Interactive effects of fishing effort reduction and climate change in a central Mediterranean fishing area: Insights from bio-economic indices derived from a dynamic food-web model
- 1National Institute of Oceanography and Applied Geophysics-OGS, Section of Oceanography, Trieste, Italy
- 2National Research Council, Institute of Anthropic Impacts and Sustainability in Marine Environment (CNR-IAS), Palermo, Italy
- 3School of Geosciences, University of Edinburgh, Edinburgh, United Kingdom
- 4Department of Integrative Marine Ecology, Stazione Zoologica Anton Dohrn, Rome, Italy
- 5National Research Council, Institute for Marine Biological Resources and Biotechnology (IRBIM), Mazara del Vallo, Italy
- 6Laboratory of Experimental Ecology and Aquaculture, Department of Biology, University of Rome Tor Vergata, Rome, Italy
- 7National Inter-University Consortium for Marine Sciences (CoNISMa), Rome, Italy
Disentangling the effects of mixed fisheries and climate change across entire food-webs requires a description of ecosystems using tools that can quantify interactive effects as well as bio-economic aspects. A calibrated dynamic model for the Sicily Channel food web, made up of 72 functional groups and including 13 fleet segments, was developed. A temporal simulation until 2050 was conducted to evaluate the bio-economic interactive effects of the reduction of bottom trawling fishing effort by exploring different scenarios that combine fishery and climate change. Our results indicate that direct and indirect effects produce a net increase in biomass of many functional groups with immediate decline of trawlers’ catches and economic incomes, followed by a long term increase mainly due to biomass rebuilding of commercial species which lasts 5-10 years after fishing reduction. Synergistic and antagonistic effects caused by changes in the fishing effort and in climate characterize a specific functional group’s response in biomass which, in turn, modulate also the catch and income of the other fleets, and especially of those sharing target resources. However, trawler’s intra-fleet competition is higher than the others fleet effects. In the medium term, the effects of fishing effort reduction are higher than those of climate change and seem to make exploitation of marine resources more sustainable over time and fishery processes more efficient by improving ecosystem health.
1 Introduction
Marine fisheries have a prominent role across the globe because they provide a huge diversity of seafood and economic income for millions of people (FAO, 2020). However, as the world population will grow to around ten billion people over the next three decades (UN, 2019) and the climate of our planet is changing, there is a need to understand and modulate fishing impacts on ecosystems and increase fisheries’ efficiency under a sustainable framework. Basically, fisheries range between two opposite sides: industrial fishing operates mainly in the high sea while Small Scale Fisheries (SSF) are predominant nearshore, especially in the emergent countries where they provide essential animal proteins (FAO, 2020).
Industrial fisheries, mainly based on bottom trawling, tend to operate at a great cost to ecosystems because of large proportions of unwanted catches of marine organisms (i.e. bycatch and discards) (FAO, 2020) and the negative impact on habitats (De Juan et al., 2011). In addition, bottom trawling is the only activity specialized in catching highly valuable demersal species such as shrimps. However, since 2000’s experimental technological advances have made progressively available potential options to reduce the impact of trawling due to unwanted catches (small-size individuals of commercial species or non-commercial species), for example by means of net modifications or benthos release panels (Soetaert et al., 2016; Vitale et al., 2018).
SSF are generally considered more sustainable because they use fishing gears that have little impact on the environment (e.g. the seabed) and incorporate traditional elements that are often in line with sustainability principles (FAO, 2019). In fact, SSF use a range of highly selective gears and are generally more flexible in terms of target species, area and season. Additionally, SSF employ 25 times more people and use three-quarters less fuel than the industrial fishery sector to catch a similar amount of edible fish (Jacquet and Pauly, 2008; Teh and Sumaila, 2013).
In the Mediterranean Sea, a wide range of fishing activities from small-scale to industrial characterizes the so-called mixed fisheries. They interact at least indirectly through the food-web (Agnetta et al., 2019) and it has also been hypothesized that they compete with each other (Raicevich et al., 2020). Nowadays, the main regulation for managing fishing in the EU waters of the Mediterranean Sea is the EU-Common Fisheries Policy (CFP), which aims at exploiting stocks at a safe level, defined according to the Maximum Sustainable Yield (MSY) paradigm. However, the resilience of a target stock relies not only on its health but also on the interactions established with other ecosystem components. Although areas with a long history of efficient and intensive stock assessments and management have shown healthy target populations (Hilborn et al., 2020), these assessments into more complex models seems to be promising for the Ecosystem Approach to Fisheries. Given that single species models tend to compress the complexity of interspecific relationships within a marine community exploited by fisheries, the adoption of multispecies and multigear models allows for a more integrated perspective of the ecosystem functioning, foresees fish and fishery patterns in mid-long temporal scales (Coll and Libralato, 2012) and helps estimate trade-offs in marine exploited ecosystems (Libralato and Agnetta, 2019).
Also, assessing ecological and economic sustainability of fisheries is urgent and awaited, particularly in places such as the Mediterranean Sea where decreased landings of shellfish and finfish observed in the last decades (Carpi et al., 2017; Colloca et al., 2017; Fiorentino and Vitale, 2021) may lead to an exacerbation of fleet competition, economically inefficient exploitation and a degradation of habitats and living resources. In addition, the fact that the Mediterranean Sea is considered a hotspot of climate change (Giorgi, 2006; Pisano et al., 2020) is leading to growing concerns for the potential negative effects on marine resources and the consequent increased critical issues for fisheries. Finally, changes in primary production driven by climate change could act in combination with fishing by varying catches and biomass of target species differently in space and time (Moullec et al., 2019) and potentially weaken the ecosystem’s capacity of recovery to threats.
In the Strait of Sicily (SoS), in order to limit the negative effects of fishing on marine life and habitats, different management measures have been discussed and adopted, including the reduction of bottom trawling, the establishment of new fishery restricted areas as well as additional technological measures (Russo et al., 2019; Jarboui et al., 2022). In particular, the Italian Multiannual Management Plan has provided for a strong reduction of the fishing effort, in terms of fleet capacity and activity, amounting to 25% from 2008 to 2013. A further reduction of fishing days by 5% in 2019 and 10% in 2020 was achieved, taking the 2018 fishing days/data as the baseline reference. Such an effort reduction is expected to determine an impressive socio-economic impact for the fishing sector, possibly affecting the medium-term survival of several enterprises. However, the effects of enforcing this measure have never been investigated in a multi-fleet context. In highly connected fishery systems such as the SoS, the intricacy of interactions among organisms, fleets and between fleets and organisms (Agnetta et al., 2019) might transfer the impact from one fleet to another and/or from one target species to another. Furthermore, given that fisheries respond to changes in bio-economic factors, it is important to take them into account in the analysis, especially in a perspective of global environmental change. It is therefore of paramount importance to evaluate the effects of the effort reduction on both the ecosystem and the fisheries performance in the short and medium term, also in relation to the future climatic scenarios for the region (Moullec et al., 2019). In this context, ecosystem food web models such as Ecopath with Ecosim represent a quantitative framework for accounting of climatic changes, fishery modifications and bioeconomy and result in an integrated modelling approach widely used (Heymans et al., 2016).
In this study, a calibrated multispecies-multifleet food web model based on Ecosim is developed and applied to quantify the ecological and bio-economic effects induced by the reduction of trawling effort under climate change scenarios. Simulations are set to disentangle potential effects of primary production changes induced by climate change, which is among the most important factor affecting ecosystem productivity over time (Moullec et al., 2019). The analysis accounts for the interaction of effects on biomass, catch and economic income in a mixed fishery Mediterranean system such as the SoS. The effort reduction is expected to produce an increase in biomass of target functional groups (fishery release) and the eventual rebuilding of stocks is quantified. Moreover, the analysis is used to test for synergism and antagonism between effort reduction and primary production levels on functional groups and the net biomass response of functional groups to changes in these two factors. Finally, the inclusion of economic aspects into the modelling allows the quantification of the extent to which group response affects catch and economic incomes of fisheries.
2 Material and methods
2.1 Modelling approach
We have used the Ecopath with Ecosim modelling approach (Christensen and Walters, 2004) by starting with a well-developed Ecopath model for the SoS (Agnetta et al., 2019). The dynamic temporal food-web model Ecosim (EwE v. 6.6.5, http://www.ecopath.org) expresses biomass dynamics based upon the initial parameters of the Ecopath master equation (Christensen and Walters, 2004) using a system of differential equations which take the form:
where dBi/dt is the biomass growth rate of group (i) during the interval dt, gi the net growth efficiency (production/consumption ratio), Ii the immigration rate, Mi and Fi the natural and fishing mortality rates of group (i), and ei the emigration rate. The consumption rates Qji are calculated based on the “foraging arena” theory (animals balance predation risk with foraging activity) where the biomass of the preys are divided into vulnerable and invulnerable components (Christensen and Walters, 2004).
In Ecosim, the interactions between predators and prey are therefore regulated by the foraging arena theory through a set of parameters including the so called “vulnerability”, which is usually considered as the tuning parameter (Christensen and Walters, 2004). The foraging arena is based on the ecological assumption that only a fraction of the biomass of prey is available to the predator, dynamically adapted through the vulnerability parameter specific for each prey-predator interaction (Walters et al., 2000). Basically, the vulnerability indicates the effect of prey and predator density on the consumption by a predator, and makes it possible to balance top-down and bottom-up processes across the trophic web (Walters and Christensen, 2007; Ahrens et al., 2012) with respect to initial conditions (Ecopath biomasses). Vulnerabilities are also tuned to fit the historical patterns of observed data under simulated dynamics forced by primary production and/or fishing effort changes (Araújo et al., 2008).
The Ecosim module was used here to integrate a massive amount of environmental, biological and fisheries information in a coherent framework and dynamically describing a series of yearly data of biomass, catch and economic income in the SoS. We started from the mass balanced Ecopath model (Agnetta et al., 2019) and, after a spin-up of 10 years (1995-2004), we fitted the dynamic food-web model for the period 2005-2017 to real data and simulated the future scenarios of climate and fisheries up to 2050. The study area, approximately 61,000 km2 wide, coincides with the northern side of the larger SoS (Figure 1), which stretches off the southern Sicily coast and is characterized in its central portion by a narrow continental shelf that separates two wider portions of shelf (i.e. the Adventure Bank to the west and the Malta Bank to the east).
