Clarifying the murk: unveiling bacterial dynamics in response to crude oil pollution, Corexit-dispersant, and natural sunlight in the Gulf of Mexico
- 1Division of Biological and Environmental Sciences, Faculty of Natural Sciences, University of Stirling, Stirling, United Kingdom
- 2Center for Environmental Diagnostics and Bioremediation, University of West Florida, Pensacola, FL, United States
- 3Sorbonne University, CNRS, Laboratoire de Biodiversité et Biotechnologies Microbiennes, USR3579, Observatoire Océanologique, Sorbonne Université, Banyuls-sur-mer, France
- 4Proteomic and Microbiology Department, University of Mons, Mons, Belgium
- 5High Performance and Cloud Computing Group, Zentrum für Datenverarbeitung (ZDV), University of Tübingen, Tübingen, Germany
The 2010 Deepwater Horizon (DwH) Oil spill released an enormous volume of oil into the Gulf of Mexico (GoM), prompting the widespread use of chemical dispersants like Corexit® EC9500A. The ecological consequences of this treatment, especially when combined with natural factors such as sunlight, remain unexplored in the context of marine bacterial communities’ dynamics. To address this knowledge gap, our study employed a unique metaproteomic approach, investigating the combined effects of sunlight, crude Macondo surrogate oil, and Corexit on GoM microbiome across different mesocosms. Exposure to oil and/or Corexit caused a marked change in community composition, with a decrease in taxonomic diversity relative to controls in only 24 hours. Hydrocarbon (HC) degraders, particularly those more tolerant to Corexit and phototoxic properties of crude oil and/or Corexit, proliferated at the expense of more sensitive taxa. Solar radiation exacerbated these effects in most taxa. We demonstrated that sunlight increased the dispersant’s toxicity, impacting on community structure and functioning. These functional changes were primarily directed by oxidative stress with upregulated proteins and enzymes involved in protein turnover, general stress response, DNA replication and repair, chromosome condensation, and cell division. These factors were more abundant in chemically treated conditions, especially in the presence of Corexit compared to controls. Oil treatment significantly enhanced the relative abundance of Alteromonas, an oil-degrading Gammaproteobacteria. In combined oil-Corexit treatments, the majority of identified protein functions were assigned to Alteromonas, with strongly expressed proteins involved in membrane transport, motility, carbon and amino acid metabolism and cellular defense mechanisms. Marinomonas, one of the most active genera in dark conditions, was absent from the light treatment. Numerous metabolic pathways and HC-degrading genes provided insights into bacterial community adaptation to oil spills. Key enzymes of the glyoxylate bypass, enriched in contaminant-containing treatments, were predominantly associated with Rhodobacterales and Alteromonadales. Several proteins related to outer membrane transport, photosynthesis, and nutrient metabolisms were characterized, allowing predictions of the various treatments on biogeochemical cycles. The study also presents novel perspectives for future oil spill clean-up processes.
1 Introduction
The Deepwater Horizon (DwH) spill occurred in the Gulf of Mexico (GoM) in spring 2010, a season characterized by intense sunlight in that region. Over 4.9 million barrels of crude oil were released into the surrounding waters, making this marine oil spill the largest one in history (Crone and Tolstoy, 2010; Lamendella et al., 2014). The acute pollution that occurred during the DwH oil spill posed an unparalleled threat to the GoM biota. Indigenous microorganisms in the GoM were significantly impacted by the oil spill (Lamendella et al., 2014). Any alterations in marine bacterial functioning are of great ecological impact. Indeed, bacteria are at the bottom of the oceanic food chain, regulate key biogeochemical cycles and play an essential role in the degradation of complex organic substances (Azam et al., 1983). The bacterial community can respond rapidly to environmental changes. In this way, understanding bacterial responses to oil spill is urgently needed, because of their large population sizes and short generation times.
1.1 Consequences of crude oil pollution
Crude oil is a complex mixture primarily composed of HC, roughly two-thirds of which are alkanes, cycloalkanes, and their derivatives, while polycyclic aromatic hydrocarbons (PAHs) and their derivatives make up the remainder (Tissot and Welte, 1984). Aromatic hydrocarbons such as PAHs are thought to be the most toxic compounds of oil (Neff and Anderson, 1981). The impact of an oil spill varies according to several factors. Environmental conditions such as mixing (Sterling et al., 2004), temperature, available nutrients, salinity (Horel et al., 2014), pressure (Marietou et al., 2018), and UV exposure (Liu et al., 2012; Bridges et al., 2018) affect the weathering process of crude oil, thus alter oil spill properties and the composition of microbial communities (Valencia-Agami et al., 2019).
The solubility of HCs determines their bioavailability to microorganisms. The water accessible fraction (WAF) of crude oil generally contains more volatile, and therefore more toxic, HCs, including low molecular weight (LMW) PAHs (Ghosal et al., 2016). High molecular weight (HMW) or more complex HCs, such as branched or polyaromatic HCs, are more persistent, their degradation sometimes require consortia of bacterial or microalgal strains (Ghosal et al., 2016; Ma et al., 2021). Petroleum toxicity may be stronger for smaller microorganisms, with a high surface/volume ratio (Urakawa et al., 2012). In our study, we used Macondo oil, also identified by its well designation MC-252 (from the Deepwater Horizon oil spill), which is a light crude oil. Light crude oils generally have lower density and viscosity compared to heavy crude oils, affecting their toxic impact on marine bacteria.
Most marine HCs, including PAHs, undergo biodegradation by HC-degrading microorganisms (Leahy and Colwell, 1990; Prince, 2010). Alkane-degrading enzymes, called alkane hydroxylases, are present in various bacteria, yeast, fungal, and algal species (Peixoto et al., 2011). Microorganisms often harbor multiple hydroxylases to degrade a diverse range of HCs, spanning from low to high molecular weight compounds, in addition to other enzymes like dioxygenases for PAH degradation, facilitating the breakdown of compounds found in crude oil (Van Beilen et al., 2003).
The diversity of microbial communities was found to be reduced as a consequence of oil exposure with a rapid shift in community composition, selecting for successive consortia of HC-degrading bacteria (Miralles et al., 2007; Orcutt et al., 2010; Hamdan and Fulmer, 2011; Bae et al., 2018; Valencia-Agami et al., 2019). Overall oil exposure enhanced oil-degrading heterotroph populations, but inhibited nitrifiers and denitrifiers (Urakawa et al., 2012; Pietroski et al., 2015; Levine et al., 2017; Bae et al., 2018). The GoM region is exposed to a significant quantity of HCs annually, from both anthropogenic and natural sources (Mason and Hazen, 2011). The GoM features an extensive natural hydrocarbon (HC) seepage, with over 600 occurrences, leading to an annual release of approximately 80,000 to 200,000 tonnes of crude oil. Consequently, microorganisms inhabiting regions with chronic HC exposure are believed to possess the capability for rapid responses to crude oil spills (Hill et al., 2006). Biodegradation played a pivotal role in determining the fate of the oil within the DwH resulting from subsurface dispersant application (Hill et al., 2006; Dubinsky et al., 2013). Within the GoM, HC-degrading microorganisms were characterized, including representatives from Oceanospirillales and Colwellia sp, all of which were notably enriched in the water column (Chakraborty et al., 2012).
1.2 Chemical dispersants - Corexit® EC9500A
The DwH spill witnessed an unprecedented scale of dispersant application, with 7 million liters mainly comprising Corexit® EC9500A sprayed onto surface slicks and injected into the wellhead (Chakraborty et al., 2012; Zuijdgeest and Huettel, 2012). Corexit accelerates oil weathering by reducing surface tension, breaking slicks into smaller droplets, thus facilitating bacterial aggregation rates (Sterling et al., 2004; Techtmann et al., 2017; Doyle et al., 2018). This dispersion process not only enhances microbial access to the oil but also promotes the diffusion and dilution of oil concentrations, providing sufficient oxygen, nitrogen, and phosphorus for microbial growth and oil consumption (Prince et al., 2013).
While some heterotrophs metabolized these components, stimulating aerobic oil biodegradation (Bruheim et al., 1999; Kujawinski et al., 2011; Chakraborty et al., 2012), other studies reported the toxicity of Corexit® EC9500A components, such as ethoxylated sorbitan monooleate, sorbitan monooleate, and dioctyl sodium sulfosuccinate (DOSS; Radniecki et al., 2013), toward numerous marine microorganisms (Rosal et al., 2010; Hamdan and Fulmer, 2011; Kujawinski et al., 2011; Zuijdgeest and Huettel, 2012; Kleindienst et al., 2015). Corexit increases the immediate presence of toxic HCs in the water accessible fraction (WAF), particularly HMW PAHs (Radniecki et al., 2013).
The impact of dispersants on microbial communities is species-specific (Kleindienst et al., 2015; Overholt et al., 2016) and remains a topic of debate. The efficiency of dispersants can also be inconsistent, influenced by various factors, including chemical structure, hydrocarbon concentration, and the metabolic potential of microbial populations (Overholt et al., 2016) as natural environmental factors such as sunlight.
1.3 Effect of solar radiation on oil spill and HC-degrading bacteria
The GoM surface waters are subjected to intense solar radiation, particularly during the Deepwater Horizon (DwH) oil spill (Liu et al., 2016). This strong solar irradiance significantly influences the weathering of oil, playing a pivotal role in photooxidation and enhancing oil dispersion within the water column (Liu et al., 2016; Bridges et al., 2018; Snyder et al., 2021). The penetration of UV radiation into the water is influenced by the optical properties of both the surface oil slick and the water itself (King et al., 2014; Bridges et al., 2018; Quigg et al., 2021). The composition of hydrocarbons (HCs) in water changes as weathering progresses, affecting its bioavailability and toxicity, thus leading to varying pressures and responses among aquatic microorganisms (King et al., 2014; Zito et al., 2014; Tarr et al., 2016; Snyder et al., 2021).
Aromatic HCs are generally more susceptible to UV radiation and photodegradation than aliphatic HCs (King et al., 2014; Zito et al., 2014; Bacosa et al., 2015a). Sunlight not only alters the chemical structure of oil but also produces significant amounts of singlet oxygen, hydroxyl radicals, and various oxygenated compounds (Ray and Tarr, 2014; Zito et al., 2014) as well as increased reactive oxygen species (ROS) known to cause DNA, protein, and lipid damage (Sinha and Häder, 2002), that can indirectly impact marine microbial communities (Bertilsson and Widenfalk, 2002; Matallana-Surget and Wattiez, 2013). Some of these UV-induced photoproducts are even more toxic to microbial communities than the original compounds (Lacaze and Villedon de Naïde, 1976; Vaughan et al., 2016; Bridges et al., 2018).
While sunlight intensifies the adverse effects of crude oil on diversity in the GoM and contributes to changes in community structure (Bacosa et al., 2015b), our knowledge of how microbial degradation of HCs changes under these GoM conditions remains limited. The specific metabolic pathways affected during oil degradation under high solar radiation doses are yet to be fully characterized.
1.4 The combined effects of three factors: crude oil, Corexit and solar radiation
The combined impact of HCs, dispersant and solar radiation on microbial communities has received limited attention. It was first reported that sunlight exposure increased chemical toxicity by a staggering factor of 30 in an equal mixture of weathered crude oil and Corexit 8666 (Lacaze and Villedon de Naïde, 1976). More recent research has shed light on the pivotal role of solar radiation in shaping the response of GoM bacterial communities to oil, Corexit® EC9500A, or their combination (Bacosa et al., 2015b). While it is worth noting that solar radiation may amplify the toxicity of Corexit through photooxidation processes (Lacaze and Villedon de Naïde, 1976; Bacosa et al., 2015b), sunlight did not enhance degradation of dispersed HCs (Bacosa et al., 2015a). In summary, sufficient light exposure promotes dispersion and dissolution of crude oil, however it can also increase the toxicity of HCs and Corexit. To date, the combined effects of solar radiation, oil, and dispersant have not been thoroughly examined in terms of protein regulation, which is crucial for understanding microbial activity and responses in the context of an oil spill accident.
1.5 (Meta)proteomics methods to study the molecular effects of oil spill on marine microorganisms
The great power of proteomics is the identification of enzymes and proteins expressed by organisms, which provides accurate biochemical responses to oil/dispersant inputs and physical effects. Proteomic studies of individual oil-degrading bacteria gave an insight into proteins involved in the degradation of a range of HC-alkanes (Sabirova et al., 2006; Feng et al., 2007). The proteome of Alcanivorax borkumensis showed that alkanes were degraded by several routes involving AlkB hydroxylases, a putative Flavin-binding monooxygenase and P450 cytochromes (Sabirova et al., 2006). This organism adapted to the influx of alkanes in many ways including inducing glyoxylate bypass and gluconeogenesis routes to adapt the cell to produce key cellular precursor metabolites directly from the fatty acids produced by alkane oxidation (Sabirova et al., 2006). Feng and colleagues gave an account of the genome and proteome of Geobacillus thermodenitrificans NG80-2 and how it can adapt in oil reservoirs due to it possessing genes for a broad range of energy sources (Feng et al., 2007). The proteome revealed a long-chain alkane degrading pathway with a key enzyme LadA which could be used for treating environmental oil pollutions and biosynthesizing complex molecules (Feng et al., 2007). It was also found to have the ability to carry out denitrification which is a survival advantage in an oil reservoir.
Microorganisms function as a consortium within their natural habitat, owing to cell-cell communication and syntrophism. Therefore, focusing on one or few microorganisms in controlled conditions could never predict the microbial response that would occur in the natural environment (Little et al., 2008). Metaproteomic approaches aim to identify the complex microbial protein profile within a given environment at a specific point of time. This gives metaproteomic approaches a unique advantage over other ‘omics’ approaches, as they can determine the functional expression of proteins (Rudney et al., 2015), and thus enables to further the understanding of the relationships between microbial diversity, functioning of an ecosystem and the networks of interactions (Allen and Banfield, 2005; DeLong, 2005). Until now, only one metaproteomics study has successfully described a list of proteins involved in the response to oil (Bargiela et al., 2015). Since then, environmental metaproteomics has been greatly improved thanks to development of high resolution mass spectrometry, protein search database construction, and bioinformatic tools to accurately annotate and link the taxonomy and functions of identified proteins, limiting the well-known protein inference issue (Matallana-Surget et al., 2018; Géron et al., 2019; Saito et al., 2019; Werner et al., 2019).
The objective of this study is to examine, for the first time, the combined impact of oil, Corexit® EC9500A, and solar radiation on marine microbial communities using a comparative metaproteomic technique. We used the most up to date methodological workflow and provide unique insights into the interactive effects of sunlight, oil, and Corexit-dispersant on natural bacterial communities in GoM. Identifying the functional responses of the microbial community, and the degradation pathways observed in situ provides essential metadata for bioremediation strategies. Our approach goes beyond mere descriptive analysis of microbial diversity and taxonomic structure; it provides specific details about which bacteria are involved in various biogeochemical processes and the pathways of those key players. This metaproteomics study introduces new proxies that can enhance the oil spill model for more accurate risk identification. Additionally, considering the importance of cleaning up accidental oil spills, our approach allows for the prediction of key proteins involved in bioremediation, reducing the need for chemical use and lowering associated costs.
