Ecotrophic perspective in fisheries management: a review of Ecopath with Ecosim models in European marine ecosystems
- 1Laboratory of Ichthyology, Department of Zoology, School of Biology, Aristotle University of Thessaloniki, Thessaloniki, Greece
- 2Department of Biology, Dalhousie University, Halifax, NS, Canada
- 3Department of Biology, Woods Hole Oceanographic Institution, Falmouth, MA, United States
- 4Kinneret Limnological Laboratory, Israel Oceanographic and Limnological Research, Migdal, Israel
- 5Marine Ecology Research Division, GEOMAR Helmholtz Centre for Ocean Research Kiel, Kiel, Germany
- 6Institute of Biosciences and Bioresources, National Research Council of Italy, Sesto Fiorentino, Firenze, Italy
The aim of this work is to present the food web models developed using the Ecopath with Ecosim (EwE) software tool to describe structure and functioning of various European marine ecosystems (eastern, central and western Mediterranean Sea; Black Sea; Bay of Biscay, Celtic Sea and Iberian coast; Baltic Sea; North Sea; English Channel, Irish Sea and west Scottish Sea; and Norwegian and Barents Seas). A total of 195 Ecopath models based on 168 scientific publications, which report original, updated and modified versions, were reviewed. Seventy models included Ecosim temporal simulations while 28 implemented Ecospace spatiotemporal dynamics. Most of the models and publications referred to the western Mediterranean Sea followed by the English Channel, Irish Sea and west Scottish Sea sub-regions. In the Mediterranean Sea, the western region had the largest number of models and publications, followed by the central and eastern regions; similar trends were observed in previous literature reviews. Most models addressed ecosystem functioning and fisheries-related hypotheses while several investigated the impact of climate change, the presence of alien species, aquaculture, chemical pollution, infrastructure, and energy production. Model complexity (i.e., number of functional groups) increased over time. Main forcing factors considered to run spatial and temporal simulations were trophic interactions, fishery, and primary production. Average scores of ecosystem indicators derived from the Ecopath summary statistics were compared. Uncertainty was also investigated based on the use of the Ecosampler plug-in and the Monte Carlo routine; only one third of the reviewed publications incorporated uncertainty analysis. Only a limited number of the models included the use of the ECOIND plug-in which provides the user with quantitative output of ecological indicators. We assert that the EwE modelling approach is a successful tool which provides a quantitative framework to analyse the structure and dynamics of ecosystems, and to evaluate the potential impacts of different management scenarios.
1 Introduction
Due to the complex nature of interactions within marine ecosystems, it is imperative to view and study them as a whole, complementing single-species assessments with ecosystem considerations (Marshall et al., 2019; Howell et al., 2021). The factors that compose marine ecosystems, as biological entities (fauna and flora) and environmental elements (e.g., nutrients), perform important processes either individually or synergistically (Borja et al., 2014; Armoškaitė et al., 2020). Worldwide, marine ecosystems have been facing a multitude of perturbations that threaten their structure and functioning (Elliott et al., 2015), with most important stressors being related to fisheries (Froese et al., 2018), climate change (Bruno et al., 2018) and non-indigenous species (Galil et al., 2018). Recent assessments demonstrate that marine ecosystems are being degraded or altered (Newton et al., 2020; Korpinen et al., 2021). Climate change has brought about rising temperatures that may trigger regime shift dynamics (Möllmann et al., 2021) while ocean acidification may further weaken the ecological resilience of European seas (Galdies et al., 2020). The introduction of alien species can affect entire ecosystems, especially in regional semi-enclosed seas such as the Baltic Sea (Dobrzycka-Krahel and Medina-Villar, 2020), the Black Sea (Shalovenkov, 2019) and the Mediterranean Sea (Zenetos et al., 2017). Much of the pressure also comes from anthropogenic activities; i.e., exploration and extraction of natural resources, transportation, infrastructure and pollution, including the discarding of fishing gears (Korpinen et al., 2021). In addition to natural resources removals, intensive bottom trawling physically damages the seabed by destroying habitats (Woods and Verones, 2019).
The European continent is characterized by great geomorphological complexity and diversity, both on land and at sea (Costello et al., 2006). A multitude of marine ecosystems exists, ranging from lower latitudes, with the closed and relatively warm Mediterranean Sea, to higher latitudes, with the eutrophic Baltic Sea and the Barents Sea, which is frozen during winter (Heiskanen et al., 2016). Marine ecosystems can be divided into two main categories, the deep seas and the shallow seas (Galparsoro et al., 2012). The deep seas (Mediterranean Sea and Black Sea) were created by geotectonic activity while the shallow seas (Adriatic Sea, Baltic Sea and North Sea) were created by the melting of glaciers covering large areas of land (Galparsoro et al., 2012). Moreover, there are the ecosystems related to the Atlantic Ocean (Bay of Biscay and Celtic Sea) on the western European coasts, which are directly affected by its geophysical and environmental conditions (Holt et al., 2014). European marine ecosystems are also distinguished by the many estuarine wetlands (Newton et al., 2014), which are important to local communities and exploited for aquaculture (Gamito and Erzini, 2005; Díaz López et al., 2008; Izquierdo-Gomez et al., 2016) and wind power plants (Halouani et al., 2020).
Software programs simulating ecosystem dynamics have facilitated the holistic study of marine ecosystems. Ecosystem modelling has provided a means for incorporating management perspectives (Fath et al., 2019); i.e., by implementing scenarios related to fisheries management, climatic and other environmental conditions (e.g., Scotti et al., 2022a), as well as the establishment of marine protected areas (MPAs; Dahood et al., 2020). Ecopath with Ecosim (EwE: Polovina, 1984; Christensen and Walters, 2004a) is the most widely used software tool for modelling marine food webs. It is suitable for examining the impacts of human and environmental stressors on the food web, as it is easy to use and offers a wide range of freely available modules and plug-ins (Pauly et al., 2000). It is based on the static depiction of a food web in a mass-balanced state over a specific time period (Ecopath) and uses differential equations for time simulations (Ecosim: Walters et al., 1997). Further extensions integrate temporal simulations with spatial dynamics and visualization (Ecospace: Walters et al., 1999), and support trophic conversion, configuring the food web as biomass movements from lower to higher trophic levels (EcoTroph: Gascuel and Pauly, 2009).
Ecological Network Analysis (ENA) is a toolkit of matrix manipulation techniques for the modelling of mass-balanced networks (Ulanowicz, 2004). Some ENA algorithms are available in EwE and enable calculating indicators summarizing ecosystem structure and functioning; the ENAtool routine also allows incorporating uncertainty levels in input data (Guesnet et al., 2015). Ecological indicators from ENA can be used to identify the state and health of the ecosystem (Heymans et al., 2016). This routine has been further expanded with a plug-in calculating standardised ecological indicators (ECOIND: Coll and Steenbeek, 2017). Additional plug-ins were established and incorporated into the main software program over the years (1) to assess parameters’ uncertainty (Ecosampler: Steenbeek et al., 2018), and (2) to estimate concentrations and densities of contaminants and radioisotopes in aquatic environments (Ecotracer: Walters and Christensen, 2018). Other modules available in the base EwE version include (1) policy optimization of fisheries management (Christensen and Walters, 2004b), (2) spatial optimization of marine protected areas (Christensen et al., 2009), and (3) value chain modelling evaluating the socioeconomic benefits of fisheries (Christensen et al., 2011).
Reviews of EwE applications have previously been completed for the Mediterranean Sea (Coll and Libralato, 2012) and at global scale (Pikitch et al., 2014; Colléter et al., 2015; Coll et al., 2015; Vasslides et al., 2017; Craig and Link, 2023; Stock et al., 2023), highlighting that EwE has the lion’s share in food web modelling with an increasing number of models through the years; i.e., over 430 models stored in an open-access database of EwE models called EcoBase (Colléter et al., 2015) and over 500 publications (Coll et al., 2015). The EcoBase initiative (http://ecobase.ecopath.org) is a collaborative project that aims at gathering published EwE models in a digital open-access repository where the models and their metadata are discoverable and easily accessible, with the goal of facilitating further meta-analyses and reviews (Colléter et al., 2015). However, since EcoBase is based on the principle of optional self-uploading by model developers, it does not cover all EwE models exhaustively, especially when it comes to modified versions of original models that examine new research items.
Our review aims to fill the gaps from the EcoBase repository by including all published models to date, original and updated versions, focusing on European seas’ ecosystems, which are regulated by common mechanisms and rules for achieving the sustainable management of European fishing fleets and conservation of fish stocks. The EU Marine Strategy Framework Directive (MSFD; EU, 2008) and the revised EU Common Fisheries Policy (CFP; EU, 2013) represent relevant regulations for the management of the European seas. They recognise the need of an integrated understanding of food web characteristics, ecosystem functioning and fisheries management, an intent that can be facilitated by the EwE modelling approach. The main objective of this work was to review all published EwE models across European seas (Ecopath, Ecosim, Ecospace, and EcoTroph), aiming to (1) gather and present all EwE models to provide an overview of their main research topics, (2) discuss the hypotheses and objectives of each model by compiling their metadata, (3) identify the modelled areas, (4) highlight gaps in research, and (5) perform a comparative synthesis by assessing ecosystem health and resilience with ecosystem indicators derived from the models. To facilitate these objectives, we divided the EwE models according to nine major areas: (1) eastern Mediterranean Sea, (2) central Mediterranean Sea, (3) western Mediterranean Sea, (4) Black Sea, (5) Bay of Biscay, Celtic Sea and Iberian coast, (6) Baltic Sea, (7) North Sea, (8) English Channel, Irish Sea and west Scottish Sea, and (9) Norwegian and Barents Seas. The ECOIND plug-in (Coll and Steenbeek, 2017), which is integrated in the EwE software tool to calculate ecological indicators, was also included in the analysis.
2 European areas and overview of published EwE models
This review was based on an overview of all EwE models that have been developed for European marine ecosystems to date. At first, an inventory of the models stored in the EcoBase repository was made and metadata were cross-referenced with the corresponding publications (Tables S1, S2). An exhaustive and thorough search was then conducted using literature search engines (Scopus, Google Scholar and Web of Science) to find all available publications with EwE applications in peer-reviewed journals. This systematic search was executed following the PRISMA (Preferred Reporting Items for Systematic Reviews and Meta-Analyses) methodology, which is based on article selection, screening and the extraction of information (Moher et al., 2010), and has been successfully applied in other review exercises (Corrales et al., 2020a). The starting year of eligibility was not defined but a cut-off date of available publications was set as to the first quarter of 2023. For a more efficient realisation of this task, keywords (e.g., “Ecopath”, “Ecosim”, “Ecospace”, and “EcoTroph”) were used in correspondence with the nine European areas and their sub-regions. The goal was to identify all subsequent publications that might be based on one original base model modifying or updating it, and that have not yet been included in EcoBase. Values of the indicators derived from the Ecopath summary statistics were compiled to infer ecosystem health and state, and for comparative analysis (Table 1). Finally, analyses of the publications and models were performed in relation to research topic (i.e., ecosystem functioning and ecological roles, fisheries, aquaculture, alien species, environment and climate) and software modules used; cumulative trends of EwE publications over time were highlighted. It is, however, noteworthy that several publications explored more than one topic. Moreover, forcing factors used to drive the spatio-temporal simulations with Ecosim and Ecospace were examined (Vasslides et al., 2017; Stock et al., 2023). Emphasis was also given to publications that used additional analyses based on the ECOIND plug-in to further demonstrate the utility of ecological indicators. These indicators are widely applied by scientists to characterize environmental and fishing impacts related to management (Coll and Steenbeek, 2017). A panel map of the nine European regions and the areas modelled was created to visualize EwE models and modules used. European areas and an overview of the models are described in the following sections.
2.1 Eastern Mediterranean Sea
The Levantine Sea is part of the eastern Mediterranean basin, which is strongly affected by increasing sea temperature and faces the greatest invasion of marine species in the world (Edelist et al., 2013). The warm, saline and ultra-oligotrophic ecosystem of the Levantine Sea results in very low primary production (Varkitzi et al., 2020). This heavily impacted ecosystem is exposed to climate change and multiple pressures and might benefit of using ecological and trophic models to understand its responses to concurrent stress factors and anthropogenic activities (Albouy et al., 2013; Corrales et al., 2018; Shabtay et al., 2018; Grossowicz et al., 2020). A large part of the Levantine Sea, the Israeli Mediterranean coast, was modelled with Ecopath (Corrales et al., 2017a), Ecosim (Corrales et al., 2017b; Corrales et al., 2018) and Ecospace (Ofir et al., submitted)1to determine the impacts that alien species, sea warming and fisheries have on the ecosystem. The consequences of alien species and fisheries have also been studied in other parts of the Levantine basin such as the insular shelf ecosystem of Cyprus (Michailidis et al., 2019; Michailidis et al., 2023) and the Gulf of Mersin in Turkey (Saygu et al., 2020). Moreover, EwE was applied to model the effects of aquaculture (Livne et al., 2020), to inform marine spatial planning (Shabtay et al., 2018), and to assess energy- (Grossowicz et al., 2020) and economy-related (Peled et al., 2020; Michael-Bitton et al., 2022) questions.
The Aegean Sea is a relatively closed area in the western part of the eastern Mediterranean Sea, located between Greece and Turkey, with numerous islands and islets (Keramidas et al., 2022). It is one of the most productive areas of the eastern Mediterranean basin, especially its northern part (Tsiaras et al., 2012). It is, however, facing the threats of increasing temperature and alien species invasion, although at a lesser extent than the Levantine Sea (Katsanevakis et al., 2020). In the north, the inflow of nutrient-rich and lower salinity waters originating from the Black Sea and large rivers (Lykousis et al., 2002) supports large biomasses of commercially important small and medium pelagic fishes. This complex interplay between stress factors and biodiversity stimulated the implementation of trophic models to assess the state of the food web and the impact of fisheries (Tsagarakis et al., 2010; Papapanagiotou et al., 2020; Tsagarakis et al., 2021; Tsagarakis et al., 2022). Ecopath and Ecosim models were also developed at a regional scale to assess trophic interactions and fisheries impacts in Thermaikos Gulf (Dimarchopoulou et al., 2022), Pagasitikos Gulf (Dimarchopoulou et al., 2019), and Saronikos Gulf (Papantoniou et al., 2021). Moreover, an Ecopath model was built to examine the effects that fish overexploitation, due to commercial fisheries in Greece and Turkey, has on the whole Aegean ecosystem (Keramidas et al., 2022).
2.2 Central Mediterranean Sea
The Adriatic Sea is a body of water in the northernmost part of the Mediterranean Sea, which separates the Italian Peninsula from the Balkans (Kourafalou, 1999). It is divided into two basins, the northern being the shallowest and the southern being the deepest (Russo and Artegiani, 1996). The Adriatic’s salinity is lower than in other Mediterranean basins because the Adriatic collects a third of the freshwater flowing into the Mediterranean Sea thus acting as a dilution basin (Russo and Artegiani, 1996). The northern and central parts of the Adriatic Sea were studied extensively over past years with Ecopath and Ecosim because of their ecosystem characteristics and commercial fisheries concentration (Coll et al., 2007; Barausse et al., 2009; Coll et al., 2009a; Pranovi and Link, 2009; Coll et al., 2010; Libralato et al., 2010; Libralato et al., 2015; Celić et al., 2018); spatial-explicit versions were constructed using Ecospace (Fouzai et al., 2012; Steenbeek et al., 2013). Two Ecopath models, on the microbenthic loop (Vassallo et al., 2006) and the benthopelagic fauna (Vassallo et al., 2017) were developed in southern Adriatic coasts, while an Ecopath model was built for the Strait of Sicily to evaluate the extent of bentho-pelagic coupling in the food web (Agnetta et al., 2019).
In the eastern Ionian Sea, EwE models were built to investigate ecosystem functioning (Piroddi et al., 2016), fisheries (Piroddi et al., 2010; Piroddi et al., 2011a; Moutopoulos et al., 2013; Moutopoulos et al., 2018) and aquaculture (Piroddi et al., 2011b). Four Ecopath models were constructed for the areas of Salento (northeastern sector) and Calabria (southwestern sector) to characterize ecosystem functioning (Ricci et al., 2019; Ricci et al., 2021), while one model of the Gulf of Taranto was assembled to assess interactions between cetaceans and commercial fisheries (Carlucci et al., 2021). Outside of the European waters, an Ecopath model was developed for the Gulf of Gabes, Tunisia (Hattab et al., 2013). It was extended to implement spatio-temporal simulations (Abdou et al., 2016; Halouani et al., 2016; Abdou et al., 2020) and trophic analysis (Halouani et al., 2015). These models, even though were developed for the northern African coasts, were included in the review because they are located in the central Mediterranean Sea and adjacent to European waters.
2.3 Western Mediterranean Sea
The Balearic Sea is part of the wider north-west Mediterranean Sea and is considered to be the most productive area of the otherwise oligotrophic Mediterranean Sea (D'Ortenzio and Ribera d'Alcalà, 2009). It is a breeding ground for small pelagic fishes such as the European anchovy (Engraulis encrasicolus). Moreover, it represents a major fishing ground for commercial fisheries and a highly exploited ecosystem (Papaconstantinou and Farrugio, 2000). The south Catalan Sea is in the northern part of the Balearic archipelago and is the most modelled area of the Mediterranean Sea (Coll and Libralato, 2012). It has been extensively modelled using EwE, either considering a static framework (Ecopath: Coll et al., 2006a; Coll et al., 2006b; Coll et al., 2009b; Navarro et al., 2011) or implementing temporal (Ecosim: Coll et al., 2008a; Coll et al., 2008b; Coll et al., 2012; Forrestal et al., 2012; Coll et al., 2013; Tecchio et al., 2013) and spatiotemporal (Ecospace: Coll et al., 2016a; Pennino et al., 2020) simulations. An Ecopath model that describes food web traits and resilience to fisheries exploitation was developed for the wider area of the northwestern Mediterranean Sea (Corrales et al., 2015). Smaller-scale models were constructed for other regions of the western Mediterranean Sea: the Gulf of Lions (Bănaru et al., 2013; Vilas et al., 2021; Seyer et al., 2023), the Gulf of Alicante (García-Rodríguez et al., 2021), the Santa Pola Bay (Bayle-Sempere et al., 2013; Izquierdo-Gomez et al., 2016), the Cerbère-Banyuls (France), Cap de Creus (Spain) and Medes Islands (Spain) MPAs (Corrales et al., 2020b), and the Port Cros; case studies integrating the EcoTroph plug-in are also available (Valls et al., 2012; Prato et al., 2014). Further east, in the wider areas of the Tyrrhenian and Ligurian seas, EwE models were built focusing on fisheries (Pinnegar and Polunin, 2004; Albouy et al., 2010; Vanalderweireldt et al., 2022), aquaculture (Díaz López et al., 2008),MPAs (Prato et al., 2016) and the microbenthic loop (Fabiano et al., 2004; Vassallo et al., 2012; Vassallo et al., 2013; Vassallo et al., 2022).
2.4 Black Sea
The Black Sea is a marginal sea located at the northeast of the Mediterranean Sea, which is connected with the Aegean Sea through the Sea of Marmara. It is the world’s largest body of water with a meromictic basin as the deep waters do not mix with the upper layers that receive oxygen from the atmosphere (Sabatino et al., 2020). As a result, over 90% of the deeper Black Sea volume is anoxic and water circulation is primarily controlled by basin topography and fluvial inputs, which result in a strongly stratified vertical structure (Reeburgh et al., 1991). In the previous decades, significant trophic transformations occurred in the basin, with the most remarkable being the introduction and extreme expansion of the warty comb jelly (Mnemiopsis leidyi), an invasive ctenophore from the western Atlantic. In particular, the outburst of M. leidyi in the late 1980s was facilitated by the simultaneous action of overfishing, climate and nutrient enrichment (Bodini et al., 2018). The abrupt increase in the size of M. leidyi population led to a dramatic drop in commercially important small pelagics like the European anchovy due to competition for the same food sources (mostly copepods) and intraguild predation on its eggs (Kideys, 2002).
Two EwE models were created for the Black Sea, focusing on fishing effects on the food web along a timeline of 30 years, starting from the 1960s (Daskalov, 2002), and assessing the relationships that later linked overfishing to the successful establishment of the warty comb jelly (Gucu, 2002). Moreover, for better understanding food web dynamics, four Ecopath models of the Black Sea were constructed. These models focus on reference periods that match significant structural changes in the food web: (1) the pre-eutrophication period of 1960s, (2) the intense eutrophication phase of 1980s, (3) the regime shift of early-1990s with warty comb jelly displacing the European anchovy, and (4) the post-eutrophication period of the late-1990s with increasing top-down control of another invader, i.e., the brown comb jelly (Beroe ovata) over M. leidyi (Akoglu et al., 2014). Finally, three Ecopath models were recently developed in the Sea of Marmara, the connecting water body between the Black Sea and the Aegean Sea, investigating fisheries impacts in the food web during the period of three decades (Saygu et al., 2023).
