Large-scale coupling of prefrontal activity patterns as a mechanism for cognitive control in health and disease: evidence from rodent models
- 1Instituto de Fisiología, Facultad de Ciencias, Universidad de Valparaíso, Valparaíso, Chile
- 2Programa de Doctorado en Ciencias Mención en Neurociencia, Facultad de Ciencias, Universidad de Valparaíso, Valparaíso, Chile
Cognitive control of behavior is crucial for well-being, as allows subject to adapt to changing environments in a goal-directed way. Changes in cognitive control of behavior is observed during cognitive decline in elderly and in pathological mental conditions. Therefore, the recovery of cognitive control may provide a reliable preventive and therapeutic strategy. However, its neural basis is not completely understood. Cognitive control is supported by the prefrontal cortex, structure that integrates relevant information for the appropriate organization of behavior. At neurophysiological level, it is suggested that cognitive control is supported by local and large-scale synchronization of oscillatory activity patterns and neural spiking activity between the prefrontal cortex and distributed neural networks. In this review, we focus mainly on rodent models approaching the neuronal origin of these prefrontal patterns, and the cognitive and behavioral relevance of its coordination with distributed brain systems. We also examine the relationship between cognitive control and neural activity patterns in the prefrontal cortex, and its role in normal cognitive decline and pathological mental conditions. Finally, based on these body of evidence, we propose a common mechanism that may underlie the impaired cognitive control of behavior.
1 The prefrontal cortex and cognitive control of behavior
1.1 Cognitive control of behavior
Animals are immersed in complex and challenging environments. To ensure its survivance and wellbeing, they are able to implement a wide repertoire of adaptive behavioral responses. Some challenges can be solved by the implementation of rapid and simple stimulus-response behaviors, as for example, escape from a predator, or finding a shelter during a sudden natural disaster. Since these behavioral responses are automatically implemented once the stimulus is detected, they allow immediate and fast adaptation (Graybiel, 2008; Buzsáki et al., 2014). However, given that are triggered by particular stimulus, these responses are rigid, stereotyped and lack of voluntarily control, which makes these cognitive operations restricted to be extrapolated to other surrounding events (Buzsáki et al., 2014).
On the other hand, some challenges are directed to the obtention of non-immediate goals, which cannot be successfully solved by stimulus-response behavior (Engel et al., 2001). Of special relevance are those in which current circumstances are new, unknown, or under constant change, and therefore, there is a significant possibility that expectations cannot be accomplished. Under these circumstances, animals require to implement more sophisticated cognitive processes in which acquired information and behavioral responses are constantly updated and accommodated according to current internal (expectations) and external (environmental) conditions. This adaptive guidance and organization of behavioral responses according to current and prospective circumstances in a goal-directed manner is known as cognitive control of behavior (Miller, 2000). It has been postulated that impairment of cognitive control is the core of several normal and pathological mental declines, which is manifested as the reduced ability to implement goal-directed adaptive behavioral responses (Diamond, 2013). Therefore, the understanding of the neurophysiological bases of cognitive control is tremendously relevant not only for the treatment of recovery of mental health, but also as a preventive strategy to ensure and promote well-being. In this article, we review the neurophysiological mechanism that allows the implementation of cognitive control in rodent models to integrate this evidence with findings in normal and pathological conditions observed in humans.
Cognitive control is directed toward the achievement of goals. Therefore, it is voluntarily implemented and self-generated, as it can be implemented without relying on external cues. These goals are commonly based on expectations derived from previous experiences (Miller, 2000; Engel et al., 2001; Diamond, 2013; Buzsáki et al., 2014). Hence, it requires the generation of internal models of environmental conditions extracted from commonalities found across previous experiences (Schlichting and Preston, 2015). Optimal behavioral outcomes are predicted by contrasting and updating the stored internal model (i.e., memory) with the current circumstances, by which plans and strategies emerges from covert internal computations and heuristic processes oriented to goal achievement (Redish, 2016). Thus, even when subjects face novel and ambiguous conditions, successful behavioral responses can be implemented through the interpolation or extrapolation of past and present environmental patterns. As a result, cognitive control is prospective, probabilistic, and generalized (Buzsáki et al., 2014). Given that environmental conditions are far from being stable over multiple temporal scales, cognitive control requires the transient integration of different levels of information. This allows continuous updating of covert computations and behavioral responses (Helfrich and Knight, 2016). This feature allows animals to respond in robust and flexible ways to the continuously fluctuating environment in a goal-directed manner (Buzsáki et al., 2014). This ability, known as behavioral flexibility (Diamond, 2013) is considered one of the hallmarks of cognitive control (Mikhalevich et al., 2017). Loss of behavioral flexibility may lead to profound consequences, manifested as the execution of perseverative and maladaptive behavioral responses, a feature observed in several situations that compromise well-being and mental health, including cognitive decline during aging (Richard’s et al., 2021), mood disorders (Kashdan and Rottenberg, 2010) and schizophrenia (Waltz, 2017), among others (Diamond, 2013; Uddin, 2021).
Cognitive control requires the coordination of several simultaneous neural processes. For example, it involves the integration (binding), interaction and “on-line” temporal maintenance of multiple levels and modalities of information. Computational processes as the evaluation and weighing the relevance of these modalities of information, its comparison with similar occurrences in the past, and the generation of heuristics and prospective responses are simultaneously implemented (Buzsáki et al., 2014). These processes are supported by the synchronization and coordination of several discrete cognitive sub-processes known as “executive functions,” such as focused attention (selecting relevant information), working memory (temporal maintenance of relevant information), recall of experiences (memory recall), inhibitory control, valence interpretation, establishment of stimulus-response associations, goal-setting, strategy implementation, error monitoring, and decision-making (Diamond, 2013). Thus, cognitive control depends on the simultaneous and dynamical cooperation among multiple parallel computations and sources of information.
