Aquatic species shows asymmetric distribution range shifts in native and non-native areas
- 1Institute of Marine Sciences - OKEANOS, University of the Azores, Horta, Portugal
- 2Department of Oceanography and Fisheries, Institute of Marine Research (IMAR), University of Azores, Horta, Portugal
- 3Centre of Marine Sciences (CCMAR), University of Algarve, Campus de Gambelas, Faro, Portugal
- 4Sustainable and Smart Aquaculture Collaborative Laboratory, Olhão, Portugal
- 5Institute of Environment, Florida International University, Miami, FL, United States
Introduction: Climate change is reshaping the geographical distribution of species across the globe. In marine ecosystems, climate change creates novel challenges to an environment impacted by numerous anthropogenic stressors. Forecasting shifts in species distribution, including the expansion of non-indigenous species under climate change scenarios, is a management challenge for today’s world.
Methods: We applied Bayesian Additive Regression Tree (BART) models to investigate the environmental factors modulating the occurrence and habitat preferences of the Atlantic blue crab Callinectes sapidus Rathbun, 1896 in native and non-native areas. With BART models, we also aimed to predict its current and future distributions under two climate change scenarios (RCPs 4.5 and 8.5). BART models were performed using global occurrences of the Atlantic blue crab – recorded from 1830 to 2022 – and several environmental covariates (i.e., water temperature, salinity, current velocity, and rugosity). Model accuracy was assessed with the Area Under the Curve (AUC) and True Skill Statistics (TSS) criteria. Cross-validation experiments were made to balance accuracy prediction and uncertainty model prediction intervals.
Results and discussion: AUC and TSS values indicated that data validation was successful for the BART model. Water temperature was the most critical variable affecting the presence probability of the Atlantic blue crab. The BART model predicts asymmetric distribution range shifts on both sides of the Atlantic Ocean. The non-native populations will experience broader shifts in their distribution range than in the native range, and the RCP 8.5 scenario model outputs predict a wider distribution range by the end of the century. Overall, we anticipate significant ecological changes in native and non-native areas. The range expansion in native areas is often equivalent to the ecological shifts induced by invasive species, so lessons learned by ecologists and managers in non-native areas will provide actionable insights to managers in native areas.
1 Introduction
The abundance and distribution of marine species changed quickly throughout the 20th century due to multiple anthropogenic stressors, including habitat modification and destruction, overexploitation, pollution, and the introduction of non-indigenous species (Halpern et al., 2015; Corrales et al., 2018). In the 21st century, global climate steers species distribution in terrestrial and aquatic ecosystems has become more evident (Poloczanska et al., 2013; Molinos et al., 2015; Pecl et al., 2016; Molinos, 2020). For some species, their distribution range contracted (e.g., alpine trees (Engler et al., 2011; Charbonnel et al., 2016), others were extirpated (e.g., Bay checkerspot butterfly Euphydryas editha bayensis) (Sternitsky, 1937), southern sea lions Otaria flavescens (Shaw, 1800), mountain wildflower Androsace septentrionalis Linnaeus 1753)) (McLaughlin et al., 2002; Baylis et al., 2015; Panetta et al., 2018), while many others experienced a degradation of their conservation status because they now live under sub-optimal temperature conditions that disrupt fitness, reproduction, survival, or established biotic interactions (e.g., white-beaked dolphin Lagenorhynchus albirostris (Gray, 1846), reef-buiding coral Diploastrea heliopore (Lamarck, 1816), golden kelp Laminaria ochroleuca Bachelot Pylaie 1824, brook trout Salvelinus fontinalis (Mitchill, 1814), and Chinese giant salamander Andrias davidianus (Blanchard, 1871) – Elliott and Simmonds, 2007; Cantin et al., 2010; Warren et al., 2012; Blois et al., 2013; Franco et al., 2018; Zhang et al., 2020). The poleward expansion and tropicalization of temperate regions is another phenomenon indicative of the global changes we are witnessing in terrestrial and aquatic ecosystems, from terrestrial plants to invertebrates, but also reptiles, fish, and mammals in multiple regions across the globe (Zeidberg and Robison, 2007; Azzurro et al., 2011; Encarnação et al., 2019; Pinsky et al., 2020; Osland et al., 2021).
The continuous change of world ecosystems due to global climate change, in tandem with other anthropogenic impacts, reshapes communities while opening the door to non-indigenous species to settle or become invasive (Willis et al., 2010; King et al., 2021; Wang et al., 2021). The factors involved in the expansion of non-indigenous species go beyond habitat suitability since biotic resistance and stochastic events may play a significant part in halting or slowing colonization and invasiveness (Brown and Barney, 2021). The Atlantic blue crab Callinectes sapidus Rathbun, 1896 is native to the western Atlantic Ocean, from the coasts of Massachusetts (United States) to central Argentina (Mancinelli et al., 2021). In Europe, it has become one of the fastest-spreading marine invasive species (Mancinelli et al., 2017; Mancinelli et al., 2021; Fuentes et al., 2019; Encarnação et al., 2021a; Encarnação et al., 2022), the reason why it is on the list of the 100 worst invasive species in the Mediterranean Sea (Streftaris and Zenetos, 2006). The oldest records in Europe date from 1900 in the Bay of Biscay (Atlantic Ocean), 1935 in Greece (Mediterranean Sea), 1951 in the Baltic Sea, and 1967 in the Black Sea and Portugal (Atlantic Ocean) (Nehring, 2011; Encarnação et al., 2022). On the western coast of Portugal, the 15 scattered records reported between 1967 and 2019 suggest that the species had not established a viable population (Encarnação et al., 2022), but this changed in 2016 after the first specimens were found in southern Portugal (Morais et al., 2019; Vasconcelos et al., 2019). Soon after, the Atlantic blue crab rapidly colonized the entire southern coast and adjacent estuarine ecosystems (Encarnação et al., 2021a) and then expanded into the west coast to locations where previous introductions have failed (Encarnação et al., 2022).
In the native range, the Atlantic blue crab stocks are overexploited along the northwest Atlantic coast (Aguilar et al., 2008; Semmler et al., 2021), while biotic interactions with the invasive European green crab Carcinus maenas (Linnaeus, 1758) exert additional pressure, mainly to juveniles (MacDonald et al., 2007). Nevertheless, at higher abundances, the Atlantic blue crab may be able to limit the invasion of the European green crab in North America (DeRivera et al., 2005). In Europe, and particularly in the Gulf of Cadiz, the native European green crab does not seem to represent a threat to the Atlantic blue crab because it has become a successful invader in locations where native crabs are abundant (Rodríguez et al., 1997; Oliveira et al., 2000; Sprung, 2001; Encarnação et al., 2013; Morais et al., 2019; González-Ortegón et al., 2022). The Atlantic blue crab has also become a profitable fishery in local fishing communities in Portugal, Spain, Greece, Turkey, and Tunisia (Nehring, 2011; Mancinelli et al., 2017; Vasconcelos et al., 2019; Encarnação et al., 2021a; Clavero et al., 2022).
Predicting changes in the distribution of a species in native and non-native regions has conservation as a common goal. Predicting where and when a species will expand/contract its distribution, or become more or less abundant, adds valuable information about species under fishery management, like the Atlantic blue crab in the native range. The prediction of species distribution allows for the prioritization of conservation resources and increases fishers’ preparedness for changes in fisheries. Failure to do so may result in the collapse of regional fisheries, as happened with the Atlantic cod Gadus morhua (Linnaeus, 1758) in the Gulf of Maine (North America) (Pershing et al., 2015). The poleward range shifts of other commercially important marine species have been documented for the common snook Centropomus undecimalis (Bloch, 1792) in North America and predicted for others, such as the dolphinfish Coryphaena hippurus Linnaeus, 1758 (Salvadeo et al., 2020; Purtlebaugh et al., 2020). Fishing communities must then adapt to changes in species distribution, either by following the fishery or focusing on new resources in their fishing grounds (Rogers et al., 2019; Paukert et al., 2021; Galappaththi et al., 2022). In non-native areas, predicting the invasion pathway of a species is one of the most valuable tools for improving its management and minimizing/mitigating future impacts (Barbet-Massin et al., 2018; Liu et al., 2020; Lyons et al., 2020). The commercial exploitation of invasive species has been used as a mitigation measure when eradication measures are unfeasible (e.g., lionfish Pterois miles (Bennett, 1828) in the Caribean Sea (Barbour et al., 2011; Morris et al., 2011) and Mediterranean Sea (Ulman et al., 2022), weakfish Cynoscion regalis in estuaries of central Portugal (Cerveira et al., 2021), the Atlantic blue crab in the Mediterranean Sea (Mancinelli et al., 2017).
Thus, our objective was to investigate the environmental factors modulating the occurrence and habitat preferences of the Atlantic blue crab in native and non-native areas using Bayesian Additive Regression Trees (BART model), as well as predict the current and future distribution of the species under two climate change scenarios: RCPs 4.5 and 8.5. We hypothesize that 1) water temperature will be closely related to the distribution of the Atlantic blue crab in native and non-native habitats and 2) rising ocean temperature will reshape the current distribution. To test our hypotheses, we used Species Distribution Modeling (SDM) because it allows for addressing issues in conservation planning (Franklin, 2013; Villero et al., 2017), evolution (Pinsky et al., 2020), biogeography (Torn et al., 2020; Pickens et al., 2021), invasiveness potential of non-native species (Jiménez-Valverde et al., 2011; Mainali et al., 2015), and even inferring the impacts of climate change on species distribution (Lezama-Ochoa et al., 2016; Zhang et al., 2019; Guerra et al., 2021). We chose BARTs because it is a flexible SDM tool offering a wide range of options to extract relationships between species and abiotic conditions, elucidating the roles of predictive variables in determining species distributions, environmental condition preferences (two-dimensional range of environmental conditions where the species showed the highest probabilities of presence), posterior distributions on predictions as a measure of uncertainty, and optimal spatial locations for species expansion or invasion (Chipman et al., 2010; Carlson, 2020; Carlson et al., 2022). With this information, we also aim to contribute to more efficiently managing this commercially valuable species in the native and non-native ranges.
2 Materials and methods
2.1 Species data
The use of Species Distribution Models (SDMs) to predict current and future distribution ranges of marine organisms has increased substantially since the mid-2010s (Robinson et al., 2017; Guerra et al., 2021; Hernández-Ucera et al., 2021; Pickens et al., 2021). SDMs rely on the relationship between a species’ georeferenced record (e.g., presence/absence data) and environmental variables to predict the probability of being found elsewhere (Robinson et al., 2017). So, we downloaded global spatial occurrences data for the Atlantic blue crab from 1830 to 2020 (data made available by Mancinelli et al. (2021) – https://doi.org/10.6084/m9.figshare.12896309). These authors compiled data from multiple databases/sources of published and unpublished records: Global Biodiversity Information System (GBIF, www.gbif.org), Ocean Biogeographic Information System (OBIS, www.obis.org), Biodiversity Information Serving Our Nation (BISON, https://bison.usgs.gov), iNaturalist (www.inaturalist.org). Additional records made between 2019 and March 2022 were retrieved from GBIF, scientific literature (Encarnação et al., 2021a; Chaouti et al., 2022; Encarnação et al., 2022; Encarnação et al., 2023), and unpublished records submitted to our citizen science campaign New Marine Species of the Algarve (NEMA – Novas Espécies Marinhas do Algarve, www.NEMAlgarve.com). The datasets were merged, and then the duplicates, doubtful identifications, and mismatched geographic coordinates (i.e., coordinates outside the marine environment) were removed. In the end, we were left with 27,664 valid records for subsequent analyses (Figure 1).
