GEO dataset mining analysis reveals novel Staphylococcus aureus virulence gene regulatory networks and diagnostic targets in mice
- 1College of Pharmacy, Beihua University, Jilin, China
- 2School of Basic Medical Sciences, Beihua University, Jilin, China
- 3College of Pharmacy, Jilin Medical University, Jilin, China
Staphylococcus (S.) aureus infection is a serious, worldwide health concern, particularly in many communities and hospitals. Understanding the S. aureus pathogenetic regulatory network will provide significant insights into diagnostic target screening to improve clinical treatment of diseases caused by S. aureus. We screened differentially expressed genes between normal mice and S. aureus-infected mice. We used the Gene Expression Omnibus (GEO) DataSets database for functional analysis (GO-analysis) and the DAVID and KEGG databases for signaling pathway analyses. We next integrated the gene and pathway analyses with Transcriptional Regulatory Element Database (TRED) to build an antimicrobial resistance gene regulatory network of S. aureus. We performed association analysis of network genes and diseases using DAVID online annotation tools. We identified a total of 437 virulence genes and 15 transcription factors (TFs), as well as 444 corresponding target genes, in the S. aureus TF regulatory network. We screened seven key network nodes (Met, Mmp13, Il12b, Il4, Tnf, Ptgs2, and Ctsl), four key transcription factors (Jun, C3, Spil, and Il6) and an important signaling pathway (TNF). We hypothesized that the cytokine activity and growth factor activity of S. aureus are combinatorically cross-regulated by Met, Mmp13, Il12b, Il4, Tnf, Ptgs2, and Ctsl genes, the TFs Jun, C3, Spi1, and Il6, as well as the immune response, cellular response to lipopolysaccharide, and inflammatory response. Our study provides information and reference values for the molecular understanding of the S. aureus pathogenetic gene regulatory network.
1 Introduction
Infection caused by Staphylococcus (S.) aureus is an endemic health problem worldwide (Chen et al., 2022; Park et al., 2021; Miller et al., 2020). S. aureus is a common invasive bacterial pathogen that produces staphylococcal enterotoxin (SE), which causes intestinal tract dysfunction (Dicks et al., 2021; Omar et al., 2021) and is responsible for almost all staphylococcal food poisoning. Staphylococcal food poisoning results from food contamination by S. aureus enterotoxin, accounting for 33% of total bacterial foodborne infections in the United States (Haghi et al., 2021). More than 45% of foodborne diseases are caused by S. aureus in Canada (Greco et al., 2020). Therefore, S. aureus pathogenicity and its underlying virulence mechanisms have been a primary research focus. Staphylococcus aureus causes high mortality, which is associated with early excessive inflammation of unknown mechanisms (Wang et al., 2023). Staphylococcus aureus has a powerful virulence secretion system to evade the host’s immune response, and may even promote excessive inflammatory response (Mu et al., 2023); therefore, the host’s regulation of the immune response, especially the key mechanisms controlling inflammation, is crucial for successful resistance to Staphylococcus aureus.
A pathogenic gene permits a pathogen to cause disease (Kotnik et al., 2023). Understanding the role of virulence genes in disease has become a central focus of medical research for the purpose of developing preventive measures, diagnostic tools, treatment approaches, and therapeutic strategies (Jonas et al., 2020). Previous studies on S. aureus pathogenicity have been mainly focused on the expression and function of a single gene using gene knockout, gene silencing, RNA interference, and other genetic approaches (Kane et al., 2018; Scherr et al., 2015). However, these methods are typically laborious and time-consuming, inefficient, and require extensive training, leading to limited success in meeting the needs of clinical medicine. Advanced omics technologies, including gene chip and big data analytics (Giulieri et al., 2020) particularly CRISPR/CAS9 (Chen et al., 2017; Penewit et al., 2018), can simultaneously identify nearly one million sites in genomic DNA, which allows for association analysis between S. aureus-infected diseases and genetic variation. Thus, these technologies provide powerful tools to investigate pathogenic gene regulatory networks and diagnostic targets. However, scientists are challenged by the increasing amounts of transcriptomic data created by high-throughput techniques, including how best to handle and analyze the millions of data points identified by genetic studies of S. aureus-infected diseases with appropriate mathematical and statistical strategies.
The objective of this study was to identify novel S. aureus pathogenic gene regulatory networks and diagnostic targets using the NCBI GEO DataSets database and functional enrichment analysis. In the present study, the gene expression profiles of S. aureus-infected mice were selected from the NCBI GEO Datasets database to assess differentially expressed genes (DEG) using a combination of the linear models and empirical Bayesian methods in limma, an R software package (https://www.r-project.org/), with the traditional t-test. Gene function analysis (GO-analysis) and signal pathway analysis (Pathway-Analysis) were performed using DAVID (Database for Annotation, Visualization and Integrated Discovery) and KEGG (Kyoto Encyclopedia of Genes and Genomes) to select DEG sets that were integrated into TRED (Transcriptional Regulatory Element Database) to construct the S. aureus antimicrobial resistance gene regulatory network. Associations between a given disease and network genes were analyzed using the DAVID online annotation tool.