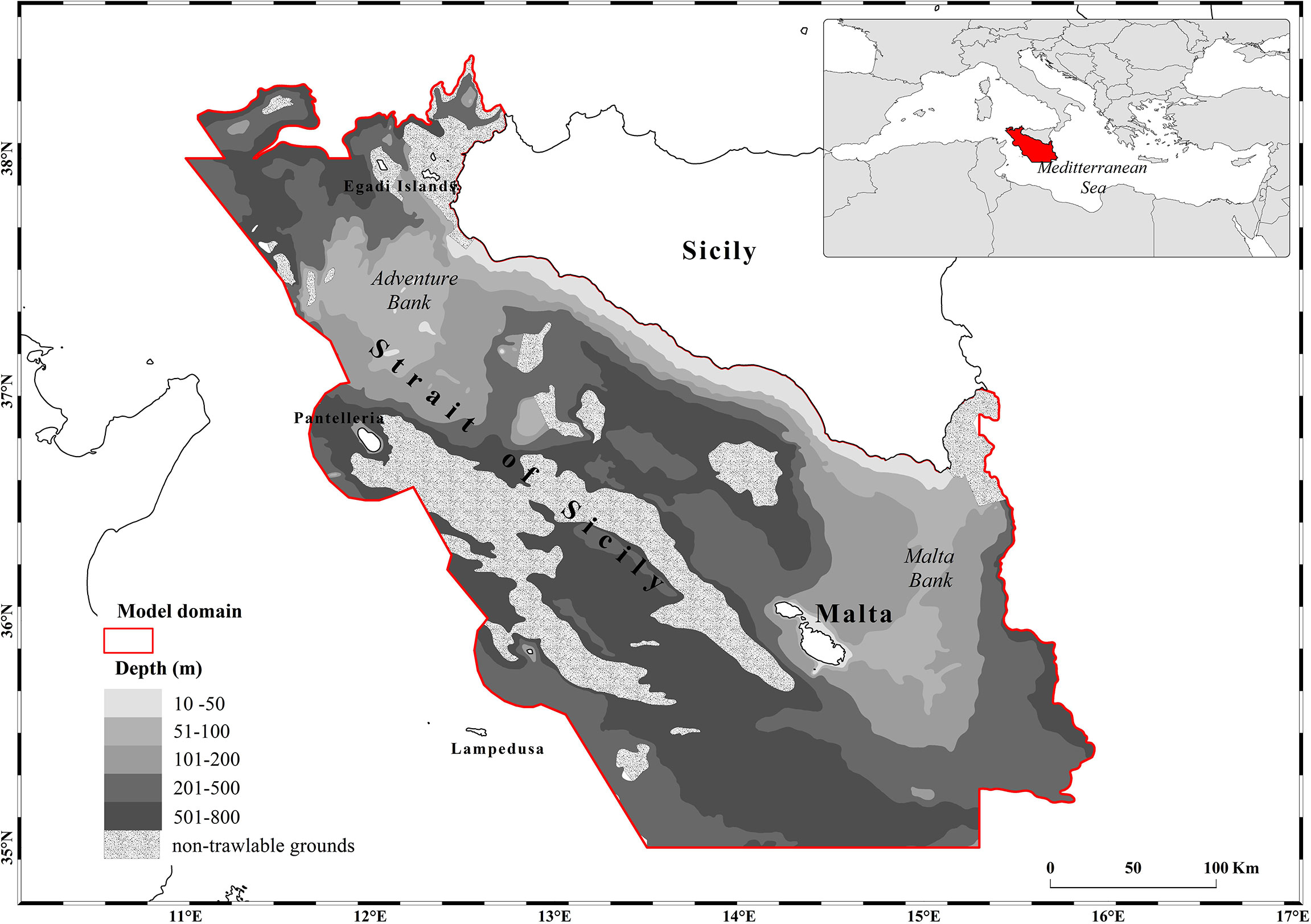
Figure 1 Area of the Strait of Sicily where the dynamic food web model (about 61,000 km2) is applied.
2.2 Input data
2.2.1 Biological data
The model comprises 69 living functional groups (from bacteria to large pelagic species), and 3 non-living organic matter compartments (Table 1). Biomass of phytoplankton, zooplankton, and the concentration of suspended detritus were estimated by the Copernicus Marine Service (CMEMS) product as obtained from the 3D coupled physical and biogeochemical model for the Mediterranean Sea (Cossarini et al., 2021). Original georeferenced CMEMS data expressed as gCm-3 were extracted for the studied area (years 2005-2017) and integrated over depth (10-800m) in order to obtain the relative biomass as gCm-2, data for plankton functional types were aggregated to represent the groups of the EwE model and then conversion factors were used in order to transform gC to wet weight (Bonanno et al., 2013; Kiørboe, 2013). Fishery-independent biomass density estimates encompassing demersal fish and shellfish were mainly obtained from bottom trawl surveys carried out in spring/summer from the mid 2000’s to 2017 in the model area by the MEDITS program (Mediterranean International bottom Trawl Surveys) (Bertrand et al., 2002; Spedicato et al., 2019) and expressed as kg km-2 wet weight. For highly migratory species (bluefin tuna and swordfish), we used average biomass estimates provided by stock assessments in the Mediterranean Sea (ICCAT, 2010) and adapted parameters by taking into account eventual lifetime spent outside the SoS area (see more details in Agnetta et al., 2019).
2.2.2 Fishery data
Fishery landings by species and gear in the SoS during 2005-2017 were drawn from the national monitoring of commercial fleets within the European Data Collection Framework (DCF - https://datacollection.jrc.ec.europa.eu/). Data were aggregated into 13 fleet segments resulting from a combination of fishing gear (i.e. trawlers, purse-seiners, long-liners, set netters) and vessel size classes based on the length overall (LOA:<12m; 12-24m; >24m) (Table 2). Furthermore, the bottom otter trawlers were also distinguished into categories according to the main target species. Compared to 18 fleets used in the original Ecopath model (Agnetta et al., 2019), a slight reshaping of several fishing activities was necessary due to the lack of details for some fisheries.
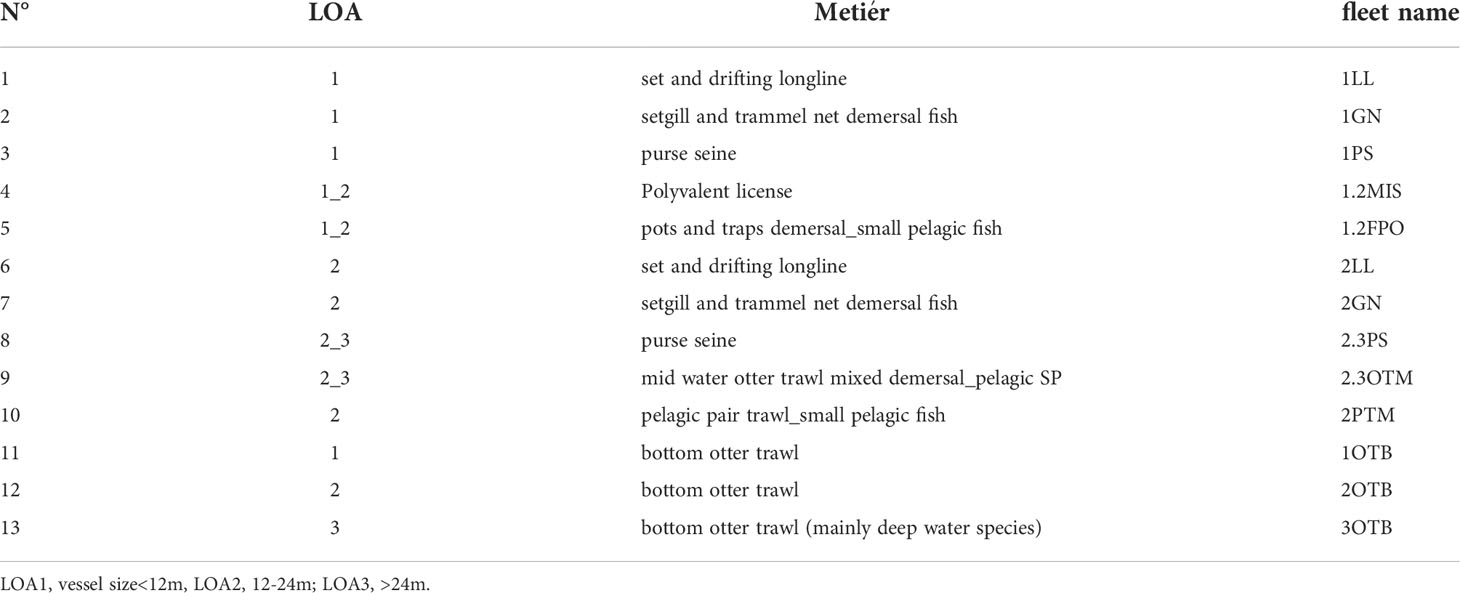
Table 2 List of the fleet segments considered by the combination of vessel size (i.e. length overall =LOA) and fishing gear.
Since the fishing activity of larger bottom trawlers (i.e. fleet 13, Table 2) targeting deep-water species (i.e. deep-water rose shrimp Parapenaeus longirostris and giant red shrimp Aristaeomorpha foliacea) spans over a larger space than the model area (Garofalo et al., 2007; Milisenda et al., 2017), about 60-70% of their important species catches was retained inside the model area (Agnetta et al., 2019). Empirical discard ratio for commercial fish and invertebrates by species and by fleet was drawn from studies carried out in the SoS or from nearby Mediterranean areas (Agnetta et al., 2019 and references therein).
2.2.3 Economic data for income per unit effort (IPUE)
Since landings (L) change dynamically in Ecosim, the revenues (R) are estimated from landings and off-vessel prices (offprice) per fleet (fl) and functional group (FG) at each time step:
The most detailed estimates of prices (per species, fleet and year) were taken into account whenever possible. The price of the main target species making up about 60% in catches of bottom trawlers such as hake (HKE), striped mullet (MUT), pandora (PAC), giant red shrimp (ARS) and deep-water rose shrimp (DPS) was updated for each year of the hindcast (2005-2017) by using data collected within the DCF. In the projections (years 2018-2050) prices were kept to the last values available (2018).
These dynamically estimated revenues were standardized by using the relative effort (rel_f) of each fleet:
where f is the effort of each fleet (fl) at any time step and t0 corresponded to the first year of the hindcast (i.e. 2005) when the model was mass balanced (ecopath model), in this way an index of income per unit effort (IPUE), was calculated as:
and expressed in millions of euros (mln EUR).
2.3 Fitting procedure and basic validation of the model
A combination of trophic interaction strength (vulnerability of prey to predators), fishing effort over time and primary production changes were used for fitting the model to past biomass and catch data for the period 2005-2017 (hindcast). The hindcast EwE model was bottom-up forced using net primary production of large (PL) and small (PS) phytoplankton. Total net primary production of the CMEMS reanalysis (Cossarini et al., 2021) was partitioned into PL and PS production anomaly by using the relative biomass of the phytoplankton functional groups included in the Biogeochemical Flux Model (BFM) (Lazzari et al., 2012; Salon et al., 2019), namely diatoms of the BFM for the PL group in EwE and the sum of picophytoplankton and nanoflagellates in BFM for PS.
In order to reconstruct fishing pressure per fleet (top-down forcing) over the years (2005-2019), we used complementary information collected under the umbrella of DCF, i.e. the vessel monitoring system (VMS) and data from the fleet register relative to the model area (https://webgate.ec.europa.eu/fleet-europa/index_en). However, VMS allows to assess the fishing activity of vessels with LOA larger than 15 m, while the fleet segment 12m< LOA< 15m is only partially covered. Hence, for this fleet segment, the observed effort in space and time was rescaled by using the official number of vessels and the footprint of VMS-equipped vessels (Russo et al., 2014; Russo et al., 2019). Moreover, fishing activity as days at sea per year was used for vessels<12 LOA.
The fitting procedure has followed an approach that foresees a series of trials for tuning the vulnerabilities (Heymans et al., 2016) and was evaluated through the sum of squared differences (SS) between the log-observed and log-predicted data (i.e. biomass or catch) (Christensen and Walters, 2004) and the Akaike’s information criterion (AIC) (Alexander et al., 2015). Vulnerability by predators (i.e. common vulnerability for all preys of a given predator) were used to perform sensitivity search of the most influential vulnerabilities and nine trials for the best fit were conducted using a different number (k) of tuned vulnerabilities (Table S1), keeping remaining vulnerabilities to default values (v=2). Comparison of model outputs and data was done using annual biomass of 27 functional groups (Figure S2A) and annual catches of the most important commercial species European hake (HKE), red mullet (MUT), pandora (PAC), giant red shrimp (ARS), deep water rose shrimp (DPS), European anchovy (ENG), European pilchard (SAR), swordfish (XIP), bluefin tuna (THU) (14 FGs, Figure S2B) for a total of 41 series (S) and 511 data points (N). Assuming autocorrelation between time series and no cross-correlation, these data corresponds to S-1 = 40 degree of freedom (df).