2 Materials and methods
2.1 Mesocosms incubations and sample collection
Surface waters were collected off Pensacola Beach, FL (30.29° N; 87.28°W) in July 2016 and incubated in 70L-mesocosms with amendments of crude oil with and without Corexit-dispersant for 24 hours under sunlight as well as in the dark condition. Before addition to the mesocosms, sampled indigenous communities of microorganisms were filtered through 5µm filters, to remove grazing zooplankton and larger phytoplankton. The 8 mesocosm conditions were incubated in Tedlar® PVF bags, DuPont, with dark condition bags covered in black plastic sheeting before incubation, and “full sun” (UV+PAR) conditions in Tedlar® bags left uncovered, allowing for approximately 40-60% transmission of wavelengths 300-400 nm and approximately 60% of radiation transmitted in wavelengths >400 nm. For each dataset consisting of dark or sunlight-exposed variants for seawater controls, other condition variables included oil-only treatments, dispersant-only treatments, and combined oil and dispersant treatments. A Water Accommodated Fraction (WAF) was prepared using Macondo oil, a light crude oil also known by its well designation MC-252 surrogate (from the Deepwater Horizon oil spill), at a concentration of 1%, with sterile seawater as previously described (Headrick et al., 2023). This WAF was subsequently added to the mesocosms treated with oil. Oil-only mesocosms had a total concentration of approximately 1.1-2.2 x 10-3% Macondo oil by volume. Corexit-only mesocosms contained 0.1% Corexit by volume. The combined treatment mesocosm had both 1.1-2.2 x 10-3% Macondo oil and 0.1% Corexit by volume. Mesocosm bags were either transparent (uncovered) to allow sunlight exposure, or opaque for dark conditions. All mesocosms were incubated in surface waters of Santa Rosa Sound (30.34° N; 87.16° W) at the US EPA Gulf Ecology Division laboratory for 24 hours, of which “Light Condition” mesocosms were exposed to solar radiation for approximately 12 hours. For 16S amplicon analysis, a total of 500 mL to 2 L of water from each mesocosm were filtered through a 0.22 µM Millipore Durapore GVWP filter then placed in cryo-tubes with sterile 0.1 mm silica and 0.5 mm glass beads and sucrose buffer (20 mM EDTA, 400 mM NaCl, 0.75 M sucrose, 50 mM Tris-HCl pH 9.0). For metaproteomics analysis, large volumes of seawater are required, as detailed previously (Matallana-Surget et al., 2018; Géron et al., 2019). Consequently, the complete volume of each bag (~60 to 68L) was filtered to guarantee a high-quality proteomics dataset. In this process, microbial biomass was collected through sequential in-line filtration of the seawater sample using two successive 142mm PES-filters with the following cut-off sizes: 0.8μm and 0.2μm. All filters used for both 16S rRNA analysis and Metaproteomics were flash frozen in liquid nitrogen and stored at -80°C until further analyses (Figure 1).
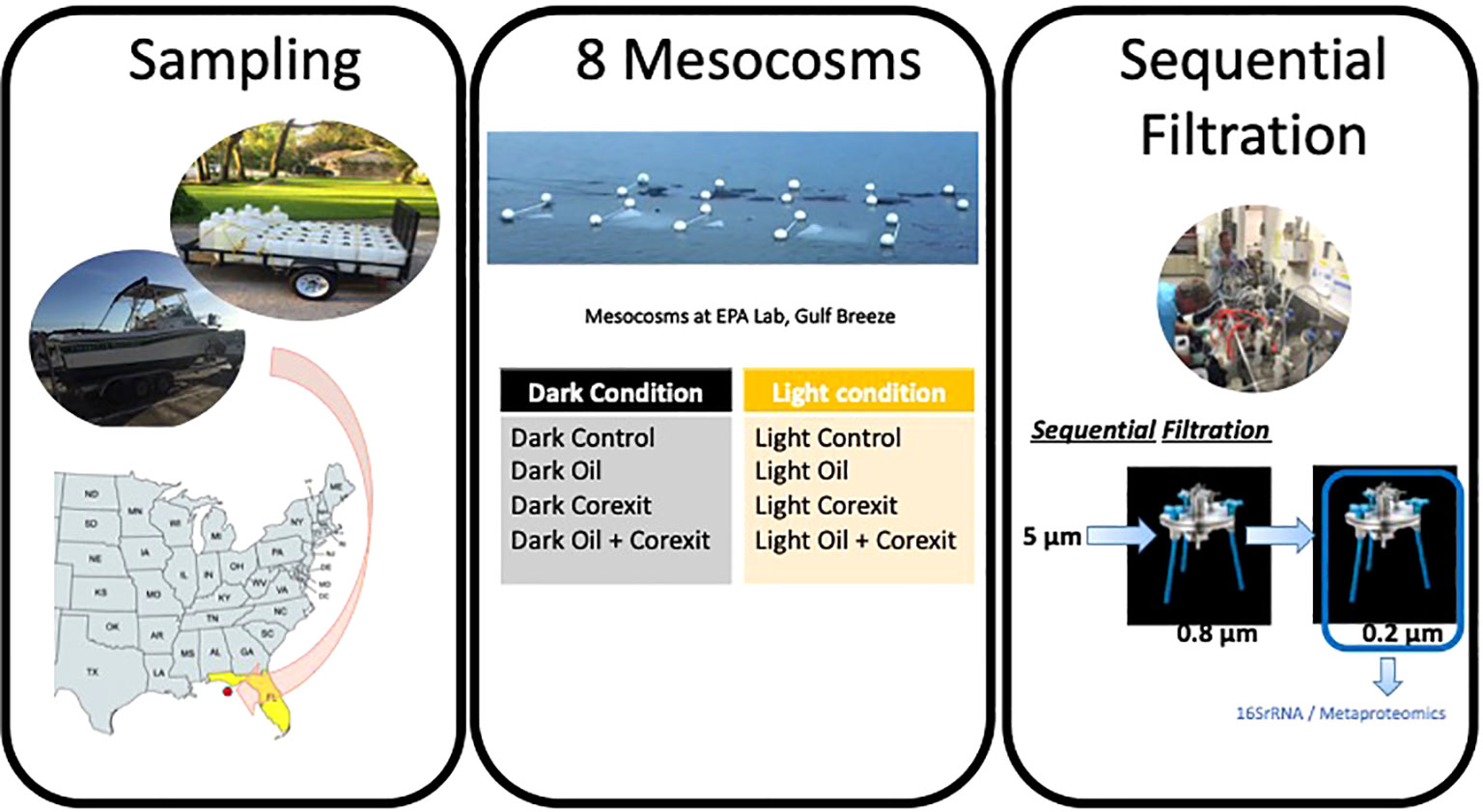
Figure 1 Sampling and mesocosm setup at the Gulf Ecology Division- Environmental Protection Agency (EPA), Pensacola Beach, FL.
2.2 DNA extraction and processing
Biomass on filters was homogenized in an Omni BeadRuptor at 2,000 rpm for two 1 minute cycles and subjected to 3 freeze thaw cycles (-80°C and room temperature). Samples were incubated with lysozyme (1.5 mg/mL final concentration) for 90 minutes at 37°C and then proteinase K (3 mg/mL final concentration) at 50°C for 90 minutes. NaCl was added to a final concentration of 0.5 M. The CTAB based EZNA Mollusc Kit (Omega) was utilized to further extract and purify the DNA, following the manufacturer’s instructions. Purified DNA concentrations were determined, and each then diluted to approximately 5 ng/uL. Technical duplicates from the same filters were analyzed for the 16S amplicon analysis. Subsequently, the samples were sequenced at the University of Illinois at Chicago’s DNA core facility, where partial 16S rRNA genes were amplified with the universal prokaryotic 16S rRNA gene primers 515F/926R (Walters et al., 2015) and sequenced on the Illumina Miseq (2x300 cycle). Illumina sequences were analyzed with QIIME v1.9.1 (Caporaso et al., 2011). Paired ends were joined with the fastq-join method. Sequences were grouped into operational taxonomic units (OTUs) and putative chimeras were removed with UPARSE (Edgar, 2013). Singletons were removed before further analysis. Taxonomic classifications for OTU sequences were determined using QIIME’s UCLUST approach and Greengenes v.13_18 reference and taxonomic mapping files. Sequences were aligned with the NAST alignment algorithm (Caporaso et al., 2011). Phylogenetic trees were constructed using the FastTree method. Beta diversity was estimated using UniFrac weighted and unweighted metrics (Hamady et al., 2010) and visualized with Principal Component Analysis (PCA).
2.3 Protein extraction and sample concentration
To enable protein extraction, the 142mm PES filters, containing the prokaryotic biomass, were cut into smaller fragments with sterile scissors and forceps, and placed into 50mL-Falcon tubes. A volume of 9mL of Lysis Buffer (covering the filter fragments) was added to each tube (6M urea, 2M thiourea, 10Mm HEPES). Cells were mechanically broken by sonication on ice (amplitude 60%, 0.5 pulse rate) and subsequently centrifuged at 16,000 × g at 4°C for 35 min. To remove particles that did not pellet during the centrifugation step, we filtered the protein suspension through a 0.22 μm syringe filter and transferred into a 3 kDa cutoff Amicon Ultra-15 filter unit (Millipore) for protein concentration. Proteins were precipitated with cold acetone overnight at −80°C, with an acetone/aqueous protein solution ratio of 4:1. Following the overnight acetone precipitation, samples were centrifuged at 15,000g at 4°C for 20 minutes to form protein pellets.
2.4 Bradford assay and gel electrophoresis
Concentrated protein pellets were re-suspended in a solution of 2M urea and 100mM K2HPO4 for protein quantitation. Total protein concentration was determined by a Bradford assay, using the Bio-Rad Protein Assay kit (Bio-Rad, Hertfordshire, United Kingdom) according to the manufacturer’s instructions, with bovine γ-globulin as a protein standard. Following protein quantification, the SDS-PAGE of 30μg of protein mixtures for each condition was conducted using 4–12% precast Bis-Tris polyacrylamide mini-gels (NuPage, Thermo Fisher Scientific). The protein bands were visualized with staining using the Imperial Protein Stain (Thermo Fisher Scientific) according to the manufacturer’s instructions. The gel electrophoresis was used to assess the accuracy of the Bradford protein concentration prior to gel-free proteomic shotgun analysis, described below.
2.5 Gel-free shotgun metaproteomic and liquid chromatography tandem mass spectrometry analysis
Based on the results of the Bradford assay and the NuPAGE gels, acetone precipitations were further performed on a total of 200μg of proteins for the eight conditions, using cold acetone precipitation at a ratio of 1:4 of sample/acetone. The samples were then re-suspended in a suspension buffer consisting of 1M Guanidine HCL and 25mM NH4 HCO3, and then reduced with 25mM dithiothreitol (DTT) for 30 minutes at 60°C and alkylated with 50mM iodoacetamide for 30 minutes at 25°C. Proteins were digested with a solution of trypsin at a ratio of 1:25 (enzyme: substrate) and incubated overnight at 37°C. Trypsin digest was then stopped with 1μl 5% (v/v) formic acid. Tryptic peptides were analyzed by liquid chromatography tandem mass spectrometry. Peptide samples were analyzed on an ultra-high-performance liquid chromatography–high-resolution tandem mass spectroscopy (UHPLC-HRMS/MS) system, including a Eksigent NanoLC 400 and AB SCIEX TripleTOF 5600. Two micrograms of peptides were analyzed using acquisition parameters previously reported (Géron et al., 2019), and MS/MS spectra were acquired with the instrument operating in data-dependent acquisition (DDA), with micro injection (75 min LC separation) modes. The metaproteomic raw data are available in the iProx public platform (Project ID: PXD048022).
2.6 Protein searches database construction and protein identification
Protein searches were performed using ProteinPilot (ProteinPilot Software 5.0.1; Revision: 4895; Paragon Algorithm: 5.0.1.0.4874; AB SCIEX, Framingham, MA, United States) (Matrix Science, London, United Kingdom; v. 2.2). Paragon searches were conducted using LC MS/MS Triple TOF 5600 System instrument settings. Other parameters used for the search were as follows: Sample Type: Identification, Cys alkylation: Iodoacetamide, Digestion: Trypsin, ID Focus: Biological Modifications and Amino acid substitutions, Search effort: Thorough ID, Detected Protein Threshold [Unused ProtScore (Conf)]>: 0.05 (10.0%).
The OTU tables obtained by the 16S rRNA reads were used to create the protein search databases for the analysis of the 8 metaproteomes. Those fasta files were generated with mPies v 0.9, our recently in house developed mPies program is freely available at https://github.com/johanneswerner/mPies/(Werner et al., 2019), following the same TAX-DB workflow, as previously described in our recent methodological publications (Géron et al., 2019; Werner et al., 2019). Taxonomic classification based on the 16S amplicon sequencing for this metaproteomics workflow is only needed as a guide for the phylum, class, or order level, as previously described (Géron et al., 2019; Werner et al., 2019). The database searching with Protein Pilot was done against 8 distinct databases (one for each condition). A FDR threshold of 1%, calculated at the protein level, was used for each protein searches. Proteins identified with one single peptide were validated by manual inspection of the MS/MS spectra, ensuring that a series of at least five consecutive sequence-specific b-and y-type ions was observed.
Identified proteins were further annotated using mPies as previously described in our former papers (Géron et al., 2019; Werner et al., 2019). For taxonomic and functional annotation, mPies used Diamond to align each identified protein sequences against the non-redundant NCBI DB and the UniProt DB (Swiss-Prot), respectively, and retrieved up to 20 best hits based on alignment score (>80). For taxonomic annotation, mPies returned the LCA among the best hits via MEGAN (bit score >80) (Werner et al., 2019). For functional annotation, mPies returned the most frequent protein name, with a consensus tolerance threshold >80% of similarity among the 20 best blast hits. Proteins annotated with a score below this threshold were manually validated. Manual validation was straightforward as the main reasons leading to low annotation score were often explained by the characterization of protein isoforms or different sub-units of the same protein.
3 Results
3.1 Taxonomic composition of the active microbial communities
This study presents extensive datasets, encompassing thousands of proteins identified under eight different treatments. To ensure clarity, we will primarily concentrate on both the taxonomic composition and functions of microbial communities assessed through metaproteomics, representing active communities in the mesocosms. It is essential to emphasize here that we used 16S rRNA gene reads to construct a Protein Search Database for our metaproteomics workflow (Géron et al., 2019; Werner et al., 2019). As a result, the 16S diversity obtained in this study will only be briefly discussed in terms of treatment clustering.
A Principal Component Analysis (PCA) of the microbial diversity based on the 16S rRNA sequences revealed three major clusters of microbial communities: Control, Corexit, and Oil alone. Community compositions of control samples were the closest to the initial communities (T0), regardless of the presence of sunlight (Figure 2). Interestingly, all samples treated with Corexit grouped together including both treatments namely: Corexit alone and Oil and Corexit. Furthermore, subclusters emerged among mesocosms exposed to the dispersant, suggesting that sunlight influenced microbial diversity when combined with Corexit.
