Emotional Instability Relates to Ventral Striatum Activity During Reward Anticipation in Females
- 1Department of Clinical Neuroscience, Karolinska Institutet, Stockholm, Sweden
- 2Department of Neuroradiology, Karolinska University Hospital, Stockholm, Sweden
- 3Department of Clinical Science, Umeå University, Umeå, Sweden
Both non-emotional symptoms, such as inattention, and symptoms of emotional instability (EI) are partially co-varying and normally distributed in the general population. Attention Deficit Hyperactivity Disorder (ADHD), which is associated with both inattention and emotional instability, has been related to lower reward anticipation activation in the ventral striatum. However, it is not known whether non-emotional dysregulation, such as inattention, or EI—or both—are associated with this effect. We hypothesized that altered reward processing relates specifically to EI. To test this, 29 healthy participants were recruited to this functional MRI study (n = 15 females). Reward processing was studied using a modified version of the Monetary Incentive Delay (MID) task. Brown Attention-Deficit Disorder Scales questionnaire was used to assess EI and inattention symptoms on a trait level. We observed less ventral striatal activation during reward anticipation related to the EI trait in females, also when controlling for the inattention trait, but not in the whole sample or males only. Our study suggests the existence of sex differences in the relationship between reward processing and EI/inattention traits.
Introduction
Non-emotional symptoms such as inattention, impulsivity, and hyperactivity typically define Attention Deficit Hyperactivity Disorder (ADHD) according to standardized diagnostic criteria (DSM-5, 2013). ADHD affects a substantial proportion of people worldwide—5–7% of children (Spencer et al., 2007) and 2.5% of adults (Simon et al., 2009). However, in clinical practice, emotion dysregulation, including emotional instability (EI), has been reported in a subpopulation of ADHD patients (Skirrow and Asherson, 2013; Shaw et al., 2014; Moukhtarian et al., 2018). It is still in dispute whether this is part of the core ADHD disorder (Sjöwall et al., 2013; Shaw et al., 2014), or rather reflects psychiatric comorbidity, such as conduct disorder (CD), emotional instability personality disorder (EIP), anxiety disorders or major depressive disorder (MDD; Rubia, 2011; Shaw et al., 2014; Katzman et al., 2017).
The cognitive core capacity theory proposes a way to unify non-emotional aspects of ADHD with emotional instability (EI), i.e., rapidly shifting and intense emotional responses rather than a prolonged lowered mood (Petrovic and Castellanos, 2016). The theory also suggests that ADHD and EI related symptoms—or traits—are normally distributed across the general population and depend on the underlying regulatory capacities for non-emotional and emotional processes. When these traits result in the functional loss they are regarded as clinically relevant symptoms constituting a psychiatric diagnosis. Thus, to fully describe the mechanism underlying ADHD and EI disorders, the full spectrum ranging from subclinical states to the clinical disorders must be studied. This reasoning is in line with the concept of Research Domain Criteria (RDoC; Insel et al., 2010; Cuthbert and Insel, 2013), where different aspects of disorders are broken down into components that may be investigated separately on a dimensional level, and then tied together for a more consistent and structured understanding of the underlying mechanisms. It has been suggested that non-emotional ADHD symptoms and EI have both common (domain-general) and distinct (domain-specific) underlying neural mechanisms, mirrored in partially overlapping but also unique neuronal networks (Rubia, 2011; Petrovic and Castellanos, 2016). Apart from emotional top-down regulation, it has been suggested that reward processing is altered in patients with ADHD and EI (Castellanos et al., 2006; Scheres et al., 2007; Thorell, 2007; Strohle et al., 2008; Stark et al., 2011; Carmona et al., 2012; Costa Dias et al., 2013; Edel et al., 2013; Furukawa et al., 2014; White et al., 2014; Kappel et al., 2015; Yu et al., 2015; Bayard et al., 2018). Non-emotional ADHD symptoms and EI, as well as reward processing, have often been investigated in clinical populations without taking the dimensionality of symptoms in the normal population into account. The RDoC approach suggests that similar alterations could be coupled to non-emotional and EI traits also at a subclinical level. Additionally, studies including patients often include various confounds such as secondary effects of medication and co-morbidities as well as a larger effect of illicit drug use. This further highlights the importance of complementing the existing research with a dimensional approach where these confounds are not present.
Prefrontal and anterior cingulate cortex (ACC) regions, as well as the insular cortex, are involved in the representation of intrinsic reward value and subjective feeling states elicited by receiving a reward (O’Doherty et al., 2001, 2006; Craig, 2002, 2003; Kringelbach and Rolls, 2004; Kable and Glimcher, 2007; Petrovic et al., 2008; Naqvi and Bechara, 2009; Dillon et al., 2010; Namkung et al., 2017; Oldham et al., 2018). Dopaminergic circuits and the ventral striatum (VS) are essential for reward learning by mediating the reward error signal (Schultz et al., 1997; Pessiglione et al., 2006; Daniel and Pollmann, 2014; Schultz, 2016) and for motivation and salience (Berridge, 2018; Oldham et al., 2018). Functional imaging studies of reward anticipation, as well as reward receipt, involve VS activation in humans (Oldham et al., 2018).
Reduced activation of VS during reward anticipation has been shown repeatedly in ADHD patients, while activation during reward outcome has been less extensively investigated with varying results (Scheres et al., 2007; Strohle et al., 2008; Stark et al., 2011; Carmona et al., 2012; Edel et al., 2013; Furukawa et al., 2014; Kappel et al., 2015). Patients with ADHD show a relatively high preference for smaller immediate rewards over larger delayed rewards (Castellanos et al., 2006; Thorell, 2007; Yu et al., 2015)—a behavior that has been associated with altered connectivity between the nucleus accumbens (NAcc) and prefrontal regions in children with ADHD (Costa Dias et al., 2013). Since ADHD is highly correlated with EI traits, it remains unclear whether the aberrant activation of VS is related to EI rather than to typical non-emotional ADHD symptoms. This question pertains both to ADHD patients and to ADHD traits. In line with this reasoning, the tendency to discount the value of a delayed reward is associated with both ADHD traits and CD traits (characterized by EI) respectively in a large community sample (Bayard et al., 2018) and similar results have been verified in CD and ADHD patients without psychiatric co-morbidity (White et al., 2014).
The overall aim of the present study was to investigate the relationship between EI and non-emotional traits in reward anticipation in a non-clinical sample. Inattention was chosen to best represent the non-emotional dimension, as previously done (Petrovic et al., 2016). We hypothesized that the EI trait would be uniquely linked to a lower reward anticipation signal in the VS also when controlling for the inattention trait. We also hypothesized that ACC and insular related activity during reward outcome would be associated with the EI trait, rather than inattention. We used a modified version of the Monetary Incentive Delay (MID) task (Knutson et al., 2001a,b), which has been specifically designed to study neural correlates of reward anticipation and outcome with fMRI. Since sex differences have been reported both about ADHD symptom profiles and reward processing (Rucklidge, 2010; Bobzean et al., 2014; Davies, 2014; Mowlem et al., 2018; Becker and Chartoff, 2019), we also explored sex differences in reward processing concerning EI and inattention traits.