2.5 Bay of Biscay, Celtic Sea and Iberian coast
The Bay of Biscay is a relatively large and deep gulf of the northeast Atlantic Ocean, located south of the Celtic Sea. It is considered a very productive area due to the upwelling characteristics and the drainage waters of large rivers (Borja et al., 2008). It displays a high biodiversity with many habitats and marine species, including endangered and protected species like cetaceans and seabirds (Matear et al., 2019). It faces intense human pressure, including industrialised commercial fisheries from France and Spain, and significant marine transportation (Lorance et al., 2009). EwE models were developed for the Bay of Biscay/Celtic Sea ecosystem (Bentorcha et al., 2017), the Bay of Biscay as a whole (Moullec et al., 2017; Corrales et al., 2022), including a specific focus on its continental shelf only (Lassalle et al., 2011; Lassalle et al., 2012; Lassalle et al., 2014; Le Marchand et al., 2022), and the Celtic Sea (Hernvann et al., 2020). The southern part of the Bay of Biscay, the Cantabrian Sea, is comparatively less studied, with an Ecopath model developed for 1994 to assess fisheries impacts on the food web of the continental shelf (Sánchez and Olaso, 2004). A single Ecopath model was developed describing the trophic network of the Gironde Estuary for the late-1990s/early-2000s (Lobry et al., 2008). The Gironde Estuary is one of the largest and least-polluted estuaries in northern Europe (Sautour and Castel, 1995) and its basin is connected with the Bay of Biscay.
South of the Bay of Biscay and Celtic Sea lies the west coast of the Iberian Peninsula. An Ecopath model was developed for the Gulf of Cadiz, located at the southern part of the west coast, investigating the trophic relationships and fishing impacts in an exploited ecosystem (Torres et al., 2013). Further north, EwE models were constructed for the Portuguese and Galician coasts, focusing on food web structure and ecological roles (Veiga-Malta et al., 2019; Giralt Paradell et al., 2020; Giralt Paradell et al., 2021), small pelagic fisheries (Szalaj et al., 2021) and climate (Szalaj et al., 2022). Smaller parts of the west coast of the Iberian Peninsula and lagoon ecosystems were modelled separately; namely, the Ria Formosa (Gamito and Erzini, 2005), the Mondego Estuary (Patrício and Marques, 2006; Baeta et al., 2011), the Ria de Aveiro (Bueno-Pardo et al., 2018) and the Ria de Arousa (Outeiro et al., 2018). In the southernmost part of the NE Atlantic is the Azores archipelago, composed of nine islands under Portuguese jurisdiction, where an Ecopath model was developed to describe the food web and vulnerabilities of an open-ocean ecosystem (Morato et al., 2016). Finally, a theoretical seamount ecosystem of the NE Atlantic was used as the basis for a trophic model to examine the potential effects of an increase in primary productivity (Morato et al., 2009).
2.6 Baltic Sea
The Baltic Sea is a marginal sea of the northeastern Atlantic Ocean, with limited water exchange between the two water bodies (Barale, 2008). It is considered one of the largest brackish water bodies in the world and its salinity is significantly lower than that of ocean waters (Vuorinen et al., 2015), defining its inherent low biodiversity (Ojaveer et al., 2010). As saltwater is denser than freshwater, the bottom of the Baltic Sea is saltier than the surface (Sohlenius et al., 2001). This condition creates vertical stratification of the water column, i.e., a halocline, which represents a barrier to the exchange of oxygen and nutrients and fosters completely separate marine environments (Väli et al., 2013). The Baltic Sea has been exposed to the simultaneous action of multiple interacting pressures, a feature that qualifies this ecosystem as an ideal site to predict the impact and formulate mitigation of future coastal perturbations (Reusch et al., 2018). As the Baltic proper is subject to most of the human pressures, such as fishing, nutrient pollution and climate change, scientific research and development of ecosystem models in the Baltic Sea have been concentrated mostly there (Korpinen et al., 2022; Reckermann et al., 2022). Several EwE models have been developed in this area, including Ecopath (Sandberg et al., 2000; Harvey et al., 2003; Tomczak et al., 2012; Tomczak et al., 2013), Ecosim (Österblom et al., 2007; Niiranen et al., 2013; Costalago et al., 2019) and Ecospace (Bauer et al., 2018; Bauer et al., 2019) applications, focusing mostly on primary production fluctuations and climate change impacts on the food webs. Recently, an EwE model of the western Baltic Sea was constructed to assess the impact that alternative fisheries management strategies have on the status of commercial stocks, diversity of top predators and fishing yield (Scotti et al., 2022a). At a smaller scale, an Ecopath model was developed for an open coastal area in the southwestern part of the Bothnian Sea to evaluate radioactive fluxes in the food web using the Ecotracer tool (Sandberg et al., 2007). Finally, separate Ecopath models were created for five southeastern Baltic Sea ecosystems to compare the structure of their carbon flow networks: the Puck Bay, the Curonian Lagoon, the Lithuanian coast, the coastal area of the Gulf of Riga and the Pärnu Bay (Tomczak et al., 2009).
2.7 North Sea
The North Sea is a shelf sea on the European continental shelf, connected to the Atlantic Ocean through the English Channel in the south and the Norwegian Sea in the north (Lüdmann et al., 2021). It is a relatively shallow sea, with a mean depth of 90 meters (Lee, 1980). Due to the large human population and high level of industrialization along its shores, the wildlife of the North Sea has suffered from pollution, overhunting, and overfishing, with most of the commercial fisheries stocks considered to be either fully exploited or overexploited (Akbari et al., 2022). The North Sea is Europe’s main fishing ground, accounting for over 5% of international commercial fish caught, concentrated mostly in the southern part of the coastal waters (Mackinson et al., 2018). An Ecopath model representing the total area of the North Sea was created for 1981, known as the ‘Year of the Stomach’, when more than 55,000 fish were sampled and analysed for their stomach contents through an ICES-coordinated project (Christensen, 1995a). Other EwE models were developed for different periods (Beattie et al., 2002; Mackinson and Daskalov, 2007; Heymans et al., 2011a; Romagnoni et al., 2015) while an Ecosim model of the same area, using multiple environmental drivers, served as the basis for multi-annual plans regarding fisheries and management in the North Sea (Mackinson, 2014). An EwE model was constructed for the southern part of the North Sea to focus on fisheries management (Stäbler et al., 2016; Stäbler et al., 2018); the model was then used as a backbone to develop an Ecospace application integrating habitat preferences (Püts et al., 2020; Püts et al., 2023). In the western part of the North Sea, Ecopath models were developed for the northeastern coast of Scotland (Otogo et al., 2015) and the Eden Estuary (Watson et al., 2020).
2.8 English Channel, Irish Sea and west Scottish Sea
The English Channel is a strait of the Atlantic Ocean that separates southern England from northern France, and is linked to the southern part of the North Sea by the Strait of Dover (Smith, 1989). It is the busiest shipping area in the world (Glegg et al., 2015) and has been exposed to environmental problems caused by accidents involving ships with toxic cargos and oil spills (Vieites et al., 2004). Despite the intense marine traffic, it remains an important ecosystem for marine species that inhabit its numerous bays and estuaries, especially in the western part, including marine mammals such as the grey seal (Halichoerus grypus) and the harbour seal (Phoca vitulina; Vincent et al., 2017). Separate EwE models were developed for the western part (Araújo et al., 2005; Araújo et al., 2006; Araújo et al., 2008) and the eastern part (Villanueva et al., 2009; Daskalov et al., 2011; Metcalfe et al., 2015). An area of the eastern English Channel, the Bay of Seine, has been extensively modelled with EwE because of its ecological characteristics and multiple anthropogenic disturbances. Several models have been constructed giving insights on food web structure (Rybarczyk and Elkaıüm, 2003; Tecchio et al., 2015), energy facilities disturbance (Pezy et al., 2017; Raoux et al., 2017; Raoux et al., 2019; Halouani et al., 2020; Noguès et al., 2022) and climate change (Bourdaud et al., 2021). At a smaller scale, food web models were created for the Bay of Somme (Rybarczyk et al., 2003), the Bay of Mont Saint Michel (Arbach Leloup et al., 2008), the Tamar Estuary (Watson et al., 2020) and the Canche Estuary (Selleslagh et al., 2012)
Further north, the continental shelf of the west coast of Scotland, including the waters around the islands in the Sea of the Hebrides, has been extensively modelled with Ecopath (Alexander et al., 2015), Ecosim (Heymans et al., 2011b; Serpetti et al., 2017; Baudron et al., 2019) and Ecospace (Sayer et al., 2005; Alexander et al., 2016; Serpetti et al., 2021). The Irish Sea is an extensive body of water that separates the islands of Ireland and Great Britain and is linked to the sea of the west coast of Scotland in the north by the North Channel, engulfing numerous estuaries with great biodiversity. However, it is considered to be one of most radioactively contaminated seas in the world with high amounts of nuclear waste, like 137Cs, Pu, and 241Am, discharged daily into it from the Sellafield nuclear site (Ray et al., 2020). This discharge contaminates seawater, sediments and marine life, and several EwE models were developed to address these issues (Tierney et al., 2018; Bentley et al., 2019a; Bentley et al., 2019b; Bentley et al., 2019c; Bentley et al., 2020). Finally, an Ecopath model was built for the Faroe Islands marine ecosystem in 1997 and extended with spatio-temporal simulations to 2007 for modelling spatial fishing restrictions (Zeller and Reinert, 2004).
2.9 Norwegian and Barents Seas
The Norwegian Sea is a marginal sea of the Atlantic Ocean, located northwest of Norway and north of the North Sea. Unlike the majority of the world seas, most of the bottom of the Norwegian Sea is not part of a continental shelf and therefore it is characterised by deep waters (Sætre, 1999). It is in fact a transition zone between Boreal and Arctic conditions, and is thus inhabited by biodiversity of both climatic regions, including many seabirds and marine cetaceans (Jørgensen et al., 2022). In the northeast, its waters are linked to the Barents Sea, which is located in the southern part of the Arctic Ocean (Johannesen et al., 2012). Contrarily to the Norwegian Sea, the Barents Sea is a relatively shallow shelf sea (Gudlaugsson, 1993). The Barents Sea contains the world’s largest remaining population of Atlantic cod (Gadus morhua) while the Norwegian Sea is one of the most important spawning grounds of the Atlantic herring (Clupea harengus), defining them as very important commercial fishing grounds (Ottersen et al., 2014; Pampoulie et al., 2015). EwE models were developed to describe these systems, focussing on the Norwegian Sea and Barents Sea ecosystem as a whole (Dommasnes et al., 2001; Bentley et al., 2017), on the Barents Sea ecosystem (Blanchard et al., 2002; Megrey and Aydin, 2009; Pedersen et al., 2021; Pedersen, 2022) and the Pechora Sea (Larsen et al., 2016). The coastal areas of the Norwegian Sea, known as fjords, are unique and important and have been modelled using EwE to study the Arctic ecosystem functioning (Pedersen et al., 2008; Pedersen et al., 2016), the red king crab (Paralithodes camtschaticus) invasion (Pedersen et al., 2018), and ocean warming impacts on kelp forests (Vilas et al., 2020a; Vilas et al., 2020b).
3 Results
Overall, 195 Ecopath models, 70 Ecosim models, 28 Ecospace models and 8 EcoTroph models from the European seas were extracted from 168 peer-reviewed journal publications (Figure 1). Altogether, a total of 301 EwE models were reviewed and analysed, including original models (n=212, 70%), their updated versions with the inclusion of Supplementary Data and information (n=32, 11%), and variant versions with modifications of the original or updated models according to the research subject and needs (n=57, 19%). While the models covered nine different regions, most publications referred to the western Mediterranean Sea (n=31, 18%) while the Black Sea had the fewest (n=4, 2%). More than half of all publications dealt with the investigation of food web structure, functioning and ecological roles (35%), and fisheries (28%; Figure 2). The majority of the models were mass-balanced Ecopath base models, most of which were in the western Mediterranean Sea (n=37, 19%), followed by the Bay of Biscay, Celtic Sea and Iberian coast region (n=35, 18%). In the Mediterranean Sea, the western region was described by most models (n=57, 46%) and publications (n=31, 40%), and is followed by central (n=39, 31% and n=27, 35%) and eastern regions (n=29, 23% and n=19, 25%). The cumulative number of EwE models increased with time, with the highest rate of increase observed for Ecopath models (Figure 3). Almost half of the models were published in the 2010s (49%), with a high number of publications appearing after 2020 (28%). The number of functional groups (FGs) included in the models exhibited similar patterns, with an increasing trend (i.e., higher resolution in food web construction) over time; their number ranged from 8 up to 108, although most of the models (32%) were composed of 31 to 40 FGs (Figure 4). Regarding forcing factors driving spatio-temporal dynamics and used for hindcasting, most of them were related to trophic interactions with the estimation of vulnerabilities (74%), fisheries (74%) and primary production (61%) (Figure 5). The bulk of Ecopath models describes trophic networks in the 1990s (n=58, 30%) and 2000s (n=67, 34%) while no model has a starting year before 1950. Ecosim models had a simulation timeframe reaching up to 150 years, monitoring biomass, fisheries and climate changes over a two-century period while Ecospace displayed a shorter simulation timeframe; i.e., less than 100 years, because of its spatial nature and resulting data and computational requirements, demanding longer simulation runs (Walters et al., 1999).
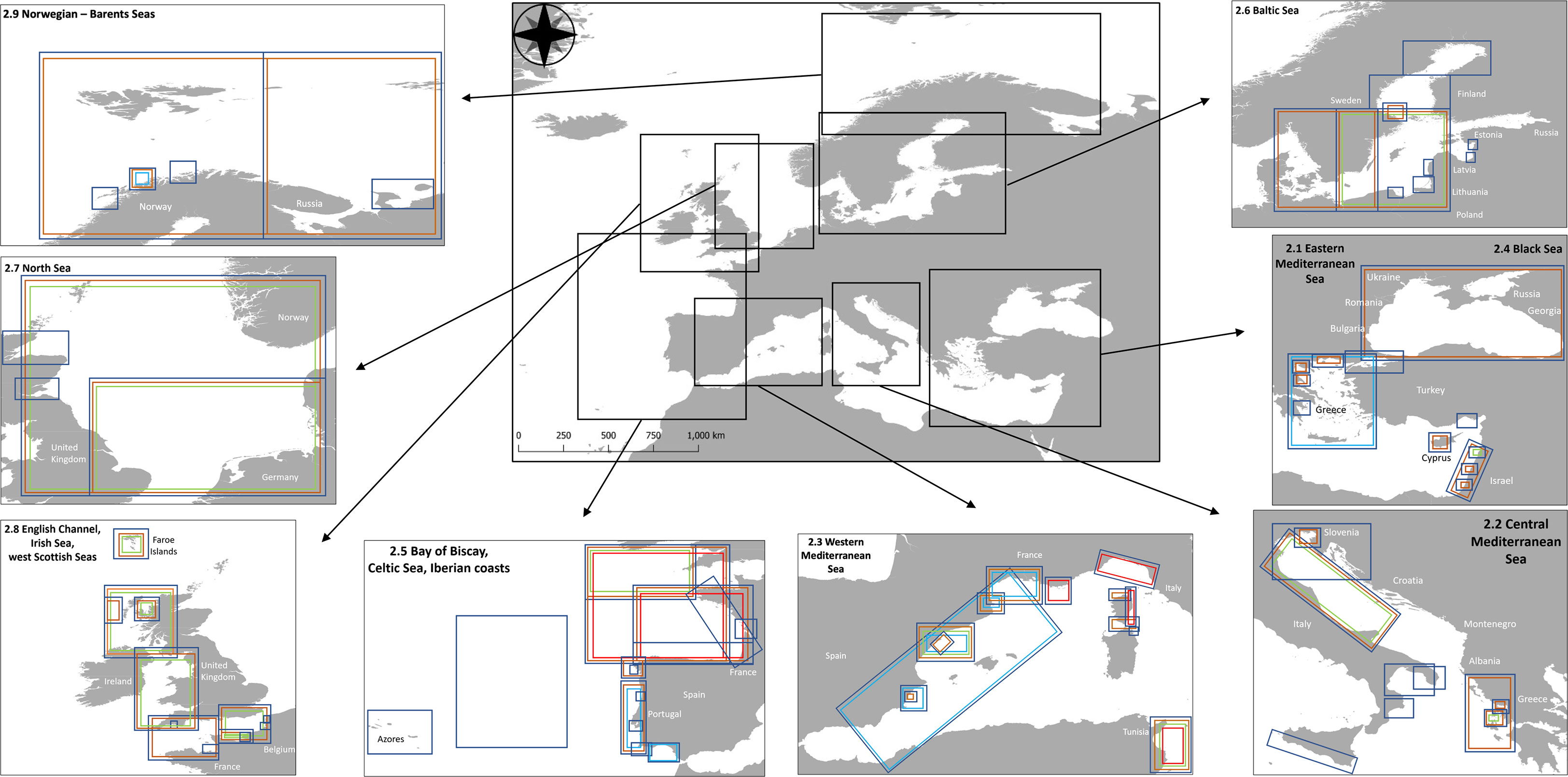
Figure 1 Map showing the nine European marine regions considered in this review. Colored rectangles encompass the approximate area modelled with EwE in all nine regions, including Ecopath models (blue rectangles), Ecosim models (orange rectangles), Ecospace models (green rectangles), EcoTroph models (red rectangles), and ECOIND plug-in expansions (light blue rectangles).
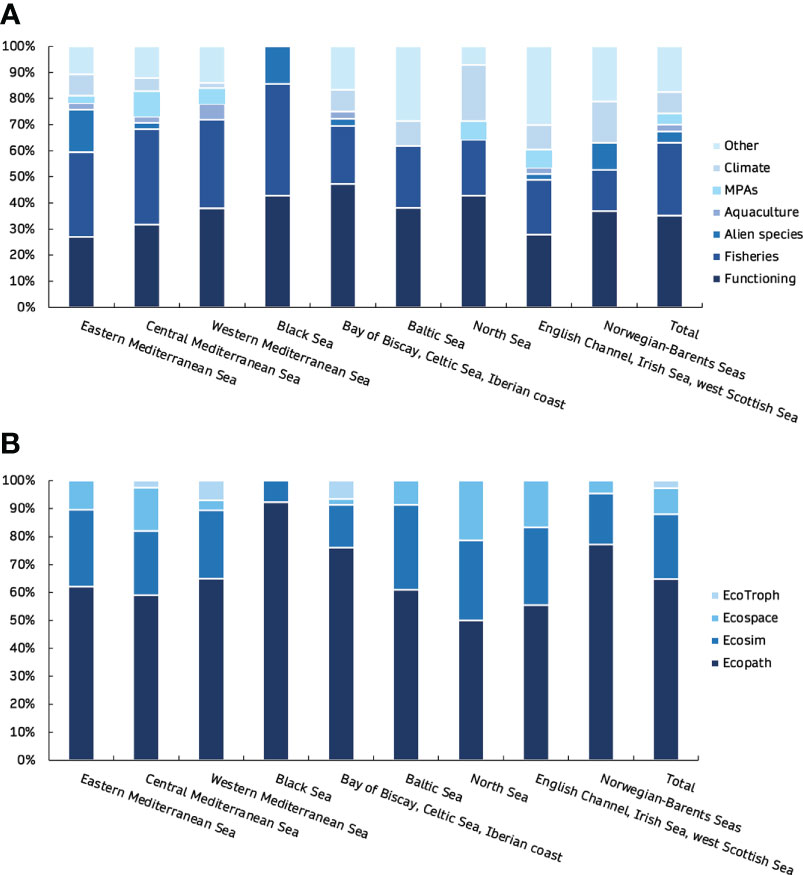
Figure 2 Percentage (y-axis) of EwE model publications in peer-reviewed journals classified by topic (A) and by EwE module (B) for the nine European marine regions.