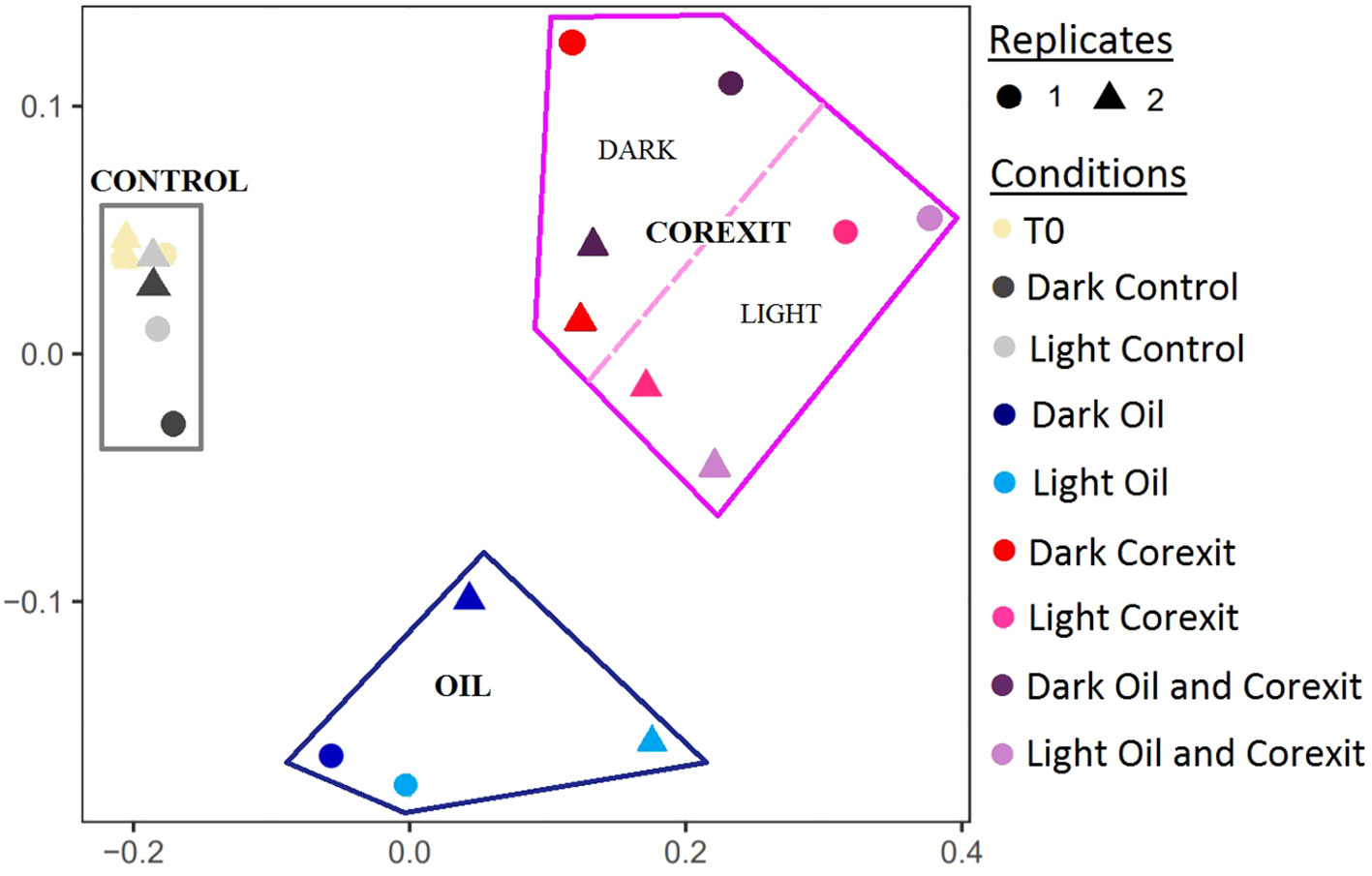
Figure 2 PCA analysis of the OTU composition based on the 16sRNA reads. Circles (•) and triangles (▴) represent first and second replicates of the experiment, respectively. Conditions are shown in different colors, with conditions under sunlight represented by lighter colors.
The taxonomic composition obtained by Metaproteomic allows for the characterization of active taxa and will be referenced as such throughout the manuscript. Identified proteins were annotated using mPies (Werner et al., 2019). As demonstrated previously in our former metaproteomics papers (Géron et al., 2019), the proportion of annotated proteins decreased with lowering taxonomic ranks as follows: Phylum (98%) > Class (94%) > Order (86%) > Family (79%) > Genus (52%) > Species (8%), respectively (Figure 3). This is due to the well-known Protein Inference limitation, extensively discussed in our former papers (Géron et al., 2019; Werner et al., 2019). Proteins characterized at lower taxonomic ranks, such as genus or species, provide an incomplete view of community composition. To gain a more accurate understanding of community dynamics, data were analyzed at multiple taxonomic ranks.
Proteins of Proteobacteria dominated across all conditions, representing on average 86% of all identified prokaryotic taxa (Figure 4A). Notably, Actinobacteria protein abundances decreased in Corexit treatments compared to control treatments. At the order level, the most abundant taxa, namely Alteromonadales and Rhodobacterales from Gammaproteobaceria and Alphaproteobacteria, respectively, exhibited higher relative abundances in experimental treatments compared to controls (Figures 4B, C). A shift in community structures was observed between controls and oil and/or Corexit-exposed mesocosms, as shown in Figures 4B, C. In contrast, Oceanospirillales appeared more abundant in control conditions than in chemically treated mesocosms, while Vibrionales thrived in the presence of Corexit relative to controls (Figure 4B). Sphingomonadales were abundant in control conditions but less represented in treated conditions.
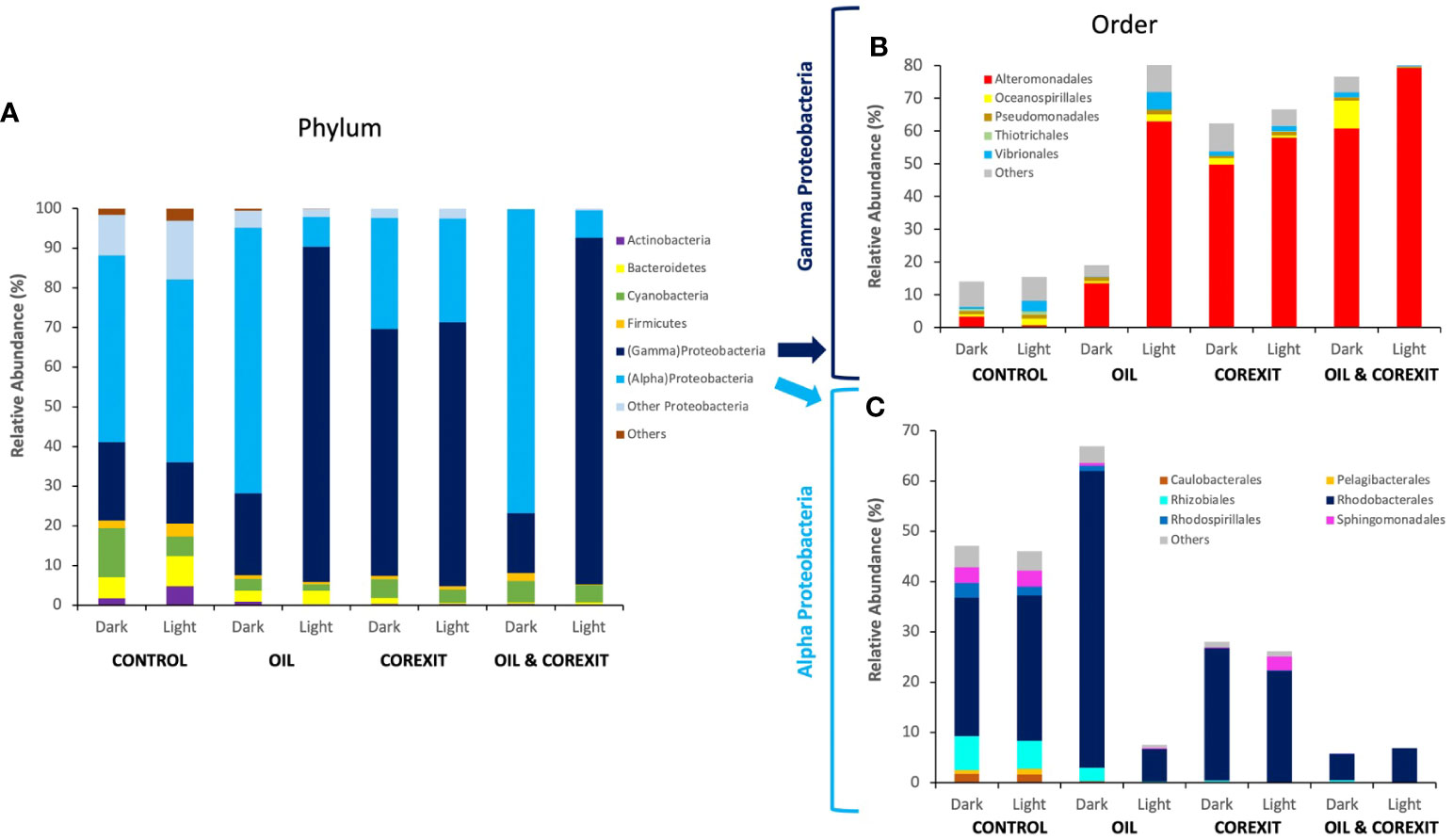
Figure 4 Bacterial community structure across different mesocosm experiments, at phylum rank (A), at order level for Gammaproteobacteria (B) and Alphaproteobacteria (C). The relative abundance (RA) is determined from proportions of different taxa present (identified via taxonomic protein annotation; ≥80% confidence) based on the total number of peptide spectra for each metaproteome. Taxa assigned as “Unknown” and the least abundant taxa representing an average of <0.5% of peptide spectra were categorized as “Others”.
3.1.1 Effect of individual treatments on microbial taxonomy
At the phylum (Figure 4A) and order level (Figures 4B, C), sunlight exposure had minor effects on control treatments. While the relative abundance of total Proteobacteria (alpha and gamma) remained largely unaffected by light treatment in each condition, significant variations in the alpha/gamma Proteobacteria ratio emerged in oil-containing treatments (Figure 4A).
Gammaproteobacteria showed minimal variations at the order level, while Alphaproteobacteria remained relatively stable (Figures 4B, C). Cyanobacteria, primarily abundant in control conditions, were more prevalent in the absence of sunlight. Overall, sunlight alone had a limited impact on surface water communities compared to contaminants, as observed in Figure 2.
In contrast, oil substantially altered microbial community structures. In the absence of light, oil favored Alphaproteobacteria, particularly Rhodobacterales (Figure 4B). Rhodobacteriales proteins constituted over half of the metaproteome in the Dark Oil treatment, primarily represented by unidentified genera within Rhodobacteraceae. The relative abundance of Gammaproteobacteria including known oil-degraders, such as Acinetobacter, Pseudomonas, Alcanivorax, and Marinobacter were sparsely abundant in the oil treatment (Supplementary Data Sheet 1). Furthermore, oil inhibited Oceanospirillales, although none of the inhibited members were previously reported as oil degraders. Alteromonas, a well-known oil-degrading Gammaproteobacteria, was the sole group that thrived in response to oil treatment. Oil also led to a 10-fold reduction in Cyanobacteria protein expression, primarily Synechococcus, indicating their susceptibility to oil (Figure 4A). Verrucomicrobia, Planctomycetes, and Actinobacteria were also reduced in the presence of oil, while Flavobacteriaceae, including Flavobacterium, experienced an increase (Supplementary Information 1).
Corexit induced significant modifications in microbial communities, favoring Gammaproteobacteria and increasing their abundance threefold in Dark Corexit conditions. Gammaproteobacteria dominated in all Corexit-containing conditions, while other Proteobacteria classes decreased to below 2% (Figure 4A). Bacteroidetes, including Flavobacteria and unidentified members of Flavobacteriaceae, Cryomorphaceae, and Saprospiraceae, as well as Actinomycetales (Actinobacteria) were notably suppressed by Corexit (Supplementary Data Sheet 1).
3.1.2 Effect of combined treatments on microbial diversity
At both phylum and order levels, combined contaminants closely mirrored Corexit alone, supporting the 16S diversity results (Figure 4). Gammaproteobacteria thrived in Corexit treatments, with similar trends at the Order level (Figures 4B, C) and alignment at family and genus levels, such as Alteromonadaceae and Thalassomonas. This trend was also observed in Alphaproteobacteria whose structure, despite a reduction in unidentified Rhodobacteraceae genera, was similar than in the Corexit treatments (Figure 4). Furthermore, as in Corexit treatments, the combined contaminants reduced the abundance of every other phylum below 3%, besides Cyanobacteria in the absence of sunlight. Cyanobacteria were almost equally represented in both Dark Corexit-containing treatments, and they were indistinguishable at the order level. The distinct, relative abundances observed for Alphaproteobacteria, Gammaproteobacteria, and Cyanobacteria concur that Corexit was the driving factor for the variance in combined contaminant treatments.
The combination of contaminants and sunlight induced significant variations in microbial communities in Light treatments versus their Dark counterparts. Gammaproteobacteria were exceptionally efficient in coping with these combined effects, as their numbers increased substantially (Figure 4B). The positive effects of sunlight were particularly evident on Alteromonas and other unidentified members of family Alteromonadaceae. On the contrary, Alphaproteobacteria declined in the presence of light and oil, mainly due to the inhibition of Rickettsiales and unspecified Alphaproteobacteria. Light and Corexit favored both Alpha- and Gammaproteobacteria, particularly when combined (93% microbial abundance; Figure 4). Additionally, sunlight and dispersant inhibited Synechococcus (Cyanobacteria; Supplementary Information 1). Light and chemicals negatively impacted other phyla, notably in Light Oil & Corexit (<2% abundance). Bacteroidetes responded positively, increasing Flavobacteriaceae, Marinomonas, Vibrio, but reducing Marinobacter (absent in the Light condition). This underscores solar radiation’s role in shaping dispersant-containing community structures. Sunlight, combined with contaminants, influenced active community structures (characterized by metaproteomics), although less markedly than in 16S analysis of Corexit-containing treatments.
3.2 Functional trait variations in response to oil, Corexit and sunlight
In both the Dark and Light conditions, the total number of proteins was 1792 and 1383, leading to 573 unique protein functions. A small core metaproteome, consisting of only 12 proteins, was found to be common in all metaproteomes, and these proteins were primarily ribosomal proteins, constitutively expressed chaperones, ATPase, flagellin, and transporters (Supplementary Information 2; Venn Diagram Data Sheet). Among all conditions, the Corexit-containing conditions exhibited the highest diversity of unique protein functions, regardless of the light treatments, with the lowest diversity observed in the control treatments. This demonstrates the active response of microbial communities to chemicals, including both oil and Corexit. A PCA analysis of the metaproteomics functional diversity mirrored the clustering observed in the 16S rRNA analysis (Figure 5). Notably, Corexit induced similar protein regulation compared to combined Oil and Corexit treatments, irrespective of light conditions.