Validation was based on a graphical evaluation of the model outputs against the observed mean yearly biomass (MEDITS) of HKE, MUT, PAC, ARS and DPS, accompanied by the calculation of the Spearman correlation coefficient. The comparison was done therefore for several commercial groups whose catches were instead used for fitting, over the hindcast period (2005-2017). Total landings and revenues relative to 6 years (2013-2018) were also compared to data collected within the DCF.
2.4 Setting of scenarios
In order to disentangle the role of fishing effort reduction and primary production driven by climate change, a set of 4 scenarios were run: they all have in common the hindcast period 2005-2017 and have specific forcing functions for the years 2018-2050, adapted and combined to address the research aims.
The scenarios implemented were i) reference scenario (REF), ii) fishing effort reduction scenario (F), iii) climate change scenario (C), and iv) combined fishing reduction and climate change (FC) (Table 3). The REF scenario was simulated with a constant effort after the year 2019 and a constant annual primary production after year 2017 (using seasonal cycles derived from climatology, years 2005-2017).

Table 3 Breakdown of forcing used to the reference (REF) and the alternative projected scenarios, F=fishing effort reduction, C= climate, FC= fishing effort reduction+climate.
The F scenario was represented by reducing simultaneously the three fleets of bottom trawlers (1OTB, 2OTB and 3OTB respectively with LOA<12m, LOA 12-24m, LOA>24m) by 10% each year from 2022 to 2024 (each 10% yearly cut always referred to the fishing effort level in 2021, so that the total reduction over the 3-year period is 30% per fleet) and considering the climatological primary production as in the REF scenario.
The C and FC scenarios were run with the same fishing pressure of REF and F respectively, but incorporating variations in primary production induced by climate change. In these cases, a monthly primary production anomaly for phytoplankton groups (PL and PS) was estimated as done for hindcast using the results of the biogeochemical scenario simulation based on Representative Concentration Pathway (RCP) 8.5 (Reale et al., 2022). The scenario simulation features the same model configuration as in the Copernicus reanalysis: a coupling between the NEMO ocean dynamics model and the BFM biogeochemical model (Reale et al., 2022). Scenario setups (e.g., boundaries, land input) are consistent with the reanalysis (Cossarini et al., 2021) except for resolution and assimilation (Reale et al., 2022). A detrendization was also applied as in Solidoro et al. (2022), in order to remove numerical trends and eventual bias between climate scenario and reanalysis by comparing the series over the 2005-2017 period. The RCP8.5 from the IPCC is the highest greenhouse gas emissions scenario over time. It assumes a slow income growth with no important rates of technological change and energy intensity improvements (Riahi et al., 2011), a high population growth rate and a “business as usual” fisheries management (i.e., current fishing mortality). It was chosen here as the scenario was the most frequently explored in the region (Hattab et al., 2016; Corrales et al., 2018; Moullec et al., 2019), hence facilitating comparisons with other findings.
2.5 Analysis of biomass, landings and income across scenarios
Trends of biomass, landings and IPUE along temporal projection (2018-2050) and across scenarios were summarized per FG and/or per fleet. In order to assess changes between each alternative scenario (F, C, FC) and the reference one (REF), basic statistics (mean, standard deviation, minimum and maximum) were estimated after calculating ratios year by year of the projection (2018-2050) and in specific time ranges (2026-2030, 2046-2050) as: ratio (t) = scenario (t)/REF (t).
Effects of fishery and climate on FG biomass were quantified by the interaction effect index (IEI), which compares the cumulative mean size effect of two paired factors with the sum of their individual effects. IEI is given by:
where Abs = absolute value, effect FC = FC-REF, effect F = F-REF, effect C= C-REF (Allgeier et al., 2011; Villar-Argaiz et al., 2018). Values of IEI>0 allowed identifying synergistic effects (i.e. larger than purely additive), while IEI<0 enabled disentangling antagonistic effects between fisheries and climate (lower than additive effects).
The mean FG biomasses at the end of the simulation (2046-2050) were clustered by considering positive or negative FG response at each scenario with respect to REF. The FGs were also ranked for each cluster by summing mean ratio across scenarios.
3 Results
3.1 Fitting and validation of the model
The first trial was done considering the default setting for all vulnerabilities (k=0) and the fitting indices were SS= 157.96 and AIC = -559.93 (Table S1). The best fit was obtained by tuning 20 vulnerabilities despite the 40 df: SS was reduced by more than 50% with respect to the starting trial (SS= 80.12) and AIC was -905.10 (Table S1). Fitting of biomass and functional group catches was fairly successful although in several cases, such as for cephalopods (CEBH, CEPH, CEPS) and decapods (DNH, DNS), the model fails in accounting for the observed interannual fluctuations but simulates a mean value through the observed data (Figure S2A). Among catches, medium-size red mullet (MUT2) showed the worst fitting (Figure S2B).
Biomass validation of the most important commercial groups showed that the model satisfactorily fits the observed trends with the exception of juvenile red mullet (MUT1) (Figure S3).
Landings and revenues from observations (DCF) and the model were in good accordance and showed the same order of magnitude between source of datasets throughout the years available (2013-2018) (Figures S4A, B).
3.2 Effort reduction vs reference scenario
Trends of the REF scenario show that keeping the fishing pressure at the current level will result in decreased biomass for most FGs, especially those targeted by bottom trawling (blue lines in Figure 2, Figures S5, S6). Large top predators such as swordfish (XIP), bluefin tuna (THU), and large pelagic fish (LPL) after an increase due to a prey release of horse mackerel (TRA), reach a plateau in 5-10 years. Benthic cephalopods (CEBH, CEBS) and crustacean decapods (DNH, DNS) were estimated to slightly increase because of a predatory relaxation resulting from depletion of carnivorous demersal (mainly hake) by fisheries (Figure 2, in blue).
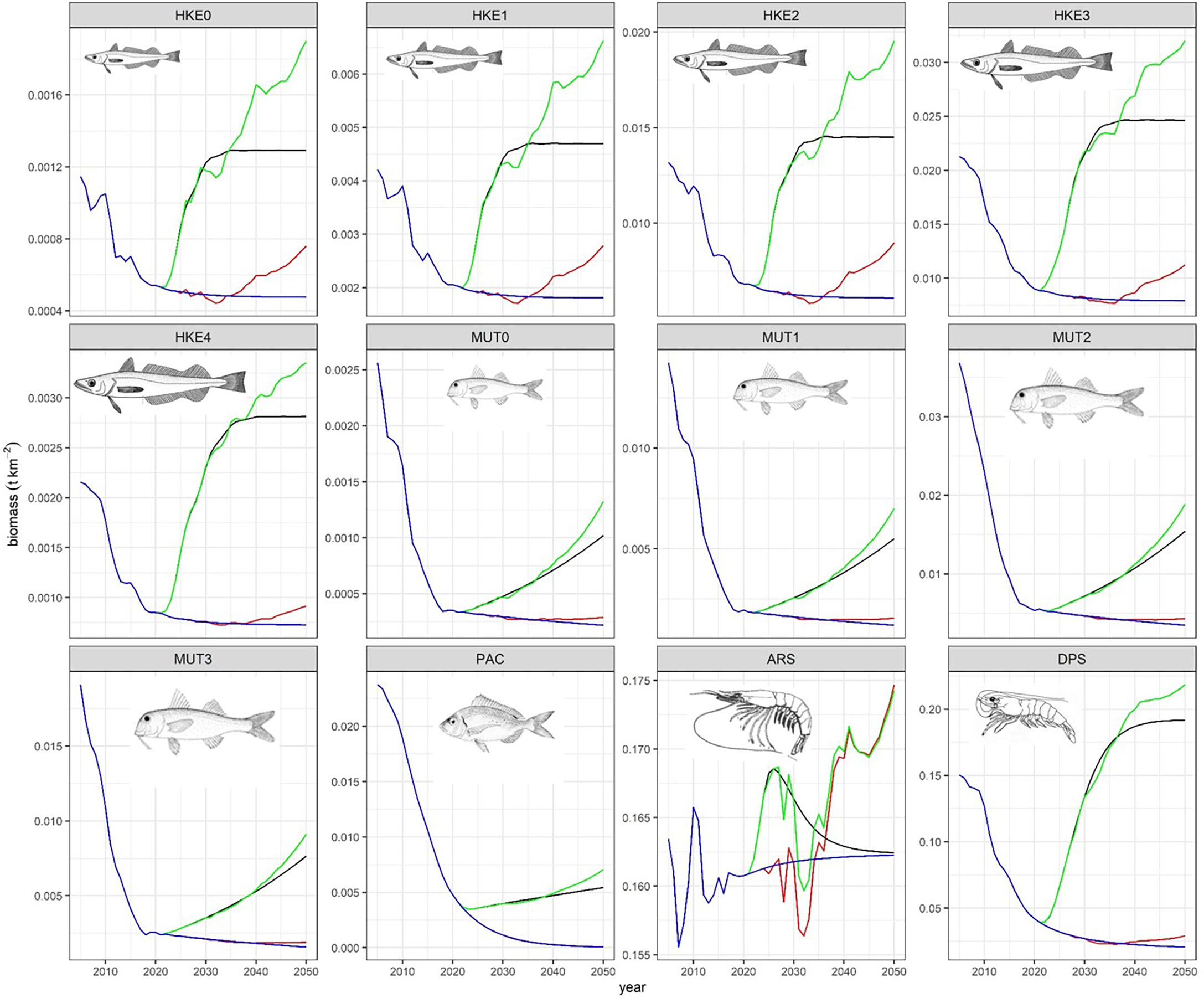
Figure 2 Trends of biomass along projection (2018-2050) and across scenarios, blue=REF, black= F, red= C, green= FC. The 12 groups comprise about 60% of bottom trawlers catches and 50% of total catches.
When a reduction of effort was applied (scenario F, black lines in figures), valuable commercial species target of bottom trawlers such as pandora (PAC), deep-water rose shrimp (DPS), hake (HKE0-4), red mullet (MUT0-3), giant red shrimp (ARS) underwent a quick rebuilding just after 2024 (i.e. after complete effort reduction). These FGs responded with positive changes one order of magnitude higher than the rest of FGs (Figure 2). The biomass of other FGs belonging to cephalopods (CEBH, CEBS, CEPH) and rays (RSS) also resulted in an exceptional recovery. In some cases, such as for Hake, an equilibrium was reached after about 15 years, but the giant red shrimp (ARS) decreased after the year 2030. Comparing F to REF, FGs showed a mean 1.40 ± 1.10 fold increase with a minimum (0.85 ± 0.09) for horse mackerel (TRA) and a maximum (16.53 ± 21.11) for pandora (PAC) (Figure 3). Horse mackerel and large top predators (LPL and THU) assumed low values in mean increase by resulting at the bottom of the ordered group’s list (Figure 3).