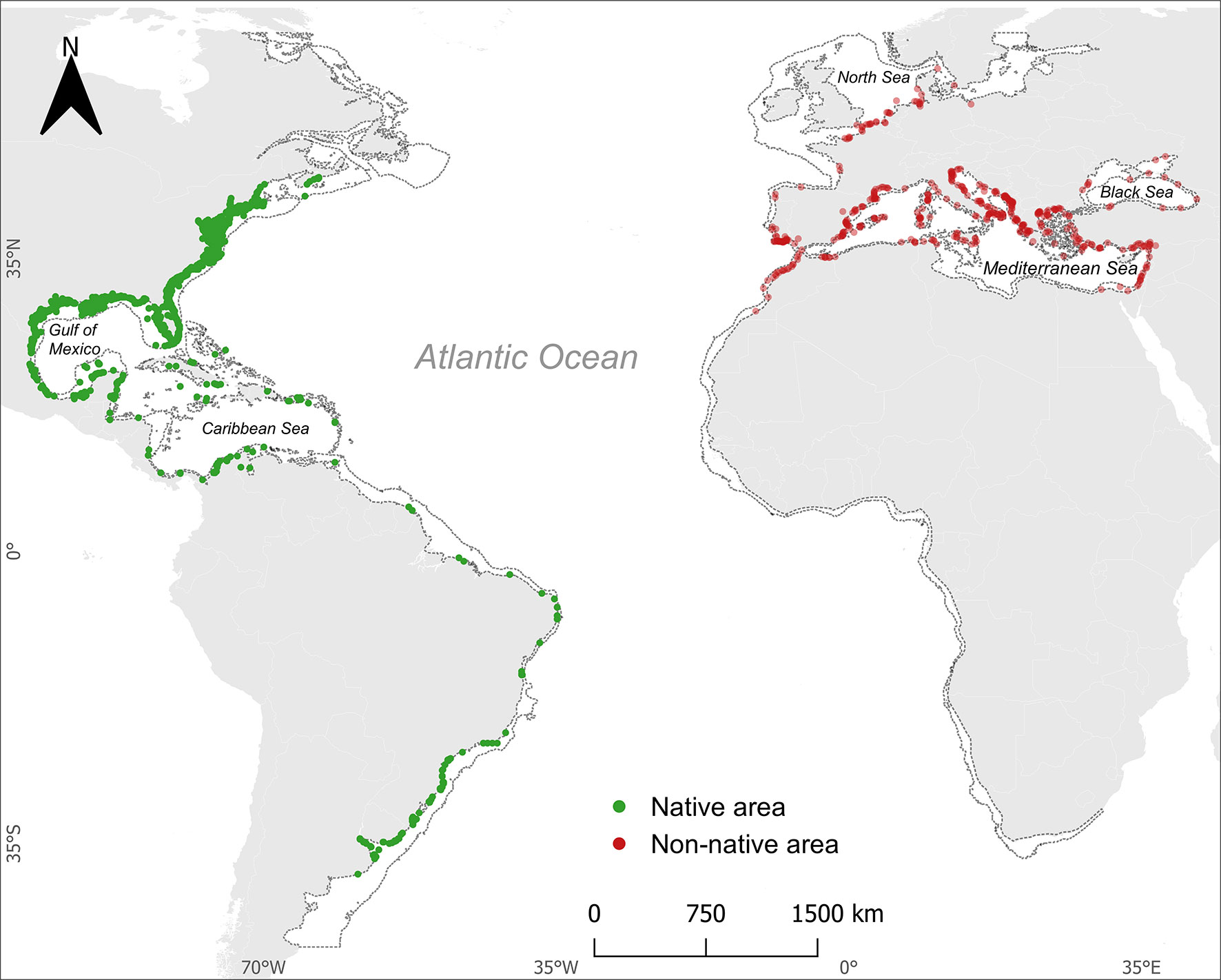
Figure 1 Location of the 2130 Atlantic blue crab Callinectes sapidus Rathbun, 1896 presence points used for Bayesian Additive Regression Tree modeling. Dotted lines represent the maximum depth of 200 m. The red dots represent the records collected in the non-native area, while the green dots represent the records collected in native area.
We used spatial filtering to reduce overfitting and improve model quality (Boria et al., 2014; Tourne et al., 2019) and kept one record per grid cell (spatial resolution of 0.083° × 0.083°) to reduce record clustering, which often results from sampling bias (Boria et al., 2014; Sillero and Barbosa, 2021). Thus, we were left with 2130 presence points to use in model training.
2.2 Environmental predictor variables
The environmental predictor variables, i.e., the covariates, were selected based on data availability and their physiological and ecological importance to marine species. So, we included water temperature (°C), salinity, current velocity (m.s-1), and depth (m) as covariates. The first three variables were downloaded as mean for the surface marine realm and the latter as mean depth. We used depth to estimate rugosity, an index often used to predict species distribution and suitable habitats, as well as an indicator of hard-bottom habitat (seafloor heterogeneity) (Dunn and Halpin, 2009; McArthur et al., 2010; Fonseca et al., 2017; Guerra et al., 2021). The rugosity index was derived from the depth layer using the ‘Terrain Ruggedness Index’ of the raster package in the R software (R Core Team, 2021). The rugosity index was calculated as the mean of absolute differences between a cell’s value and its eight surrounding cells. Low rugosity values indicate unconsolidated substrate (i.e., mud and sand), whereas high rugosity values are associated with rocky substrates (Riley et al., 1999; Fonseca et al., 2017). All covariate layers were downloaded from the public database Bio-Oracle v2.0 which provides environmental variables under current conditions (2000-2014) and projections for the 2040s (2040-2049) and 2090s (2090-2099), considering the concentration of greenhouse gas emissions in the Representative Concentration Pathway (RCP) trajectories of climate change adopted by the IPCC (Intergovernmental Panel on Climate Change) (Tyberghein et al., 2012; Assis et al., 2018). We used the moderate (RCP 4.5) and the most pessimistic trajectory projections (RCP 8.5) to predict areas of occurrence of the Atlantic blue crab for the 2040s (mid-term) and 2090s (long-term) (Supplementary Table 1; Supplementary Figure 1).
Pearson’s correlation coefficient (r) and Variation Inflation Factor (VIF) were applied to detect correlation and collinearity between covariates by using the R packages corrplot and usdm, respectively (Zuur et al., 2010; Naimi et al., 2014; Wei and Simko, 2021). The lowest and highest correlation recorded were 0.18 and 0.39, respectively (Supplementary Figure 2). VIF values were lower than 2, ranging from 1.13 to 1.28 (Supplementary Table 2). Therefore, no significant correlations and collinearity were detected, and no covariate was excluded before data modeling. The Bio-ORACLE dataset was retrieved on March 19, 2022, from the Bio-ORACLE’s website (Bio-ORACLE, 2022).
2.3 BART modeling
Bayesian Additive Regression Trees (BARTs) were performed to predict the probability of the Atlantic blue crab’s presence as a function of a set of environmental covariates. BART is a Bayesian approach to non-parametric function estimation with the ability to capture complex interactions and nonlinear relationships among predictors. This is accomplished by using a sum-of-trees framework (Chipman et al., 2010). BART model can be expressed as:
where is the vector of response variable, is the design matrix (predictors); represents the distinct regression trees, each composed of a tree structure , and parameters of the terminal nodes (leaves), as an ensemble , …, , denoted by ; is each prediction of a set of n trees; and = is vector noise, representing independent mean-zero normal error with variance σ. A set of posterior draws of sum-of-trees functions, generated by the Markov Chain Monte Carlo process, create the posterior probability for . For binary response , like absence (0) presence (1) data, BART uses a logit link: , where Φ denotes the standard normal cumulative distribution function. A complete description of BART models can be found in Chipman et al. (2010).
The absence data were randomly generated using the function ‘randomPoints()’ (R package dismo), as pseudo-absences. The total number of pseudo-absences was the same as the number of presences, considering known species distribution and the statistical modeling approach, as recommended by Babet-Massin et al. (2012) for tree-based models (Supplementary Figure 3). Then, both presence and absence data were merged into the BART model.
BART was performed with the R package embarcadero, an SDM wrapper for the dbarts package (Chipman et al., 2010; Carlson, 2020; Dorie, 2022). The BART model was performed with all covariates (current scenario from both native and non-native areas), followed by an automated variable selection procedure using the ‘step.model()’ function. This model was trained on current data and fitted 100 times for six different settings of ensemble size (n= 10, 20, 50, 100, 150, and 200 trees) using the ‘bart()’ function (Carlson et al., 2022). This procedure was conducted to select the model with the most informative covariates and the lowest average model root mean square error (RMSE). Then, the final model was performed with 200 trees, 1000 posterior draws with a burn-in of 100 draws, and values for hyperparameters of power equal to 2.0 and base equal to 0.95 for tree regularization prior, as recommended by Chipman et al. (2010). The final BART model was used to pedict the probability of occurrence in future scenarios using the function ‘predict()’.
2.4 Model validation and evaluation
The three hyperparameters, which govern priors on Tj, Mj, and σ, were tuned in for a full cross-validation experiment to balance accuracy prediction and uncertainty model prediction intervals. The ‘retune()’ function was used to run the experiment across the k hyperprior (values of 1, 2, and 3), power parameter (1.5, 1.6, 1.7, 1.8, 1.9, and 2.0), and the base parameter (0.75, 0.80, 0.85, 0.90, and 0.95). Model accuracy was assessed by using the Area Under the Curve (AUC) of the Receiver Operational Characteristic (ROC) approach and True Skill Statistics (TSS), which considered commission (false positives: type I error) and omission errors (false negatives: type II error) (Allouche et al., 2006). Both AUC and TSS methods measure the ability of the model to correctly discriminate presence and absence data; however, TSS also accounts for both model’s sensitivity (i.e., the proportion of presences accurately predicted) and specificity (i.e., the proportion of absences accurately predicted). AUC values between 0.5 and 0.7 indicate poor prediction, from 0.7 to 0.8 is an acceptable prediction, from 0.8 to 0.9 is excellent, and greater than 0.9 is outstanding (Hosmer and Lemeshow, 2000). TSS is defined as ‘sensitivity + specificity - 1’ and ranges from -1 to +1, where +1 indicates perfect agreement and values of zero or less indicate a performance no better than random (Allouche et al., 2006).
The raster maps of the predicted posterior mean, first (0.025), and third (0.975) probability quantiles were generated from the BART model. Spatial uncertainty was calculated by mapping the differences between the two quantile rasters that generate a credible interval width (Carlson, 2020). Future layers of the predicted values were subtracted from the current predicted layer to obtain habitat change trends. The distribution shifting map under each climate change scenario was generated with positive, zero, and negative grid values that represent habitat range expansion, no change, and habitat range retraction, respectively.
All raster layers had a spatial resolution of 0.083° × 0.083° cell size (approximately 9.2 km at the equator) and were cropped to depths up to 200 m in the coastal zone of the Atlantic Ocean, encompassing the depth range of the Atlantic blue crab. This species occupies shallow estuarine and coastal waters, down to 100 m depth in offshore habitats (Hill et al., 1989; WoRMS, 2022). However, the maximum depth of the calibration area also considered that the species may shift its distribution towards deeper waters under climate change scenarios (Dulvy et al., 2008; Hastings et al., 2020). All statistical analyses were performed using R (version 4.0.0) (R Core Team, 2021). All maps were created using the QGIS Geographic Information System, version 3.24 (Open-Source Geospatial Foundation Project, http://qgis.osgeo.org).
3 Results
3.1 Presence probability and covariates
In general, the TSS (0.69) and training AUC (0.92) values indicated that the BART models performed very well on data validation with cut-off thresholds of 0.54 (Supplementary Figure 4). The final BART model included temperature as the most informative variable with the highest average importance value, followed by salinity and current velocity (Supplementary Figure 5A). (Supplementary Figure 6) On the other hand, rugosity was dropped during the covariates selection process due to the lowest RMSE value (~0.3315) (Supplementary Figure 5B).
The relationship between the probability of presence and temperature showed that the Atlantic blue crab occurred in areas with water temperatures higher than 10°C, with a high probability of being found in marine habitats between ~20 and 25°C (Figure 2A). The species also occurred in a wide range of salinity, but its presence probability decreased in waters with values higher than 31 (Figure 2B). The probability of presence decreased with increasing current velocity since over 70% of occurrences happened in calm waters (Figure 2C). The relationships between temperature – the most important covariate – and other covariates revealed the two-dimensional range of environmental conditions where the species showed the highest presence probabilities (Figure 3). Generally, high presence probabilities were associated with temperatures between ~20 to 25 °C, salinity between 25 and 32 (Figure 3A), and current velocity slower than 0.20 m.s-1 (Figure 3B).