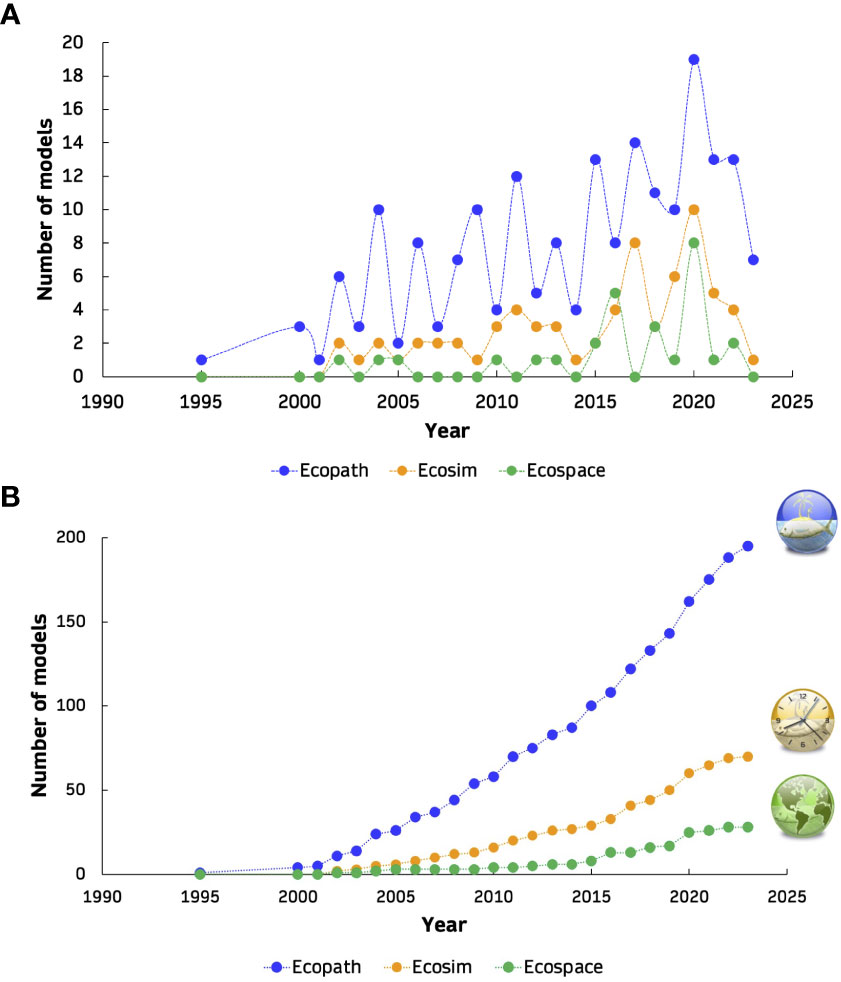
Figure 3 Absolute (A) and cumulative (B) annual number of Ecopath (blue), Ecosim (orange) and Ecospace (green) models in peer-reviewed publications and referring to European waters since 1995.
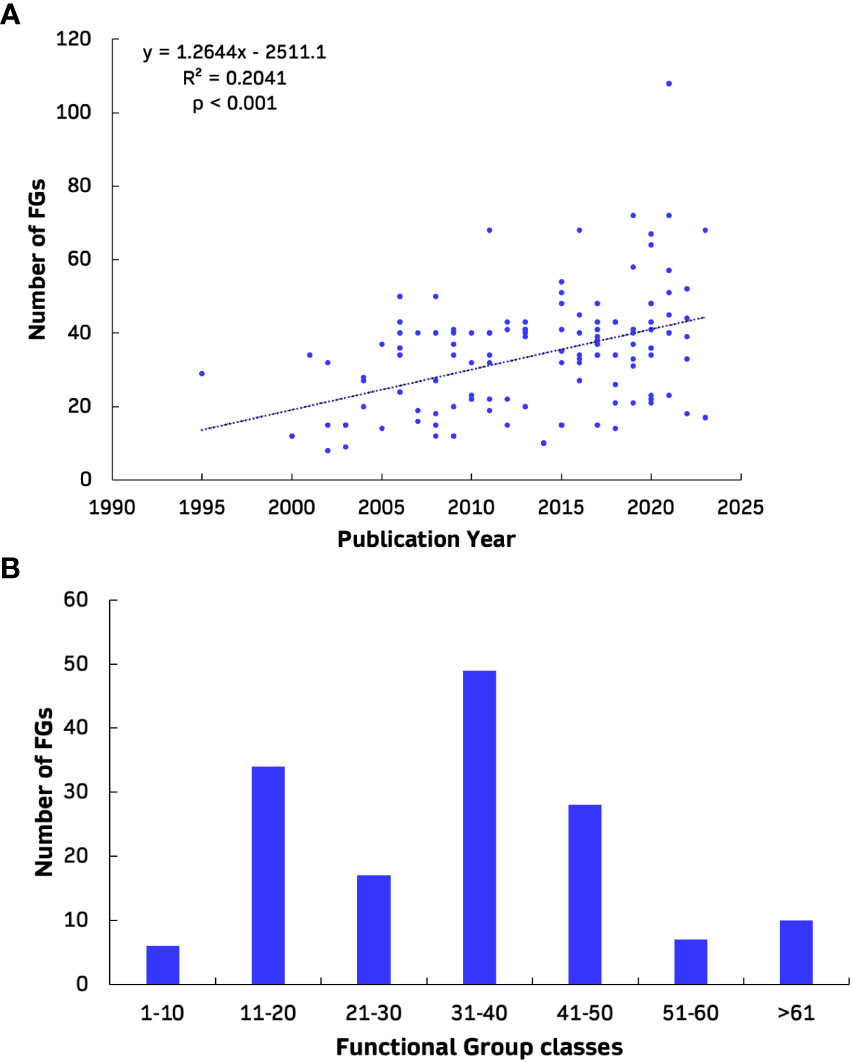
Figure 4 Number of functional groups (y-axis) in relation with time of Ecopath models (A) and divided by size classes (B) in all nine reviewed European marine regions. The dotted line illustrates the trend identified with a linear model, indicating a correlation with highly statistical significance (p value < 0.001).
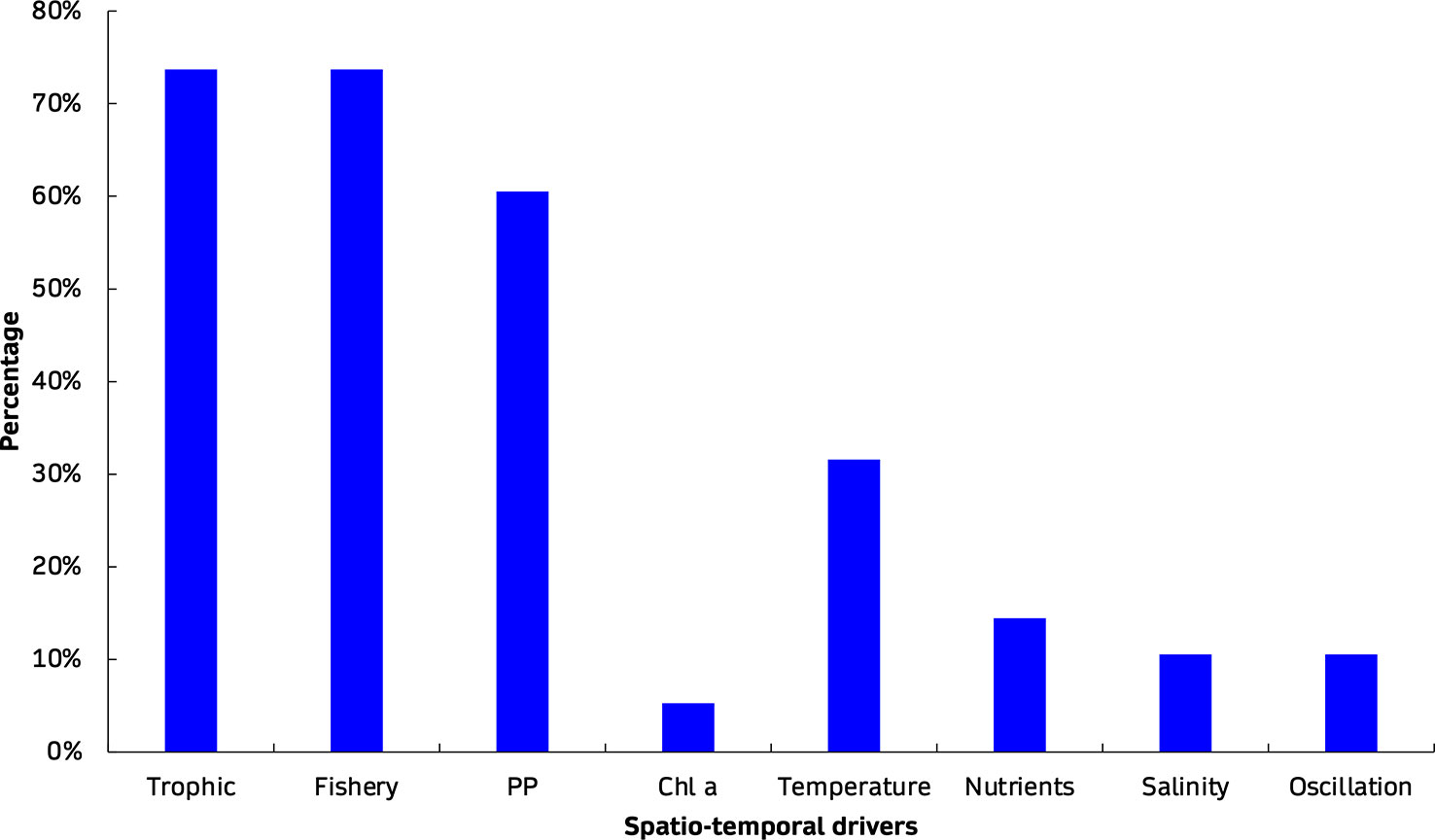
Figure 5 Forcing factors used as drivers for spatio-temporal simulations (i.e., Ecosim, Ecospace) in the calibration and fitting procedures; the y-axis informs on their percentage of occurrence.
3.1 Ecosystem indicators and summary statistics
Ecological indicators obtained from Ecopath summary statistics for the models in the nine European marine regions are given as weighted averages for each area, including the minimum and maximum range limits (Table 2). These indicators were scaled and visualised as radar charts for comparisons (Figure 6). The largest average Ecopath modelling area was found for the Norwegian-Barents Sea region (almost 1 million km2) while the smallest was that of the western Mediterranean Sea region (below 5,000 km2), indicating the presence of many smaller models. A pattern of increasing modelling area with decreasing latitude was observed. The Norwegian-Barents Sea and the North Sea regions had the highest number of FGs (47 each), followed by the western Mediterranean Sea (41); the Black Sea had the fewest (13) and total average number of FGs was 34.
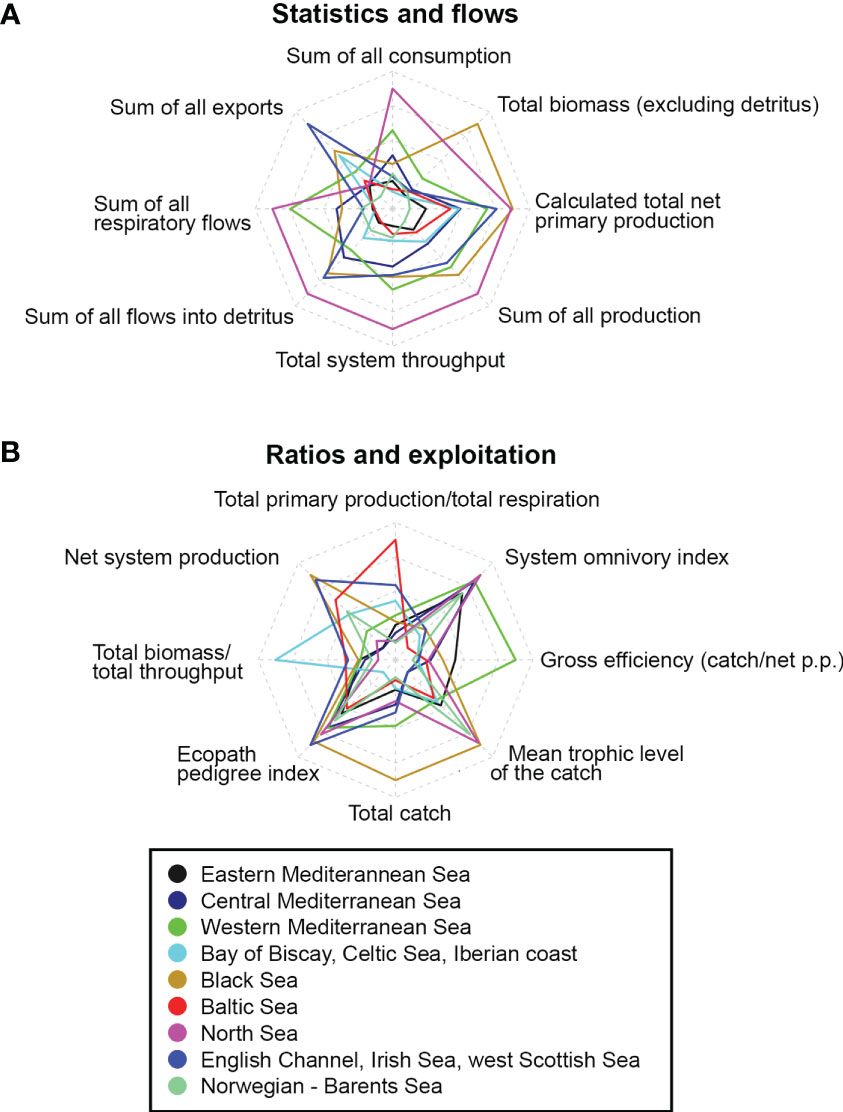
Figure 6 Radar charts of ecological indicators and average summary divided in statistics and flows (A) and ratios and exploitation (B). Scoring scale ranges from -2 to 2 for Ecopath models of the nine reviewed European marine areas. The units of the indicators are in accordance with Table 2.
The Total System Throughput (TST) expresses the sum of all system’s flows (total consumption + total export + total respiration + total flows to detritus), representing the size of the system (Ulanowicz, 1986). The North Sea region had the highest average TST (10,285.1 tkm-2year-1) while the eastern Mediterranean Sea had the lowest (1,767.8 tkm-2year-1), a pattern that is in line with expectation as TST depends on the number of flows in a network (Scharler et al., 2015). The Bay of Biscay, the Celtic Sea and Iberian coast had the highest total primary production, followed by other upwelling or eutrophic areas like the North Sea and the west Scottish Sea. Ecosystem maturity can be expressed as the ratio of total primary production over total respiration (Odum, 1971); the less mature ecosystems were in the Baltic Sea region (8.48) while those closest to maturity were in the Norwegian-Barents Sea region (1.01). In less mature systems the production exceeds the respiration for most groups, leading to ratios greater than 1 (Odum, 1971). Average estimates of total biomass (excluding detritus) were higher in the Bay of Biscay, Celtic Sea and Iberian coast models (851.1 tkm-2) while those in the Black Sea had the lowest values (22.7 tkm-2). Regarding commercial fisheries, the ecosystems with most catches (landings plus discards) were observed in the Bay of Biscay, Celtic Sea and Iberian coast region (14.1 tkm-2year-1) following biomass trends, while lowest levels of fish extraction were found in the Norwegian-Barents Sea region (0.08 tkm-2year-1). Mean trophic level of the catch (MTLc) provides clues about the effect of fisheries on the ecosystem; it is calculated as the weighted average trophic level of the species removed, with their relative biomass contribution to the catch used as a scaling factor (Shannon et al., 2014). A decreasing trend of this indicator in time relates to the progressive overexploitation of fish stocks along the trophic chain, from top predators to lower trophic-level consumers (Pauly et al., 1998). The Bay of Biscay, Celtic Sea and Iberian coast ecosystems had the highest average MTLc scores (3.59), indicating the presence of large predatory species in the catch while central Mediterranean, English Channel, Irish Sea and west Scottish Sea regions had the lowest average scores (<3), which represents highly exploited fish stocks. The System Omnivory index (SOi) is a measure of the distribution of feeding interactions among trophic levels, developed for complexity and connectivity evaluations in the food web (Pauly et al., 1993). The North Sea region had the highest SOi (0.24), indicating that various trophic groups obtain energy from trophic chains of different lengths. However, all models were characterised by low values compared to temperate ecosystems close to the tropics (Christensen, 1995b).
3.2 Ecological indicators of the ECOIND plug-in
A series of algorithms enabling the calculation of various ecological indicators was added to EwE with the ECOIND plug-in (Coll and Steenbeek, 2017). This plug-in returns standardised indicators incorporating a series of biological traits and features, and provides useful tools for further analysis of the ecosystem, in both static and spatio-temporal dynamics. Due to the relatively recent integration of ECOIND into EwE, it was not widely used in the EwE models reviewed here (Table S3). ECOIND results can be extracted adopting a static (Coll and Steenbeek, 2017; Vilas et al., 2020a; García-Rodríguez et al., 2021; Keramidas et al., 2022) or a spatio-temporal dynamic (Bentley et al., 2019a; Corrales et al., 2020b; Hernvann et al., 2020; Vilas et al., 2020b; Vilas et al., 2021; Le Marchand et al., 2022; Piroddi et al., 2022; Szalaj et al., 2022) perspective.
The ECOIND plug-in can facilitate the comparison between ecosystems with respect to food web structure and functioning (Coll and Steenbeek, 2017). In the latest ECOIND edition, indicators are classified into five categories: (1) biomass, (2) catch, (3) trophic, (4) species, and (5) size-based. Various assessments related to the MSFD have already been successfully implemented with ecological indicators derived from models of various types (e.g., biogeochemical, hydrodynamic, multi-species, end-to-end) in a number of software programs, including EwE (Lynam and Mackinson, 2015; Susini and Todd, 2021), with encouraging results (Piroddi et al., 2015a). There is a multitude of ecological indicators, which can also inform ecosystem-based fisheries management (EBFM), that have been used to successfully address fisheries (Fay et al., 2013; Lassen et al., 2013; Halouani et al., 2019; Link and Watson, 2019) and climate (Shin et al., 2018) issues. As the stressors affecting marine ecosystems increase, so is the need for testing and validating ecological indicators (Rombouts et al., 2013; Ito et al., 2023). A key issue facing the scientific community is the identification of indicators that are the most suitable to effectively describe the status of ecosystems, helping decision-making bodies (Safi et al., 2019). EwE is based on an interactive approach within the scientific community and ECOIND represents a quick and user-friendly tool for assessments based on ecological indicators (Coll et al., 2016b).
4 Discussion
This review of all published Ecopath with Ecosim (EwE) models of European seas aims to serve as an easily accessible and quick guide for researchers working on ecosystem modelling. This centralised aggregation of European EwE models and their metadata highlights the evolution of models and research topics over time and across space. European seas and the NE Atlantic have been extensively modelled, compared to other regions like the Indian and Antarctic Oceans. Also, the Mediterranean Sea has been modelled as a whole ecosystem with two Ecopath models representing the 1950s and the 2000s, sub-divided into four separate sections: western, Adriatic, Ionian and eastern region (Piroddi et al., 2015b). These sub-models were simulated temporally (Piroddi et al., 2017) with Ecosim to demonstrate the effects of climate change and fisheries, and spatiotemporally with Ecospace (Piroddi et al., 2022) to inform ecosystem-based management (EBM). Scientific projects with adequate funding schemes and data availability can support this trend in European regions (Colléter et al., 2015). However, there are still some European marine ecosystems with no existing models; this is the case of several Mediterranean countries (e.g., Malta, Albania and Montenegro) and the southern part of the Norwegian Sea. As many models assessed by this review are not in EcoBase, we strongly encourage the scientific community to use this repository to store metadata of EwE models and take advantage of this large network of users (Colléter et al., 2015). However, the existence of a quality control evaluating the models stored in the EcoBase, similar with the Ecopath pedigree routine, including also Ecosim and Ecospace models (e.g., a quality measure with a traffic light system), would be beneficial for scientists implementing various meta-analyses. Ecopath models are the most numerous because they are a prerequisite for further developing temporal (Ecosim) and spatial (Ecospace) simulations (Christensen and Walters, 2004a). As several Ecopath models have been already developed in the European seas, a good future suggestion can be their temporal or spatio-temporal expansion with Ecosim and Ecospace, which are comparatively less developed, recognizing nevertheless the difficulties of obtaining reliable spatial biological and environmental data for validation and hindcasting (Townsend et al., 2014; Colléter et al., 2015).
In line with the need for more effective and holistic considerations in fisheries management, the EBFM approach is key to moving forward. According to Pikitch et al. (2004), the aim of EBFM is threefold: (1) to maintain ecosystem health and resilience, and fisheries sustainability; (2) to take into account all ecosystem components; and (3) to establish a network of indicators to measure ecosystem degradation. EwE can inform on ecosystem-based management (EBM; Piroddi et al., 2022) and more specifically EBFM, with many representative studies (e.g., Guénette et al., 2006; Bourdaud et al., 2016) supporting practical implementations (Smith et al., 2007; Ofir et al., 2022). EBFM takes into consideration the complex dynamics of ecological, fisheries and human interactions in management (Long et al., 2015). EwE models embraced this direction, including aspects of previously ignored ecosystem elements (e.g., species interactions, discards and bycatch) to implement a holistic approach to managing fisheries (Fulton et al., 2014; Trochta et al., 2018). The EwE modelling framework incorporates fisheries, species interactions, environmental drivers, human activities, complexity and uncertainty, all important aspects of EBFM (Collie et al., 2016). In the future, more emphasis can be placed on plug-ins such as EcoTroph and/or ECOIND. EcoTroph can provide in fact more insights into the distribution of biomass and catch among trophic levels, taking into account the whole trophic spectrum, while ECOIND can further expand the framework of ecological analyses.