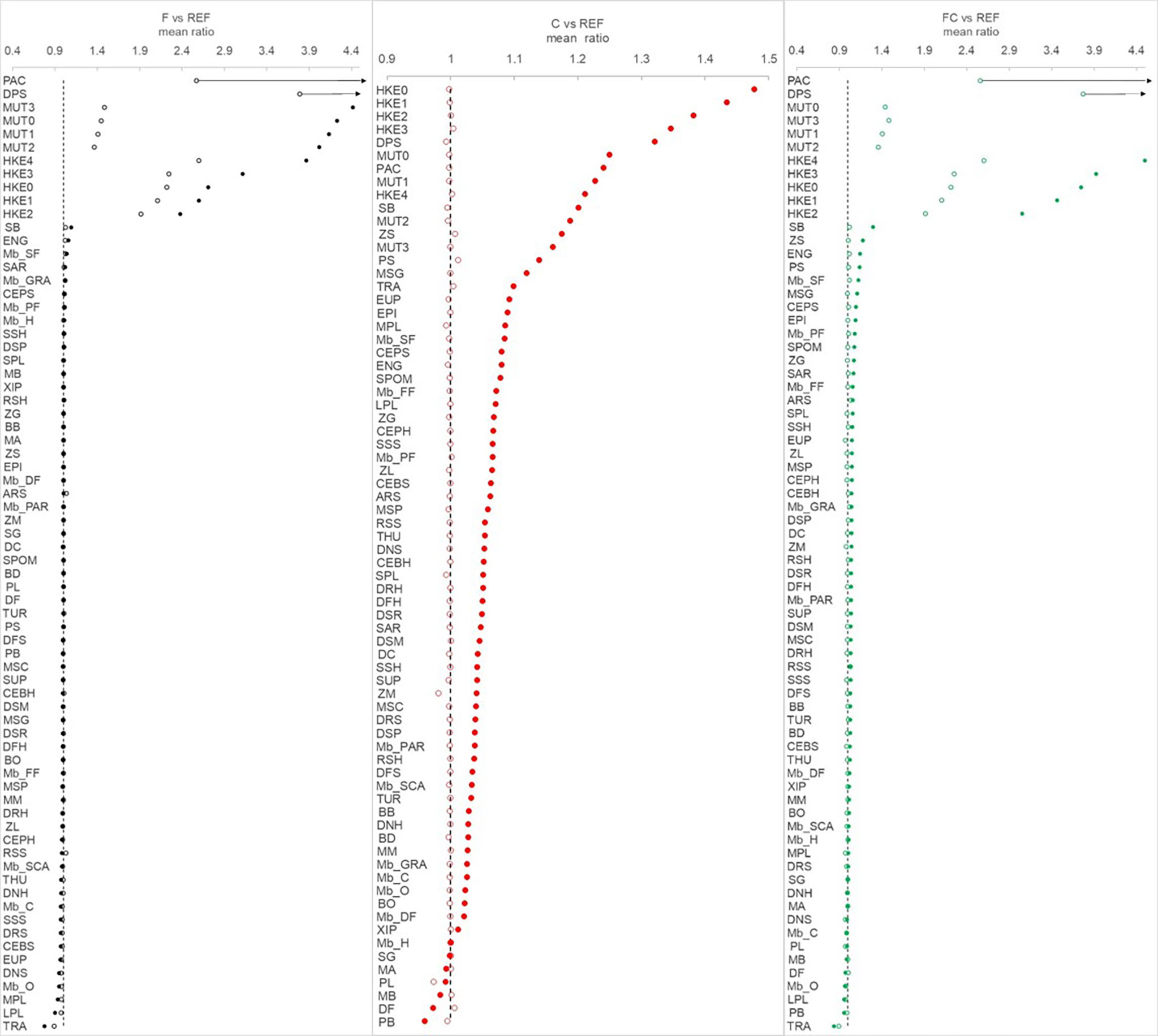
Figure 3 Mean ratio of biomass calculated for each functional group by comparing alternative scenarios (F, C, FC) with the reference (REF, y=1, dotted black line) across the years 2026-2030 (open circle) and 2046-2050 (solid circle). Ending values of PAC and DPS in F and FC scenario are outside of the scale (values reported in the main text).
As concerns the impact on fleet production, in scenario F the reduction of effort in years 2022-2024 clearly caused an initial drop in bottom trawl landings (Figure 4, fleets 11-13), but from 2025, since an important rebuilding was recorded for the main target species (Figure 5), a rise of landings to or above REF can be observed (Figures 4, 6). The maximum increase was 1.28 ± 0.20 fold for 3.OTB_MDD (fleet 13, Figure 6), in spite of a mean ratio of 1.03 ± 0.09 and a minimum of 0.94 ± 0.03 fold for 2.OTM (fleet 9) with respect to the REF scenario. Effort reduction of the bottom trawlers had an indirect positive effect on landings of gillnets (2.GNS) and on small-scale longline (1.LLD) that increased by 5% and 4% respectively. On the contrary, a moderate negative effect (-4%) resulted for small purse seiners (1.PS) and mid-water trawlers (2.OTM), and a slight negative effect (-2%) was also evident on the landings of polyvalent-license vessels (1.2.MIS) (Figure 6).
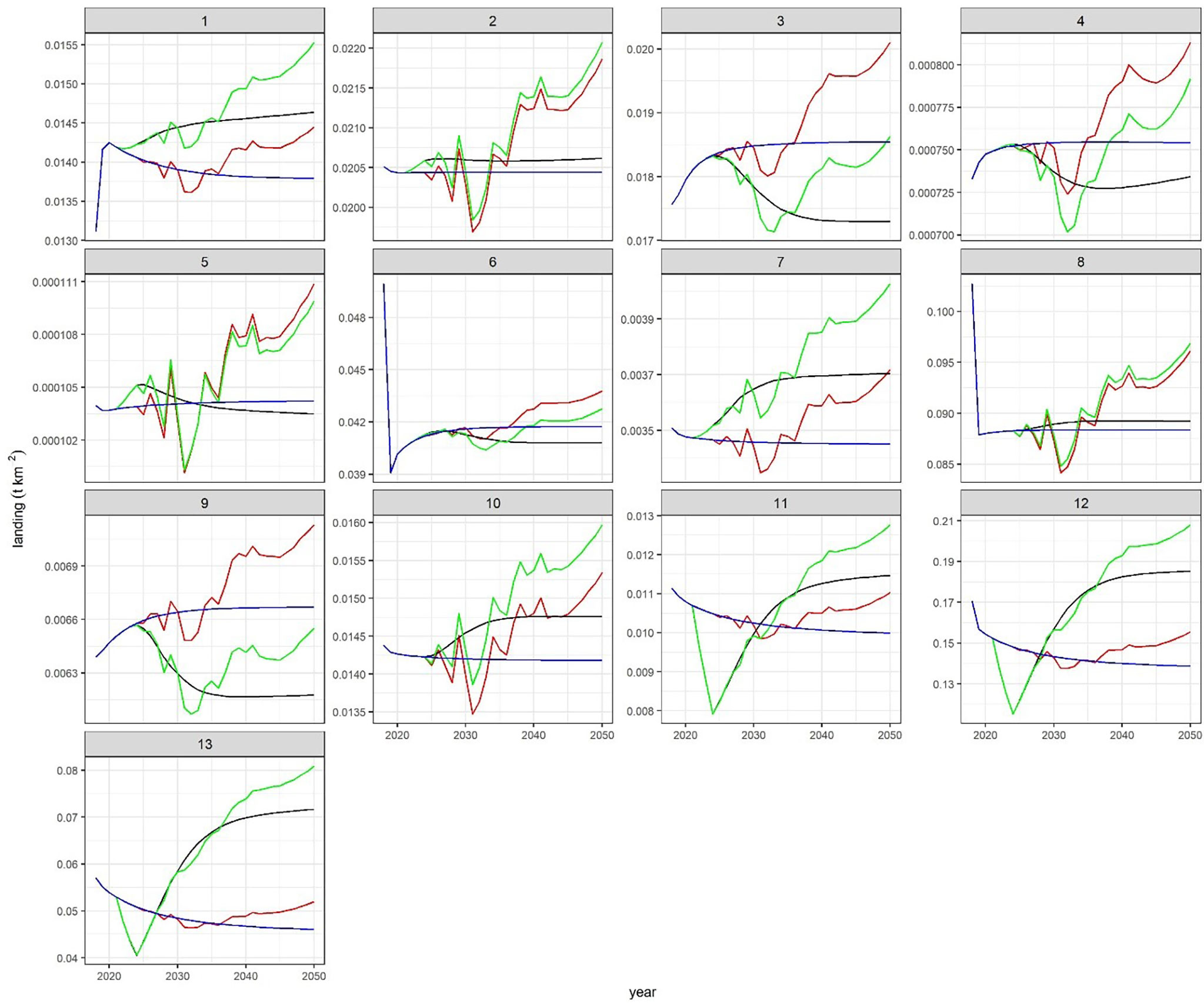
Figure 4 Trends of landings per fleet across reference (REF) and alternative scenarios (F,C,FC). Fleet: 1 = 1LL, 2 = 1GN, 3 = 1PS, 4 = 1.2MIS, 5 = 1.2FPO, 6 = 2LL, 7 = 2GN, 8 = 2.3PS, 9 = 2.3OTM, 10 = 2PTM, 11 = 1OTB, 12 = 2OTB, 13 = 3OTB. REF = blue, F = black, C = red, FC = green.
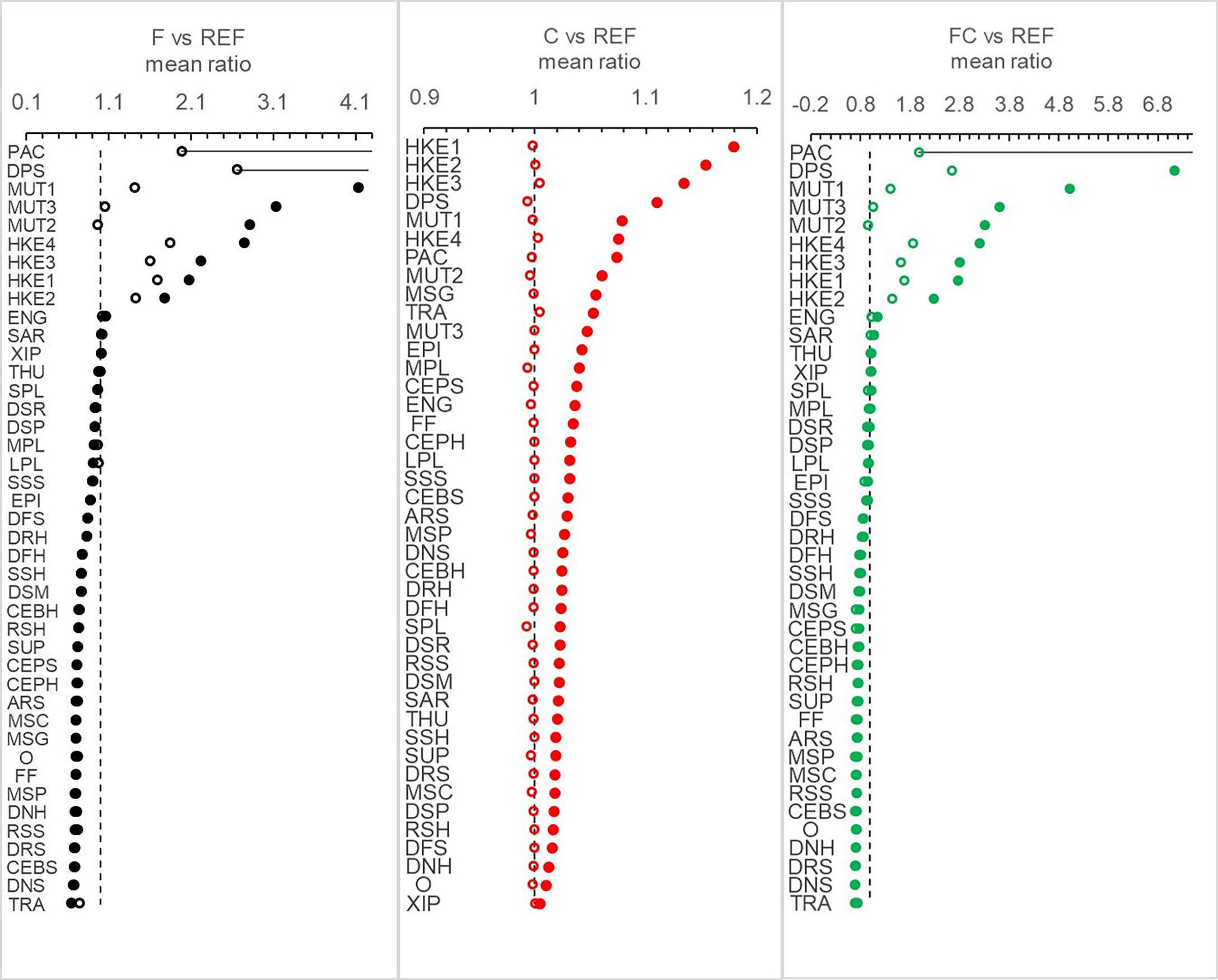
Figure 5 Mean ratio of landings per functional group calculated between the alternative scenarios (F, C, FC) and the reference (REF) in the years 2026-2030 and 2046-2050.
