Model-Free or Not?
- Institute for Medical Physics and Biophysics, Medical Faculty, Leipzig University, Leipzig, Germany
Relaxation in nuclear magnetic resonance is a powerful method for obtaining spatially resolved, timescale-specific dynamics information about molecular systems. However, dynamics in biomolecular systems are generally too complex to be fully characterized based on NMR data alone. This is a familiar problem, addressed by the Lipari-Szabo model-free analysis, a method that captures the full information content of NMR relaxation data in case all internal motion of a molecule in solution is sufficiently fast. We investigate model-free analysis, as well as several other approaches, and find that model-free, spectral density mapping, LeMaster’s approach, and our detector analysis form a class of analysis methods, for which behavior of the fitted parameters has a well-defined relationship to the distribution of correlation times of motion, independent of the specific form of that distribution. In a sense, they are all “model-free.” Of these methods, only detectors are generally applicable to solid-state NMR relaxation data. We further discuss how detectors may be used for comparison of experimental data to data extracted from molecular dynamics simulation, and how simulation may be used to extract details of the dynamics that are not accessible via NMR, where detector analysis can be used to connect those details to experiments. We expect that combined methodology can eventually provide enough insight into complex dynamics to provide highly accurate models of motion, thus lending deeper insight into the nature of biomolecular dynamics.
Introduction
Study of biomolecular function requires understanding the dynamics of the biological system. Nuclear magnetic resonance (NMR), despite many recent technological advances in other techniques, remains a premier method for detailed dynamics characterization. In NMR, one may measure a variety of site-specific relaxation experiments, which provide timescale sensitive information about the motion. By varying the type of experiment (T1, T1ρ, NOE, etc.) or experimental conditions (external magnetic field, applied field strength, magic-angle spinning (MAS) frequency, etc.), the timescale sensitivity of the measurement is modified. Then, one may resolve the dynamics both in space, via site resolution, and in timescale, via multiple experiments (Palmer, 2004; Schanda and Ernst, 2016).
However, is it possible to fully characterize the motions leading to the observed relaxation behavior? Many relaxation experiments in NMR are sensitive to the reorientational motion of anisotropic NMR interaction tensors (NMR relaxation can also be sensitive to change in scalar terms, e.g., isotropic chemical shift). For a given spin, relaxation is usually dominated by only one to two interactions. For example, relaxation of 15N in a protein backbone is determined almost entirely by the reorientation of the one-bond 1H–15N dipole coupling and the 15N chemical shift anisotropy (CSA). But, multiple sources of motion lead to reorientation of the bond. For example, if we suppose the H–N bond to be in a protein, within a helix, then we would have local distortion of the peptide plane (one-bond libration), motion of the peptide plane within the helix, motion of the helix within the protein, and motion of the protein either in solution, in a crystal, a fibril, a membrane, etc.
This degree of complexity is illustrated in Figure 1. For a given bond in a molecule, and a given motion acting on that bond, a distribution of orientations is sampled as illustrated in Figure 1A. The orientational distribution determines the contribution of that motion to the total order parameter,
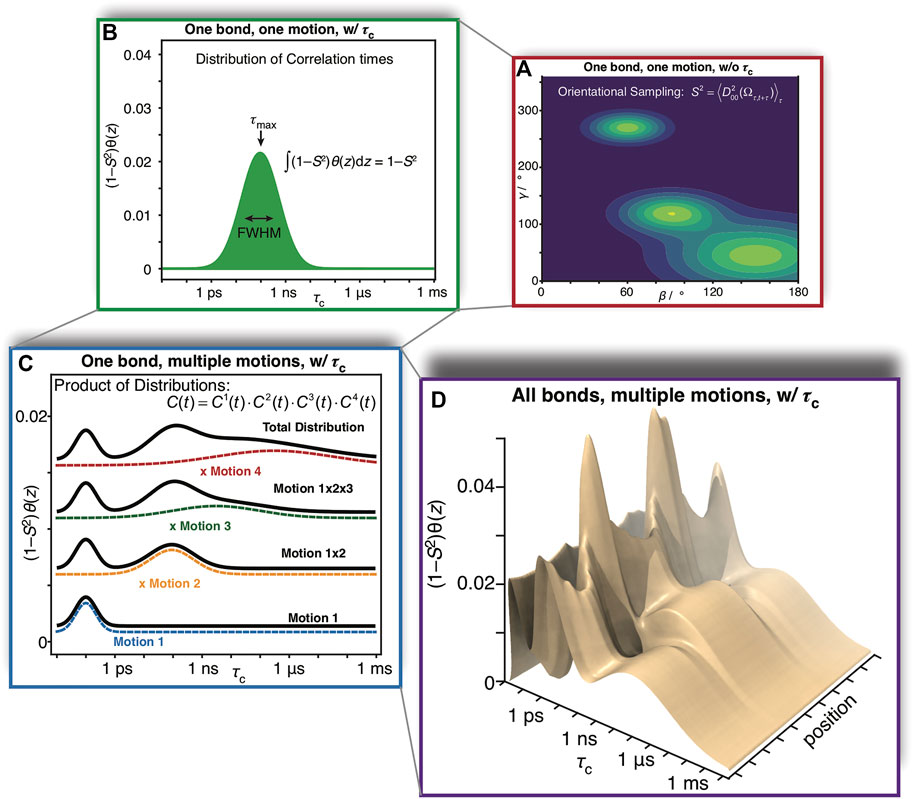
FIGURE 1. Complexity of reorientational dynamics. For each bond in a molecule, multiple types of motion result in orientational sampling, where the distribution of angles for each motion result in a generalized order parameter, S2. Therefore, in (A) we plot a possible distribution of Euler angles for a single type of motion (population is plotted as a function of angles β and γ, where α is not required for a symmetric interaction tensor). A single motion is furthermore described by a correlation time, and may be distributed over a range of correlation times. In (B) we plot a possible distribution of correlation times
While NMR is powerful, obtaining a complete description of the complex dynamics stretches beyond the limit of what is possible based on experimental data alone, especially for large molecules such as proteins. This problem is a familiar one, addressed almost 40 years ago by Lipari and Szabo (Lipari and Szabo, 1982a), who developed a method known as the model-free approach. While we will discuss the details of this approach below, the name tells us a critical advantage of such an approach: model-free analysis allows the extraction of dynamics information from NMR relaxation data without having knowledge of the specific model of motion. Furthermore, the resulting parameters have a well-defined relationship to the distribution of orientations sampled and the distribution of correlation times.
Lipari and Szabo described the internal motion of a molecule with just two parameters: a generalized order parameter related to the amplitude of motion,
The advantage of model-free analysis is that it does not require knowing the model of motion. For example, for relatively low fields (∼90 MHz, as used by Lipari and Szabo), all distributions of orientations and correlation times shown in Figure 2 should yield identical relaxation rate constants for the set of experiments. If we do not know which model is the correct model, the best we can do is to parameterize the results in a way that does not depend on the model of motion, as can be done with the model-free parameters
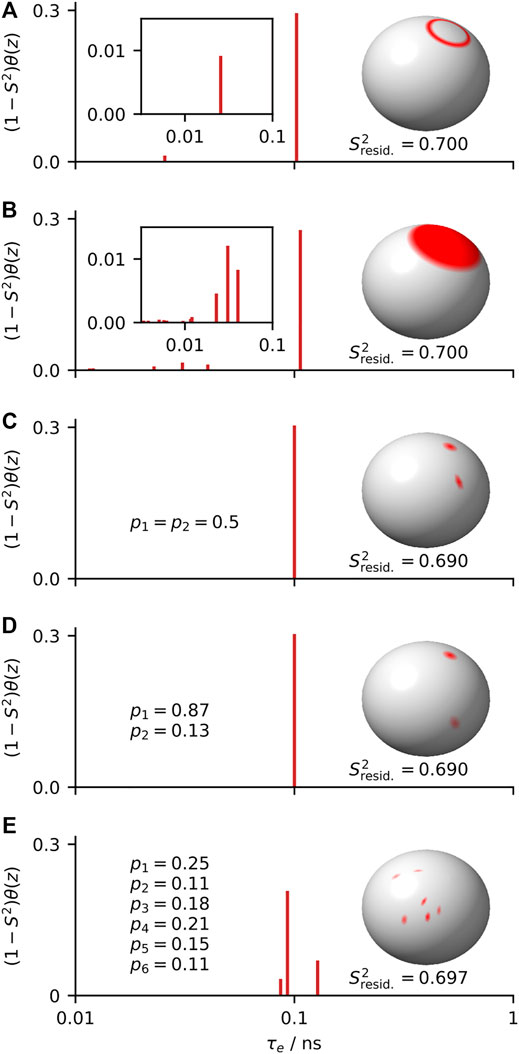
FIGURE 2. Five distributions of orientations and correlation times that yield the same model-free parameters (
When analyzing data, a model provides a framework for understanding the data, and by using a model we are always adding some information to the experimental data. In some cases, we add further information depending on how we interpret a model. A model is advantageous if that information is correct, and disadvantageous if that information is wrong. Suppose, for example, we know that the correct model in Figure 2 is a symmetric two-site hop, shown in Figure 2C; then we may extract the hop angle and exchange rates from
In this review, we will first discuss the original model-free approach, and then examine methods descended from it, including discussion of our own detector analysis, a relatively new approach that also provides a model-free analysis in the spirit of the original Lipari-Szabo approach, but can extract the full information content of relaxation data sets in instances where the model-free approach cannot. We discuss analysis of microsecond motions using R1ρ relaxation, and finally consider how other methods, in particular molecular dynamics (MD) simulation, may be used to supply the information that NMR lacks, thus improving the interpretation of NMR parameters.