Materials and Methods
Participants
Twenty-nine healthy participants were included in the present study (see Supplementary Material for details of recruitment). All subjects gave written and oral consent for participation in the study, which was conducted according to the Helsinki Declaration and approved by the regional ethics committee in Stockholm (application number 2015/127-31/1, amendment number 2016/1711-32). Each participant received a total of 840 SEK (≈84 Euro) for participation in the study. This sum was equivalent to the total win in the MID task (see below).
Assessment
Inattention and EI traits were assessed with the Brown Attention-Deficit Disorder Scales (Brown-ADD) self-report questionnaire (Brown, 1996). The questionnaire contains 40 items, divided into five subscales assessing different aspects of ADD symptoms—Activation (Organizing, Prioritizing and Activating to Work), Attention, herein referred to as Inattention (Focusing, Sustaining and Shifting Attention to Tasks), Effort (Regulating Alertness, Sustaining Effort, and Processing Speed), Affect, herein referred to as Emotion Instability (Managing Frustration and Modulating Emotions), Memory (Utilizing Working Memory and Accessing Recall). A total score combining all five subscales can be obtained. Answers range from 0 (“never”) to 3 (“almost daily”). For this study, we were interested in the Inattention and the Emotion Instability subscales to represent non-emotional ADHD trait and EI trait, respectively, as done previously (Petrovic et al., 2016). The maximum score for Inattention is 27, the maximum score for Emotion Instability is 21. Higher subscale scores indicate more difficulties in that particular domain.
Experimental Stimuli and Task
We used a modified version of the MID task, originally developed by Knutson and colleagues (Knutson et al., 2001a,b). Two pseudo-randomized presentation orders were used to avoid possible order effects. The details of the modified version of the MID task are presented in Figure 1.
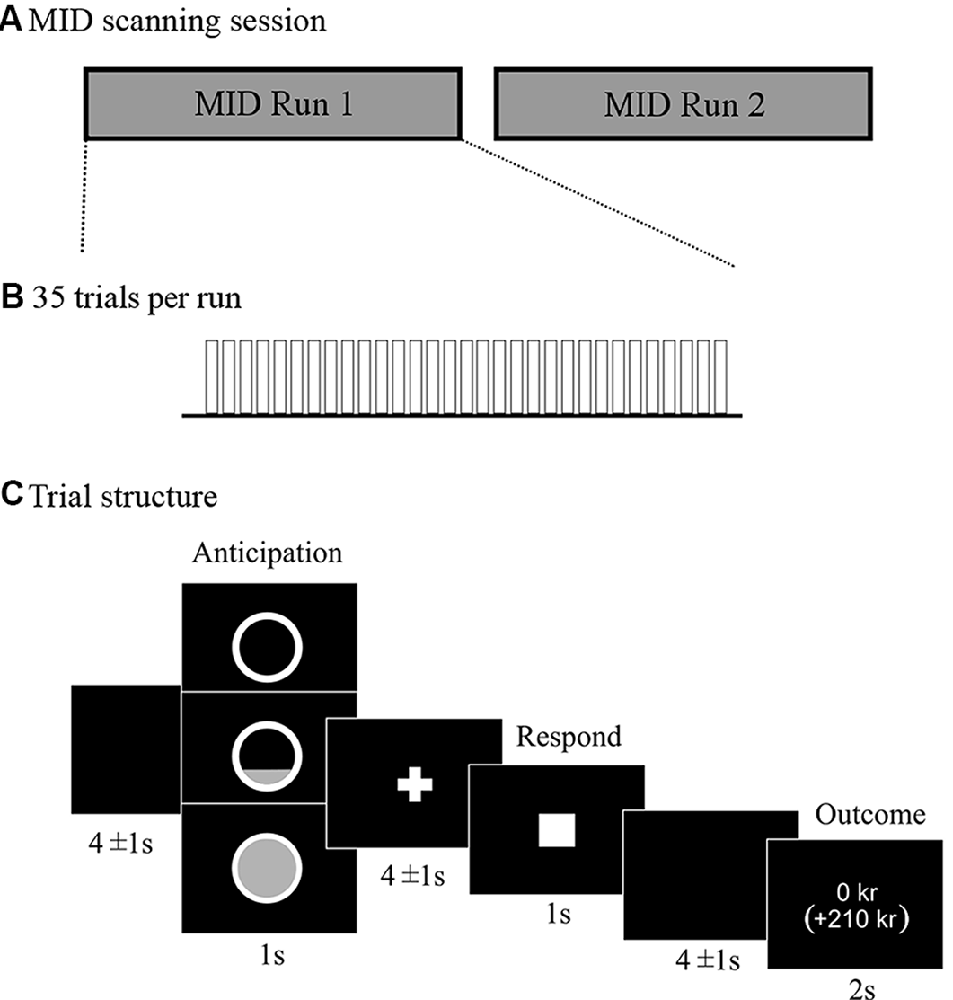
Figure 1. Description of the monetary incentive delay (MID) scanning session (A) and trial structure of the modified version of the MID task (B,C). Presentation software (Neurobehavioral Systems) was used to present the task in the scanner. The whole MID task required ≈35 min of scanning. Each of the two runs consisted of 35 trials of ≈16 s each, which resulted in 9.3 min per run. This led to an approximate scanning time of 10 min per run including instructions at the beginning of each run and repetition of trials due to possible mistakes of the participant. There was a total of 70 trials. The outcome was not dynamically adjusted but fixed to yield the same number of wins for each participant with a success rate of 50% for each reward level. Participants were told that different trials would vary in difficulty, and they were instructed to always respond as fast as they could to win as much as possible, even if some trials would require an impossibly fast response and be highly unlikely to result in a win. Participants were explicitly instructed to only press the button once for each trial. There were 14 Baseline trials where participants anticipated no win, and could not win anything, but were encouraged to press the button nevertheless in the same way as in the reward trials. There were 28 trials with the opportunity to win 10 SEK (subsequently referred to as Low win, equivalent to ≈1 Euro), and 28 trials with the opportunity to win 50 SEK (subsequently referred to as High win, equivalent to ≈5 Euro). As the actual winning rate was fixed, 50% of the trials resulted in “Failed” win and consequently no reward outcome. The uncertainty of the outcome was important to keep participants motivated to perform. The outcome phase included a presentation of the winning amount in the current trial, as well as the total accumulated sum below in parentheses.
MRI Data Acquisition
All participants practiced a test version of the MID task of about 2.5 min on a computer and were subsequently placed inside a 3T GE scanner (Discovery MR750; GE, Fairfield, CT). Anatomical and fMRI scans were acquired using an eight-channel head coil. In addition to the fMRI scan, a T1-weighted scan was performed and later used for co-registration of functional MRI data for each participant.
For the functional scans, 45 slices were acquired in an interleaved order using a gradient-echo EPI sequence (flip angle = 70°, echo time = 30 ms, repetition time = 2,200 ms) with a field of view of 23.0 cm, matrix size of 76 × 76, slice thickness of 3 mm, slice spacing 0.3 mm. The T1-weighted images were acquired using a 3D-BRAVO sequence (TR = 6.40 ms, TE = 2.81 ms, FOV = 24.0 cm, flip angle = 12°, inversion time 450 ms, acquisition matrix 240 × 240 × 180, with isotropic voxel size 1 × 1 × 1 mm3. The B0 Fieldmap sequence was used to acquire 45 slices in an interleaved manner using a gradient-echo EPI sequence (TR = 755.6 ms, TE = 7.0 ms, flip angle = 45°, FOV = 22.0 cm, acquisition matrix 64 × 64, slice thickness 3.0 mm, slice spacing 0.3 mm).
