Patterns of Transposable Element Expression and Insertion in Cancer
- 1Integrated Cancer Research Center, School of Biology, Georgia Institute of Technology, Atlanta, GA, USA
- 2Ovarian Cancer Institute, Atlanta, GA, USA
- 3School of Biology, Georgia Institute of Technology, Atlanta, GA, USA
- 4PanAmerican Bioinformatics Institute, Cali, Colombia
- 5Applied Bioinformatics Laboratory, Atlanta, GA, USA
- 6Department of Computational Mathematics, Science and Engineering, Michigan State University, East Lansing, MI, USA
Human transposable element (TE) activity in somatic tissues causes mutations that can contribute to tumorigenesis. Indeed, TE insertion mutations have been implicated in the etiology of a number of different cancer types. Nevertheless, the full extent of somatic TE activity, along with its relationship to tumorigenesis, have yet to be fully explored. Recent developments in bioinformatics software make it possible to analyze TE expression levels and TE insertional activity directly from transcriptome (RNA-seq) and whole genome (DNA-seq) next-generation sequence data. We applied these new sequence analysis techniques to matched normal and primary tumor patient samples from the Cancer Genome Atlas (TCGA) in order to analyze the patterns of TE expression and insertion for three cancer types: breast invasive carcinoma, head and neck squamous cell carcinoma, and lung adenocarcinoma. Our analysis focused on the three most abundant families of active human TEs: Alu, SVA, and L1. We found evidence for high levels of somatic TE activity for these three families in normal and cancer samples across diverse tissue types. Abundant transcripts for all three TE families were detected in both normal and cancer tissues along with an average of ~80 unique TE insertions per individual patient/tissue. We observed an increase in L1 transcript expression and L1 insertional activity in primary tumor samples for all three cancer types. Tumor-specific TE insertions are enriched for private mutations, consistent with a potentially causal role in tumorigenesis. We used genome feature analysis to investigate two specific cases of putative cancer-causing TE mutations in further detail. An Alu insertion in an upstream enhancer of the CBL tumor suppressor gene is associated with down-regulation of the gene in a single breast cancer patient, and an L1 insertion in the first exon of the BAALC gene also disrupts its expression in head and neck squamous cell carcinoma. Our results are consistent with widespread somatic activity of human TEs leading to numerous insertion mutations that can contribute to tumorigenesis in a variety of tissues.
Introduction
More than 50% of the human genome sequence is derived from transposable element (TE) insertions (Lander et al., 2001; de Koning et al., 2011). The vast majority of TE-derived sequences in the human genome correspond to relatively ancient insertions that are no longer capable of transposition (Mills et al., 2007). However, there are several families of human TEs that remain active to this day. The most abundant families of active TEs in the human genome are the Alu and SVA short interspersed nuclear elements (SINEs) along with the L1 Long Interspersed Nuclear Element (LINE) family (Kazazian et al., 1988; Batzer and Deininger, 1991; Batzer et al., 1991; Brouha et al., 2003; Ostertag et al., 2003; Wang et al., 2005). Alu and SVA SINEs are non-autonomous TEs that are mobilized via the transpositional machinery encoded by the autonomous L1 family of LINEs. Recent evidence indicates that a handful of HERV-K endogenous retroviral elements also remain active in the human genome (Wildschutte et al., 2016).
Active TE families are of great interest since they have the ability to generate de novo mutations, many of which have been linked to human disease (Hancks and Kazazian, 2012; Solyom and and Kazazian, 2012). For instance, TE insertions have been shown to contribute to the etiology of a variety of different cancer types (Belancio et al., 2010a; Carreira et al., 2014). Numerous recent studies have used a combination of next-generation sequence analysis, followed by validation with PCR and/or Sanger sequencing, to elucidate connections between TE activity and cancer (Solyom et al., 2012; Shukla et al., 2013; Tubio et al., 2014; Doucet-O'Hare et al., 2015; Ewing et al., 2015). L1 insertions in particular have been implicated as potential cancer causing mutations in those and other studies (Morse et al., 1988; Miki et al., 1992; Iskow et al., 2010; Lee et al., 2012; Scott et al., 2016). L1 activity is thought to promote tumor development by causing genomic instability, via impaired chromosomal pairing during mitosis, and/or by disrupting coding or regulatory sequences (Kemp and Longworth, 2015).
Many of the studies that have related TEs to cancer have considered TE expression, at the transcript or protein level, and TE insertional activity separately. A number of different cancer types are positive for L1 transcript expression (Belancio et al., 2010b), and L1 proteins have been shown to be ubiquitously expressed in both normal and tumor samples from the same individuals (Bratthauer and Fanning, 1992, 1993; Bratthauer et al., 1994; Asch et al., 1996; Doucet-O'Hare et al., 2015, 2016). There is also evidence suggesting that L1 protein expression can be limited to tumor tissues and thereby serve as a useful cancer biomarker; nearly half of all human cancers are exclusively immunoreactive for L1-ORF1 encoded proteins (Rodic et al., 2014). The expression of L1 proteins in tumors has been shown to affect the expression of a number of cancer-related genes, including the down-regulation of tumor suppressors (Rangasamy et al., 2015). With respect to TE insertional activity, studies on matched normal and tumor tissues have found that novel L1 insertions occur at high frequencies in lung cancer genomes (Iskow et al., 2010). Such insertions frequently occur in oncogenes and tumor suppressors, underscoring their putative role in tumorigenesis (Lee et al., 2012).
A principal challenge when interpreting cancer genomes is distinguishing between so-called passenger and driver mutations. While passenger mutations are present in cancer genomes, they are not considered to contribute to cancer progression; instead, they are simply somatic mutations that arise during carcinogenesis and are carried along during clonal expansion. Driver mutations, on the other hand, are causal mutations that are directly implicated in carcinogenesis and the promotion of cancer growth (Stratton et al., 2009; Marx, 2014; Pon and Marra, 2015). To date, only a few studies have directly implicated TE insertions as cancer driver mutations. One such study analyzed 19 hepatocellular carcinoma genomes utilizing the RC-Seq methodology (Baillie et al., 2011) and discovered two separate L1 insertions that initiate tumorigenesis via distinct oncogenic pathways (Shukla et al., 2013). This study found L1 insertions in two different tumor suppressor genes: Mutated in Colorectal Cancers (MCC) and Suppression of Tumorigenicity (ST18). Most recently, a role for L1 insertional activity was conclusively demonstrated for colorectal cancer caused by an insertion in the APC tumor suppressor gene (Scott et al., 2016). This paper describes a somatic L1 insertion into one copy of the APC gene that, when coupled with a point mutation in the other copy of the gene, initiates tumorigenesis through the two hit colorectal cancer pathway.
Owing to parallel developments in genomics and bioinformatics, it is now possible to jointly analyze the patterns of TE transcript expression and TE insertional activity in human cancers. The Cancer Genome Atlas (TCGA) provides access to both transcriptome sequence data (RNA-seq) and whole genome sequence data (DNA-seq) for a number of matched normal and primary tumor sample pairs from individual patients (Weinstein et al., 2013). In addition, recently developed bioinformatics algorithms allow for the detection of TE transcripts directly from RNA-seq data (Jin et al., 2015) as well as for the characterization of novel TE insertions from DNA-seq data (Thung et al., 2014; Sudmant et al., 2015). We took advantage of these developments in order to evaluate the patterns of both TE expression and insertional activity in three cancer types: breast invasive carcinoma, head, and neck squamous cell carcinoma, and lung adenocarcinoma (Figure 1 and Supplementary Figure 1). We observed a simultaneous increase of L1 transcript expression and L1 insertional activity for primary tumor samples for all three cancers, and we evaluate individual cases of TE insertions that are implicated as potential cancer causing mutations.