Model-Free
While dynamics analysis methods have existed for application to solid-state NMR for some years now (Chevelkov et al., 2009b; Schanda et al., 2010; Zinkevich et al., 2013; Lamley et al., 2015a; Smith et al., 2016; Lakomek et al., 2017; Kurauskas et al., 2017), most of the approaches applied have evolved from methodology first developed for solution-state NMR. Probably the most important advance in solution-state analysis was the development of the model-free approach (Lipari and Szabo, 1982a; Lipari and Szabo, 1982b), and related two-step techniques (Wennerström et al., 1979; Halle and Wennerström, 1981; Brown, 1982). Then, we begin by reviewing some of the existing methodology, to understand advantages and disadvantages to various approaches.
Model-Free Theory
Typical solution-state NMR data sets consist of relaxation rate constants for R1 (1/T1), R2 (1/T2), and nuclear Overhauser effect (NOE,
Here,
Then, model-free analysis makes a few assumptions about the correlation function:
1) The total motion of a given bond is the result of overall tumbling of the molecule in solution and internal motion of the bond within the molecule, and these two motions are statistically independent.
2) Decay of the correlation function due to internal motion is fast compared to all
The decay of the correlation due to internal motion does not need to be mono-exponential (or even multi-exponential, although we will later apply this assumption). Instead of the second assumption, we may assume that the correlation function due to internal motion is mono-exponential, in which case we do not require its decay to be fast (we will visit this case only briefly, as it is less likely to occur in practice). We also assume tumbling is isotropic, although this is not necessarily required. Note that separate methods exist in case overall tumbling and internal motion are coupled (Tugarinov et al., 2001), although we will not consider these here. As a set of equations, this yields
The first equation is the result of statistical independence of internal and overall motion, such that we may write the total correlation function,
We calculate
We see that if
Instead of assuming fast decay of
In the extreme narrowing limit, where decay of the correlation function is fast, we have
The notation
Since
Applying this model does not require that the true correlation function has exactly this form, but rather, the model correlation function simply must have the same values of
A Few Notes on Linearity
We will later note that many of the methods used for analyzing relaxation rate constants result in parameters that are linear functions of the distribution of correlation times,
That is, for every correlation time,
We define the function
One may determine the
Then, the model-free parameters
Note that
Fitting With Model-Free
In Figure 3, we test the performance of model-free fitting under a number of conditions. In Figure 3A, we calculate a number of relaxation rate constants from motion having a single internal correlation time and overall tumbling with
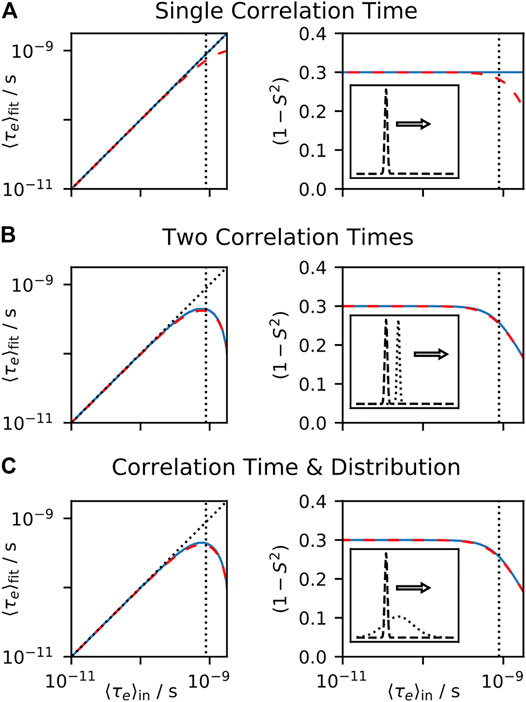
FIGURE 3. Model-free fit parameters as a function of input parameters. For each plot, a data set is calculated, using the experiments found in from Table I of Lipari and Szabo (1982b), and the resulting rate constants are fit using the model-free approach, with the resulting
In Figure 3B, we include two correlation times in the input, each with equal amplitude, where one correlation time is fixed (10 ps), and a second correlation time is swept. We calculate the mean effective correlation time directly on the x-axis (
Determining S2
For model-free analysis,
Then, if we have a precise description of the internal dynamics, we may calculate parameters
In solid-state NMR, we no longer have overall tumbling motion, so the term
This prevents us from separating
One usually equates
Alternative Methods
In the case that all internal motion is fast, such that the correlation function decays quickly, model-free analysis is an ideal approach for extracting dynamics information from relaxation data: the full information content of the relaxation data is captured in the parameters
The first step is to combine the two exponential terms, where we define the log-effective correlation time,
The integral has a complex dependence on
Extended Model-Free
Clore and coworkers found that when measuring relaxation data at higher fields (up to 600 MHz) that not all backbone motion could be well fit using the model-free approach for staphylococcal nuclease and interleukin-1β (Clore et al., 1990). They found that the simplest correlation function that could fit the data was obtained by adding another decaying exponential term, yielding the EMF correlation function.
In this correlation function, the total internal motion is separated into fast and slow components, with order parameters
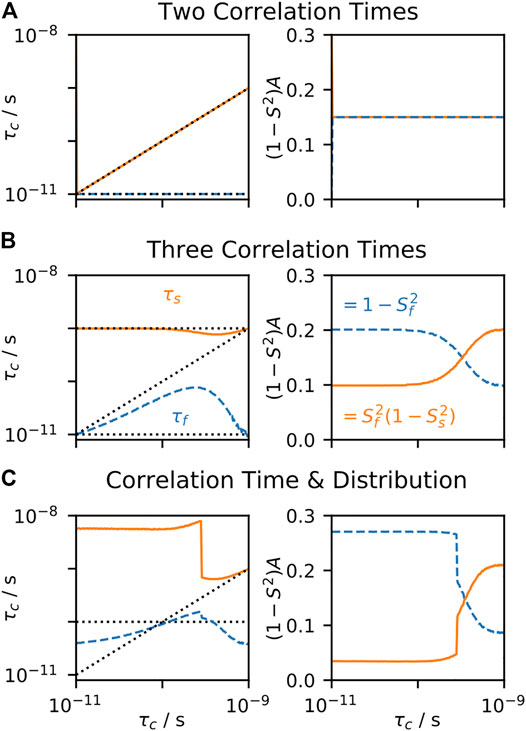
FIGURE 4. EMF parameters as a function of input correlation time (solution-state). For each plot, a data set is calculated, using the set of experiments from Clore et al. (1990), and the resulting rate constants are fitted using the EMF approach. For all plots,
To the best of our knowledge, the behavior of the fit parameters has no well-defined relationship to the distribution of correlation times,
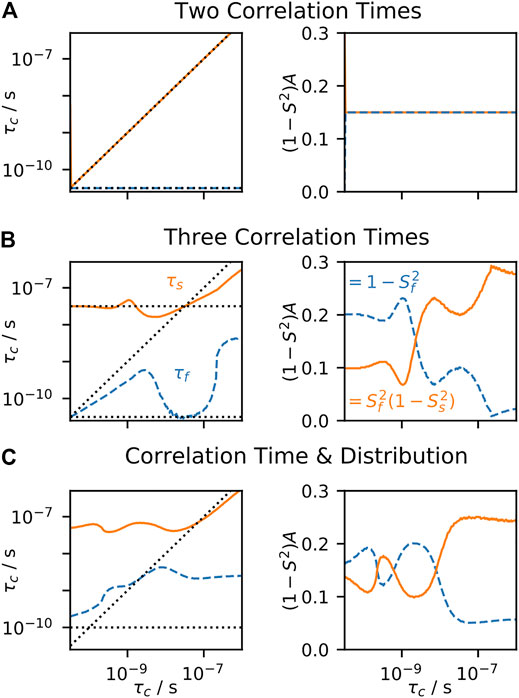
FIGURE 5. EMF parameters as a function of input correlation time (solid-state). For each plot, a data set is calculated, including direct measurement of Sresid. via residual couplings (Eq. 17), 15N T1 at 400, 500, and 850 MHz, and T2 with MAS of 60 kHz. The resulting rate constants are fitted using the EMF approach. For all plots,
Spectral Density Mapping
In contrast to EMF, SDM is achieved by simple linear combination of sets of relaxation data at a single magnetic field (Peng and Wagner, 1992; Ishima et al., 1999). From a set of R1, R2, and NOE relaxation rate constants, one calculates
The above expressions yield very close approximations of the spectral density at specific frequencies: 0,
The parameters resulting from SDM always behave the same way in response to a given correlation time, regardless of other correlation times present, and is the consequence of properties of linearity discussed in A Few Notes on Linearity. This is seen in Figure 6A, where we calculate relaxation rate constants resulting from a single correlation time and analyze with SDM. In Figure 6B, we split motion over two correlation times, and observe how the terms respond to sweeping one of them, and in Figure 6C, we split motion into a distribution and a single, swept correlation time and determine how the terms respond to the swept correlation time. The result is always identical (scaling by 0.5 results from dividing the total amplitude into two parts), a very useful property occurring when data is analyzed strictly by linear combination of data. Unlike EMF analysis, behavior of SDM is independent of the form of the distribution of correlation times.