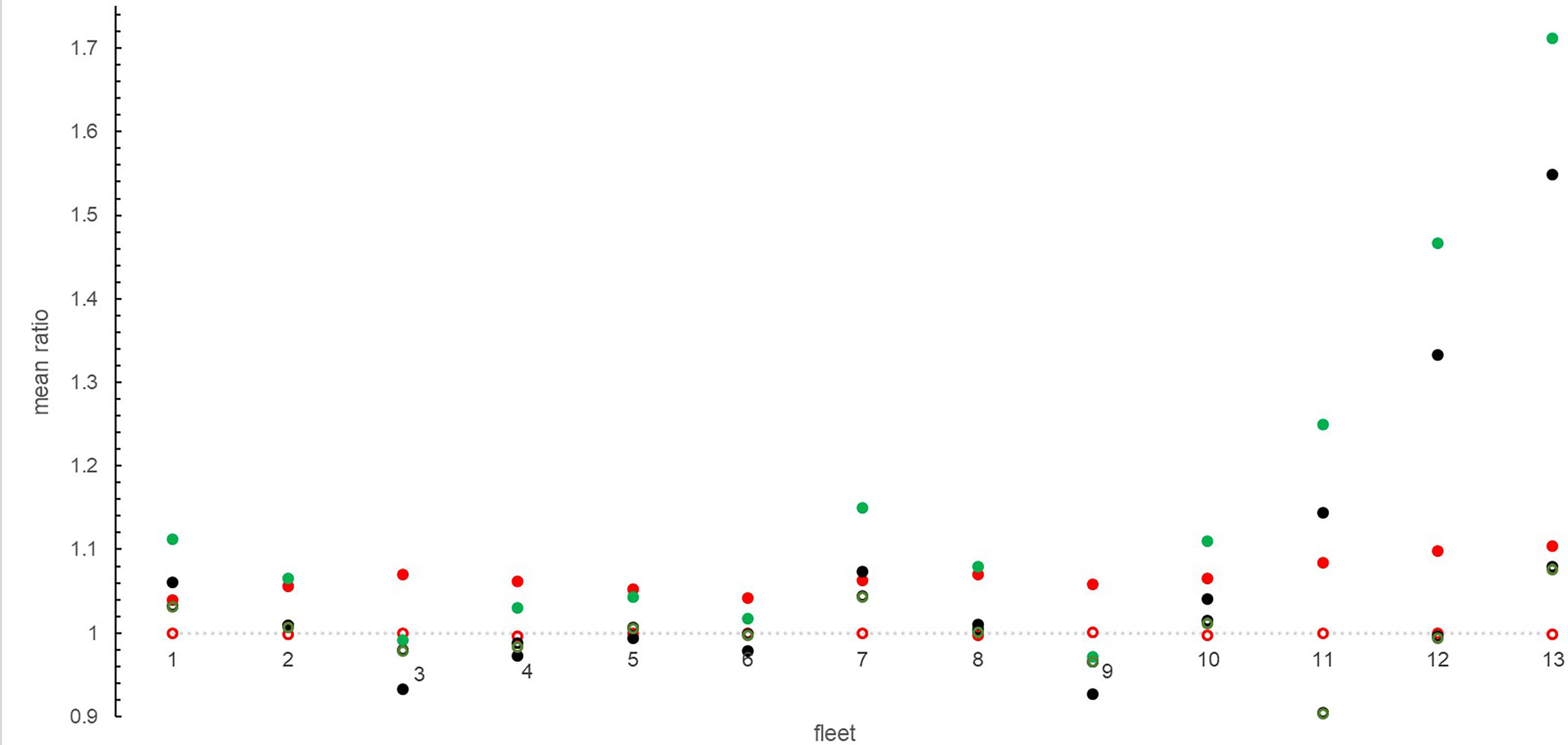
Figure 6 Mean ratio of fleet landings calculated between the alternative scenarios (F, C, FC) and the reference (REF) in the years 2026-2030 (open circle) and 2046-2050 (solid circle). F = black, C = red, FC = green.
The effects of the scenario on IPUE were analogous to what was observed for landings (Figure 7), but during the phase of progressive effort reduction (2022-2024) bottom trawlers did not lose IPUE. Higher positive changes resulted for bottom trawling (1.31 ± 0.18 fold) and slight to moderate negative effects (maximum IPUE loss< 10%) for small purse seiners (1.PS and 2.3PS), polyvalent-license vessels (1.2MIS) and mid-water trawlers (2.OTM) (Figure 8).
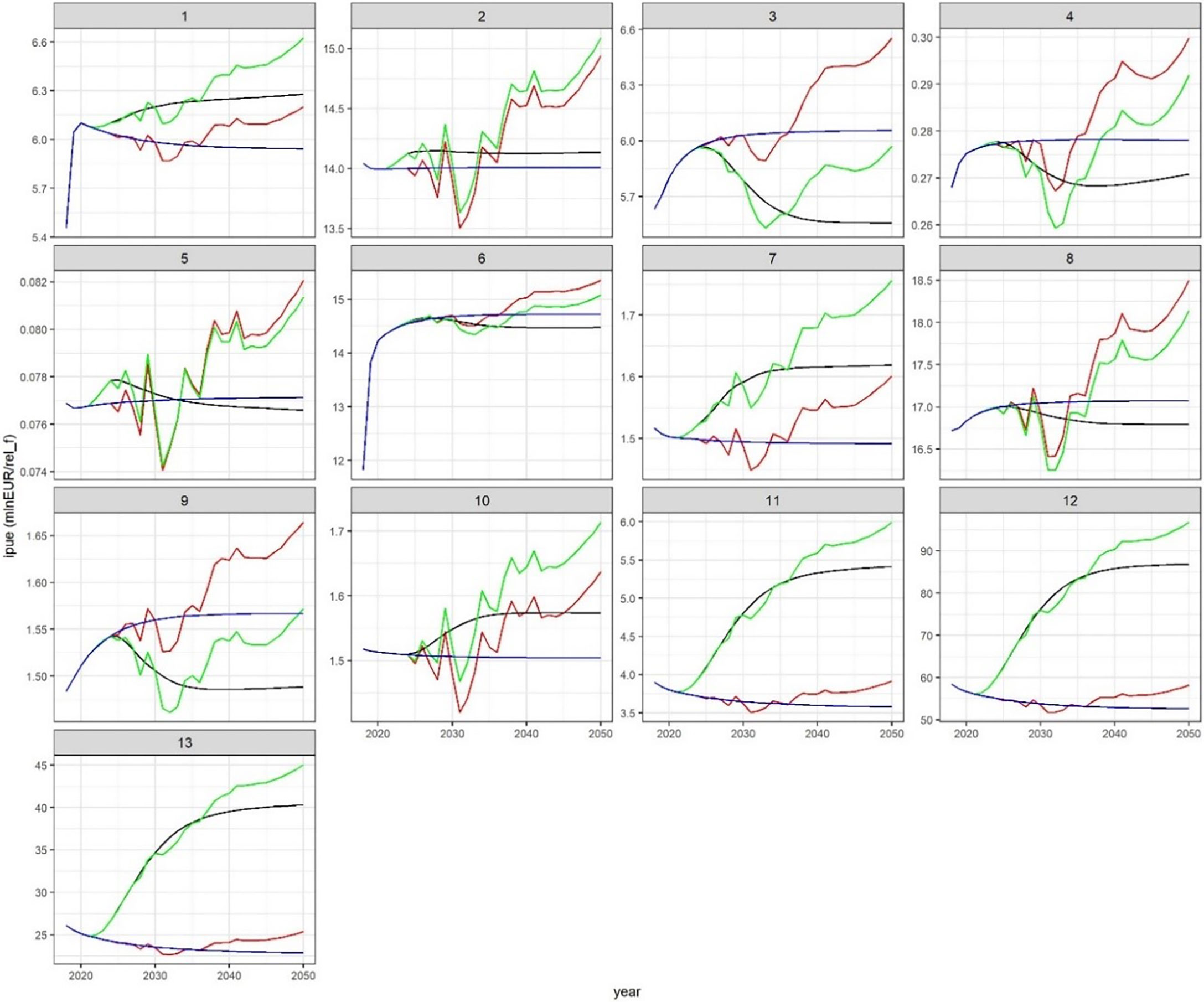
Figure 7 Projection (2018-2050) of income per unit of relative effort (IPUE) across scenarios for each fleet. Fleet: 1 = 1LL, 2 = 1GN, 3 = 1PS, 4 = 1.2MIS, 5 = 2FPO, 6 = 2LL, 7 = 2GN, 8 = 2.3PS, 9 = 2.3OTM, 10 = 2PTM, 11 = 1OTB, 12 = 2OTB, 13 = 3OTB.