MRI Data Pre-processing
The collected data was arranged to follow the Brain Imaging Data Standard (BIDS; Gorgolewski et al., 2016). The re-organized data was run through the fMRIPrep pipeline, version 1.0.11 (Esteban et al., 2019). Processing steps included skull-stripping, brain tissue segmentation, normalization to MNI space, brain-mask extraction of BOLD images, motion-correction, segmentation, and co-registration of BOLD images to T1. The B0 field map was included to account for susceptibility distortion fields. For details on fMRIPrep’s processing steps, see Supplementary Material Section 2.2.1. The output from fMRIPrep was further smoothed in SPM12 (Statistical parametric mapping, The Welcome Department of Imaging Neuroscience, Institute of Neurology, University College London) running on Matlab 14 (MATLAB 2014, The MathWorks Inc., MA, USA), using a Gaussian smoothing kernel of FWHM = 6 mm. An automated quality control procedure included in the fMRIPrep pipeline enabled the quality check of all participants’ scans, focusing on framewise displacement outliers.
Data Analysis
Methods to confirm that our version of the MID-task-evoked similar behavioral and fMRI responses as previous MID studies are described in detail in Supplementary Materials.
Behavioral Data
To test our main hypothesis that altered reward processing is related to EI rather than inattention trait on a behavioral level, we correlated mean reaction time (RT; Baseline and Win trials), reaction time variability (RTV; defined as the standard deviation of RT) and response time speeding (RTS; Baseline RT—mean Win RT) with B-ADD scores of Inattention and Emotion Instability. For these analyses, we ran multiple regressions using the “lm” function in R version 3.1.3 (R Core Team, 2015). RT, RTV, and RTS were dependent variables, and Inattention and Emotion Instability were independent variables in the models. We further controlled for age and sex by adding those as independent variables. RTS represents the relative difference between a non-incentive condition and the high and low incentive conditions for each participant and a similar approach has been used previously to quantify differences in behavior in the MID task (Veroude et al., 2016; van Hulst et al., 2017). When response times were longer than 1 s the target was no longer presented on the screen and such trials did not lead to a reward. Since too slow responses during low-incentive conditions could reflect low motivation, removing these trials could result in incorrect representations of average RT and RTV and they were therefore included in the analyses. Participants were explicitly instructed to press the response button only once for each trial, and multiple response trials were excluded since they represented the inability to follow these instructions.
fMRI Data
The onset times of events of interest in the MID task were those of anticipation of reward and reward outcomes. The anticipation phase was modeled as a stick function and convoluted with the canonical hemodynamic response function (HRF) to the onset of the reward anticipation cue of either Baseline, Low win, or High win. In line with the behavioral analysis, trials with an RT of >1 s were included in the anticipation phase analysis, while multiple response trials or trials with omitted responses were excluded. Similarly, successful outcome conditions of Low win and High win were modeled separately as stick functions, convolved with the HRF. Even if the Baseline outcome and the outcome of “Failed” win trials all resulted in no-win, these conditions were modeled separately since they were thought to represent different underlying neural processes. Button press and motion parameters were included in the model as regressors of no interest.
Main first-level contrasts were defined by combining the two win conditions (Low win and High win) and contrasting those against Baseline (Win vs. Baseline) both for the reward anticipation phase and the reward outcome phase. The two win conditions were combined since our hypothesis only concerned reward in general and not a specific level of reward. The “Failed” win trials resulting in no-win outcome were not considered in the a priori hypotheses, but brain activation associated with “failed” reward outcome (“Failed” win vs Baseline contrast) was explored in follow-up analyses and reported in Supplementary Materials for completeness.
We used an ROI approach for our main analyses. The pre-defined ROI for the anticipation of reward was based on peak activations in left and right VS reported in a recent meta-analysis on MID task brain activation (Oldham et al., 2018). We created a bilateral ROI around the reported peak activations (spherical ROIs, radius = 6 mm, Supplementary Figure S1A). For the reward outcome phase, we based the ROIs on peak activations located in rostral ACC (rACC) and bilateral insula (spherical ROIs, radius = 10 mm, the left and right insula were combined as one ROI) reported in a study (Dillon et al., 2010) that applied similar jittering and baseline strategies for the reward outcome phase as in the present study (Supplementary Figure S1B).
To test our a priori hypotheses, we ran t-tests on the second level including B-ADD Inattention and Emotion Instability scores as regressors of interest within the pre-defined ROIs. Small volume correction was applied within these regions, and clusters were considered significant if the FWE corrected peak p-value was <0.05. As a next step, we tested whether correcting for age and sex affected brain activation. Since reward processing differs between sexes (Bobzean et al., 2014; Becker and Chartoff, 2019) and the symptomatology profile differs between female and male ADHD patients (Rucklidge, 2010; Trent and Davies, 2012; Davies, 2014) we explored the effects of sex-by-Emotion Instability and sex-by-Inattention interaction on brain activity. Finally, we ran a whole-brain analysis to explore whether activation of additional regions showed correlations with B-ADD Emotion Instability or Inattention scores.
We complemented the small volume correction ROI approach by analyzing extracted mean parameter estimates from the pre-defined ROIs. This analysis was used to compare activation related to the different reward levels, as well as for follow-up analysis of the reward anticipation signal changes in VS. This commonly used approach in fMRI analyses provides information on more general increases or decreases in signal over a larger brain region, enabling noise reduction especially in relatively small, functionally distinct regions (Poldrack, 2007; Poldrack et al., 2011).
Results
Description of the Sample
Twenty-nine participants were included in the analyses (15 females), mean age 28.94 (SD = 6.47, min = 18.70, max = 46.50). Distribution of B-ADD subscales Inattention and Emotion Instability and the correlation between these two dimensions in each participant (r = 0.27, p = 0.16) are shown in Figure 2. There was no significant statistical difference between sexes in these measures (Inattention: t(27) = 0.60, p = 0.55, Emotion Instability: t(27) = 0.97, p = 0.34). Additional descriptive characteristics of the sample can be found in Supplementary Table S1.