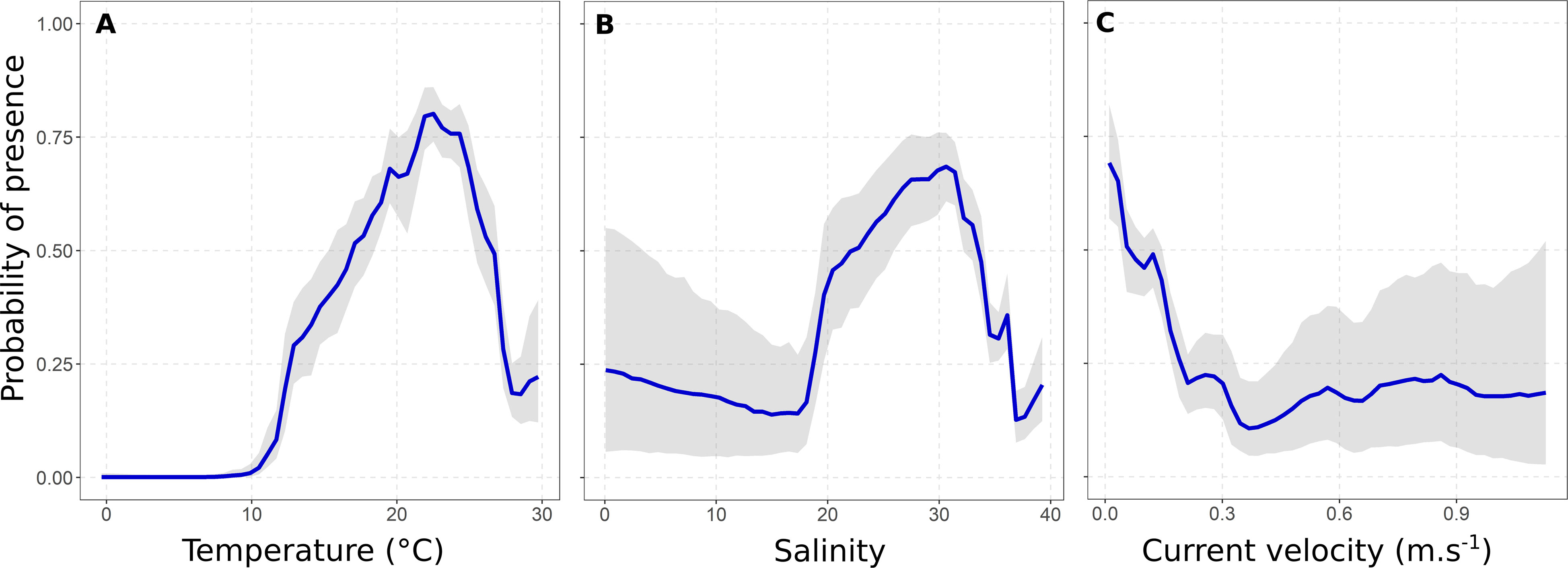
Figure 2 Smoothed fits of covariates modeling the spatial distribution of the Atlantic blue crab Callinectes sapidus Rathbun, 1896 for temperature (A), salinity (B), and current velocity (C). Gray shading indicates a 95% credible interval from the posterior distribution of the current scenario BART model.
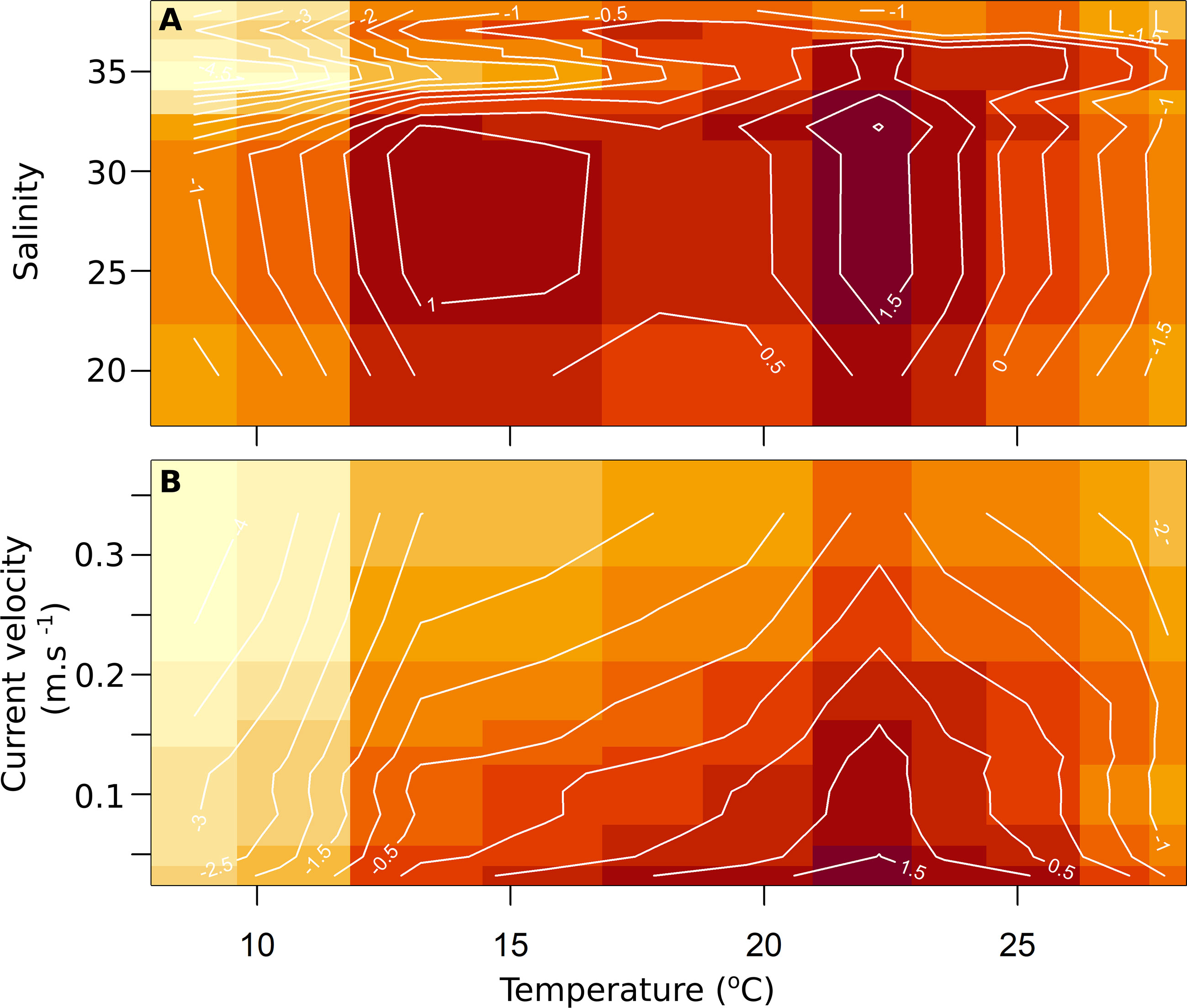
Figure 3 Two-dimensional partial dependence plots for the Atlantic blue crab Callinectes sapidus Rathbun, 1896 distribution model. The contour plots show the relationship between temperature and the other covariates in predicting the species distribution, namely salinity (A), and current velocity (B). The contour lines represent the posterior median of the draws.
3.2 Prediction maps, climate change scenarios, and uncertainty
The BART model for current conditions identified areas of importance for the Atlantic blue crab. The highest presence probabilities were found in the Gulf of Mexico, the southeast coast of the United States, and along the southeast Brazilian coast to the Rio de la Plata estuary (Uruguay and Argentina) in the native area, and in the Mediterranean Sea and off the Mauritanian coast in non-native areas. Sites with a medium to high presence probability were also identified in native and non-native habitats (Figure 4A).
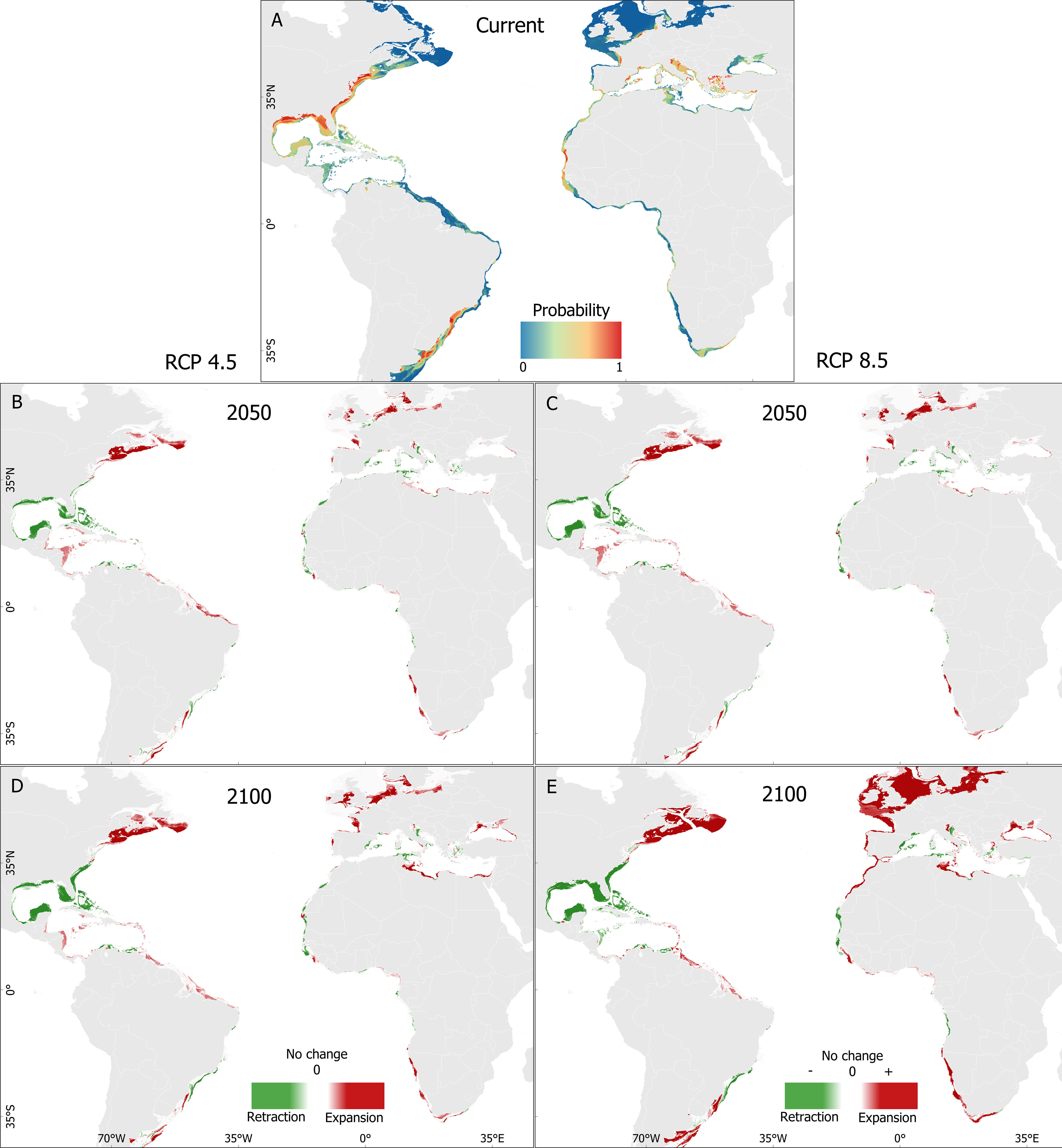
Figure 4 Predicted distribution of the Atlantic blue crab Callinectes sapidus Rathbun, 1896 (1832-2022) for posterior predictive mean for the BART model in the current scenario (A) and habitat range changes for the RCP 4.5 2050 (B), RCP 8.5 2050 (C), RCP 4.5 2100 (D) and RCP 8.5 2100 (E) scenarios.
For both climate change scenarios, the distribution shifts of the Atlantic blue crab will be less pronounced in native than in non-native areas. Overall, changes in habitat suitability (retraction or expansion) affect the probability presence under the extreme RCP 8.5 in a higher degree scenario than in the moderate RCP 4.5 scenario. Habitat changes in the 2090s were greater than in the 2040s as well. For both scenarios, the model predicted pronounced habitat retraction in the Gulf of Mexico and expansion from the Caribbean Sea to the northern Brazilian coast and eastern Canada (Figures 4B, C). In the non-native area, and particularly by the end of the century, extensive habitat expansion is predicted to occur from the coast of Mauritania to Morocco and from southern Portugal towards northern Atlantic regions, including the North and Baltic Seas, as well as a retraction in the western areas of the Mediterranean basin and expansion in the eastern Mediterranean basin, including the Baltic Sea (Figures 4D, E) (Supplementary Figure 7).