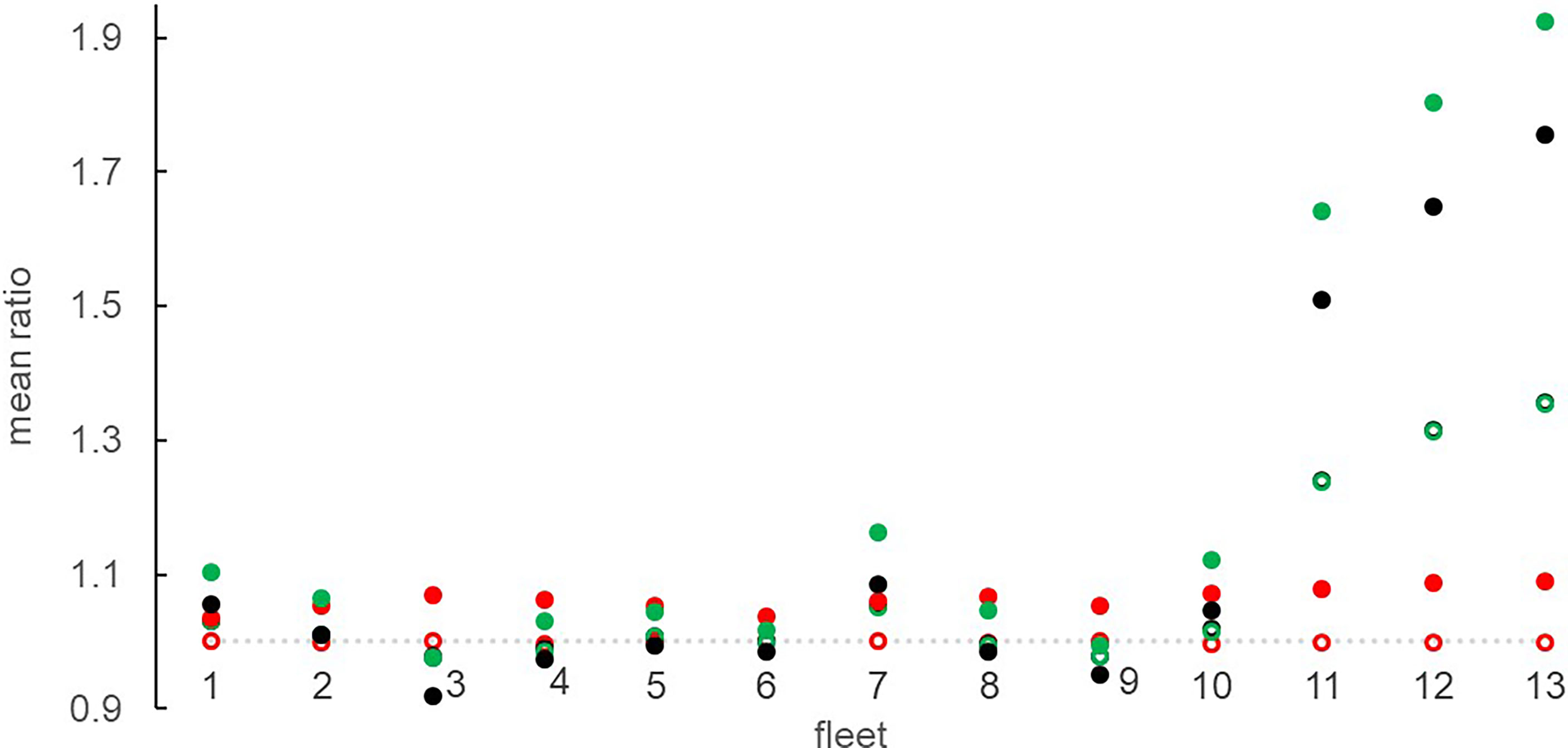
Figure 8 Mean IPUE ratio of 13 fleets, calculated between the alternative scenarios (F, C, FC) and the reference (REF) in the years 2026-2030 (open circle) and 2046-2050 (solid circle). F = black, C = red, CF = green.
3.3 Climate (C and FC) vs reference scenario
Considering climate scenarios (C and FC vs. REF, red and green lines, respectively), most FGs showed an increase in biomass (Figures 2, S6) with a mean ratio of 1.021 ± 0.004 fold in C and 1.51 ± 2.23 fold in FC (Figure 3). Among the low trophic level FGs, small phytoplankton (PS, ~ +5%) and zooplankton small (ZS, ~ +6%) resulted in the top 15 increased FGs, whereas large phytoplankton (PL) and pelagic bacteria (PB) showed the largest biomass reduction (< -2%). As a result, total biomass of phytoplankton did not significantly change (<2%) across scenarios. Horse mackerel (TRA) and pelagic fish such as large pelagic fish (LPL) and bluefin tuna (THU) appeared high in the ordered list only in C scenario, suggesting bottom-up effects linked to prey release (Figure 3).
Climate scenarios showed an overall increase of landings per fleet (Figure 4) and per FG (Figure 5), but combined effects of effort reduction and climate change caused contrasting responses. PAC, DPS, HKE, and MUT showed the highest increase in C and FC scenarios (Figure 5) with mean ratios ranging between 1.03 (MUT3, in C) and 4.2 (DPS, in FC), though PAC in FC registered an exceptional mean increase of about 14-fold. In the FC scenario, a slight enhancement of the positive effects of trawling reduction (for example in OTBs) was also evident, as well as smoothing effect of the negative ones (for example in 1.PS) (Figure 6).
In agreement to what was observed for landings, IPUE was positively affected in the climate scenarios (Figure 7), with higher ratios in FC for bottom trawling (from 1.36 ± 0.23 to 1.52 ± 0.34 fold) than in any other fleet. Moreover, in FC a positive reinforced effect of bottom trawling reduction was evident on IPUE of small longlines (fleet 1), gillnet (fleet 7) and pair trawlers (fleet 10), while a smoothing effect resulted for all fleets affected by IPUE loss (Figure 8).
3.4 Interactive effects of effort reduction and climate on biomass
The analysis of differences in biomasses at the end of the simulation (2046-2050) for F, C and FC scenarios with respect to REF resulted in 5 clusters that are reported in Figure 9, together with IEI highlighting synergistic and antagonistic effects. Results show that synergistic effect of effort reduction and climate change was detected for 34 FGs, that is 47% out of total cases (72 FGs) covering the whole spectrum of trophic levels. The highest synergistic climate-fisheries effect was detected for natant decapods from the slope (DNS,IEI=0.6) and the highest antagonistic for reptant decapods from the slope (DRS) and medium pelagic fish (MPL) (IEI of -1.37 and -1.33, respectively).

Figure 9 Increase (+) or decrease (-) in mean ratio (2046-2050) of functional group (FG) biomass was evaluated as an FG response to the different scenarios and used for a qualitative clustering. In each cluster, FGs are in ascendant order by considering a cumulative response (algebraic sum of mean ratio) across scenarios. Interactive Effect Index (IEI) is given to show synergistic (blue) and antagonistic (red) effect between effort reduction and climate on FG.
The blue cluster, made up of 37 out of 72 FGs having increases in biomass in all scenarios (F, C and FC), showed that climate change and fishing effort reduction mainly had a positive synergistic effect i.e. an increase of biomass in all scenarios. The most important commercial target species of bottom trawling were at the top ten of the cluster because direct fishing release effects were predominant. This caused values of change to be orders of magnitude higher in F and FC than in C (Figure 3). The 18 FGs belonging to the yellow cluster increased in biomass in climatic scenarios (C and FC) but showed biomass reductions in F scenario. In this cluster, positive effects of climate in terms of biomass through the food web (i.e. relaxation of predation and/or release of prey) were higher than the negative ones caused by reducing fishing. The grey cluster was comprised three primary producer groups (SG, MA, MB), which benefited only from the reduction of their consumers (i.e. a mesopredator release) in scenario F. Antagonistic effects dominated yellow and grey clusters.
Eleven FGs are listed in the red cluster, which encompasses the FGs that responded positively to C scenario only, showing that the effect of reduced fishing effort embedded in the other two scenarios drives a biomass reduction. A mix of high synergistic and high antagonistic effects characterize the red cluster.
Finally, three small planktonic groups i.e. dinoflagellates (DF), phytoplankton large (PL) and pelagic bacteria (PB) (green cluster), showed a biomass decrease in all alternative scenarios indicating a negative synergistic effect of effort reduction and climate.
4 Discussion
Sustaining wild fish production, maintaining jobs and revenues of fishing enterprises while protecting biodiversity is a relevant issue to be faced by the Mediterranean fishing sector. Following the growing body of scientific evidence, the EU, national commissions and Regional Fisheries Management Organization, such as the FAO General Fisheries Commision for the Mediterranean Sea (GFCM), are supporting the development of management plans aimed at including combinations of measures to achieve sustainable fisheries. These plans are mainly based on the reduction of fishing effort and discards for trawlers and the implementation of fisheries restricted areas to protect nurseries of commercial species (see the CFP and the GFCM strategy), and should induce the reduction of fishing mortality and the rebuilding of overexploited stocks (Fiorentino and Vitale, 2021). However, recent global analyses highlighted the overfished status of resources (Froese et al., 2018) and a lack of effective rebuilding plans for the Mediterranean Sea (Melnychuk et al., 2021). This suggests the need for understanding which management measures could be considered to increase the sustainability of fisheries, also by taking the impact of global climate change in action into account.
A dynamic food web model applied to the SoS was developed to quantify, in a multifleet-multispecies framework, the potential effects of the reduction of bottom trawling effort in combination with changes of primary production induced by climate changes.
The ongoing fisheries exploitation pattern simulated in the reference scenario showed a decreasing biomass trend for most FGs in the SoS, confirming the overexploitation status of many target species in the Mediterranean Sea as reported in several syntheses (Colloca et al., 2017; FAO, 2020). Results of the reference scenario highlight a decrease in biomass for the main target species until 2050 under the current (2019) exploitation rate.
When a 30% reduction of bottom trawling effort (i.e. a yearly 10% decrease for 3 consecutive years) was applied, an overall increase in biomass for the main target species (hake, red mullet, deep water rose shrimp) resulted in 6-7 years. In particular, European hake reached about a 2-fold steady state biomass after 10 years since the completion of fishing effort reduction. The trend of most fished species was similar to that observed in effective marine managed areas, in coastal (Edgar et al., 2014; Dimarchopoulou et al., 2019) as well as in open sea systems (Pipitone et al., 2014). In particular, the main demersal fish species targeted by bottom trawling showed a biomass rebuilding of the same order of magnitude reported in a Sicilian trawl exclusion area (mean 8-fold increase) (Pipitone et al., 2000). In addition, a SMART model simulation considering a 15% reduction of trawl fishing effort in the SoS showed an increase of spawning biomass of red mullet and giant red shrimp after 6 years (Russo et al., 2019).
The overall fish biomass increase led to an important mid-term increase (after 2024) of landings and IPUE mainly due to direct rebuilding of target species, but also indirect food web effects deriving from the reduction of trawling. After a short time of transition (3 years of effort reduction implementation), favorable conditions for higher catch and incomes resulted especially for bottom trawlers but also for gillnets with which they share a main target species (i.e. hake). In the scenario of fishing effort reduction for trawlers (scenario F), landings and IPUE of longlines and purse seiners would be instead negatively affected in a slight/moderate (<5%) indirect way. In fact, the trawling effort reduction caused a release of predators impacting on preys 1) directly targeted by a fleet, e.g. impairing the interactions among HKE_SAR_purse seine, and/or 2) whose target species feed on, as in the case of longline (example HKE_TRA_THU_longline). These findings suggest that bottom trawlers suffer mainly from an intra-fleet competition and secondarily a competition with the fleets sharing the main resources (GNS) or accessory catches. Results suggest that direct and indirect effects of trawl effort reduction are thus minimal on small scale fisheries (Figures 6 and 8, fleets 2, 4 and 5 in particular), although further analyses are required to explore effects of fishers adaptations (e.g., changing from trawling to SSF). However, bottom trawlers could also play a role of stabilizer on the landings of purse seiners by relaxing natural top-down effects on small pelagic fish.