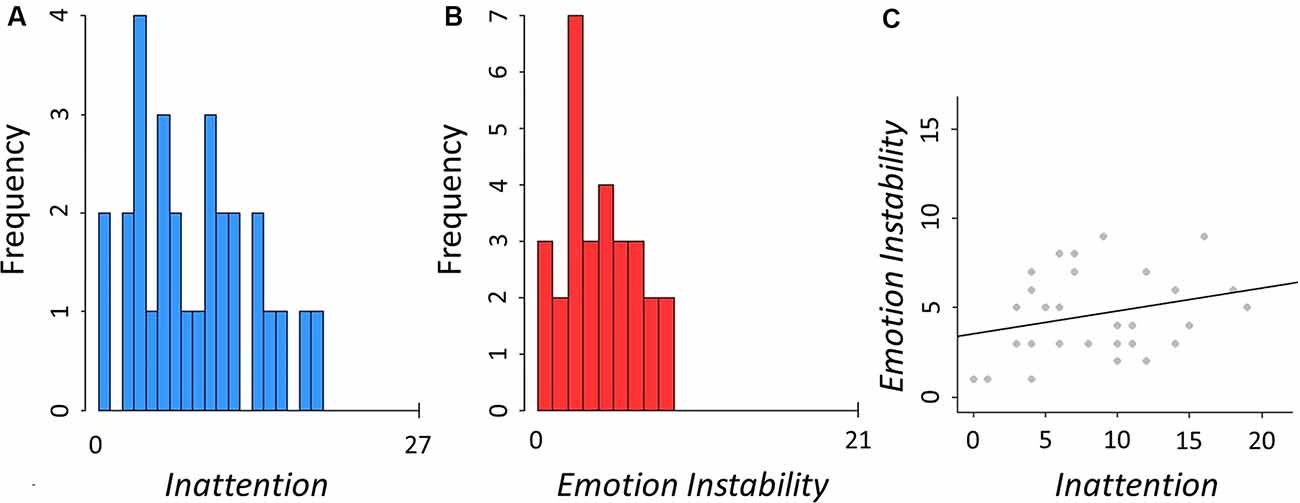
Figure 2. Descriptives of the assessed traits. (A) Distribution of B-ADD Inattention, mean = 8.59 (SD = 5.03, min = 0, max = 19). (B) Distribution of B-ADD Emotion Instability, mean = 4.59 (SD = 2.37, min = 1, max = 9). (C) Correlation between Inattention and Emotion Instability (r = 0.27, p = 0.16).
Behavioral Results
To confirm that our version of the MID task evoked similar behavioral responses as previous versions we ran a linear mixed effects model (described in Supplementary Materials). This analysis showed a significant effect of Win vs. Baseline on RT (MRT Win = 0.26 s (s), SD = 0.05); MRT Baseline = 0.29 s, SD = 0.10); t(1,936) = −5.72, p < 0.001; Figure 3A). Further detailed results of the different reward levels are presented in Supplementary Materials, Supplementary Figure S2 and Supplementary Table S2. The main behavioral analysis showed no significant correlations between the traits of interest (B-ADD Inattention or Emotion Instability) and RT or RTV and adjusting for age and sex did not change the results. There was no main effect of sex or a sex-by-Emotion Instability/Inattention interaction on RT or RTV.
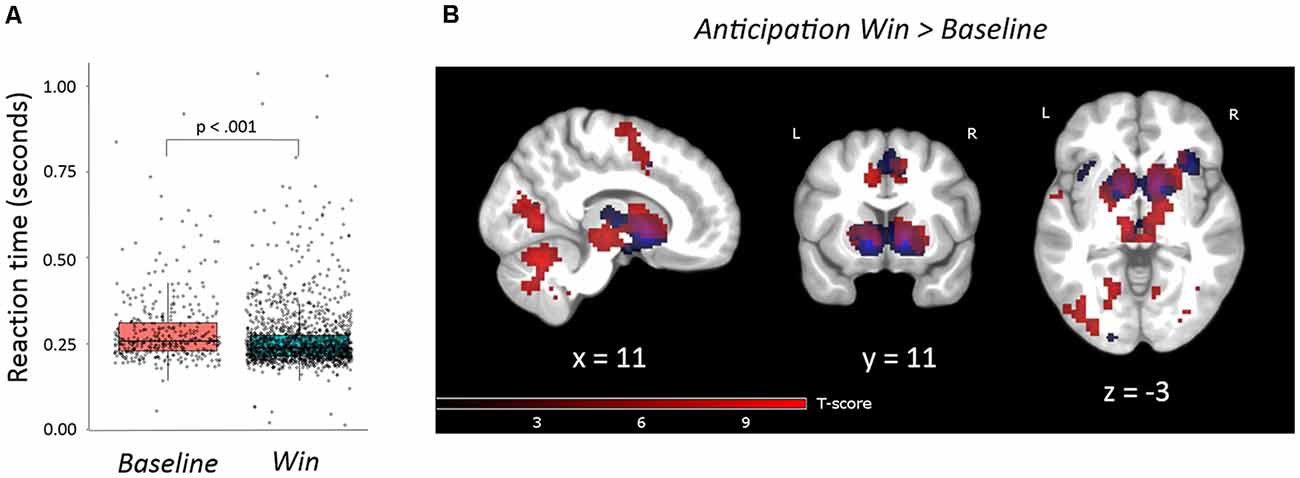
Figure 3. (A) Reaction times for win trials were statistically shorter than for baseline trials (beta = −0.03, t(1936) = −5.72, p < 0.001). The linear mixed-effects model was statistically significant (F = 32.70, p < 0.001). (B) Task activations of contrast anticipation Win vs. Baseline. FWE, p = 0.05, clusters >20 voxels. Activations are overlaid on an average T1 image based on our 29 participants. Task activations (red color) in this study overlap with activations reported by Oldham and colleagues (blue color; Oldham et al., 2018). For the exact location of activations, see Supplementary Table S3.
A trend level moderate correlation was observed between RTS and Emotion Instability (r = 0.32, p = 0.09), which was still present when controlling for Inattention (standardized beta-weight = 0.35, p = 0.08). There was no significant correlation between Inattention and RTS and no main effect of sex or sex by Emotion Instability/Inattention interaction.
fMRI Results
Main Activations
During reward anticipation, the whole-brain analysis showed nine large significant main activation clusters (FWE-corrected) for the contrast Win vs. Baseline expanding over bilateral VS and adjacent regions, thalamus, ACC, right anterior insula, pre-frontal, motor, parietal, occipital, cerebellar and brain stem regions (Figure 3B and Supplementary Table S3). In the reward outcome condition (excluding “Failed” win trials, see “Materials and Methods” section) the whole-brain analysis showed significant activations (FWE-corrected) for Win vs. Baseline in a network including the bilateral anterior insula and rACC extending into dorsal ACC (dACC; Figure 4, Supplementary Table S4). Results were similar for “Failed” win outcomes (Supplementary Figure S6, Supplementary Table S5, see Supplementary Materials for more detail), though medial activations were slightly more dorsal than for successful reward outcomes. Further detailed results of the different reward levels are presented in Supplementary Materials and Supplementary Figures S3, S4 and S5.
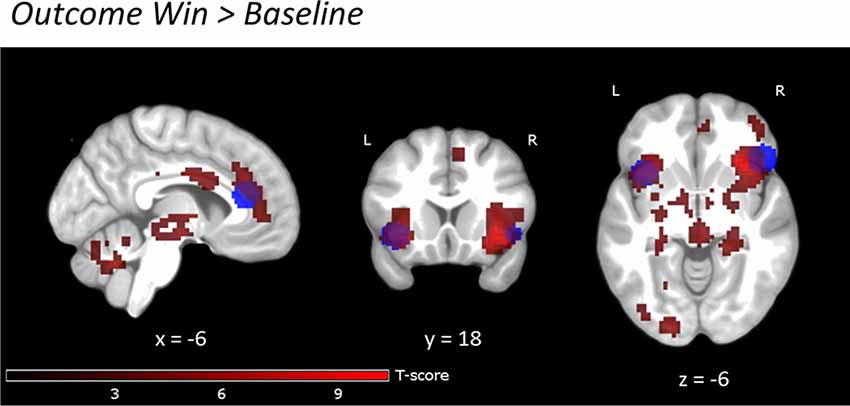
Figure 4. Reward outcome Win vs. Baseline. Uncorrected, p < 0.001, clusters >20 voxels. Activations are overlaid on an average T1 image based on our 29 participants. Task activations (red color) overlap with activations in the study by Bayard et al., 2018 (blue color) in bilateral insula and rACC. For the exact location of activations, see Supplementary Table S4.