The spatial analysis, which considered the temperature effect as an indicator of thermal habitat suitability on the predicted probability of the Atlantic blue crab over time (current, the 2040s, and the 2090s), also disclosed that temperature plays a decisive role in the spread of the species along the south and north Atlantic Ocean, and Mediterranean Sea (Figures 5A–E). Additionally, thermal habitat suitability showed a similar pattern for the 2040s and 2090s in the native range, while in the non-native range, it increased drastically in the 2090s (Figures 4D, E).
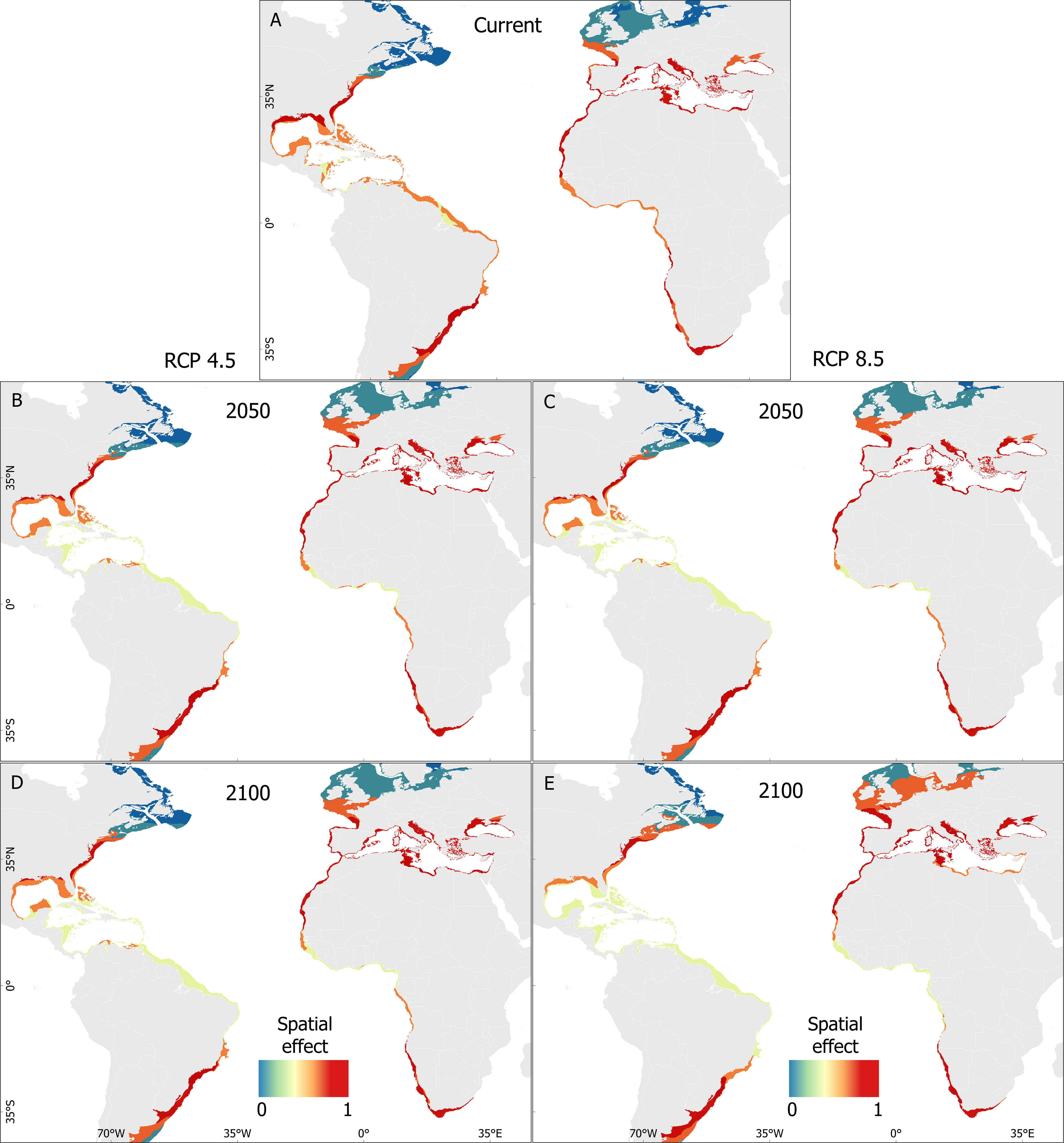
Figure 5 Partial spatial dependence plots for the Atlantic blue crab Callinectes sapidus Rathbun, 1896 showing the average contribution of temperature to the BART model predictions: current scenario (A), RCP 4.5 2050 (B), RCP 8.5 2050 (C), RCP 4.5 2100 (D), and RCP 8.5 2100 (E).
Model uncertainty also varied over time and between native and non-native habitats. Uncertainty was higher in the 2090s for both RCP 4.5 and 8.5 scenarios, and it was generally associated with deeper waters. In the native range, uncertainty predictions were higher in areas located in the Caribbean Sea (e.g., coasts of Honduras, Nicaragua, and Costa Rica), northern Brazil, and eastern Canada. In the non-native range, the highest uncertainties areas were mainly found in western Africa (Guinea-Bisau, Cameron), Morocco, and the Baltic Sea (Figure 6). The 2.5% and 97.5% predicted intervals of distribution probability used to estimate uncertainty across models and climate change scenarios are shown in Figure 6.
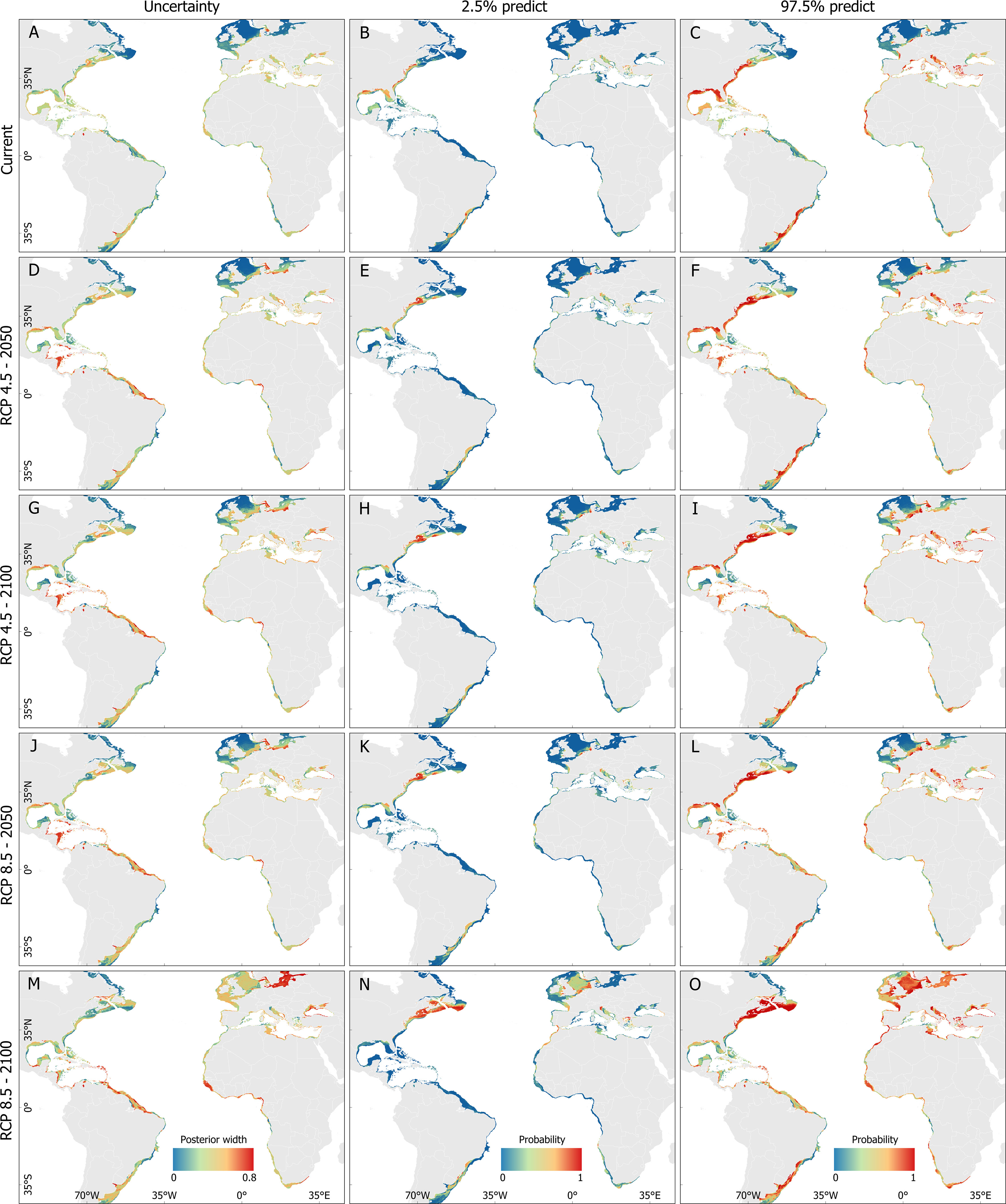
Figure 6 Prediction of the Atlantic blue crab Callinectes sapidus Rathbun, 1896 distribution between 1832 and 2022 (A–C) and under the influence of RCP 4.5 2050 (D–F), RCP 4.5 2100 (G–I), RCP 8.5 2050 (J–L), and RCP 8.5 2100 (M–O). The uncertainty areas are shown in the left column, the 2.5% quantile in the center column, and the 97.5% quantile in the right column.
4 Discussion
4.1 Advantages and limitations of BART models
This study shows that BARTs amplify our understanding of how environmental factors affect the occurrence and habitat preferences of the Atlantic blue crab in native and non-native areas. Equally important, BARTs projected the species distribution under two climate change scenarios, and showcases the applicability of a high-performance machine learning algorithm to study the distribution of an aquatic species.
Our results about the current and future distributions of the Atlantic blue crab were based on the assumption of changes in thermal boundaries. However, other factors affect species distribution, including biotic interactions, adaptative genetic drift, evolutionary changes, migration and dispersal, and anthropogenic stressors (e.g., fishing pressure, species introductions) (Hines et al., 2010; Gamliel et al., 2020; Pinsky et al., 2020; Seaborn et al., 2020; Encarnação et al., 2021a; Mancinelli et al., 2021). Therefore, as modeling and computing capabilities improve the inclusion of such data in future studies will improve prediction accuracy and reduce uncertainty. Yet, current results already provide timely information to managers to elaborate data-driven management policies.
We must also stress that we used environmental data representing the realized niche in the native range and that recently introduced non-indigenous species are often in non-equilibrium with the dynamics of the ecosystem. Invasive ecology theory reports numerous situations when non-indigenous species became invasive and then saw the population numbers collapse (Hui and Richardson, 2017). Therefore, projections about non-indigenous species distribution shifts must always be cautious because of a mosaic of introduction timelines along the non-native area (Hui, 2022). This is the case of the Atlantic blue crab, first reported in Europe in 1900 and with dissimilar invasion timelines between the North Sea, the eastern Atlantic coasts, and the Mediterranean Sea (Welk, 2004; Gallien et al., 2012; Pili et al., 2020; Freeman et al., 2022). Conscious of this limitation, we cannot guarantee that the niche-environment equilibrium assumption of SDM models have always been met.
We opted to analyze the distribution range shifts of the Atlantic blue crab at an ocean scale. At such scale, we are unable to capture the fine spatial resolution required to evaluate the expansion or retreat into and from estuarine ecosystems, and even freshwater ecosystems further inland, despite their importance for the species (Mangum and Amende, 1972; Taylor and Fehon, 2021; Scalici et al., 2022). Another factor precluding us from a finer resolution is the poor global database of environmental information covering inland ecosystems, despite the inclusion of data from several large estuarine ecosystems in the Bio-ORACLE database (e.g., Black Bay, Corpus Christi Bay, Chesapeake, Bay, Mississippi Sound estuary, and Lake Borgner in the US, Rio de La Plata in Uruguay and Argentina, Términos Lagoon in Mexico, and Maracaibo Lake in Venezuela) (Tyberghein et al., 2012; Assis et al., 2018). Therefore, the absence of environmental information on inland ecosystems in the raster layers does not invalidate the main results and conclusions of our study.