Several behavioral paradigms in rodent models have been designed to evaluate neural operations related to cognitive control. For example, focused attention and impulse control is often evaluated by 5-choice serial reaction time test (Asinof and Paine, 2014), working memory and decision making are evaluated by delay-match to sample test (Dudchenko, 2004), and inhibitory control can be evaluated by extinction of conditioned-fear (Chang et al., 2009). However, these tests allow the evaluation of a single and discrete cognitive function and are normally based on a particular sensory modality, restricting the evaluation of multiple parallel processes, as occurs during cognitive control. Also, several of these tests are considered of low ethological validity, as they are far from real life circumstances (Shemesh and Chen, 2023). Thus, despite their undeniable value, these tests have remarkable limitations to study neural operations associated to cognitive control.
On the other hand, behavioral paradigm involving goal-directed spatial memory, as the Morris-water maze test, the Barnes maze test, cheese-board test, and radial-arm maze test (Redish, 2016) offers several advantages to study neural processes supporting cognitive control. These tasks are based on learning and memorizing the spatial locations of relevant places in the environment (Ito, 2018). Spatial memory tests are ethologically valid, as it reproduces challenges commonly faced in the natural environments of rodent and primates, including humans (d’Isa and Gerlai, 2022). Given that spatial memory is not acquired in a single trial but it requires the repeated exploration of relatively fixed environment, they allow to evaluate memory generalization and strategy progression along the learning process (Ruediger et al., 2012; Richards et al., 2014). To accomplish the task, animals require to integrate several sets of information as multisensory (visual, olfactory, vestibular), spatial-temporal, emotional valence (aversive and appetitive), and self-motion. Additionally, spatial learning process requires the implementation of discrete executive functions, as extraction of rules and contingences, online maintenance of relevant information (working memory), and decision-making, which can be easily evaluated on spatial memory tasks. Thus, several parallel perceptual and cognitive processes can be evaluated in goal-directed spatial memory tests. Moreover, through a detailed behavioral analysis, they also allow the evaluation of more sophisticated cognitive operations, such as strategy switching (Rich and Shapiro, 2009), vicarious behavior (Redish, 2016), planning (Dragoi and Tonegawa, 2011), path integration (Collett and Graham, 2004), or even imagination (Lai et al., 2023) and deliberation (Blumenthal et al., 2011). Importantly, with slight modifications, it is possible to evaluate behavioral flexibility (Hamilton and Brigman, 2015). For example, in spatial set-shifting tasks, the rule is changed (i.e., navigate using egocentric cues instead of allocentric cues). Similarly, in spatial reversal-learning tasks, the spatial position of the goal is changed after several training sessions, allowing the evaluation of goal-directed adjustment of behavioral. And finally, the neural systems involved in goal-directed spatial memory are relatively well known (Buzsáki and Moser, 2013). Therefore, spatial memory testing in rodent models, though well-designed paradigms, together with a detailed behavioral analysis, is an appropriate behavioral tool for the study of neural mechanisms involved in cognitive control (Pezzulo et al., 2014).
1.2 The role of the prefrontal cortex in cognitive control of behavior
The prefrontal cortex (PFC) is the association cortex localized in the frontal lobe of mammals (Carlén, 2017). Lesion and functional studies demonstrate the relevance of the mPFC in several cognitive operations required for goal-directed adaptation, which has led to the proposal that the main and single prefrontal function is “to structure the present to serve the future” (Fuster, 2001, 2008). Therefore, the PFC is considered the main structure supporting cognitive control of behavior (Miller, 2000).
Early studies defined the PFC as the area of the frontal pole that do not evokes motor responses to electrical stimulation (Uylings et al., 2003). In humans, the PFC constitutes a 30% of the entire cortical area. It can be subdivided following cytoarchitectonic criteria into the dorsolateral PFC, ventrolateral PFC, rostral parts of the orbitofrontal PFC and frontal pole. Other regions, such as the caudal orbitofrontal PFC, the anterior cingulate cortex and the ventromedial PFC are also included (Haber et al., 2022). Although rodents do not possess anatomical features of the primate PFC (Carlén, 2017), the rodent medial-PFC (mPFC) subserves a range of cognitive and behavioral processes homologous to those mediated by the primate PFC (Uylings et al., 2003; Carlén, 2017). However, the rodent mPFC has been controversial to define (Carlén, 2017; Laubach et al., 2018). Initially, the rodent mPFC was described as prefrontal areas connected with the mediodorsal nucleus (MD) of the thalamus (Rose and Woolsey, 1948). Later, the homology between the PFC from primates and rodents was established using several criteria, as the cytoarchitecture (presence of granular cortex in the frontal pole), the pattern of specific connections (reciprocal connection with MD), the functional behavioral and electrophysiological properties (similar impairments to prefrontal lesions and comparable activity patterns, as the presence of “delay cells”) and the embryological development (late ontogenetic development) (Uylings et al., 2003). Most of these features are shared between the primate PFC and rodent mPFC. However, taking into account that rodent mPFC is agranular (layer IV is not present), the MD also projects to areas non-related to rodent mPFC (Donoghue and Wise, 1982) and the differences of spatial arrangement between rodent and primate PFC (the rodent mPFC lies next to the allocortex) has led to the view that the primate and rodent PFC are not homologues, but these areas emerged differentially during evolution to accomplish class-common behavioral need, (i.e., the cognitive control of behavior) (Carlén, 2017; Hanganu-Opatz et al., 2023). Also, through comparative studies of gene expression patterns, it has evidenced differences between homologous cortical areas in humans and rodents (Bernard et al., 2012; Zeng et al., 2012). However, recent data based on clustering of internal microcircuitry (Harris et al., 2019) and large-scale hierarchical gradients (Fulcher et al., 2019) show that the rodent and primate PFC have similar laminar-gene expression, cell density and local and large-scale connectivity. Indeed, it was found a high conserved expression pattern of orthologous genes between the human and mouse PFC (Chen et al., 2016). Thus, despite this issue is a current matter of discussion, these data support the idea of “functional homologues” between rodent and primate PFC.