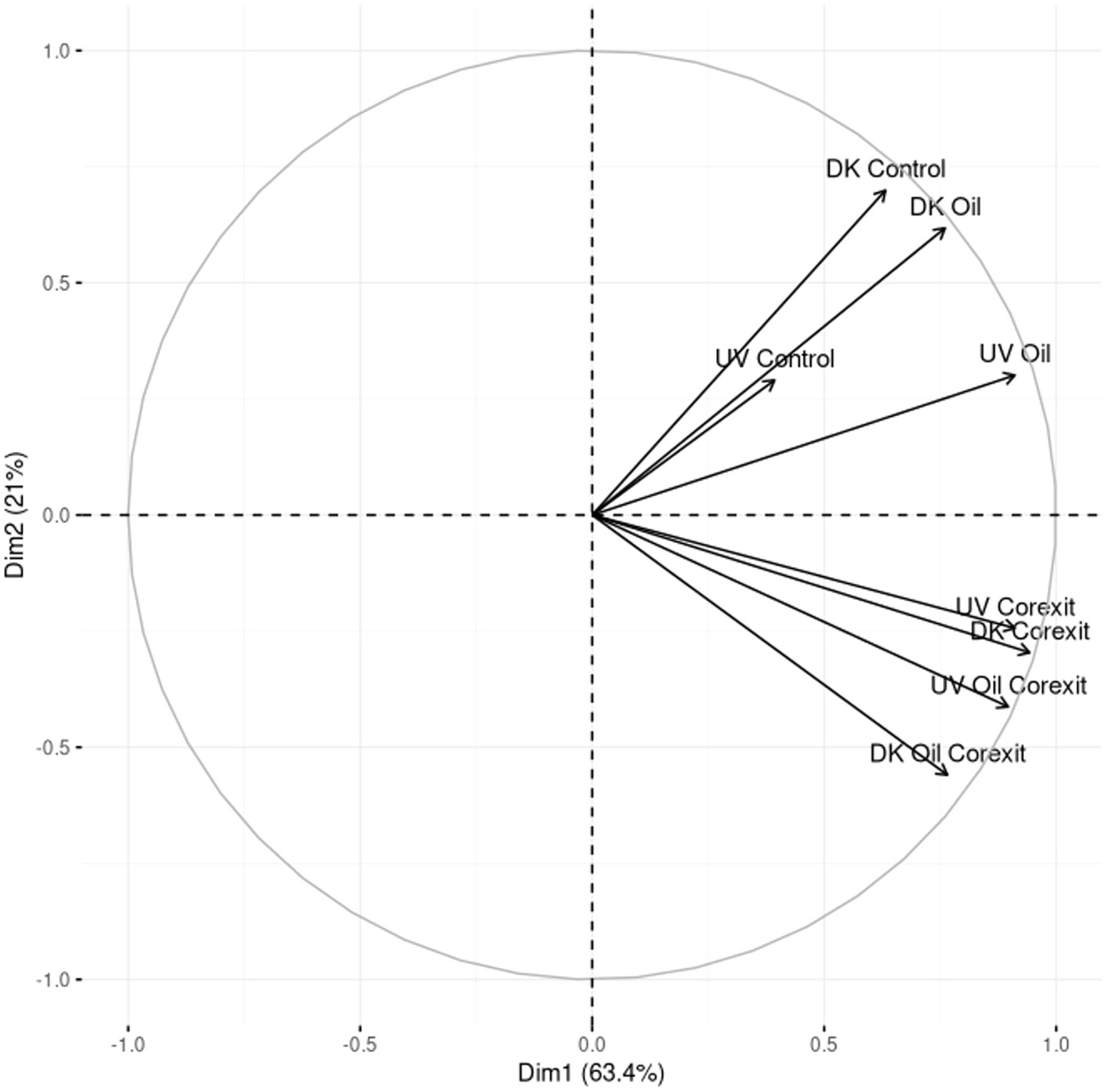
Figure 5 PCA analysis of the functional profiles obtained by metaproteomics in the different treatments.
All non-redundant protein functions were classified into functional groups for all conditions as presented in Figure 6. The most consistently abundant functional proteins were involved in the metabolism of nucleic acids and protein synthesis (Figure 6; Supplementary S1). The vast majority of these were involved in transcription or translation (averaging 25% of total identified peptides for each condition), such as transcriptional regulators (15%) or ribosomal protein (7%).
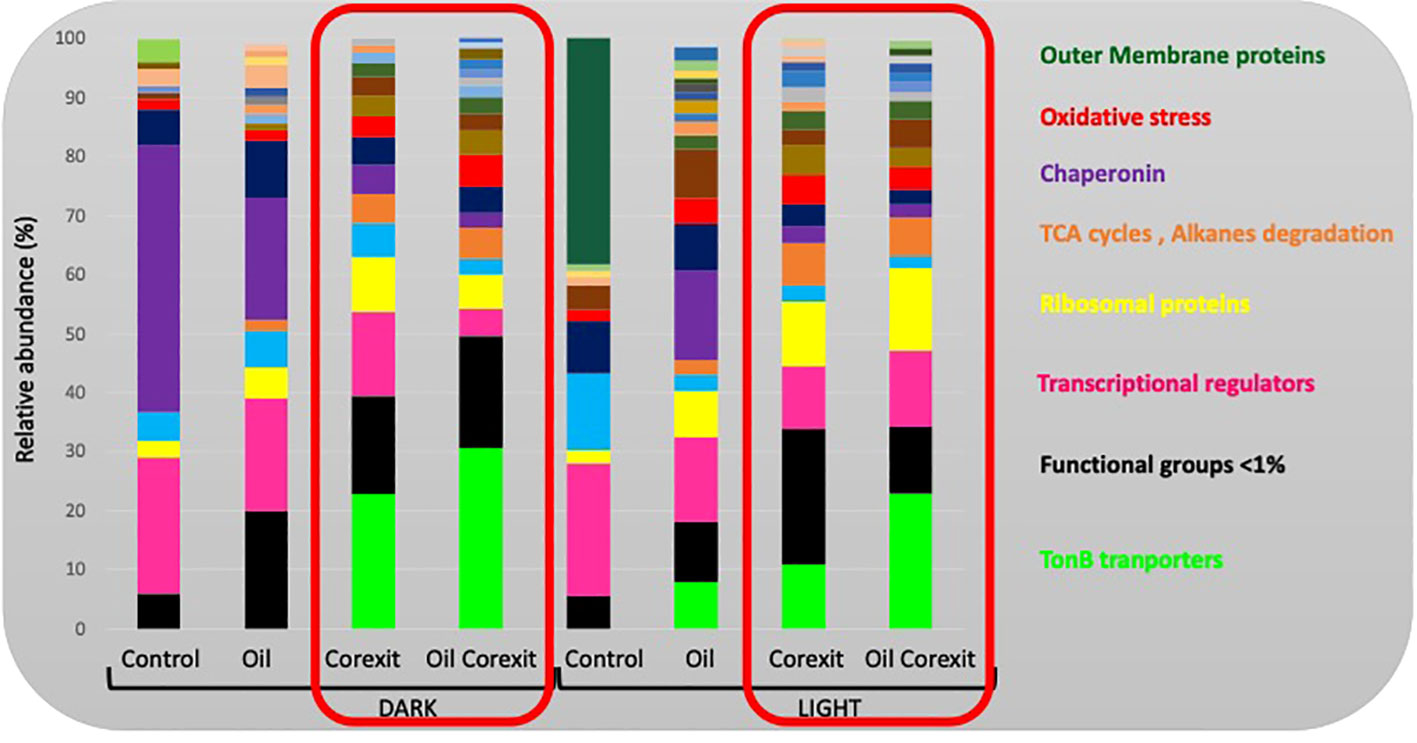
Figure 6 Relative functional composition for each condition. Values represent the proportion of peptides within different related functional groupings. Unknown functions, and functional categories represented by <1% of total detected peptides were classed as “Other”. Functional groups discussed in this paper are provided in the margin, but details of all functional groups are provided as Supplementary Information in Supplementary Figure S1.
Transporters were the next most abundant group, with slightly higher expression in all Corexit-exposed conditions (Supplementary Information 2). TonB-dependent transporters increased in oil and/or Corexit conditions (30% in the dark combined condition), while ABC transporters showed variable abundance across conditions (Figure 6). Amino acid transport was more abundant in Light Control than Dark Control but less abundant in irradiated conditions. Nitrate and dicarboxylate ABC transporters were upregulated in Dark Corexit and/or Oil treatments. Proteins associated with electron transport and transfer processes were consistently expressed, with the highest abundance in Oil-only conditions (11.01% and 8.34%, respectively), and the lowest in Corexit-exposed conditions (3.57-5.46%). These proteins were primarily associated with oxidative phosphorylation, including cytochromes (~5.9%).
Chaperonins were most abundant in the Dark Control (~45%) but absent in the Light Control. They were more abundant in response to oil-only exposure than Corexit treatments, and less abundant in UV-exposed conditions. Proteins associated with oxidative stress responses were more abundant in Corexit-exposed conditions compared to controls (Figure 6).
Carbon metabolism was upregulated in all Corexit and/or oil-exposed communities, in both dark and light conditions. Pyruvate metabolism, including the tricarboxylic acid (TCA) cycle, contributed significantly (~4.4%) (Figure 6). Carbon fixation processes were generally more abundant in treated dark conditions compared to UV. In combined treatments, the pentose phosphate, carbon fixation, and Entner-Doudoroff pathways were all overrepresented in the dark. Other less abundant proteins included amino and nucleotide sugar metabolism, polysaccharide biosynthesis, methylotrophic pathways, and other HC-degradation (Supplementary Figure S1). Photosynthesis-related proteins were more abundant in dark conditions, with the highest expression in the Dark Control (5.31%). They were also well-expressed in dark treatment conditions, particularly in oil-only and combined treatments, mainly for light-harvesting phycobiliproteins (~0.73%) and CO2-concentrating mechanisms associated with Cyanobacteria (~0.56%). On average, 1.2% of identified proteins were associated with nitrogen metabolism, 0.59% with phosphorus metabolism, and 0.42% with iron metabolism, with no clear pattern observed. Proteins associated with sulfur metabolism were identified in Corexit-associated conditions only.
Motility-associated protein responses varied across different treatment conditions, with flagellar/motility proteins overrepresented in Light control or Oil-only conditions (~3.47% overall). Chemotaxis-associated proteins were absent in control conditions but present (~1.05%) in Oil and/or Corexit-treated conditions.
3.3 Who’s doing what? Linking taxonomy and function of key players during an oil spill
Control conditions exhibited a diverse taxonomic composition enriched with essential housekeeping functions such as like photosynthesis, respiratory chain proteins, transcriptional factors, transporters, and chaperonins (Supplementary Heatmaps 1-2). Conversely, the Dark Oil treatment exhibited the most extensive diversity, both in terms of taxa and non-redundant protein functions (Supplementary Heatmaps 3-4). Interestingly, Corexit and Oil+Corexit conditions displayed similar diversity patterns (Supplementary Heatmaps 5-8). In the Oil and/or Corexit treatments, the majority of identified protein functions were assigned to Alteromonas, and the detailed regulations for this genus are presented below for the combined treatments.
In the combined oil and Corexit treatment, a wide diversity of functional categories was assigned to Alteromonas - a versatile responder under combined treatments (Figure 7). Highly expressed proteins included those related to TonB-dependent transporters, chemotaxis, motility, ribosomal proteins, aspects of carbon metabolism, and cellular defense mechanisms. Outer membrane proteins, various forms of amino acid, peptide and protein metabolism, and respiratory chain protein ATP synthase also displayed high expression. Generally, proteins were more abundant in the dark than in UV conditions, except for transcriptional and translational regulators, which were more prevalent in the light.
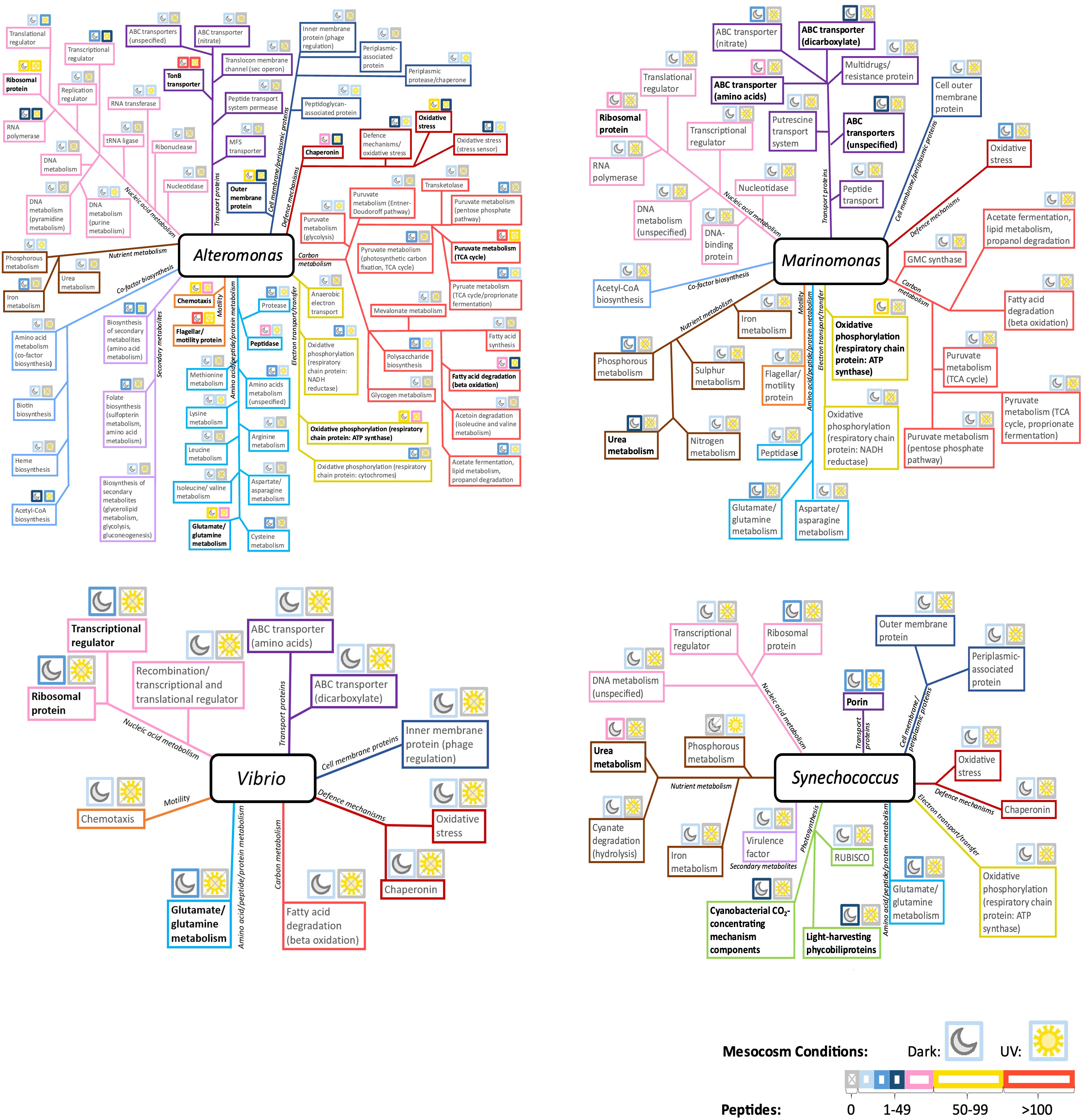
Figure 7 Distribution of identified protein functions in Alteromonas, Marinomonas, Vibrio and Synechococcus, in the combined oil and Corexit treatment conditions. Above each function, moon and sun icons represent the Dark and Light conditions, respectively. The border color for each icon indicates peptide abundance, and thus protein expression, within these conditions. Highly expressed protein functions are emphasized in black boldface. MFS, Major facilitator superfamily of transporter; GMC synthase, glucose methanol choline synthase.