4.2 Model performance and effect of covariate
Overall, the BART model performed very well and the robust results illustrated the significance of climate change on the distribution shifts of an aquatic species. Both the AUC (0.92) and TSS (0.62) derived from the BART model achieved excellent performance, showing a high degree of discrimination between the sites where the Atlantic blue crab was present and those where it was absent. In addition, TSS values indicated the models’ high ability to correctly predict true negative (specificity) and true positive rates (sensitivity). The high performance of machine learning techniques has been reported for other tree-based models used to predict the distribution of other marine species (e.g., boosted regression trees, random forest) (Pittman and Brown, 2011; Mainali et al., 2015; Hernández-Ucera et al., 2021; Rahmanian et al., 2022).
The distribution of marine species can be influenced by several environmental predictors. However, only four to five variables can accurately predict the current and future distributions of marine species (Lezama-Ochoa et al., 2016; Zhang et al., 2019; Guerra et al., 2021; Hernández-Ucera et al., 2021). Temperature, salinity, current velocity, primary productivity, and seafloor parameters (e.g., slope, roughness, rugosity) are amongst the most informative predictors used in species distribution models in marine ecosystems (Bosch et al., 2018; Guerra et al., 2021; Pickens et al., 2021). Water temperature was the most informative environmental variable in controlling the potential distribution of the Atlantic blue crab, which aligns with the species’ temperature-dependent trait (Brylawski and Miller, 2006; Hines, 2007; Hines et al., 2010; Azra et al., 2020; Rogers et al., 2022). The life history traits of the Atlantic blue crab vary latitudinally because temperature strongly affects growth, molting, reproduction (e.g., maturation, fecundity, larval development time, frequency of spawning), and settlement. The drastic decrease in the species occurrence probability below 10°C and above 25°C in the BART model coincides with the species’ optimal water temperature range. Indeed, the Atlantic blue crab enters a period of dormancy in waters below 10°C (Brylawski and Miller, 2006; Hines et al., 2010), and their mean thermal preference for the Gulf of Mexico population ranges from 26 to 33°C with an optimum temperature at 24°C (García-Rueda et al., 2021; Marchessaux et al., 2022). Yet, we should always be aware that local adaptations reshape populations’ optimal temperature range.
Like many decapod species, the Atlantic blue crab requires various environmental conditions during its life cycle, which coincides with different habitat use strategies (Harris, 1979; Hines et al., 2010). For instance, the growth phase occurs in low-salinity estuarine waters, while ovigerous females migrate toward higher-salinity waters to release the eggs (Hines, 2003; Hines, 2007). The occurrence probability was closely related to temperature but also influenced by the other environmental predictors considered in this study. Thus, the variability in occurrence probabilities (between 25% and 80%) concerning salinity may be explained by the species’ migratory behavior during which they inhabit ecosystems with striking different water characteristics. Our analysis also shows that the highest occurrence probabilities were registered in calm water areas. Although the BART model did not indicate rugosity as an informative critical variable, these calm waters may be showing unconsolidated substrate. The Atlantic blue crab prefers nearshore aquatic submerged vegetation beds, also making use of unvegetated sandy and muddy bottoms for shelter, refuge from predators, and feeding grounds (Wilson et al., 1990; Hines et al., 2010).
4.3 Current distribution
The current prediction for the Atlantic blue crab distribution in the native range indicated three zones with the highest occurrence probabilities that match with areas with a high concentration of observations (Figure 1). These zones consist of diverse habitats, including bays, estuaries, coastal lagoons, tidal marshes, shallow inshore waters with soft bottoms, rocky bottoms, and mangrove wetlands (Miloslavich et al., 2011; Love et al., 2013; NMFS, 2015). The abundance in the Gulf of Mexico is considerably higher in salt marshes than in open waters (Minello et al., 2008), similar to observations on the Virginia coast, United States, where they are associated with seagrass meadows, salt marshes, channels, and oceanic inlets (Cheng et al., 2022). Therefore, vegetation zones likely enhance habitat suitability for the Atlantic blue crab because it provides refuge from predators and a bounty of food.
Our BART model also predicted high presence probabilities for true absence areas off the coasts of west France, Mauritania, Senegal, Gambia, and South Africa. The environmental conditions in these areas, with bays, salt marshes, mangroves, estuaries, and lagoons, seem suitable for the Atlantic blue crab despite the absence of records. These results should be evaluated with caution due to the lack of comprehensive studies in these regions while underscoring the high invasion probability of these areas under the current climate scenario.
Invasion success is often dictated by propagule pressure (Holle and Simberloff, 2005) to circumvent or minimize biological resistance (Brown and Barney, 2021). On the northwestern coast of Africa, propagule pressure is increasing from northern populations along the Atlantic coast of Morocco (Chaouti et al., 2022; Chairi and González-Ortegón, 2022). Our present-day habitat suitability predictions already identified the coast of Mauritania with a high presence probability, meaning that the species may colonize this area in the upcoming years. This prediction is of particular concern for the Banc d’Arguin National Park in Mauritania, a highly productive ecosystem, the largest marine protected area in western Africa, and a UNESCO World Heritage Site (Trégarot et al., 2020). At these latitudes, non-i Atlantic blue crab populations may compete with three native congeners Callinectes amnicola (de Rochebrune, 1883), Callinectes marginatus (A. Milne-Edwards, 1861), and Callinectes pallidus (Rochebrune, 1883) (Manning and Holthuis, 1981).
4.4 Future distribution and climate change
The shifts in aquatic species distribution due to climate change are multidirectional, either towards the poles or the equator or between shallower and deeper waters (Dulvy et al., 2008; Hastings et al., 2020). The Atlantic blue crab distribution is limited by cold water at higher latitudes (Molina et al., 2021), yet climate change will expand its distribution polewards to escape warmer waters at lower latitudes (Azra et al., 2020; Hastings et al., 2020). Our data shows that the temperature-driven expansion/contraction of the species distribution will vary between native and non-native areas as a function of climate change scenarios.
The asymmetric nature of the future distribution on both sides of the Atlantic Ocean owes to the populations’ intrinsic status, native or non-native. In native habitats – especially in the Gulf of Mexico, the Caribbean Sea, and off South America – and under the RCP 4.5 and 8.5 scenarios, our models predicted an expansion towards the equator (e.g., Caribbean Sea, and from the coasts of Trinidad and Tobago to the North of Brazil. The population retraction predicted in the Gulf of Mexico may be associated with water temperatures above 30°C, disrupting the species’ metabolism when it reaches the upper thermal tolerance limits (Marchessaux et al., 2022). The poleward expansion will be more significant later in the century and particularly in eastern Canada, as supported by recent observations in the Gulf of Maine (Johnson, 2015). In non-native ecosystems, the most significant distribution shifts are expected to occur in the 2090s under the RCP 8.5 scenario. The species will be widespread along most of the Mediterranean Sea, between Mauritania and the Bay of Biscay, the North Sea, and even reaching the Baltic Sea. Indeed, there have been sporadic records between the Bay of Biscay and the North Sea (Cabal et al., 2006; Nehring, 2011; Mancinelli et al., 2021) and some already identified established populations off Belgium and the Netherlands since the 1990s (Nehring, 2011). The coastal areas in the North Sea and Baltic Sea are geographically attractive with their numerous estuaries and salt marsh areas, which may favor a steep increase in abundance when coupled with more amenable sea temperatures (Dijkema, 1990).
The environmental selection for traits that enhance reproduction and survival under climate change scenarios may benefit species’ range expansion (Finch et al., 2021). For the Atlantic blue crab, we expect that two mechanistic phenomena will act in tandem to favor the poleward-expansion along the eastern Canadian waters and northern European seas by the end of the 21st century. First, the poleward-increased water temperature is conducive to a fundamental niche expansion. Second, decreasing water temperature-induced mortality during winter allows consolidation of the expansion obtained during warmer months (Molina et al., 2021). Additionally, its plasticity to adjust the reproductive strategy and behavior according to temperature changes allows the Atlantic blue crab to tolerate, adapt, or move to deal with environmental shifts in native and non-native habitats.
The expansion of another invasive species to northern European estuaries will disturb ecosystems that already endure significant anthropogenic impacts (e.g., excessive nutrient load, habitat degradation, river flow regularization, urbanization) (Damme et al., 2005; Nehring, 2006; Courrat et al., 2009; Dauvin et al., 2009). The range expansion of the Atlantic blue crab in the native area will set new ecological dynamics often equivalent to a biological invasion process (Tulis et al., 2023).
Overall, environmental and fishery managers should be proactively vigilant because the expansion of the Atlantic blue crab in native and non-native areas will likely shift ecological dynamics and increase ecological stress. Managers from the native area will undoubtedly obtain precious insights from lessons learned in the non-native area. Finally, managers should promote or sponsor citizen science campaigns, not only because of their significant role in reporting the distribution of non-indigenous species and tracking their expansion (Encarnação et al., 2021b) but also because they generate data with great value to improve model validation and applicability (Matutini et al., 2021).
5 Conclusions
This study anticipates that climate change will lead the Atlantic blue crab to expand their distribution in native and non-native areas. An asymmetric range expansion will occur on both sides of the Atlantic Ocean, with temperature playing a decisive role in setting such asymmetry. In native habitats and considering the RCP 4.5 and 8.5 scenarios, we predict an expansion toward the equator and deeper areas during the 2040s. A poleward range expansion will be particularly noticeable by the end of the 21st century off eastern Canada. In non-native ecosystems and considering the RCP 8.5 scenario, the most significant distribution shifts will occur in the 2090s when the species become common even in the North Sea. The range expansion of native species is often equivalent to the dynamics and impacts of invasive species, so lessons learned by ecologists and environmental managers about the invasive Atlantic blue crab will provide precious insights to colleagues in the native areas.
Data availability statement
The datasets presented in this study can be found in online repositories. The names of the repository/repositories and accession number(s) can be found below: All occurrence data of the Atlantic blue crab Callinectes sapidus used in the study are available for download from the Global Biodiversity Information System (GBIF- www.gbif.org/), iNaturalist (https://www.inaturalist.org/). Occurrences from 1832 to 2020 can be downloaded from the link: https://www.nature.com/articles/s41597-021-00888-w#additional-information. Additional records made between 2019 and March 2022 are available at https://zenodo.org/record/7421549#.Y6mYdnbx4mI. GIS rasters providing environmental data for current and future scenarios are available at Bio-ORACLE website (https://www.bio-oracle.org/).
Author contributions
EC: conceptualization, methodology, data curation, performed the analyses, writing - original draft, writing - review & editing. JE: investigation, data curation, methodology, writing - review & editing. MT: writing - review & editing. PM: conceptualization, writing - review & editing. All authors contributed to the article and approved the submitted version.
Funding
This research received no specific funding agency in the public, commercial, or not-for-profit sectors. However, the NEMA is funded and supported by the Portuguese national funds from Foundation for Science and Technology (FCT) (Projects: UIDB/04326/2020, UIDP/04326/2020 and LA/P/0101/2020), ATLAZUL (Poctep/Interreg 0755/ATLAZUL/6/E) and ALFCORAZUL projects (Fundo Ambiental, candidature n° 48). JE has a Ph.D. scholarship (SFRH/BD/140556/2018) funded by Foundation for Science and Technology (FCT, Portugal).
Acknowledgments
The authors express their gratitude to the referees for their thoughtful comments and effort toward improving our article.
Conflict of interest
The authors declare that the research was conducted in the absence of any commercial or financial relationships that could be construed as a potential conflict of interest.
Publisher’s note
All claims expressed in this article are solely those of the authors and do not necessarily represent those of their affiliated organizations, or those of the publisher, the editors and the reviewers. Any product that may be evaluated in this article, or claim that may be made by its manufacturer, is not guaranteed or endorsed by the publisher.