Relation Between Reward Processing Brain Activation and Emotion Instability/Inattention Traits
Reward anticipation: we did not find any significant correlations between the traits of interest (Emotion Instability or Inattention) and activations in the contrast anticipation Win vs. Baseline within the VS ROI. There were no significant correlations of reward anticipation activation and age within the predefined ROI.
Reward outcome: there were no correlations of Emotion Instability or Inattention within the pre-defined ROIs in rACC and bilateral insula for successful reward outcomes (Win vs. Baseline). There were no significant correlations of reward outcome activation and age within our pre-defined ROIs.
Sex Differences in Correlations Between Brain Activation and Emotion Instability/Inattention
We found a significant effect of sex (controlling for Emotion Instability and Inattention) on activation during reward anticipation in the left VS (Supplementary Figure S7). Analysis of the mean parameter estimates, i.e., the average signal change, from the pre-defined bilateral VS ROIs during reward anticipation revealed a trend significant effect of sex (controlling for Emotion Instability and Inattention, standardized beta-weight = 0.43, p = 0.053) as well as an additional interaction effect of sex-by-Emotion Instability (controlling for sex, Emotion Instability, and Inattention, p = 0.01) on average signal change in VS during reward anticipation. See also Supplementary Figure S8 for visualization of the corresponding small volume corrected voxel-wise analysis within the bilateral VS ROI.
There were no effects of sex, sex-by-Emotion Instability interaction, or sex-by-Inattention interaction on reward outcome activation within our pre-defined ROIs.
In females, we observed statistically significant negative correlations between Emotion Instability and activation bilaterally within the pre-defined VS ROI for the anticipation of Win vs. Baseline, applying small volume correction (Table 1). The results were similar when controlling for Inattention (Figure 5, Table 1) or age. There were no significant correlations of Inattention and brain activation during anticipation of reward when controlling for Emotion Instability in the female subsample.
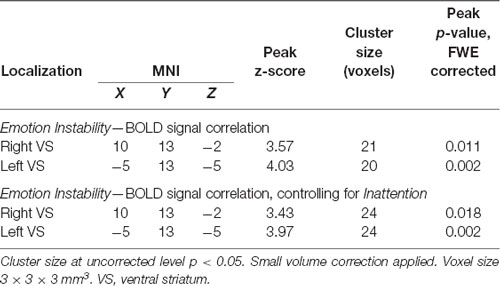
Table 1. Correlations of Emotion Instability and BOLD signal within the bilateral VS during reward anticipation in females.
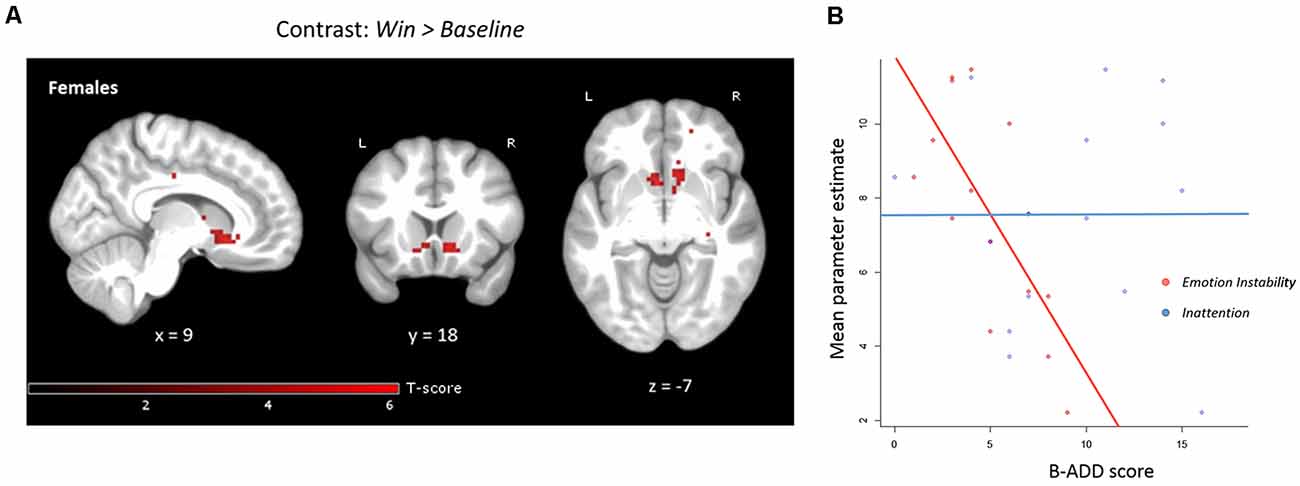
Figure 5. (A) Negative correlation with Emotion Instability, controlling for Inattention in anticipation Win vs. Baseline for females only (n = 15). Both the right and left VS clusters were significant when applying small volume correction (within the bilateral pre-defined VS ROI). Note that no mask is applied in the above figure for clarity, uncorrected level, p < 0.001. (B) Mean parameter estimates extracted from the pre-defined bilateral VS ROI during reward anticipation correlated negatively with Emotion Instability (r = −0.71, p < 0.01, when controlling for Inattention: standardized beta-weight = 0.73, p < 0.01). There was no significant correlation of mean parameter estimates extracted from bilateral VS and Inattention (r = 0.006, p = 0.98). The maximum B-ADD score for Emotion Instability was 21 (maximum in the current sample: 9) and for Inattention 27 (maximum in the current sample: 16).
In males, there were no significant correlations between Emotion Instability/Inattention and brain activation during anticipation of reward.
Discussion
The present study found partial support for the hypothesis that emotional instability (EI) is related to decreased activity in the ventral striatum (VS) during reward anticipation. There was an interaction effect of sex by Emotion Instability score and in female subjects, VS activity related negatively to Brown Attention-Deficit Disorder Scales (B-ADD) Emotion Instability score, even when adjusting for non-emotional B-ADD Inattention score. However, this relation could not be observed in the sample as a whole or males. Activation in the rostral ACC (rACC) and bilateral insula during reward outcome did not correlate with Emotion Instability, and there was no indication of a sex-by-Inattention/Emotion Instability interaction for the outcome phase.
The here applied MID task produced similar main activations as in previous studies for the reward anticipation phase, including VS activation (Oldham et al., 2018). However, in contrast to previous studies, we did not observe VS activation during the outcome phase. One reason for the diverse results may be that most studies have not jittered between all phases of the MID task, which makes it difficult to separate brain activations from the different task events. Thus, VS activation during the outcome phase observed in previous studies might be confounded by VS activation associated with anticipation. The present version of the task overcomes this issue and indicates that VS activation is related to anticipation rather than a rewarding outcome. Also, we separated the “failed” reward outcome from a successful reward outcome and found that both conditions elicit overlapping activations in ACC and insula. This implies a more general involvement of these regions in different types of emotional and feeling states.