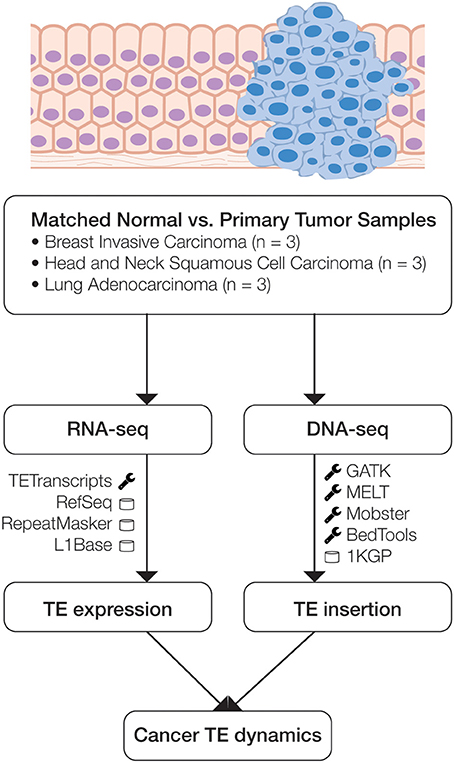
Figure 1. Scheme of the analytical design used in this study. Matched normal and primary tumor samples for three cancer types were analyzed using transcriptome (RNA-seq) and whole genome (DNA-seq) data. RNA-seq data was analyzed to compare normal versus cancer expression levels, and DNA-seq data was analyzed to identify somatic TE insertion events. The main bioinformatics programs (wrench) and databases (cylinder) used for each phase of the analysis are indicated.
Materials and Methods
Genome and Transcriptome Sequence Data
Whole genome sequence data (DNA-seq), transcriptome sequence data (RNA-seq) and patient metadata for matched normal and primary tumor tissue samples from nine cancer patients were acquired from The TCGA (Weinstein et al., 2013) via the Cancer Genomics Hub (CGHub) using the download client GeneTorrent (Maltbie et al., 2013). The nine participants included three breast invasive carcinoma patients, three head and neck squamous cell carcinoma patients and three lung adenocarcinoma patients (Table 1). DNA-seq and RNA-seq data were accessed as BAM files of paired-end Illumina sequence data aligned against the human genome reference sequence (build hg19). BAM files containing sequence alignments were validated for quality using FASTQC (Andrews, 2011), and autosomes were extracted from the BAM files for downstream analysis using SAMtools (Li et al., 2009).
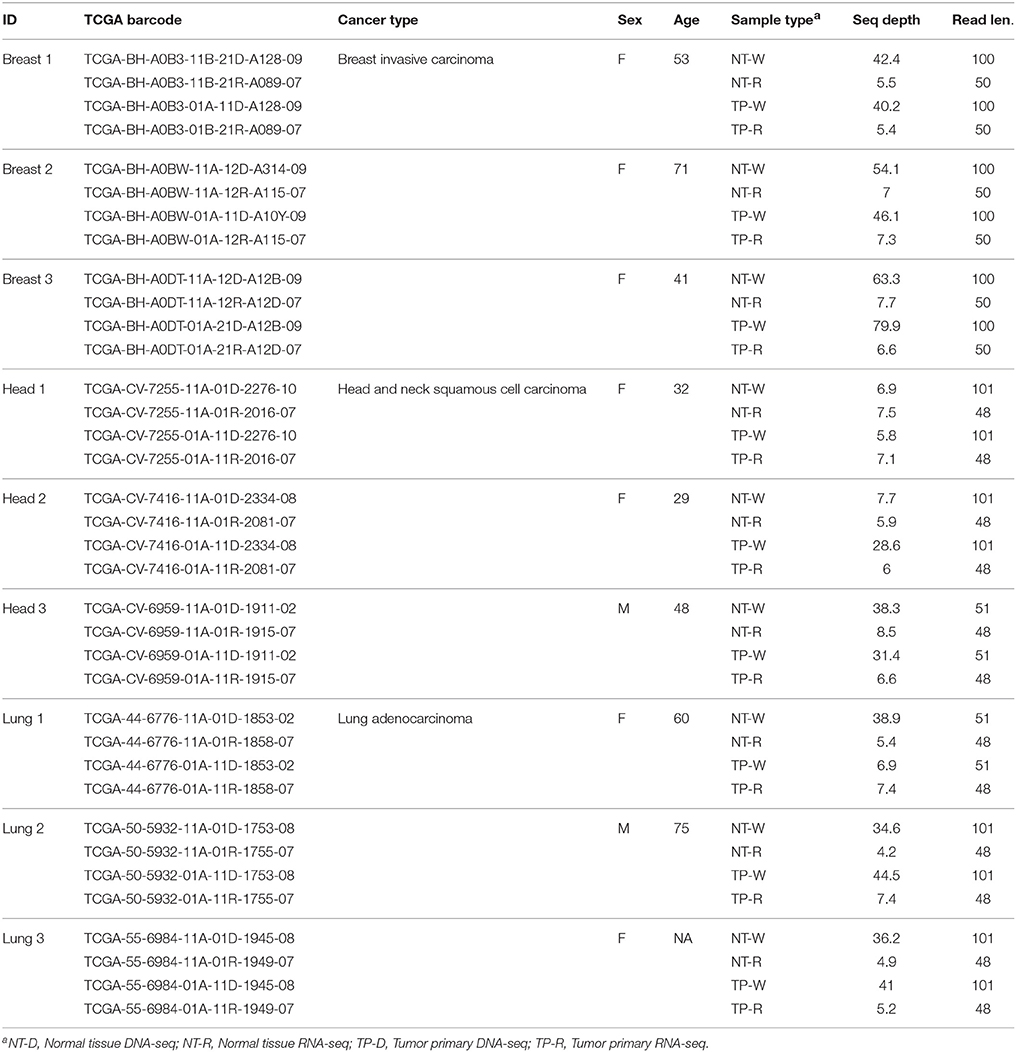
Table 1. TCGA whole genome (DNA-seq) and transcriptome (RNA-seq) data sources for the patients analyzed in this study.
Gene and Transposable Element (TE) Expression Levels
Gene and TE expression levels were measured using RNA-seq data for the nine matched normal and primary tumor tissue samples. Gene expression levels were quantified as read counts mapped to NCBI RefSeq gene annotations (Pruitt et al., 2012). TE expression levels—for Alu, L1 and SVA elements—were quantified using reads mapped to RepeatMasker annotations, which were subsequently analyzed with the TEtranscripts package (Jin et al., 2015). The TEtranscripts program uses an expectation maximization (EM) algorithm to choose optimal unique TE locations for multi-mapped reads, thereby allowing for accurate expression level measurements for active TE families. The TEtranscripts method was recently shown to yield more reliable measures of TE transcription levels compared to previously published methods, such as HTSeq-count, Cufflinks, and RepEnrich (Trapnell et al., 2010; Criscione et al., 2014; Anders et al., 2015). The L1Base database was used to identify the genomic locations of 145 full length, intact elements from the most recently active L1 subfamily (Penzkofer et al., 2005). The set of full-length intact L1 sequences from the L1Base was generated by performing a BLAST search using the human genomic DNA sequences against the L1 template sequence (Penzkofer et al., 2005). L1Base was used to facilitate measures of active L1 element expression by limiting our analysis to RNA-seq reads that map to full-length, intact L1 sequences which retain the potential to be transpositionally active. This was done in an effort to ensure that the reads we analyzed were taken from potentially active L1 elements as opposed to older fixed elements, which could represent read-through transcripts initiated from nearby genomic promoters. The expression levels of these potentially active L1 elements were analyzed separately using the TEtranscripts method.