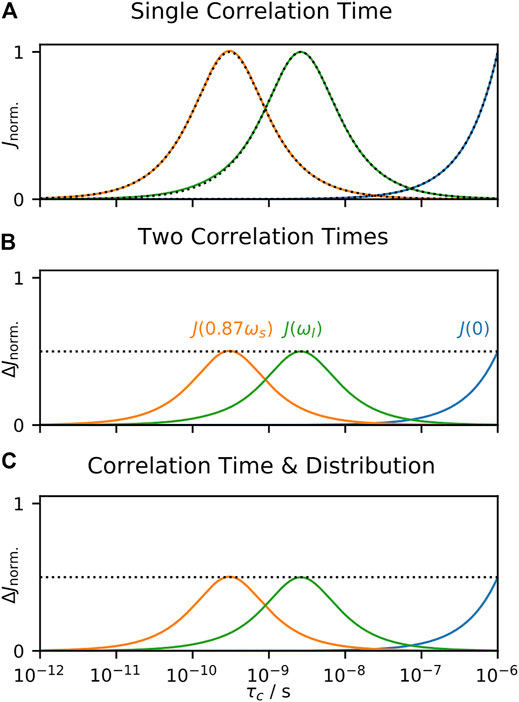
FIGURE 6. Behavior of SDM as a function of correlation time. In each subplot, we calculate 15N T1, T2, and σNH at 600 MHz, and analyze the results using Eq. 21. In (A), the input total correlation function consists of a single decaying exponential term (with amplitude 1), where the terms
Note that this approach describes the total motion, and does not separate out tumbling from internal motion in the case of solution-state NMR, which has an especially strong influence on
LeMaster’s Approach
LeMaster proposed an alternative to SDM analysis of R1, R2, and NOE data from one field, in order to separate overall tumbling from internal motion (LeMaster, 1995). In this case, LeMaster proposed fitting data to the following correlation function:
It is assumed that
In the latter formulation, we find that the spectral density becomes a linear combination of terms, weighted by
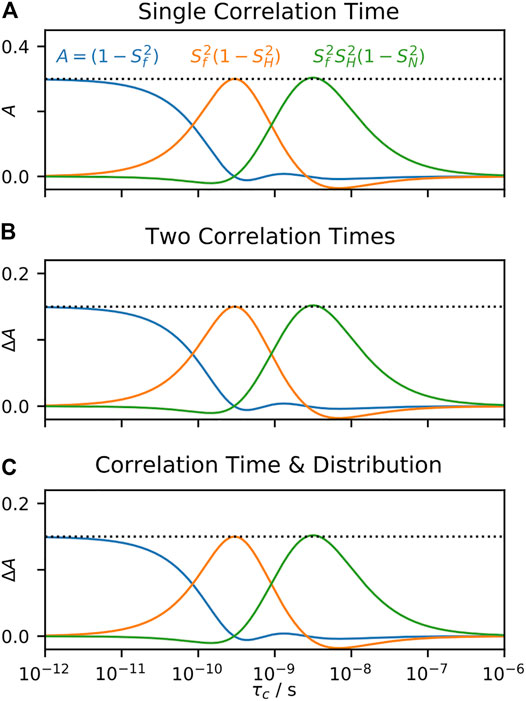
FIGURE 7. Behavior of LeMaster’s approach as a function of correlation time. In each subplot, we calculate 15N T1, T2, and σNH at 600 MHz for motion with
LeMaster’s approach is a linear fit, without priors; as discussed in A Few Notes on Linearity, this means that the fitted parameters may also be obtained by a linear combination of the experimental relaxation rate constants. Therefore, the parameters
Interpretation of Motions by a Projection onto an Array of Correlation Times Approach
Limitations of the approaches above have led Ferrage and coworkers to develop the interpretation of motions by a projection onto an array of correlation times (IMPACT) approach (Khan et al., 2015), which was applied to a protein with intrinsically disordered regions (IDR). A challenge of IDRs is that the lack of structure potentially yields a large number of distinct motions and therefore many correlation times, so that EMF approach is not appropriate for data analysis, but the limited number of parameters obtained with SDM fails to provide a complete description of the dynamics. Then, the IMPACT approach allows analysis of large, multi-field data sets, by taking the total correlation function to be a sum of several fixed correlation times,
Because
Following our procedures for SDM and LeMaster’s approach, we also examine the behavior of the IMPACT approach in Figure 8. When fitting a correlation function having a single correlation time in Figure 8A, we obtain ideal behavior from the IMPACT approach. When the input correlation time matches one of the correlation times in the IMPACT array, the corresponding amplitude is one, and all other amplitudes are zero. When the input correlation time is in between correlation times in the IMPACT array, then only the two nearest correlation times to the input value have non-zero amplitudes, and those two amplitudes sum to one (a minor deviation from this behavior occurs at 10 ns). However, if we input two correlation times in Figure 8B, or one correlation time and one distribution in Figure 8C, with motion split equally between the two correlation times or correlation time and distribution, the fit parameters’ response to the swept correlation time is not an exact reproduction of the behavior in Figure 8A, in contrast to SDM and LeMaster’s approach. While SDM and LeMaster’s approach are both linear combinations of relaxation rate constants, IMPACT is a linear fit for which its behavior depends heavily on restricting the values of the fit parameters (priors), which as discussed in A Few Notes on Linearity, means that the fit parameters are no longer linear to
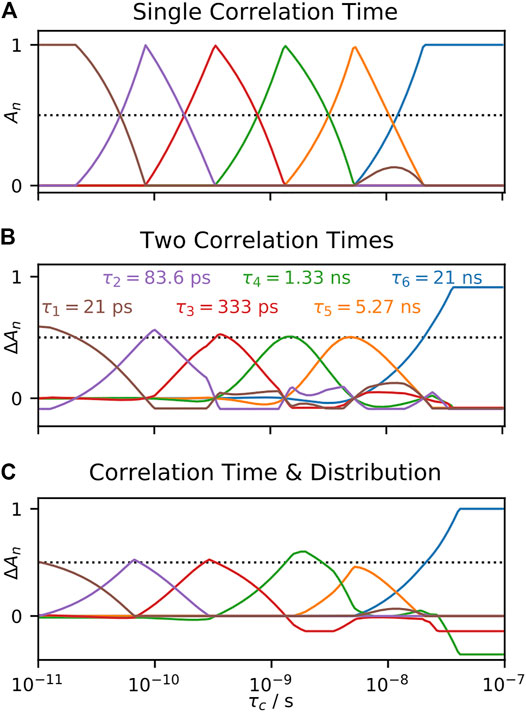
FIGURE 8. Behavior of the IMPACT approach as a function of correlation time. In each plot, we fit calculated relaxation rate constants, and fit the amplitudes in Eq. 25 according to the IMPACT procedure, using the set of experiments from Khan et al. (2015). In (A), the input total correlation function consists of a single decaying exponential term (with amplitude 1), where the amplitudes are plotted as the correlation time is varied. In (B), the total correlation function uses two correlation times (equal amplitudes), with one fixed at 1 ns, and the second swept (x-axis). On the y-axis, we plot contributions to the
IMPACT has not been developed for application to solid-state NMR, but it is worth investigating how such a method could work. In Figure 9A, we use an IMPACT-type approach to fitting R1 at three fields and S2, using an array of three correlation times. We restrict the fitted amplitudes (
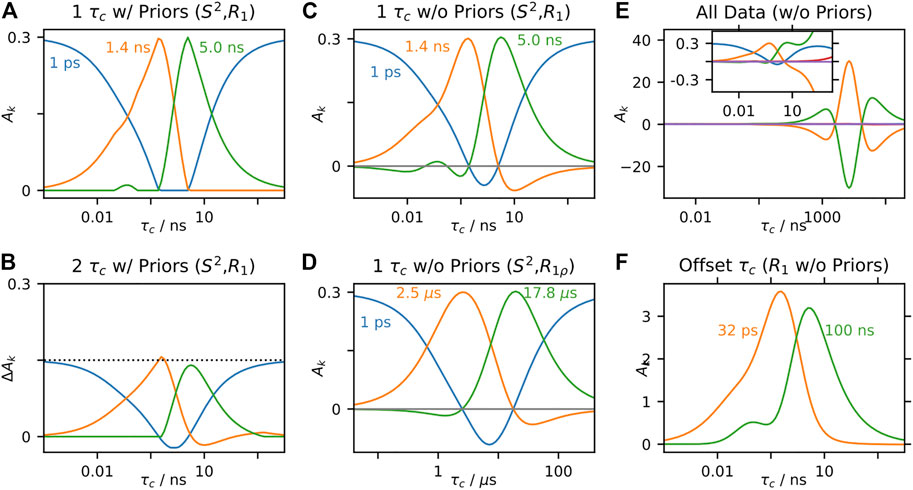
FIGURE 9. IMPACT behavior in solids. In each plot, we test the behavior of the amplitudes,
In Figures 9C,D, we have fairly good performance, excepting that some of the amplitudes become slightly negative. Interestingly, these negative amplitudes may be eliminated by placing two correlation times further away from each other. Then, in Figure 9F, we fit only R1 data, using correlation times of 32 ps and 100 ns. The fitted correlation times no longer correspond to the center of the sensitive range of the
A New Approach for Solid-State Nuclear Magnetic Resonance
In the previous section, we investigated the behavior of a number of approaches to processing relaxation data. Of those approaches, model-free, SDM, and LeMaster’s approach provide parameters which are linear to the distribution of correlation times,
Linear Combination of Data
As we have emphasized for the above examples, one may obtain parameters that have a well-defined (linear) relationship to the distribution of correlation times by taking linear combinations of relaxation rate constants. Thus far, we have limited ourselves to very specific linear combinations: combinations that yield the spectral density, or combinations that are related to specific correlation times. However, why shouldn’t we use any linear combination that is optimized to give an ideal linear relationship to the distribution of correlation times,
Here, we take
Then, as is the case for model-free, SDM, and LeMaster’s approach, any sum of relaxation constants maintains linearity. Following our previous convention (Smith et al., 2018), we denote the sum as
Then,
Optimizing Detectors: The Relaxation-Rate Space Approach
While any linear combination of experimental relaxation rate constants yields a linear relationship between
The question, then, is how to obtain optimized linear combinations satisfying the above requirements. Our initial answer to this question is the result of identifying a similar problem in a completely different field: When one sees the color of an object, its appearance depends on the distribution of wavelengths reflected (or emitted) by the object. The distribution of wavelengths is given by the spectral power distribution,
The functions
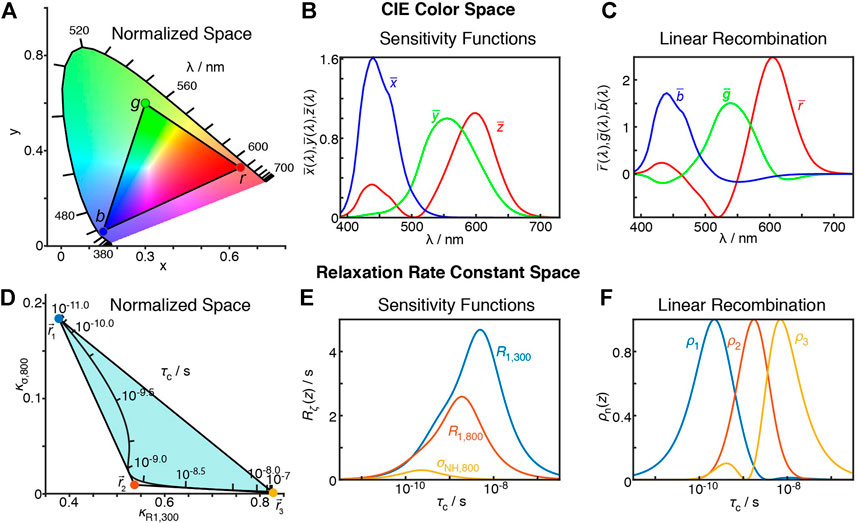
FIGURE 10. Similarity between the CIE XYZ colorspace and the relaxation rate constant space. (A) plots the XYZ colorspace, black lines indicate where single wavelengths fall in the colorspace (z not shown, space is normalized such that x + y + z = 1). Points connected by a triangle indicate the definition of red, green, and blue colors as defined by the sRGB standard (Anderson et al., 1996). (B) plots the sensitivity of the
The XYZ color space can be represented as a 2D space, shown in Figure 10A. Only x and y are shown, and z is selected so that
Realizing that the mathematics of relaxation rate constants was essentially equivalent to color spaces, we created analogous relaxation rate constant spaces, replacing the X, Y, and Z values with normalized rate constants. However, instead of placing points within the relaxation rate space, we surrounded the space in Figure 10D, since we wanted to describe all points in the space with positive parameters. Interestingly, by surrounding the space as closely as possible, without crossing into the space, we obtained a transformation to functions with well-separated and non-negative sensitivities, see Figure 10F. In the example here, we use three points to transform the three experimental sensitivities into detector sensitivities, resulting in three detectors. However, redundancy in the information of larger data sets often results in the space becoming narrow in a given dimension, so that the full space may also be approximately described using fewer points, resulting in fewer detectors than experimental data points, but better signal-to-noise in the resulting parameters. Full details of this approach are described in Smith et al. (2018).