Supplementary material
The Supplementary Material for this article can be found online at: https://www.frontiersin.org/articles/10.3389/fmars.2023.1158206/full#supplementary-material
References
Aguilar R., Johnson E., Hines A. H., Kramer M. A., Goodison M. R. (2008). Importance of blue crab life history for stock enhancement and spatial management of the fishery in Chesapeake bay. Rev. Fish. Sci. 13, 117–124. doi: 10.1080/10641260701681599
Allouche O., Tsoar A., Kadmon R. (2006). Assessing the accuracy of species distribution models: prevalence, kappa and the true skill statistic (TSS). J. Appl. Ecol. 43, 1223–1232. doi: 10.1111/j.1365-2664.2006.01214.x
Assis J., Tyberghein L., Bosch S., Verbruggen H., Serrão E. A., Clerck O. (2018). Bio-oricle v2.0: extending marine data layers for bioclimatic modelling. Glob. Ecol. Biogeogr. 27, 277–284. doi: 10.1111/geb.12693
Azra M. N., Amr-Aaqillah-Amr M. A., Ikhwanuddin M., Ma. H., Waiho K., Ostrensky A., et al. (2020). Effects of climate-induced water temperature changes on the life history of brachyuran crabs. Rev. Aquac. 12, 1211–1216. doi: 10.1111/raq.12380
Azzurro E., Moschella P., Maynou F. (2011). Tracking signals of change in Mediterranean fish diversity based on local ecological knowledge. PloS One 6 (9), e24885. doi: 10.1371/journal.pone.0024885
Babet-Massin M., Jiguet F., Albert C. H., Thuiller W. (2012). Selecting pseudo-absences distribution models: how, where and how many? Methods Ecol. Evol. 3, 327–338. doi: 10.1111/j.2041-210X.2011.00172.x
Barbet-Massin M., Rome Q., Villemant C., Courchamp F. (2018). Can species distribution models really predict the expansion of invasive species? PloS One 13 (3), e0193085. doi: 10.1371/journal.pone.0193085
Barbour A. B., Allen M. S., Frazer T. K., Sherman K. D. (2011). Evaluating the potential efficacy of invasive lionfish (Pterois volitans) removals. PloS One 6, e19666. doi: 10.1371/journal.pone.0019666
Baylis A. M. M., Orben R. A., Arnould J. P. Y., Christiansen F., Hays G. C., Staniland I. J. (2015). Disentangling the cause of a catastrophic population decline in a large marine mammal. Ecology 96, 2834–2847. doi: 10.1890/14-1948.1
Bio-ORACLE (2022). Available at: https://www.bio-oracle.org/downloads-to-email.php.
Blois J. L., Zarnetske P. L., Fitzpatrick M. C., Finnegan S. (2013). Climate change and the past, present, and future of biotic interactions. Science 341 (6145), 499–504. doi: 10.1126/science.12371
Boria R. A., Olson L. E., Goodman S. M., Anderson R. P. (2014). Spatial filtering to reduce sampling bias can improve the performance of ecological niche models. Ecol. Modell. 275, 73–77. doi: 10.1016/j.ecolmodel.2013.12.012
Bosch S., Tyberghein L., Deneudt K., Hernandez F., Clerk O. (2018). In search of relevant predictors for marine species distribution modelling using the MarineSPEED benchmark dataset. Divers. Distrib. 24, 144–157. doi: 10.1111/ddi.12668
Brown B. L., Barney J. N. (2021). Rethinking biological invasions as a metacommunity problem. Front. Ecol. Evol. 8. doi: 10.3389/fevo.2020.584701
Brylawski B. J., Miller T. J. (2006). Temperature-dependent growth of the blue crab (Callinectes sapidus): a molt process approach. Can. J. Fish. Aquat. 63, 1298–1308. doi: 10.1139/f06-011
Cabal J., Millán J. A. P., Arronte J. C. (2006). A new record of callinectes sapidus rathbu (Crustacea: Decapoda: Brachyura) from the cantabrian Sea, bay of Biscay, Spain. Aquat. Invasions 1, 186–187. doi: 10.3391/ai.2006.1.3.14
Cantin N. E., Cohen A. L., Karnauskas K. B., Tarrant A. M., McCorkle D. C. (2010). Ocean warming slows coral growth in the central red Sea. Science 329, 322–325. doi: 10.1126/science.1190182
Carlson C. J. (2020). Embarcadero: species distribution modelling with Bayesian additive regression trees in r. Methods Ecol. Evol. 11, 850–858. doi: 10.1111/2041-210X.13389
Carlson C. J., Bevins S. N., Schimid B. V. (2022). Plague risk in the western united states oven seven decades of environmental change. Glob. Change Biol. 28, 753–769. doi: 10.1111/gcb.15966
Cerveira I., Baptista V., Teodósio M. A., Morais P. (2021). What’s for dinner? assessing the value of an edible invasive species and outreach actions to promote its consumption. Biol. Invasions. 24, 815–829. doi: 10.1007/s10530-021-02685-3
Chairi H., González-Ortegón E. (2022). Additional records of the blue crab Callinectes sapidus rathbun 1896 in the Moroccan Sea, Africa. Bioinvasions Rec. 11, 776–784. doi: 10.3391/bir.2022.11.3.19
Chaouti A., Belattmania Z., Nadri A., Serrão E. A., Encarnação J., Sabour B. (2022). The invasive Atlantic blue crab Callinectes sapidus rathbun 1896 expands its distributional range southward to Atlantic African shores: first records along the Atlantic coast of Morocco. Bioinvasions Rec. 11, 227–237. doi: 10.3391/bir.2022.11.1.23
Charbonnel A., Laffaille P., Biffi M., Blanc F., Maire A., Nemoz M., et al. (2016). Can recent global changes explain the dramatic range contraction of an endangered semi-aquatic mammal species in the French pyrenees? PloS One 11 (7), e0159941. doi: 10.1371/journal.pone.0159941
Cheng S. L., Tedford K. N., Smith R. S., Hardison S., Cornish M. R., Castorani M. C. N. (2022). Coastal vegetation and bathymetry influence blue crab abundance across spatial scales. Estuaries Coast 45 (6), 1701–1715. doi: 10.1007/s12237-021-01039-5
Chipman H. A., George E. I., McCulloch R. E. (2010). BART: Bayesian regression trees. Ann. Appl. Stat. 4, 266–298. doi: 10.1214/09-AOAS285
Clavero M., Franch N., Bernardo-Madrid R., López V., Abelló P., Mancinelli G. (2022). Severe, rapid and widespread impacts of an Atlantic blue crab invasion. Mar. pollut. Bull. 176, 113479. doi: 10.1016/j.marpolbul.2022.113479
Corrales X., Coll M., Ofir E., Heymans J. J., Steenbeek J., Goren M., et al. (2018). Future scenarios of marine resources and ecosystem conditions in the Eastern Mediterranean under the impacts of fishing, alien species and sea warming. Sci. Rep. 8, 1–16. doi: 10.1038/s41598-018-32666-x
Courrat A., Lobry J., Nicolas D., Laffargue P., Amara R., Le Pape O. (2009). Anthropogenic disturbance on nursery function of estuarine areas for marine species. Estuar. Coast. Shelf Sci. 81, 179–190. doi: 10.1016/j.ecss.2008.10.017
Damme S. V., Struyf E., Maris T., Ysebaert T., Dehairs F., Meire P. (2005). Spatial and temporal patterns of water quality along the estuarine salinity gradient of the scheldt estuary (Belgium and the netherlands): results of an integrated monitoring approach. Hydrobiologia 540, 29–45. doi: 10.1007/s10750-004-7102-2
Dauvin J. C., Tous Rius A., Ruellet T. (2009). Recent expansion of two invasive crabs species Hemigrapsus sanguineus (de haan 1835) and H. takanoi asakura and Watanabe 2005 along the opal coast, France. Aquat. Invasions 4, 451–465. doi: 10.3391/ai.2009.4.3.3
DeRivera C. E., Ruiz G. M., Hines A. H., Jivoff P. (2005). Biotic resistance to invasion: native predator limits abundance and distribution of an introduced crab. Ecology 86, 3364–3376. doi: 10.1890/05-0479
Dijkema K. S. (1990). Salt and brackish marshes around the Baltic Sea and adjacent parts of the north Sea: their vegetation and management. Biol. Conserv. 51, 191–209. doi: 10.1016/0006-3207(90)90151-E
Dorie V. (2022). Dbarts: discrete Bayesian additive regression trees sampler, R package version 0.9-22.
Dulvy N. K., Rogers S. I., Jennings S., Stelzenmüller V., Dye S. R., Skjoldal H. R. (2008). Climate change and deepening of the north Sea fish assemblage: a biotic indicator of warming seas. J. Appl. Ecol. 45, 1029–1039. doi: 10.1111/j.1365-2664.2008.01488.x
Dunn D. C., Halpin P. N. (2009). Rugosity-based regional modeling of hard-bottom habitat. Mar. Ecol. Prog. Ser. 377, 1–11. doi: 10.3354/meps07839
Elliott W., Simmonds M. (2007). Whales in hot water? the impact of a changing climate on whales, dolphins and porpoises: a call for action (Chippenham, UK: WWF-International, Gland Switz./WDCS).
Encarnação J., Baptista V., Teodósio M. A., Morais P. (2021a). Low-cost citizen science effectively monitors the rapid expansion of marine invasive species. Front. Environ. Sci. 9. doi: 10.3389/fenvs.2021.752705
Encarnação J., Costa E. F. S., Teodósio M. A., Morais P. (2023). New records of the Atlantic blue crab Callinectes sapidus rathbun 1896 in European waters (Zenodo). doi: 10.5281/zenodo.7421549
Encarnação J., Krug L. N., Teodósio M. A., Morais P. (2022). Coastal countercurrents increase propagule pressure of an aquatic invasive species to an area where previous introductions failed. Estuaries Coast 45, 2504–2518. doi: 10.1007/s12237-022-01092-8
Encarnação J., Leitão T., Morais P., Pilo D., Range P., Chicharo A. (2013). Effects of inter-annual freshwater inflow shifts on the community structure of estuarine decapods. Cah. Biol. Mar. 54, 181–189. doi: 10.21411/CBM.A.782BAAB8
Encarnação J., Morais P., Baptista V., Cruz J., Teodósio M. A. (2019). New evidence of marine fauna tropicalization off the southwestern Iberian peninsula (Southwest Europe). Diversity 11 (4), 48. doi: 10.3390/d11040048
Encarnação J., Teodósio M. A., Morais P. (2021b). Citizen science and biological invasions: a review. Front. Environ. Sci. 8. doi: 10.3389/fenvs.2020.602980
Engler R., Randin C. F., Thuiller W., Dullinger S., Zimmermann N. E., Araújo M. B., et al. (2011). 21st century climate change threatens mountain flora unequally across Europe. Glob. Change Biol. 17 (7), 2330–2341. doi: 10.1111/j.1365-2486.2010.02393.x
Finch D. M., Butler J. L., Runyon J. B., Fettig C. J., Kilkenny F. F., Jose S., et al. (2021). “Effects of climate change on invasive species,” in Invasive species in forests and rangelands of the united states. Eds. Poland T. M., Patel-Weynand T., Finch D. M., Miniat C. F., Hayes D. C., Lopez V. M. (Cham: Springer). doi: 10.1007/978-3-030-45367-1_4
Fonseca V. P., Pennino M. G., Nóbrega M. F., Oliveira J. E. L., Mendes L. F. (2017). Identifying fish diversity hot-spots in data-poor situations. Mar. Environ. Res. 129, 365–373. doi: 10.1016/j.marenvres.2017.06.017
Franco J. N., Tuya F., Bertocci I., Rodríguez L., Martínez B., Arenas F. (2018). The ‘golden kelp Laminaria ochroleuca under global change: integrating multiple eco-physiological responses with species distribution models. J. Ecol. 106 (1), 47–58. doi: 10.1111/1365-2745.12810
Franklin J. (2013). Species distribution models in conservation biogeography: developments and challenges. Divers. Distrib. 19, 1217–1223. doi: 10.1111/ddi.12125
Fuentes M. A., Torrent L., Barrera S., Boix D. (2019). Rapid invasion of the American blue crab Callinectes sapidus Rathbun, 1896 in the North-East of the Iberian Peninsula. Bioinvasions Rec. 8 (1), 113–118. doi: 10.3391/bir.2019.8.1.12
Freeman M. S., Dick J. T., Reid N. (2022). Dealing with non-equilibrium bias and survey effort in presence-only invasive species distribution models (iSDM); predicting the range of muntjac deer in Britain and Ireland. Ecol. Inform. 69, 101683. doi: 10.1016/j.ecoinf.2022.101683
Galappaththi E. K., Susarla V. B., Loutet S. J., Ichien S. T., Hyman A. A., Ford J. D. (2022). Climate change adaptation in fisheries. Fish Fish. 23 (1), 4–21. doi: 10.1111/faf.12595
Gallien L., Douzet R., Pratte S., Zimmermann N. E., Thuiller W. (2012). Invasive species distribution models–how violating the equilibrium assumption can create new insights. Glob. Ecol. Biogeogr. 21 (11), 1126–1136. doi: 10.1111/j.1466-8238.2012.00768.x
Gamliel I., Buba Y., Guy-Haim T., Garval T., Willette D., Belmaker J. (2020). Incorporating physiology into species distribution models moderates the projected impact of warming on selected Mediterranean marine species. Ecography 43, 1090–1106. doi: 10.1111/ecog.04423
García-Rueda A., Tremblay N., Mascaró M., Díaz F., Paschke K., Rosas C. (2021). The thermal tolerance of a tropical population of blue crab (Callinectes sapidus) modulates aerobic metabolism during hypoxia. J. Therm. Biol. 102, 103078. doi: 10.1016/j.jtherbio.2021.103078
González-Ortegón E., Berger S., Encarnação J., Chairi H., Morais P., Teodósio M. A., et al. (2022). Free pass through the pillars of Hercules? genetic and historical insights into the recent expansion of the Atlantic blue crab Callinectes sapidus to the West and the East of the strait of Gibraltar. Front. Mar. Sci. 9. doi: 10.3389/fmars.2022.918026
Guerra T. G., Santos J. M. F. F., Pennino M. G., Lopes P. F. M. (2021). Damage or benefit? how future scenarios of climate change may affect the distribution of small pelagic fishes in the coastal seas of the americas. Fish. Res. 234, 105815. doi: 10.1016/j.fishres.2020.105815
Halpern B. S., Frazier M., Potapenko J., Casey K. S., Koenig K., Longo K., et al. (2015). Spatial and temporal changes in cumulative human impacts on the world’s ocean. Nat. Commun. 6 (1), 1–7. doi: 10.1038/ncomms8615
Harris R. E. (1979). Life history, ecology and stock assessment of the blue crab Callinectes sapidus of the united states Atlantic coast- a review. Proc. Blue Crab Colloquium, 59–69.