Differential expression levels between normal and cancer tissue pairs, for genes and TEs, were evaluated by comparing distributions of log10 transformed RNA-seq expression levels characterized as described above. The statistical significance levels of the observed differential expression between normal and cancer pairs were evaluated by comparing these distributions using the non-parametric Kolmogorov-Smirnov test. Statistical comparisons were done separately for each tissue (cancer) type: breast invasive carcinoma, head and neck squamous cell carcinoma and lung adenocarcinoma.
Transposable Element Insertion Detection
The genomic locations of novel TE insertions from matched normal and primary tumor tissue samples were predicted based on discordant read-pair mapping of DNA-seq data (Ewing, 2015) (Table 2). A scheme of our TE insertion detection analysis pipeline is shown in Supplementary Figure 2. DNA-seq BAM files were realigned according to GATK's standard indel realignment method (Van der Auwera et al., 2013) to facilitate TE insertion detection. The programs MELT (Sudmant et al., 2015) and Mobster (Thung et al., 2014) were used together for TE insertion detection. These two programs were selected owing to their previously demonstrated superior performance for human TE insertion detection (Rishishwar et al., 2016). Only TE insertion sites that were found by both methods (i.e., the intersection of the predictions) were used for subsequent analysis. TE insertion predictions made by the individual programs were considered to represent the same insertion if they were found within ±100 bp of each other. An additional filtering step was applied based on the number of mapped sequence reads (coverage) that support each TE insertion prediction. Only predictions with a minimum coverage of 5 reads and a maximum coverage of 4X the average sequencing depth of the sample were used for subsequent analysis. These upper and lower cut-off thresholds were empirically chosen based on the observed distributions of the numbers of discordant mapped read pairs used to call individual TE insertions. Read count distributions were computed individually for each program (MELT, Mobster) used and for each sample (Supplementary Figure 3). The resulting distributions were typically bimodal with a lower peak (i.e., with lower read count support) that we considered to be enriched for potential false positive TE insertion calls. The lower cut-off threshold of 5 reads was chosen to minimize such false positives, and the upper cut-off threshold was chosen to remove calls made in genomic regions that show anomalously high numbers of mapped reads, which tend to be enriched for ambiguously mapped reads.
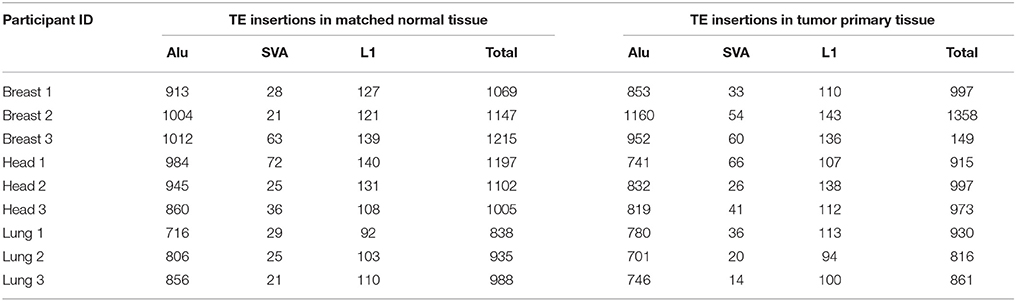
Table 2. Numbers of MELT and Mobster predicted TE insertions in matched normal (N) and primary tumor (T) samples across 9 individuals.
The number of observed versus expected counts of unique L1 insertions were compared for matched normal and primary tumor tissue samples. The observed counts were taken from the TE detection pipeline, and the expected counts were computed as the ratio of unique insertions seen in matched normal vs. primary tissue for all TEs multiplied by the total number of observed L1 insertions. The significance of the difference between the observed versus expected counts of unique L1 insertions was evaluated using the Fisher's exact test. Counts of TE insertions for matched normal and primary tumor tissue samples were characterized based on their frequencies from the 1000 Genomes Project (1KGP) (Sudmant et al., 2015) and grouped into three distinct frequency bins. The distributions of TE insertion counts across the three frequency bins were compared for matched normal and cancer samples for the different tissue types analyzed here, and the significance of the differences between these distributions were evaluated using the Kolmogorov-Smirnov test.
TE Insertion Genome Feature Analysis
The genomic locations of novel TE insertions were considered with respect to several genomic features using the BEDTools program (Quinlan, 2014): RefSeq genes (Pruitt et al., 2012), COSMIC tumor suppressor genes (Forbes et al., 2015), and enhancer elements defined by chromatin states (Roadmap Epigenomics et al., 2015). The population allele frequencies of the predicted TE insertions were computed from the Phase 3 release of the 1KGP (Sudmant et al., 2015) as previously described (Rishishwar et al., 2015).
Results and Discussion
TE Expression Levels in Matched Normal vs. Primary Tumor Tissue Samples
RNA-seq data were used to evaluate the differences in TE expression levels between matched normal and primary tumor tissue samples as described in the Materials and Methods. The observed differences in gene expression levels between normal and tumor tissue were compared to differences in TE expression levels for breast invasive carcinoma, head, and neck squamous cell carcinoma and lung adenocarcinoma. There are no significant differences observed for the distributions of gene expression levels between matched normal and primary tumor tissue pairs for any of the three cancer types analyzed here (Figure 2). Similarly, when all three families of potentially active TEs (Alu, L1, and SVA) are considered together, there is no significant difference seen for the overall levels of expression between matched normal and tumor tissue. However, when full-length, potentially active L1 sequences are considered alone, we observe statistically significant increases in L1 expression levels for all three cancer types.
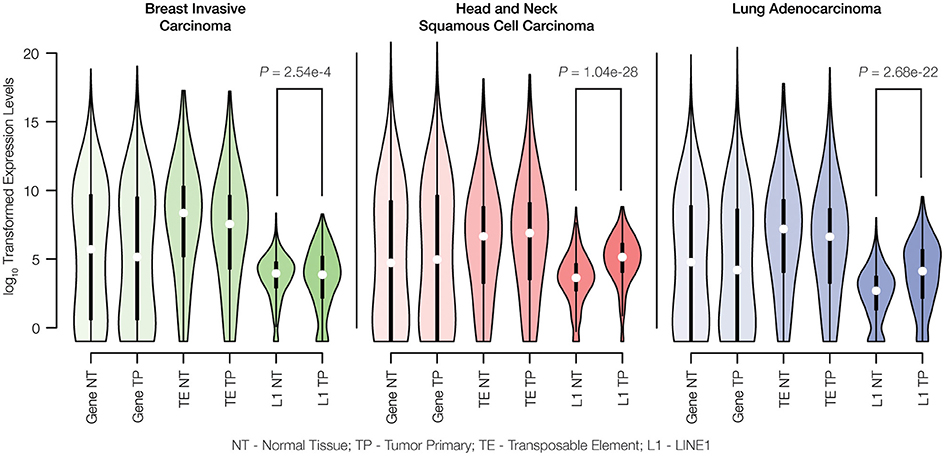
Figure 2. Gene expression levels for matched normal vs. primary tumor tissue pairs. Normal tissue (NT) and tumor primary (TP) expression levels were measured for genes, transposable elements (TEs) and LINE1 elements (L1s) via analysis of RNA-seq data as described in the Materials and Methods. Expression levels are shown as distributions of log10 transformed read counts, and normal versus tumor comparisons are shown for breast invasive carcinoma (green), head and neck squamous cell carcinoma (red), and lung adenocarcinoma (blue). For each tissue type, the significance levels of the differences in L1 expression between normal and cancer pairs are indicated with P-values from the Kolmogorov-Smirnov test.