Optimizing Detector Sensitivities: Automated Approach
Investigating the relaxation rate space is a powerful way to grasp the information content of a relaxation data set, however, detector optimization using this method requires manual selection of points in the space. This quickly became excessively tedious for large data sets, as is the case for analysis of relaxometry data (Smith A. A. et al., 2021), so that we have also automated the optimization of linear combination (Smith et al., 2019a).
For automation, one still has the requirements that we capture the information in the experiments (that is, we can fit the data), while minimizing the number of parameters to describe that data, and second, that we obtain detector sensitivities that are narrow and non-negative. The first requirement may be met using singular value decomposition (Golub and Kahan, 1965). Suppose we have a matrix, M, for which each row is a sensitivity of one of our experiments (
The t rows of
Then, T defines the linear recombination of the
In the detector analysis, once we have optimized the detectors, we apply the same linear combination to the experimental relaxation rate constants as were applied to the sensitivities in order to obtain optimized detector responses. Note in practice that this is implemented as a fit, allowing one to prioritize fitting relaxation rate constants with lower measurement error. Furthermore, we place bounds on the fitted detector responses,
Model-Free, or Not?
We see that the original model-free approach, SDM, LeMaster’s approach, and detector analysis all belong to a family of methods that yield parameters with well-defined relationships to the distribution of correlation times, here defined by
Case 1: Extended Model-Free
Using detectors, we may better understand how EMF parameters in solid-state NMR depend on amplitudes of motion for particular windows of correlation times. We re-analyze relaxation data of HET-s (218–289) fibrils (Smith et al., 2016), by first performing a detector analysis on the data, shown in Figure 11A and then iteratively fitting detector responses to correlation times and amplitudes in Figure 11B, resulting in the EMF analysis in Figure 11C.
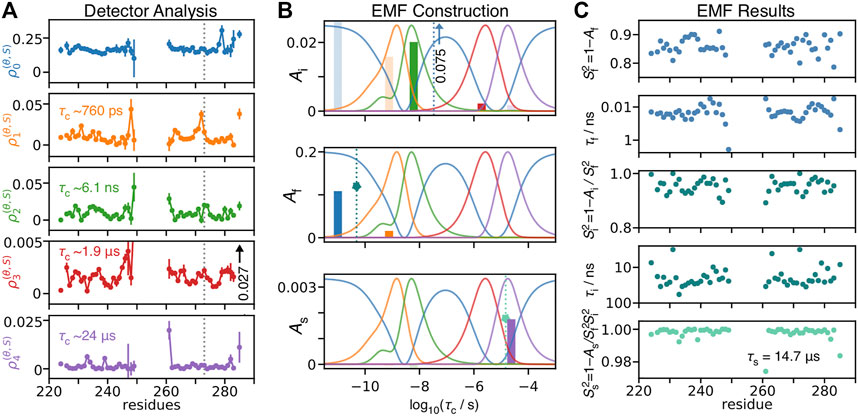
FIGURE 11. Model-free analysis from detectors. (A) shows a detector analysis of HET-s (218–289) fibrils (Smith et al., 2016), with sensitivities shown in (B) (amplitude scale not shown; sensitivities have a maximum of 1). (B) illustrates the procedure to convert 273Ser detector responses into model-free parameters. Bars give the detector responses (y-axis), plotted at the center of the corresponding detector’s sensitivity (x-axis, note that ρ0, blue, does not have a well-defined center). At top, we find the ratio of
Using the following procedure, we are able to reproduce our previous model-free results, illustrated in Figure 11B for residue 273Ser. The procedure is given below as a set of simple equations, where results are a good reproduction of our previous direct fit using the model-free approach.
In the first and second steps, we find a correlation time for which the ratio of sensitivities of ρ2 and ρ3 matches the ratio of the detector responses, and then subsequently find the correct amplitude to reproduce these correlation times. With
In the third and fourth steps, we subtract the contributions from
In the final step, one fixes the slow correlation time to 14.7 μs (based on a fit optimization over the whole data set). In this case, the amplitude of
Then, the major problems with this EMF analysis are intermediate correlation times falling within the NMR blind spot (∼20–600 ns), along with correspondingly inflated amplitudes, as well as similar problems due to fitting fast correlation times to
Case 2: Model-Free Analysis of μs-Motion
Microsecond motion is the result of processes having higher free-energy cost than nanosecond and picosecond dynamics. We suggest dividing these motions into local and collective motions, where the free energy cost of local motions comes from higher amplitude motions (∼10°) that require traversing a large energy barrier. In contrast, collective motions tend to be very low amplitude motion, where the high free-energy cost of the motion is not due to large amplitude dynamics or a significant energy barrier, but rather diffusive dynamics involving large numbers of atoms. Such dynamics are characterized by modes of motion, where a continuum of possible correlation lengths leads to a distribution of correlation times. In contrast, some local microsecond dynamics can be reasonably well approximated as a hopping motion between two orientations, and therefore described with a single correlation time (although effort should be made to determine whether relaxation might be due to multi-site exchange, and understand how this changes the interpretation of data analysis).
Local Dynamics
The availability of R1ρ data, including formulas for its analysis (Trott and Palmer, 2002; Abergel and Palmer, 2003; Miloushev and Palmer, 2005; Kurbanov et al., 2011; Rovo and Linser, 2017) and improving methods for its collection (Kurauskas et al., 2017; Lakomek et al., 2017; Keeler et al., 2018; Krushelnitsky et al., 2018) has recently resulted in considerable improvement in the ability to characterize local micro- to millisecond motions (Rovó, 2020). We consider two categories of R1ρ experiments: the first is Bloch-McConnell relaxation dispersion experiments (BMRD), for which R1ρ relaxation is the result of motion modulating the isotropic chemical shift, and the NEar Rotary-resonance Relaxation Dispersion (NERRD, (Kurauskas et al., 2017)), for which orientational changes in anisotropic tensors leads to R1ρ relaxation. For two-site exchange, BMRD R1ρ relaxation rate constants depend on exchange rate (
The total
Then, the question is, how may we most efficiently extract the exchange rate (
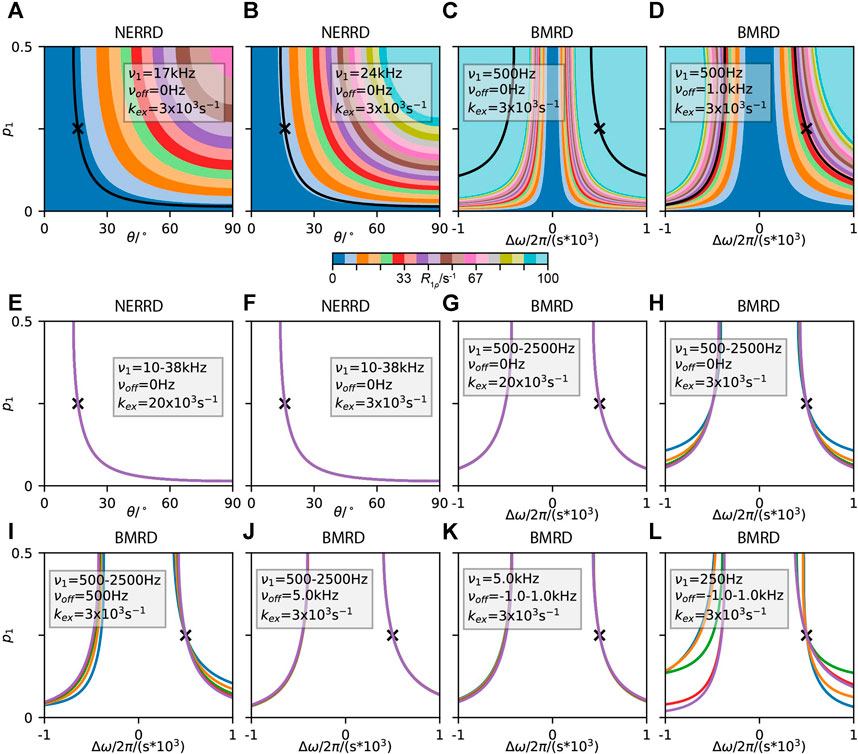
FIGURE 12. Separating population from hop angle and change in chemical shift in NERRD and BMRD experiments. Relevant parameters are shown as insets (
In contrast,
Separability occurs only when
In case we are in the fast exchange limit for BMRD experiments, we are left only with the terms
A final consideration when analyzing BMRD and NERRD data is whether a two-site exchange model is reasonable. In a true two-site exchange, all moving residues should have identical exchange rates and populations, but differing
Collective Dynamics
NERDD relaxation also appears throughout the whole protein in the absence of BMRD relaxation, depending on sample conditions, and is attributed to low amplitude rocking of the whole protein. This is observed very weakly in GB1 crystals (Krushelnitsky et al., 2018), and strongly in GB1 complexed with IgG (Lamley et al., 2015b), HET-s (218–289) (Smith et al., 2016), ubiquitin crystals with amplitude depending heavily on crystal form (Ma et al., 2015; Kurauskas et al., 2017; Lakomek et al., 2017), and SH3 (Krushelnitsky et al., 2018). The apparent global nature of this motion led all of these studies, with the exception of Lakomek et al., to fit R1ρ relaxation using a slow motion with a single correlation time for all residues. In our HET-s analysis, we proposed fitting R1ρ data using a global, slow correlation time, where the corresponding order parameter could vary, and additionally an offset term that would account for faster motion that could not be fully parameterized from R1ρ data alone. Kurauskas et al. also followed this procedure, whereas Krushelnitsky and coworkers included explicit fitting of an additional fast motion with a distribution of correlation times. By including an offset term, and using a single correlation time globally, we again have a linear fit.