Marinomonas was one of the most active genera present in the dark condition but was absent from mesocosms exposed to sunlight. The most represented protein functions were involved in oxidative phosphorylation, various ABC transporters, nutrient metabolism, and ribosomal protein (Figure 7).
Vibrio, which remained active not in the dark but in the light conditions, exhibited a diverse range of protein functions (Figure 7). Among these functions, transcriptional regulators, ribosomal proteins, and amino acid metabolism, particularly related to glutamate and glutamine, showed the highest levels of expression.
Synechococcus harbored diverse protein functions (Figure 7). These were primarily those involved in photosynthesis, and in nutrient metabolism. Most functions were expressed in the dark, except for porins and phosphorus metabolism. Carbon metabolism was not characterized in Synechococcus in either condition. Synechococcus displayed few proteins involved in cellular defense mechanism as shown in Figure 7.
4 Discussion
The 16S rRNA analysis provides insights into complex microbial community composition, while metaproteomics allows us to decode microbial functioning and identify the most active and abundant taxa. Both methods, 16S rRNA and metaproteomics, revealed that the chemicals used in this study—oil and dispersant—significantly influenced microbial composition and activities. Notably, the taxonomy identified through 16S analysis closely mirrored the results obtained from metaproteomics, indicating that the most abundant bacterial groups were also the most active. Among these chemicals, Corexit (either alone or in combined treatments) exerted a more pronounced influence on community structure than oil treatment. Interestingly, while 16S diversity suggested that irradiance primarily affected the taxonomic composition of Corexit-treated communities, metaproteomic data revealed consistent impacts of sunlight exposure on the relative abundance of dominant taxa (including Alteromonadales, Oceanospirillales, and Synechococcales) and overall diversity. As expected, sunlight exposure impacted the relative abundance and diversity of Rhodobacteraceae, a major group that includes copiotrophic photoheterotrophs, confirming previous findings (Gómez-Consarnau et al., 2019).
4.1 Impact of the chemicals – oil and Corexit - on the composition of the active microbiome
Microbial communities respond rapidly to oil and/or Corexit in warm GoM surface waters (Doyle et al., 2018). In our study, our mesocosm experiment ran for 24 hours, thus assessing the initial responses to oil and/or Corexit exposure. Multiple studies reported a pattern of succession in oil-exposed communities in surface waters (Doyle et al., 2018; Valencia-Agami et al., 2019) and at depth (Mason et al., 2012; Techtmann et al., 2017; Marietou et al., 2018). A number of studies found that, similar to our results, diversity rapidly decreased in response to oil (Miralles et al., 2007; Orcutt et al., 2010; Mason et al., 2012; Bae et al., 2018) or Corexit treatment (Bacosa et al., 2015b).
Oil increased the abundance of HC-degrading microorganisms, but simultaneously reduced microbial diversity, thus disrupting the interdependence within the microbial consortia (Nyman, 1999). This disruption is primarily attributed to the toxicity exerted by oil on specific microbial clades, and a notable illustration of this is the suppression of Synechococcus and Pelagibacteraceae. This inhibition of Synechococcus was also supported by Liu and Liu (2013) and Bacosa et al. (2015b). Alphaproteobacteria adapted quicker to oil than Gammaproteobacteria. The increase in abundance in Rhodobacteriales is not surprising (Figure 4), since members of that order are known HC-degraders and were extensively implicated in DwH spill-related studies (Redmond et al., 2010; Dubinsky et al., 2013; Liu and Liu, 2013). Other studies observed the same results, serving as proof that Rhodobacteraceae members are crucial to oil degradation (Brakstad and Lødeng, 2005; Kostka et al., 2011). The greatest increase in diversity between the control and the oil samples were Vibrio species which were highly expressed in samples containing oil and/or Corexit in both the dark and UV conditions (Figure 4). Vibrio species are ubiquitous and abundant in marine ecosystems and are associated with HC degradation (Chakraborty et al., 2012). In addition, Alteromonas was the only oil-degrading Gammaproteobacterium whose abundance increased in Oil (Jin et al., 2012) (Figure 4). Bacosa et al. (2015b) observed that Alteromonas predominates over other degraders in the early stages of oil exposure, suggesting that the observed active microbiome, assessed by metaproteomics, is likely to change over time. Similarly, the relative abundance of Bacteroidetes was no greater in oil-only conditions than in controls. Although Bacteroidetes was found to proliferate following oil exposure, this occurred in experiments with longer incubation time (Valencia-Agami et al., 2019). Therefore, they appear to be largely uninvolved in earlier stages of crude oil degradation (Brakstad and Lødeng, 2005).
The dispersant played a significant role in shaping the community structure. Members of Alpha- and Gammaproteobacteria have the capability to utilize different Corexit compounds (Yoshida et al., 2006; Chakraborty et al., 2012), which may explain their success in Corexit-treated conditions. Vibrio species, known for their moderate tolerance to Corexit, were not adversely impacted (Bacosa et al., 2015b; Kamalanathan et al., 2018). In contrast, Corexit’s species-specific toxicity (Yoshida et al., 2006; Overholt et al., 2016), and its implication in causing phosphate stress (Edwards et al., 2011), can explain the Corexit-induced inhibition on Flavobacteria, other families of Bacteroidetes, Pelagibacteraceae, and Actinomycetales (Figure 4). Natural formation of marine-snow-like particles upon the addition of Corexit to seawater (Quigg et al., 2021) would also potentially lead to an increase in particle-associated copiotrophs like those in Rhodobacteraceae and Vibrio, naturally found at higher abundance associated with particles (Crump et al., 1999; Gómez-Consarnau et al., 2019). Interestingly, the Phylum Bacteroidetes generally appeared sensitive to Corexit but not oil and further research is needed to investigate the impact of Corexit on Bacteroidetes species.
In the combined chemical treatments, Corexit accounted for most of the community structure variation. Relative abundance of the orders Sphingomonadales and Betaproteobacteria, and of the phylum Firmicutes, was reduced across all treated conditions, indicating sensitivity to oil and to Corexit. In the PCA analyses of both 16sRNA and metaproteomics, Oil & Corexit treatments grouped together with Corexit alone, regardless of sunlight (Figures 3, 6). Even though the community structures were very similar in Corexit-containing conditions, their functioning was distinct. A hypothesis would be that the dispersant acts as a preferred carbon source (Kleindienst et al., 2015), thus delaying oil-induced variation. However, the microbial composition is anticipated to change over time in favor of an oil-driven structure, as Bacosa et al. (2015b) showed that in Dark conditions the concentrations of n-alkanes, PAHs, and alkylated PAHs did not decline prior to the 5th day, regardless of the addition of dispersant. This implies that more than a day’s cycle would be needed to notice changes induced by oil degradation. Pseudomonas was also highly expressed in all the samples containing oil and/or Corexit in both the dark and sunlight conditions (Figure 3). The order of Pseudomonadales, which was best represented in oil-treated conditions, is known to include HC-degraders (Lamendella et al., 2014), and to have some tolerance of Corexit (Kamalanathan et al., 2018), indicating its importance in an oil spill.
4.2 Sunlight amplifies oil and Corexit toxicity
Sunlight alone caused only minor alterations in the community structure (Figure 4), likely due to microorganisms’ efficient radiation coping mechanisms (Ruiz-Gonzalez et al., 2013). Previous studies demonstrated that the combination of oil and Corexit (Doyle et al., 2018) and additional sunlight exposure (Bacosa et al., 2015a; Bacosa et al., 2015b) led to a more significant reduction in diversity compared to oil alone. Combining oil, Corexit, and sunlight led to significant diversity reduction, suggesting strong interactions between contaminants and solar radiation. This interaction affected the relative abundance of Alpha- and Gammaproteobacteria, with Vibrio absent from light exposed combined treatments. Members of Alpha- and Gammaproteobacteria would outgrow other bacteria by utilizing UV-degraded contaminant compounds as a carbon source. This is supported by photooxidation playing a major role in oil weathering and degradation (Aeppli et al., 2014; Bacosa et al., 2015b). In this way, in our study, Vibrio was absent from the light exposed and chemical treatments. Interestingly, a related taxa Aliivibrio fischeri is known to be susceptible to toxic water-soluble photoproducts of crude oil (Griffiths et al., 2014).
Oceanospirillales, primarily represented by Marinomonas, appeared sensitive to phototoxic effects, as did Actinobacteria (Chakraborty et al., 2012; Bacosa et al., 2015b; Gómez-Consarnau et al., 2019). Actinobacteria has previously been found to represent a significant proportion of oil and Corexit-exposed microbial communities, especially in deeper waters rather than surface conditions in the GoM (Chakraborty et al., 2012).
4.3 Functional traits and their regulation to environmental stressors
Many of the identified functional protein categories were associated with essential cellular processes, including nucleic acid metabolism (including gene expression, replication, and repair), cellular defense mechanisms, carbon metabolism, amino acid metabolism, nutrient metabolism, transport proteins, and co-factors. While shifts in community structure were evident across conditions, changes in protein expression were generally less pronounced. Notably, proteins related to nucleic acid metabolism exhibited consistent high expression levels across all conditions. In this study, we also observed the presence of numerous proteins associated with hydrocarbon (HC) degradation pathways, consistent with previous analyses of samples from the DwH plume that revealed expression of proteins related to hydrocarbon degradation, carbon metabolism, and methanogenesis (Lu et al., 2012; Mason et al., 2012).
4.3.1 Hydrocarbon degraders: insights into their roles and adaptations to sunlight and Corexit
Alkane hydrocarbon (HC) degradation involves alkane monooxygenases to convert alkanes into primary alcohols. These primary alcohols are further oxidized to fatty acids by aldehyde and alcohol dehydrogenases, which can enter the beta-oxidation pathway (Van Beilen et al., 2003). Interestingly, we observed the expression of monooxygenases in presence of oil, Corexit or control treatment, for the mesocosms kept in the dark. The Alkane-1-monoxygenase was characterized in the Dark-Corexit treatment. Those results could indicate ongoing alkane degradation in those conditions, even in the control treatment possibly due to natural HC seepage in the GoM (Joye et al., 2014; Kleindienst et al., 2015). The monooxygenases were annotated as being expressed by Thioclava spp. (DK Corexit), Neptuniibacter (DK Control) and Clostridium Paraputrificum (DK Oil) (Supplementary Figure S1; Supplementary Information 2). Sulfur oxidizing bacteria, including Thioclava spp. were previously characterized in water flooded petroleum reservoir in China (Tian et al., 2017). Bacosa et al. (2021) also reported an increase of Neptuniibacter in presence of oil, making those bacteria novel potential candidate for environmental oil pollution treatment. Methylmalonyl-coA epimerase, an enzyme known to be used by bacteria such as Desulfatibacillum alkenivorans for alkane degradation (Callaghan et al., 2012), was expressed in the Dark Oil and Light Corexit samples by Ebipacterium multivorans and a bacterium from the family of Rhodobacteraceae (Supplementary Information 2).
Enzymes related to the glyoxylate bypass (isocitrate lyase, malate synthase, and succinate dehydrogenase), an alternative pathway for growth solely on alkanes, were enriched in all contaminant-containing treatments, primarily associated with Rhodobacterales and Alteromonadales. This enrichment may be a response to suppress oxidative stress, indicating the importance of boosting DNA repair mechanisms, oxidative stress resistance, and utilization of newly supplied carbon sources (Liu et al., 2003). These adaptations could contribute to an increased relative abundance of Proteobacterial orders, although a substantial reduction in bacterial diversity was observed (Figure 4). Furthermore, enzymes indicating a complete TCA cycle were enriched in contaminant-containing treatments, possibly due to bacteria utilizing newly supplied carbon sources. This includes malate dehydrogenase, which converts malate to pyruvate or oxaloacetate. These enzymes were more abundant in dark conditions than in light, suggesting sunlight’s influence on HC-degrading enzymes. Dehydrogenases, vital for PAH degradation (Van Beilen et al., 2003; Ghosal et al., 2016), were more prevalent in oil and/or Corexit-containing treatments, indicating potential short-term biodegradation of PAHs within 24 hours.
HC-degrading taxa were consistently present across treatment conditions, with a higher relative abundance in response to oil exposure. Alteromonadales increased significantly in response to oil, Corexit, and UV exposure. These findings indicate the rapid response of Alteromonadales to spills (Techtmann et al., 2017), including Corexit-treated conditions (Kamalanathan et al., 2018). Alteromonas and Marinobacter, known hydrocarbonoclasts, are among the first to proliferate in crude oil degradation, typically within days of exposure (Techtmann et al., 2017; Valencia-Agami et al., 2019). Extracellular polymeric substance (EPS) production may contribute to their resilience, aiding in biofilm formation and aggregation around oil droplets, which helps protect against environmental stressors such as PAHs or Corexit. Members of Alteromonadaceae are known to upregulate EPS production and extracellular enzyme activity in response to Corexit and oil exposure (Kamalanathan et al., 2018), as well as UV exposure (Sun et al., 2018). In our study, Alteromonas expressed protein functions potentially involved in EPS production, including polysaccharide biosynthesis, secondary metabolite biosynthesis, and transport. Additionally, some Alteromonadaceae may have the ability to degrade Corexit components (Kamalanathan et al., 2018). However, further experiments are needed to determine the toxicity of dispersant-oil mixtures compared to dispersants alone (Overholt et al., 2016).
4.3.2 Corexit unveiled: evidence of enhanced oxidative stress
Oxidative stress responses were upregulated in treated conditions compared to controls. UV exposure further increased the expression of oxidative stress responses in the presence of oil or Corexit, leading to the production of more toxic metabolites and reactive oxygen species (ROS) from these contaminants (Bacosa et al., 2015b). Understanding the regulation of these proteins in response to stressors can provide insights into bacterial adaptation mechanisms, which may be relevant for bioremediation in oil spill cleanups. Our study revealed that oil and especially Corexit triggered various responses to maintain DNA integrity and protect cells from damage.
