., sp. nov., and Pararhodobacter aggregans gen. nov., sp. nov., non-phototrophic rhodobacteraceae from the biofilter of a marine aquaculture. Syst, Appl. Microbiol. 34, 498–502. doi: 10.1016/j.syapm.2011.08.006
Foesel B. U., Gieseke A., Schwermer C., Stief P., Koch L., Cytryn E., et al. (2008). Nitrosomonas Nm143-like ammonia oxidizers and Nitrospira marina-like nitrite oxidizers dominate the nitrifier community in a marine aquaculture biofilm. FEMS Microbiol. Ecol. 63, 192–204. doi: 10.1111/j.1574-6941.2007.00418.x
Gao Y., Wang X., Li J., Lee C. T., Ong P. Y., Zhang Z., et al. (2020). Effect of aquaculture salinity on nitrification and microbial community in moving bed bioreactors with immobilized microbial granules. Bioresour. Technol. 297, 122427. doi: 10.1016/j.biortech.2019.122427
Garrity G. M., Bell J. A., Lilburn T. (2015). “Helicobacteraceae fam. nov,” in Bergey’s manual of systematics of archaea and bacteria (New Jersey, EUA: John Wiley & Sons, Inc.) 1–1.
Goldford J. E., Lu N., Bajić D., Estrela S., Tikhonov M., Sanchez-Gorostiaga A., et al. (2018). Emergent simplicity in microbial community assembly. Science 361, 469–474. doi: 10.1126/science.aat1168
Handayani D. P., Isnansetyo A., Istiqomah I., Jumina J. (2021). Anti-vibrio activity of Pseudoalteromonas xiamenensis STKMTI. 2, a new potential vibriosis biocontrol bacterium in marine aquaculture. Aquacult. Res. (2022) 53.5, 1800–13. doi: 10.1111/are.15708
Hatzenpichler R. (2012). Diversity, physiology, and niche differentiation of ammonia-oxidizing archaea. Appl. Environ. Microbiol. 78, 7501–7510. doi: 10.1128/AEM.01960-12
Head I. M., Hiorns W. D., Embley T. M., McCarthy A. J., Saunders J. R. (1993). The phylogeny of autotrophic ammonia-oxidizing bacteria as determined by analysis of 16S ribosomal RNA gene sequences. Microbiology 139, 1147–1153. doi: 10.1099/00221287-139-6-1147
Howell B. R. (1997). A re-appraisal of the potential of the sole, Solea solea (L.), for commercial cultivation. Aquaculture 155, 355–365. doi: 10.1016/S0044-8486(97)00103-8
Hüpeden J., Wemheuer B., Indenbirken D., Schulz C., Spieck E. (2020). Taxonomic and functional profiling of nitrifying biofilms in freshwater, brackish and marine RAS biofilters. Aquacult. Eng. 90, 102094. doi: 10.1016/j.aquaeng.2020.102094
Infante-Villamil S., Huerlimann R., Jerry D. R. (2020). Microbiome diversity and dysbiosis in aquaculture. Rev. Aquacult. (2021) 13.2, 1077–1096. doi: 10.1111/raq.12513
Jiang W., Tian X., Li L., Dong S., Zhao K., Li H., et al. (2019). Temporal bacterial community succession during the start-up process of biofilters in a cold-freshwater recirculating aquaculture system. Bioresour. Technol. 287, 121441. doi: 10.1016/j.biortech.2019.121441
Kanamuro M., Sato-Takabe Y., Muramatsu S., Hirose S., Muramatsu Y., Takaichi S., et al. (2021). Litoreibacter roseus sp. nov., a novel bacteriochlorophyll a-containing bacterium. Int. J. Syst, Evol. Microbiol. 71, 004679. doi: 10.1099/ijsem.0.004679
Kawaichi S., Ito N., Kamikawa R., Sugawara T., Yoshida T., Sako Y. (2013). Ardenticatena maritima gen. nov., sp. nov., a ferric iron-and nitrate-reducing bacterium of the phylum ‘Chloroflexi’ isolated from an iron-rich coastal hydrothermal field, and description of Ardenticatenia classis nov. Int. J. Syst. Evol. Microbiol. 63, 2992–3002. doi: 10.1099/ijs.0.046532-0
Liu Q., Zhi Y., He Y., Ren Z., Chen H., Yang R. (2020). Changes in phycospheric and environmental microbes associated with an outbreak of yellow spot disease on pyropia yezoensis. Aquaculture 529, 735651. doi: 10.1016/j.aquaculture.2020.735651