Then, for each residue,
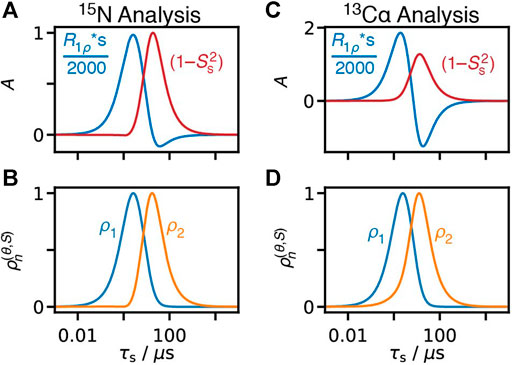
FIGURE 13. Behavior of fitting R1ρ data to an offset and a fixed correlation time. (A) shows the offset term,
In Figure 13A, we calculate R1ρ relaxation rate constants for 15N relaxation, and fit to Eq. 36.
The sensitivity of the offset term in Figure 13A to motion near 2.5 μs as opposed to faster motions may be surprising, although perhaps it should not be. NERRD experiments are most sensitive in the μs-range of correlation times, and rate constants under different experimental conditions have nearly converged to the same value at 1.9 μs (all rate constants within 5% of each other)– only slightly faster than the 2.5 μs where we find the maximum. Then, we would expect the offset term to be sensitive both near where R1ρ is most sensitive, but also near where it converges, which is roughly what we find.
It is then important to note that fitting
Outlook: Combining Methods
We have seen that relaxation data in NMR may be processed by a variety of different methods, however, only some of these methods can really be thought of as “model-free,” such that we can establish a well-defined (linear) behavior for each parameter as a function of correlation time, independent of the actual model of the correlation function. These methods are the original model-free analysis, under the assumption that
So, are detectors the last word in NMR dynamics analysis? We certainly hope not. Each detector response provides a “window” into the total reorientational motion of some NMR tensor, with the window width and center defined by
Molecular dynamics simulation is particularly powerful as a complimentary method to NMR. One obtains positions of all atoms as a function of time, allowing first, the direct calculation of the NMR-relevant correlation functions, and second, in principle allowing one to connect those correlation functions to specific motion in the molecule.
This is the discrete form of Eq. 14, as would be applied to an MD trajectory. To obtain the nth time point in the correlation function,
When analyzing MD with detectors, one has two options: find the optimal set of detectors for describing correlation time distributions found with MD (that is, as many as possible with good signal-to-noise, and as narrow/non-overlapping as possible), or optimize the detectors to match some or all of the NMR-derived detectors. The latter approach is shown in Figure 14, where sensitivities of seven NMR experiments in Figure 14A are optimized to yield five detectors in Figure 14C, and the linear combination used to yield
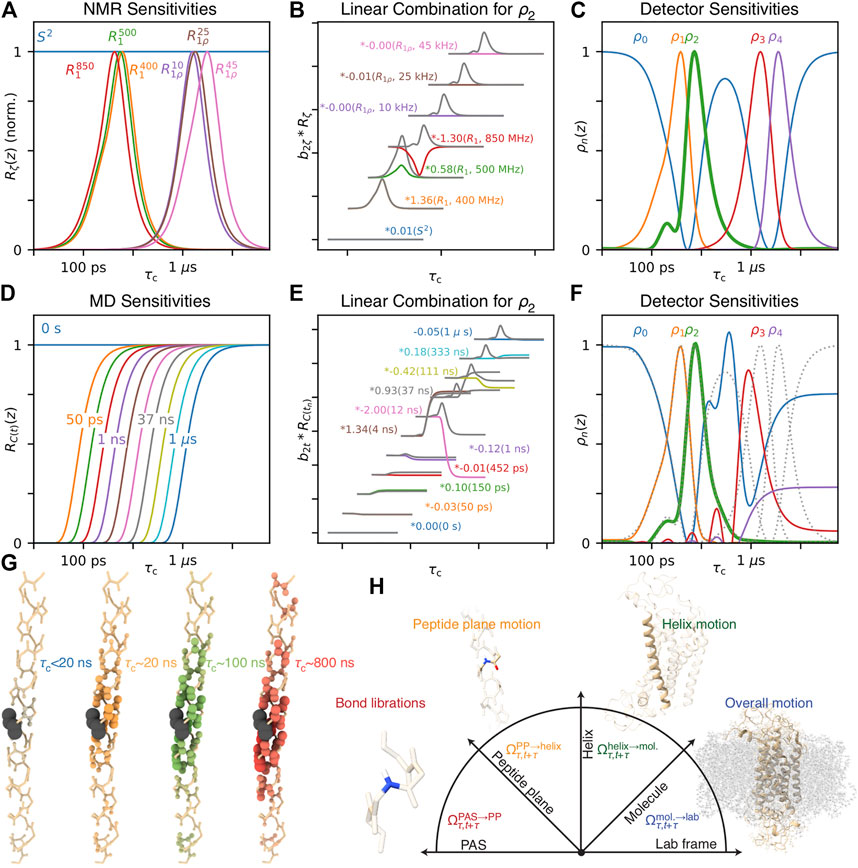
FIGURE 14. Combining NMR and MD. (A) plots normalized NMR sensitivities for a selection of experiments (S2, 15N R1 at 400, 500, 850 MHz, 15N R1ρ at 850 MHz, 60 kHz MAS, ν1 at 10, 25, and 45 kHz). (B) shows a linear combination of the normalized sensitivities (x, y positions shifted to reduce plot overlap), which yields the sensitivity of ρ2, shown in (C) (green, bold). In color are the weighted contributions from each rate constant, and grey shows the cumulative sum (summing all sensitivities at and below the grey line). (C) shows the five sensitivities optimized from NMR data. (D) plots sensitivities of time points from MD-derived correlation functions (0 s, 10 points log-spaced from 50 ps to 1 μs). (E) shows a linear combination of those sensitivities, optimized to match the sensitivity of ρ2 (x, y positions shifted to reduce plot overlap). (F) shows detectors optimized to match the NMR-derived detectors in (C). (G) shows spatial correlation of motion in a helix as a function of correlation time (windows for <20, ∼20, ∼100, ∼800 ns). Color intensity and bond radii indicate the correlation coefficient between that residue’s H–N motion and the motion of the black residue. (H) illustrates frames used to separate transformation from the PAS to the lab frame into four steps: a peptide plane frame, a helix frame, and a molecule frame (illustration inspired by Brown (1996), molecule plots created with ChimeraX (Pettersen et al., 2021)).
The detector analysis then provides a very reliable means of comparing NMR results to MD simulation. The ability to easily compare results across multiple methods is one of the primary advantages of detector analysis. We should note that carefully executed fitting of MD-derived correlation functions, followed by calculation of relaxation rate constants should yield similarly reliable rate constants, if the trajectory is sufficiently long (Mollica et al., 2012). However, the rate constants themselves are sensitive to a broader range of correlation times than detectors, so that the comparison has lower timescale resolution than detectors.