The methods that we used to characterize TE expression levels include several analytical controls aimed to ensure that only genuine TE-initiated transcripts, from members of potentially active families, are measured. Nevertheless, the lack of a difference between normal and tumor expression levels observed when all three active TE families were considered together could reflect technical difficulties with identifying bona fide TE transcripts that are initiated from element promoters as opposed to TE sequences that are passively expressed as part of longer genic transcripts. This is particularly true for Alu elements, many of which are found in the introns of human genes and transcribed as read-through transcripts initiated from RNA Pol II gene promoters (Deininger, 2011). Our confidence in the ability to measure L1-initiated transcripts is higher owing to the focus on previously identified full-length, intact elements that are located in intergenic regions. In any case, the up-regulation of L1s in cancer that we observed has potential implications for increased TE insertional activity for all three families, since L1 encoded proteins are responsible for the cis retrotransposition of L1s as well as the trans activation of Alu and SVA elements (Batzer and Deininger, 2002; Hancks and Kazazian, 2010). We analyzed the same pairs of matched normal and primary tumor tissues to evaluate whether the observed increase in L1 expression corresponds to increased transpositional activity of human TEs.
Novel TE Insertions in Matched Normal and Primary Tumor Tissue Samples
It is now possible to characterize the genomic locations and copy numbers of individual TE insertions from whole genome DNA-seq data owing to recent developments in computational genomics software (Ewing, 2015; Rishishwar et al., 2016). This technological advance is exemplified by the recent Phase 3 release of the 1KGP, which includes a complete genome-wide census of polymorphic TE insertion sites for 2504 individuals across 26 human populations (Sudmant et al., 2015). We analyzed whole genome DNA-seq data using computational methods for TE insertion detection (see Materials and Methods) in order to compare TE insertional activity between matched normal versus primary tumor tissue samples.
When all three families of active human TEs are considered together, we observed a total of 3672 TE insertions across the nine individuals analyzed for normal and cancer tissue pairs, 693 of which are unique insertions found in only one individual and one tissue type. In other words, we observe an average of ~77 unique somatic TE insertions per person, i.e., “private” TE insertions. This estimate is similar to the value of ~90 unique (presumably germline) TE insertions that we previously observed for individuals from the 1KGP (Rishishwar et al., 2015). A large majority of the observed TE insertions—81% for all TEs and 62% for L1s alone—are shared between the normal and tumor tissue types of an individual, suggesting that they represent germline insertions (Figure 3A). There are 1.3x more unique TE insertions seen for tumor compared to normal tissue, and this effect is more pronounced for L1s alone, which are 2x more abundant in tumor tissue samples. Accordingly, there is a statistically significant excess of observed versus expected L1 insertions in tumor versus normal tissue (P = 0.019) (Figure 3B). These results are consistent with a potential role for L1 transpositional activity in tumorigenesis for the cancer types analyzed here, as has been previously suggested for several different cancers (Morse et al., 1988; Iskow et al., 2010; Lee et al., 2012; Scott et al., 2016).
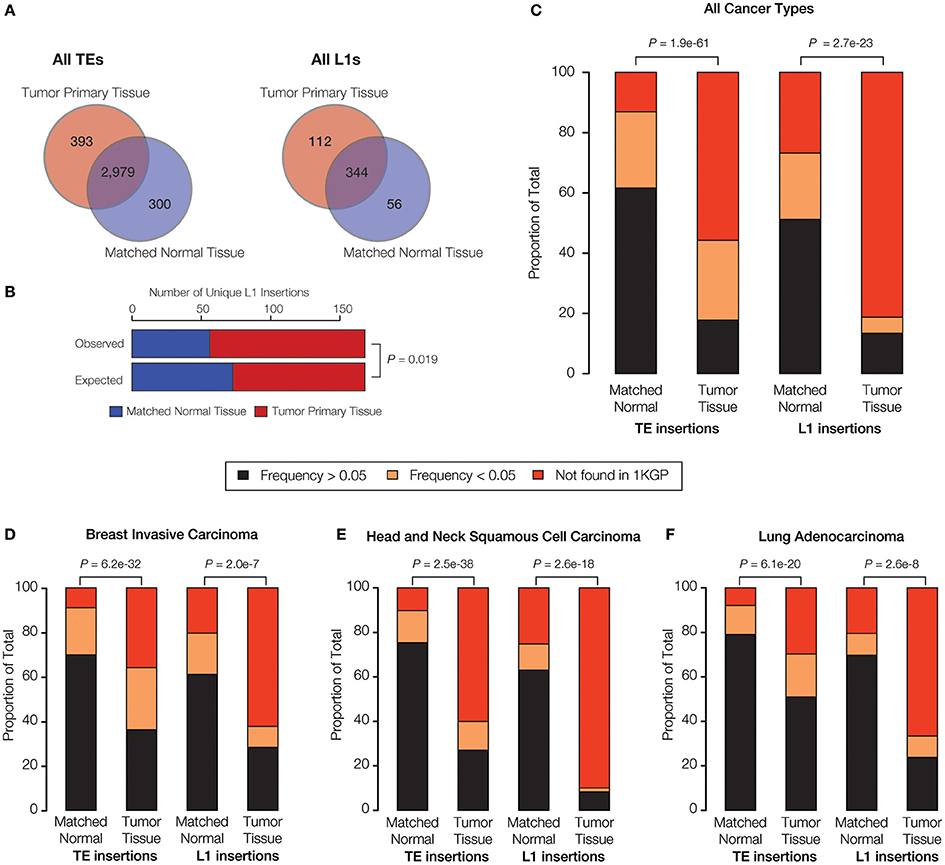
Figure 3. TE insertional activity in matched normal vs. primary tumor tissue pairs. The number of TE insertions were measured for normal and primary tumor tissue pairs for breast invasive carcinoma, head, and neck squamous cell carcinoma and lung adenocarcinoma via analysis of whole genome DNA-seq data as described in the Materials and Methods. (A) The total number of predicted TE insertions, pooled for all nine individuals over the three cancer types analyzed here, are shown for normal vs. tumor tissue. Venn diagrams show the numbers of unique versus shared TE insertions for the two tissue types. (B) Comparison of the observed versus expected numbers of unique L1 insertions for normal vs. tumor tissue. (C) Comparison of the population frequencies of observed TE insertions in matched normal vs. tumor tissue pairs are shown for all of the TEs analyzed here and for L1s alone. (D–F) The same comparisons of TE insertion population frequencies are shown individually for each cancer type analyzed here. TE insertion population frequencies are color coded as shown in the key. P-values show the significance of the differences for observed distributions based on the Fisher's exact test (B) and the Kolmogorov-Smirnov test (C–F).
Given the relatively high level of L1 insertional activity in the tumor tissue samples analyzed here, we tested whether tumor-specific L1 insertions are found at lower frequencies among the (presumably) healthy donors from the 1KGP compared to L1 insertions found in matched normal tissue. The idea was to evaluate whether the tumor-specific L1 insertions represent mutations that are private, and thereby more likely to be deleterious or disease-causing. To do this, individual TE insertions were classified as high frequency (>0.05), low frequency (<0.05) or private (absent) according to their previously characterized population (allele) frequencies from the 1KGP (Rishishwar et al., 2015; Sudmant et al., 2015).
When all three cancer types are considered together, there is a statistically significant excess of private and low frequency TE insertions observed for tumor compared to normal tissue (P = 1.9e-61) (Figure 3C). This effect is even more pronounced when L1 insertions are considered alone (P = 2.7e-23). The same pattern of an increased frequency of private L1 insertions in tumor tissue is observed (P < 2.0e-7) when all three cancer types are analyzed for sets of patients (Figures 3D–F) and when samples for individual patients are analyzed separately (Supplementary Figure 4). The strongest effect is seen for head and neck squamous cell carcinoma. The pattern of a significant excess of private L1 insertions in tumor compared to normal tissue, observed for all three cancer types studied here, provides further evidence in support of a possible role for L1 activity in tumorigenesis.