The main finding in the present study was the negative correlation between Emotion Instability scores and VS activation during reward anticipation in females, also when controlling for Inattention scores. Previous functional imaging studies have shown that both clinical ADHD and high ADHD traits are related to abnormally low activation in VS during reward anticipation (Scheres et al., 2007; Strohle et al., 2008; Stark et al., 2011; Carmona et al., 2012; Edel et al., 2013; Furukawa et al., 2014; Kappel et al., 2015). Those studies have rarely considered that emotional regulation capacity could potentially influence the results. Our findings are in line with the cognitive core capacity theory, which suggests that mechanisms underlying non-emotional ADHD traits, such as inattention, and EI are partially shared and partially domain-specific (Petrovic and Castellanos, 2016). The current study supports the suggestion that emotional and non-emotional symptoms should be considered separately. Also, our results may have clinical implications, since the core ADHD diagnostic criteria do not consider emotional aspects of psychopathology which could potentially identify ADHD subtypes requiring different treatments. Also, our dimensional approach to parse different traits of ADHD in a population sample and investigate their neural correlates is supported by the Research Domain Criteria (RDoC) concept (Insel et al., 2010; Cuthbert and Insel, 2013) as a way to better understand psychiatric disorders.
Our results suggest that there is an association between the capacity of emotion regulation (mirrored in the degree of EI) and low-level reward processing (mirrored in VS hypoactivation during reward anticipation) and that this relationship is independent of non-emotional regulation capacity (mirrored in the degree of non-emotional inattention symptoms). To the best of our knowledge, it is the first time that this association has experimental support. We suggest that our observations may result from suboptimal dopaminergic signaling. The relation between emotion regulation capacity and reward anticipation processing may be mirrored in the function of different dopamine pathways projecting towards prefrontal regions and ACC (such as medial PFC, orbitofrontal cortex and rACC), and dopamine pathways projecting towards VS—involved in simple reward anticipation and reward error signaling (Hauser et al., 2016). Besides, individuals may also present with varying functions in dopamine projections primarily towards either emotional or non-emotional top-down regulatory networks (Petrovic and Castellanos, 2016). Although these interact, the functional balance between them may partially differ between individuals and subsequently affect also reward processing to a varying degree.
Finally, our results underscore the need to disentangle the underlying mechanisms of emotional and non-emotional symptomatology related to ADHD and EI about sex. This is also emphasized by previous research suggesting sex differences in reward processing (Bobzean et al., 2014; Becker and Chartoff, 2019), ADHD symptomatology (Rucklidge, 2010; Davies, 2014), and ADHD treatment efficacy (Hodgson et al., 2014; Mowlem et al., 2018).
There were several limitations to this study. Due to small subsamples in the explorative analyses, potential sex differences indicated here should be treated with caution until further replication. Further, the B-ADD self-rating scale used in this study might only partly reflect the constructs of interest. To better understand underlying neural processes, there is a need to develop tools that better quantify and successfully isolate EI and non-emotional ADHD traits.
Also, depressive symptoms are known to influence reward processing (Whitton et al., 2015), which could potentially have contributed to our findings. However, since all participants reported no previous or current depression this is unlikely. Also, fluctuations of hormonal levels over the menstrual cycle could have influenced reward processing (Sacher et al., 2013; Bobzean et al., 2014; Becker and Chartoff, 2019).
General Conclusion and Implications
We observed that less ventral striatal activation during reward anticipation was related to subclinical emotional instability in females. The study suggests that emotional and non-emotional symptoms should be disentangled, concerning sex, in both non-clinical and clinical study groups. The use of a dimensional approach when quantifying psychopathology may be a step towards the development of more effective treatment paradigms.
Data Availability Statement
The datasets generated for this study are available on request to the corresponding author.
Ethics Statement
The studies involving human participants were reviewed and approved by the ethics committee in Stockholm (application number 2015/127-31/1, amendment number 2016/1711-32). The patients/participants provided their written informed consent to participate in this study.
Author Contributions
FB and PP were mainly responsible for the conception and design of the study. FB, CA and NW acquired and analyzed the data. FB, CA, NW, MI, EH and PP all contributed to interpreting the results and writing the manuscript. All authors have approved the submitted version.
Funding
PP was funded by grants from Karolinska Institutet (2-70/2014-97), ALF Medicine (20160039), Hjärnfonden, Marianne och Marcus Wallenbergs Stiftelse (MMW2014.0065), VR (2014-30186-113005-19) and Projektmedel för forskning och utveckling inom psykiatri, primärvård och geriatrik, PPG (20160038). FB was funded by CSTP from Karolinska Institutet.
Supplementary Material
The Supplementary Material for this article can be found online at: https://www.frontiersin.org/articles/10.3389/fnbeh.2020.00076/full#supplementary-material.
Conflict of Interest
The authors declare that the research was conducted in the absence of any commercial or financial relationships that could be construed as a potential conflict of interest.
References
Bayard, F., Nymberg Thunell, C., Abe, C., Almeida, R., Banaschewski, T., Barker, G., et al. (2018). Distinct brain structure and behavior related to ADHD and conduct disorder traits. Mol. Psychiatry doi: 10.1093/med/9780198739258.003.0020
Becker, J. B., and Chartoff, E. (2019). Sex differences in neural mechanisms mediating reward and addiction. Neuropsychopharmacology 44, 166–183. doi: 10.1038/s41386-018-0125-6
Berridge, K. C. (2018). Evolving concepts of emotion and motivation. Front. Psychol. 9:1647. doi: 10.3389/fpsyg.2018.01647
Bobzean, S. A., DeNobrega, A. K., and Perrotti, L. I. (2014). Sex differences in the neurobiology of drug addiction. Exp. Neurol. 259, 64–74. doi: 10.1016/j.expneurol.2014.01.022
Brown, T. E. (1996). Brown Attention-Deficit Disorder Scales (Manual). San Antonio, TX: The Psychological Corporation.
Carmona, S., Hoekzema, E., Ramos-Quiroga, J. A., Richarte, V., Canals, C., Bosch, R., et al. (2012). Response inhibition and reward anticipation in medication-naïve adults with attention-deficit/hyperactivity disorder: a within-subject case-control neuroimaging study. Hum. Brain Mapp. 33, 2350–2361. doi: 10.1002/hbm.21368
Castellanos, F. X., Sonuga-Barke, E. J., Milham, M. P., and Tannock, R. (2006). Characterizing cognition in ADHD: beyond executive dysfunction. Trends Cogn. Sci. Regul. Ed. 10, 117–123. doi: 10.1016/j.tics.2006.01.011
Costa Dias, T. G., Wilson, V. B., Bathula, D. R., Iyer, S. P., Mills, K. L., Thurlow, B. L., et al. (2013). Reward circuit connectivity relates to delay discounting in children with attention-deficit/hyperactivity disorder. Eur Neuropsychopharmacol 23, 33–45. doi: 10.1016/j.euroneuro.2012.10.015
Craig, A. D. (2002). How do you feel? Interoception: the sense of the physiological condition of the body. Nat. Rev. Neurosci. 3, 655–666. doi: 10.1038/nrn894
Craig, A. D. (2003). Interoception: the sense of the physiological condition of the body. Curr. Opin. Neurobiol. 13, 500–505. doi: 10.1016/s0959-4388(03)00090-4
Cuthbert, B. N., and Insel, T. R. (2013). Toward the future of psychiatric diagnosis: the seven pillars of RDoC. BMC Med. 11:126. doi: 10.1186/1741-7015-11-126
Daniel, R., and Pollmann, S. (2014). A universal role of the ventral striatum in reward-based learning: evidence from human studies. Neurobiol. Learn. Mem. 114, 90–100. doi: 10.1016/j.nlm.2014.05.002
Davies, W. (2014). Sex differences in attention Deficit Hyperactivity Disorder: candidate genetic and endocrine mechanisms. Front Neuroendocrinol 35, 331–346. doi: 10.1016/j.yfrne.2014.03.003
Dillon, D. G., Bogdan, R., Fagerness, J., Holmes, A. J., Perlis, R. H., and Pizzagalli, D. A. (2010). Variation in TREK1 gene linked to depression-resistant phenotype is associated with potentiated neural responses to rewards in humans. Hum. Brain Mapp. 31, 210–221. doi: 10.1002/hbm.20858
DSM-5. (2013). Diagnostic and Statistical Manual of Mental Disorders: DSM-5. Arlington, VA: American Psychiatric Association.