From the behavioral perspective, early studies showed that prefrontal lesions in humans led to the inability to override prepotent responses, which manifested as an impairment in the organization of behavior (Bechara et al., 1994; Damasio, 2006). Detailed behavioral analysis have revealed that prefrontal lesions in humans led to impairments in several executive functions that support cognitive control, as working memory, setting, sustained attention, inference control, decision making, inhibitory control, planning, and strategy implementation (Milner, 1963; Eslinger and Damasio, 1985; Chao and Knight, 1995; Bechara et al., 1998; Burgess, 2000). Importantly, studies developed by Milner (1965) showed that prefrontal lesioned patients showed deficits in spatial mazes, which appeared to be non-spatial in nature but had deficits in the correct strategy to solve the mazes, showing perseverative and impulsive behaviors. Behavioral flexibility is one of the strongest cognitive functions impaired by prefrontal lesions (Wegener and Stamm, 1966). This idea is supported by functional neuroimaging studies in humans showing that behavioral flexibility is associated with the activation of the PFC (Remijnse et al., 2005; Boehme et al., 2017; Uddin, 2021). An extensive body of evidence shows that lesions in the rodent mPFC impairs several executive functions similarly as lesions of human PFC. For example, lesions on the rodent mPFC produced deficiencies in working memory (Ragozzino and Kesner, 2001), focused attention (Kahn et al., 2012), decision-making (Croxson et al., 2014), strategy switching (de Bruin et al., 2001; Floresco et al., 2009) and inhibitory control (Brockett et al., 2022). Evidence from spatial memory tasks has shown that the mPFC supports strategy progression during spatial learning (de Bruin et al., 1997). Notably, the mPFC seems to be required for memory generalization in spatial memory tasks (Richards et al., 2014). Similarly as humans, lesions of the rodent mPFC strongly impair behavioral flexibility [extensive review in Hamilton and Brigman (2015)]. This body of evidence supports the idea of the rodent mPFC as a “functional homologue” to the human PFC.
The rodent mPFC has been subdivided into several areas following cytoarchitectonic criteria. However, different delineations and nomenclatures have been established over the years (Le Merre et al., 2021). The most accepted delineation subdivides the mPFC into three main sections: the infralimbic mPFC (IL), the prelimbic mPFC (PL), and the anterior cingulate cortex (ACC) (Laubach et al., 2018). Some authors include the orbitofrontal cortex (OFC) as part of the rodent mPFC, whereas the ACC is sometimes excluded from the prefrontal criteria (Carlén, 2017; Le Merre et al., 2021). Furthermore, some authors include areas of the dorsal portion of the frontal poles, as the secondary motor cortex (M2), also known as the frontal orienting field (FOF), second frontal area (Fr2), or medial agranular cortex (AGm) as part of the mPFC (Barthas and Kwan, 2017). This region receives afferents from the MD; however, electrical stimulation evokes motor response (Donoghue and Wise, 1982). These discrepancies show the difficulty of demarcate the mPFC in rodents (Carlén, 2017). Thus, the PL and IL are considered the “core” of the rodent mPFC.
Functional differences have been found between these prefrontal subdivisions: for example, during fear conditioning, the PL is involved in the expression of conditioned fear, whereas the IL is required for its extinction (Sierra-Mercado et al., 2011). This has led to a dorsal-to-ventral parcellation of the mPFC, in which the dorsal portion (ACC, PL) is associated with limbic and cognitive operations, whereas the ventral portion (the IL) is associated with visceral and autonomic functions (Vertes, 2004; Peters et al., 2009). However, neuronal firing in the PL and IL seems to represent similar behavioral elements in spatial tasks (Baeg et al., 2003; Hok et al., 2005; Rich and Shapiro, 2009), suggesting similar computing properties between these areas. Indeed, current evidence has challenged this parcellation of the mPFC. For example, diverse prefrontal neuronal populations with differential and opposed representational features coexist in the same prefrontal region (Ye et al., 2016). Analysis of wiring and molecular properties did not found differences between the prefrontal subdivisions (Ye et al., 2016; Ortiz et al., 2020), and behavioral evidence non-related to fear conditioning and extinction suggests functional similarities between the PL and IL (Riaz et al., 2019). Further, using optogenetic tools, it has been shown that activation of PL enhanced fear extinction, whereas inactivation of IL has no effect on extinction, challenging the classical roles of PL and IL (Do-Monte et al., 2015; Marek et al., 2018). Thus, considering the dense reciprocal connectivity between the PL and IL (Hoover and Vertes, 2007; van Aerde et al., 2008), it is possible that these structures work together as a single and unified processing system (Le Merre et al., 2021).
The rodent mPFC is composed by excitatory pyramidal neurons (PN; 80–90% of the total population) positioned in cortical layers II/III and V/VI (Riga et al., 2014) and GABAergic inhibitory neurons (IN; 10–20% of the total population) subdivided into different neuronal sub-types distributed across all cortical layers (Kawaguchi and Kubota, 1997). Importantly, the rodent mPFC is agranular, lacking the layer IV (Uylings et al., 2003). While PN are the main target of afferents from distributed neural systems and constitutes the output from the mPFC to other cortical and subcortical structures (Elston, 2003), IN synapse predominantly locally with PN [but not exclusively, see (Cho et al., 2023)], thus controlling and synchronizing the input and outputs of the prefrontal network (Riga et al., 2014). Among PN, intratelencephalic neurons (IT, neurons projecting to other cortical areas) are distributed between layer II to VI, pyramidal tract PN (PT, neurons projecting to subcortical nucleus) are located in layer V, and corticothalamic PN (CT, which project to thalamus) are located in layer V and IV (Anastasiades and Carter, 2021). Despite the local circuitry of the mPFC has not been studied in detail, it has been suggested that it shares an organization similar to other frontal cortices (Anastasiades and Carter, 2021). In this local connectivity, PN of layer II/III send descending projections to PN in layer V, which also send ascendent projections to layer II/III. Lateral projections are particularly strong in the mPFC, as robust connection exist between PN of layer II/III and between PN of layer V (Anastasiades and Carter, 2021). Indeed, the mPFC is the cortical region of the highest proportion of feedback projections (Le Merre et al., 2021). This high internal excitatory connectivity may be relevant for local neural operations performed in the mPFC. On the other hand, GABAergic IN are mainly subdivided in parvalbumin (PV) and somatostatin (SOM) expressing neurons (Kawaguchi and Kubota, 1997). PV cells synapse preferentially at the soma and axons of PN, contributing to feedforward inhibition that controls signal transmission, whereas SOM cells inhibits the dendrites of PN, providing feedback inhibition (Anastasiades and Carter, 2021). Importantly, cortical and subcortical areas projecting to the mPFC also synapse local IN, which are activated before projecting neurons (Anastasiades et al., 2018). Thus, considering that IN are relevant for the synchronization of neural populations and the emergence of neural activity patterns (see below), the feedback and feedforward inhibition triggered by local and long-range activity may support complex network dynamics in the mPFC.