It should be noted TE insertions found in low copy numbers may not be detectable using next-generation sequence analysis, whereas such insertions may be uncovered using more sensitive PCR-based approaches. False negatives of this kind will be more prevalent at low levels of sequence coverage. We have tried to control for this by using relatively high sequence coverage (~35X) studies here, but the conservative lower read count cut-off of 5 reads per TE insertion call that we used may still lead to missing TE insertion calls. Sequence based predictions can also yield false-positive TE insertion calls. In an effort to deal with this issue, we have only used high-confidence calls produced by two independent programs—MELT and Mobster—that we have recently shown to be most reliable for the detection of human TE insertions (Rishishwar et al., 2016).
One other potential problem with the sequence based analysis relates to the base pair resolution with which TE insertions can be called via computational analysis of next-generation sequence data. Currently, the most accurate programs for calling TE insertions from next-generation sequence data do not yet allow for the insertions to be precisely located to genomic regions at single base pair resolution. To account for this fact, TE insertions called within a window of ±100 bp are considered to be co-located (Supplementary Figure 2). It is possible that this approximation can lead to multiple TE insertion events being collapsed into a single event. Subsequent experimental confirmation of individual TE insertion calls of interest (e.g., potentially tumorigenic TE insertions) should help to provide certainty with respect to both their validity and their precise genomic locations.
Potentially Tumorigenic TE Insertions
Having established a potential role for transpositional activity in tumorigenesis using the genome-wide approaches described above, we wanted to search for specific examples where individual TE insertions could be implicated as possible cancer driver mutations. To do so, we performed an integrated analysis of TE insertion, gene expression and chromatin data (see Materials and Methods) in an effort to identify the cancer-specific TE insertions that are most likely to play a causal role in tumorigenesis. We considered TE insertions that are co-located with either exons or regulatory elements of previously characterized tumor suppressor genes to have the highest likelihood of being functionally relevant. We observed a total of 141 intragenic (35.9%) insertions and 246 intronic insertions (62.6%) out of the 393 total cancer-specific insertions in our dataset. None of these intergenic or intronic cancer-specific TE insertions were found to disrupt any known functional (regulatory) sequence element. Thus, consistent with previous studies, the vast majority of TE insertions that we observed are not likely to affect gene function or expression in cancer. We did find 4 exonic TE insertions, along with 2 insertions located in regulatory elements, for known tumor suppressor genes (1.5% of the total). Here, we focus on two of these potential cases of cancer driver TE insertions, which could prove to be of interest to the TE and/or cancer research communities.
There is a private, breast cancer tumor-specific Alu insertion that is located within an upstream enhancer element that helps to regulate the expression of the Cbl Proto-Oncogene (CBL) gene (Figure 4A). CBL is classified as a tumor suppressor gene by the COSMIC database (Forbes et al., 2015). It has been found to be mutated or translocated in a number of cancers including acute myeloid leukemia (Abbas et al., 2008; Naramura et al., 2011; Aranaz et al., 2013); mutations in CBL are also the cause of Noonan syndrome-like disorder (Martinelli et al., 2010). The CBL encoded protein functions as a negative regulator of signal transduction pathways (Schmidt and Dikic, 2005), activation of which have been associated with cancer (Sever and Brugge, 2015). The tumor-specific Alu enhancer insertion that we characterized is associated with down-regulation of CBL expression, consistent with a potential role in tumorigenesis via the activation of signal transduction pathways associated with cell proliferation (Sever and Brugge, 2015).
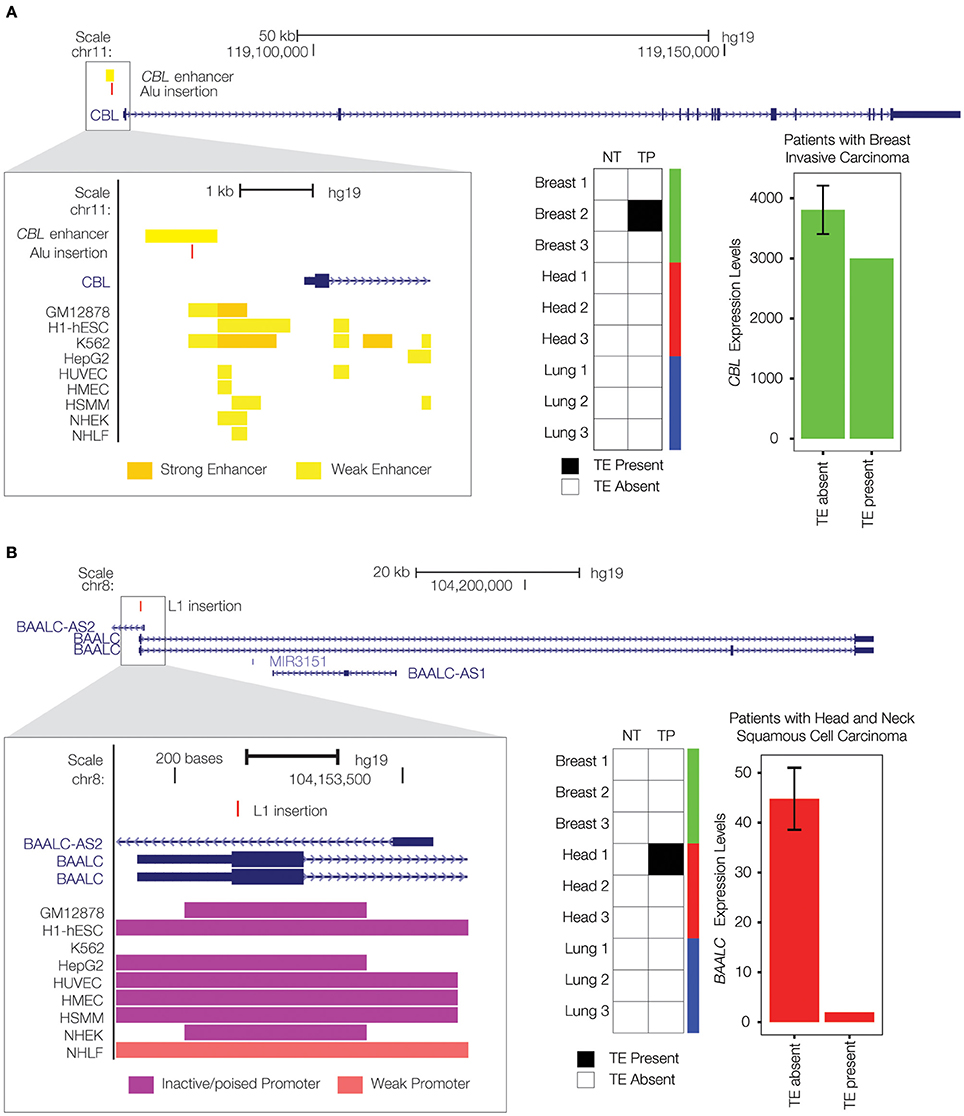
Figure 4. Private TE insertions implicated as potential cancer driver mutations. (A) A tumor-specific Alu insertion (red) is found in a single breast cancer patient. The insertion is located within an upstream enhancer for the CBL gene on chromosome 11 (gene model shown in blue), as indicated by enhancer-associated chromatin marks (inset yellow bars). Presence of the Alu insertion is associated with down-regulation of CBL (expression levels in green). (B) A tumor-specific L1 insertion (red) is located within the first exon of the BAALC gene on chromosome 8 (gene model shown in blue). Co-location of the L1 insertion with promoter-associated chromatin marks (purple bars) is shown in the inset. Presence of the L1 insertion is associated with down-regulation of BAALC (expression levels in red).