Hastings R. A., Rutterford L. A., Freer J. J., Collins R. A., Simpson S. D., Genner M. J. (2020). Climate change drives poleward increases and equatorward declines in marine species. Curr. Biol. 30, 1572–1577. doi: 10.1016/j.cub.2020.02.043
Hernández-Ucera J., Murillo F. J., Regueira M., Cabanellas-Reboredo M., Planas M. (2021). Preferential habitats prediction in sygnathids using species distribution models. Mar. Environ. Res. 172, 105488. doi: 10.1016/j.marenvres.2021.105488
Hill J., Fowler D. L., van den Avyle M. V. (1989). Species profiles: life histories and environmental requirements of coastal fishes and invertebrates (Mid-Atlantic) - blue crab (Vicksburg: U.S. Army Corps of Engineers). doi: 10.21236/ADA226928
Hines A. H. (2003). Ecology of juvenile and adult blue crabs: summary of discussion of research themes and directions. Bull. Mar. Sci. 72 (2), 423–433.
Hines A. H. (2007). “Ecology of juvenile and adult blue crabs,” in Biology of the blue crab. Eds. Kennedy V. S., Cronin L. E. (Maryland: Maryland Sea Grant College Program, College Park, MD), 565–654.
Hines A. H., Johnson E. G., Darnell M. Z., Rittschof D., Miller T. J., Bauer L. J., et al. (2010). “Predicting effects of climate change on blue crabs in Chesapeake bay,” in Biology and management of exploited crab populations under climate change. Eds. Kruse G. H., Eckert G. L., Foy R. J., Lipcius R. N., Sainte-Marie B., Woodby D. (Fairbanks: Alaska Sea Grant, University of Alaska Fairbanks). doi: 10.4027/bmecpcc.2010.22
Holle B. V., Simberloff D. (2005). Ecological resistance to biological invasion overwhelmed by propagule pressure. Ecology 86, 3212–3218. doi: 10.1890/05-0427
Hosmer D. W., Lemeshow S. (2000). Assessing the fit of the model. chapter 5. in: applied logistic regression. 2nd Ed (New York, NY: John Wiley and Sons), Pp. 143–202. doi: 10.1002/0471722146
Hui C. (2022). The dos and don´ts for predicting invasion dynamics with species distribution models. Biol. Invasions 25, 947–953. doi: 10.1007/s10530-022-02976-3
Jiménez-Valverde A., Peterson A. T., Soberón J., Overton J. M., Aragón P., Lobo J. M. (2011). Use of niche models invasive species risk assessments. Biol. Invasions 13, 2785–2797. doi: 10.1007/s10530-011-9963-4
Johnson S. S. (2015). The savory swimmer swims north: a northerm range extension of the blue crab Callinectes sapidus. J. Crustac. Biol. 35, 105–110. doi: 10.1163/1937240X-00002293
King N. G., Wilmes S. B., Smyth D., Tinker J., Robins P. E., Malham S. K. (2021). Climate change accelerates range expansion of the non-native species, the pacific oyster, Crassostrea gigas. ICES J. Mar. Sci. 78, 70–81. doi: 10.1093/icesjms/fsaa189
Lezama-Ochoa N., Murua H., Chust G., Van Loon E., Ruiz J., Hall M., et al. (2016). Present and future potential habitat distribution of Carcharhinus falciformis and Canthidermis maculata by-catch species in the tropical tuna purse-seine fishery under climate change. Front. Mar. Sci. 3. doi: 10.3389/fmars.2016.00034
Liu C., Wolter C., Xian W. (2020). And jeschke, J Most invasive species largely conserve their climatic niche. PNAS 117 (38), 23643–23651. doi: 10.1073/pnas.200428911
Love M. S., Baldera A., Yeung C., Robbins C. (2013). The gulf of Mexico ecosystem: a coastal and marine atlas (New Orleans, LA: Ocean Conservancy).
Lyons D. A., Lowen J. B., Therriault T. W., Brickman D., Guo L., Moore A. M., et al. (2020). Identifying marine invasion hotspots using stacked species distribution models. Biol. Invasions 22, 3403–3423. doi: 10.1007/s10530-020-02332-3
MacDonald J. A., Roudez R., Glover T., Weis J. S. (2007). The invasive green crab and Japanese shore crab: behavioral interactions with native crab species, the blue crab. Biol. Invasions 9, 837–848. doi: 10.1007/s10530-006-9085-6
Manning R. B., Holthuis L. B. (1981). West African brachyuran crabs (Crustacea: Decapoda). doi: 10.1016/0198-0254(81)91632-0
Mainali K. P., Warren D. L., Dhileepan K., Mcconnachie A., Strathie L., Hassan G., et al. (2015). Projecting future expansion of invasive species: comparing and improving methodologies for species distribution modeling. Glob. Change Biol. 21, 4461–4480. doi: 10.1111/gcb.13038
Mancinelli G., Bardelli R., Zenetos A. (2021). A global occurrence database of the Atlantic blue crab Callinectes sapidus. Sci. Data 8, 111. doi: 10.1038/s41597-021-00888-w
Mancinelli G., Chainho P., Cilenti L., Falco S., Kapiris K., Ribeiro F. (2017). On the Atlantic blue crab (Callinectes sapidus rathbun 1896) in southern European coastal waters: time to turn a threat into a resource? Fish. Res. 194, 1–8. doi: 10.1016/j.fishres.2017.05.002
Mangum C. P., Amende L. M. (1972). Blood osmotic concentration of blue crabs (Callinectes sapidus rathbun) found in fresh water. Chesapeake Sci. 13, 318–320. doi: 10.2307/1351114
Marchessaux G., Bosch-Belmar M., Cilenti L., Lago N., Mangano M. C., Marsiglia N., et al. (2022). The invasive blue crab Callinectes sapidus thermal response: predicting metabolic suitability maps under future warming Mediterranean scenarios. Front. Mar. Sci. 9. doi: 10.3389/fmars.2022.1055404
Matutini F., Baudry J., Pain G., Sineau M., Pithon J. (2021). On the use of abiotic surrogates to describe marine benthic biodiversity. Ecol. Evol. 11, 3028–3039. doi: 10.1002/ece3.7210
McArthur M. A., Brooke B. P., Przeslawski R., Ryan D. A., Lucieer V. L., Nichol S., et al. (2010). On the use of abiotic surrogates to describe marine benthic biodiversity. Estuar. Coast. Shelf Sci. 88, 21–32. doi: 10.1016/j.ecss.2010.03.003
McLaughlin J. F., Hellmann J. J., Boggs C. L., Ehrlich P. R. (2002). Climate change hastens population extinctions. PNAS 99, 6070–6074. doi: 10.1073/pnas.052131199
Miloslavich P., Klein E., Díaz J. M., Hernández C. E., Bigatti G., Campos L., et al. (2011). Marine biodiversity in the Atlantic and pacific coasts of south America: knowledge and gaps. PloS One 6, e14631. doi: 10.1371/journal.pone.0014631
Minello T. J., Matthews G. A., Caldwell P. A., Rozas L. P. (2008). Population and production estimates for decapod crustaceans in wetlands of Galveston bay, Texas. Trans. Am. Fish. Soc 137, 129–146. doi: 10.1577/T06-276.1
Molina A. I., Cerrato R. M., Nye J. A. (2021). Population level differences in overwintering survivorship of blue crabs (Callinectes sapidus): a caution on extrapolating climate sensitivities along latitudinal gradients. PloS One 16, e0257569. doi: 10.1371/journal.pone.0257569
Molinos J. G. (2020). Global marine warming in a new dimension. Nat. Ecol. Evol. 4, 16–17. doi: 10.1038/s41559-019-1037-5
Molinos J. G., Halpern B. S., Schoeman D. S., Brown C. J., Kiessling W., Moore P. J., et al. (2015). Climate velocity and the future global redistribution of marine biodiversity. Nat. Clim. Change 6, 83–88. doi: 10.1038/nclimate2769
Morais P., Gaspar M., Garel E., Baptista V., Cruz J., Cerveira I., et al. (2019). The Atlantic blue crab Callinectes sapidus Rathbun, 1896 expands its non-native distribution into the Ria Formosa lagoon and the Guadiana estuary (SW-Iberian Peninsula, Europe). Bioinvasions Rec. 8 (1), 123–133. doi: 10.3391/bir.2019.8.1.14
Morris J. A., Thomas A., Rhyne A. L., Breen N., Akins L., Nash B. (2011). Nutritional properties of the invasive lionfish: a delicious and nutritious approach for controlling the invasion. Aquac. Aquar. Conserv. Legis. 5, 99–102.
Naimi B., Hamm N. A. S., Groen T. A., Skidmore A. K., Toxopeus A. G. (2014). Where is positional uncertainty a problem for species distribution modelling? Ecography 37 (2), 191–203. doi: 10.1111/j.1600-0587.2013.00205.x
Nehring S. (2006). Four arguments why so many alien species settle into estuaries, with special reference to the German river Elbe. Helgol. Mar. Res. 60 (2), 127–134. doi: 10.1007/s10152-006-0031-x
Nehring S. (2011). “Invasion history and success of the American blue crab Callinectes sapidus in European and adjacent waters,” in In the wrong place-alien marine crustaceans: distribution, biology and impacts (Dordrecht: Springer), (pp. 607–624). doi: 10.1007/978-94-007-0591-3_21
NMFS. (2015). Our living oceans: habitat. status of the habitat of U.S. living marine resources (U.S. Dep. Commer., NOAA Tech. Memo. NMFS-F/SPO-75).