The mPFC is structurally positioned between the perception and execution of actions, allowing the integration of perceptual information about the current context required for the execution of appropriate behavioral responses (Fuster, 2001). The mPFC receives projections from structures processing sensory, motivational, contextual, spatial, temporal, and internal information necessary to update internal representations (Fuster, 2001; Euston et al., 2012). Indeed, among cortical regions, the mPFC receive projections from the largest number of brain areas (Le Merre et al., 2021). Simultaneously, the mPFC projects to distributed associative, sensory, motor, neuromodulatory, and visceral brain systems to generate and modulate behavioral responses (Vertes, 2004; Gabbott et al., 2005; Euston et al., 2012). One of the most relevant structures innervating the mPFC is MD of the thalamus, which has been considered as a definitory feature of the mPFC (Vertes et al., 2007; Delevich et al., 2015; Ketz et al., 2015; Bolkan et al., 2017; Shepherd and Yamawaki, 2021). Afferences from the MD project to superficial layers of the mPFC (Anastasiades and Carter, 2021). As the MD does not receive sensory or motor inputs, it is considered a high order nucleus. Instead, the MD receives inputs from the mPFC, thalamocortical neurons from other associative cortices, and several subcortical structures (Mitchell and Chakraborty, 2013; Ketz et al., 2015). Afferents from the mPFC emerges exclusively from layer VI of ACC, PL and IL (Gabbott et al., 2005). Lesion or inhibition of the MD leads to deficits similar to prefrontal lesions (Mitchell and Chakraborty, 2013; Parnaudeau et al., 2013), suggesting a close association between the MD and cognitive functions governed by the PFC.
The mPFC also receives dense projections from other associative cortical structures. One of the most studied connections of the mPFC is with the hippocampus (HPC) (Eichenbaum, 2017), structure involved in the representation of spatial and temporal sequences (Buzsáki and Tingley, 2018). Prefrontal-hippocampal interaction is relevant for object- and place-recognition memory (Chao et al., 2020, 2022), goal-directed spatial navigation and memory (Ito, 2018) and long-term consolidation of declarative memories (Girardeau and Zugaro, 2011; Euston et al., 2012). The HPC is subdivided into dorsal-HPC (dHPC) and ventral-HPC (vHPC). The dHPC is associated with cognitive operations, while the vHPC is mostly related with emotional and contextual-spatial processing (Lee et al., 2017). The vHPC is directly connected with the mPFC, in which excitatory neurons from the CA1 and the subiculum projects to the deep layers (V and VI) of the IL and PL (Hoover and Vertes, 2007; Anastasiades and Carter, 2021). Through this pathway, the vHPC may send contextual information to the mPFC (Cohen and Meyer, 2020). On the other hand, the dHPC is bidirectionally connected with the mPFC through the nucleus reuniens (RE) of the thalamus (Baker and Bird, 2002; Vertes, 2004; Joyce et al., 2022). The RE display bidirectional connectivity with the mPFC and the dHPC (Vertes et al., 2007). Simultaneously, the RE is the major thalamic input to the HPC, which distributes densely to CA1, the ventral subiculum, and entorhinal cortex (EC) (Jay and Witter, 1991; Vertes et al., 2007). This connectivity of the mPFC with the dHPC has been associated with the integration of spatial-temporal information (Eichenbaum, 2017).
The EC, cortical area that constitutes the hippocampal-entorhinal loop associated with spatial cognition and memory (Kitamura et al., 2015), send direct projections to the ACC and PL (Hoover and Vertes, 2007). These projections emerge from PN located in layer V and VI of the EC, innervating the superficial layers of the mPFC (Insausti et al., 1997). Given that the main output from the HPC is the EC, afferences from the EC may inform the mPFC about spatial-temporal features. On the other hand, the PL and IL project directly to the EC (Vertes, 2004). The posterior parietal cortex (PPC), area involved in the active guidance of the body through the visual space (Whitlock et al., 2008), projects densely to the ACC, but much less to the PL and IL (Kolb and Walkey, 1987; Vertes, 2004). This pathway may integrate information relative to self-motion into the mPFC (Whitlock et al., 2008). Direct projections from the ACC, PL and IL to PPC are scarce, although strong projection to the PPC emerges from the OFC (Olsen et al., 2019). The retrosplenial cortex (RSC) is another associative cortical structure connected with the mPFC. Although the specific function of the RSC has been difficult to clarify, it seems involved in the cross-modal integration during spatial navigation processing (Vann et al., 2009). The RSC is reciprocally connected with the ACC and PL (Jones et al., 2005; Hoover and Vertes, 2007). Interestingly, the RSC is also reciprocally connected with the EC and PPC, and receives unidirectional projections from the dHPC (Mitchell et al., 2018). Therefore, the RSC may participate in the integration of spatial and action-based information into the mPFC. The mPFC also receives restricted projections from the M2 cortex (Hoover and Vertes, 2007), area involved in the transformation of sensory cues into motor actions (Olson et al., 2020). The M2 is one main outputs from the mPFC, in which prefrontal afferences to M2 emerges from PL and ACC (Bedwell et al., 2014). Thus, M2 may participate in the organization of goal-directed motor actions.