2 Experimental methods
2.1 Chip data
Chip data were pooled from the NCBI GEO DataSets database (https://www.ncbi.nlm.nih.gov/gds) using the keywords (Staphylococcus aureus) AND “Mus musculus” [porgn: txid9606], while data only from S aureus-infected mice and gene chips with Affymetrix CEL files were adopted (Davis et al., 2007).
2.2 Chip data processing
First, we performed background correction on the chip data, and then the probe fluorescence values were converted into gene expression values using the Expression Console™ software tool (Affymetrix, Santa Clara, California, United States) (www.affymetrix.com). The chip data were logged and normalized by Transcriptome Analysis Console (Affymetrix, Santa Clara, California, United States). Differentially expressed mRNAs were compared between the normal and S. aureus-infected mice using SAM (Significance Analysis of Microarray) (http://www-stat.stanford.edu/∼tibs/SAM/index.html). DEGs with fold change > 2.0 or fold change < −2.0 and a p-value < 0.05 were selected for further study. DEGs overlapping in two or more platforms were further screened with Venn diagrams to account for differences among the chip platforms (Lu et al., 2007).
2.3 Transcription factor (TF) and corresponding target gene screening
We first selected the “Search TF Target Genes” option in http://rulai.cshl.edu/TRED. Next, we selected Factor Name in the Type of search key option and entered the Gene symbol name. In the third step, we selected Mus musculus in Target Gene Organism and selected “all” at Promoter Quality and Binding Quality. At the final step, we searched for corresponding target genes (Zhao et al., 2005).
2.4 Gene co-expression network
We identified a total of 15 TFs and their predicted corresponding target genes. A total of 444 target genes were paired to analyze TF-to-target regulatory relationships. Differential co-expression correlations between gene pairs were estimated by differential co-expression analysis (DCEA) (Markus et al., 2017) and then mapped to mouse TF-to-target pairs to identify TF-gene transcriptional regulatory pairs that were visualized using Cytoscape software (Sun et al., 2017).
2.5 GO function annotation analysis
A set of 437 genes were submitted to the DAVID database (http://david.abcc.ncifcrf.gov/) for enrichment analysis (Huang et al., 2007) of DEG sets with the Functional Annotation Tool, where OFFICIAL_GENE_SYMBOL was selected and the whole genome of Mus musculus was used as the background genes.
2.6 Significance analysis of DEGs
DEGs were annotated based on the NCBI GO database (http://www.geneontology.org/). The significance level and misjudgment rate of each GO were estimated by Fisher’s exact test and chi-squared test (χ2), and the p-value was calibrated with the misjudgment rate to determine significance (p < 0.05) of GOs. The significant GOs (p < 0.05) were manually selected using the European Bioinformatics Institute (EBI) database (https://www.ebi.ac.uk/) (Ashburner et al., 2000).
3 Experimental results
3.1 Chip data
In the present study, chip data were pooled from the NCBI GEO DataSets database using both S aureus-infected mice and Affymetrix gene chips with CEL files as the selection criteria. Three platforms of gene chips were selected and the detailed information is listed in Table 1.
3.2 Chip data processing
After background correction of chip data, the probe fluorescence values were converted into gene expression values using the Expression Console™ software tool and then logged and normalized by Transcriptome Analysis Console. 299, 738, and 385 DEGs were respectively identified from the three platforms by SAM to compare differentially expressed miRNAs between the normal and S. aureus-infected mice. DEGs were then cross-screened; 33 DEGs overlapped in the three platforms, and 324, 22, and 58 DEGs co-existed in two platforms, respectively (Figure 1). Therefore, a total of 437 DEGs that overlapped in more than two platforms were used in subsequent analyses.
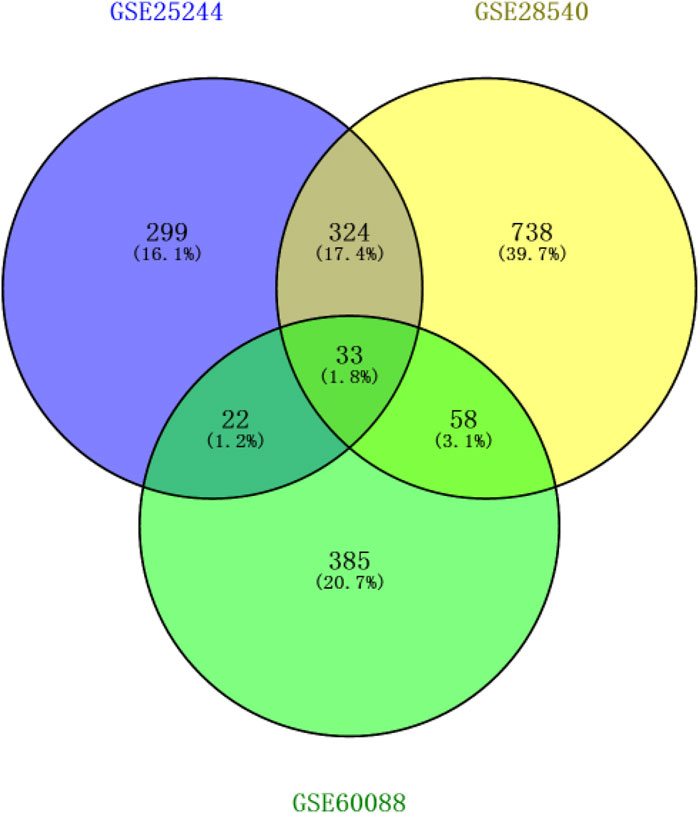
Figure 1. Screening results of genes overlapping in two or more platforms (blue circle represents GSE25244, yellow circle represents GSE28540, and green circle represents GSE60088).