We also found a private L1 insertion that was unique to a head and neck squamous cell carcinoma tissue sample, located within the first exon of the Brain and Acute Leukemia, Cytoplasmic (BAALC) gene (Figure 4B). As its name implies, the BAALC gene is expressed in the brain and related neural tissues, and it was first identified by association with acute myeloid leukemia where it was shown to be overexpressed (Damiani et al., 2013; Zhou et al., 2015). TE insertions within exons are extremely rare and would presumably have a dramatic effect on gene function. Indeed, this particular insertion is associated with nearly complete inactivation of the BAALC gene. This is consistent with previous results showing that the presence of fixed L1 insertions genome-wide is strongly associated with the down-regulation of human gene expression (Han et al., 2004). A recent study has demonstrated that BAALC can inhibit extracellular signal-regulated kinase (ERK) mediated monocytic differentiation of AML cells (Morita et al., 2015). Thus, down-regulation of BAALC would presumably result in a loss of control over cellular differentiation, consistent with a possible role in tumorigenesis. A recent study discovered a role for the change in methylation status of a cancer-specific L1 insertion in tumorigenesis (Scott et al., 2016); this could be an additional mechanism by which the BAALC L1 insertion observed here exerts a regulatory effect.
Conclusion
The results of our analysis show a surprisingly high level of somatic TE activity in the human genome. Abundant transcripts from members of all three active human TE families analyzed here—Alu, SVA and L1—can be identified for both normal and cancer tissue samples. In addition, after filtering for high confidence TE insertion calls, we identified an average of close to 80 unique insertions for each tissue among the individual patients in our study. Thus, active human TE families retain the ability to transpose in somatic tissue thereby generating substantial levels of cellular heterogeneity among diverse tissues.
We also observe a correlated increase in both transcript expression levels and transpositional activity for L1 elements in cancer tissue samples when compared to matched normal tissue. Increased cancer expression of L1 elements is particularly relevant for TE insertional activity, since the L1 transpositional machinery is responsible for transposing non-autonomous Alu and SVA elements in trans along with L1 elements in cis. Our results are consistent with previous studies showing expression of L1 transcripts in lung cancer (Belancio et al., 2010b) and expression of L1 ORF1p in breast cancer (Harris et al., 2010), and tumor-specific L1 insertions have also previously been found in breast (Morse et al., 1988), head and neck (Helman et al., 2014), and lung tumors (Helman et al., 2014). We confirmed the presence of numerous tumor-specific L1 insertions in these three cancer types and identify two potentially tumorigenic TE insertions, an Alu insertion in the enhancer region of the tumor suppressor gene CBL and an L1 insertion in the first exon of the BAALC gene. These results underscore the potential for somatic TE activity to generate cellular heterogeneity and to contribute to the etiology of cancer across a wide range of human tissues.
Ethics Statement
Ethical approval was not required for this study on restricted access, de-identified data in accordance with the guidelines of the Cancer Genome Atlas (TCGA). Access to the data was approved by the data access committee of the TCGA.
Author Contributions
EC, LW, and LR performed all of the analyses described in the study. JW contributed to the genome feature analysis. IJ and JM conceived of designed and supervised the study. All authors contributed to the drafting and revision of the manuscript.
Funding
EC and LW were supported by the Georgia Tech Bioinformatics Graduate Program. LR and IJ were supported by the IHRC-Georgia Tech Applied Bioinformatics Laboratory (ABiL).
Conflict of Interest Statement
The authors declare that the research was conducted in the absence of any commercial or financial relationships that could be construed as a potential conflict of interest.
Acknowledgments
The results published here are in whole or part based upon data generated by The Cancer Genome Atlas managed by the NCI and NHGRI. Information about TCGA can be found at http://cancergenome.nih.gov. The authors thank Emily Norris for feedback on the manuscript.
Supplementary Material
The Supplementary Material for this article can be found online at: https://www.frontiersin.org/article/10.3389/fmolb.2016.00076/full#supplementary-material
References
Abbas, S., Rotmans, G., Löwenberg, B., and Valk, P. J. (2008). Exon 8 splice site mutations in the gene encoding the E3-ligase CBL are associated with core binding factor acute myeloid leukemias. Haematologica 93, 1595–1597. doi: 10.3324/haematol.13187
Anders, S., Pyl, P. T., and Huber, W. (2015). HTSeq–a Python framework to work with high-throughput sequencing data. Bioinformatics 31, 166–169. doi: 10.1093/bioinformatics/btu638
Andrews, S. (2011). FastQC A Quality Control Tool for High Throughput Sequence Data. Cambridge: Babraham Institute.
Aranaz, P., Miguéliz, I., Hurtado, C., Erquiaga, I., Larrayoz, M. J., Calasanz, M. J., et al. (2013). CBL RING finger deletions are common in core-binding factor acute myeloid leukemias. Leuk. Lymphoma 54, 428–431. doi: 10.3109/10428194.2012.709629
Asch, H. L., Eliacin, E., Fanning, T. G., Connolly, J. L., Bratthauer, G., and Asch, B. B. (1996). Comparative expression of the LINE-1 p40 protein in human breast carcinomas and normal breast tissues. Oncol. Res. 8, 239–247.
Baillie, J. K., Barnett, M. W., Upton, K. R., Gerhardt, D. J., Richmond, T. A., De Sapio, F., et al. (2011). Somatic retrotransposition alters the genetic landscape of the human brain. Nature 479, 534–537. doi: 10.1038/nature10531
Batzer, M. A., and Deininger, P. L. (1991). A human-specific subfamily of Alu sequences. Genomics 9, 481–487. doi: 10.1016/0888-7543(91)90414-A
Batzer, M. A., and Deininger, P. L. (2002). Alu repeats and human genomic diversity. Nat. Rev. Genet. 3, 370–379. doi: 10.1038/nrg798
Batzer, M. A., Gudi, V. A., Mena, J. C., Foltz, D. W., Herrera, R. J., and Deininger, P. L. (1991). Amplification dynamics of human-specific (HS) Alu family members. Nucleic Acids Res. 19, 3619–3623. doi: 10.1093/nar/19.13.3619
Belancio, V. P., Roy-Engel, A. M., and Deininger, P. L. (2010a). All y'all need to know 'bout retroelements in cancer. Semin. Cancer Biol. 20, 200–210. doi: 10.1016/j.semcancer.2010.06.001
Belancio, V. P., Roy-Engel, A. M., Pochampally, R. R., and Deininger, P. (2010b). Somatic expression of LINE-1 elements in human tissues. Nucleic Acids Res. 38, 3909–3922. doi: 10.1093/nar/gkq132
Bratthauer, G. L., Cardiff, R. D., and Fanning, T. G. (1994). Expression of LINE-1 retrotransposons in human breast cancer. Cancer 73, 2333–2336.
Bratthauer, G. L., and Fanning, T. G. (1992). Active LINE-1 retrotransposons in human testicular cancer. Oncogene 7, 507–510.
Bratthauer, G. L., and Fanning, T. G. (1993). LINE-1 retrotransposon expression in pediatric germ cell tumors. Cancer 71, 2383–2386.