Oliveira R. F., Machado J. L., Jordão J. M., Burford F. L., Latruffe C., McGregor P. K. (2000). “Human exploitation of male fiddler crab claws: behavioural consequences and implications for conservation,”, Animal Conservation (Animal Conservation forum: Cambridge University Press) vol. 3, 1–5). doi: 10.1111/j.1469-1795.2000.tb00081.x
Osland M. J., Stevens P. W., Lamont M. M., Brusca R. C., Hart K. M., Seminoff J. A. (2021). Tropicalization of temperate ecosystems in north America: the northward range expansion of tropical organisms in response to warming winter temperatures. Glob. Change Biol. 27 (13), 3009–3034. doi: 10.1111/gcb.15563
Panetta A. M., Stanton M. L., Harte J. (2018). Climate warming drives local extinction: evidence from observation and experimentation. Sci. Adv. 355, eaai9214. doi: 10.1126/sciadv.aaq1819
Paukert C., Olden J. D., Lynch A. J., Breshears D. D., Chambers C. R., Chu C., et al. (2021). Climate change effects on north American fish and fisheries to inform adaptation strategies. Fisheries 46 (9), 449–464. doi: 10.1002/fsh.10668
Pecl G. T., Araújo M. B., Bell J. D., Blanchard J., Bonebrake T. C., Chen I., et al. (2016). Biodiversity redistribution under climate change: impacts on ecosystems and human well-being. Science 4, eaaq1819. doi: 10.1126/science.aai92
Pershing A. J., Alexander M. A., Hernandez C. M., Kerr L. A., Le Bris A., Mills K. E., et al. (2015). Slow adaptation in the face of rapid warming leads to collapse of the gulf of Maine cod fishery. Science 350 (6262), 809–812. doi: 10.1126/science.aac9819
Pickens B. A., Carroll R., Schirripa M. J., Forrestal F., Friedland K. D., Taylor J. C. (2021). A systematic review of spatial habitat associations and modeling of marine fish distribution: a guide to predictors, methods, and knowledge gaps. PloS One 16 (5), e0251818. doi: 10.1371/journal.pone.0251818
Pili A. N., Tingley R., Sy E. Y., Diesmos M. L. L., Diesmos A. C. (2020). Niche shifts and environmental non-equilibrium undermine the usefulness of ecological niche models for invasion risk assessments. Sci. Rep. 10 (1), 1–18. doi: 10.1038/s41598-020-64568-2
Pinsky M. L., Selden R. L., Kitchel Z. J. (2020). Climate-driven shifts in marine species ranges: scaling from organisms to communities. Ann. Rev. Mar. Sci. 12, 153–179. doi: 10.1146/annurev-marine-010419-010916
Pittman S., Brown K. A. (2011). Multi-scale approach for predicting fish species distributions across coral reef seascapes. PloS One 6, e20583. doi: 10.1371/journal.pone.0020583
Poloczanska E. S., Brown C. J., Sydeman W. J., Kiessling W., Schoeman D. S., Moore P. J., et al. (2013). Global imprint of climate change on marine life. Nat. Clim. Change 3, 919–925. doi: 10.1038/nclimate1958
Purtlebaugh C. H., Martin C. W., Allen M. S. (2020). Poleward expansion of common snook Centropomus undecimalis in the northeastern gulf of Mexico and future research needs. PloS One 15 (6), e0234083. doi: 10.1371/journal.pone.0234083
Rahmanian S., Pouyan S., Karami S., Pourghasemi H. R. (2022). “Predictive habitat suitability models for Teucrium polium l. using boosted regression trees. chapter 17,” in Pourghasemi, H.R. (Ed.). computers in earth environmental sciences (Elsevier), 245–254. doi: 10.1016/B978-0-323-89861-4.00029-4
R Core Team (2021). R: a language and environment for statistical computing (Vienna, Austria: R Foundation for Statistical Computing).
Riley S. J., DeGloria S. D., Elliot R. (1999). A terrain ruggedness index that quantifies topographic heterogeneity. Intermt. J. Sci. 5, 1–4.
Robinson N. M., Nelson W. A., Costello M. J., Sutherland J. E., Lundquist C. J. (2017). A systematic review of marine-based species distribution models (SDMs) with recommendations for best practice. Front. Mar. Sci. 4. doi: 10.3389/fmars.2017.00421
Rodríguez A., Drake P., Arias A. M. (1997). Reproductive periods and larval abundance patterns of the crabs Panopeus africanus and Uca tangeri in a shallow inlet (SW Spain). Mar. Ecol. Prog. Ser. 149, 133–142. doi: 10.3354/meps149133
Rogers T. L., Gouhier T. C., Kimbro D. L. (2022). Distinct temperature stressors acting on multiple ontogenetic stages influence the biogeography of Atlantic blue crabs. Mar. Ecol. Prog. Ser. 690, 97–111. doi: 10.3354/meps14039
Rogers L. A., Griffin R., Young T., Fuller E., St Martin K., Pinsky M. L. (2019). Shifting habitats expose fishing communities to risk under climate change. Nat. Clim. Change 9 (7), 512–516. doi: 10.1038/s41558-019-0503-z
Salvadeo C., Auliz-Ortiz D. M., Petatán-Ramírez D., Reyes-Bonilla H., Ivanova-Bonchera A., Juárez-León E. (2020). Potential poleward distribution shift of dolphinfish (Coryphaena hippurus) along the southern California current system. Environ. Biol. Fishes 103 (8), 973–984. doi: 10.1007/s10641-020-00999-0
Scalici M., Chiesa S., Mancinelli G., Rontani P. M., Voccia A., Nonnis Marzano F. (2022). Euryhaline aliens invading Italian inland waters: the case of the Atlantic blue crab Callinectes sapidus rathbun 1896. Appl. Sci. 12, 4666. doi: 10.3390/app12094666
Seaborn T., Goldberg C. S., Crespi E. J. (2020). Integration of dispersal data into distribution modeling: what have we done and what have we learned? Front. Biogeogr. 12, e43130. doi: 10.21425/F5FBG43130
Semmler R. F., Ogburn M. B., Aguilar R., North E. W., Reaka M. L., Hines A. H. (2021). The influence of blue crab movement on mark-recapture estimates of recreational harvest and exploitation. Can. J. Fish. Aquat. Sci. 78, 371–385. doi: 10.1139/cjfas-2020-0112
Sillero N., Barbosa M. (2021). Common mistakes in ecological niche models. Int. J. Geogr. Inf. Sci. 35, 213–226. doi: 10.1080/13658816.2020.1798968
Sprung M. (2001). “Larval abundance and recruitment of Carcinus maenas l. close to its southern geographic limit: a case of match and mismatch,” in Advances in decapod crustacean research (Dordrecht: Springer), (pp. 153–158). doi: 10.1007/978-94-017-0645-2_16
Streftaris N., Zenetos A. (2006). Alien marine species in the Mediterranean-the 100 ‘Worst invasives’ and their impact. mediterr. Mar. Sci. 7 (1), 87–118. doi: 10.12681/mms.180
Taylor D. L., Fehon M. M. (2021). Blue crab (Callinectes sapidus) population structure in southern new eng-land tidal rivers: patterns of shallow-water, unvegetated habitat use and quality. Estuar. Coast. 44 (5), 1320–1343. doi: 10.1007/s12237-020-
Torn K., Peterson A., Herkül K. (2020). “Predicting the impact of climate change on the distribution of the key habitat-forming species in the NE Baltic Sea,” in Global coastal issues of 2020. Eds. Malvárez G., Navas F.(Coconut Creek, Florida: Coastal Education & Research Foundation, Inc.), 177–181, ISBN: ISSN 0749-0208. Journal of Coastal Research, Special Issue No. 95. doi: 10.2112/SI95-035.1
Tourne D. C. M., Ballester M. V. R., James P. M. A., Martorano L. G., Guedes M. C., Thomas E. (2019). Strategies to optimize modeling habitat suitability of Bertholletia excelsa in the pan-Amazonia. Ecol. Evol. 9, 12623–12638. doi: 10.1002/ece3.5726
Trégarot E., Meissa B., Gascuel D., Sarr O., Valy Y. E., Wagne O. H., et al. (2020). The role of marine protected areas in sustaining fisheries: The case of the national park of banc d’Arguin, Mauritania. Aquac. Fish. 5, 253–264. doi: 10.1016/j.aaf.2020.08.004
Tulis F., Michal Š., Jánošíková R., Ivan B., Michal A., Gyözö H. (2023). The impact of the striped field mouse’s range expansion on communities of native small mammals. Sci. Rep. 13, 753. doi: 10.1038/s41598-022-26919-z
Tyberghein L., Verbruggen H., Pauly K., Troupin C., Mineur F., Clerck O. (2012). Bio-oricle: a global environmental dataset for marine species modelling. Glob. Ecol. Biogeogr. 21, 272–281. doi: 10.1111/j.1466-8238.2011.00656.x
Ulman A., Ali F., Harris H., Adel M., Al Mabruk S., Bariche M., et al. (2022). Lessons from the Western Atlantic lionfish invasion to inform management in the Mediterranean. Front. Mar. Sci. 9. doi: 10.3389/fmars.2022.865162
Vasconcelos P., Carvalho A. N., Piló D., Pereira F., Encarnação J., Teodósio M. A. (2019). Recent and consecutive records of the Atlantic blue crab (Callinectes sapidus rathbun 1896): rapid westward expansion and confirmed establishment along the southern coast of Portugal. Thalassas 35, 485–494. doi: 10.1007/s41208-019-00163-1
Villero D., Pla M., Camps D., Ruiz-Olmo J., Brotons L. (2017). Integrating species distribution modeling into decision-making to inform conservation actions. Biodivers. Conserv. 26, 251–271. doi: 10.1007/s10531-016-1243-2
Wang Y., Tan W., Li B., Wen L., Lei G. (2021). Habitat alteration facilitates the dominance of invasive species through disrupting niche partitioning in floodplain wetlands. Divers. Distrib. 27, 1861–1871. doi: 10.1111/ddi.13376
Warren D. R., Robinson J. M., Josephson D. C., Sheldon D. R., Kraft C. E. (2012). Elevated summer temperatures delay spawning and reduce redd construction for resident brook trout (Salvelinus fontinalis). Glob. Change Biol. 18 (6), 1804–1811. doi: 10.1111/j.1365-2486.2012.02670.x
Wei T., Simko V. (2021). R package ‘corrplot’: visualization of a correlation matrix. (Version 0.92).
Welk E. (2004). Constraints in range predictions of invasive plant species due to non-equilibrium distribution patterns: purple loosestrife (Lythrum salicaria) in north America. Ecol. Modell. 179 (4), 551–567. doi: 10.1016/j.ecolmodel.2004.04.020
Willis C. G., Ruhfel B. R., Primack R. B., Miller-Rushing A. J., Losos J. B., Davis C. C. (2010). Favorable climate change response explains non-native species’ success in thoreau’s woods. PloS One 5 (1), e8878. doi: 10.1371/journal.pone.0008878
Wilson K. A., Able K. W., Heck K. L. (1990). Predation rates on juvenile blue crabs in estuarine nursery habitats: evidence for the importance of macroalgae (Ulva lactuca). Mar. Ecol. Prog. Ser. 58, 243–251. doi: 10.3354/meps058243
WoRMS (2022). Callinectes sapidus rathbun 1896. Available from https://www.marinespecies.org at VLIZ Accessed 2022-04-21.
Zeidberg L. D., Robison B. H. (2007). Invasive range expansion by the Humboldt squid, Dosidicus gigas, in the eastern north pacific. PNAS 104, 12948–12950. doi: 10.1073/pnas.0702043104
Zhang Z., Mammola S., Liang Z., Capinha C., Wei Q., Wu Y., et al. (2020). Future climate change will severely reduce habitat suitability of the critically endangered chinese giant salamander. Freshw. Biol. 65 (5), 971–980. doi: 10.1111/fwb.13483
Zhang Z., Xu S., Capinha C., Weterings R., Gao T. (2019). Using species distribution model to predict the impact of climate change on the potential distribution of Japanese whiting Sillago japonica. Ecol. Indic. 104, 333–340. doi: 10.1016/j.ecolind.2019.05.023
Keywords: Atlantic blue crab, invasive species, species distribution modeling, Atlantic Ocean, global warming
Citation: Costa EFS, Encarnação J, Teodósio MA and Morais P (2023) Aquatic species shows asymmetric distribution range shifts in native and non-native areas. Front. Mar. Sci. 10:1158206. doi: 10.3389/fmars.2023.1158206
Received: 03 February 2023; Accepted: 17 April 2023;
Published: 02 May 2023.
Edited by:
João Canning-Clode, Center for Marine and Environmental Sciences (MARE), PortugalReviewed by:
Raül Triay-Portella, University of Las Palmas de Gran Canaria, SpainJim R. Muirhead, Smithsonian Environmental Research Center (SI), United States
Copyright © 2023 Costa, Encarnação, Teodósio and Morais. This is an open-access article distributed under the terms of the Creative Commons Attribution License (CC BY). The use, distribution or reproduction in other forums is permitted, provided the original author(s) and the copyright owner(s) are credited and that the original publication in this journal is cited, in accordance with accepted academic practice. No use, distribution or reproduction is permitted which does not comply with these terms.
*Correspondence: Eudriano F. S. Costa, eudrianocosta@gmail.com