Peptidases and proteases were abundant in contaminant-containing treatments, indicating protein damage and breakdown. Sunlight and contaminants showed higher enrichment of those enzymes suggesting an elevated level of oxidative stress in these conditions. Chaperonin proteins, including the 60 kDa, 10 kDa, and DnaK chaperonins, were consistently abundant in all mesocosms exposed to the challenging environmental conditions in the Gulf of Mexico (regular oil seepages, high doses of UV irradiation), indicating their crucial role in preventing cellular damage. These proteins play a crucial role in preventing cellular damage by averting the accumulation of misfolded or unfolded proteins that have lost their function. This observation implies the necessity for a stringent regulation of critical protein folding within the microbial community in this region to deal with the accumulation of carbonylated amino acids, a characteristic indicator of oxidative protein damage (Matallana-Surget et al., 2013; Chang et al., 2020).
The presence of Corexit led to an increase in the expression of TonB-dependent transport proteins. These proteins are known to be associated with either oil exposure (Knapik et al., 2020) or the application of Corexit (Peña-Montenegro et al., 2023). Starvation-related proteins were expressed in Corexit-containing treatments, indicating potential stress in nutrient-limited waters.
Proteins involved in cell redox homeostasis, such as peroxiredoxins, thioredoxins, hydroperoxide reductases, catalase-peroxidases, and superoxide dismutases were significantly more abundant in the presence of contaminants in comparison to the controls, highlighting the presence of ROS. Three proteins of peroxiredoxin and six catalase-peroxidases were abundant in Light Corexit (Supplementary Information 2). Glutathione reductase, another indicator of oxidative stress response, was abundant in Light Corexit-treated conditions. Its role is to maintain a strong reducing environment in the cell in response to ROS (Ziegelhoffer and Donohue, 2009).
DNA replication and repair protein Endonuclease MutS2 (DK Oil Corexit), DNA gyrase subunit B (Light Corexit, Light Oil Corexit), RecA (Light Oil, Light Corexit), LexA (DK Oil) and RecBCD enzyme subunit RecC (DK Oil Corexit), UvrABC system protein A (UvrA; Light Corexit, Light Oil Corexit) were absent in control samples and prevalent in contaminant-containing conditions, especially Corexit treatments, indicating acute stress responses that includes DNA damage repair and protection against oxidative stress (Morita et al., 2010). It was striking to observe numerous enzymes involved in distinct types of DNA repair pathways in presence of Corexit and/or Oil (absent in controls) that are essential for maintaining DNA integrity and preventing mutations. The DNA repair pathways involved DNA mismatch repair (Endonuclease MutS2), DNA homologous recombination (RecA, RecBCD), SOS Response (Lex A, transcriptional repressor regulating the SOS response), Nucleotide Excision Repair (NER) (UvrABC system) (Morita et al., 2010). Moreover, RecA was expressed in Light Corexit and Light Oil, while RecBCD enzyme subunit RecC was found in Light Oil & Corexit. Both RecA and RecBCD are part of a DNA lesion repair system (Anderson and Kowalczykowski, 1997), indicating the necessity for DNA damage repair under these conditions.
Numerous proteins responsible for maintaining chromosome integrity and DNA stability compaction were identified almost exclusively in the contaminants-containing conditions that included: DNA gyrase, DNA topoisomerases, Nucleoid occlusion factor SlmA, and several Histone Binding HU proteins. Divalent cations such as (Ca2+ or Mg2+) enriched in petroleum-containing waters are known to inhibit DNA compaction induced by trivalent cation such as spermidine (Gao et al., 2019). In other words, in the absence of spermidine, divalent cations, cause DNA condensation (Gao et al., 2019). Interestingly, we also characterized a high number of spermidine/putrescine ABS transporter proteins (44 proteins), exclusively present in contaminant-containing mesocosms, suggesting a role in balancing increased divalent cation concentrations and maintaining DNA stability, in a context of enhanced oxidative stress.
Cell Division FtsZ protein was prevalent in oil and/or Corexit treated samples, indicating its role in cell division under stress conditions. Under stress conditions, the SOS response may be activated, leading to the inhibition of cell division to allow time for DNA repair. FtsZ proteins can be regulated by diverse stress-induced pathways. Interestingly, it was demonstrated in E. coli that the nucleoid occlusion SlmA interferes with FtsZ polymerization and blocks the Z-ring formation over the nucleoid and help coordinate cell division with chromosome segregation (Cho et al., 2011; Monterroso et al., 2019; Robles-Ramos et al., 2021).
4.3.3 Nutrient cycling in the presence of light, oil, and Corexit
The oceanic environment is primarily limited by nitrogen, phosphorus, and iron availability (Moore et al., 2013). Picocyanobacteria, such as Synechococcus, play a significant role by producing dissolved organic matter (DOM), rich in nitrogen and phosphorus. Heterotrophic microorganisms contribute to nutrient cycling by regenerating inorganic nutrients, thus making them readily available to cyanobacteria in mutualistic consortia (Buchan et al., 2014; Christie-Oleza et al., 2017). Previous research conducted at the DwH plume site identified gene expressions associated with nitrogen assimilation, sulfate reduction, and phosphorus transport (Lu et al., 2012).
Synechococcus exhibited active metabolism involving iron, phosphorus, and nitrogen, primarily in the form of urea, a common nitrogen source for primary producers (Solomon et al., 2010). However, the presence of oil and Corexit, especially in irradiated conditions, had inhibitory effects on photosynthesis and the most abundant and active order of Cyanoabacteria (Synechococcales). This outcome is unsurprising, given that hydrocarbons (HCs) found in oil have inhibitory effects on most Cyanobacteria (Megharaj et al., 2000), including photosynthesis (Gordon and Prouse, 1973; Karydis, 1979). Corexit® 9500 is toxic to Synechococcus, and many other phytoplankton species, either alone or in combination with oil (Bacosa et al., 2015b; Quigg et al., 2021). The reduced representation and activity of Cyanobacteria, particularly Synechococcus, in irradiated conditions, likely result from the additional phototoxicity induced by crude oil and Corexit (Lacaze and Villedon de Naïde, 1976; Bacosa et al., 2015b). While Cyanobacteria fix carbon through photosynthesis and perform dark respiration (Shinde et al., 2020), no specific forms of carbon metabolism were observed in Synechococcus, Prochlorococcus, or Nostoc. However, ATP synthase required for respiration was detected in Synechococcus.
Marinomonas also expressed a range of nutrient acquisition strategies which aligns with the findings of Mason and colleagues (2012). Most of the expressed proteins were involved in transport and metabolism of nitrogen, phosphorus, iron, and sulfur, albeit to a lesser extent in the presence of sunlight. Additionally, metabolic pathways responsible for converting phosphonates (a common component of the oceanic organic phosphorous reservoir; Villarreal-Chiu et al., 2012) to phosphates were expressed at low levels in all conditions, as were pathways related to iron metabolism. Macondo oil has a low sulphur content, and sulphur metabolism was exclusively expressed at low levels in the Corexit treatment conditions. This is likely due to heterotrophic metabolism of the Corexit component DOSS. Sulphur metabolism was expressed in Marinomonas, although Oceanospirillales are not known for Corexit degradation.
Glutamate and glutamine metabolism were highly expressed relative to other forms of amino acid, peptide and protein metabolism, and strongly expressed across various taxa, including Alteromonas, Pseudoalteromonas, Marinomonas, Vibrio, and Synechococcus, indicating their role in nitrogen assimilation and biosynthesis of essential cellular components (Walker and van der Donk, 2016). Species-specific responses to oil and Corexit exposure have been observed in relation to glutamate and glutamine metabolism (Peña-Montenegro et al., 2023).
5 Conclusion
This study unveils novel insights into the remarkable abilities of indigenous bacteria in the GoM to biodegrade oil, even in the presence of Corexit dispersant and intense UV irradiation. Leveraging advanced metaproteomic techniques, we have delved deeper into the intricate functioning of marine microbial communities. For the first time, we harnessed metaproteomics to decipher the combined repercussions of oil pollution, the dispersant Corexit, and UV radiation on the natural microbial communities in the GoM, in the aftermath of the 2010 Deepwater Horizon oil spill.
Exposure to oil and/or Corexit initiates a profound transformation in the taxonomic structure of bacterioplankton communities, a transformation that sunlight further exacerbates due to enhanced oxidative stress. In the presence of oil, the growth of hydrocarbonoclastic heterotrophs such as Alteromonadales and Oceanospirillales was enhanced, while other less-adapted heterotrophs were inhibited. Consequently, the dominance of Alpha- and Gammaproteobacteria under sunlight exposure and with contaminant treatments was explained by their efficient DNA repair mechanisms, tolerance to oxidative stress, and the switch to alternative metabolic pathways. We observed a surge in carbon and amino acid metabolism in response to oil or Corexit. In our study, we demonstrated that Corexit does affect the expression of HC-degrading enzymes and enhanced oxidative stress.
Moreover, we provide compelling evidence that sunlight plays a pivotal role in the response and activity of the marine indigenous microbiome in the GoM. In the presence of sunlight and oil, the diversity of many crucial HC-degrading bacteria, such as Rhodovulum and Roseobacter, was significantly reduced compared to the dark and oil-only samples. This suggests that sunlight can alter the chemical structure of oil, making it more toxic to specific bacterial species. We also reported that cyanobacterial photosynthesis was impacted by the phototoxicity of oil and Corexit.
As a perspective, we advocate for further studies to incorporate a time series approach, comparing short-term versus longer incubations, while also assessing the impact of varying concentrations of crude oil and Corexit. Enhanced understanding of these factors would be invaluable in shaping future oil spill cleanup procedures, which are indispensable for preserving the health of our oceans.
Data availability statement
The mass spectrometry proteomics data have been deposited to the ProteomeXchange Consortium (https://proteomecentral.proteomexchange.org/) via the iProX partner repository (Ma et al., 2019; Chen et al, 2021) with the dataset identifier PXD048022.
Author contributions
SM: Conceptualization, Investigation, Methodology, Supervision, Validation, Visualization, Writing – original draft, Writing – review & editing, Data curation, Formal analysis, Project administration, Software. LN: Data curation, Formal analysis, Methodology, Writing – review & editing. LW: Data curation, Formal Analysis, Methodology, Writing – review & editing. PL: Data curation, Methodology, Writing – review & editing. RuW: Formal analysis, Methodology, Resources, Writing – review & editing. JW: Data curation, Formal analysis, Software, Visualization, Writing – review & editing. RF: Data curation, Writing – review & editing. DD: Data curation, Formal analysis, Methodology, Visualization, Writing – review & editing. RoW: Data curation, Formal analysis, Methodology, Visualization, Writing – review & editing. WJ: Funding acquisition, Methodology, Project administration, Resources, Supervision, Writing – review & editing.
Funding
The author(s) declare financial support was received for the research, authorship, and/or publication of this article. This research was made possible in part by a grant from BP/The Gulf of Mexico Research Initiative as part of the C-IMAGEII Consortium and the Belgian Fund for Scientific Research (Grand equipment - F.R.S - FNRS). Open access was funded by the University of Stirling.
Acknowledgments
We thank Melissa Brock, Melissa Egerington-Hagy, and Rachel Richardson for assistance with field sampling. We are indebted to Mike Murrell for hosting us at the EPA laboratory facilities.
Conflict of interest
The authors declare that the research was conducted in the absence of any commercial or financial relationships that could be construed as a potential conflict of interest.
Publisher’s note
All claims expressed in this article are solely those of the authors and do not necessarily represent those of their affiliated organizations, or those of the publisher, the editors and the reviewers. Any product that may be evaluated in this article, or claim that may be made by its manufacturer, is not guaranteed or endorsed by the publisher.
Supplementary material
The Supplementary Material for this article can be found online at: https://www.frontiersin.org/articles/10.3389/fmars.2023.1337886/full#supplementary-material
Supplementary Figure 1 | Functional composition for each experimental condition based on the percentage of proteins annotated and associated with a function. Functions where the number of proteins annotated represented less than 1% of the total number of proteins in an experimental group were labelled as other.
Supplementary Heatmaps 1-8 | Heatmaps of the taxonomic (bottom clusters) and the functional (right clusters) linkages for each methodology. Proteins annotated at both order and functional levels were ranked according to the number of identified peptides. Protein isoforms and/or sub-unit were grouped under the same function. Clusters were determined using complete linkage hierarchical clustering and Euclidean distance metric.