3.3 TF regulatory networks
We used the TRED database to predict possible TFs for 437 DEGs. We identified 15 TFs and 444 corresponding target genes (Table 2). As shown in Figure 2, we visualized these TFs and genes using Cytoscape to develop S. aureus pathogenic gene TF regulatory networks. This analysis demonstrated that 16 target genes were co-regulated by at least three TFs (Table 3). Among these, me was regulated by five TFs, and mmp13, il12b, il4, tnf, ptgs2, and ctsl were regulated by four TFs.
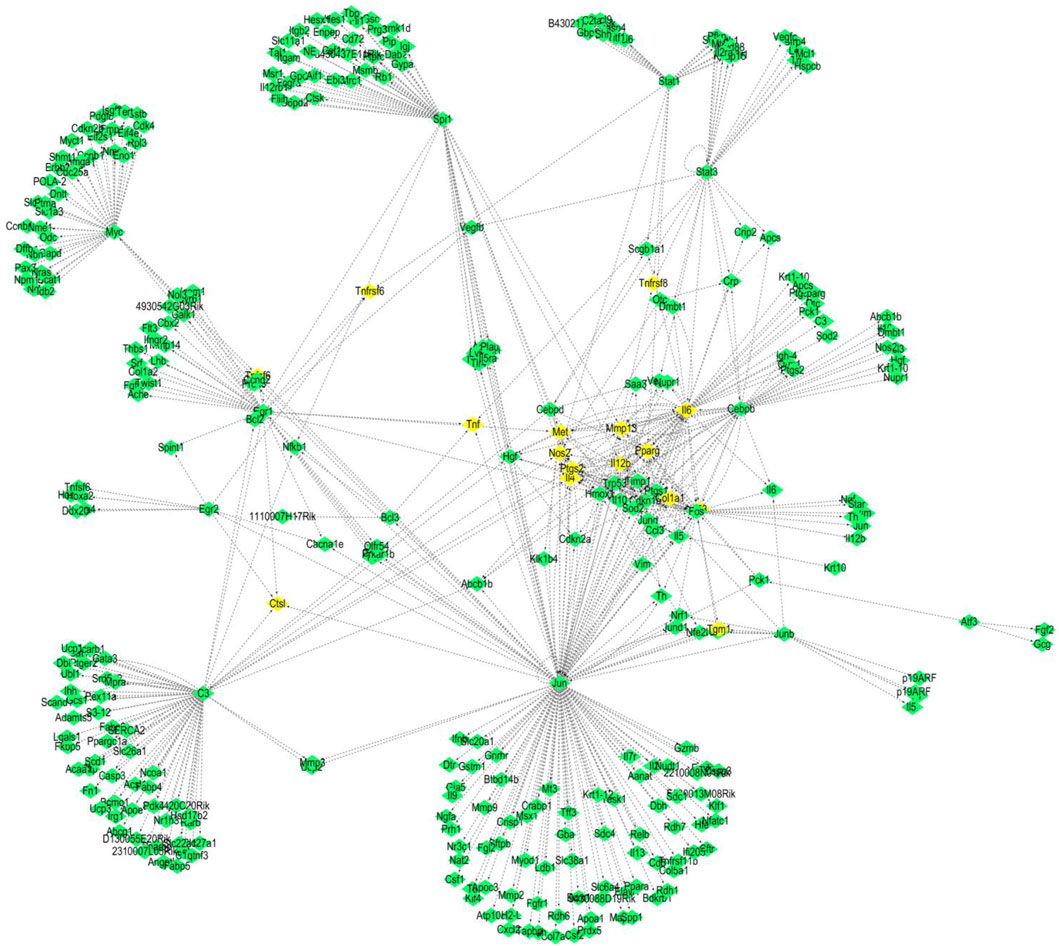
Figure 2. TF regulatory networks of 15 S. aureus virulence genes (yellow represents TFs; green represents corresponding target proteins).
3.4 Analysis of network nodes in TF regulatory networks
As shown in Figure 2, we statistically recorded the network nodes in TF regulatory networks, finding 14 genes with more than 10 nodes. Among these genes, six (ptgs2, trp53, mmp13, hmox1, il4, and pparg) contained more than 15 nodes (Table 4). Met, ptgs2, and mmp13 were regulated by five, four, and four TFs, respectively, showing close association with S. aureus virulence genes.