Much of the cognitive control implemented by the mPFC is mediated through projections to several subcortical structures. Emotional control, for example, seems to be mediated by the connection between the mPFC with the amygdala (LeDoux, 2000; Marek et al., 2013). The amygdala is subdivided into the basal (BA), lateral (LA), and central nucleus (CeA) (LeDoux, 2000). The lateral and basal amygdala conform the basolateral complex of the amygdala (BLA), which is strongly innervated by the mPFC; these projections emerge preferably from layer II and V from ACC, PL and IL (Vertes, 2004; Gabbott et al., 2005). These prefrontal afferents to the amygdala may have a key role in goal-directed responses to threats (Alexandra Kredlow et al., 2022). Importantly, the BLA also send excitatory projections to the layer V of the PL and IL (Orozco-Cabal et al., 2006; Hoover and Vertes, 2007), whereas the CeA, considered the output of the amygdala, sends GABAergic afferent to the mPFC (Seo et al., 2016). These projection may integrate emotional (especially aversive) information into the mPFC. The nucleus accumbens (NAc), part of the mesolimbic dopaminergic reward circuitry (Floresco, 2015), is also strongly innervated by the mPFC (Vertes, 2004; Gabbott et al., 2005). Excitatory afferents to NAc emerges bilaterally from layer II, V and VI from PL and IL (Gabbott et al., 2005). Projections from the NAc to the mPFC seems to be absent (Hoover and Vertes, 2007). This mPFC-NAc circuitry may be relevant for guiding of behaviors according to rewards. The mPFC also projects to the lateral hypothalamus (LH), structure involved in the control of food intake and motivated behaviors (Stuber and Wise, 2016). The LH receive strong projections from layers II, III, V, and V from the ACC, PL and IL (Gabbott et al., 2005). This circuit participates in the cognitive control of food intake (Azevedo et al., 2022).
The mPFC also receive projections from several subcortical neuromodulatory nucleus. The dorsal raphe nucleus (DRN), the pedunculopontine tegmental nucleus (PPT), the locus coeruleus (LC), ventral-tegmental area (VTA) and the basal forebrain strongly project to the PL and IL (Hoover and Vertes, 2007; Henny and Jones, 2008). This monoaminergic and cholinergic innervation may modulate prefrontal network dynamics (Cools and Arnsten, 2022). Also the mPFC project to several of these nucleus. The DRN receive projections from layer V of the ACC, PL and IL (Gabbott et al., 2005), and the VTA receive projections from layer V of PL and IL (Gabbott et al., 2005). The mPFC also projects to the locus coeruleus (Cardenas et al., 2021) and the basal forebrain (Gaykema et al., 1991). This prefrontal innervation of monoaminergic and cholinergic nucleus may have a relevant role in global state of arousal (Mashour et al., 2022).
2 Neurophysiological basis for prefrontal cognitive control of behavior
2.1 Cognitive relevant features are represented by synchronized neuronal firing in the mPFC
How does the mPFC participate in the implementation of cognitive control? The most accepted hypothesis postulates that brain operations are supported by the transient, discrete, and strongly interconnected active ensembles of neurons, known as “neuronal assembly” (NA) (Hebb, 1949). This hypothesis proposes that NAs are made up of a relatively small set of distributed neurons that, by synchronized firing, encode relevant behavioral parameters (Buzsáki, 2010). Experimentally, a NA is a task-related synchronized overlapping firing of multiple single-neurons (Sakurai, 1999). Given that detection of NAs requires highly invasive intracerebral multielectrode recordings (Buzsáki, 2004) or fluorescent cell-imaging in behaving subjects (Carrillo-Reid et al., 2017), most studies relating NAs to cognitive features have been performed in animal models.
To date, the best characterized NA are the “place cells” in the HPC that encode the spatial position of the subject in the environment (Moser et al., 2008). Considering the role of the mPFC, NAs in this structure would represent multiple sets of discrete information reflecting cognitive-relevant elements, such as maintenance of information, strategies, decisions, and goals (Sakurai et al., 2013). Consequently, it has been found that prefrontal NAs encode a wide range of behavioral requirements for the task (Jung et al., 1998) including prospective goal choices (Baeg et al., 2003; Fujisawa et al., 2008; Benchenane et al., 2010), spatial goals (Hok et al., 2005) or strategy selection and switching (Rich and Shapiro, 2009; Powell and Redish, 2016). These NAs in the mPFC have been shown to be highly dynamical, as they are progressively formed in parallel with learning (Baeg et al., 2007; Benchenane et al., 2010); are transiently activated, reflecting the emergent dynamics of cognitive operations (Fujisawa et al., 2008), and once formed, can be activated at remote temporal scales, representing the long-term memory of the task (Baeg et al., 2007). Importantly, given their dynamic nature, prefrontal NAs support cognitive flexibility through abrupt changes in firing patterns related to the accommodation of new behavioral strategies as animals detect variations in their environment (Rich and Shapiro, 2009; Powell and Redish, 2016; Malagon-Vina et al., 2018). Thus, the formation, activation, and dynamic modulation of synchronized firing patterns of neuronal populations in the mPFC seem to be relevant for the implementation of cognitive control.
2.2 Oscillatory activity in the mPFC
Together with synchronized neuronal spiking, oscillatory patterns in the mPFC seems to support cognitive operations. Brain oscillations refer to rhythmic electrical activity detected as periodic fluctuations of the extracellular electric potential (i.e., local field potential, LFP) (Buzsáki et al., 2012; Yener and Başar, 2013). It reflects the non-linear summation of post-synaptic potentials that emerge from the synchronized interplay between excitatory and inhibitory synaptic transmembrane ion currents of the neural population (Pesaran et al., 2018). As brain oscillations are intrinsically periodic, they are classified into different bandwidths (between 0.5 and 200 Hz) that are related with different brain states (Buzsáki and Draguhn, 2004; Buzsáki and Watson, 2012). The amplitude and frequency of oscillations depends on the identity and neural composition of the neural network, the sum of the synchronized activity of these neurons, and the neuronal morphology and disposition of neurons in the cerebral space (Buzsáki and Draguhn, 2004; Maling and McIntyre, 2016). Thus, different oscillatory frequencies reflect the synchronized recruitment of different levels of neural populations, in which low-frequency oscillations are the manifestation of the synchronized activity of large-scale neural populations, whereas high-frequency oscillations represent the coordinated activity of local neural populations (Buzsáki and Draguhn, 2004; Rosanova et al., 2009).