The assessment of ecosystem summary indicators highlighted some interesting trends. Highest average estimates of total biomass (excluding detritus) and of trophic flows were observed in ecosystems at higher latitudes. Positive correlations between biomass and fishery yields at colder, higher latitude ecosystems have been observed in the literature (Friedland et al., 2012). Such systems appear to have high seasonal productivity, being favoured by advection processes to sustain large shoals of commercially important fishes (Hunt et al., 2016). However, these systems are among the first to be affected by ocean warming (Kjesbu et al., 2014; Lotze et al., 2019). Many publications from the current review (e.g., Bentley et al., 2017; Vilas et al., 2020b; Pedersen et al., 2021) presented models considering the impacts of climate change and ocean warming (Stock et al., 2023). Consequently, the majority of the climate-related publications were focused on higher-latitude ecosystems (63%). In the Mediterranean Sea, chlorophyll a and primary production gradually decline from the western to the eastern basin, according to field and satellite data (e.g., Lavigne et al., 2015; Mattei and Scardi, 2022). As a result, a declining trend of total biomass and ecosystem size (TST) was observed from west to east; the Black Sea displayed instead the lowest estimates of total biomass. As the water moves eastward, it becomes more saline and oligotrophic, which means there is less organic matter and fewer nutrients to support abundance (D'Ortenzio and Ribera d'Alcalà, 2009). The highest number of models on alien species was found for Mediterranean Sea regions, and especially the eastern Mediterranean Sea, an outcome due to the nature of the basin that is one of the most invaded seas in the world (Giakoumi et al., 2019). As expected, upwelling and eutrophic ecosystems (i.e., Bay of Biscay, Celtic Sea, Iberian coast and North Sea) showed the highest total primary production. A similar finding was observed in total catches of these areas, with systems connected to the NE Atlantic Ocean exhibiting the greatest values. However, the Barents Sea represents an exception compared to other North Atlantic ecosystems due to a combination of factors, including lower species diversity, limited accessibility, shorter fishing season due to the sea ice, and underestimations, since unreported landings as well as discards miscalculations are very common (Popov and Zeller, 2018). The waters of NE Atlantic have upwelling characteristics, encouraging the reproduction of small pelagic fishes and the creation of large shoals that drive high fisheries yields and catch (Bjørndal, 2009; Feijó et al., 2018). The Mean Trophic Level of the Catch (MTLc) was highest in the Barents Sea (>4) and generally higher in high latitude regions. These findings are corroborated by the literature on large boreal ecosystems and may be explained by the abundance of large marine predators and elasmobranchs in the catch (Conti and Scardi, 2010). A same pattern was observed in lower latitude ecosystems; for instance, the oligotrophic eastern Mediterranean Sea has intermediate total yields associated with the highest average MTLc in the basin. However, high biomasses of top predators have not been observed in the eastern Mediterranean Sea compared to the central and western parts of the basin, suggesting the MTLc score in the east can be attributed to lower productivity and density of low trophic level organisms (e.g., small pelagics) in the catch (Tsagarakis et al., 2010).
The complexity of EwE models has increased along time, with a higher number of compartments found in most recent works compared to the oldest. Nevertheless, the number of FGs in most of the models ranged from 30 to 50 (Stock et al., 2023), an aspect that acknowledges the challenges posed by increasing model complexity (Green et al., 2005) and software tools’ limitations to achieve mass-balance conditions (Villasante et al., 2016). Ecosim and Ecospace are used to implement simulations in time and space, respectively. Their performance, however, depends on the choice of the forcing factors, which influences calibration and fitting procedures (Mackinson et al., 2009). The majority of the models examined in this review incorporated trophic (vulnerabilities), fishery and primary production drivers, due to the automated fitting procedure available in EwE that includes these factors (Scott et al., 2016). Regarding the environmental drivers, most of the models incorporated temperature (e.g., SST, SBT), as most taxa have a strong connection between temperature and metabolic rates such as growth and consumption (Chabot et al., 2016). Also temperature data are the most easily accessible environmental data (Assis et al., 2018), however they play a crucial role in investigating the effects of climate change, confirming the ecosystem-level relevance of ocean warming, especially at higher latitudes (Grimm et al., 2013).
An issue related to the ecosystem indicators and the development of food web models like EwE is the uncertainty analysis (Evans et al., 2013). Ecosystem models are based on three principles (Levins, 1966): (1) generality (the tolerance limits of modelling applications); (2) realism (the degree of correspondence to reality); and (3) accuracy (the level of uncertainty). The latter is an integral part of complex ecosystem modelling (Milner-Gulland and Shea, 2017) and is considered in Ecopath, through the pedigree routine (Christensen and Walters, 2004a) to assess the integrity of input parameters and the quality of the model. However, the pedigree routine is not used by many models (Colléter et al., 2015). Understanding and addressing model uncertainty is the key to implement management scenarios and supporting robust decision making (Refsgaard et al., 2007). According to Rounsevell et al. (2021), uncertainty is divided in three categories: (1) scenario-based, including linguistic variations, alternative forms of implementation and input parameters interpretation; (2) model-based, encompassing structural limitations, input data reliability and error propagation; and (3) decision making-based, involving data interpretation, communication and translation of results. A newer approach to assess uncertainty in EwE was attained with the development of the Ecosampler plug-in (Steenbeek et al., 2018). Ecosampler is based on a Monte Carlo method (Metropolis and Ulam, 1949), built in EwE for automated input parameter optimization (Kavanagh et al., 2004). The Monte Carlo routine scans potential parameters that can ensure preserving the mass-balanced state of the ecosystem, and Ecosampler stores these plausible sets of models in its repository (Steenbeek et al., 2018). However, only 30% of the reviewed publications used the Monte Carlo routine and its results to perform uncertainty analysis on input parameters. Despite having its own limitations (e.g., modification of the base model may lead to invalid stored samples), Ecosampler is an add-on option that aims to account for uncertainty. Although uncertainty is often considered a threat, it can improve predictions obtained from ecological models (Larrosa et al., 2016; Rounsevell et al., 2021) and represents an essential condition for using EwE models to inform and support ecosystem-based management (Heymans et al., 2016).
Model validation, also known as skill assessment, is an essential step in evaluating the performance of coupled trophic/physical models, including EwE models (Stow et al., 2009). As a rule of thumb, some steps can be followed to achieve the best possible fit (Heymans et al., 2016). Ecopath input parameters, including data on biomass and catch, can be cross-checked with those from stock assessments and other sources (i.e., acoustic surveys, trawling samplings), enhancing model credibility (Agnetta et al., 2019; Natugonza et al., 2020). The PREBAL routine incorporated on the EwE software tool ensures a diagnostic check in which biomass and trophic flows are calculated for each group in the food web by balancing the input and output (Link, 2010). In the same way, Ecosim calibration is an essential step in Ecosim modelling to verify that the model accurately represents the temporal dynamics of the food web (Haputhantri et al., 2008; Tomczak et al., 2012). The stepwise fitting procedure is an automated routine for vulnerabilities and primary production drivers, which can be used as a skill assessment metric, based on an optimization algorithm (Mackinson et al., 2009; Scott et al., 2016). Ecospace has a multi-frame nature, as it is designed to capture the interactions between multiple species, fisheries, and the environment across a spatially-explicit domain. Model inter-comparison with other species distribution models (SDMs), like generalised linear models (GLMs), generalised additive models (GAMs) or boosted regression trees (BRTs) and cross-validation (Roberts et al., 2017) are skill assessment metrics used to achieve Ecospace modelling validation (Püts et al., 2020; Steenbeek et al., 2021).Simulating complex marine ecosystems and the impact of stressors acting on them is a challenging feat required to enhance EBFM. Despite existing difficulties in modelling ecosystems, this review reported a marked increase of EwE models in recent years, in all European marine areas. The increase in modelling efforts highlights the embracing of the holistic ecosystem management approach, primarily in vulnerable systems where single-species assessments and management alone have proven to be inadequate (Skern-Mauritzen et al., 2016). According to Craig and Link (2023), EwE modelling approach can support EBFM by following certain criteria, including (1) a clear management objective, (2) an accessible and well-constructed model, (3) a communication channel among scientists and decision-making bodies and (4) a versatile modelling approach (i.e., software modules and addressed topics). Other reviews have also assessed ecosystem models and applications globally, regarding the definition of the best suitable modelling approach according to ecosystems’ characteristics and issues (Geary et al., 2020; Perryman et al., 2021). EwE is a successful modelling approach allowing to describe the whole food web state. It enables simulating food web changes in time and space, implementing forecast scenarios that can support management decisions. In the future, other reviews of EwE models, indicators and statistics could be implemented to inform about geographical gaps (e.g., areas with no existing models), possibly assisting global meta-analyses and identification of emerging ecosystem properties.
Author contributions
IK and AT conceived the study. IK collected the data, performed the meta-analysis and wrote the manuscript. DD visualized the graphic illustrations. EO, MS and GG reviewed the manuscript. AT supervised the manuscript and acquired funding. All authors contributed to the article and approved the submitted version.
Funding
The present work was supported by the H2020 funded project “EcoScope” (Contract No. 101000302). MS was also supported by the German Federal Agency for Nature Conservation (Bundesamt für Naturschutz, BfN) with funds from the Federal Ministry of the Environment, Nature Conservation and Nuclear Safety (BMU), under the grant agreement FKZ: 3521532201. MS is thankful to the balt_ADAPT project (Adaptation of the Western Baltic Coastal Fishery to Climate Change, grant no. 03F0863), which received funding from the German Federal Ministry of Education and Research (BMBF).
Conflict of interest
The authors declare that the research was conducted in the absence of any commercial or financial relationships that could be construed as a potential conflict of interest.
Publisher’s note
All claims expressed in this article are solely those of the authors and do not necessarily represent those of their affiliated organizations, or those of the publisher, the editors and the reviewers. Any product that may be evaluated in this article, or claim that may be made by its manufacturer, is not guaranteed or endorsed by the publisher.
Supplementary material
The Supplementary Material for this article can be found online at: https://www.frontiersin.org/articles/10.3389/fmars.2023.1182921/full#supplementary-material
Footnotes
- ^ Ofir, E., Corrales, X., Coll, M., Heymans, J. J., Goren, M., Steenbeek, J., et al. (under review). Evaluation of fisheries management policies in the alien species-rich Eastern Mediterranean under climate change. Front. Mar. Sci.
References
Abdou K., Halouani G., Hattab T., Romdhane M. S., Ben Rais Lasram F., Le Loc’h F. (2016). Exploring the potential effects of marine protected areas on the ecosystem structure of the gulf of gabes using the ecospace model. Aquat. Living Resour. 29 (2), 202. doi: 10.1051/alr/2016014
Abdou K., Le Loc’h F., Gascuel D., Romdhane M. S., Aubin J., Ben Rais Lasram F. (2020). Combining ecosystem indicators and life cycle assessment for environmental assessment of demersal trawling in Tunisia. Int. J. Life Cycle Assess. 25, 105–119. doi: 10.1007/s11367-019-01651-5
Agnetta D., Badalamenti F., Colloca F., D’ Anna G., Di Lorenzo G., Fiorentino F., et al. (2019). Benthic-pelagic coupling mediates interactions in Mediterranean mixed fisheries: an ecosystem modeling approach. PloS One 14 (1), e0210659. doi: 10.1371/journal.pone.0210659
Akbari N., Bjørndal T., Failler P., Forse A., Taylor M. H., Drakeford B. (2022). A multi-criteria framework for the sustainable management of fisheries: a case study of UK’s north Sea Scottish fisheries. Environ. Manage. 70, 79–96. doi: 10.1007/s00267-022-01607-w
Akoglu E., Salihoglu B., Libralato S., Oguz T., Solidoro C. (2014). An indicator-based evaluation of black Sea food web dynamics during 1960–2000. J. Mar. Syst. 134, 113–125. doi: 10.1016/j.jmarsys.2014.02.010
Albouy C., Mouillot D., Rocklin D., Culioli J. M., Le Loc’h F. (2010). Simulation of the combined effects of artisanal and recreational fisheries on a Mediterranean MPA ecosystem using a trophic model. Mar. Ecol. Prog. Ser. 412, 207–221. doi: 10.3354/meps08679
Albouy C., Velez L., Coll M., Colloca F., Le Loc’h F., Mouillot D., et al. (2013). From projected species distribution to food-web structure under climate change. Global Change Biol. 20 (3), 730–741. doi: 10.1111/gcb.12467
Alexander K. A., Heymans J. J., Magill S., Tomczak M. T., Holmes S. J., Wilding T. A. (2015). Investigating the recent decline in gadoid stocks in the west of Scotland shelf ecosystem using a food web model. ICES J. Mar. Sci. 72 (2), 436–449. doi: 10.1093/icesjms/fsu149
Alexander K. A., Meyjes S. A., Heymans J. J. (2016). Spatial ecosystem modelling of marine renewable energy installations: gauging the utility of ecospace. Ecol. Model. 331, 115–128. doi: 10.1016/j.ecolmodel.2016.01.016
Araújo J. N., Mackinson S., Ellis J. R., Hart P. J. B. (2005). An ecopath model of the western English channel ecosystem with an exploration of its dynamic properties. Lowestoft: CEFAS Cefas Sci. Ser. Tech. Rep. 125, 45.
Araújo J. N., Mackinson S., Stanford R. J., Hart P. J. B. (2008). Exploring fisheries strategies for the western English channel using an ecosystem model. Ecol. Model. 210, 465–477. doi: 10.1016/j.ecolmodel.2007.08.015
Araújo J. N., Mackinson S., Stanford R. J., Sims D. W., Southward A. J., Hawkins S. J., et al. (2006). Modelling food web interactions, variation in plankton production, and fisheries in the western English channel ecosystem. Mar. Ecol. Prog. Ser. 309, 175–187. doi: 10.3354/meps309175
Arbach Leloup F., Desroy N., Le Mao P., Pauly D., Le Pape O. (2008). Interactions between a natural food web, shellfish farming and exotic species: the case of the bay of mont saint Michel (France). Estuarine Coast. Shelf Sci. 76, 111–120. doi: 10.1016/j.ecss.2007.06.011
Armoškaitė A., Puriņa I., Aigars J., Strāķe S., Pakalniete K., Frederiksen P., et al. (2020). Establishing the links between marine ecosystem components, functions and services: an ecosystem service assessment tool. Ocean Coast. Manage. 193, 105229. doi: 10.1016/j.ocecoaman.2020.105229
Assis J., Tyberghein L., Bosch S., Verbruggen H., Serrão E. A., De Clerck O. (2018). Bio-ORACLE v2.0: extending marine data layers for bioclimatic modelling. Global Ecol. Biogeogr. 27 (3), 277–284. doi: 10.1111/geb.12693
Baeta A., Niquil N., Marques J. C., Patrício J. (2011). Modelling the effects of eutrophication, mitigation measures and an extreme flood event on estuarine benthic food webs. Ecol. Model. 222 (6), 1209–1221. doi: 10.1016/j.ecolmodel.2010.12.010
Bănaru D., Mellon-Duval C., Roos D., Bigot J. L., Souplet A., Jadaud A., et al. (2013). Trophic structure in the gulf of lions marine ecosystem (north-western Mediterranean Sea) and fishing impacts. J. Mar. Syst. 111-112, 45–68. doi: 10.1016/j.jmarsys.2012.09.010
Barale V. (2008). “The European marginal and enclosed seas: an overview” in: Remote sensing of the European Seas. Eds. Barale V., Gade M. (Dordrecht: Springer), 463–474.
Barausse A., Duci A., Mazzoldi C., Artioli Y., Palmeri L. (2009). Trophic network model of the northern Adriatic Sea: analysis of an exploited and eutrophic ecosystem. Estuarine Coast. Shelf Sci. 83 (4), 577–590. doi: 10.1016/j.ecss.2009.05.003
Baudron A. R., Serpetti N., Fallon N. G., Heymans J. J., Fernandes P. G. (2019). Can the common fisheries policy achieve good environmental status in exploited ecosystems: the west of Scotland demersal fisheries example. Fish. Res. 211, 217–230. doi: 10.1016/j.fishres.2018.10.024
Bauer B., Gustafsson B. G., Hyytiäinen K., Meier H. E. M., Müller-Karulis B., Saraiva S., et al. (2019). Food web and fisheries in the future Baltic Sea. Ambio 48, 1337–1349. doi: 10.1007/s13280-019-01229-3
Bauer B., Meier H. E. M., Casini M., Hoff A., Margoński P., Orio A., et al. (2018). Reducing eutrophication increases spatial extent of communities supporting commercial fisheries: a model case study. ICES J. Mar. Sci. 75 (4), 1306–1317. doi: 10.1093/icesjms/fsy003
Bayle-Sempere J. T., Arreguín-Sánchez F., Sanchez-Jerez P., Salcido-Guevara L. A., Fernandez-Jover D., Zetina-Rejón M. J. (2013). Trophic structure and energy fluxes around a Mediterranean fish farm. Ecol. Model. 248, 135–147. doi: 10.1016/j.ecolmodel.2012.08.028
Beattie A., Sumaila U. R., Christensen V., Pauly D. (2002). A model for the bioeconomic evaluation of marine protected area size and placement in the north Sea. Natural Res. Model. 15 (4), 413–437. doi: 10.1111/j.1939-7445.2002.tb00096.x
Bentley J. W., Hines J. W., Borrett S., Serpetti N., Fox C., Reid D. G., et al. (2019a). Diet uncertainty analysis strengthens model-derived indicators of food web structure and function. Ecol. Indic. 98, 239–250. doi: 10.1016/j.ecolind.2018.11.008
Bentley J. W., Hines D. E., Borrett S. R., Serpetti N., Hernandez-Milian G., Fox C., et al. (2019c). Combining scientific and fishers’ knowledge to co-create indicators of food web structure and function. ICES J. Mar. Syst. 76 (7), 2218–2234. doi: 10.1093/icesjms/fsz121
Bentley J. W., Serpetti N., Fox C., Heymans J. J., Reid D. G. (2019b). Fishers’ knowledge improves the accuracy of food web model predictions. ICES J. Mar. Sci. 76 (4), 897–912. doi: 10.1093/icesjms/fsz003
Bentley J. W., Serpetti N., Fox C. J., Heymans J. J., Reid D. G. (2020). Retrospective analysis of the influence of environmental drivers on commercial stocks and fishing opportunities in the Irish Sea. Fish. Oceanogr. 29 (5), 415–435. doi: 10.1111/fog.12486
Bentley J. W., Serpetti N., Heymans J. J. (2017). Investigating the potential impacts of ocean warming on the Norwegian and barents seas ecosystem using a time-dynamic food-web model. Ecol. Model. 360, 94–107. doi: 10.1016/j.ecolmodel.2017.07.002
Bentorcha A., Gascuel D., Guénette S. (2017). Using trophic models to assess the impact of fishing in the bay of Biscay and the celtic Sea. Aquat. Living Resour. 30, 7. doi: 10.1051/alr/2017006
Bjørndal T. (2009). Overview, roles, and performance of the north East Atlantic fisheries commission (NEAFC). Mar. Policy 33 (4), 685–697. doi: 10.1016/j.marpol.2009.01.007
Blanchard J. L., Pinnegar J. K., Mackinson S. (2002). Exploring marine mammal-fishery interactions using ‘Ecopath with ecosim’: modelling the barents Sea ecosystem. Lowestoft: CEFAS Cefas Sci. Ser. Tech. Rep. 117, 52.