3.5 Annotation analysis of GO functions of DEGs
437 DEGs were annotated for GO functions (http://www.geneontology.org/), from which the top 10 pathways with the highest p values were further analyzed (Table 5). Ten pathways were primarily associated with disease-related pathways, among which the S. aureus infection pathway ranked first.
4 Discussion
Staphylococcus aureus predominantly resides on the skin and in the nasopharynx of humans, and it is the most prevalent cause of nosocomial and community-acquired bloodstream infections, skin and soft tissue infections, and pneumonia in almost all geographic areas. Thus, S. aureus poses a serious threat to human health and global stability. Methicillin-resistant S. aureus is unresponsive to 60% of antibiotics (Mitevska et al., 2021; Paudel et al., 2021), which is a major underlying cause of several difficult-to-treat life-threatening infections. Therefore, there is an unmet need to understand S. aureus pathogenesis to develop effective prevention and treatment of infection. In the present study, we identified 437 DEGs, from which 15 TFs and their predicted corresponding target genes were used to develop a TF regulatory network. We found several key factors closely related to inflammation and the immune system that are regulated by S. aureus TF regulatory networks. Our findings provide new information and reference values for virulence genes in the transcriptional regulation of S. aureus infection.
We hypothesized that inflammatory and immune system diseases caused by S. aureus are likely regulated by three genes: jun, c3, and spil. Staphylococcus aureus is the most common pathogen that causes inflammatory and immune system diseases, including a variety of suppurative (pus-forming) infections (Carrel et al., 2017), pneumonia, pseudomembranous colitis, pericarditis, and even sepsis (Agarwal et al., 2018). Jun, c3, and spi1 are believed to be involved in inflammation and immune responses. Jun has been reported to inhibit inflammatory factors and participate in immune system regulation, which is supported by Xie et al. (2014) who showed that downregulation of jun decreased expression of pro-inflammatory cytokines such as tumor necrosis factor-α (TNF-α), interferon β (IFN-β), and interleukin 6 (IL-6), but upregulated expression of anti-inflammatory cytokines, including IL-10. Jun is also closely associated with systemic lupus erythematosus (SLE), an autoimmune disease involving multiple organs and systems (Maria et al., 2017). Doníz et al. (2011) reported that jun expression was significantly elevated in peripheral blood mononuclear cells (PBMC) in SLE patients compared to normal controls. C3, the most important molecule encoded by gene c3 in the complement system (Lu et al., 2018), is located at the intersection node of both classical and alternative complement activation pathways, as well as the mannose-binding lectin pathway, indicating that C3 plays an important regulatory role in the complement system, inflammation, and the immune system (Yuan et al., 2024). The complex and diverse C3 cleavage fragments and their binding proteins regulate the complement system via activating the complement cascade and through self-activation and cleavage, as well as by interacting with a variety of relevant factors that promote immune adhesion and pathogen phagocytosis (Fagan et al., 2017). Spi1 affects the immune system by regulating mature B cells in the spleen (Batista et al., 2017). In the present study, we first reported that jun (corresponding to 120 target genes), c3 (corresponding to 62 target genes), and spi1 (corresponding to 49 target genes) regulated most of the target genes, strengthening our hypothesis that jun, c3 and spi1 regulate inflammation and the immune system.
We further revealed that jun and spil are core genes in the S. aureus regulatory network, while c3 plus il6 are secondary core genes. Regulation of core genes in inflammation and immune system diseases is executed mainly by regulating target genes met, mmp13, il12b, il4, tnf, ptgs2, and ctsl. In the present study, we found that all of these genes were regulated by at least four TFs (Figure 2). Met, mmp13, il12b, il4, and ptgs2 were regulated by jun and spil. Met, mmp13, and il12b were simultaneously regulated by il6. Ctsl, ptgs2, and il4 were regulated by jun and c3. In addition to jun, c3, and spil, il6 was has been shown to be involved in inflammatory and immune system diseases, where il6 acted not only as a TF but also as a target gene regulated by a TF. A previous study revealed a close association of il6 with the immune system (Leal et al., 1999) by activating proinflammatory and other cytokines in B cells, hepatocytes, hybridoma cells, and plasma cells to improve the body’s resistance to S. aureus infection. However, it has also been reported that il6 inhibits the adverse effects of macrophages to IFN-γ responsiveness (Saleh et al., 2021). Met, mmp13, and ctsl are all related to cancer (Mo et al., 2017; Yang et al., 2017; Zhang et al., 2016). Further analysis should focus on understanding the molecular mechanism of the core genes that interact with TFs and their corresponding target genes in inflammation and the immune system (Gao et al., 2024).