Brouha, B., Schustak, J., Badge, R. M., Lutz-Prigge, S., Farley, A. H., Moran, J. V., et al. (2003). Hot L1s account for the bulk of retrotransposition in the human population. Proc. Natl. Acad. Sci. U.S.A. 100, 5280–5285. doi: 10.1073/pnas.0831042100
Carreira, P. E., Richardson, S. R., and Faulkner, G. J. (2014). L1 retrotransposons, cancer stem cells and oncogenesis. FEBS J. 281, 63–73. doi: 10.1111/febs.12601
Criscione, S. W., Zhang, Y., Thompson, W., Sedivy, J. M., and Neretti, N. (2014). Transcriptional landscape of repetitive elements in normal and cancer human cells. BMC Genomics 15:583. doi: 10.1186/1471-2164-15-583
Damiani, D., Tiribelli, M., Franzoni, A., Michelutti, A., Fabbro, D., Cavallin, M., et al. (2013). BAALC overexpression retains its negative prognostic role across all cytogenetic risk groups in acute myeloid leukemia patients. Am. J. Hematol. 88, 848–852. doi: 10.1002/ajh.23516
Deininger, P. (2011). Alu elements: know the SINEs. Genome Biol. 12:236. doi: 10.1186/gb-2011-12-12-236
de Koning, A. P., Gu, W., Castoe, T. A., Batzer, M. A., and Pollock, D. D. (2011). Repetitive elements may comprise over two-thirds of the human genome. PLoS Genet. 7:e1002384. doi: 10.1371/journal.pgen.1002384
Doucet-O'Hare, T. T., Rodic, N., Sharma, R., Darbari, I., Abril, G., Choi, J. A., et al. (2015). LINE-1 expression and retrotransposition in Barrett's esophagus and esophageal carcinoma. Proc. Natl. Acad. Sci. U.S.A. 112, E4894–E4900. doi: 10.1073/pnas.1502474112
Doucet-O'Hare, T. T., Sharma, R., Rodic, N., Anders, R. A., Burns, K. H., and Kazazian, H. H. Jr. (2016). Somatically Acquired LINE-1 Insertions in Normal Esophagus Undergo Clonal Expansion in Esophageal Squamous Cell Carcinoma. Hum. Mutat. 37, 942–954. doi: 10.1002/humu.23027
Ewing, A. D. (2015). Transposable element detection from whole genome sequence data. Mob. DNA 6, 24. doi: 10.1186/s13100-015-0055-3
Ewing, A. D., Gacita, A., Wood, L. D., Ma, F., Xing, D., Kim, M. S., et al. (2015). Widespread somatic L1 retrotransposition occurs early during gastrointestinal cancer evolution. Genome Res. 25, 1536–1545. doi: 10.1101/gr.196238.115
Forbes, S. A., Beare, D., Gunasekaran, P., Leung, K., Bindal, N., Boutselakis, H., et al. (2015). COSMIC: exploring the world's knowledge of somatic mutations in human cancer. Nucleic Acids Res. 43(Database issue), D805–D811. doi: 10.1093/nar/gku1075
Han, J. S., Szak, S. T., and Boeke, J. D. (2004). Transcriptional disruption by the L1 retrotransposon and implications for mammalian transcriptomes. Nature 429, 268–274. doi: 10.1038/nature02536
Hancks, D. C., and Kazazian, H. H. Jr. (2010). SVA retrotransposons: evolution and genetic instability. Semin. Cancer Biol. 20, 234–245. doi: 10.1016/j.semcancer.2010.04.001
Hancks, D. C., and Kazazian, H. H. Jr. (2012). Active human retrotransposons: variation and disease. Curr. Opin. Genet. Dev. 22, 191–203. doi: 10.1016/j.gde.2012.02.006
Harris, C. R., Normart, R., Yang, Q., Stevenson, E., Haffty, B. G., Ganesan, S., et al. (2010). Association of nuclear localization of a long interspersed nuclear element-1 protein in breast tumors with poor prognostic outcomes. Genes Cancer 1, 115–124. doi: 10.1177/1947601909360812
Helman, E., Lawrence, M. S., Stewart, C., Sougnez, C., Getz, G., and Meyerson, M. (2014). Somatic retrotransposition in human cancer revealed by whole-genome and exome sequencing. Gen. Res. 24, 1053–1063. doi: 10.1101/gr.163659.113
Iskow, R. C., McCabe, M. T., Mills, R. E., Torene, S., Pittard, W. S., Neuwald, A. F., et al. (2010). Natural mutagenesis of human genomes by endogenous retrotransposons. Cell 141, 1253–1261. doi: 10.1016/j.cell.2010.05.020
Jin, Y., Tam, O. H., Paniagua, E., and Hammell, M. (2015). TEtranscripts: a package for including transposable elements in differential expression analysis of RNA-seq datasets. Bioinformatics 31, 3593–3599. doi: 10.1093/bioinformatics/btv422
Kazazian, H. H. Jr., Wong, C., Youssoufian, H., Scott, A. F., Phillips, D. G., and Antonarakis, S. E. (1988). Haemophilia A resulting from de novo insertion of L1 sequences represents a novel mechanism for mutation in man. Nature 332, 164–166. doi: 10.1038/332164a0
Kemp, J. R., and Longworth, M. S. (2015). Crossing the LINE Toward Genomic Instability: LINE-1 Retrotransposition in Cancer. Front. Chem. 3:68. doi: 10.3389/fchem.2015.00068
Lander, E. S., Linton, L. M., Birren, B., Nusbaum, C., Zody, M. C., Baldwin, J., et al. (2001). Initial sequencing and analysis of the human genome. Nature 409, 860–921. doi: 10.1038/35057062
Lee, E., Iskow, R., Yang, L., Gokcumen, O., Haseley, P., Luquette, L. J. III, et al. (2012). Landscape of somatic retrotransposition in human cancers. Science 337, 967–971. doi: 10.1126/science.1222077
Li, H., Handsaker, B., Wysoker, A., Fennell, T., Ruan, J., Homer, N., et al. (2009). The Sequence Alignment/Map format and SAMtools. Bioinformatics 25, 2078–2079. doi: 10.1093/bioinformatics/btp352
Maltbie, D., Ganeshalingam, L., and Allen, P. (2013). System and Method for Secure, High-Speed Transfer of Very Large Files. Google Patents.
Martinelli, S., De Luca, A., Stellacci, E., Rossi, C., Checquolo, S., Lepri, F., et al. (2010). Heterozygous germline mutations in the CBL tumor-suppressor gene cause a Noonan syndrome-like phenotype. Am. J. Hum. Genet. 87, 250–257. doi: 10.1016/j.ajhg.2010.06.015
Marx, V. (2014). Cancer genomes: discerning drivers from passengers. Nat. Methods 11, 375–379. doi: 10.1038/nmeth.2891
Miki, Y., Nishisho, I., Horii, A., Miyoshi, Y., Utsunomiya, J., Kinzler, K. W., et al. (1992). Disruption of the APC gene by a retrotransposal insertion of L1 sequence in a colon cancer. Cancer Res. 52, 643–645.