Although global climate models project that net primary productivity will very likely decline by 4–11% for RCP8.5 at the end of this century (Couespel et al., 2021; Bindoff et al., 2022) with negative consequences for fish catches (Tittensor et al., 2021), the decline has expected to show regional variations due to biological and modeling factors (Bindoff et al., 2022). For the Mediterranean Sea, the specific biogeochemical model projections under high emission scenario (Solidoro et al., 2022) suggest an increment in both plankton gross productivity and system total respiration, yielding a decrement in the concentrations of total plankton biomass, chlorophyll and particulate organic carbon, among other variables. However, our results have showed that local variations of primary production related to climate change in the mid-term (2050) resulted in a small increase in total biomass (<2%) of primary producers and in a more important rearrangement of relative biomass of the lowest trophic levels. In fact, pico-nano phytoplankton (PS, +5%) and nano-micro zooplankton (ZS, +6%) increased at the expense of the larger phytoplankton (PL) and mesozooplankton (ZM) which remained quite stable or in slight decrement on average. These modifications in biomass of primary producers fuelled bottom-up energetic pathways through the food web and triggered a slight (about 1%) increase in biomass of higher trophic levels by medium-small pelagic fish groups. Similar projections have recently been obtained with the application of an end-to-end model in the Mediterranean as whole (Moullec et al., 2019) and have been suggested by other authors (Marquis et al., 2011) also in relation to past extreme global warming phases (Britten and Sibert, 2020). Keeping the fishing effort constant, the effect of climate change alone on landings and income per unit of effort was small but positive for all the fleets considered. However in this scenario, target species did not undergo any important recovery.
Notably, our results also show an interplay between fishing management and climate mediated by specific responses of food web components (i.e. FGs), so that the effects of the two drivers could act either synergistically or antagonistically.
Although uncertainty characterize projections of primary production (Kwiatkowski et al., 2020), we have showed that its changes could play a relevant role in affecting the biomass of different functional groups and their predator-prey relationships. Moreover our analysis support the conclusion that, effort reduction is the most important driver in determining the SoS ecosystem mid-term sustainable evolution. Complex and nested interactions through the SoS food web were expected (Agnetta et al., 2019), but the reliability of the results might be improved by implementing the model with processes and effects linked to spatial and habitat modifications as well as the effects of other physical factors not explicitly used here (e.g. temperature, oxygen and alkalinity changes). However, we believe this study is an important contribution towards disentangling the effects of fishery and climate change in an intricate food web such as that of the SoS.
Rebuilding exploited populations is a management objective that starts with assessing single species targets to identify specific goals, but then it has to lead to a broad ecosystem approach to fisheries management, in order to preserve the community size-structure, the ecological role of different species and the integrity of habitat functioning (Link et al., 2002; Jennings and Rice, 2011).
Here, we showed that under the current fishing regime, stock productivity and fleet profitability are generally impaired by high fishing mortality. Although climate change and fishing interact, fishing impacts are faster in producing change on marine ecosystems, therefore implementing sustainability by means of fisheries management plans is urgent. An essential aspect, however, is that of management at the transnational level (Mackelworth et al., 2019): the reduction of fishing effort in an area involving shared stocks such as the one examined in this study can be effective only if implemented to all active vessels (i.e. trawlers) in order to avoid spatial imbalances which could undermine the local management strategy measures. This aspect is even more important in areas, such as the SoS, where main stocks, such as hake, deep water rose shrimp and giant red shrimp, are shared between several coastal countries (Jarboui et al., 2022).
The reduction of the bottom trawling effort includes a short transitional period with loss in catches and incomes as reported by other authors (Russo et al., 2019) with potential short-term negative repercussions on socio-economic aspects, which should be adequately addressed by compensatory measures during the transitional period, but positive long-term effects that could outweigh the negative ones including those affecting the other fleets. Although sustainability is a trade-off process merging many aspects of our society, the reduction of the trawling effort seems a way, possibly accompanied by adaptation of fisheries and other management measures, to support the efficiency of fishery processes and the goals for sustainability. Subsidiarity, illegal fishing and slavery at sea are modern issues (Sumaila et al., 2020) not taken into account here, but we also showed that the maintenance of fixed prices during biomass rebuilding might contribute to compensate for the negative social effects produced by bottom trawl fishing reductions.
Data availability statement
The original contributions presented in the study are included in the article/Supplementary Material. Further inquiries can be directed to the corresponding author.
Author contributions
DA and SL conceptualized the work and wrote the paper. DA developed the model and analyzed the data. All the authors contributed to the development of the model by sharing important datasets and revised the manuscript.
Funding
Catch, effort, price, and abundance at sea data were collected within the European Data Collection Framework (DCF) funded by the European Union and the Italian Ministry for Agricultural, Food and Forestry Policies.
Acknowledgments
The authors acknowledge the anonymous English reviewer provided by OGS services for its constructive comments and suggestions and others colleagues (Giovanni D’Anna and Michele Gristina from CNR) that shared some data for the previous mass balanced version of the model. A special thanks to Marco Reale (OGS) who contributed with biogeochemical model analysis for climate scenario.
Conflict of interest
The authors declare that the research was conducted in the absence of any commercial or financial relationships that could be construed as a potential conflict of interest.
Publisher’s note
All claims expressed in this article are solely those of the authors and do not necessarily represent those of their affiliated organizations, or those of the publisher, the editors and the reviewers. Any product that may be evaluated in this article, or claim that may be made by its manufacturer, is not guaranteed or endorsed by the publisher.
Supplementary material
The Supplementary Material for this article can be found online at: https://www.frontiersin.org/articles/10.3389/fmars.2022.909164/full#supplementary-material
References
Agnetta D., Badalamenti F., Colloca F., D’Anna G., Di Lorenzo M., Fiorentino F., et al. (2019). Benthic-pelagic coupling mediates interactions in Mediterranean mixed fisheries: An ecosystem modeling approach. PloS One 14, e0210659. doi: 10.1371/journal.pone.0210659
Ahrens R. N. M., Walters C. J., Christensen V. (2012). Foraging arena theory. Fish. Fish. 13, 41–59. doi: 10.1111/j.1467-2979.2011.00432.x
Alexander K. A., Heymans J. J., Magill S., Tomczak M. T., Holmes S. J., Wilding T. A. (2015). Investigating the recent decline in gadoid stocks in the west of Scotland shelf ecosystem using a foodweb model. ICES. J. Mar. Sci. 72, 436–449. doi: 10.1093/icesjms/fsu149
Allgeier J. E., Rosemond A. D., Layman C. A. (2011). The frequency and magnitude of non-additive responses to multiple nutrient enrichment. J. Appl. Ecol. 48, 96–101. doi: 10.1111/j.1365-2664.2010.01894.x
Araújo J. N., Mackinson S., Stanford R. J., Hart P. J. B. (2008). Exploring fisheries strategies for the western English channel using an ecosystem model. Ecol. Modell. 210, 465–477. doi: 10.1016/j.ecolmodel.2007.08.015
Bertrand J. A., Gil de Sola L., Papaconstantinou C., Relini G., Souplet A., Souplet A. (2002). The general specifications of the MEDITS surveys. Sci. Mar. 66, 9. doi: 10.3989/scimar.2002.66s29
Bindoff N. L., Cheung W. W. L., Kairo J. G., Arístegui J., Guinder V. A., Hallberg R., et al. (2022). Changing ocean, marine ecosystems, and dependent communities In: IPCC Special Report on the Ocean and Cryosphere in a Changing ClimatePörtner H. O., Roberts D. C., Masson-Delmotte V., Zhai P., Tignor M., Poloczanska E., Mintenbeck K, et al. (eds.) ( Cambridge, UK and New York, NY, USA:Cambridge University Press), pp. 447–587. doi: 10.1017/9781009157964.007
Bonanno A., Zgozi S., Cuttitta A., El Turki A., Di Nieri A., Ghmati H., et al. (2013). Influence of environmental variability on anchovy early life stages (Engraulis encrasicolus) in two different areas of the central Mediterranean Sea. Hydrobiologia 701, 273–287. doi: 10.1007/s10750-012-1285-8
Britten G. L., Sibert E. C. (2020). Enhanced fish production during a period of extreme global warmth. Nat. Commun. 11(5636), 1–6. doi: 10.1038/s41467-020-19462-w
Carpi P., Scarcella G., Cardinale M. (2017). The saga of the management of fisheries in the adriatic sea: History, flaws, difficulties, and successes toward the application of the common fisheries policy in the mediterranean. Front. Mar. Sci. 4. doi: 10.3389/fmars.2017.00423
Christensen V., Walters C. J. (2004). Ecopath with ecosim: Methods, capabilities and limitations. Ecol. Modell. 172, 109–139. doi: 10.1016/j.ecolmodel.2003.09.003
Coll M., Libralato S. (2012). Contributions of food web modelling to the ecosystem approach to marine resource management in the Mediterranean Sea. Fish. Fish. 13, 60–88. doi: 10.1111/j.1467-2979.2011.00420.x
Colloca F., Scarcella G., Libralato S. (2017). Recent trends and impacts of fisheries exploitation on Mediterranean stocks and ecosystems. Front. Mar. Sci. 4. doi: 10.3389/fmars.2017.00244
Corrales X., Coll M., Ofir E., Heymans J. J., Steenbeek J., Goren M., et al. (2018). Future scenarios of marine resources and ecosystem conditions in the Eastern Mediterranean under the impacts of fishing, alien species and sea warming. Sci. Rep. 8, 14284. doi: 10.1038/s41598-018-32666-x
Cossarini G., Feudale L., Teruzzi A., Bolzon G., Coidessa G., Solidoro C., et al. (2021). High-resolution reanalysis of the Mediterranean Sea biogeochemistry, (1999–2019). Front. Mar. Sci. 8. doi: 10.3389/fmars.2021.741486
Couespel D., Lévy M., Bopp L. (2021). Oceanic primary production decline halved in eddy-resolving simulations of global warming. Biogeosciences 18, 4321–4349. doi: 10.5194/bg-18-4321-2021
De Juan S., Demestre M., Sanchez P. (2011). Exploring the degree of trawling disturbance by the analysis of benthic communities ranging from a heavily exploited fishing ground to an undisturbed area in the NW Mediterranean. Sci. Mar. 75, 507–516. doi: 10.3989/scimar.2011.75n3507
Dimarchopoulou D., Keramidas I., Tsagarakis K., Tsikliras A. C. (2019). Ecosystem models and effort simulations of an untrawled gulf in the central Aegean Sea. Front. Mar. Sci. 6, 648. doi: 10.3389/fmars.2019.00648
Edgar G. J., Stuart-Smith R. D., Willis T. J., Kininmonth S., Baker S. C., Banks S., et al. (2014). Global conservation outcomes depend on marine protected areas with five key features. Nature 506, 216–220. doi: 10.1038/nature13022
FAO. (2019). Social protection for small-scale fisheries in the Mediterranean region – a review. (Rome:FAO). Available at: https://www.fao.org/3/ca4711en/ca4711en.pdf.
FAO. (2020). “The state of world fisheries and aquaculture 2020,” in Sustainability in action. (Rome:FAO).
Fiorentino F., Vitale S. (2021). How can we reduce the overexploitation of the Mediterranean resources? Front. Mar. Sci. 8. doi: 10.3389/fmars.2021.674633
Froese R., Winker H., Coro G., Demirel N., Tsikliras A. C., Dimarchopoulou D., et al. (2018). Status and rebuilding of European fisheries. Mar. Policy 93, 159–170. doi: 10.1016/j.marpol.2018.04.018
Garofalo G., Giusti G. B., Cusumano S., Igrande G., Sinacori G., Gristina M., et al. (2007). Catch per unit effort of red shrimp in bathyal fishing grounds fo the Eastern Mediterranean. Biol. Mar. Mediterr. 14, 250–251.