With MD and NMR data sets, one may then use NMR data via detectors (or relaxation rate constants) as a means of validating the MD, and potentially refining it; methods include selecting sections of trajectories that best reproduce experiment (Salvi et al., 2016), selecting the best force fields for a system (Antila et al., 2021), or validating the refinement of a force-field itself (Hoffmann et al., 2018a; Hoffmann et al., 2018b). One may also use NMR data (specifically order parameters) as a means of directing the simulation, so that the simulation returns parameters matching the experiment (Hansen et al., 2014). One should note that a major challenge of combining NMR and MD data is that, while NMR is highly sensitive to microsecond motions, for example, via R1ρ measurements, it is challenging to obtain accurate dynamics on the microsecond timescale from MD simulations. Although MD simulations now regularly extend for multiple microseconds, or longer via enhanced sampling (Bernardi et al., 2015), one still lacks sufficient statistics to obtain reliable dynamics behavior. Consider, if we investigate a 1 μs motion, using a 10 μs trajectory, we should observe 10 events, but the variance in number of events is also 10 (assuming Poisson statistics), so that large errors easily occur. Additionally, correct replication of slower motions requires all the faster motion leading up to the slow motion to occur at approximately the correct rates, so that the slower motions are more susceptible to influences like force field inaccuracies, starting structure of the system, etc. This remains a significant challenge for combining experiment in simulation, requiring creative solutions to take advantage of simulation where reproduction of experimental observables is poor.
With experimental validation or refinement of an MD simulation, one may analyze the simulation further, with improved confidence of the accuracy of the simulation. However, we want to use the simulation specifically to improve our interpretation of the experimental parameters. For example, we recently showed that it was possible to calculate the spatial correlation of motions within a given detector window between different residues in HET-s (218–289) fibrils (Smith et al., 2019b), using a modified iRED analysis (Prompers and Brüschweiler, 2001; Prompers and Brüschweiler, 2002). The result is that we could see that detector windows corresponding to longer correlation times tended to result in correlation over longer distances, providing at least some explanation for the presence of slow, low amplitude motion in fibrils. A similar correlation analysis is shown in Figure 14G, in this case for residues in an α-helix, where similarly, detector windows corresponding to longer correlation times yield longer correlation lengths. We suspect this behavior to be nearly universal: even in well-defined structures, there is always some residual flexibility. Then, both short- and long-range modes of motion should be thermally populated (in terms of modes, these are more accurately described as having short and long wavelengths). However, the longer-range modes usually have longer correlation times, resulting in the trends in Figure 14G. Note that this implies that there should almost always be distributions of correlation times due to varying correlation length, further complicating the interpretation of the two to three correlation times provided by the EMF approach.
For fairly rigid regions of a molecule, we expect detector-specific correlation analysis to help explain dynamic trends. However, what should we do for regions that are more mobile, with multiple types of motion contributing? Having all of the atom positions in an MD simulation should provide the detail that would allow us to separate different motions. Then, we could define the total motion of a bond as resulting from the product of these motions. For example, for an H–N dipole coupling in an α-helix, the total rotation of the dipole is the result of the reorientation of the principal axis system (PAS) of the dipole within the peptide plane (PP), the peptide plane reorienting within the helix, the helix reorienting with the molecule, and the molecule reorienting within the lab frame.
This concept is illustrated in Figure 14H. In the case that it is possible to derive a correlation function from each rotation, one then may effectively achieve an in silico model-free type separation of the correlation functions motion. A similar approach for the specific separation of librations, φ/ψ reorientation, and peptide plane tumbling in intrinsically disorded proteins has been demonstrated by Salvi et al. (2017), however we find that it is possible to fully generalize this concept for separation of arbitrary definitions of independent motions (manuscript under revision, (Smith et al., 2021b)). Then, separated motions may also be analyzed with detectors, to determine how both experimental and simulated detector responses depend on both timescale and position in the molecule. Separation of motions could also be coupled with mode analyses such as iRED (Prompers and Brüschweiler, 2001, 2002) or principal component analysis (Amadei et al., 1993; Altis et al., 2007), providing a method to better characterize distributions of correlation times arising from different motions and complex mode-like dynamics. In each proposed case, comparison of the different MD analyses is possible via the detector analysis. Our eventual goal is that one may extract enough detail from the MD to build explicit models of motion for direct application to the NMR experimental results, so that the final characterizations are no longer model-free at all, but rather yield highly detailed models based on the combined information from experiment and simulation.
Conclusion
We show that the original model-free approach, SDM, LeMaster’s approach, and detectors all belong to a class of methods where fit parameters are resulting from a linear combination of experimental relaxation rate constants (potentially requiring an additional arithmetic step to yield the final parameters). IMPACT is a close approximation to this behavior, whereas EMF parameters exhibit significantly different behavior. Analysis methods belonging to this class are particularly useful because it is straightforward to estimate the resulting parameters if the distribution of correlation times,
The detector analysis is the most general of these approaches, being applicable to any collection of NMR relaxation experiments probing reorientational motion, and can be generalized for other methods such as MD simulation, requiring very little modification of the analysis. Then, the resulting detector responses from NMR and MD are easily compared. With experimental validation of MD, one may then use the wealth of detail in MD simulation to better understand how experimentally derived parameters are related to specific motion, via correlation of motion, separation of motion, and other existing and yet-to-be developed techniques. This has the potential to lead to improved models of motion for NMR analysis, which in turn can help obtain a more fundamental understand of dynamics in biomolecular systems.
Author Contributions
AS has prepared the manuscript text and KZ has prepared the figures.
Funding
The authors acknowledge funding by the Deutsche Forschungsgemeinschaft (DFG) grant SM 576/1-1 and by European Social Funds (ESF) and the Free State of Saxony (Junior Research Group UniDyn, Project No. SAB 100382164). The authors also acknowledge support from the German Research Foundation (DFG) and Universität Leipzig within the program of Open Access Publishing.
Conflict of Interest
The authors declare that the research was conducted in the absence of any commercial or financial relationships that could be construed as a potential conflict of interest.
Publisher’s Note
All claims expressed in this article are solely those of the authors and do not necessarily represent those of their affiliated organizations, or those of the publisher, the editors and the reviewers. Any product that may be evaluated in this article, or claim that may be made by its manufacturer, is not guaranteed or endorsed by the publisher.
Supplementary Material
The Supplementary Material for this article can be found online at: https://www.frontiersin.org/articles/10.3389/fmolb.2021.727553/full#supplementary-material
References
Abergel, D., and Palmer, A. G. (2003). On the Use of the Stochastic Liouville Equation in Nuclear Magnetic Resonance: Application to R1ρ Relaxation in the Presence of Exchange. Concepts Magn. Reson. 19A, 134–148. doi:10.1002/cmr.a.10091
Altis, A., Nguyen, P. H., Hegger, R., and Stock, G. (2007). Dihedral Angle Principal Component Analysis of Molecular Dynamics Simulations. J. Chem. Phys. 126, 244111. doi:10.1063/1.2746330
Amadei, A., Linssen, A. B. M., and Berendsen, H. J. C. (1993). Essential Dynamics of Proteins. Proteins 17, 412–425. doi:10.1002/prot.340170408
Anderson, M., Motta, R., Chandrasekar, S., and Stokes, M. (1996). “Proposal for a Standard Default Color Space for the Internet—sRGB,” in Color and Imaging Conference (Society for Imaging Science and Technology), 238–245.
Antila, H. S., Ferreira, T. M., Ollila, O. H. S., and Miettinen, M. S. (2021). Using Open Data to Rapidly Benchmark Biomolecular Simulations: Phospholipid Conformational Dynamics. J. Chem. Inf. Model. 61, 938–949. doi:10.1021/acs.jcim.0c01299
Beckmann, P. A. (1988). Spectral Densities and Nuclear Spin Relaxation in Solids. Phys. Rep. 171, 85–128. doi:10.1016/0370-1573(88)90073-7
Bernardi, R. C., Melo, M. C. R., and Schulten, K. (2015). Enhanced Sampling Techniques in Molecular Dynamics Simulations of Biological Systems. Biochim. Biophys. Acta (Bba) - Gen. Subjects 1850, 872–877. doi:10.1016/j.bbagen.2014.10.019
Brown, M. (1982). Theory of Spin-Lattice Relaxation in Lipid Bilayers and Biological Membranes. 2H and 14N Quadrupolar Relaxation. J. Chem. Phys. 77 (3), 1576–1599. doi:10.1063/1.443940
Brown, M. F. (1996). “Biological Membranes,” in Biological Membranes: A Molecular Perspective From Computation And Experiment. Editors K. M. Merz, and B. Roux (Birkhäuser Basel). doi:10.1007/978-1-4684-8580-6
Chevelkov, V., Fink, U., and Reif, B. (2009a). Accurate Determination of Order Parameters from 1H,15N Dipolar Couplings in MAS Solid-State NMR Experiments. J. Am. Chem. Soc. 131, 14018–14022. doi:10.1021/ja902649u
Chevelkov, V., Fink, U., and Reif, B. (2009b). Quantitative Analysis of Backbone Motion in Proteins Using MAS Solid-State NMR Spectroscopy. J. Biomol. NMR 45, 197–206. doi:10.1007/S10858-009-9348-5
Clore, G. M., Szabo, A., Bax, A., Kay, L. E., Driscoll, P. C., and Gronenborn, A. M. (1990). Deviations from the Simple Two-Parameter Model-free Approach to the Interpretation of Nitrogen-15 Nuclear Magnetic Relaxation of Proteins. J. Am. Chem. Soc. 112, 4989–4991. doi:10.1021/ja00168a070
d'Auvergne, E. J., and Gooley, P. R. (2003). The Use of Model Selection in the Model-free Analysis of Protein Dynamics. J. Biomol. NMR 25, 25–39. doi:10.1023/A:1021902006114
Dantzig, G. B. (1982). Reminiscences about the Origins of Linear Programming. Operations Res. Lett. 1, 43–48. doi:10.1016/0167-6377(82)90043-8
Gill, M. L., Byrd, R. A., and Palmer, A. G. (2016). Dynamics of GCN4 Facilitate DNA Interaction: a Model-free Analysis of an Intrinsically Disordered Region. Phys. Chem. Chem. Phys. 18, 5839–5849. doi:10.1039/c5cp06197k
Golub, G., and Kahan, W. (1965). Calculating the Singular Values and Pseudo-inverse of a Matrix. J. Soc. Ind. Appl. Maths. Ser. B Numer. Anal. 2, 205–224. doi:10.1137/0702016
Gullion, T., and Schaefer, J. (1989). Rotational-Echo Double-Resonance NMR. J. Magn. Reson. (1969) 81, 196–200. doi:10.1016/0022-2364(89)90280-1
Halle, B., and Wennerström, H. (1981). Interpretation of Magnetic Resonance Data from Water Nuclei in Heterogeneous Systems. J. Chem. Phys. 75, 1928–1943. doi:10.1063/1.442218
Haller, J. D., and Schanda, P. (2013). Amplitudes and Time Scales of Picosecond-To-Microsecond Motion in Proteins Studied by Solid-State NMR: a Critical Evaluation of Experimental Approaches and Application to Crystalline Ubiquitin. J. Biomol. NMR 57, 263–280. doi:10.1007/s10858-013-9787-x
Hansen, N., Heller, F., Schmid, N., and van Gunsteren, W. F. (2014). Time-averaged Order Parameter Restraints in Molecular Dynamics Simulations. J. Biomol. NMR 60, 169–187. doi:10.1007/s10858-014-9866-7
Hoffmann, F., Mulder, F. A. A., and Schäfer, L. V. (2018a). Accurate Methyl Group Dynamics in Protein Simulations with AMBER Force Fields. J. Phys. Chem. B. 122, 5038–5048. doi:10.1021/acs.jpcb.8b02769
Hoffmann, F., Xue, M., Schäfer, L. V., and Mulder, F. A. A. (2018b). Narrowing the gap between Experimental and Computational Determination of Methyl Group Dynamics in Proteins. Phys. Chem. Chem. Phys. 20, 24577–24590. doi:10.1039/c8cp03915a
Hsu, A., O'Brien, P. A., Bhattacharya, S., Rance, M., and Palmer, A. G. (2018). Enhanced Spectral Density Mapping through Combined Multiple-Field Deuterium 13CH2D Methyl Spin Relaxation NMR Spectroscopy. Methods 138-139, 76–84. doi:10.1016/j.ymeth.2017.12.020
Ishima, R., Louis, J. M., and Torchia, D. A. (1999). Transverse 13C Relaxation of CHD2 Methyl Isotopmers to Detect Slow Conformational Changes of Protein Side Chains. J. Am. Chem. Soc. 121, 11589–11590. doi:10.1021/ja992836b
Judd, D. B. (1951). Report of U.S. Secretariat Committee on Colorimetry and Artificial Daylight, Proceedings of the Twelfth Session of the CIE. Stockholm: Paris: Bureau Central de la CIE, 11.