Theta oscillation (6–12 Hz) is the most prominent low-frequency oscillatory activity observed in the mPFC. It is evident during locomotion and high cognitive demands (Colgin, 2011; Gordon, 2011). It is classically proposed that neocortical theta is driven by the HPC, which is thought to be sustained by inputs from the medial septum (cholinergic and GABAergic) and entorhinal cortex (GABAergic) (Buzsáki, 2002). However, hippocampal-independent theta has also been shown in the neocortex (Benchenane et al., 2011) although the exact circuit involved in its origin is still matter of discussion (Mitchell et al., 1982; Jeffery et al., 1995; Buzsáki, 2002; Schlesiger et al., 2015; Headley and Paré, 2017). During the last decade, a 4-Hz low-frequency oscillation has been described in the mPFC (Fujisawa and Buzsáki, 2011; Biskamp et al., 2017; Karalis and Sirota, 2022). Although the cellular origin of this rhythm is still unknown, it has been shown that it is locally generated in cortical networks by respiratory influence through the afferents from olfactory bulb (Folschweiller and Sauer, 2021). Given that this rhythm is also detected in several structures connected with the mPFC, as the HPC (Yanovsky et al., 2014), PPC (Jung et al., 2022), VTA (Fujisawa and Buzsáki, 2011), BLA (Karalis et al., 2016) and striatum (Oberto et al., 2022), this rhythm may synchronize distant structures with the mPFC. This 4-Hz oscillation is particularly evident in moments of immobility, suggesting that it may supports distributed coordination of neural networks when locomotion is absent (Biskamp et al., 2017). Evidence suggests that 4-Hz may be relevant for cognitive functions (Fujisawa and Buzsáki, 2011; Karalis et al., 2016; Bagur et al., 2021; Oberto et al., 2022). On the other hand, beta (15–30 Hz) and gamma (30–100 Hz) oscillations are the most prominent high-frequency oscillatory patterns in the mPFC. The origin of these interplayed oscillatory rhythms seems to depends in the interaction of local GABAergic and glutamatergic neurons (Bitzenhofer et al., 2017; Cardin, 2018). Even more, some studies link their origin to different subtypes of local GABAergic INs. For example, in the primary visual cortex of behaving mice, optogenetic differential stimulation of SST-IN or PV-IN are preferentially correlated with enhancement of beta or gamma activity, respectively (Chen et al., 2017). Although classical works provide support to the role of peri-somatic inhibition in gamma rhythmogenesis (Cardin et al., 2009; Sohal et al., 2009), it has also been shown that gamma can be driven by SST-IN, PNs or long-range GABAergic neurons projecting to the cortex (Adesnik and Scanziani, 2010; Kim et al., 2015; Veit et al., 2017). In summary, the neuronal composition (subtypes of neurons) and architecture (cell morphology and disposition of cells in space), as well as synchronized activity of cells are important factors needed for the emergence of oscillations at particular frequencies.
Astrocytes (a subtype of glial cell) also participate in the maintenance and modulation of brain rhythms (Buskila et al., 2019). Since they have a close association with synapses, they regulate the concentration of extracellular ions and neurotransmitters (tight control of the extracellular K+, glutamate uptake and gliotransmission). Also they communicate through calcium waves (astrocytic communication via gap junction), mechanisms by can influence brain activity and synchronization (Amzica et al., 2002; Lee et al., 2014; Bellot-Saez et al., 2018; Buskila et al., 2019). Indeed, astrocytic calcium dynamic is relevant for the modulation of hippocampal theta activity, and attenuation of IP3-mediated Ca+2 signaling in astrocytes increase theta power, especially during REM sleep (Foley et al., 2017). Higher frequency rhythms are also modulated by astrocytes; blockade of glutamate vesicular release from astrocytes induces a decrease in gamma power in vitro and in vivo, thus demonstrating their relevance for cortical gamma oscillations (Lee et al., 2014). Moreover, in a mouse model of astrocyte-specific exocytosis impairment (blockade of gliotransmitter release, presumably D-serine), the mPFC-HPC theta synchronization was impaired, as well as cognitive tasks associated with spatial learning and reference memory (Sardinha et al., 2017). Thus, these result evidence the modulation of network dynamics by astrocytes and their impact in functional communication (Sardinha et al., 2017).
Together with cell composition and the architecture of neural networks, the genetic background of cells is relevant for the generation of brain rhythms (Buzsáki et al., 2013). Indeed, it has been shown that brain oscillations are highly heritable (Peeters et al., 1992; van Beijsterveldt et al., 1996; Franken et al., 1998; Buzsáki et al., 2013; Müller et al., 2017). In humans, EEG profiles show higher similarity between monozygotic twins compared with between dizygotic twins or unrelated people (Landolt, 2011). Additionally, genetic mutations can generate disease states; for example deletions or duplications of the SCN1A gene cause Dravet syndrome; these patients show an impaired ability to generate gamma activity in response to auditory stimuli compared to healthy controls (Sanchez-Carpintero et al., 2020). Moreover, current research has identified genes in the neocortex correlated with oscillatory activity linked to successful memory encoding (Berto et al., 2018, 2021). These genes are expressed mainly in neurons, which encode ion channels and synaptic proteins (Berto et al., 2018, 2021). In a recent work performed in humans, it was observed that genes encoding for ion channel activity, chromatin remodeling, synaptic scaffolding, and alternative splicing were related to successful memory encoding (Berto et al., 2021; Khanna and Williams, 2021). Future research will clarify the mechanisms of genetic control of brain oscillations.