This study provides evidence that immune functions, including immune response, cellular response to lipopolysaccharide, and the inflammatory response, are regulated by network nodes that contain ptgs2, mmp13, il12b, and met, together with TF jun. In the present study, we identified 14 genes in 10 nodes, of which only four genes (ptgs2, mmp13, il12b, and met) were regulated by four TFs (Table 4). In addition to met, which was regulated by most TFs, ptgs2, mmp13, and il12b significantly regulated TFs and network nodes. All network nodes were compared to previous studies (Kondo et al., 2000; Yamaguchi et al., 2005; Gokulnath et al., 2017; Utsugi et al., 2010; Cui et al., 2013) and we found that most of the network nodes were related on a certain level and strongly correlated with TF jun. The main GO terms of these network nodes are cytokine activity and growth factor activity, functioning immune response, cellular response to lipopolysaccharide, and inflammatory response. In addition, the most significant 10 pathways were associated with immune-related diseases, including S. aureus infection (Dusane et al., 2018), leishmaniasis (Jaton et al., 2016; Cui et al., 2017), rheumatoid arthritis (Insa et al., 2017), and tuberculosis (Ashtekar et al., 2016); however, immune-related TFs and information pathways, including the chemokine signaling (Joanna et al., 2017) and TNF signaling (Nandi et al., 2017) pathways, were closely associated with other diseases. Therefore, in general, these diseases and pathways were associated with immune function.
5 Conclusion
We successfully identified 437 DEGs from the GEO database to develop a TF regulatory network of S. aureus. We analyzed the genes met, mmp13, il12b, il4, tnf, ptgs2 and ctsl and transcription factors Jun, C3, Spil and il6 pathways, and found that most of these genes were on the TNF signaling pathway. At last, we hypothesized that met, mmp13, il12b, il4, tnf, and ptgs2 function together with TFs jun, c3, spil, and il6 to regulate inflammation and the immune system. The present study thus provides information and reference values for understanding the regulatory mechanisms of TFs and their network of S. aureus virulence genes.
Data availability statement
The original contributions presented in the study are included in the article/supplementary material, further inquiries can be directed to the corresponding authors.
Author contributions
GX: Data curation, Writing–original draft. YY: Formal Analysis, Writing–original draft. YL: Data curation, Writing–original draft, Writing–review and editing. YB: Funding acquisition, Writing–original draft, Writing–review and editing.
Funding
The authors declare that financial support was received for the research, authorship, and/or publication of this article. This work was supported by the Jilin Province Science and Technology Development Plan project (YDZJ202201ZYTS206), Science and Technology Research Project of Education Department of Jilin Province (JJKH20220468KJ, JJKH20230078KJ), 2022 Annual graduate Innovation Plan project of Beihua University Research ([2022] 028), Jilin City Science and Technology innovation Development plan project (20190104135).
Conflict of interest
The authors declare that the research was conducted in the absence of any commercial or financial relationships that could be construed as a potential conflict of interest.
Publisher’s note
All claims expressed in this article are solely those of the authors and do not necessarily represent those of their affiliated organizations, or those of the publisher, the editors and the reviewers. Any product that may be evaluated in this article, or claim that may be made by its manufacturer, is not guaranteed or endorsed by the publisher.
References
Agarwal, R., Bartsch, S. M., Kelly, B. J., Prewitt, M., Liu, Y., Chen, Y., et al. (2018). Newer glycopeptide antibiotics for treatment of complicated skin and soft tissue infections: systematic review, network meta-analysis and cost analysis. Clin. Microbiol. Infect. 24, 361–368. doi:10.1016/j.cmi.2017.08.028
Ashburner, M., Ball, C. A., Blake, J. A., Botstein, D., Butler, H., Cherry, J. M., et al. (2000). Gene ontology: tool for the unification of biology. The Gene Ontology Consortium. Nat. Genet. 25, 25–29. doi:10.1038/75556
Ashtekar, R., and Shah, I. (2016). Tuberculosis in a case of hyper immunoglobulin Esyndrome. J. Fam. Med. Prim. Care 5, 871–872. doi:10.4103/2249-4863.201167