Kantorovich, L. (1960). On the Calculation of Production Inputs. Probl. Econ. 3, 3–10. doi:10.2753/PET1061-199103013
Kay, L. E., Torchia, D. A., and Bax, A. (1989). Backbone Dynamics of Proteins as Studied by Nitrogen-15 Inverse Detected Heteronuclear NMR Spectroscopy: Application to Staphylococcal Nuclease. Biochemistry 28, 8972–8979. doi:10.1021/bi00449a003
Keeler, E. G., Fritzsching, K. J., and McDermott, A. E. (2018). Refocusing CSA during Magic Angle Spinning Rotating-Frame Relaxation Experiments. J. Magn. Reson. 296, 130–137. doi:10.1016/j.jmr.2018.09.004
Khan, S. N., Charlier, C., Augustyniak, R., Salvi, N., Déjean, V., Bodenhausen, G., et al. (2015). Distribution of Pico- and Nanosecond Motions in Disordered Proteins from Nuclear Spin Relaxation. Biophysical J. 109, 988–999. doi:10.1016/j.bpj.2015.06.069
Kinosita, K., Kawato, S., and Ikegami, A. (1977). A Theory of Fluorescence Polarization Decay in Membranes. Biophysical J. 20, 289–305. doi:10.1016/S0006-3495(77)85550-1
Korzhnev, D. M., Neudecker, P., Mittermaier, A., Orekhov, V. Y., and Kay, L. E. (2005). Multiple-Site Exchange in Proteins Studied with a Suite of Six NMR Relaxation Dispersion Experiments: An Application to the Folding of a Fyn SH3 Domain Mutant. J. Am. Chem. Soc. 127, 15602–15611. doi:10.1021/ja054550e
Koss, H., Rance, M., and Palmer, A. G. (2018). General Expressions for Carr-Purcell-Meiboom-Gill Relaxation Dispersion for N-Site Chemical Exchange. Biochemistry 57, 4753–4763. doi:10.1021/acs.biochem.8b00370
Krushelnitsky, A., Gauto, D., Rodriguez Camargo, D. C., Schanda, P., and Saalwächter, K. (2018). Microsecond Motions Probed by Near-Rotary-Resonance R1ρ 15N MAS NMR Experiments: the Model Case of Protein Overall-Rocking in Crystals. J. Biomol. NMR 71, 53–67. doi:10.1007/s10858-018-0191-4
Kurauskas, V., Izmailov, S. A., Rogacheva, O. N., Hessel, A., Ayala, I., Woodhouse, J., et al. (2017). Slow Conformational Exchange and Overall Rocking Motion in Ubiquitin Protein Crystals. Nat. Commun. 8, 145. doi:10.1038/s41467-017-00165-8
Kurbanov, R., Zinkevich, T., and Krushelnitsky, A. (2011). The Nuclear Magnetic Resonance Relaxation Data Analysis in Solids: General R1/R1ρ Equations and the Model-free Approach. J. Chem. Phys. 135 (1–9), 184104. doi:10.1063/1.3658383
Lakomek, N.-A., Penzel, S., Lends, A., Cadalbert, R., Ernst, M., and Meier, B. H. (2017). Microsecond Dynamics in Ubiquitin Probed by Solid-State 15N NMR Spectroscopy R1ρ Relaxation Experiments under Fast Mas (60-110 Khz). Chem. Eur. J. 23, 9425–9433. doi:10.1002/chem.201701738
Lamley, J. M., Lougher, M. J., Sass, H. J., Rogowski, M., Grzesiek, S., and Lewandowski, J. R. (2015a). Unraveling the Complexity of Protein Backbone Dynamics with Combined 13C and 15N Solid-State NMR Relaxation Measurements. Phys. Chem. Chem. Phys. 17, 21997–22008. doi:10.1039/c5cp03484a
Lamley, J. M., Öster, C., Stevens, R. A., and Lewandowski, J. R. (2015b). Intermolecular Interactions and Protein Dynamics by Solid‐State NMR Spectroscopy. Angew. Chem. Int. Ed. 54, 15374–15378. doi:10.1002/anie.201509168
LeMaster, D. (1995). Larmor Frequency Selective Model Free Analysis of Protein NMR Relaxation. J. Biomol. NMR 6, 366–374. doi:10.1007/BF00197636
Lipari, G., and Szabo, A. (1982a). Model-free Approach to the Interpretation of Nuclear Magnetic Resonance Relaxation in Macromolecules. 1. Theory and Range of Validity. J. Am. Chem. Soc. 104, 4546–4559. doi:10.1021/ja00381a009
Lipari, G., and Szabo, A. (1982b). Model-free Approach to the Interpretation of Nuclear Magnetic Resonance Relaxation in Macromolecules. 2. Analysis of Experimental Results. J. Am. Chem. Soc. 104, 4559–4570. doi:10.1021/ja00381a010
Ma, P., Haller, J. D., Zajakala, J., Macek, P., Sivertsen, A. C., Willbold, D., et al. (2014). Probing Transient Conformational States of Proteins by Solid-State R1ρ Relaxation-Dispersion NMR Spectroscopy. Angew. Chem. Int. Ed. 53, 4312–4317. doi:10.1002/anie.201311275
Ma, P., Xue, Y., Coquelle, N., Haller, J. D., Yuwen, T., Ayala, I., et al. (2015). Observing the Overall Rocking Motion of a Protein in a Crystal. Nat. Commun. 6, 8361. doi:10.1038/ncomms9361
Mandel, A. M., Akke, M., and Palmer, A. G. (1995). Backbone Dynamics of Escherichia coli Ribonuclease HI: Correlations with Structure and Function in an Active Enzyme. J. Mol. Biol. 246, 144–163. doi:10.1006/jmbi.1994.0073
Marion, D., Gauto, D. F., Ayala, I., Giandoreggio-Barranco, K., and Schanda, P. (2019). Microsecond Protein Dynamics from Combined Bloch-McConnell and Near-Rotary-Resonance R1p Relaxation-Dispersion MAS NMR. ChemPhysChem 20, 276–284. doi:10.1002/cphc.201800935
Mendelman, N., and Meirovitch, E. (2021). Structural Dynamics from NMR Relaxation by SRLS Analysis: Local Geometry, Potential Energy Landscapes, and Spectral Densities. J. Phys. Chem. B. 125, 6130–6143. doi:10.1021/acs.jpcb.1c02502
Miloushev, V. Z., and Palmer, A. G. (2005). R1ρ Relaxation for Two-Site Chemical Exchange: General Approximations and Some Exact Solutions. J. Magn. Reson. 177, 221–227. doi:10.1016/j.jmr.2005.07.023
Mollica, L., Baias, M., Lewandowski, J. R., Wylie, B. J., Sperling, L. J., Rienstra, C. M., et al. (2012). Atomic-Resolution Structural Dynamics in Crystalline Proteins from NMR and Molecular Simulation. J. Phys. Chem. Lett. 3, 3657–3662. doi:10.1021/Jz3016233
Munowitz, M. G., Griffin, R. G., Bodenhausen, G., and Huang, T. H. (1981). Two-dimensional Rotational Spin-echo Nuclear Magnetic Resonance in Solids: Correlation of Chemical Shift and Dipolar Interactions. J. Am. Chem. Soc. 103, 2529–2533. doi:10.1021/ja00400a007
Neudecker, P., Korzhnev, D. M., and Kay, L. E. (2006). Assessment of the Effects of Increased Relaxation Dispersion Data on the Extraction of 3-site Exchange Parameters Characterizing the Unfolding of an SH3 Domain. J. Biomol. NMR 34, 129–135. doi:10.1007/s10858-006-0001-2
Öster, C., Kosol, S., and Lewandowski, J. R. (2019). Quantifying Microsecond Exchange in Large Protein Complexes with Accelerated Relaxation Dispersion Experiments in the Solid State. Sci. Rep. 9, 11082. doi:10.1038/s41598-019-47507-8
Palmer, A. G. (2004). NMR Characterization of the Dynamics of Biomacromolecules. Chem. Rev. 104, 3623–3640. doi:10.1021/cr030413t
Peng, J. W., and Wagner, G. (1992). Mapping of Spectral Density Functions Using Heteronuclear NMR Relaxation Measurements. J. Magn. Reson. (1969) 98, 308–332. doi:10.1016/0022-2364(92)90135-T
Penzel, S., Smith, A. A., Agarwal, V., Hunkeler, A., Org, M.-L., Samoson, A., et al. (2015). Protein Resonance Assignment at MAS Frequencies Approaching 100 kHz: a Quantitative Comparison of J-Coupling and Dipolar-Coupling-Based Transfer Methods. J. Biomol. NMR 63, 165–186. doi:10.1007/s10858-015-9975-y
Pettersen, E. F., Goddard, T. D., Huang, C. C., Meng, E. C., Couch, G. S., Croll, T. I., et al. (2021). UCSF ChimeraX : Structure Visualization for Researchers, Educators, and Developers. Protein Sci. 30, 70–82. doi:10.1002/pro.3943
Polimeno, A., and Freed, J. H. (1992). Advances In Chemical Physics. John Wiley & Sons, 89–206. doi:10.1002/9780470141410.ch3A Many-Body Stochastic Approach to Rotational Motions in Liquids.