Edel, M. A., Enzi, B., Witthaus, H., Tegenthoff, M., Peters, S., Juckel, G., et al. (2013). Differential reward processing in subtypes of adult attention deficit hyperactivity disorder. J. Psychiatr. Res. 47, 350–356. doi: 10.1016/j.jpsychires.2012.09.026
Esteban, O., Markiewicz, C. J., Blair, R. W., Moodie, C. A., Isik, A. I., Erramuzpe, A., et al. (2019). fMRIPrep: a robust preprocessing pipeline for functional MRI. Nat. Methods 16, 111–116. doi: 10.1038/s41592-018-0235-4
Furukawa, E., Bado, P., Tripp, G., Mattos, P., Wickens, J. R., Bramati, I. E., et al. (2014). Abnormal striatal BOLD responses to reward anticipation and reward delivery in ADHD. PLoS One 9:e89129. doi: 10.1371/journal.pone.0089129
Gorgolewski, K. J., Auer, T., Calhoun, V. D., Craddock, R. C., Das, S., Duff, E. P., et al. (2016). The brain imaging data structure, a format for organizing and describing outputs of neuroimaging experiments. Sci. Data 3:160044. doi: 10.1038/sdata.2016.44
Hauser, T. U., Fiore, V. G., Moutoussis, M., and Dolan, R. J. (2016). Computational Psychiatry of ADHD: Neural Gain Impairments across Marrian Levels of Analysis. Trends Neurosci. 39, 63–73. doi: 10.1016/j.tins.2015.12.009
Hodgson, K., Hutchinson, A. D., and Denson, L. (2014). Nonpharmacological treatments for ADHD: a meta-analytic review. J. Atten. Disord. 18, 275–282. doi: 10.1177/1087054712444732
Insel, T., Cuthbert, B., Garvey, M., Heinssen, R., Pine, D. S., Quinn, K., et al. (2010). Research domain criteria (RDoC): toward a new classification framework for research on mental disorders. Am J. Psychiatry 167, 748–751. doi: 10.1176/appi.ajp.2010.09091379
Kable, J. W., and Glimcher, P. W. (2007). The neural correlates of subjective value during intertemporal choice. Nat. Neurosci. 10, 1625–1633. doi: 10.3410/f.1097059.553030
Kappel, V., Lorenz, R. C., Streifling, M., Renneberg, B., Lehmkuhl, U., Strohle, A., et al. (2015). Effect of brain structure and function on reward anticipation in children and adults with attention deficit hyperactivity disorder combined subtype. Soc. Cogn. Affect. Neurosci. 10, 945–951. doi: 10.1093/scan/nsu135
Katzman, M. A., Bilkey, T. S., Chokka, P. R., Fallu, A., and Klassen, L. J. (2017). Adult ADHD and comorbid disorders: clinical implications of a dimensional approach. BMC Psychiatry 17:302. doi: 10.1186/s12888-017-1463-3
Knutson, B., Adams, C. M., Fong, G. W., and Hommer, D. (2001a). Anticipation of increasing monetary reward selectively recruits nucleus accumbens. J Neurosci 21:RC159. doi: 10.1523/jneurosci.21-16-j0002.2001
Knutson, B., Fong, G. W., Adams, C. M., Varner, J. L., and Hommer, D. (2001b). Dissociation of reward anticipation and outcome with event-related fMRI. Neuroreport 12, 3683–3687. doi: 10.1097/00001756-200112040-00016
Kringelbach, M. L., and Rolls, E. T. (2004). The functional neuroanatomy of the human orbitofrontal cortex: evidence from neuroimaging and neuropsychology. Prog. Neurobiol. 72, 341–372. doi: 10.1016/j.pneurobio.2004.03.006
Moukhtarian, T. R., Mintah, R. S., Moran, P., and Asherson, P. (2018). Emotion dysregulation in attention-deficit/hyperactivity disorder and borderline personality disorder. Borderline Personal. Disord. Emot. Dysregul. 5:9. doi: 10.1186/s40479-018-0086-8
Mowlem, F. D., Rosenqvist, M. A., Martin, J., Lichtenstein, P., Asherson, P., and Larsson, H. (2018). Sex differences in predicting ADHD clinical diagnosis and pharmacological treatment. Eur. Child Adolesc. Psychiatry 28, 481–489. doi: 10.1007/s00787-018-1211-3
Namkung, H., Kim, S. H., and Sawa, A. (2017). The insula: an underestimated brain area in clinical neuroscience, psychiatry, and neurology. Trends Neurosci. 40, 200–207. doi: 10.1016/j.tins.2017.02.002
Naqvi, N. H., and Bechara, A. (2009). The hidden island of addiction: the insula. Trends Neurosci. 32, 56–67. doi: 10.1016/j.tins.2008.09.009
O’Doherty, J. P., Buchanan, T. W., Seymour, B., and Dolan, R. J. (2006). Predictive neural coding of reward preference involves dissociable responses in human ventral midbrain and ventral striatum. Neuron 49, 157–166. doi: 10.1016/j.neuron.2005.11.014
O’Doherty, J., Kringelbach, M. L., Rolls, E. T., Hornak, J., and Andrews, C. (2001). Abstract reward and punishment representations in the human orbitofrontal cortex. Nat. Neurosci. 4, 95–102. doi: 10.1038/82959
Oldham, S., Murawski, C., Fornito, A., Youssef, G., Yucel, M., and Lorenzetti, V. (2018). The anticipation and outcome phases of reward and loss processing: a neuroimaging meta-analysis of the monetary incentive delay task. Hum. Brain Mapp. 39, 3398–3418. doi: 10.1002/hbm.24184
Pessiglione, M., Seymour, B., Flandin, G., Dolan, R. J., and Frith, C. D. (2006). Dopamine-dependent prediction errors underpin reward-seeking behaviour in humans. Nature 442, 1042–1045. doi: 10.1038/nature05051
Petrovic, P., and Castellanos, F. X. (2016). Top-down dysregulation-from adhd to emotional instability. Front. Behav. Neurosci. 10:70. doi: 10.3389/fnbeh.2016.00070
Petrovic, P., Pleger, B., Seymour, B., Kloppel, S., De Martino, B., Critchley, H., et al. (2008). Blocking central opiate function modulates hedonic impact and anterior cingulate response to rewards and losses. J. Neurosci. 28, 10509–10516. doi: 10.3410/f.1124546.581753
Petrovic, P., Ekman, C. J., Klahr, J., Tigerstrom, L., Ryden, G., Johansson, A. G., et al. (2016). Significant grey matter changes in a region of the orbitofrontal cortex in healthy participants predicts emotional dysregulation. Soc. Cogn. Affect. Neurosci. 11, 1041–1049. doi: 10.1093/scan/nsv072
Pinheiro J, B. D., DebRoy, S., Sarkar, D., R Core Team (2019). nlme: Linear and Nonlinear Mixed Effects Models-R package version 3.1-147. Available online at: https://CRAN.R-project.org/package=nlme. Accessed 29 May 2019.