Martínez-Porchas M., Vargas-Albores F. (2017). Microbial metagenomics in aquaculture: a potential tool for a deeper insight into the activity. Rev. Aquacult. 9, 42–56. doi: 10.1111/raq.12102
Martins C. I. M., Eding E. H., Verdegem M. C. J., Heinsbroek L. T. N., Schneider O., Blancheton J.-P., et al. (2010). New developments in recirculating aquaculture systems in Europe: A perspective on environmental sustainability. Aquacult. Eng. 43, 83–93. doi: 10.1016/j.aquaeng.2010.09.002
Marx C. J. (2009). Getting in touch with your friends. Science 324, 1150–1151. doi: 10.1126/science.1173088
Michaud L., Blancheton J.-P., Bruni V., Piedrahita R. (2006). Effect of particulate organic carbon on heterotrophic bacterial populations and nitrification efficiency in biological filters. Aquacult. Eng. 34, 224–233. doi: 10.1016/j.aquaeng.2005.07.005
Michaud L., Lo Giudice A., Troussellier M., Smedile F., Bruni V., Blancheton J.-P. (2009). Phylogenetic characterization of the heterotrophic bacterial communities inhabiting a marine recirculating aquaculture system. J. Appl. Microbiol. 107, 1935–1946. doi: 10.1111/j.1365-2672.2009.04378.x
Morais S., Aragão C., Cabrita E., Conceição L. E., Constenla M., Costas B., et al. (2016). New developments and biological insights into the farming of Solea senegalensis reinforcing its aquaculture potential. Rev. Aquacult. 8, 227–263. doi: 10.1111/raq.12091
Mu D.-S., Wang S., Liang Q.-Y., Du Z.-Z., Tian R., Ouyang Y., et al. (2020). Bradymonabacteria, a novel bacterial predator group with versatile survival strategies in saline environments. Microbiome 8, 1–15. doi: 10.1186/s40168-020-00902-0
Newman M. E. (2003). The structure and function of complex networks. SIAM. Rev. 45, 167–256. doi: 10.1137/S003614450342480
Parada A. E., Needham D. M., Fuhrman J. A. (2016). Every base matters: assessing small subunit rRNA primers for marine microbiomes with mock communities, time series and global field samples. Environ. Microbiol. 18, 1403–1414. doi: 10.1111/1462-2920.13023
Paungfoo C., Prasertsan P., Burrell P. C., Intrasungkha N., Blackall L. L. (2007). Nitrifying bacterial communities in an aquaculture wastewater treatment system using fluorescence in situ hybridization (FISH), 16S rRNA gene cloning, and phylogenetic analysis. Biotechnol. Bioeng. 97, 985–990. doi: 10.1002/bit.21270
Petersen C., Round J. L. (2014). Defining dysbiosis and its influence on host immunity and disease. Cell. Microbiol. 16, 1024–1033. doi: 10.1111/cmi.12308
Piedrahita R. H. (2003). Reducing the potential environmental impact of tank aquaculture effluents through intensification and recirculation. Aquaculture 226, 35–44. doi: 10.1016/S0044-8486(03)00465-4
Pujalte M. J., Lucena T., Rodrigo-Torres L., Arahal D. R. (2018). Comparative genomics of Thalassobius including the description of thalassobius activus sp. nov., and Thalassobius autumnalis sp. nov. Front. Microbiol. 8, 2645. doi: 10.3389/fmicb.2017.02645
Pujalte M. J., Lucena T., Ruvira M. A., Arahal D. R., Macián M. C. (2014). The family rhodobacteraceae (Berlin: Springer).
Quast C., Pruesse E., Yilmaz P., Gerken J., Schweer T., Yarza P., et al. (2012). The SILVA ribosomal RNA gene database project: improved data processing and web-based tools. Nucleic Acids Res. 41, D590–D596. doi: 10.1093/nar/gks1219
R Core Team (2019). R: A language and environment for statistical computing. Vienna, Austria: R Foundation for Statistical Computing. URL https://www.R-project.org/.