Prompers, J. J., and Brüschweiler, R. (2002). General Framework for Studying the Dynamics of Folded and Nonfolded Proteins by NMR Relaxation Spectroscopy and MD Simulation. J. Am. Chem. Soc. 124, 4522–4534. doi:10.1021/ja012750u
Prompers, J. J., and Brüschweiler, R. (2001). Reorientational Eigenmode Dynamics: A Combined MD/NMR Relaxation Analysis Method for Flexible Parts in Globular Proteins. J. Am. Chem. Soc. 123, 7305–7313. doi:10.1021/ja0107226
Rovó, P., and Linser, R. (2017). Microsecond Time Scale Proton Rotating-Frame Relaxation under Magic Angle Spinning. J. Phys. Chem. B. 121, 6117–6130. doi:10.1021/acs.jpcb.7b03333
Rovó, P. (2020). Recent Advances in Solid-State Relaxation Dispersion Techniques. Solid State. Nucl. Magn. Reson. 108, 101665. doi:10.1016/j.ssnmr.2020.101665
Salvi, N., Abyzov, A., and Blackledge, M. (2017). Analytical Description of NMR Relaxation Highlights Correlated Dynamics in Intrinsically Disordered Proteins. Angew. Chem. Int. Ed. 56, 14020–14024. doi:10.1002/anie.201706740
Salvi, N., Abyzov, A., and Blackledge, M. (2016). Multi-Timescale Dynamics in Intrinsically Disordered Proteins from NMR Relaxation and Molecular Simulation. J. Phys. Chem. Lett. 7, 2483–2489. doi:10.1021/acs.jpclett.6b00885
Schanda, P., and Ernst, M. (2016). Studying Dynamics by Magic-Angle Spinning Solid-State NMR Spectroscopy: Principles and Applications to Biomolecules. Prog. Nucl. Magn. Reson. Spectrosc. 96, 1–46. doi:10.1016/j.pnmrs.2016.02.001
Schanda, P., Meier, B. H., and Ernst, M. (2011). Accurate Measurement of One-Bond H-X Heteronuclear Dipolar Couplings in MAS Solid-State NMR. J. Magn. Reson. 210, 246–259. doi:10.1016/j.jmr.2011.03.015
Schanda, P., Meier, B. H., and Ernst, M. (2010). Quantitative Analysis of Protein Backbone Dynamics in Microcrystalline Ubiquitin by Solid-State NMR Spectroscopy. J. Am. Chem. Soc. 132, 15957–15967. doi:10.1021/Ja100726a
Schanda, P. (2019). Relaxing with Liquids and Solids - A Perspective on Biomolecular Dynamics. J. Magn. Reson. 306, 180–186. doi:10.1016/j.jmr.2019.07.025
Shapiro, Y. E., and Meirovitch, E. (2012). Slowly Relaxing Local Structure (SRLS) Analysis of 15N-H Relaxation from the Prototypical Small Proteins GB1 and GB3. J. Phys. Chem. B. 116, 4056–4068. doi:10.1021/jp300245k
Skrynnikov, N. R., Millet, O., and Kay, L. E. (2002). Deuterium Spin Probes of Side-Chain Dynamics in Proteins. 2. Spectral Density Mapping and Identification of Nanosecond Time-Scale Side-Chain Motions. J. Am. Chem. Soc. 124, 6449–6460. doi:10.1021/ja012498q
Smith, A. A., Bolik-Coulon, N., Ernst, M., Meier, B. H., and Ferrage, F. (2021a). How Wide Is the Window Opened by High-Resolution Relaxometry on the Internal Dynamics of Proteins in Solution? J. Biomol. NMR 75, 119–131. doi:10.1007/s10858-021-00361-1
Smith, A. A., Ernst, M., and Meier, B. H. (2017). Because the Light Is Better Here: Correlation-Time Analysis by NMR Spectroscopy. Angew. Chem. 129, 13778–13783. doi:10.1002/ange.201707316
Smith, A. A., Ernst, M., Meier, B. H., and Ferrage, F. (2019a). Reducing Bias in the Analysis of Solution-State NMR Data with Dynamics Detectors. J. Chem. Phys. 151, 034102. doi:10.1063/1.5111081
Smith, A. A., Ernst, M., and Meier, B. H. (2018). Optimized "detectors" for Dynamics Analysis in Solid-State NMR. J. Chem. Phys. 148, 045104. doi:10.1063/1.5013316
Smith, A. A., Ernst, M., Riniker, S., and Meier, B. H. (2019b). Localized and Collective Motions in HET‐s(218‐289) Fibrils from Combined NMR Relaxation and MD Simulation. Angew. Chem. 131, 9483–9488. doi:10.1002/ange.201901929
Smith, A. A., Testori, E., Cadalbert, R., Meier, B. H., and Ernst, M. (2016). Characterization of Fibril Dynamics on Three Timescales by Solid-State NMR. J. Biomol. NMR 65, 171–191. doi:10.1007/s10858-016-0047-8
Smith, A. A., Vogel, A., Engberg, O., Hildebrand, P. W., and Huster, D. (2021b). A Method to Construct the Dynamic Landscape of a Bio-Membrane with experiment and Simulation. ResearchSquare, 1–28. doi:10.21203/rs.3.rs-645823/v1
Smith, T., and Guild, J. (1931). The C.I.E. Colorimetric Standards and Their Use. Trans. Opt. Soc. 33, 73–134. doi:10.1088/1475-4878/33/3/301
Tikhonov, A. N., and Arsenin, V. I. A. (1977). Solutions of Ill-Posed Problems. Washington, DC: Winston and Sons.
Trott, O., Abergel, D., and Palmer, A. G. (2003). An Average-Magnetization Analysis of R1ρ Relaxation outside of the Fast Exchange Limit. Mol. Phys. 101, 753–763. doi:10.1080/0026897021000054826
Trott, O., and Palmer, A. G. (2002). R1ρ Relaxation outside of the Fast-Exchange Limit. J. Magn. Reson. 154, 157–160. doi:10.1006/jmre.2001.2466
Tugarinov, V., Liang, Z., Shapiro, Y. E., Freed, J. H., and Meirovitch, E. (2001). A Structural Mode-Coupling Approach to 15N NMR Relaxation in Proteins. J. Am. Chem. Soc. 123, 3055–3063. doi:10.1021/ja003803v
Virtanen, P. (2020). SciPy 1.0: Fundamental Algorithms for Scientific Computing in Python. Nat. Methods 17, 261–272. doi:10.1038/s41592-019-0686-2
Vos, J. J. (1978). Colorimetric and Photometric Properties of a 2° Fundamental Observer. Color Res. Appl. 3, 125–128. doi:10.1002/col.5080030309
Wennerström, H., Lindman, B., Soederman, O., Drakenberg, T., and Rosenholm, J. B. (1979). Carbon-13 Magnetic Relaxation in Micellar Solutions. Influence of Aggregate Motion on T1. J. Am. Chem. Soc. 101, 6860–6864. doi:10.1021/ja00517a012
Keywords: solid-state NMR, dynamics detectors, model-free analysis, NMR relaxation, molecular dynamics simulation
Citation: Zumpfe K and Smith AA (2021) Model-Free or Not?. Front. Mol. Biosci. 8:727553. doi: 10.3389/fmolb.2021.727553
Received: 18 June 2021; Accepted: 21 September 2021;
Published: 25 October 2021.
Edited by:
Józef Romuald Lewandowski, University of Warwick, United KingdomReviewed by:
Tharin Blumenschein, University of East Anglia, United KingdomPaul Schanda, Institute of Science and Technology Austria, Austria
Copyright © 2021 Zumpfe and Smith. This is an open-access article distributed under the terms of the Creative Commons Attribution License (CC BY). The use, distribution or reproduction in other forums is permitted, provided the original author(s) and the copyright owner(s) are credited and that the original publication in this journal is cited, in accordance with accepted academic practice. No use, distribution or reproduction is permitted which does not comply with these terms.
*Correspondence: Albert A. Smith, albert.smith-penzel@medizin.uni-leipzig.de