Bodini A., Rocchi M., Scotti M. (2018). Insights into the ecology of the black Sea through the qualitative loop analysis of the community structure. Limnol. Oceanogr. 63 (2), 968–984. doi: 10.1002/lno.10713
Borja A., Fontán A., Sáenz J., Valencia V. (2008). Climate, oceanography, and recruitment: the case of the bay of Biscay anchovy (Engraulis encrasicolus). Fish. Oceanogr. 17 (6), 477–493. doi: 10.1111/j.1365-2419.2008.00494.x
Borja A., Prins T. C., Simboura N., Andersen J. H., Berg T., Marques J.-C., et al. (2014). Tales from a thousand and one ways to integrate marine ecosystem components when assessing the environmental status. Front. Mar. Sci. 1, 72. doi: 10.3389/fmars.2014.00072
Bourdaud P., Ben Rais Lasram F., Araignous E., Champagnat J., Grusd S., Halouani G., et al. (2021). Impacts of climate change on the bay of seine ecosystem: forcing a spatio-temporal trophic model with predictions from an ecological niche model. Fish. Oceanogr. 30 (5), 471–489. doi: 10.1111/fog.12531
Bourdaud P., Gascuel D., Bentorcha A., Brind’Amour A. (2016). New trophic indicators and target values for an ecosystem-based management of fisheries. Ecol. Indic. 61 (2), 588–601. doi: 10.1016/j.ecolind.2015.10.010
Bruno J. F., Bates A. E., Cacciapaglia C., Pike E. P., Amstrup S. C., Van Hooidonk R., et al. (2018). Climate change threatens the world’s marine protected areas. Nat. Climate Change 8, 499–503. doi: 10.1038/s41558-018-0149-2
Bueno-Pardo J., García-Seoane E., Sousa A. I., Coelho J. P., Morgado M., Frankenbach S., et al. (2018). Trophic web structure and ecosystem attributes of a temperate coastal lagoon (Ria de aveiro, Portugal). Ecol. Model. 378, 13–25. doi: 10.1016/j.ecolmodel.2018.03.009
Carlucci R., Capezzuto F., Cipriano G., D’ Onghia G., Fanizza C., Libralato S., et al. (2021). Assessment of cetacean–fishery interactions in the marine food web of the gulf of taranto (Northern Ionian Sea, central Mediterranean Sea). Rev. Fish Biol. Fish. 31, 135–156. doi: 10.1007/s11160-020-09623-x
Celić I., Libralato S., Scarcella G., Raicevich S., Marčeta B., Solidoro C. (2018). Ecological and economic effects of the landing obligation evaluated using a quantitative ecosystem approach: a Mediterranean case study. ICES J. Mar. Sci. 75 (6), 1992–2003. doi: 10.1093/icesjms/fsy069
Chabot D., Steffensen J. F., Farrell A. P. (2016). The determination of standard metabolic rate in fishes. J. Fish Biol. 88 (1), 81–121. doi: 10.1111/jfb.12845
Christensen V. (1995a). A model of trophic interactions in the north Sea in 1981, the year of the stomach. Dana 11 (1), 1–28.
Christensen V. (1995b). Ecosystem maturity - towards quantification. Ecol. Model. 77 (1), 3–32. doi: 10.1016/0304-3800(93)E0073-C
Christensen V., Ferdaña Z., Steenbeek J. (2009). Spatial optimization of protected area placement incorporating ecological, social and economical criteria. Ecol. Model. 220 (19), 2583–2593. doi: 10.1016/j.ecolmodel.2009.06.029
Christensen V., Steenbeek J., Failler P. (2011). A combined ecosystem and value chain modeling approach for evaluating societal cost and benefit of fishing. Ecol. Model. 222 (3), 857–864. doi: 10.1016/j.ecolmodel.2010.09.030
Christensen V., Walters C. J. (2004a). Ecopath with ecosim: methods, capabilities and limitations. Ecol. Model. 172 (2-4), 109–139. doi: 10.1016/j.ecolmodel.2003.09.003
Christensen V., Walters C. J. (2004b). Trade-offs in ecosystem-scale optimization of fisheries management policies. Bull. Mar. Sci. 74 (3), 549–562.
Coll M., Akoglu E., Arreguín-Sánchez F., Fulton E. A., Gascuel D., Heymans J. J., et al. (2015). Modelling dynamic ecosystems: venturing beyond boundaries with the ecopath approach. Rev. Fish Biol. Fish. 25, 413–424. doi: 10.1007/s11160-015-9386-x
Coll M., Bahamon N., Sardà F., Palomera I., Tudela S., Suuronen P. (2008b). Improved trawl selectivity: effects on the ecosystem in the south Catalan Sea (NW Mediterranean). Mar. Ecol. Prog. Ser. 355, 131–147. doi: 10.3354/meps07183
Coll M., Libralato S. (2012). Contributions of food web modelling to the ecosystem approach to marine resource management in the Mediterranean Sea. Fish Fish. 13 (1), 60–88. doi: 10.1111/j.1467-2979.2011.00420.x
Coll M., Navarro J., Olson R. J., Christensen V. (2012). Assessing the trophic position and ecological role of squids in marine ecosystems by means of food-web models. Deep-Sea Res. II 95, 21–36. doi: 10.1016/j.dsr2.2012.08.020
Coll M., Navarro J., Palomera I. (2013). Ecological role, fishing impact, and management options for the recovery of a Mediterranean endemic skate by means of food web models. Biol. Conserv. 157, 108–120. doi: 10.1016/j.biocon.2012.06.029
Coll M., Palomera I., Tudela S. (2009b). Decadal changes in a NW Mediterranean Sea food web in relation to fishing exploitation. Ecol. Model. 220 (17), 2088–2102. doi: 10.1016/j.ecolmodel.2009.04.049
Coll M., Palomera I., Tudela S., Dowd M. (2008a). Food-web dynamics in the south Catalan Sea ecosystem (NW Mediterranean) for 1978–2003. Ecol. Model. 217, 95–116. doi: 10.1016/j.ecolmodel.2008.06.013
Coll M., Palomera I., Tudela S., Sardà F. (2006a). Trophic flows, ecosystem structure and fishing impacts in the south Catalan Sea, northwestern Mediterranean. J. Mar. Syst. 59, 63–96. doi: 10.1016/j.jmarsys.2005.09.001
Coll M., Santojanni A., Palomera I., Arneri E. (2009a). Food-web changes in the Adriatic Sea over the last three decades. Mar. Ecol. Prog. Ser. 381, 17–37. doi: 10.3354/meps07944
Coll M., Santojanni A., Palomera I., Arneri E. (2010). Ecosystem assessment of the north-central Adriatic Sea: towards a multivariate reference framework. Mar. Ecol. Prog. Ser. 417, 193–210. doi: 10.3354/meps08800
Coll M., Santojanni A., Palomera I., Tudela S., Arneri E. (2007). An ecological model of the northern and central Adriatic Sea: analysis of ecosystem structure and fishing impacts. J. Mar. Syst. 67, 119–154. doi: 10.1016/j.jmarsys.2006.10.002
Coll M., Shannon L. J., Kleisner K. M., Juan-Jordá M. J., Bundy A., Akoglu A. G., et al. (2016b). Ecological indicators to capture the effects of fishing on biodiversity and conservation status of marine ecosystems. Ecol. Indic. 60, 947–962. doi: 10.1016/j.ecolind.2015.08.048
Coll M., Shannon L. J., Moloney C. L., Palomera I., Tudela S. (2006b). Comparing trophic flows and fishing impacts of a NW Mediterranean ecosystem with coastal upwelling systems by means of standardized models and indicators. Ecol. Model. 198, 53–70. doi: 10.1016/j.ecolmodel.2006.04.009
Coll M., Steenbeek J. (2017). Standardized ecological indicators to assess aquatic food webs: the ECOIND software plug-in for ecopath with ecosim models. Environ. Model. Softw. 89, 120–130. doi: 10.1016/j.envsoft.2016.12.004
Coll M., Steenbeek J., Sole J., Palomera I., Christensen V. (2016a). Modelling the cumulative spatial–temporal effects of environmental drivers and fishing in a NW Mediterranean marine ecosystem. Ecol. Model. 331, 100–114. doi: 10.1016/j.ecolmodel.2016.03.020
Colléter M., Valls A., Guitton J., Gascuel D., Pauly D., Christensen V. (2015). Global overview of the applications of the ecopath with ecosim modelling approach using the EcoBase models repository. Ecol. Model. 302, 42–53. doi: 10.1016/j.ecolmodel.2015.01.025
Collie J. S., Botsford L. W., Hastings A., Kaplan I. C., Largier J. L., Livingston P. A., et al. (2016). Ecosystem models for fisheries management: finding the sweet spot. Fish Fish. 17, 101–125. doi: 10.1111/faf.12093
Conti L., Scardi M. (2010). Fisheries yield and primary productivity in large marine ecosystems. Mar. Ecol. Prog. Ser. 410, 233–244. doi: 10.3354/meps08630
Corrales X., Coll M., Ofir E., Heymans J. J., Steenbeek J., Goren M., et al. (2018). Future scenarios of marine resources and ecosystem conditions in the Eastern Mediterranean under the impacts of fishing, alien species and sea warming. Sci. Rep. 8, 14284. doi: 10.1038/s41598-018-32666-x
Corrales X., Coll M., Ofir E., Piroddi C., Goren M., Edelist D., et al. (2017b). Hindcasting the dynamics of an Eastern Mediterranean marine ecosystem under the impacts of multiple stressors. Mar. Ecol. Prog. Ser. 580, 17–36. doi: 10.3354/meps12271
Corrales X., Coll M., Tecchio S., Bellido J. M. (2015). Ecosystem structure and fishing impacts in the northwestern Mediterranean Sea using a food web model within a comparative approach. J. Mar. Syst. 148, 183–199. doi: 10.1016/j.jmarsys.2015.03.006
Corrales X., Katsanevakis S., Coll M., Heymans J. J., Piroddi C., Ofir E., et al. (2020a). Advances and challenges in modelling the impacts of invasive alien species on aquatic ecosystems. Biol. Invasions 22, 907–934. doi: 10.1007/s10530-019-02160-0
Corrales X., Ofir E., Coll M., Goren M., Edelist D., Heymans J. J., et al. (2017a). Modeling the role and impact of alien species and fisheries on the Israeli marine continental shelf ecosystem. J. Mar. Syst. 170, 88–102. doi: 10.1016/j.jmarsys.2017.02.004
Corrales X., Preciado I., Gascuel D., Lopez de Gamiz-Zearra A., Hernvann P.-Y., Mugerza E., et al. (2022). Structure and functioning of the bay of Biscay ecosystem: a trophic modelling approach. Estuarine Coast. Shelf Sci. 264, 107658. doi: 10.1016/j.ecss.2021.107658
Corrales X., Vilas D., Piroddi C., Steenbeek J., Claudet J., Lloret J., et al. (2020b). Multi-zone marine protected areas assessment of ecosystem and fisheries benefits using multiple ecosystem models. Ocean Coast. Manage. 193, 105232. doi: 10.1016/j.ocecoaman.2020.105232
Costalago D., Bauer B., Tomczak M. T., Lundström K., Winder M. (2019). The necessity of a holistic approach when managing marine mammal–fisheries interactions: environment and fisheries impact are stronger than seal predation. Ambio 48, 552–564. doi: 10.1007/s13280-018-1131-y
Costello M. J., Bouchet P., Emblow C. S., Legakis A. (2006). European Marine biodiversity inventory and taxonomic resources: state of the art and gaps in knowledge. Mar. Ecol. Prog. Ser. 316, 257–268. doi: 10.3354/meps316257
Craig J. K., Link J. S. (2023). It is past time to use ecosystem models tactically to support ecosystem-based fisheries management: case studies using ecopath with ecosim in an operational management context. Fish Fish. 00, 1–26. doi: 10.1111/faf.12733
D'Ortenzio F., Ribera d'Alcalà M. (2009). On the trophic regimes of the Mediterranean Sea: a satellite analysis. Biogeosciences 6, 139–148. doi: 10.5194/bg-6-139-2009
Dahood A., de Mutsert K., Watters G. M. (2020). Evaluating Antarctic marine protected area scenarios using a dynamic food web model. Biol. Conserv. 251, 108766. doi: 10.1016/j.biocon.2020.108766
Daskalov G. M. (2002). Overfishing drives a trophic cascade in the black Sea. Mar. Ecol. Prog. Ser. 225, 53–63. doi: 10.3354/meps225053
Daskalov G. M., Mackinson S., Mulligan B. (2011). Modelling possible food-web effects of aggregate dredging in the eastern English channel. Mar. Environ. Prot. Fund (MEPF). Ref No: MEPF. 8, 37.
Díaz López B., Bunke M., Bernal Shirai J. A. (2008). Marine aquaculture off Sardinia island (Italy): ecosystem effects evaluated through a trophic mass-balance model. Ecol. Model. 212 (3-4), 292–303. doi: 10.1016/j.ecolmodel.2007.10.028
Dimarchopoulou D., Keramidas I., Tsagarakis K., Tsikliras A. C. (2019). Ecosystem models and effort simulations of an untrawled gulf in the central Aegean Sea. Front. Mar. Sci. 6, 648. doi: 10.3389/fmars.2019.00648
Dimarchopoulou D., Tsagarakis K., Sylaios G., Tsikliras A. C. (2022). Ecosystem trophic structure and fishing effort simulations of a major fishing ground in the northeastern Mediterranean Sea (Thermaikos gulf). Estuarine Coast. Shelf Sci. 264, 107667. doi: 10.1016/j.ecss.2021.107667
Dobrzycka-Krahel A., Medina-Villar S. (2020). Alien species of Mediterranean origin in the Baltic Sea region: current state and risk assessment. Environ. Rev. 28 (3), 339–356. doi: 10.1139/er-2019-0074
Dommasnes A., Christensen V., Ellertsen B., Kvamme C., Melle W., Nøttestad L., et al. (2001). An ecopath model for the Norwegian Sea and barents Sea. Fish. Impacts North Atlantic Ecosys.: Models Anal. 9 (4), 213–240.
Edelist D., Rilov G., Golani D., Carlton J. T., Spanier E. (2013). Restructuring the Sea: profound shifts in the world's most invaded marine ecosystem. Diversity Distributions 19 (1), 69–77. doi: 10.1111/ddi.12002
Elliott M., Borja Á, McQuatters-Gollop A., Mazik K., Birchenough S., Andersen J. H., et al. (2015). Force majeure: will climate change affect our ability to attain good environmental status for marine biodiversity? Mar. pollut. Bull. 95 (1), 7–27. doi: 10.1016/j.marpolbul.2015.03.015
EU (2008). Directive 2008/56/EC of the European parliament and of the council establishing a framework for community action in the field of marine environmental policy (Marine strategy framework directive). Off. J. Eur. Union 164, 9–40.
EU (2013). Regulation (EU) no 1380/2013 of the European parliament and of the council of 11 December 2013 on the common fisheries policy, amending council regulations (EC) no 1954/2003 and (EC) no 1224/2009 and repealing council regulations (EC) no 2371/2002 and (EC) no 639/2004 and council decision 2004/585/EC. Off. J. Eur. Union 354, 22–61.
Evans M. R., Grimm V., Johst K., Knuuttila T., de Langhe R., Lessells C. M., et al. (2013). Do simple models lead to generality in ecology? Trends Ecol. Evol. 28 (10), 578–583. doi: 10.1016/j.tree.2013.05.022
Fabiano M., Vassallo P., Vezzulli L., Salvo V. S., Marques J. C. (2004). Temporal and spatial change of exergy and ascendency in different benthic marine ecosystems. Energy 29 (11), 1697–1712. doi: 10.1016/j.energy.2004.03.051
Fath B. D., Asmus H., Asmus R., Baird D., Borrett S. R., De Jonge V. N., et al. (2019). Ecological network analysis metrics: the need for an entire ecosystem approach in management and policy. Ocean Coast. Manage. 174, 1–14. doi: 10.1016/j.ocecoaman.2019.03.007
Fay G., Large S. I., Link J. S., Gamble R. J. (2013). Testing systemic fishing responses with ecosystem indicators. Ecol. Model. 265, 45–55. doi: 10.1016/j.ecolmodel.2013.05.016
Feijó D., Marçalo A., Bento T., Barra J., Marujo D., Correia M., et al. (2018). Trends in the activity pattern, fishing yields, catch and landing composition between 2009 and 2013 from onboard observations in the Portuguese purse seine fleet. Regional Stud. Mar. Sci. 23, 97–106. doi: 10.1016/j.rsma.2017.12.007
Finn J. T. (1976). Measures of ecosystem structure and function derived from analysis of flows. J. Theor. Biol. 56 (2), 363–380. doi: 10.1016/S0022-5193(76)80080-X
Forrestal F., Coll M., Die D. J., Christensen V. (2012). Ecosystem effects of bluefin tuna Thunnus thynnus thynnus aquaculture in the NW Mediterranean Sea. Mar. Ecol. Prog. Ser. 456, 215–231. doi: 10.3354/meps09700
Fouzai N., Coll M., Palomera I., Santojanni A., Arneri E., Christensen V. (2012). Fishing management scenarios to rebuild exploited resources and ecosystems of the northern-central Adriatic (Mediterranean Sea). J. Mar. Syst. 102-104, 39–51. doi: 10.1016/j.jmarsys.2012.05.003
Friedland K. D., Stock C., Drinkwater K. F., Link J. S., Leaf R. T., Shank B. V., et al. (2012). Pathways between primary production and fisheries yields of Large marine ecosystems. PloS One 7 (1), e28945. doi: 10.1371/journal.pone.0028945
Froese R., Winker H., Coro G., Demirel N., Tsikliras A. C., Dimarchopoulou D., et al. (2018). Status and rebuilding of European fisheries. Mar. Policy 93, 159–170. doi: 10.1016/j.marpol.2018.04.018
Fulton E. A., Smith A. D. M., Smith D. C., Johnson P. (2014). An integrated approach is needed for ecosystem based fisheries management: insights from ecosystem-level management strategy evaluation. PloS One 9 (1), e84242. doi: 10.1371/journal.pone.0084242
Galdies C., Bellerby R., Canu D., Chen W., Garcia-Luque E., Gašparović B., et al. (2020). European Policies and legislation targeting ocean acidification in european waters - current state. Mar. Policy 118, 103947. doi: 10.1016/j.marpol.2020.103947
Galil B. S., Marchini A., Occhipinti-Ambrogi A. (2018). East Is east and West is west? management of marine bioinvasions in the Mediterranean Sea. Estuarine Coast. Shelf Sci. 201, 7–16. doi: 10.1016/j.ecss.2015.12.021
Galparsoro I., Connor D. W., Borja Á, Aish A., Amorim P., Bajjouk T., et al. (2012). Using EUNIS habitat classification for benthic mapping in European seas: present concerns and future needs. Mar. pollut. Bull. 64 (12), 2630–2638. doi: 10.1016/j.marpolbul.2012.10.010
Gamito S., Erzini K. (2005). Trophic food web and ecosystem attributes of a water reservoir of the ria Formosa (south Portugal). Ecol. Model. 181 (4), 509–520. doi: 10.1016/j.ecolmodel.2004.02.024
García-Rodríguez E., Coll M., Vivas M., Bellido J. M., Esteban A., Torres M. A. (2021). A food-web comparative modeling approach highlights ecosystem singularities of the gulf of alicante (Western Mediterranean Sea). J. Sea Res. 174, 102073. doi: 10.1016/j.seares.2021.102073
Gascuel D., Pauly D. (2009). EcoTroph: modelling marine ecosystem functioning and impact of fishing. Ecol. Model. 220, 2885–2898. doi: 10.1016/j.ecolmodel.2009.07.031
Geary W. L., Bode M., Doherty T. S., Fulton E. A., Nimmo D. G., Tulloch A. I. T., et al. (2020). A guide to ecosystem models and their environmental applications. Nat. Ecol. Evol. 4, 1459–1471. doi: 10.1038/s41559-020-01298-8
Giakoumi S., Pey A., Di Franco A., Francour P., Kizilkaya Z., Arda Y., et al. (2019). Exploring the relationships between marine protected areas and invasive fish in the world's most invaded sea. Ecol. Appl. 29 (1), e1809. doi: 10.1002/eap.1809
Giralt Paradell O., Díaz López B., Methion S., Rogan E. (2020). Food-web interactions in a coastal ecosystem influenced by upwelling and terrestrial runoff off north-West Spain. Mar. Environ. Res. 157, 104933. doi: 10.1016/j.marenvres.2020.104933
Giralt Paradell O., Methion S., Rogan E., Díaz López B. (2021). Modelling ecosystem dynamics to assess the effect of coastal fisheries on cetacean species. J. Environ. Manage. 285, 112175. doi: 10.1016/j.jenvman.2021.112175
Glegg G., Jefferson R., Fletcher S. (2015). Marine governance in the English channel (La manche): linking science and management. Mar. pollut. Bull. 95 (2), 707–718. doi: 10.1016/j.marpolbul.2015.02.020
Green J. L., Hastings A., Arzberger P., Ayala F. J., Cottingham K. L., Cuddington K., et al. (2005). Complexity in ecology and conservation: mathematical, statistical, and computational challenges. BioScience 55 (6), 501–510. doi: 10.1641/0006-3568(2005)055[0501:CIEACM]2.0.CO;2
Grimm N. B., Chapin F. S. III, Bierwagen B., Gonzalez P., Groffman P. M., Luo Y., et al. (2013). The impacts of climate change on ecosystem structure and function. Front. Ecol. Environ. 11 (9), 474–482. doi: 10.1890/120282
Grossowicz M., Ofir E., Shabtay A., Wood J., Biton E., Belkin N., et al. (2020). Modeling the effects of brine outflow from desalination plants on coastal food-webs of the levantine basin (eastern Mediterranean Sea). Desalination 496, 114757. doi: 10.1016/j.desal.2020.114757
Gucu A. C. (2002). Can overfishing be responsible for the successful establishment of Mnemiopsis leidyi in the black Sea? Estuarine Coast. Shelf Sci. 54, 439–451. doi: 10.1006/ecss.2000.0657
Gudlaugsson S. T. (1993). Large Impact crater in the barents Sea. Geology 21 (4), 291–294. doi: 10.1130/0091-7613(1993)021<0291:LICITB>2.3.CO;2
Guénette S., Heymans J. J., Christensen V., Trites A. W. (2006). Ecosystem models show combined effects of fishing, predation, competition, and ocean productivity on steller sea lions (Eumetopias jubatus) in Alaska. Can. J. Fish. Aquat. Sci. 63 (11), 2495–2517. doi: 10.1139/f06-136