Batista, C. R., Li, S. K. H., Xu, L. S., Solomon, L. A., and DeKote, R. P. (2017). PU.1 regulates ig light chain transcription and rearrangement in pre-B cells during B cell development. J. Immunol. 198, 1565–1574. doi:10.4049/jimmunol.1601709
Carrel, M., Zhao, C., Thapaliya, D., Bitterman, P., Kates, A. E., Hanson, B. M., et al. (2017). Assessing the potential for raw meat to influence human colonization with Staphylococcus aureus. Sci. Rep. 7, 10848. doi:10.1038/s41598-017-11423-6
Chen, H. Q., Zhang, J. Y., He, Y., Lv, Z. Y., Liang, Z. T., Chen, J. Z., et al. (2022). Exploring the role of Staphylococcus aureus in inflammatory diseases. Toxins (Basel) 14, 464–474. doi:10.3390/toxins14070464
Chen, W., Zhang, Y., Yeo, W. S., Bae, T., and Ji, Q. (2017). Rapid and efficient genome editing in Staphylococcus aureus by using an engineered CRISPR/Cas9 system. J. Am. Chem. Soc. 139, 3790–3795. doi:10.1021/jacs.6b13317
Cui, G. H., Chen, S. Y., Jiang, B., Zhang, Y., Qiu, N. N., Satoh, T., et al. (2013). Synthesis and characterization of novel thermoresponsive fluorescence complexes based on copolymers with rare earth ions. Opt. Mater. 35 (12), 2250–2256. doi:10.1016/j.optmat.2013.06.010
Cui, G. H., Zhao, D. H., Lv, P., Gao, Z. G., Chen, S. Y., Qiu, N. N., et al. (2017). Synthesis and characterization of Eu(III)-based coordination complexes of modified D-glucosamine and poly(N-isopropylacrylamide). Opt. Mater. 72, 115–121. doi:10.1016/j.optmat.2017.05.051
Davis, S., and Meltzer, P. S. (2007). GEOquery: a bridge between the gene expression Omnibus (GEO) and BioConductor. Bioinformatics 23, 1846–1847. doi:10.1093/bioinformatics/btm254
Dicks, J., Turnbull, J. D., Russell, J., Parkhill, J., and Alexander, S. (2021). Genome sequencing of a historic Staphylococcus aureus collection reveals new enterotoxin genes and sheds light on the evolution and genomic organization of this key virulence gene family. J. Bacteriol. 203, 005877. doi:10.1128/JB.00587-20
Doníz, P. L., Martínez, J. V., Niño, M. P., Abud, M. C., Hernández, C. B., González, A. R., et al. (2011). Expression and function of Cbl-b in T cells from patients with systemic lupus erythematosus, and detection of the 2126 A/G Cblb gene polymorphism in the Mexican mestizo population. Lupus. 20, 628–635. doi:10.1177/0961203310394896
Dusane, D. H., Kyrouac, D., Petersen, I., Bushrow, L., Calhoun, J. H., Jeffrey, F. G., et al. (2018). Targeting intracellular Staphylococcus aureus to lower recurrence of orthopaedic infection. J. Orthop. Res. 36, 1086–1092. doi:10.1002/jor.23723
Fagan, K., Crider, A., Ahmed, A. O., and Pillai, A. (2017). Complement C3 expression is decreased in autism spectrum disorder subjects and contributes to behavioral deficits in rodents. Mol. Neuropsychiatry 3, 19–27. doi:10.1159/000465523
Gao, L. J., Du, B. Y., Ma, Q. H., Ma, Y. H., Yu, W. Y., Li, T., et al. (2024). Multiplex-PCR method application to identify duck blood and its adulterated varieties. Food Chem. 2024, 138673. doi:10.1016/j.foodchem.2024.138673
Giulieri, S. G., Tong, S. Y. C., and Williamson, D. A. (2020). Using genomics to understand meticillin- and vancomycin-resistant Staphylococcus aureus infections. Microb. Genom 6, e000324. doi:10.1099/mgen.0.000324
Gokulnath, M., Swetha, R., Thejaswini, G., Shilpa, P., and Selvamurugan, N. (2017). Transforming growth factor-β1 regulation of ATF-3, c-Jun and JunB proteins for activation of matrix metalloproteinase-13 gene in human breast cancer cells. Int. J. Biol. Macromol. 94, 370–377. doi:10.1016/j.ijbiomac.2016.10.026
Greco, S. L., Drudge, C., Fernandes, R., Kim, J. H., and Copes, R. (2020). Estimates of healthcare utilisation and deaths from waterborne pathogen exposure in Ontario, Canada. Epidemiol. Infect. 148, e70. doi:10.1017/S0950268820000631
Haghi, F., Zeighami, H., Hajiloo, Z., Torabi, N., and Derakhshan, S. (2021). High frequency of enterotoxin encoding genes of Staphylococcus aureus isolated from food andclinical samples. J. Health Popul. Nutr. 40, 27. doi:10.1186/s41043-021-00246-x
Huang, D. W., Sherman, B. T., Tan, Q., Collins, J. R., Alvord, W. G., Roayaei, J., et al. (2007). The DAVID Gene Functional Classification Tool: a novel biological module-centric algorithm to functionally analyze large gene lists. Genome Biol. 8, R183. doi:10.1186/gb-2007-8-9-r183
Insa, J., Achim, K., Christine, P., Gabriele, P. H., Christian, S., Reinhard, E. V., et al. (2017). Staphylococcus aureus bacteremia in patients with rheumatoid arthritis - data from the prospective INSTINCT cohort. J. Infect. 74, 575–584. doi:10.1016/j.jinf.2017.03.003