A key feature of cortical brain oscillations is that they are internally generated, even in the absence of external cues; hence, brain oscillations may represent internal-generated neural operations (Buzsáki et al., 2014). Thus, particular oscillatory patterns may emerge in the mPFC according to cognitive requirements, representing relevant features related to diverse cognitive and behavioral tasks (Hyman et al., 2005; Benchenane et al., 2010; Buzsáki, 2010; O’Neill et al., 2013). For example, theta oscillations appear in the rodent mPFC largely associated with the performance of spatial tasks (Siapas et al., 2005; O’Neill et al., 2013), and learning and memory consolidation (Benchenane et al., 2010; Alekseichuk et al., 2016). This prefrontal theta is usually coupled with hippocampal theta (see below) (Hyman et al., 2005; Jones and Wilson, 2005; O’Neill et al., 2013). On the other hand, high-frequency oscillations also emerges in the mPFC, which may represent local neural operations underlying information processing (Fries, 2009; Engel and Fries, 2010; Fernandez-Ruiz et al., 2023). For example, synchronization of mPFC with other cortical areas in the beta band in primates, humans and rodents is associated to top-down attention (i.e., expectation based attention), while synchronization in gamma prevails during bottom-up attention (i.e., states more focused in features of the presented stimuli) (Buschman and Miller, 2007). Similarly, during cognitive flexibility, increases in gamma activity (anterior cingulate and right temporo-parietal cortex) and decreases in alpha and beta (frontal and inferior-parietal cortex), have been related to improved performance during task-switching paradigms in humans (Proskovec et al., 2019). Cortical low-frequency rhythms, as theta oscillations, modulate the timing and amplitude of high-frequency rhythms, a phenomenon known as cross-frequency coupling (CFC) (Canolty and Knight, 2010; Lisman and Jensen, 2013; Aru et al., 2015). This phase-to-amplitude modulation allows the coordination of fast-local computations by slower oscillations at larger spatial scales, offering windows of efficient communication between different neural networks, allowing the integration of distributed local computations into large-scale processes (Canolty and Knight, 2010). This theta-gamma CFC modulation has been evidenced in the mPFC during the performance of several cognitive functions in rodents (Fujisawa and Buzsáki, 2011; Li et al., 2012; Tamura et al., 2017) and non-human and human primates (Voloh et al., 2015; Daume et al., 2017; Jones et al., 2020). Also 4-Hz rhythm is capable of synchronizing gamma oscillation in the mPFC, although its role on cognitive operations is still under research (Fujisawa and Buzsáki, 2011; Zhong et al., 2017; Karalis and Sirota, 2022). Interestingly, spiking neurons in the mPFC are also entrained by theta-gamma CFC (Fujisawa and Buzsáki, 2011; Li et al., 2012; Tamura et al., 2017) which has been hypothesized as a mechanism for integration and segregation of task-relevant neural populations. Thus, CFC seems to be critical for cognitive control (Helfrich and Knight, 2016).
Importantly, oscillations modulate the timing of the membrane potential of recruited neurons, synchronizing the timing of action potentials (Anastassiou et al., 2011). Thus, the synchronization of neural firing and oscillatory rhythms are not independent phenomena; contrarily, the synchronization of neuronal firing by oscillations seems to support the formation and activation of NAs through the integration of activity-dependent synaptic plasticity (Fell and Axmacher, 2011; Buzsáki and Watson, 2012). Therefore, oscillatory synchronization of neuronal spiking seems to be a requisite for the formation of NAs (Buzsáki, 2010). As an example, the timing and sequence of place cells in the HPC are synchronized by theta and gamma oscillations (Buzsáki and Moser, 2013). The capability of brain oscillations to modulate the timing and occurrence of neuronal spiking activity is commonly measured through phase-locking (Lowet et al., 2016). It has been shown that prefrontal oscillations, throughout phase-locking, synchronized prefrontal spiking that encode relevant event-related information for the behavioral task, promoting the generation and activation of cognitive relevant NAs (Benchenane et al., 2010; Negrón-Oyarzo et al., 2018). Thus, cognitive control of behavior may be supported by the coupling of NAs by different and complementary patterns of oscillatory activity in the mPFC.
2.3 Functional connectivity between the mPFC and distributed neural networks for the cognitive control of behavior
For the implementation of cognitive control, the mPFC requires rapid and flexible information exchange among anatomically connected structures that represents and store relevant information required for task performance (Euston et al., 2012; Buzsáki et al., 2014; Helfrich and Knight, 2016). It has been proposed the large-scale synchronization of activity patterns, known as functional connectivity (FC), is a neural mechanism for neural communication, allowing the integration of local computations across different spatio-temporal scales (Fries, 2005; Friston, 2011; Eickhoff and Müller, 2015). Consequently, FC between the mPFC and distributed neural networks may facilitate the dynamic integration and coupling of information crucial for the formation and activation of NAs involved in cognitive control (Colgin, 2011; Helfrich and Knight, 2016).