Guesnet V., Lassalle G., Chaalali A., Kearney K., Saint-Béat B., Karimi B., et al. (2015). Incorporating food-web parameter uncertainty into ecopath-derived ecological network indicators. Ecol. Model. 313, 29–40. doi: 10.1016/j.ecolmodel.2015.05.036
Halouani G., Abdou K., Hattab T., Romdhane M. S., Ben Rais Lasram F., Le Loc’h F. (2016). A spatio-temporal ecosystem model to simulate fishing management plans: a case of study in the gulf of gabes (Tunisia). Mar. Policy 69, 62–72. doi: 10.1016/j.marpol.2016.04.002
Halouani G., Gascuel D., Hattab T., Ben Rais Lasram F., Coll M., Tsagarakis K., et al. (2015). Fishing impact in Mediterranean ecosystems: an EcoTroph modeling approach. J. Mar. Syst. 150, 22–33. doi: 10.1016/j.jmarsys.2015.05.007
Halouani G., Le Loc’h F., Shin Y.-J., Velez L., Hattab T., Romdhane M. S., et al. (2019). An end-to-end model to evaluate the sensitivity of ecosystem indicators to track fishing impacts. Ecol. Indic. 98, 121–130. doi: 10.1016/j.ecolind.2018.10.061
Halouani G., Villanueva M. C., Raoux A., Dauvin J.-C., Ben Rais Lasram F., Foucher E., et al. (2020). A spatial food web model to investigate potential spillover effects of a fishery closure in an offshore wind farm. J. Mar. Syst. 212, 103434. doi: 10.1016/j.jmarsys.2020.103434
Haputhantri S. S. K., Villanueva M. C. S., Moreau J. (2008). Trophic interactions in the coastal ecosystem of Sri Lanka: an ECOPATH preliminary approach. Estuarine Coast. Shelf Sci. 76 (2), 304–318. doi: 10.1016/j.ecss.2007.07.013
Harvey C. J., Cox S. P., Essington T. E., Hansson S., Kitchell J. F. (2003). An ecosystem model of food web and fisheries interactions in the Baltic Sea. ICES J. Mar. Sci. 60, 939–950. doi: 10.1016/S1054-3139(03)00098-5
Hattab T., Ben Rais Lasram F., Albouy C., Romdhane M. S., Jarboui O., Halouani G., et al. (2013). An ecosystem model of an exploited southern Mediterranean shelf region (Gulf of gabes, Tunisia) and a comparison with other Mediterranean ecosystem model properties. J. Mar. Syst. 128, 159–174. doi: 10.1016/j.jmarsys.2013.04.017
Heiskanen A.-S., Berg T., Uusitalo L., Teixeira H., Bruhn A., Krause-Jensen D., et al. (2016). Biodiversity in marine ecosystems–European developments toward robust assessments. Front. Mar. Sci. 3, 184. doi: 10.3389/fmars.2016.00184
Hernvann P.-Y., Gascuel D., Grüss A., Druon J.-N., Kopp D., Perez I., et al. (2020). The celtic Sea trough time and space: ecosystem modeling to unravel fishing and climate change impacts on food-web structure and dynamics. Front. Mar. Sci. 7, 578717. doi: 10.3389/fmars.2020.578717
Heymans J. J., Coll M., Link J. S., Mackinson S., Steenbeek J., Walters C., et al. (2016). Best practice in ecopath with ecosim food-web models for ecosystem-based management. Ecol. Model. 331, 173–184. doi: 10.1016/j.ecolmodel.2015.12.007
Heymans J. J., Howell K. L., Ayers M., Burrows M. T., Gordon J. D. M., Jones E. G., et al. (2011b). Do we have enough information to apply the ecosystem approach to management of deep-sea fisheries? an example from the West of Scotland. ICES J. Mar. Sci. 68 (2), 265–280. doi: 10.1093/icesjms/fsq065
Heymans J. J., Mackinson S., Sumaila U. R., Dyck A., Little A. (2011a). The impact of subsidies on the ecological sustainability and future profits from north Sea fisheries. PloS One 6 (5), e20239. doi: 10.1371/journal.pone.0020239
Holt J., Allen J. I., Anderson T. R., Brewin R., Butenschön M., Harle J., et al. (2014). Challenges in integrative approaches to modelling the marine ecosystems of the north Atlantic: physics to fish and coasts to ocean. Prog. Oceanogr. 129 (B), 285–313. doi: 10.1016/j.pocean.2014.04.024
Howell D., Schueller A. M., Bentley J. W., Buchheister A., Chagaris D., Cieri M., et al. (2021). Combining ecosystem and single-species modeling to provide ecosystem-based fisheries management advice within current management systems. Front. Mar. Sci. 7, 607831. doi: 10.3389/fmars.2020.607831
Hunt G. L., Drinkwater K. F., Arrigo K., Berge J., Daly K. L., Danielson S., et al. (2016). Advection in polar and sub-polar environments: impacts on high latitude marine ecosystems. Prog. Oceanogr. 149, 40–81. doi: 10.1016/j.pocean.2016.10.004
Ito M., Halouani G., Cresson P., Giraldo C., Girardin R. (2023). Detection of fishing pressure using ecological network indicators derived from ecosystem models. Ecol. Indic. 147, 110011. doi: 10.1016/j.ecolind.2023.110011
Izquierdo-Gomez D., Bayle-Sempere J. T., Arreguín-Sánchez F., Sánchez-Jerez P. (2016). Modeling population dynamics and small-scale fisheries yields of fish farming escapes in Mediterranean coastal areas. Ecol. Model. 331, 56–67. doi: 10.1016/j.ecolmodel.2016.01.012
Jørgensen L. L., Pecuchet L., Ingvaldsen R. B., Primicerio R. (2022). Benthic transition zones in the Atlantic gateway to a changing Arctic ocean. Prog. Oceanogr. 204, 102792. doi: 10.1016/j.pocean.2022.102792
Johannesen E., Høines A. S., Dolgov A. V., Fossheim M. (2012). Demersal fish assemblages and spatial diversity patterns in the Arctic-Atlantic transition zone in the barents Sea. PloS One 7 (4), e34924. doi: 10.1371/journal.pone.0034924
Katsanevakis S., Zenetos A., Corsini-Foka M., Tsiamis K. (2020). “Biological Invasions in the Aegean Sea: Temporal Trends, Pathways, and Impacts” in: The Handbook of Environmental Chemistry. (Berlin, Heidelberg: Springer).
Kavanagh P., Newlands N., Christensen V., Pauly D. (2004). Automated parameter optimization for Ecopath ecosystem models. Ecol. Modell. 172 (2-4), 141–149. doi: 10.1016/j.ecolmodel.2003.09.004
Keramidas I., Dimarchopoulou D., Tsikliras A. C. (2022). Modelling and assessing the ecosystem of the Aegean Sea, a major hub of the eastern Mediterranean at the intersection of Europe and Asia. Regional Stud. Mar. Sci. 56, 102704. doi: 10.1016/j.rsma.2022.102704
Kideys A. E. (2002). Ecology: fall and rise of the black Sea ecosystem. Science 297 (5586), 1482–1484. doi: 10.1126/science.107300
Kjesbu O. S., Bogstad B., Devine J. A., Gjøsæter H., Howell D., Ingvaldsen R. B., et al. (2014). Synergies between climate and management for Atlantic cod fisheries at high latitudes. Proc. Natl. Acad. Sci. United States America 111 (9), 3478–3483. doi: 10.1073/pnas.1316342111
Korpinen S., Laamanen L., Bergström L., Nurmi M., Andersen J. H., Haapaniemi J., et al. (2021). Combined effects of human pressures on europe’s marine ecosystems. Ambio 50, 1325–1336. doi: 10.1007/s13280-020-01482-x
Korpinen S., Uusitalo L., Nordström M. C., Dierking J., Tomczak M. T., Haldin J., et al. (2022). Food web assessments in the Baltic Sea: models bridging the gap between indicators and policy needs. Ambio 51, 1687–1697. doi: 10.1007/s13280-021-01692-x
Kourafalou V. H. (1999). Process studies on the po river plume, north Adriatic Sea. J. Geophys. Res. 104 (C12), 29963–29985. doi: 10.1029/1999JC900217
Larrosa C., Carrasco L. R., Milner-Gulland E. J. (2016). Unintended feedbacks: challenges and opportunities for improving conservation effectiveness. Conserv. Lett. 9 (5), 316–326. doi: 10.1111/conl.12240
Larsen L.-H., Sagerup K., Ramsvatn S. (2016). The mussel path – using the contaminant tracer, ecotracer, in ecopath to model the spread of pollutants in an Arctic marine food web. Ecol. Model. 331, 77–85. doi: 10.1016/j.ecolmodel.2015.10.011
Lassalle G., Chouvelon T., Bustamante P., Niquil N. (2014). An assessment of the trophic structure of the bay of Biscay continental shelf food web: comparing estimates derived from an ecosystem model and isotopic data. Prog. Oceanogr. 120, 205–215. doi: 10.1016/j.pocean.2013.09.002
Lassalle G., Gascuel D., Le Loc’h F., Lobry J., Pierce G. J., Ridoux V., et al. (2012). An ecosystem approach for the assessment of fisheries impacts on marine top predators: the bay of Biscay case study. ICES J. Mar. Sci. 69 (6), 925–938. doi: 10.1093/icesjms/fss049
Lassalle G., Lobry J., Le Loc’h F., Bustamante P., Certain G., Delmas D., et al. (2011). Lower trophic levels and detrital biomass control the bay of Biscay continental shelf food web: implications for ecosystem management. Prog. Oceanogr. 91, 561–575. doi: 10.1016/j.pocean.2011.09.002
Lassen H., Pedersen S. A., Frost H., Hoff A. (2013). Fishery management advice with ecosystem considerations. ICES J. Mar. Sci. 70 (2), 471–479. doi: 10.1093/icesjms/fss208
Lavigne H., D’Ortenzio F., Ribera d'Alcalà M., Claustre H., Sauzède R., Gacic M. (2015). On the vertical distribution of the chlorophyll a concentration in the Mediterranean Sea: a basin-scale and seasonal approach. Biogeosciences 12, 5021–5039. doi: 10.5194/bg-12-5021-2015
Le Marchand M., Ben Rais Lasram F., Araignous E., Saint-Béat B., Lassalle G., Michelet N., et al. (2022). Potential combined impacts of climate change and non-indigenous species arrivals on bay of Biscay trophic network structure and functioning. J. Mar. Syst. 228, 103704. doi: 10.1016/j.jmarsys.2022.103704
Libralato S., Caccin A., Pranovi F. (2015). Modeling species invasions using thermal and trophic niche dynamic sunder climate change. Front. Mar. Sci. 2, 29. doi: 10.3389/fmars.2015.00029
Libralato S., Christensen V., Pauly D. (2006). A method for identifying keystone species in food web models. Ecol. Modell. 195 (3-4), 153–171. doi: 10.1016/j.ecolmodel.2005.11.029
Libralato S., Coll M., Tempesta M., Santojanni A., Spoto M., Palomera I., et al. (2010). Food-web traits of protected and exploited areas of the Adriatic Sea. Biol. Conserv. 143 (9), 2182–2194. doi: 10.1016/j.biocon.2010.06.002
Lindeman R. L. (1942). The trophic-dynamic aspect of ecology. Ecology 23, 399–418. doi: 10.2307/1930126
Link J. S. (2010). Adding rigor to ecological network models by evaluating a set of pre-balance diagnostics: a plea for PREBAL. Ecol. Model. 221 (12), 1580–1591. doi: 10.1016/j.ecolmodel.2010.03.012
Link J. S., Watson R. A. (2019). Global ecosystem overfishing: clear delineation within real limits to production. Sci. Adv. 5 (6), eaav047. doi: 10.1126/sciadv.aav0474
Livne L., Grossowicz M., Tchernov D., Ayalon O. (2020). Predicting impacts of offshore monoculture farm expansion in ultra-oligotrophic waters of the levantine basin. Front. Mar. Sci. 7, 556. doi: 10.3389/fmars.2020.00556
Lobry J., David V., Pasquaud S., Lepage M., Sautour B., Rochard E. (2008). Diversity and stability of an estuarine trophic network. Mar. Ecol. Prog. Ser. 358, 13–25. doi: 10.3354/meps07294
Long R. D., Charles A., Stephenson R. L. (2015). Key principles of marine ecosystem-based management. Mar. Policy 57, 53–60. doi: 10.1016/j.marpol.2015.01.013
Lorance P., Bertrand J. A., Brind'Amour A., Rochet M. J., Trenkel V. M. (2009). Assessment of impacts from human activities on ecosystem components in the bay of Biscay in the early 1990s. Aquat. Living Resour. 22 (4), 409–431. doi: 10.1051/alr/2009049
Lotze H. K., Tittensor D. P., Bryndum-Buchholz A., Eddy T. D., Cheung W. W. L., Galbraith E. D., et al. (2019). Global ensemble projections reveal trophic amplification of ocean biomass declines with climate change. Proc. Natl. Acad. Sci. United States America 116 (26), 12907–12912. doi: 10.1073/pnas.1900194116
Lüdmann T., Saitz Y. M., Metzing J., Emeis K.-C. (2021). Acoustic backscatter analysis of ground-fishing activity in the German north Sea sector. Continental Shelf Res. 212, 104292. doi: 10.1016/j.csr.2020.104292
Lykousis V., Chronis G., Tselepides A., Price N. B., Theocharis A., Siokou-Frangou I., et al. (2002). Major outputs of the recent multidisciplinary biogeochemical researches undertaken in the Aegean Sea. J. Mar. Syst. 33-34, 313–334. doi: 10.1016/S0924-7963(02)00064-7
Lynam C. P., Mackinson S. (2015). How will fisheries management measures contribute towards the attainment of good environmental status for the north Sea ecosystem? Global Ecol. Conserv. 4, 160–175. doi: 10.1016/j.gecco.2015.06.005
Mackinson S. (2014). Combined analyses reveal environmentally driven changes in the north Sea ecosystem and raise questions regarding what makes an ecosystem model’s performance credible? Can. J. Fish. Aquat. Sci. 71 (1), 31–46. doi: 10.1139/cjfas-2013-0173
Mackinson S., Daskalov G. (2007). An ecosystem model of the north Sea for use in research supporting the ecosystem approach to fisheries management: description and parameterisation. Lowestoft: CEFAS Cefas Sci. Ser. Tech. Rep. 142, 200.
Mackinson S., Daskalov G., Heymans J. J., Neira S., Arancibia H., Zetina-Rejón M., et al. (2009). Which forcing factors fit? using ecosystem models to investigate the relative influence of fishing and changes in primary productivity on the dynamics of marine ecosystems. Ecol. Model. 220 (21), 2972–2987. doi: 10.1016/j.ecolmodel.2008.10.021
Mackinson S., Platts M., Garcia C., Lynam C. (2018). Evaluating the fishery and ecological consequences of the proposed north Sea multi-annual plan. PloS One 13 (1), e0190015. doi: 10.1371/journal.pone.0190015
Marshall K. N., Koehn L. E., Levin P. S., Essington T. E., Jensen O. P. (2019). Inclusion of ecosystem information in US fish stock assessments suggests progress toward ecosystem-based fisheries management. ICES J. Mar. Sci. 76 (1), 1–9. doi: 10.1093/icesjms/fsy152
Marten G. G., Polovina J. J. (1982). “A comparative study of fish yields from various tropical ecosystems,” in ICLARM Conference Proceedings, Vol. 9, 255–289.
Matear L., Robbins J. R., Hale M., Potts J. (2019). Cetacean biodiversity in the bay of Biscay: suggestions for environmental protection derived from citizen science data. Mar. Policy 109, 103672. doi: 10.1016/j.marpol.2019.103672
Mattei F., Scardi M. (2022). Mining satellite data for extracting chlorophyll a spatio-temporal patterns in the Mediterranean Sea. Environ. Model. Softw. 150, 105353. doi: 10.1016/j.envsoft.2022.105353
Megrey B. A., Aydin K. Y. (2009). A macrodescriptor perspective of ecological attributes for the Bering and barents seas. Deep Sea Res. Part II: Topical Stud. Oceanogr. 56 (21-22), 2132–2140. doi: 10.1016/j.dsr2.2008.11.024
Metcalfe K., Vaz S., Engelhard G. H., Villanueva M. C., Smith R. J., Mackinson S. (2015). Evaluating conservation and fisheries management strategies by linking spatial prioritization software and ecosystem and fisheries modelling tools. J. Appl. Ecol. 52, 665–674. doi: 10.1111/1365-2664.12404
Metropolis N., Ulam S. (1949). The Monte Carlo method. J. Am. Stat. Assoc. 44 (247), 335–341. doi: 10.1080/01621459.1949.10483310
Michael-Bitton G., Gal G., Corrales X., Ofir E., Shechter M., Zemah-Samir S. (2022). Economic aspects of fish stock accounting as a renewable marine natural capital: the Eastern Mediterranean continental shelf ecosystem as a case study. Ecol. Econ. 200, 107539. doi: 10.1016/j.ecolecon.2022.107539
Michailidis N., Chartosia N., Katsanevakis S. (2023). Exploring the role of fishing in a heavily bioinvaded shelf ecosystem. Fish. Res. 259, 106554. doi: 10.1016/j.fishres.2022.106554
Michailidis N., Corrales X., Karachle P. K., Chartosia N., Katsanevakis S., Sfenthourakis S. (2019). Modelling the role of alien species and fisheries in an Eastern Mediterranean insular shelf ecosystem. Ocean Coast. Manage. 175, 152–171. doi: 10.1016/j.ocecoaman.2019.04.006
Milner-Gulland E. J., Shea K. (2017). Embracing uncertainty in applied ecology. J. Appl. Ecol. 54 (6), 2063–2068. doi: 10.1111/1365-2664.12887
Moher D., Liberati A., Tetzlaff J., Altman D. G., The PRISMA Group (2010). Preferred reporting items for systematic reviews and meta-analyses: the PRISMA statement. Int. J. Surg. 8 (5), 336–341. doi: 10.1016/j.ijsu.2010.02.007
Möllmann C., Cormon X., Funk S., Otto S. A., Schmidt J. O., Schwermer H., et al. (2021). Tipping point realized in cod fishery. Sci. Rep. 11, 14259. doi: 10.1038/s41598-021-93843-z
Morato T., Bulman C., Pitcher T. J. (2009). Modelled effects of primary and secondary production enhancement by seamounts on local fish stocks. Deep Sea Res. Part II: Topical Stud. Oceanogr. 56 (25), 2713–2719. doi: 10.1016/j.dsr2.2008.12.029
Morato T., Lemey E., Menezes G., Pham C. K., Brito J., Soszynski A., et al. (2016). Food web and ecosystem structure of the open-ocean and deep-sea environments of the Azores, NE Atlantic. Front. Mar. Sci. 3, 245. doi: 10.3389/fmars.2016.00245
Moullec F., Gascuel D., Bentorcha K., Guénette S., Robert M. (2017). Trophic models: what do we learn about celtic Sea and bay of Biscay ecosystems? J. Mar. Syst. 172, 104–117. doi: 10.1016/j.jmarsys.2017.03.008
Moutopoulos D. K., Libralato S., Solidoro C., Stergiou K. I. (2013). Toward an ecosystem approach to fisheries in the Mediterranean Sea: multi-gear/multi-species implications from an ecosystem model of the Greek Ionian Sea. J. Mar. Syst. 113-114, 13–28. doi: 10.1016/j.jmarsys.2012.12.002
Moutopoulos D. K., Tsagarakis K., Machias A. (2018). Assessing ecological and fisheries implications of the EU landing obligation in Eastern Mediterranean. J. Sea Res. 141, 99–111. doi: 10.1016/j.seares.2018.08.006
Natugonza V., Ainsworth C., Sturludóttir E., Musinguzi L., Ogutu-Ohwayo R., Tomasson T., et al. (2020). Ecosystem modelling of data-limited fisheries: how reliable are ecopath with ecosim models without historical time series fitting? J. Great Lakes Res. 46 (2), 414–428. doi: 10.1016/j.jglr.2020.01.001
Navarro J., Coll M., Louzao M., Palomera I., Delgado A., Forero M. G. (2011). Comparison of ecosystem modelling and isotopic approach as ecological tools to investigate food webs in the NW Mediterranean Sea. J. Exp. Mar. Biol. Ecol. 401, 97–104. doi: 10.1016/j.jembe.2011.02.040
Nee S. (1990). Community construction. Trends Ecol. Evol. 5 (10), 337–339. doi: 10.1016/0169-5347(90)90182-D
Newton A., Icely J., Cristina S., Brito A., Cardoso A. C., Colijn F., et al. (2014). An overview of ecological status, vulnerability and future perspectives of European large shallow, semi-enclosed coastal systems, lagoons and transitional waters. Estuarine Coast. Shelf Sci. 140, 95–122. doi: 10.1016/j.ecss.2013.05.023
Newton A., Icely J., Cristina S., Perillo G. M. E., Turner R. E., Ashan D., et al. (2020). Anthropogenic, direct pressures on coastal wetlands. Front. Ecol. Evol. 8, 144. doi: 10.3389/fevo.2020.00144
Niiranen S., Yletyinen J., Tomczak M., Blenckner T., Hjerne O., MacKenzie B. R., et al. (2013). Combined effects of global climate change and regional ecosystem drivers on an exploited marine food web. Global Change Biol. 19 (11), 3327–3342. doi: 10.1111/gcb.12309
Noguès Q., Araignous E., Bourdaud P., Halouani G., Raoux A., Foucher E., et al. (2022). Spatialized ecological network analysis for ecosystem-based management: effects of climate change, marine renewable energy, and fishing on ecosystem functioning in the bay of seine. ICES J. Mar. Syst. 79 (4), 1098–1112. doi: 10.1093/icesjms/fsac026
Ofir E., Silver T., Steenbeek J. G., Shachar N., Gal G. (2022). Applying the safe operating space (SOS) approach to sustainable commercial fishing under varying lake levels and littoral zone conditions. Fisheries. 48 (3), 107–120. doi: 10.1002/fsh.10869
Ojaveer H., Jaanus A., MacKenzie B. R., Martin G., Olenin S., Radziejewska T., et al. (2010). Status of biodiversity in the Baltic Sea. PloS One 5 (9), e12467. doi: 10.1371/journal.pone.0012467
Österblom H., Hansson S., Larsson U., Hjerne O., Wulff F., Elmgren R., et al. (2007). Human-induced trophic cascades and ecological regime shifts in the Baltic Sea. Ecosystems 10, 877–889. doi: 10.1007/s10021-007-9069-0
Otogo G. A., Wangvoralak S., Pierce G. J., Hastie L. C., Scott B. (2015). The ecological role of Loligo forbesii in the Moray firth ecosystem, northeast Scotland. Int. J. Mar. Environ. Sci. 9, 7.