Giorgi F. (2006). Climate change hot-spots. Geophys. Res. Lett. 33, L08707. doi: 10.1029/2006GL025734
Hattab T., Leprieur F., Ben Rais Lasram F., Gravel D., Loc’h Le F., Albouy C. (2016). Forecasting fine-scale changes in the food-web structure of coastal marine communities under climate change. Ecography (Cop.). 39, 1227–1237. doi: 10.1111/ecog.01937
Heymans J. J., Coll M., Link J. S., Mackinson S., Steenbeek J., Walters C., et al. (2016). Best practice in ecopath with ecosim food-web models for ecosystem-based management. Ecol. Modell. 331, 173–184. doi: 10.1016/j.ecolmodel.2015.12.007
Hilborn R., Amoroso R. O., Anderson C. M., Baum J. K., Branch T. A., Costello C., et al. (2020). Effective fisheries management instrumental in improving fish stock status. Proc. Natl. Acad. Sci. U. S. A. 117, 2218–2224. doi: 10.1073/pnas.1909726116
ICCAT. (2010) Collective volume of scientific papers - international commission for the conservation of Atlantic tunas ICCAT (Madrid, Spain).
Jacquet J., Pauly D.. (2008) Funding priorities: big barriers to small-scale fisheries. Conserv. Policy 22, 832–835. doi: 10.1111/j.1523-1739.2008.00978.x
Jarboui O., Ceriola L., Fiorentino F.. (2022). “Current fisheries management in the strait of Sicily and progress towords an ecosystem approach,” in Transition towards an ecosystem approach to fisheries in the Mediterranean Sea - lessons learned through selected case studies. Eds. Vasconcellos M., Ünal V.. (Rome:FAO), 147–162.
Jennings S., Rice J. (2011). Towards an ecosystem approach to fisheries in Europe: a perspective on existing progress and future directions. Fish. Fish. 12, 125–137. doi: 10.1111/j.1467-2979.2011.00409.x
Kiørboe T. (2013). Zooplankton body composition. Limnol. Oceanogr. 58, 1843–1850. doi: 10.4319/lo.2013.58.5.1843
Kwiatkowski L., Torres O., Bopp L., Aumont O., Chamberlain M., Christian J. R., et al. (2020). Twenty-first century ocean warming, acidification, deoxygenation, and upper-ocean nutrient and primary production decline from CMIP6 model projections. Biogeosciences 17, 3439–3470. doi: 10.5194/bg-17-3439-2020
Lazzari P., Solidoro C., Ibello V., Salon S., Teruzzi A., Béranger K., et al. (2012). Seasonal and inter-annual variability of plankton chlorophyll and primary production in the Mediterranean Sea: A modelling approach. Biogeosciences 9, 217–233. doi: 10.5194/bg-9-217-2012
Libralato S., Agnetta D. (2019). From ecological trade-offs to resilience: insights from exploited marine ecosystems. Curr. Opin. Syst. Biol. 13, 136–141. doi: 10.1016/j.coisb.2018.12.005
Link J. S., Brodziak J. K., Edwards S. F., Overholtz W. J., Mountain D., Jossi J. W., et al. (2002). Marine ecosystem assessment in a fisheries management context. Can. J. Fish. Aquat. Sci. 59, 1429–1440. doi: 10.1139/f02-115
Mackelworth P. C., Teff Seker Y., Vega Fernández T., Marques M., Alves F. L., D’Anna G., et al. (2019). Geopolitics and marine conservation: Synergies and conflicts. Front. Mar. Sci. 6. doi: 10.3389/fmars.2019.00759
Marquis E., Niquil N., Vézina A. F., Petitgas P., Dupuy C. (2011). Influence of planktonic foodweb structure on a system’s capacity to support pelagic production: an inverse analysis approach. ICES. J. Mar. Sci. 68, 803–812. doi: 10.1093/icesjms/fsr027
Melnychuk M. C., Kurota H., Mace P. M., Pons M., Minto C., Osio G. C., et al. (2021). Identifying management actions that promote sustainable fisheries. Nat. Sustain. 4, 440–449. doi: 10.1038/s41893-020-00668-1
Milisenda G., Vitale S., Massi D., Enea M., Gancitano V., Giusto G. B., et al. (2017). Spatio-temporal composition of discard associated with the deep water rose shrimp fisheries (Parapenaeus longirostris, Lucas 1846) in the south-central Mediterranean Sea. Mediterr. Mar. Sci. 18, 53–63. doi: 10.12681/mms.1787
Moullec F., Barrier N., Drira S., Guilhaumon F., Marsaleix P., Somot S., et al. (2019). An end-to-end model reveals losers and winners in a warming Mediterranean Sea. Front. Mar. Sci. 6. doi: 10.3389/fmars.2019.00345
Pipitone C., Badalamenti F., D’Anna G., Patti B. (2000). Fish biomass increase after a four-year trawl ban in the gulf of castellammare (NW Sicily, Mediterranean Sea). Fish. Res. 48, 23–30. doi: 10.1016/S0165-7836(00)00114-4
Pipitone C., Badalamenti F., Vega Fernández T., D’Anna G. (2014). “Spatial management of fisheries in the Mediterranean Sea: Problematic issues and a few success stories.,”. in Marine managed areas and fisheries. Eds. Johnson M. L., Sandell J.(Oxford: Academic Press), 371–402.
Pisano A., Marullo S., Artale V., Falcini F., Yang C., Leonelli F. E., et al. (2020). New evidence of Mediterranean climate change and variability from Sea surface temperature observations. Remote Sens. 12, 132. doi: 10.3390/RS12010132
Raicevich S., Grati F., Giovanardi O., Sartor P., Sbrana M., Silvestri R., et al. (2020). “The unexploited potential of small-scale fisheries in Italy: Analysis and perspectives on the status and resilience of a neglected fishery sector,”, in Small-scale fisheries in Europe: Status, resilience and governance. Ed. Pascual-Fernández J. (Switzerland:Springer Nature), 191–211. doi: 10.1007/978-3-030-37371-9_10
Reale M., Cossarini G., Lazzari P., Lovato T., Bolzon G., Masina S., et al. (2022). Acidification , deoxygenation , nutrient and biomasses decline in a warming Mediterranean Sea. Biogeosciences. Discuss., 1–44. doi: 10.5194/bg-2021-301
Riahi K., Rao S., Krey V., Cho C., Chirkov V., Fischer G., et al. (2011). RCP 8.5-a scenario of comparatively high greenhouse gas emissions. Clim. Change 109, 33–57. doi: 10.1007/s10584-011-0149-y
Russo T., D’Andrea L., Franceschini S., Accadia P., Cucco A., Garofalo G., et al. (2019). Simulating the effects of alternative management measures of trawl fisheries in the central Mediterranean Sea: Application of a multi-species bio-economic modeling approach. Front. Mar. Sci. 6. doi: 10.3389/fmars.2019.00542
Russo T., D’Andrea L., Parisi A., Cataudella S. (2014). VMSbase: An r-package for VMS and logbook data management and analysis in fisheries ecology. PloS One 9, e100195. doi: 10.1371/journal.pone.0100195
Salon S., Cossarini G., Bolzon G., Feudale L., Lazzari P., Teruzzi A., et al. (2019). Novel metrics based on biogeochemical argo data to improve the model uncertainty evaluation of the CMEMS Mediterranean marine ecosystem forecasts. Ocean. Sci. 15, 997–1022. doi: 10.5194/os-15-997-2019
Soetaert M., Lenoir H., Verschueren B. (2016). Reducing bycatch in beam trawls and electrotrawls with (electrified) benthos release panels. ICES. J. Mar. Sci. 73, 2370–2379. doi: 10.1093/icesjms/fsw096
Solidoro C., Cossarini G., Lazzari P., Galli G., Bolzon G., Somot S., et al. (2022). Modeling carbon budgets and acidification in the Mediterranean Sea ecosystem under contemporary and future climate. Front. Mar. Sci. 8. doi: 10.3389/fmars.2021.781522
Spedicato M. T., Massutí E., Mérigot B., Tserpes G., Jadaud A., Relini G. (2019). The MEDITS trawl survey specifications in an ecosystem approach to fishery management. Sci. Mar. 83, 9. doi: 10.3989/scimar.04915.11X
Sumaila U. R., Zeller D., Hood L., Palomares M. L. D., Li Y., Pauly D. (2020). Illicit trade in marine fish catch and its effects on ecosystems and people worldwide. Sci. Adv. 6, eaaz3801. doi: 10.1126/sciadv.aaz3801
Teh L. C. L., Sumaila U. R. (2013). Contribution of marine fisheries to worldwide employment. Fish. Fish. 14, 77–88. doi: 10.1111/j.1467-2979.2011.00450.x
Tittensor D. P., Novaglio C., Harrison C. S., Heneghan R. F., Barrier N., Bianchi D., et al. (2021). Next-generation ensemble projections reveal higher climate risks for marine ecosystems. Nat. Clim. Change 11, 973–981. doi: 10.1038/s41558-021-01173-9
UN (2019) World population prospect 2019. Available at: https://population.un.org/wpp/.
Villar-Argaiz M., Medina-Sánchez J. M., Biddanda B. A., Carrillo P. (2018). Predominant non-additive effects of multiple stressors on autotroph C:N:P ratios propagate in freshwater and marine food webs. Front. Microbiol. 9. doi: 10.3389/fmicb.2018.00069
Vitale S., Milisenda G., Gristina M., Baiata P., Bonanomi S., Colloca F., et al. (2018). Towards more selective Mediterranean trawl fisheries: are juveniles and trash excluder devices effective tools for reducing undersized catches? Sci. Mar. 82, 215–223. doi: 10.3989/scimar.04751.28A
Walters C., Christensen V. (2007). Adding realism to foraging arena predictions of trophic flow rates in ecosim ecosystem models: Shared foraging arenas and bout feeding. Ecol. Modell. 209, 342–350. doi: 10.1016/j.ecolmodel.2007.06.025
Keywords: ecosystem impact, fishery sustainability, food-webs, strait of Sicily, ecosim model
Citation: Agnetta D, Badalamenti F, Colloca F, Cossarini G, Fiorentino F, Garofalo G, Patti B, Pipitone C, Russo T, Solidoro C and Libralato S (2022) Interactive effects of fishing effort reduction and climate change in a central Mediterranean fishing area: Insights from bio-economic indices derived from a dynamic food-web model. Front. Mar. Sci. 9:909164. doi: 10.3389/fmars.2022.909164
Received: 31 March 2022; Accepted: 22 July 2022;
Published: 12 August 2022.
Edited by:
Athanassios C. Tsikliras, Aristotle University of Thessaloniki, GreeceReviewed by:
Peter Goethals, Ghent University, BelgiumSion Letizia, University of Bari Aldo Moro, Italy
Copyright © 2022 Agnetta, Badalamenti, Colloca, Cossarini, Fiorentino, Garofalo, Patti, Pipitone, Russo, Solidoro and Libralato. This is an open-access article distributed under the terms of the Creative Commons Attribution License (CC BY). The use, distribution or reproduction in other forums is permitted, provided the original author(s) and the copyright owner(s) are credited and that the original publication in this journal is cited, in accordance with accepted academic practice. No use, distribution or reproduction is permitted which does not comply with these terms.
*Correspondence: Davide Agnetta, dagnetta@ogs.it