Poldrack, R. A. (2007). Region of interest analysis for fMRI. Soc. Cogn. Affect. Neurosci. 2, 67–70. doi: 10.1093/scan/nsm006
Poldrack, R. A., Mumford, J. A., and Nichols, T. E. (2011). Handbook of Functional MRI Data Analysis, New York, NY: Cambridge University Press.
R Core Team. (2015). RLanguage and Environment for Statistical Computing. R Foundation for Statistical Computing (Vienna, Austria). Available online at: http://www.R-project.org/. Accessed 29 May 2019.
Rubia, K. (2011). “Cool” inferior frontostriatal dysfunction in attention-deficit/hyperactivity disorder versus “hot” ventromedial orbitofrontal-limbic dysfunction in conduct disorder: a review. Biol. Psychiatry 69, e69–87. doi: 10.1016/j.biopsych.2010.09.023
Rucklidge, J. J. (2010). Gender differences in attention-deficit/hyperactivity disorder. Psychiatr. Clin. North Am. 33, 357–373. doi: 10.1016/j.psc.2010.01.006
Sacher, J., Okon-Singer, H., and Villringer, A. (2013). Evidence from neuroimaging for the role of the menstrual cycle in the interplay of emotion and cognition. Front. Hum. Neurosci. 7:374. doi: 10.3389/fnhum.2013.00374
Scheres, A., Milham, M. P., Knutson, B., and Castellanos, F. X. (2007). Ventral striatal hyporesponsiveness during reward anticipation in attention-deficit/hyperactivity disorder. Biol. Psychiatry 61, 720–724. doi: 10.1016/j.biopsych.2006.04.042
Schultz, W. (2016). Dopamine reward prediction error coding. Dialogues Clin. Neurosci. 18, 23–32. doi: 10.1111/psyp.12673
Schultz, W., Dayan, P., and Montague, P. R. (1997). A neural substrate of prediction and reward. Science 275, 1593–1599. doi: 10.1126/science.275.5306.1593
Shaw, P., Stringaris, A., Nigg, J., and Leibenluft, E. (2014). Emotion dysregulation in attention deficit hyperactivity disorder. Am J Psychiatry 171, 276–293. doi: 10.1126/science.275.5306.1593
Simon, V., Czobor, P., Balint, S., Meszaros, A., and Bitter, I. (2009). Prevalence and correlates of adult attention-deficit hyperactivity disorder: meta-analysis. Br. J. Psychiatry 194, 204–211. doi: 10.1192/bjp.bp.107.048827
Sjöwall, D., Roth, L., Lindqvist, S., and Thorell, L. B. (2013). Multiple deficits in ADHD: executive dysfunction, delay aversion, reaction time variability, and emotional deficits. J. Child Psychol. Psychiatry 54, 619–627. doi: 10.1111/jcpp.12006
Skirrow, C., and Asherson, P. (2013). Emotional lability, comorbidity and impairment in adults with attention-deficit hyperactivity disorder. J. Affect. Disord. 147, 80–86. doi: 10.1016/j.jad.2012.10.011
Spencer, T. J., Biederman, J., and Mick, E. (2007). Attention-deficit/hyperactivity disorder: diagnosis, lifespan, comorbidities, and neurobiology. Ambul. Pediatr. 7, 73–81. doi: 10.1016/j.ambp.2006.07.006
Stark, R., Bauer, E., Merz, C. J., Zimmermann, M., Reuter, M., Plichta, M. M., et al. (2011). ADHD related behaviors are associated with brain activation in the reward system. Neuropsychologia 49, 426–434. doi: 10.1016/j.neuropsychologia.2010.12.012
Strohle, A., Stoy, M., Wrase, J., Schwarzer, S., Schlagenhauf, F., Huss, M., et al. (2008). Reward anticipation and outcomes in adult males with attention-deficit/hyperactivity disorder. NeuroImage 39, 966–972. doi: 10.1016/j.neuroimage.2007.09.044
Thorell, L. B. (2007). Do delay aversion and executive function deficits make distinct contributions to the functional impact of ADHD symptoms? A study of early academic skill deficits. J. Child Psychol. Psychiatry 48, 1061–1070. doi: 10.1111/j.1469-7610.2007.01777.x
Trent, S., and Davies, W. (2012). The influence of sex-linked genetic mechanisms on attention and impulsivity. Biol. Psychol. 89, 1–13. doi: 10.1016/j.biopsycho.2011.09.011
van Hulst, B. M., de Zeeuw, P., Bos, D. J., Rijks, Y., Neggers, S. F., and Durston, S. (2017). Children with ADHD symptoms show decreased activity in ventral striatum during the anticipation of reward, irrespective of ADHD diagnosis. J. Child Psychol. Psychiatry 58, 206–214. doi: 10.1111/jcpp.12643
Veroude, K., von Rhein, D., Chauvin, R. J., van Dongen, E. V., Mennes, M. J., Franke, B., et al. (2016). The link between callous-unemotional traits and neural mechanisms of reward processing: an fMRI study. Psychiatry Res. Neuroimaging 255, 75–80. doi: 10.1016/j.pscychresns.2016.08.005
White, S. F., Clanton, R., Brislin, S. J., Meffert, H., Hwang, S., Sinclair, S., et al. (2014). Reward: empirical contribution. Temporal discounting and conduct disorder in adolescents. J. Pers. Disord. 28, 5–18. doi: 10.1521/pedi.2014.28.1.5
Whitton, A. E., Treadway, M. T., and Pizzagalli, D. A. (2015). Reward processing dysfunction in major depression, bipolar disorder and schizophrenia. Curr Opin Psychiatry 28, 7–12. doi: 10.1097/yco.0000000000000122
Keywords: emotional instability, emotional dysregulation, ADHD, reward anticipation, functional MRI, ventral striatum
Citation: Bayard F, Abé C, Wrobel N, Ingvar M, Henje E and Petrovic P (2020) Emotional Instability Relates to Ventral Striatum Activity During Reward Anticipation in Females. Front. Behav. Neurosci. 14:76. doi: 10.3389/fnbeh.2020.00076
Received: 26 January 2020; Accepted: 28 April 2020;
Published: 29 May 2020.
Edited by:
Walter Adriani, Istituto Superiore di Sanità (ISS), ItalyReviewed by:
Claire Jennifer Foldi, Monash University, AustraliaThomas J. Ross, National Institute on Drug Abuse (NIDA), United States
Copyright © 2020 Bayard, Abé, Wrobel, Ingvar, Henje and Petrovic. This is an open-access article distributed under the terms of the Creative Commons Attribution License (CC BY). The use, distribution or reproduction in other forums is permitted, provided the original author(s) and the copyright owner(s) are credited and that the original publication in this journal is cited, in accordance with accepted academic practice. No use, distribution or reproduction is permitted which does not comply with these terms.
*Correspondence: Predrag Petrovic, predrag.petrovic@ki.se