Mills, R. E., Bennett, E. A., Iskow, R. C., and Devine, S. E. (2007). Which transposable elements are active in the human genome? Trends Genet. 23, 183–191. doi: 10.1016/j.tig.2007.02.006
Morita, K., Masamoto, Y., Kataoka, K., Koya, J., Kagoya, Y., Yashiroda, H., et al. (2015). BAALC potentiates oncogenic ERK pathway through interactions with MEKK1 and KLF4. Leukemia 29, 2248–2256. doi: 10.1038/leu.2015.137
Morse, B., Rotherg, P. G., South, V. J., Spandorfer, J. M., and Astrin, S. M. (1988). Insertional mutagenesis of the myc locus by a LINE-1 sequence in a human breast carcinoma. Nature 333, 87–90. doi: 10.1038/333087a0
Naramura, M., Nadeau, S., Mohapatra, B., Ahmad, G., Mukhopadhyay, C., Sattler, M., et al. (2011). Mutant Cbl proteins as oncogenic drivers in myeloproliferative disorders. Oncotarget 2, 245–250. doi: 10.18632/oncotarget.233
Ostertag, E. M., Goodier, J. L., Zhang, Y., and Kazazian, H. H. Jr. (2003). SVA elements are nonautonomous retrotransposons that cause disease in humans. Am. J. Hum. Genet. 73, 1444–1451. doi: 10.1086/380207
Penzkofer, T., Dandekar, T., and Zemojtel, T. (2005). L1Base: from functional annotation to prediction of active LINE-1 elements. Nucleic Acids Res. 33(Database issue), D498–D500. doi: 10.1093/nar/gki044
Pon, J. R., and Marra, M. A. (2015). Driver and passenger mutations in cancer. Annu. Rev. Pathol. 10, 25–50. doi: 10.1146/annurev-pathol-012414-040312
Pruitt, K. D., Tatusova, T., Brown, G. R., and Maglott, D. R. (2012). NCBI Reference Sequences (RefSeq): current status, new features and genome annotation policy. Nucleic Acids Res. 40(Database issue), D130–D135. doi: 10.1093/nar/gkr1079
Quinlan, A. R. (2014). BEDTools: the Swiss-Army Tool for Genome Feature Analysis. Curr. Protoc. Bioinformatics 47, 11.12.1–11.12.34. doi: 10.1002/0471250953.bi1112s47
Rangasamy, D., Lenka, N., Ohms, S., Dahlstrom, J. E., Blackburn, A. C., and Board, P. G. (2015). Activation of LINE-1 Retrotransposon Increases the Risk of Epithelial-Mesenchymal Transition and Metastasis in Epithelial Cancer. Curr. Mol. Med. 15, 588–597. doi: 10.2174/1566524015666150831130827
Rishishwar, L., Marino-Ramirez, L., and Jordan, I. K. (2016). Benchmarking computational tools for polymorphic transposable element detection. Brief. Bioinform. doi: 10.1093/bib/bbw072. [Epub ahead of print].
Rishishwar, L., Tellez Villa, C. E., and Jordan, I. K. (2015). Transposable element polymorphisms recapitulate human evolution. Mob. DNA 6, 21. doi: 10.1186/s13100-015-0052-6
Roadmap Epigenomics, C., Kundaje, A., Meuleman, W., Ernst, J., Bilenky, M., Yen, A., et al. (2015). Integrative analysis of 111 reference human epigenomes. Nature 518, 317–330. doi: 10.1038/nature14248
Rodic, N., Sharma, R., Sharma, R., Zampella, J., Dai, L., Taylor, M. S., et al. (2014). Long interspersed element-1 protein expression is a hallmark of many human cancers. Am. J. Pathol. 184, 1280–1286. doi: 10.1016/j.ajpath.2014.01.007
Schmidt, M. H., and Dikic, I. (2005). The Cbl interactome and its functions. Nat. Rev. Mol. Cell Biol. 6, 907–918. doi: 10.1038/nrm1762
Scott, E. C., Gardner, E. J., Masood, A., Chuang, N. T., Vertino, P. M., and Devine, S. E. (2016). A hot L1 retrotransposon evades somatic repression and initiates human colorectal cancer. Genome Res. 26, 745–755. doi: 10.1101/gr.201814.115
Sever, R., and Brugge, J. S. (2015). Signal transduction in cancer. Cold Spring Harb. Perspect. Med. 5:a006098. doi: 10.1101/cshperspect.a006098
Shukla, R., Upton, K. R., Muñoz-Lopez, M., Gerhardt, D. J., Fisher, M. E., Nguyen, T., et al. (2013). Endogenous retrotransposition activates oncogenic pathways in hepatocellular carcinoma. Cell 153, 101–111. doi: 10.1016/j.cell.2013.02.032
Solyom, S., Ewing, A. D., Rahrmann, E. P., Doucet, T., Nelson, H. H., Burns, M. B., et al. (2012). Extensive somatic L1 retrotransposition in colorectal tumors. Genome Res. 22, 2328–2338. doi: 10.1101/gr.145235.112
Solyom, S., and Kazazian, H. H. (2012). Mobile elements in the human genome: implications for disease. Genome Med. 4:12. doi: 10.1186/gm311
Stratton, M. R., Campbell, P. J., and Futreal, P. A. (2009). The cancer genome. Nature 458, 719–724. doi: 10.1038/nature07943
Sudmant, P. H., Rausch, T., Gardner, E. J., Handsaker, R. E., Abyzov, A., Huddleston, J., et al. (2015). An integrated map of structural variation in 2,504 human genomes. Nature 526, 75–81. doi: 10.1038/nature15394
Thung, D. T., de Ligt, J., Vissers, L. E., Steehouwer, M., Kroon, M., de Vries, P., et al. (2014). Mobster: accurate detection of mobile element insertions in next generation sequencing data. Genome Biol. 15:488. doi: 10.1186/s13059-014-0488-x
Trapnell, C., Williams, B. A., Pertea, G., Mortazavi, A., Kwan, G., van Baren, M. J., et al. (2010). Transcript assembly and quantification by RNA-Seq reveals unannotated transcripts and isoform switching during cell differentiation. Nat. Biotechnol. 28, 511–515. doi: 10.1038/nbt.1621
Tubio, J. M., Li, Y., Ju, Y. S., Martincorena, I., Cooke, S. L., Tojo, M., et al. (2014). Mobile DNA in cancer. Extensive transduction of nonrepetitive DNA mediated by L1 retrotransposition in cancer genomes. Science 345:1251343. doi: 10.1126/science.1251343
Van der Auwera, G. A., Carneiro, M. O., Hartl, C., Poplin, R., Del Angel, G., Levy-Moonshine, A., et al. (2013). From FastQ data to high confidence variant calls: the Genome Analysis Toolkit best practices pipeline. Curr. Protoc. Bioinformatics 43, 11 10 11–33. doi: 10.1002/0471250953.bi1110s43
Wang, H., Xing, J., Grover, D., Hedges, D. J., Han, K., Walker, J. A., et al. (2005). SVA elements: a hominid-specific retroposon family. J. Mol. Biol. 354, 994–1007. doi: 10.1016/j.jmb.2005.09.085
Weinstein, J. N., Collisson, E. A., Mills, G. B., Shaw, K. M., Ozenberger, B. A., Ellrott, K., et al. (2013). The Cancer Genome Atlas Pan-Cancer analysis project. Nat. Genet. 45, 1113–1120. doi: 10.1038/ng.2764
Wildschutte, J. H., Williams, Z. H., Montesion, M., Subramanian, R. P., Kidd, J. M., and Coffin, J. M. (2016). Discovery of unfixed endogenous retrovirus insertions in diverse human populations. Proc. Natl. Acad. Sci. U.S.A. 113, E2326–E2334. doi: 10.1073/pnas.1602336113
Keywords: LINE-1, L1, Alu, SVA, retrotransposons, bioinformatics, mutation, tumorigenesis
Citation: Clayton EA, Wang L, Rishishwar L, Wang J, McDonald JF and Jordan IK (2016) Patterns of Transposable Element Expression and Insertion in Cancer. Front. Mol. Biosci. 3:76. doi: 10.3389/fmolb.2016.00076
Received: 24 August 2016; Accepted: 31 October 2016;
Published: 16 November 2016.
Edited by:
Tammy A. Morrish, Formerly affiliated with University of Toledo, USAReviewed by:
David Ray, Mississippi State University, USADavid E. Symer, Ohio State University Comp. Cancer Ctr., USA
Tara Theresa Doucet-O'Hare, National Institutes of Health, USA
Copyright © 2016 Clayton, Wang, Rishishwar, Wang, McDonald and Jordan. This is an open-access article distributed under the terms of the Creative Commons Attribution License (CC BY). The use, distribution or reproduction in other forums is permitted, provided the original author(s) or licensor are credited and that the original publication in this journal is cited, in accordance with accepted academic practice. No use, distribution or reproduction is permitted which does not comply with these terms.
*Correspondence: I. King Jordan, king.jordan@biology.gatech.edu