References
Aeppli C., Nelson R. K., Radovic J. R., Carmichael C. A., Valentine D. L., Reddy C. M. (2014). Recalcitrance and degradation of petroleum biomarkers upon abiotic and biotic natural weathering of Deepwater Horizon oil. Environ. Sci. Technol. 48 (12), 6726–6734. doi: 10.1021/es500825q
Allen E. E., Banfield J. F. (2005). Community genomics in microbial ecology and evolution. Nat. Rev. Microbiol. 3 (6), 489–498. doi: 10.1038/nrmicro1157
Anderson D. G., Kowalczykowski S. C. (1997). The translocating RecBCD enzyme stimulates recombination by directing RecA protein onto ssDNA in a χ-regulated manner. Cell 90 (1), 77–86. doi: 10.1016/S0092-8674(00)80315-3
Azam F., Fenchel T., Field J. G., Gray J. S., Meyer-Reil L. A., Thingstad F. (1983). The ecological role of water-column microbes in the sea. Mar. Ecol. Prog. Ser. 10, 257–263. doi: 10.3354/meps010257
Bacosa H. P., Erdner D. L., Liu Z. (2015a). Differentiating the roles of photooxidation and biodegradation in the weathering of Light Louisiana Sweet crude oil in surface water from the Deepwater Horizon site. Mar. pollut. Bull. 95 (1), 265–272. doi: 10.1016/j.marpolbul.2015.04.005
Bacosa H. P., Kang A., Lu K., Liu Z. (2021). Initial oil concentration affects hydrocarbon biodegradation rates and bacterial community composition in seawater. Mar. pollut. Bulletin. 162, 111867. doi: 10.1016/j.marpolbul.2020.111867
Bacosa H. P., Liu Z., Erdner D. L. (2015b). Natural sunlight shapes crude oil-degrading bacterial communities in northern Gulf of Mexico surface waters. Front. Microbiol. 6, 1325. doi: 10.3389/fmicb.2015.01325
Bae H. S., Huang L., White J. R., Wang J., DeLaune R. D., Ogram A., et al. (2018). Response of microbial populations regulating nutrient biogeochemical cycles to oiling of coastal saltmarshes from the Deepwater Horizon oil spill. Environ. pollut. 241, 136–147. doi: 10.1016/j.envpol.2018.05.033
Bargiela R., Herbst F. A., Martínez-Martínez M., Seifert J., Rojo D., Cappello S., et al. (2015). Metaproteomics and metabolomics analyses of chronically petroleum-polluted sites reveal the importance of general anaerobic processes uncoupled with degradation. Proteomics. 15 (20), 3508–3520. doi: 10.1002/pmic.201400614
Bertilsson S., Widenfalk A. (2002). Photochemical degradation of PAHs in freshwaters and their impact on bacterial growth-influence of water chemistry. Hydrobiologia 469, 23–32. doi: 10.1023/A:1015579628189
Brakstad O. G., Lødeng A. G. G. (2005). Microbial diversity during biodegradation of crude oil in seawater from the North Sea. Microbial Ecol. 49 (1), 94–103. doi: 10.1007/s00248-003-0225-6
Bridges K. N., Lay C. R., Alloy M. M., Gielazyn M. L., Morris J. M., Forth H. P., et al. (2018). Estimating incident ultraviolet radiation exposure in the northern Gulf of Mexico during the Deepwater Horizon oil spill. Environmental. Toxicol. Chem. 37 (6), 1679–1687. doi: 10.1002/etc.4119
Bruheim P., Bredholt H., Eimhjellen K. (1999). Effects of surfactant mixtures, including Corexit 9527, on bacterial oxidation of acetate and alkanes in crude oil. Appl. Environ. Microbiol. 65 (4), 1658–1661. doi: 10.1128/AEM.65.4.1658-1661.1999
Buchan A., LeCleir G. R., Gulvik C. A., González J. M. (2014). Master recyclers: features and functions of bacteria associated with phytoplankton blooms. Nat. Rev. Microbiol. 10, 686–698. doi: 10.1038/nrmicro3326
Callaghan A. V., Morris B. E., Pereira I. A., McInerney M. J., Austin R. N., Groves J. T., et al. (2012). The genome sequence of Desulfatibacillum alkenivorans AK-01: a blueprint for anaerobic alkane oxidation. Environ. Microbiol. 14 (1), 101–113. doi: 10.1111/j.1462-2920.2011.02516.x
Caporaso J. G., Lauber C. L., Walters W. A., Berg-Lyons D., Lozupone C. A., Turnbaugh P. J., et al. (2011). Global patterns of 16S rRNA diversity at a depth of millions of sequences per sample. Proc. Natl. Acad. Sci. U S A. 108 Suppl 1 (Suppl 1), 4516–4522. doi: 10.1073/pnas.1000080107
Chakraborty R., Borglin S. E., Dubinsky E. A., Andersen G. L., Hazen T. C. (2012). Microbial response to the MC-252 oil and corexit 9500 in the gulf of Mexico. Front. Microbiol. 3, 357. doi: 10.3389/fmicb.2012.00357
Chang R. L., Stanley J. A., Robinson M. C., Sher J. W., Li Z., Chan Y. A., et al. (2020). Protein structure, amino acid composition and sequence determine proteome vulnerability to oxidation-induced damage. EMBO J. 39 (23), e104523. doi: 10.15252/embj.2020104523
Chen T, Ma J, Liu Y, Chen Z, Xiao N, Lu Y, et al. (2022). iProX in 2021: connecting proteomics data sharing with big data. Nucleic Acids Res. 50 (D1), D1522–D1527. doi: 10.1093/nar/gkab1081
Cho H., McManus H. R., Dove S. L., Bernhardt T. G. (2011). Nucleoid occlusion factor SlmA is a DNA-activated FtsZ polymerization antagonist. Proc. Natl. Acad. Sci. U S A. 108 (9), 3773–3778. doi: 10.1073/pnas.1018674108
Christie-Oleza J. A., Sousoni D., Lloyd M., Armengaud J., Scanlan D. J. (2017). Nutrient recycling facilitates long-term stability of marine microbial phototroph-heterotroph interactions. Nat. Microbiol. 2, 17100. doi: 10.1038/nmicrobiol.2017.100
Crone T. J., Tolstoy M. (2010). Magnitude of the 2010 Gulf of Mexico oil leak. Science 330 (6004), 634. doi: 10.1126/science.1195840
Crump B. C., Armbrust E. V., Baross J. A. (1999). Phylogenetic analysis of particle-attached and free-living bacterial communities in the Columbia River, its estuary, and the adjacent coastal ocean. Appl. Environ. Microbiol. 65 (7), 3192–3204. doi: 10.1128/AEM.65.7.3192-3204.1999
DeLong E. F. (2005). Microbial community genomics in the ocean. Nature Reviews. Microbiology 3 (6), 459–469. doi: 10.1038/nrmicro1158
Doyle S. M., Whitaker E. A., De Pascuale V., Wade T. L., Knap A. H., Santschi P. H., et al. (2018). Rapid formation of microbe-oil aggregates and changes in community composition in coastal surface water following exposure to oil and the dispersant corexit. Front. Microbiol. 9, 689. doi: 10.3389/fmicb.2018.00689
Dubinsky E. A., Conrad M. E., Chakraborty R., Bill M., Borglin S. E., Hollibaugh J. T., et al. (2013). Succession of hydrocarbon-degrading bacteria in the aftermath of the Deepwater Horizon Oil Spill in the Gulf of Mexico. Environ. Sci. Technol. 47 (19), 10860–10867. doi: 10.1021/es401676y
Edgar R. C. (2013). UPARSE: highly accurate OTU sequences from microbial amplicon reads. Nat. Methods 10 (10), 996–998. doi: 10.1038/nmeth.2604
Edwards B. R., Reddy C. M., Camilli R., Carmichael C. A., Longnecker K., Mooy B. A. S. V. (2011). Rapid microbial respiration of oil from the Deepwater Horizon spill in offshore surface waters of the Gulf of Mexico. Environ. Res. Lett. 6 (3), 035301. doi: 10.1088/1748-9326/6/3/035301
Feng L., Wang W., Cheng J., Ren Y., Zhao G., Gao C., et al. (2007). Genome and proteome of long-chain alkane degrading Geobacillus thermodenitrificans NG80-2 isolated from a deep-subsurface oil reservoir. Proc. Natl. Acad. Sci. U S A. 104 (13), 5602–5607. doi: 10.1073/pnas.0609650104
Gao T., Zhang W., Wang Y., Yang G. (2019). DNA Compaction and Charge Neutralization Regulated by Divalent Ions in very Low pH Solution. Polymers (Basel). 11 (2), 337. doi: 10.3390/polym11020337
Géron A., Werner J., Wattiez R., Lebaron P., Matallana-Surget S. (2019). Deciphering the functioning of microbial communities: shedding light on the critical steps in metaproteomics. Front. Microbiol. 10, 2395. doi: 10.3389/fmicb.2019.02395
Ghosal D., Ghosh S., Dutta T. K., Ahn Y. (2016). Current state of knowledge in microbial degradation of polycyclic aromatic hydrocarbons (PAHs): a review. Front. Microbiol. 7, 1369. doi: 10.3389/fmicb.2016.01369
Gómez-Consarnau L., Needham D. M., Weber P. K., Fuhrman J. A., Mayali X. (2019). Influence of light on particulate organic matter utilization by attached and free-living marine bacteria. Front. Microbiol. 10, 1204. doi: 10.3389/fmicb.2019.01204
Gordon D. C., Prouse N. J. (1973). The effects of three oils on marine phytoplankton photosynthesis. Mar. Biol. 22 (4), 329–333. doi: 10.1007/BF00391389
Griffiths M. T., Da Campo R., O'Connor P. B., Barrow M. P. (2014). Throwing light on petroleum: Simulated exposure of crude oil to sunlight and characterization using atmospheric pressure photoionization Fourier transform ion cyclotron resonance mass spectrometry. Analytical Chem. 86 (1), 527–534. doi: 10.1021/ac4025335
Hamady M., Lozupone C., Knight R. (2010). Fast UniFrac: facilitating high-throughput phylogenetic analyses of microbial communities including analysis of pyrosequencing and PhyloChip data. ISME J. 4 (1), 17–27. doi: 10.1038/ismej.2009.97
Hamdan L., Fulmer P. (2011). Effects of COREXIT® EC9500A on bacteria from a beach oiled by the Deepwater Horizon spill. Aquat. Microbial Ecol. 63 (2), 101–109. doi: 10.3354/ame01482
Headrick E. L., Nigro L. M., Waidner L. A., Ederington-Hagy M., Simmering A. L., Snyder R. A., et al. (2023). Acute Inhibition of bacterial growth in coastal seawater amended with crude oils with varied photoreactivities. Front. Ecol. Evol. 11, 1113899. doi: 10.3389/fevo.2023.1113899
Hill T. C., Walsh K. A., Harris J. A., Moffett B. F. (2006). Using ecological diversity measures with bacterial communities. FEMS. Microbiol. Ecol. 43 (1), 1–11. doi: 10.1111/j.1574-6941.2003.tb01040.x
Horel A., Bernard R. J., Mortazavi B. (2014). Impact of crude oil exposure on nitrogen cycling in a previously impacted Juncus roemerianus salt marsh in the northern Gulf of Mexico. Environ. Sci. pollut. Res. 21 (11), 6982–6993. doi: 10.1007/s11356-014-2599-z
Jin H. M., Kim J. M., Lee H. J., Madsen E. L., Jeon C. O. (2012). Alteromonas as a key agent of polycyclic aromatic hydrocarbon biodegradation in crude oil-contaminated coastal sediment. Environ. Sci. Technol. 46 (14), 7731–7740. doi: 10.1021/es3018545
Joye S. B., Teske A. P., Kostka J. E. (2014). Microbial dynamics following the macondo oil well blowout across gulf of Mexico environments. BioScience 64 (9), 766–777. doi: 10.1093/biosci/biu121
Kamalanathan M., Xu C., Schwehr K., Bretherton L., Beaver M., Doyle S. M., et al. (2018). Extracellular enzyme activity profile in a chemically enhanced water accommodated fraction of surrogate oil: Toward understanding microbial activities after the Deepwater Horizon oil spill. Front. Microbiol. 9, 1–13. doi: 10.3389/fmicb.2018.00798
Karydis M. (1979). Short term effects of hydrocarbons on the photosynthesis and respiration of some phytoplankton species. Botanica Marina 22 (5), 281–286. doi: 10.1515/botm.1979.22.5.281
King S. M., Leaf P. A., Olson A. C., Ray P. Z., Tarr M. A. (2014). Photolytic and photocatalytic degradation of surface oil from the Deepwater Horizon spill. Chemosphere 95, 415–422. doi: 10.1016/j.chemosphere.2013.09.060
Kleindienst S., Paul J. H., Joye S. B. (2015). Using dispersants after oil spills: impacts on the composition and activity of microbial communities. Nat. Rev. Microbiol. 13 (6), 388–396. doi: 10.1038/nrmicro3452
Knapik K., Bagi A., Krolicka A., Baussant T. (2020). Metatranscriptomic analysis of oil-exposed seawater bacterial communities archived by an environmental sample processor (ESP). Microorganisms 8 (5), 744. doi: 10.3390/microorganisms8050744
Kostka J. E., Prakash O., Overholt W. A., Green S. J., Freyer G., Canion A., et al. (2011). Hydrocarbon-degrading bacteria and the bacterial community response in Gulf of Mexico beach sands impacted by the Deepwater Horizon oil spill. Appl. Environ. Microbiol. 77 (22), 7962–7974. doi: 10.1128/AEM.05402-11
Kujawinski E. B., Kido Soule M. C., Valentine D. L., Boysen A. K., Longnecker K., Redmond M. C. (2011). Fate of dispersants associated with the Deepwater Horizon oil spill. Environ. Sci. Technol. 45 (4), 1298–1306. doi: 10.1021/es103838p
Lacaze J. C., Villedon de Naïde O. (1976). Influence of illumination on phytotoxicity of crude oil. Mar. pollut. Bull. 7 (4), 73–76. doi: 10.1016/0025-326X(76)90018-7
Lamendella R., Strutt S., Borglin S., Chakraborty R., Tas N., Mason O. U., et al. (2014). Assessment of the Deepwater Horizon oil spill impact on Gulf Coast microbial communities. Front. Microbiol. 5, 130. doi: 10.3389/fmicb.2014.00130
Leahy J. G., Colwell R. R. (1990). Microbial degradation of hydrocarbons in the environment. Microbiological Rev. 54 (3), 305–315. doi: 10.1128/mr.54.3.305-315.1990
Levine B. M., White J. R., DeLaune R. D. (2017). Impacts of the long-term presence of buried crude oil on salt marsh soil denitrification in Barataria Bay, Louisiana. Ecol. Eng. 99, 454–461. doi: 10.1016/j.ecoleng.2016.11.017
Little A. E., Robinson C. J., Peterson S. B., Raffa K. F., Handelsman J. (2008). Rules of engagement: interspecies interactions that regulate microbial communities. Annu. Rev. Microbiol. 62, 375–401. doi: 10.1146/annurev.micro.030608.101423
Liu Z., Liu J. (2013). Evaluating bacterial community structures in oil collected from the sea surface and sediment in the northern Gulf of Mexico after the Deepwater Horizon oil spill. Microbiol. Open 2 (3), 492–504. doi: 10.1002/mbo3.89
Liu Z., Liu J., Gardner W. S., Shank G. C., Ostrom N. E. (2016). The impact of Deepwater Horizon oil spill on petroleum hydrocarbons in surface waters of the northern Gulf of Mexico. Deep-Sea Research Part II. Topical Stud. Oceanography 129, 292–300. doi: 10.1016/j.dsr2.2014.01.013
Liu Z., Liu J., Zhu Q., Wu W. (2012). The weathering of oil after the Deepwater Horizon oil spill: Insights from the chemical composition of the oil from the sea surface, salt marshes, and sediments. Environ. Res. Lett. 7 (3), 035302. doi: 10.1088/1748-9326/7/3/035302
Liu Y., Zhou J., Omelchenko M. V., Beliaev A. S., Venkateswaran A., Stair J., et al. (2003). Transcriptome dynamics of Deinococcus radiodurans recovering from ionizing radiation. Proc. Natl. Acad. Sci. 100 (7), 4191–4196. doi: 10.1073/pnas.0630387100
Lu Z., Deng Y., Van Nostrand J. D., He Z., Voordeckers J., Zhou A., et al. (2012). Microbial gene functions enriched in the Deepwater Horizon deep-sea oil plume. ISME J. 6 (2), 451–460. doi: 10.1038/ismej.2011.91
Ma M., Zheng L., Yin X., Gao W., Han B., Li Q., et al. (2021). Reconstruction and evaluation of oil-degrading consortia isolated from sediments of hydrothermal vents in the South Mid-Atlantic Ridge. Sci. Rep. 11 (1), 1456. doi: 10.1038/s41598-021-80991-5
Ma J, Chen T, Wu S, Yang C, Bai M, Shu K, et al. (2019). iProX: an integrated proteome resource. Nucleic Acids Res. 47 (D1), D1211–D1217. doi: 10.1093/nargky869
Marietou A., Chastain R., Beulig F., Scoma A., Hazen T. C., Bartlett D. H. (2018). The effect of hydrostatic pressure on enrichments of hydrocarbon degrading microbes from the Gulf of Mexico following the Deepwater Horizon Oil Spill. Front. Microbiol. 9, 808. doi: 10.3389/fmicb.2018.00808
Mason O. U., Hazen T. C. (2011). New insights into microbial responses to oil spills from the Deepwater Horizon incident. SIM News 61 (3), 60–65.