Romero M., Avendaño-Herrera R., Magariños B., Cámara M., Otero A. (2010). Acylhomoserine lactone production and degradation by the fish pathogen Tenacibaculum maritimum, a member of the cytophaga–Flavobacterium–Bacteroides (CFB) group. FEMS Microbiol. Lett. 304, 131–139. doi: 10.1111/j.1574-6968.2009.01889.x
Ruan Y.-J., Guo X.-S., Ye Z.-Y., Liu Y., Zhu S.-M. (2015). Bacterial community analysis of different sections of a biofilter in a full-scale marine recirculating aquaculture system. North Am. J. Aquacult. 77, 318–326. doi: 10.1080/15222055.2015.1017128
Rud I., Kolarevic J., Holan A. B., Berget I., Calabrese S., Terjesen B. F. (2017). Deep-sequencing of the bacterial microbiota in commercial-scale recirculating and semi-closed aquaculture systems for Atlantic salmon post-smolt production. Aquacult. Eng. 78, 50–62. doi: 10.1016/j.aquaeng.2016.10.003
Schmieder R., Edwards R. (2011). Quality control and pre-processing of metagenomic datasets. Bioinformatics 27, 863–864. doi: 10.1093/bioinformatics/btr026
Semedo M., Lopes E., Baptista M. S., Oller-Ruiz A., Gilabert J., Tomasino M. P., et al. (2021). Depth profile of nitrifying archaeal and bacterial communities in the remote oligotrophic waters of the north pacific. Front. Microbiol. 12, 319. doi: 10.3389/fmicb.2021.624071
Sharma B., Ahlert R. (1977). Nitrification and nitrogen removal. Water Res. 11, 897–925. doi: 10.1016/0043-1354(77)90078-1
Spearman C. (1987). The proof and measurement of association between two things. Am. J. Psychol. 100, 441–471. doi: 10.2307/1422689
Tal Y., Watts J. E. M., Schreier S. B., Sowers K. R., Schreier H. J. (2003). Characterization of the microbial community and nitrogen transformation processes associated with moving bed bioreactors in a closed recirculated mariculture system. Aquaculture 215, 187–202. doi: 10.1016/S0044-8486(02)00372-1
Team, R.C (2021). R: A language and environment for statistical computing. (Vienna, Austria: R Foundation for Statistical Computing).
Toranzo A. E., Magariños B., Romalde J. L. (2005). A review of the main bacterial fish diseases in mariculture systems. Aquaculture 246, 37–61. doi: 10.1016/j.aquaculture.2005.01.002
Wei G., Shan D., Li G., Li X., Tian R., He J., et al. (2020). Prokaryotic communities vary with floc size in a biofloc-technology based aquaculture system. Aquaculture 529, P735632. doi: 10.1016/j.aquaculture.2020.735632
Wynne J. W., Thakur K. K., Slinger J., Samsing F., Milligan B., Powell J. F. F., et al. (2020). Microbiome profiling reveals a microbial dysbiosis during a natural outbreak of tenacibaculosis (Yellow mouth) in Atlantic salmon. Front. Microbiol. 11, 586387. doi: 10.3389/fmicb.2020.586387
Xia L. C., Ai D., Cram J., Fuhrman J. A., Sun F. (2013). Efficient statistical significance approximation for local similarity analysis of high-throughput time series data. Bioinformatics 29, 230–237. doi: 10.1093/bioinformatics/bts668
Xia Y., Kong Y., Thomsen T. R., Halkjær Nielsen P. (2008). Identification and ecophysiological characterization of epiphytic protein-hydrolyzing saprospiraceae (“Candidatus epiflobacter” spp.) in activated sludge. Appl. Environ. Microbiol. 74, 2229–2238. doi: 10.1128/AEM.02502-07
Keywords: network, correlation, microbiome, recirculating aquaculture systems, nitrification, pathogens
Citation: Bastos Almeida D, Semedo M, Magalhães C, Blanquet I and Mucha AP (2022) The network of nitrifying and pathogenic prokaryotic interactions in a recirculating aquaculture system of a sole (Solea senegalensis) hatchery. Front. Mar. Sci. 9:1038196. doi: 10.3389/fmars.2022.1038196
Received: 06 September 2022; Accepted: 10 November 2022;
Published: 01 December 2022.
Edited by:
Andrea Tarnecki, Auburn University Shellfish Laboratory, United StatesReviewed by:
Patricia I. S. Pinto, University of Algarve, PortugalKishore Kumar Krishnani, Central Institute of Fisheries Education (ICAR), India
Copyright © 2022 Bastos Almeida, Semedo, Magalhães, Blanquet and Mucha. This is an open-access article distributed under the terms of the Creative Commons Attribution License (CC BY). The use, distribution or reproduction in other forums is permitted, provided the original author(s) and the copyright owner(s) are credited and that the original publication in this journal is cited, in accordance with accepted academic practice. No use, distribution or reproduction is permitted which does not comply with these terms.
*Correspondence: Diana Bastos Almeida, diana.almeida@ciimar.up.pt