Jaton, L., Pillonel, T., Jaton, K., Dory, E., Prod'hom, G., Blanc, D. S., et al. (2016). Common skin infection due to Panton-Valentine leucocidin-producing Staphylococcus aureus strains in asylum seekers from Eritrea: a genome-based investigation of a suspected outbreak. Clin. Microbiol. Infect. 22, 739.e5–e8. doi:10.1016/j.cmi.2016.05.026
Joanna, E. S., Joanna, Ś., Ewa, T., Katarzyna, C., Monika, L., Magdalena, R., et al. (2017). Prenatal stress affects viability, activation, and chemokine signaling in astroglial cultures. J. Neuroimmunol. 15, 79–87. doi:10.1016/j.jneuroim.2017.08.006
Jonas, L., Kathrin, H., Katharina, H., Kristina, D., Vanessa, M., Christian, R., et al. (2020). Staphylococcus aureus pathogenicity in cystic fibrosis patients-results from an observational prospective multicenter study concerning virulence genes, phylogeny, and gene plasticity. Toxins (Basel). 12, 279. doi:10.3390/toxins12050279
Kane, T. L., Carothers, K. E., and Lee, S. W. (2018). Virulence factor targeting of the bacterial pathogen Staphylococcus aureus for vaccine and therapeutics. Curr. Drug Targets 19, 111–127. doi:10.2174/1389450117666161128123536
Kondo, T., Matsuda, T., Kitano, T., Takahashi, A., Tashima, M., Ishikura, H., et al. (2000). Role of c-jun expression increased by heat shock- and ceramide-activated caspase-3 in HL-60 cell apoptosis. Possible involvement of ceramide in heat shock-induced apoptosis. J. Biol. Chem. 275, 7668–7676. doi:10.1074/jbc.275.11.7668
Kotnik, U., Maver, A., Peterlin, B., and Lovrecic, L. (2023). Assessmentof pathogenic variation in gynecologic cancer genes in a national cohort. Sci. Rep. 13, 5307. doi:10.1038/s41598-023-32397-8
Leal, I. S., Smedegârd, B., Andersen, P., and Appelberg, R. (1999). Interleukin-6 and interleukin-12 participate in induction of a type 1 protective T-cell response during vaccination with a tuberculosis subunit vaccine. Infect. Immun. 67, 5747–5754. doi:10.1128/IAI.67.11.5747-5754.1999
Lu, J., Lee, J. C., Salit, M. L., and Cam, M. C. (2007). Transcript-based redefinition of grouped oligonucleotide probe sets using AceView: high-resolution annotation for microarrays. BMC Bioinforma. 8, 108. doi:10.1186/1471-2105-8-108
Lu, X. X., Wang, X., Xu, T., Feng, Y., Wang, Y. F., Cai, Z. C., et al. (2018). Circulating C3 and glucose metabolism abnormalities in patients with OSAHS. Sleep. Breath. 22, 345–351. doi:10.1007/s11325-017-1564-8
Maria, T., and Amr, H. S. (2017). Epigenetic variability in systemic lupus erythematosus: what we learned from genome-wide DNA methylation studies. Curr. Rheumatol. Rep. 19, 32. doi:10.1007/s11926-017-0657-5
Markus, D., Ruta, S. J., Duy, N. D., Lisette, J. A. K., and Haja, N. K. (2017). Differential expression and co-expression gene networks reveal candidate biomarkers of boar taint in non-castrated pigs. Sci. Rep. 7, 12205. doi:10.1038/s41598-017-11928-0
Miller, L. S., Fowler, V. G., Shukla, S. K., Rose, W. E., and Proctor, R. A. (2020). Development of a vaccine against Staphylococcus aureus invasive infections: evidence basedon human immunity,genetics and bacterial evasion mechanisms. FEMS Microbiol. Rev. 44, 123–153. doi:10.1093/femsre/fuz030
Mitevska, E., Wong, B., Surewaard, B. G. J., and Jenne, C. N. (2021). The prevalence, risk, and management of methicillin-resistant Staphylococcus aureus infection in diverse populations across Canada: a systematic review. Pathogens 10, 393. doi:10.3390/pathogens10040393
Mo, H. N., and Liu, P. (2017). Targeting MET in cancer therapy. Chronic Dis. Transl. Med. 3, 148–153. doi:10.1016/j.cdtm.2017.06.002
Mu, Y., Fan, Y., He, L., Hu, N., Xue, H., Guan, X., and Zheng, Z. (2023). Enhanced cancer immunotherapy through synergistic ferroptosis and immune checkpoint blockade using cell membrane- coated nanoparticles. Cancer Nanotechnol. 14, 83. doi:10.1186/s12645-023-00234-2
Nandi, A., and Bishay, B. (2017). A novel CCR-2/TLR-2 triggered signaling in murine peritoneal macrophages intensifies bacterial (Staphylococcus aureus) killing by reactive oxygen species through TNF-R1. Immunol. Lett. 190, 93–107. doi:10.1016/j.imlet.2017.07.011
Omar, A. M., Daniele, C., Abdelhamid, H., Sid, A. B., Francesca, F., and Vincenzina, F. P. (2021). Prevalence, enterotoxigenic potential and antimicrobial resistance of Staphylococcus aureus and methicillin-resistant Staphylococcus aureus (MRSA) isolated from Algerian ready to eat foods. Toxins (Basel) 13, 835. doi:10.3390/toxins13120835