Mason O. U., Hazen T. C., Borglin S., Chain P. S., Dubinsky E. A., Fortney J. L., et al. (2012). Metagenome, metatranscriptome and single-cell sequencing reveal microbial response to Deepwater Horizon oil spill. ISME J. 6 (9), 1715–1727. doi: 10.1038/ismej.2012.59
Matallana-Surget S., Cavicchioli R., Fauconnier C., Wattiez R., Leroy B., Joux F., et al. (2013). Shotgun redox proteomics: identification and quantitation of carbonylated proteins in the UVB-resistant marine bacterium, Photobacterium angustum S14. PloS One 8 (7), e68112. doi: 10.1371/journal.pone.0068112
Matallana-Surget S., Jagtap P. D., Griffin T. G., Beraud M., Wattiez R. (2018). “Comparative Metaproteomics to Study Environmental Changes,” in Metagenomics: Perspectives, Methods, and Applications. Ed. Nagarajan M. (London, United Kingdom: Academic Press), 70–86.
Matallana-Surget S., Wattiez R. (2013). Impact of solar radiation on gene expression in bacteria. Proteomes 1 (2), 70–86. doi: 10.3390/proteomes1020070
Megharaj M., Singleton I., McClure N. C., Naidu R. (2000). Influence of petroleum hydrocarbon contamination on microalgae and microbial activities in a long-term contaminated soil. Arch. Environ. Contamination Toxicol. 38 (4), 439–445. doi: 10.1007/s002449910058
Miralles G., Nérini D., Manté C., Acquaviva M., Doumenq P., Michotey V., et al. (2007). Effects of spilled oil on bacterial communities of Mediterranean coastal anoxic sediments chronically subjected to oil hydrocarbon contamination. Microbial Ecol. 54 (4), 646–661. doi: 10.1007/s00248-007-9221-6
Monterroso B., Zorrilla S., Sobrinos-Sanguino M., Robles-Ramos M. A., Margolin W., Keating C. D., et al. (2019). Bacterial FtsZ protein forms phase-separated condensates with its nucleoid-associated inhibitor SlmA. EMBO Rep. 20 (2019), e45946. doi: 10.15252/embr.201845946
Moore C. M., Mills M. M., Arrigo K. R., Berman-Frank I., Bopp L., Boyd P. W., et al. (2013). Processes and patterns of oceanic nutrient limitation. Nat. Geosci. 6 (9), 701–710. doi: 10.1038/ngeo1765
Morita R., Nakane S., Shimada A., Inoue M., Iino H., Wakamatsu T., et al. (2010). Molecular mechanisms of the whole DNA repair system: a comparison of bacterial and eukaryotic systems. J. Nucleic Acids 2010, 179594. doi: 10.4061/2010/179594
Neff J. M., Anderson J. W. (1981). Response of Marine Animals to Petroleum and Specific Petroleum Hydrocarbons (United States: Halsted Press, a Division of John Wiley and Sons, Inc., New York, NY).
Nyman J. A. (1999). Effect of crude oil and chemical additives on metabolic activity of mixed microbial populations in fresh marsh soils. Microbial Ecol. 37 (2), 152–162. doi: 10.1007/s002489900139
Orcutt B. N., Orcutt B. N., Joye S. B., Kleindienst S., Knittel K., Ramette A., et al. (2010). Impact of natural oil and higher hydrocarbons on microbial diversity, distribution, and activity in Gulf of Mexico cold-seep sediments. Deep-Sea Res. Part II: Topical Stud. Oceanography 57 (21–23), 2008–2021. doi: 10.1016/j.dsr2.2010.05.014
Overholt W. A., Marks K. P., Romero I. C., Hollander D. J., Snell T. W., Kostka J. E. (2016). Hydrocarbon-degrading bacteria exhibit a species-specific response to dispersed oil while moderating ecotoxicity. Appl. Environ. Microbiol. 82 (2), 518–527. doi: 10.1128/AEM.02379-15
Peixoto R. S., Vermelho A. B., Rosado A. S. (2011). Petroleum-degrading enzymes: bioremediation and new prospects. Enzyme Res. 2011, 475193. doi: 10.4061/2011/475193
Peña-Montenegro T. D., Kleindienst S., Allen A. E., Eren A. M., McCrow J. P., Sánchez-Calderón J. D., et al. (2023). Species-specific responses of marine bacteria to environmental perturbation. ISME Communication. 3 (1), 99. doi: 10.1038/s43705-023-00310-z
Pietroski J. P., White J. R., DeLaune R. D., Wang J. J., Dodla S. K. (2015). Fresh and weathered crude oil effects on potential denitrification rates of coastal marsh soil. Chemosphere 134, 120–126. doi: 10.1016/j.chemosphere.2015.03.056
Prince R. C. (2010). “Bioremediation of Marine Oil Spills,” in Handbook of Hydrocarbon and Lipid Microbiology. (Berlin, Heidelberg: Springer), 2617–2630.
Prince R. C., Mcfarlin K. M., Butler J. D., Febbo E. J., Wang F. C., Nedwed T. J. (2013). The primary biodegradation of dispersed crude oil in the sea. Chemosphere 90 (2), 521–526. doi: 10.1016/j.chemosphere.2012.08.020
Quigg A., Parsons M., Bargu S., Ozhan K., Daly K. L., Chakraborty S., et al. (2021). Marine phytoplankton responses to oil and dispersant exposures: Knowledge gained since the Deepwater Horizon oil spill. Mar. pollut. Bull. 164, 112074. doi: 10.1016/j.marpolbul.2021.112074
Radniecki T. S., Schneider M. C., Semprini L. (2013). The influence of Corexit 9500A and weathering on Alaska North Slope crude oil toxicity to the ammonia oxidizing bacterium, Nitrosomonas europaea. Mar. pollut. Bull. 68 (1–2), 64–70. doi: 10.1016/j.marpolbul.2012.12.022
Ray P. Z., Tarr M. A. (2014). Petroleum films exposed to sunlight produce hydroxyl radical. Chemosphere 103, 220–227. doi: 10.1016/j.chemosphere.2013.12.005
Redmond M. C., Valentine D. L., Sessions A. L. (2010). Identification of novel methane-, ethane-, and propane-oxidizing bacteria at marine hydrocarbon seeps by stable isotope probing. Appl. Environ. Microbiol. 76 (19), 6412–6422. doi: 10.1128/AEM.00271-10
Robles-Ramos M. A., Zorrilla S., Alfonso C., Margolin W., Rivas G., Monterroso B. (2021). Assembly of bacterial cell division protein FtsZ into dynamic biomolecular condensates. Biochim. Biophys. Acta (BBA) - Mol. Cell Res. 1868 (5), 118986. doi: 10.1016/j.bbamcr.2021.118986
Rosal R., Rodea-Palomares I., Boltes K., Fernández-Piñas F., Leganés F., Petre A. (2010). Ecotoxicological assessment of surfactants in the aquatic environment: Combined toxicity of docusate sodium with chlorinated pollutants. Chemosphere 81 (2), 288–293. doi: 10.1016/j.chemosphere.2010.05.050
Rudney J. D., Jagtap P. D., Reilly C. S., Chen R., Markowski T. W., Higgins L., et al. (2015). Protein relative abundance patterns associated with sucrose-induced dysbiosis are conserved across taxonomically diverse oral microcosm biofilm models of dental caries. Microbiome 3 (1), 69. doi: 10.1186/s40168-015-0136-z
Ruiz-Gonzalez C.L.A.R.A., Simó R., Sommaruga R., Gasol J. M. (2013). Away from darkness: a review on the effects of solar radiation on heterotrophic bacterioplankton activity. Front. Microbiol. 4, 131. doi: 10.3389/fmicb.2013.00131
Sabirova J. S., Ferrer M., Regenhardt D., Timmis K. N., Golyshin P. N. (2006). Proteomic insights into metabolic adaptations in Alcanivorax borkumensis induced by alkane utilization. J. Bacteriology 188 (11), 3763–3773. doi: 10.1128/JB.00072-06
Saito M. A., Bertrand E. M., Duffy M. E., Gaylord D. A., Held N. A., Hervey W. J., et al. (2019). Progress and challenges in ocean metaproteomics and proposed best practices for data sharing. J. Proteome Res. 18 (4), 1461–1476. doi: 10.1021/acs.jproteome.8b00761
Shinde S., Zhang X., Singapuri S. P., Kalra I., Liu X., Morgan-Kiss R. M., et al. (2020). Glycogen metabolism supports photosynthesis start through the oxidative pentose phosphate pathway in cyanobacteria. Plant Physiol. 182 (1), 507–517. doi: 10.1104/pp.19.01184
Sinha R. P., Häder D. P. (2002). UV-induced DNA damage and repair: a review. Photochemical Photobiological Sci. 1 (4), 225–236. doi: 10.1039/b201230h
Snyder K., Mladenov N., Richardot W., Dodder N., Nour A., Campbell C., et al. (2021). Persistence and photochemical transformation of water-soluble constituents from industrial crude oil and natural seep oil in seawater. Mar. pollut. Bull. 165, 112049. doi: 10.1016/j.marpolbul.2021.112049
Solomon C. M., Collier J. L., Berg G. M., Glibert P. M. (2010). Role of urea in microbial metabolism in aquatic systems: A biochemical and molecular review. Aquat. Microbial Ecol. 59 (1), 67–88. doi: 10.3354/ame01390
Sterling M. C., Bonner J. S., Ernest A. N., Page C. A., Autenrieth R. L. (2004). Chemical dispersant effectiveness testing: Influence of droplet coalescence. Mar. pollut. Bull. 48 (9–10), 969–977. doi: 10.1016/j.marpolbul.2003.12.003
Sun L., Chiu M. H., Xu C., Lin P., Schwehr K. A., Bacosa H., et al. (2018). The effects of sunlight on the composition of exopolymeric substances and subsequent aggregate formation during oil spills. Mar. Chem. 203, 49–54. doi: 10.1016/j.marchem.2018.04.006
Tarr M. A., Zito P., Overton E. B., Olson G. M., Adhikari P. L., Reddy C. M. (2016). Weathering of oil spilled in the marine environment. Oceanography 29 (3), 126–135. doi: 10.5670/oceanog.2016.77
Techtmann S. M., Zhuang M., Campo P., Holder E., Elk M., Hazen T. C., et al. (2017). Corexit 9500 enhances oil biodegradation and changes active bacterial community structure of oil-enriched microcosms. Appl. Environ. Microbiol. 83 (10), e03462–e03416. doi: 10.1128/AEM.03462-16
Tian H., Gao P., Chen Z., Li Y., Li Y., Wang Y., et al. (2017). Compositions and abundances of sulfate-reducing and sulfur-oxidizing microorganisms in water-flooded petroleum reservoirs with different temperatures in China. Front. Microbiol. 8, 143. doi: 10.3389/fmicb.2017.00143
Tissot B. P., Welte D. H. (1984). Petroleum Formation and Occurrence: A New Approach to Oil and Gas Exploration. 2nd ed (Berlin Heidelberg: Springer).
Urakawa H., Garcia J. C., Barreto P. D., Molina G. A., Barreto J. C. (2012). A sensitive crude oil bioassay indicates that oil spills potentially induce a change of major nitrifying prokaryotes from the Archaea to the Bacteria. Environ. pollut. 164, 42–45. doi: 10.1016/j.envpol.2012.01.009
Valencia-Agami S. S., Cerqueda-García D., Putzeys S., Uribe-Flores M. M., García-Cruz N. U., Pech D., et al. (2019). Changes in the bacterioplankton community structure from southern Gulf of Mexico during a simulated crude oil spill at mesocosm Scale. Microorganisms 7 (10), 441. doi: 10.3390/microorganisms7100441
Van Beilen J. B., Li Z., Duetz W. A., Smits T. H. M., Witholt B. (2003). Diversity of alkane hydroxylase systems in the environment. Oil Gas Sci. Technol. 58 (4), 427–440. doi: 10.2516/ogst:2003026
Vaughan P. P., Wilson T., Kamerman R., Hagy M. E., McKenna A., Chen H., et al. (2016). Photochemical changes in water accommodated fractions of MC252 and surrogate oil created during solar exposure as determined by FT-ICR MS. Mar. pollut. Bulletin. 104 (1-2), 262–268. doi: 10.1016/j.marpolbul.2016.01.012
Villarreal-Chiu J. F., Quinn J. P., McGrath J. W. (2012). The genes and enzymes of phosphonate metabolism by bacteria, and their distribution in the marine environment. Front. Microbiol. 3, 19. doi: 10.3389/fmicb.2012.00019
Walker M. C., van der Donk W. A. (2016). The many roles of glutamate in metabolism. J. Ind. Microbiol. Biotechnol. 43 (2–3), 419–430. doi: 10.1007/s10295-015-1665-y
Walters W, Hyde ER, Berg-Lyons D, Ackermann G, Humphrey G, Parada A, et al. (2015). Improved bacterial 16s rrna gene (v4 and v4-5) and fungal internal transcribed spacer marker gene primers for microbial community surveys. mSystems 1 (1), e00009–15. doi: 10.1128/mSystems.00009-15
Werner J., Géron A., Kerssemakers J., Matallana-Surget S. (2019). mPies: a novel metaproteomics tool for the creation of relevant protein databases and automatized protein annotation. Biol. Direct. 14 (1), 21. doi: 10.1186/s13062-019-0253-x
Yoshida A., Nomura H., Toyoda K., Nishino T., Seo Y., Yamada M., et al. (2006). Microbial responses using denaturing gradient gel electrophoresis to oil and chemical dispersant in enclosed ecosystems. Mar. pollut. Bull. 52 (1), 89–95. doi: 10.1016/j.marpolbul.2005.08.015
Ziegelhoffer E. C., Donohue T. J. (2009). Bacterial responses to photo-oxidative stress. Nat. Rev. Microbiol. 12, 856–863. doi: 10.1038/nrmicro2237
Zito P., Chen H., Podgorski D. C., McKenna A. M., Tarr M. A. (2014). Sunlight creates oxygenated species in water-soluble fractions of Deepwater Horizon oil. J. Hazardous Materials 280, 636–643. doi: 10.1016/j.jhazmat.2014.08.059
Keywords: oil spill, dispersant, solar radiation, marine microbiome, hydrocarbon-degraders, metaproteomics
Citation: Matallana-Surget S, Nigro LM, Waidner LA, Lebaron P, Wattiez R, Werner J, Fraser R, Dimitrov D, Watt R and Jeffrey WH (2024) Clarifying the murk: unveiling bacterial dynamics in response to crude oil pollution, Corexit-dispersant, and natural sunlight in the Gulf of Mexico. Front. Mar. Sci. 10:1337886. doi: 10.3389/fmars.2023.1337886
Received: 13 November 2023; Accepted: 27 December 2023;
Published: 17 January 2024.
Edited by:
Hao Zhang, Chinese Academy of Sciences (CAS), ChinaReviewed by:
Rossanna Rodriguez-Canul, Center for Research and Advanced Studies - Mérida Unit, MexicoLi Tangcheng, Shantou University, China
Copyright © 2024 Matallana-Surget, Nigro, Waidner, Lebaron, Wattiez, Werner, Fraser, Dimitrov, Watt and Jeffrey. This is an open-access article distributed under the terms of the Creative Commons Attribution License (CC BY). The use, distribution or reproduction in other forums is permitted, provided the original author(s) and the copyright owner(s) are credited and that the original publication in this journal is cited, in accordance with accepted academic practice. No use, distribution or reproduction is permitted which does not comply with these terms.
*Correspondence: Sabine Matallana-Surget, sabine.matallanasurget@stir.ac.uk