Ottersen G., Bogstad B., Yaragina N. A., Stige L. C., Vikebø F. B., Dalpadado P. (2014). A review of early life history dynamics of barents Sea cod (Gadus morhua). ICES J. Mar. Sci. 71 (8), 2064–2087. doi: 10.1093/icesjms/fsu037
Outeiro L., Byron C., Angelini R. (2018). Ecosystem maturity as a proxy of mussel aquaculture carrying capacity in ria de arousa (NW spain): a food web modeling perspective. Aquaculture 496, 270–284. doi: 10.1016/j.aquaculture.2018.06.043
Pampoulie C., Slotte A., Óskarsson G. J., Helyar S. J., Jónsson Á, Ólafsdóttir G., et al. (2015). Stock structure of Atlantic herring Clupea harengus in the Norwegian Sea and adjacent waters. Mar. Ecol. Prog. Ser. 522, 219–230. doi: 10.3354/meps11114
Papaconstantinou C., Farrugio H. (2000). Fisheries in the Mediterranean. Mediterr. Mar. Sci. 1, 5–18. doi: 10.12681/mms.2
Papantoniou G., Giannoulaki M., Stoumboudi M. T., Lefkaditou E., Tsagarakis K. (2021). Food web interactions in a human dominated Mediterranean coastal ecosystem. Mar. Environ. Res. 172, 105507. doi: 10.1016/j.marenvres.2021.105507
Papapanagiotou G., Tsagarakis K., Koutsidi M., Tzanatos E. (2020). Using traits to build and explain an ecosystem model: ecopath with ecosim modelling of the north Aegean Sea (Eastern Mediterranean). Estuarine Coast. Shelf Sci. 236, 106614. doi: 10.1016/j.ecss.2020.106614
Patrício J., Marques J. C. (2006). Mass balanced models of the food web in three areas along a gradient of eutrophication symptoms in the south arm of the mondego estuary (Portugal). Ecol. Model. 197 (1-2), 21–34. doi: 10.1016/j.ecolmodel.2006.03.008
Pauly D., Christensen V. (1995). Primary production required to sustain global fisheries. Nature 374, 255–257. doi: 10.1038/374255a0
Pauly D., Christensen V., Dalsgaard J., Froese R., Torres J. (1998). Fishing down marine food webs. Science 279 (5352), 860–863. doi: 10.1126/science.279.5352.860
Pauly D., Christensen V., Walters C. (2000). Ecopath, ecosim, and ecospace as tools for evaluating ecosystem impact of fisheries. ICES J. Mar. Sci. 57 (3), 697–706. doi: 10.1006/jmsc.2000.0726
Pauly D., Soriano-Bartz M. L., Palomares M. L. D. (1993). “Improved construction, parametrization and interpretation of steady-state ecosystem models” in Trophic models of aquatic ecosystems. ICLARM Conf. Proc. 26, 1–13.
Pedersen T. (2022). Comparison between trophic positions in the barents Sea estimated from stable isotope data and a mass balance model. Front. Mar. Sci. 9, 813977. doi: 10.3389/fmars.2022.813977
Pedersen T., Fuhrmann M. M., Lindstrøm U., Nilssen E. M., Ivarjord T., Ramasco V., et al. (2018). Effects of the invasive red king crab on food web structure and ecosystem properties in an Atlantic fjord. Mar. Ecol. Prog. Ser. 596, 13–31. doi: 10.3354/meps12548
Pedersen T., Mikkelsen N., Lindstrøm U., Renaud P. E., Nascimento M. C., Blanchet M.-A., et al. (2021). Overexploitation, recovery, and warming of the barents Sea ecosystem during 1950–2013. Front. Mar. Sci. 8, 732637. doi: 10.3389/fmars.2021.732637
Pedersen T., Nilsen M., Nilssen E. M., Berg E., Reigstad E. (2008). Trophic model of a lightly exploited cod-dominated ecosystem. Ecol. Model. 214 (2-4), 95–111. doi: 10.1016/j.ecolmodel.2007.12.012
Pedersen T., Ramsvatn S., Nilssen E. M., Nilsen M., Morissette L., Ivarjord T., et al. (2016). Species diversity affects ecosystem structure and mass flows in fjords. Regional Stud. Mar. Sci. 3, 205–215. doi: 10.1016/j.rsma.2015.10.007
Peled Y., Zemah-Shamir S., Israel A., Shechter M., Ofir E., Gal G. (2020). Incorporating insurance value into ecosystem services assessments: mitigation of ecosystem users’ welfare uncertainty through biological control. Ecosystem Serv. 46, 101192. doi: 10.1016/j.ecoser.2020.101192
Pennino M. G., Bevilacqua A. H., Torres M. A., Bellido J. M., Sole J., Steenbeek J., et al. (2020). Discard ban: a simulation-based approach combining hierarchical Bayesian and food web spatial models. Mar. Policy 116, 103703. doi: 10.1016/j.marpol.2019.103703
Perryman H. A., Hansen C., Howell D., Olsen E. (2021). A review of applications evaluating fisheries management scenarios through marine ecosystem models. Rev. Fish. Sci. Aquacult. 29 (4), 800–835. doi: 10.1080/23308249.2021.1884642
Pezy J.-P., Raoux A., Marmin S., Balay P., Niquil N., Dauvin J.-C. (2017). Before-after analysis of the trophic network of an experimental dumping site in the eastern part of the bay of seine (English channel). Mar. pollut. Bull. 118 (1-2), 101–111. doi: 10.1016/j.marpolbul.2017.02.042
Pianka E. R. (1973). The structure of lizard communities. Annu. Rev. Ecol. System. 4, 53–74. doi: 10.1146/annurev.es.04.110173.000413
Pikitch E. K., Rountos K. J., Essington T. E., Santora C., Pauly D., Watson R., et al. (2014). The global contribution of forage fish to marine fisheries and ecosystems. Fish Fish. 15 (1), 43–64. doi: 10.1111/faf.12004
Pikitch E. K., Santora C., Babcock E. A., Bakun A., Bonfil R., Conover D. O., et al. (2004). Ecosystem-based fishery management. Science 305 (5682), 346–347. doi: 10.1126/science.1098222
Pinnegar J. K., Polunin N. V. C. (2004). Predicting indirect effects of fishing in Mediterranean rocky littoral communities using a dynamic simulation model. Ecol. Model. 172 (2-4), 249–267. doi: 10.1016/j.ecolmodel.2003.09.010
Piroddi C., Bearzi G., Christensen V. (2010). Effects of local fisheries and ocean productivity on the northeastern Ionian Sea ecosystem. Ecol. Model. 221 (11), 1526–1544. doi: 10.1016/j.ecolmodel.2010.03.002
Piroddi C., Bearzi G., Christensen V. (2011b). Marine open cage aquaculture in the eastern Mediterranean Sea: a new trophic resource for bottlenose dolphins. Mar. Ecol. Prog. Ser. 440, 255–266. doi: 10.3354/meps09319
Piroddi C., Bearzi G., Gonzalvo J., Christensen V. (2011a). From common to rare: the case of the Mediterranean common dolphin. Biol. Conserv. 144 (10), 2490–2498. doi: 10.1016/j.biocon.2011.07.003
Piroddi C., Coll M., Liquete C., Macias D., Greer K., Buszowski J., et al. (2017). Historical changes of the Mediterranean Sea ecosystem: modelling the role and impact of primary productivity and fisheries changes over time. Sci. Rep. 7, 44491. doi: 10.1038/srep44491
Piroddi C., Coll M., Macias D., Steenbeek J., Garcia-Gorriz E., Mannini A., et al. (2022). Modelling the Mediterranean Sea ecosystem at high spatial resolution to inform the ecosystem-based management in the region. Sci. Rep. 12, 19680. doi: 10.1038/s41598-022-18017-x
Piroddi C., Coll M., Steenbeek J., Macias Moy D., Christensen V. (2015b). Modelling the Mediterranean marine ecosystem as a whole: addressing the challenge of complexity. Mar. Ecol. Prog. Ser. 533, 47–65. doi: 10.3354/meps11387
Piroddi C., Moutopoulos D. K., Gonzalvo J., Libralato S. (2016). Ecosystem health of a Mediterranean semi-enclosed embayment (Amvrakikos gulf, greece): assessing changes using a modeling approach. Continental Shelf Res. 121, 61–73. doi: 10.1016/j.csr.2015.10.007
Piroddi C., Teixeira H., Lynam C. P., Smith C., Alvarez M. C., Mazik K., et al. (2015a). Using ecological models to assess ecosystem status in support of the European marine strategy framework directive. Ecol. Indic. 58, 175–191. doi: 10.1016/j.ecolind.2015.05.037
Polovina J. J. (1984). Model of a coral reef ecosystem: the ECOPATH model and its application to french frigate shoals. Coral Reefs 3, 1–11. doi: 10.1007/BF00306135
Popov S., Zeller D. (2018). Reconstructed Russian fisheries catches in the barents Sea: 1950-2014. Front. Mar. Sci. 5, 266. doi: 10.3389/fmars.2018.00266
Pranovi F., Link J. S. (2009). Ecosystem exploitation and trophodynamic indicators: a comparison between the northern Adriatic Sea and southern new England. Prog. Oceanogr. 81, 149–164. doi: 10.1016/j.pocean.2009.04.008
Prato G., Barrier C., Francour P., Cappanera V., Markantonatou V., Guidetti P., et al. (2016). Assessing interacting impacts of artisanal and recreational fisheries in a small marine protected area (Portofino, NW Mediterranean Sea). Ecosphere 7 (12), e01601. doi: 10.1002/ecs2.1601
Prato G., Gascuel D., Valls A., Francour P. (2014). Balancing complexity and feasibility in Mediterranean coastal food-web models: uncertainty and constraints. Mar. Ecol. Prog. Ser. 512, 71–88. doi: 10.3354/meps10988
Püts M., Kempf A., Möllmann C., Taylor M. (2023). Trade-offs between fisheries, offshore wind farms and marine protected areas in the southern north Sea – winners, losers and effective spatial management. Mar. Policy 152, 105574. doi: 10.1016/j.marpol.2023.105574
Püts M., Taylor M., Núñez-Riboni I., Steenbeek J., Stäbler M., Möllmann C., et al. (2020). Insights on integrating habitat preferences in process-oriented ecological models – a case study of the southern north Sea. Ecol. Model. 431, 109189. doi: 10.1016/j.ecolmodel.2020.109189
Raoux A., Lassalle G., Pezy J.-P., Tecchio S., Safi G., Ernande B., et al. (2019). Measuring sensitivity of two OSPAR indicators for a coastal food web model under offshore wind farm construction. Ecol. Indic. 96, 728–738. doi: 10.1016/j.ecolind.2018.07.014
Raoux A., Tecchio S., Pezy J.-P., Lassalle G., Degraer S., Wilhelmsson D., et al. (2017). Benthic and fish aggregation inside an offshore wind farm: which effects on the trophic web functioning? Ecol. Indic. 72, 33–46. doi: 10.1016/j.ecolind.2016.07.037
Ray D., Leary P., Livens F., Gray N., Morris K., Law K. A., et al. (2020). Controls on anthropogenic radionuclide distribution in the Sellafield-impacted Eastern Irish Sea. Sci. Total Environ. 743, 140765. doi: 10.1016/j.scitotenv.2020.140765
Reckermann M., Omstedt A., Soomere T., Aigars J., Akhtar N., Beldowska M., et al. (2022). Human impacts and their interactions in the Baltic Sea region. Earth System Dyn. 13 (1), 1–80. doi: 10.5194/esd-13-1-2022
Reeburgh W. S., Ward B. B., Whalen S. C., Sandbeck K. A., Kilpatrickt K. A., Kerkhof L. J. (1991). Black Sea methane geochemistry. Deep Sea Res. Part A. Oceanogr. Res. Papers 38 (S2), S1189–S1210. doi: 10.1016/S0198-0149(10)80030-5
Refsgaard J. C., van der Sluijs J. P., Højberg A. L., Vanrolleghem P. A. (2007). Uncertainty in the environmental modelling process – a framework and guidance. Environ. Model. Softw. 22 (11), 1543–1556. doi: 10.1016/j.envsoft.2007.02.004
Reusch T. B. H., Dierking J., Andersson H. C., Bonsdorff E., Carstensen J., Casini M., et al. (2018). The Baltic Sea as a time machine for the future coastal ocean. Sci. Adv. 4 (5), eaar8195. doi: 10.1126/sciadv.aar8195
Ricci P., Libralato S., Capezzuto F., D’ Onghia G., Maiorano P., Sion L., et al. (2019). Ecosystem functioning of two marine food webs in the north-Western Ionian Sea (Central Mediterranean Sea). Ecol. Evol. 9 (18), 10198–10212. doi: 10.1002/ece3.5527
Ricci P., Sion L., Capezzuto F., Cipriano G., D’ Onghia G., Libralato S., et al. (2021). Modelling the trophic roles of the demersal Chondrichthyes in the northern Ionian Sea (Central Mediterranean Sea). Ecol. Model. 444, 109468. doi: 10.1016/j.ecolmodel.2021.109468
Roberts D. R., Bahn V., Ciuti S., Boyce M. S., Elith J., Guillera-Arroita G., et al. (2017). Cross-validation strategies for data with temporal, spatial, hierarchical, or phylogenetic structure. Ecography 40 (8), 913–929. doi: 10.1111/ecog.02881
Romagnoni G., Mackinson S., Hong J., Eikeset A. M. (2015). The ecospace model applied to the north Sea: evaluating spatial predictions with fish biomass and fishing effort data. Ecol. Model. 300, 50–60. doi: 10.1016/j.ecolmodel.2014.12.016
Rombouts I., Beaugrand G., Artigas L. F., Dauvin J. C., Gevaert F., Goberville E., et al. (2013). Evaluating marine ecosystem health: case studies of indicators using direct observations and modelling methods. Ecol. Indic. 24, 353–365. doi: 10.1016/j.ecolind.2012.07.001
Rounsevell M. D. A., Arneth A., Brown C., Cheung W. W. L., Gimenez O., Holman I., et al. (2021). Identifying uncertainties in scenarios and models of socio-ecological systems in support of decision-making. One Earth 4 (7), 967–985. doi: 10.1016/j.oneear.2021.06.003
Rybarczyk H., Elkaıüm B. (2003). An analysis of the trophic network of a macrotidal estuary: the seine estuary (Eastern channel, Normandy, France). Estuarine Coast. Shelf Sci. 58 (4), 775–791. doi: 10.1016/S0272-7714(03)00184-7
Rybarczyk H., Elkaıüm B., Ochs L., Loquet N. (2003). Analysis of the trophic network of a macrotidal ecosystem: the bay of Somme (Eastern channel). Estuarine Coast. Shelf Sci. 58 (3), 405–421. doi: 10.1016/S0272-7714(02)00294-9
Sabatino R., Di Cesare A., Dzhembekova N., Fontaneto D., Eckert E. M., Corno G., et al. (2020). Spatial distribution of antibiotic and heavy metal resistance genes in the black Sea. Mar. pollut. Bull. 160, 111635. doi: 10.1016/j.marpolbul.2020.111635
Sætre R. (1999). Features of the central Norwegian shelf circulation. Continental Shelf Res. 19 (14), 1809–1831. doi: 10.1016/S0278-4343(99)00041-2
Safi G., Giebels D., Arroyo N. L., Heymans J. J., Preciado I., Raoux A., et al. (2019). Vitamine ENA: a framework for the development of ecosystem-based indicators for decision makers. Ocean Coast. Manage. 174, 116–130. doi: 10.1016/j.ocecoaman.2019.03.005
Sánchez F., Olaso I. (2004). Effects of fisheries on the cantabrian Sea shelf ecosystem. Ecol. Model. 172, 151–174. doi: 10.1016/j.ecolmodel.2003.09.005
Sandberg J., Elmgren R., Wulff F. (2000). Carbon flows in Baltic Sea food webs — a re-evaluation using a mass balance approach. J. Mar. Syst. 25, 249–260. doi: 10.1016/S0924-7963(00)00019-1
Sandberg J., Kumbald L., Kautsky U. (2007). Can ECOPATH with ECOSIM enhance models of radionuclide flows in food webs? - an example for 14C in a coastal food web in the Baltic Sea. J. Environ. Radioactivity 92, 96–111. doi: 10.1016/j.jenvrad.2006.09.010
Sautour B., Castel J. (1995). Comparative spring distribution of zooplankton in three macrotidal European estuaries. Hydrobiologia 311, 139–151. doi: 10.1007/BF00008577
Sayer M. D. J., Magill S. H., Pitcher T. J., Morissette L., Ainsworth C. (2005). Simulation-based investigations of fishery changes as affected by the scale and design of artificial habitats. J. Fish Biol. 67 (sB), 218–243. doi: 10.1111/j.0022-1112.2005.00928.x
Saygu I., Akoglu E., Gül G., Bedikoğlu D., Demirel N. (2023). Fisheries impact on the Sea of marmara ecosystem structure and functioning during the last three decades. Front. Mar. Sci. 9, 1076399. doi: 10.3389/fmars.2022.1076399
Saygu I., Heymans J. J., Fox C. J., Özbilgin H., Eryaşar A. R., Gökçe G. (2020). The importance of alien species to the food web and bottom trawl fisheries of the northeastern Mediterranean, a modelling approach. J. Mar. Syst. 202, 103253. doi: 10.1016/j.jmarsys.2019.103253
Scharler U. M., Ulanowicz R. E., Fogel M. L., Wooler M. J., Jacobson-Meyers M. E., Lovelock C. E., et al. (2015). Variable nutrient stoichiometry (carbon:nitrogen:phosphorus) across trophic levels determines community and ecosystem properties in an oligotrophic mangrove system. Oecologia 179, 863–876. doi: 10.1007/s00442-015-3379-2
Scott E., Serpetti N., Steenbeek J., Heymans J. J. (2016). A stepwise fitting procedure for automated fitting of ecopath with ecosim models. SoftwareX 5, 25–30. doi: 10.1016/j.softx.2016.02.002
Scotti M., Bondavalli C., Rossetti G., Bodini A. (2022b). Flow network indices signal a directional change in ecosystems: evidence from a small mountain lake (Lake Santo, northern Italy). Ecol. Indic. 139, 108896. doi: 10.1016/j.ecolind.2022.108896
Scotti M., Opitz S., MacNeil L., Kreutle A., Pusch C., Froese R. (2022a). Ecosystem-based fisheries management increases catch and carbon sequestration through recovery of exploited stocks: the western Baltic Sea case study. Front. Mar. Sci. 9, 879998. doi: 10.3389/fmars.2022.879998
Selleslagh J., Lobry J., Amara R., Brylinski J.-M., Boët P. (2012). Trophic functioning of coastal ecosystems along an anthropogenic pressure gradient: a French case study with emphasis on a small and low impacted estuary. Estuarine Coast. Shelf Sci. 112, 73–85. doi: 10.1016/j.ecss.2011.08.004
Serpetti N., Baudron A. R., Burrows M. T., Payne B. L., Helaouët P., Fernandes P. G., et al. (2017). Impact of ocean warming on sustainable fisheries management informs the ecosystem approach to fisheries. Sci. Rep. 7, 13438. doi: 10.1038/s41598-017-13220-7
Serpetti N., Benjamins S., Brain S., Collu M., Harvey B. J., Heymans J. J., et al. (2021). Modeling small scale impacts of multi-purpose platforms: an ecosystem approach. Front. Mar. Sci. 8, 694013. doi: 10.3389/fmars.2021.694013
Seyer T., Bănaru D., Vaz S., Hattab T., Labrune C., Booth S., et al. (2023). Ecosystem modelling in the northwestern Mediterranean Sea: structure and functioning of a complex system. J. Mar. Syst. 240, 103877. doi: 10.1016/j.jmarsys.2023.103877
Shabtay A., Portman M. E., Ofir E., Carmel Y., Gal G. (2018). Using Ecol. Modell. in marine spatial planning to enhance ecosystem-based management. Mar. Policy 95, 14–23. doi: 10.1016/j.marpol.2018.06.018
Shalovenkov N. (2019). “Chapter 31 - alien species invasion: case study of the black Sea,” in Coasts and estuaries, 547–568.