Park, S., and Ronholm, J. (2021). Staphylococcus aureus in agriculture: lessons in evolution from a multispecies pathogen. Clin. Microbiol. Rev. 34, e00182-20. doi:10.1128/CMR.00182-20
Paudel, S., Bagale, K., Patel, S., Kooyers, N. J., and Kulkarni, R. (2021). Human urine alters methicillin-resistant Staphylococcus aureus virulence and transcriptome. Appl. Environ. Microbiol. 87, e0074421. doi:10.1128/AEM.00744-21
Penewit, K., Holmes, E. A., McLean, K., Ren, M., Waalkes, A., and Salipante, S. J. (2018). Efficient and scalable precision genome editing in Staphylococcus aureus through conditional recombineering and CRISPR/Cas9-Mediated counterselection. mBio 9, 000677. doi:10.1128/mBio.00067-18
Saleh, H. A., Ramdan, E., Elmazar, M. M., Azzazy, H. M. E., and Abdelnaser, A. (2021). Comparing the protective effects of resveratrol, curcumin and sulforaphane against LPS/IFN-γ-mediated inflammation in doxorubicin-treated macrophages. Sci. Rep. 11, 545. doi:10.1038/s41598-020-80804-1
Scherr, T. D., Hanke, M. L., Huang, O., James, D. B. A., Horswill, A. R., Bayles, K. W., Fey, P. D., Torres, V. J., and Kielian, T. (2015). Staphylococcus aureus biofilms induce macrophage dysfunction through leukocidin AB and alpha-toxin. mBio 6, 010211. doi:10.1128/mBio.01021-15
Sun, C., Yuan, Q., Wu, D., Meng, X., and Wang, B. (2017). Identification of core genes and outcome in gastric cancer using bioinformatics analysis. Oncotarget 8, 70271–70280. doi:10.18632/oncotarget.20082
Utsugi, M., Dobashi, K., Ono, A., Ishizuka, T., Hisada, T., Koga, Y., Shimizu, Y., Kawata, T., Matsuzaki, S. I., Aoki, H., Kamide, Y., and Mori, M. (2010). JNK1 and JNK2 differently regulate IL-12 production in THP-1 macrophage cells. Cytokine 51, 127–131. doi:10.1016/j.cyto.2010.04.002
Wang, M., Wang, Y., Mu, Y., Yang, F., Yang, Z., Liu, Y., Huang, L., Liu, S., Guan, X., Xie, Z., and Gu, Z. (2023). Engineering SIRPα cellular membrane-based nanovesicles for combination immunotherapy. Nano Res. 16, 7355–7363. doi:10.1007/s12274-023-5397-4
Xie, J. J., Zhang, S. P., Hu, Y. X., Li, D. R., Cui, J. M., Xue, J., Zhang, G. Z., Khachigian, L. M., Wong, J., Sun, L. Q., and Wang, M. (2014). Regulatory roles of c-jun in H5N1 influenza virus replication and host inflammation. Biochim. Biophys. Acta 1842, 2479–2488. doi:10.1016/j.bbadis.2014.04.017
Yamaguchi, K., Lantowski, A., Dannenberg, A. J., and Subbaramaiah, K. (2005). Histone deacetylase inhibitors suppress the induction of c-Jun and its target genes including COX-2. J. Biol. Chem. 280, 32569–32577. doi:10.1074/jbc.M503201200
Yang, X., Liao, H. Y., and Zhang, H. H. (2017). Roles of MET in human cancer. Clin. Chim. Acta. 15 (525), 69–83. doi:10.1016/j.cca.2021.12.017
Yuan, G. X., Wang, Y. T., Niu, H. M., Ma, Y., and Song, J. X. (2024). Isolation, purification, and physicochemical characterization of Polygonatum polysaccharide and its protective effect against CCl4-induced liver injury via Nrf2 and NF-κB signaling pathways. Int. J. Biol. Macromol. 261, 129863. doi:10.1016/j.ijbiomac.2024.129863
Zhang, H. M., Zhang, L. S., Wei, L. X., Gao, X. W., Tang, L. I., Gong, W., Min, N. A., Zhang, L. I., and Yuan, Y. W. (2016). Knockdown of cathepsin L sensitizes ovarian cancer cells to chemotherapy. Oncol. Lett. 11, 4235–4239. doi:10.3892/ol.2016.4494
Keywords: Staphylococcus aureus, pathogenic gene, transcription factor, regulatory network, network node
Citation: Xu G, Yang Y, Lin Y and Bai Y (2024) GEO dataset mining analysis reveals novel Staphylococcus aureus virulence gene regulatory networks and diagnostic targets in mice. Front. Mol. Biosci. 11:1381334. doi: 10.3389/fmolb.2024.1381334
Received: 03 February 2024; Accepted: 18 March 2024;
Published: 28 March 2024.
Edited by:
Shahzad Zafar Iqbal, Government College University, Faisalabad, PakistanReviewed by:
Xingang Guan, Taizhou University, ChinaMadhavi Annamanedi, West Virginia University, United States
Copyright © 2024 Xu, Yang, Lin and Bai. This is an open-access article distributed under the terms of the Creative Commons Attribution License (CC BY). The use, distribution or reproduction in other forums is permitted, provided the original author(s) and the copyright owner(s) are credited and that the original publication in this journal is cited, in accordance with accepted academic practice. No use, distribution or reproduction is permitted which does not comply with these terms.
*Correspondence: Yan Lin, lyaner0122@163.com; Yu Bai, baiyu218@163.com