Shannon L. J., Coll M., Bundy A., Gascuel D., Heymans J. J., Kleisner K., et al. (2014). Trophic level-based indicators to track fishing impacts across marine ecosystems. Mar. Ecol. Prog. Ser. 512, 115–140. doi: 10.3354/meps10821
Sheldon R. W., Prakash A., Sutcliffe Jr. (1972). The size distribution of particles in the ocean. Limnol. Oceanogr. 17 (3), 327–340. doi: 10.4319/lo.1972.17.3.0327
Shin Y.-J., Houle J. E., Akoglu E., Blanchard J. L., Bundy A., Coll M., et al. (2018). The specificity of marine ecological indicators to fishing in the face of environmental change: a multi-model evaluation. Ecol. Indic. 89, 317–326. doi: 10.1016/j.ecolind.2018.01.010
Skern-Mauritzen M., Ottersen G., Handegard N. O., Huse G., Dingsør G. E., Stenseth N. C., et al. (2016). Ecosystem processes are rarely included in tactical fisheries management. Fish Fish. 17 (1), 165–175. doi: 10.1111/faf.12111
Smith A. J. (1989). The English channel–by geological design or catastrophic accident? Proc. Geol. Assoc. 100 (3), 325–337. doi: 10.1016/S0016-7878(89)80052-5
Smith A. D. M., Fulton E. J., Hobday A. J., Smith D. C., Shoulder P. (2007). Scientific tools to support the practical implementation of ecosystem-based fisheries management. ICES J. Mar. Sci. 64 (4), 633–639. doi: 10.1093/icesjms/fsm041
Sohlenius G., Emeis K.-C., Andrén E., Andrén T., Kohly A. (2001). Development of anoxia during the Holocene fresh–brackish water transition in the Baltic Sea. Mar. Geol. 177 (3-4), 221–242. doi: 10.1016/S0025-3227(01)00174-8
Stäbler M., Kempf A., Mackinson S., Poos J. J., Garcia C., Temming A. (2016). Combining efforts to make maximum sustainable yields and good environmental status match in a food-web model of the southern north Sea. Ecol. Model. 331, 17–30. doi: 10.1016/j.ecolmodel.2016.01.020
Stäbler M., Kempf A., Temming A. (2018). Assessing the structure and functioning of the southern north Sea ecosystem with a food-web model. Ocean Coast. Manage. 165, 280–297. doi: 10.1016/j.ocecoaman.2018.08.017
Steenbeek J., Buszowski J., Chagaris D., Christensen V., Coll M., Fulton E. A., et al. (2021). Making spatial-temporal marine ecosystem modelling better – a perspective. Environ. Model. Softw. 145, 105209. doi: 10.1016/j.envsoft.2021.105209
Steenbeek J., Coll M., Gurney L., Mélin F., Hoepffner N., Buszowski J., et al. (2013). Bridging the gap between ecosystem modeling tools and geographic information systems: driving a food web model with external spatial–temporal data. Ecol. Model. 263, 139–151. doi: 10.1016/j.ecolmodel.2013.04.027
Steenbeek J., Corrales J., Platts M., Coll M. (2018). Ecosampler: a new approach to assessing parameter uncertainty in ecopath with ecosim. Softw. X 7, 198–204. doi: 10.1016/j.softx.2018.06.004
Stock A., Murray C. C., Gregr E. J., Steenbeek J., Woodburn E., Micheli F., et al. (2023). Exploring multiple stressor effects with ecopath, ecosim, and ecospace: research designs, modeling techniques, and future directions. Sci. Total Environ. 869, 161719. doi: 10.1016/j.scitotenv.2023.161719
Stow C. A., Jolliff J., McGillicuddy Jr. D. J., Doney S. C., Allen J. I., Friedrichs M. A. M., et al. (2009). Skill assessment for coupled biological/physical models of marine systems. J. Mar. Syst. 76 (1-2), 4–15. doi: 10.1016/j.jmarsys.2008.03.011
Susini I., Todd V. L. G. (2021). Predictive capacity of ecopath with ecosim: model performance and ecological indicators’ response to imprecision. Environ. Model. Softw. 143, 105098. doi: 10.1016/j.envsoft.2021.105098
Szalaj D., Silva A., Ré P., Cabral H. (2022). Predictions of sardine and the Portuguese continental shelf ecosystem dynamics under future fishing, forced-biomass and SST scenarios. Mar. pollut. Bull. 178, 113594. doi: 10.1016/j.marpolbul.2022.113594
Szalaj D., Torres M. A., Veiga-Malta T., Angélico M. M., Sobrinho-Gonçalves L., Chaves C., et al. (2021). Food-web dynamics in the Portuguese continental shelf ecosystem between 1986 and 2017: unravelling drivers of sardine decline. Estuarine Coast. Shelf Sci. 251, 107259. doi: 10.1016/j.ecss.2021.107259
Tecchio S., Coll M., Christensen V., Company J. B., Ramírez-Llodra E., Sardà F. (2013). Food web structure and vulnerability of a deep-sea ecosystem in the NW Mediterranean Sea. Deep Sea Res. Part I: Oceanogr. Res. Papers 75, 1–15. doi: 10.1016/j.dsr.2013.01.003
Tecchio S., Rius A. T., Dauvin J.-C., Lobry J., Lassalle G., Morin J., et al. (2015). The mosaic of habitats of the seine estuary: insights from food-web modelling and network analysis. Ecol. Model. 312, 91–101. doi: 10.1016/j.ecolmodel.2015.05.026
Tierney K. M., Heymans J. J., Muir G. K. P., Cook G. T., Buszowski J., Steenbeek J., et al. (2018). Modelling marine trophic transfer of radiocarbon (14C) from a nuclear facility. Environ. Model. Softw. 102, 138–154. doi: 10.1016/j.envsoft.2018.01.013
Tomczak M. T., Heymans J. J., Yletyinen J., Niiranen S., Otto S. A., Blenckner T. (2013). Ecological network indicators of ecosystem status and change in the Baltic Sea. PloS One 8 (10), e75439. doi: 10.1371/journal.pone.0075439
Tomczak M. T., Müller-Karulis B., Järv L., Kotta J., Martin G., Minde A., et al. (2009). Analysis of trophic networks and carbon flows in south-eastern Baltic coastal ecosystems. Prog. Oceanogr. 81, 111–131. doi: 10.1016/j.pocean.2009.04.017
Tomczak M. T., Niiranen S., Hjerne O., Blenckner T. (2012). Ecosystem flow dynamics in the Baltic proper–using a multi-trophic dataset as a basis for food–web modelling. Ecol. Model. 230, 123–147. doi: 10.1016/j.ecolmodel.2011.12.014
Torres M. A., Coll M., Heymans J. J., Christensen V., Sobrino I. (2013). Food-web structure of and fishing impacts on the gulf of cadiz ecosystem (South-western Spain). Ecol. Indic. 265, 26–44. doi: 10.1016/j.ecolmodel.2013.05.019
Townsend M., Thrush S. F., Lohrer A. M., Hewitt J. E., Lundquist C. J., Carbines M., et al. (2014). Overcoming the challenges of data scarcity in mapping marine ecosystem service potential. Ecosystem Serv. 8, 44–55. doi: 10.1016/j.ecoser.2014.02.002
Trochta J. T., Pons M., Rudd M. B., Krigbaum M., Tanz A., Hilborn R. (2018). Ecosystem-based fisheries management: perception on definitions, implementations, and aspirations. PloS One 13 (1), e0190467. doi: 10.1371/journal.pone.0190467
Tsagarakis K., Coll M., Giannoulaki M., Somarakis S., Papaconstantinou C., Machias A. (2010). Food web traits of the north Aegean Sea ecosystem (Eastern Mediterranean) and comparison with other Mediterranean ecosystems. Estuarine Coast. Shelf Sci. 88 (2), 233–248. doi: 10.1016/j.ecss.2010.04.007
Tsagarakis K., Libralato S., Giannoulaki M., Touloumis K., Somarakis S., Machias A., et al. (2022). Drivers of the north Aegean Sea ecosystem (Eastern Mediterranean) through time: insights from multidecadal retrospective analysis and future simulations. Front. Mar. Sci. 9, 919793. doi: 10.3389/fmars.2022.919793
Tsagarakis K., Panigada S., Machias A., Giannoulaki M., Foutsi A., Pierantonio N., et al. (2021). Trophic interactions in the "small pelagic fish - dolphins - fisheries" triangle: outputs of a modelling approach in the north Aegean Sea (Eastern Mediterranean, Greece). Ocean Coast. Manage. 204, 105474. doi: 10.1016/j.ocecoaman.2020.105474
Tsiaras K. P., Kourafalou V. H., Raitsos D. E., Triantafyllou G., Petihakis G., Korres G. (2012). Inter-annual productivity variability in the north Aegean Sea: influence of thermohaline circulation during the Eastern Mediterranean transient. J. Mar. Syst. 96-97, 72–81. doi: 10.1016/j.jmarsys.2012.02.003
Ulanowicz R. E. (1986). Growth and development: ecosystem phenomenology (New York: Springer Verlag (reprinted by iUniverse, 2000), 203.
Ulanowicz R. E. (2004). Quantitative methods for ecological network analysis. Comput. Biol. Chem. 28 (5-6), 321–339. doi: 10.1016/j.compbiolchem.2004.09.001
Ulanowicz R. E., Kemp W. M. (1979). Toward canonical trophic aggregations. Am. Nat. 114, 871–883. doi: 10.1086/283534
Väli G., Meier H. E. M., Elken J. (2013). Simulated halocline variability in the Baltic Sea and its impact on hypoxia during 1961–2007. J. Geophys. Res. 118 (12), 6982–7000. doi: 10.1002/2013JC009192
Valls A., Coll M., Christensen V. (2015). Keystone species: toward an operational concept for marine biodiversity conservation. Ecol. Monogr. 85 (1), 29–47. doi: 10.1890/14-0306.1
Valls A., Gascuel D., Guénette S., Francour P. (2012). Modeling trophic interactions to assess the effects of a marine protected area: case study in the NW Mediterranean Sea. Mar. Ecol. Prog. Ser. 456, 201–214. doi: 10.3354/meps09701
Vanalderweireldt L., Albouy C., Le Loc’h F., Millot R., Blestel C., Patrissi M., et al. (2022). Ecosystem modelling of the Eastern Corsican coast (ECC): case study of one of the least trawled shelves of the Mediterranean Sea. J. Mar. Syst. 235, 103798. doi: 10.1016/j.jmarsys.2022.103798
Varkitzi I., Psarra S., Assimakopoulou G., Pavlidou A., Krasakopoulou E., Velaoras D., et al. (2020). Phytoplankton dynamics and bloom formation in the oligotrophic Eastern Mediterranean: field studies in the Aegean, levantine and Ionian seas. Deep Sea Res. Part II: Topical Stud. Oceanogr. 171, 104662. doi: 10.1016/j.dsr2.2019.104662
Vassallo P., Bellardini D., Castellano M., Dapueto G., Povero P. (2022). Structure and functionality of the mesozooplankton community in a coastal marine environment: portofino marine protected area (Liguria). Diversity 14 (1), 19. doi: 10.3390/d14010019
Vassallo P., D’ Onghia G., Fabiano M., Maiorano P., Lionetti A., Paoli C., et al. (2017). A trophic model of the benthopelagic fauna distributed in the Santa maria di leuca cold-water coral province (Mediterranean Sea). Energy Ecol. Environ. 2, 114–124. doi: 10.1007/s40974-016-0047-2
Vassallo P., Fabiano M., Vezzulli L., Sandulli R., Marques J. C., Jørgensen S. E. (2006). Assessing the health of coastal marine ecosystems: a holistic approach based on sediment micro and meio-benthic measures. Ecol. Indic. 6 (3), 525–542. doi: 10.1016/j.ecolind.2005.07.003
Vassallo P., Paoli C., Fabiano M. (2012). Ecosystem level analysis of sandy beaches using thermodynamic and network analyses: a study case in the NW Mediterranean Sea. Ecol. Indic. 15 (1), 10–17. doi: 10.1016/j.ecolind.2011.09.016
Vassallo P., Paoli C., Schiavon G., Albertelli G., Fabiano M. (2013). How ecosystems adapt to face disruptive impact? the case of a commercial harbor benthic community. Ecol. Indic. 24, 431–438. doi: 10.1016/j.ecolind.2012.07.021
Vasslides J. M., de Mutsert K., Christensen V., Townsend H. (2017). Using the ecopath with ecosim modeling approach to understand the effects of watershed-based management actions in coastal ecosystems. Coast. Manage. 45 (17), 44–55. doi: 10.1080/08920753.2017.1237241
Veiga-Malta T., Szalaj D., Angélico M. M., Azevedo M., Farias I., Garrido S., et al. (2019). First representation of the trophic structure and functioning of the Portuguese continental shelf ecosystem: insights into the role of sardine. Mar. Ecol. Prog. Ser. 617-618, 323–340. doi: 10.3354/meps12724
Vieites D. R., Nieto-Román S., Palanca A., Ferrer X., Vences M. (2004). European Atlantic: The hottest oil spill hotspot worldwide. Sci. Nat. 91, 535–538. doi: 10.1007/s00114-004-0572-2
Vilas D., Coll M., Corrales X., Steenbeek J., Piroddi C., Macias D., et al. (2021). Current and potential contributions of the gulf of lion fisheries restricted area to fisheries sustainability in the NW Mediterranean Sea. Mar. Policy 123, 104296. doi: 10.1016/j.marpol.2020.104296
Vilas D., Coll M., Pedersen T., Corrales X., Filbee-Dexter K., Pedersen T., et al. (2020a). Kelp-carbon uptake by Arctic deep-sea food webs plays a noticeable role in maintaining ecosystem structural and functional traits. J. Mar. Syst. 203, 103268. doi: 10.1016/j.jmarsys.2019.103268
Vilas D., Coll M., Pedersen T., Corrales X., Filbee-Dexter K., Wernberg T. (2020b). Future trajectories of change for an Arctic deep-sea ecosystem connected to coastal kelp forests. Restor. Ecol. 29 (S2), e13327. doi: 10.1111/rec.13327
Villanueva M. C., Ernande B., Mackinson S. (2009). Trophic network. channel habitat atlas for marine resource management, final report. Eds. Carpentier A., Martin C. S., Vaz S. (France: IFREMER, Boulogne-sur-mer), 547–562.
Villasante S., Arreguín-Sánchez F., Heymans J. J., Libralato S., Piroddi C., Christensen V., et al. (2016). Modelling marine ecosystems using the ecopath with ecosim food web approach: new insights to address complex dynamics after 30 years of developments. Ecol. Model. 331, 1–4. doi: 10.1016/j.ecolmodel.2016.04.017
Vincent C., Huon M., Caurant F., Dabin W., Deniau A., Dixneuf S., et al. (2017). Grey and harbour seals in France: distribution at sea, connectivity and trends in abundance at haulout sites. Deep Sea Res. Part II: Topical Stud. Oceanogr. 141, 294–305. doi: 10.1016/j.dsr2.2017.04.004
Vuorinen I., Hänninen J., Rajasitla M., Laine P., Eklund J., Montesino-Pouzols F., et al. (2015). Scenario simulations of future salinity and ecological consequences in the Baltic Sea and adjacent north Sea areas–implications for environmental monitoring. Ecol. Indic. 50, 196–205. doi: 10.1016/j.ecolind.2014.10.019
Walters W. J., Christensen V. (2018). Ecotracer: analyzing concentration of contaminants and radioisotopes in an aquatic spatial-dynamic food web model. J. Environ. Radioactivity 181, 118–127. doi: 10.1016/j.jenvrad.2017.11.008
Walters C., Christensen V., Pauly D. (1997). Structuring dynamic models of exploited ecosystems from trophic mass-balance assessments. Rev. Fish Biol. Fish. 7, 139–172. doi: 10.1023/A:1018479526149
Walters C., Pauly D., Christensen V. (1999). Ecospace: prediction of mesoscale spatial patterns in trophic relationships of exploited ecosystems, with emphasis on the impacts of marine protected areas. Ecosystems 2, 539–554. doi: 10.1007/s100219900101
Watson S. C. L., Beaumont N. J., Widdicombe S., Paterson D. M. (2020). Comparing the network structure and resilience of two benthic estuarine systems following the implementation of nutrient mitigation actions. Estuarine Coast. Shelf Sci. 244, 106059. doi: 10.1016/j.ecss.2018.12.016
Woods J. S., Verones F. (2019). Ecosystem damage from anthropogenic seabed disturbance: a life cycle impact assessment characterisation model. Sci. Total Environ. 649, 1481–1490. doi: 10.1016/j.scitotenv.2018.08.304
Zeller D., Reinert J. (2004). Modelling spatial closures and fishing effort restrictions in the faroe islands marine ecosystem. Ecol. Model. 172 (2-4), 403–420. doi: 10.1016/j.ecolmodel.2003.09.020
Keywords: ecopath with ecosim, european marine ecosystems, ecological Indicators, food web modelling, meta - analysis
Citation: Keramidas I, Dimarchopoulou D, Ofir E, Scotti M, Tsikliras AC and Gal G (2023) Ecotrophic perspective in fisheries management: a review of Ecopath with Ecosim models in European marine ecosystems. Front. Mar. Sci. 10:1182921. doi: 10.3389/fmars.2023.1182921
Received: 09 March 2023; Accepted: 12 April 2023;
Published: 02 May 2023.
Edited by:
Nazli Demirel, Istanbul University, TürkiyeReviewed by:
Ekin Akoglu, Middle East Technical University, TürkiyePaolo Vassallo, University of Genoa, Italy
Copyright © 2023 Keramidas, Dimarchopoulou, Ofir, Scotti, Tsikliras and Gal. This is an open-access article distributed under the terms of the Creative Commons Attribution License (CC BY). The use, distribution or reproduction in other forums is permitted, provided the original author(s) and the copyright owner(s) are credited and that the original publication in this journal is cited, in accordance with accepted academic practice. No use, distribution or reproduction is permitted which does not comply with these terms.
*Correspondence: Ioannis Keramidas, keraioan@bio.auth.gr