The mPFC shows strong FC with the HPC. FC is commonly measured as spectral coherence defined as the cross-correlation of both amplitude and phase as a function of frequency and time between two LFP signals (Gordon, 2011). Coherence in the theta frequency between the mPFC and HPC has been widely described, which increases in relationship with cognitive performance, such as decision making, spatial memory acquisition and inhibitory control (Adhikari et al., 2010b; Benchenane et al., 2010; O’Neill et al., 2013; Negrón-Oyarzo et al., 2018). Interestingly, this interaction modulates the spike timing of prefrontal neurons (Jones and Wilson, 2005; Siapas et al., 2005; Benchenane et al., 2010; Negrón-Oyarzo et al., 2018), allowing the emergence of neural spiking representations of relevant cognitive features during learning (Adhikari et al., 2010a,b; Benchenane et al., 2010). Also, there is a prominent coherence in the low-gamma band (20–40 Hz), which progressively increases over time through task acquisition, suggesting that the FC between mPFC-HPC supports strategy progression during learning (Negrón-Oyarzo et al., 2018). Hippocampal theta oscillation also coordinates prefrontal gamma oscillations though CFC (Sirota et al., 2008). This phenomenon has been associated with working memory (Fujisawa and Buzsáki, 2011; Li et al., 2012; Tamura et al., 2017). Given that gamma oscillations represent local computations (Fries, 2009; Fernandez-Ruiz et al., 2023) this long-range synchronization may allow the coordination and integration of distributed computations, favoring neural communication and plasticity required for cognitive control (Hyafil et al., 2015; Helfrich and Knight, 2016). The mPFC-HPC coupling may be relevant for the integration of spatial and temporal information into the mPFC required for cognitive control. However, it has been shown that the directionality of mPFC-HPC coupling may represents different processes: for example, HPC-to-mPFC participates in the transference of contextual information to the mPFC, whereas mPFC-to-HPC coupling guides successful retrieval of memories in the HPC (Place et al., 2016). Therefore, mPFC-HPC coupling may also represent cognitive control exerted by the mPFC. The coordination between the mPFC and HPC is also attained by 4-Hz oscillation (Karalis and Sirota, 2022). Similarly to theta, 4-Hz oscillation also coordinates gamma activity and neuronal spiking at long-range. However, contrary to theta coordination, 4-Hz synchronization emerges during offline states, when locomotion is not present (Karalis and Sirota, 2022). This coordination may be a complementary mechanism for neural communication when theta is absent (Folschweiller and Sauer, 2021).
As an accumulative process, the acquisition of goal-directed memory requires access to previous experiences stored in long-term memory and the formation of new long-term memories through memory consolidation (Mecklinger, 2010). The mPFC-HPC axis plays a pivotal role in supporting memory consolidation (Rothschild et al., 2017; Shin et al., 2019; Wagner et al., 2019). The most accepted current model form memory consolidation is the “two-stage model” (Buzsaki, 1989) that proposes an online stage, where environmental information is acquired, and an offline stage, where recently acquired information is transferred to distributed cortical modules for long-term storage (Nieuwenhuis and Takashima, 2010; Preston and Eichenbaum, 2013). During spatial memory formation, the online stage is characterized by a peak of coherence in theta between the HPC and mPFC, facilitating the coordination of neuronal firing in the mPFC and the formation of NAs to store relevant task-related information (Siapas et al., 2005; Sirota et al., 2008; Benchenane et al., 2010; Wang et al., 2020). Theta oscillations are believed to play a crucial role in “tagging” NAs for later consolidation (Peyrache et al., 2009; Girardeau and Zugaro, 2011; Jadhav et al., 2016). The offline stage, occurring during sleep or quiet wakefulness, involves reduced external stimulation (Wamsley, 2019). This stage is associated with the interaction of three major structures: the mPFC through slow oscillations (SO: <1 Hz), the thalamo-cortical circuit through spindles (10–16 Hz), and the HPC through sharp-wave ripples (SWR: 120–250 Hz) (Binder et al., 2019; Oyanedel et al., 2020). SWR in the HPC leads to the reactivation of hippocampal sequences formed during the online stage (Peyrache et al., 2009). Importantly, prefrontal neurons are reactivated during SWR, indicating the interaction between the mPFC and HPC during the consolidation process (Jadhav et al., 2012; Rothschild et al., 2017; Tang et al., 2017). This hippocampal reactivation during the offline stage allows the transfer of relevant information acquired during the online stage, contributing to the strengthening of synaptic connections and the consolidation of acquired information in the long-term (Frankland and Bontempi, 2005; Papale et al., 2016; Binder et al., 2019). Inhibition of SWR during the offline stage impairs the acquisition of goal-directed spatial memory, reinforcing the crucial role of the mPFC-HPC axis in the memory consolidation process (Girardeau et al., 2009; Jadhav et al., 2012; Binder et al., 2019). This evidence suggests the relevance of the mPFC-HPC coupling for several processes related to cognitive control.
It also has been documented FC between the mPFC and MD. For example, it has been observed coherence at beta frequency in the mPFC-MD circuit during working memory and decision-making (Parnaudeau et al., 2013; Bolkan et al., 2017). Specifically, in working memory tasks, it has been found that MD is related with the “online holding” of relevant information, whereas the mPFC is related with the execution of actions (Bolkan et al., 2017). The MD also may play a role in memory consolidation (Mitchell and Gaffan, 2008; Cross et al., 2012). Given that the MD decrease their firing rate when SWR emerges in the HPC (Logothetis et al., 2012; Yang et al., 2019), it has been suggested that that MD contributes to increase the mPFC reactivity to hippocampal SWR. This mechanisms may promote hippocampal-cortical communication for the consolidation of declarative memory (Yang et al., 2019).
FC between the mPFC and the amygdala may contribute to cognitive control of emotional processing (Alexandra Kredlow et al., 2022). Most of studies concerning mPFC-amygdala coupling have been performed using fear conditioning and extinction task. Early studies showed that theta oscillation is evident in the rodent LA during retrieval of fear memory (Pape et al., 2005). Interestingly, theta coherence between BLA and mPFC predict freezing (Popa et al., 2010) and successful fear discrimination (Likhtik et al., 2014). This has also been observed in human and non-human primates (Taub et al., 2018; Chen et al., 2021). However, as well as the mPFC-HPC interaction, the directionality of mPFC-amygdala coupling may signal different cognitive processes. For example, BLA-to-mPFC coordination has been associated with communication of aversiveness to the mPFC (Popa et al., 2010; Taub et al., 2018), whereas mPFC-to-BLA modulation was associated with prefrontal control of fear expression (Popa et al., 2010; Courtin et al., 2014) and successful threat evaluation (Likhtik et al., 2014). Interestingly, it has been shown that the directionality of mPFC-amygdala coordination in the theta band depends on fear responses during different stages of fear memory and extinction (Lesting et al., 2013). This suggests that long-range communication between the mPFC and the amygdala through theta oscillations depend on the current cognitive process. The mPFC and amygdala also interact through of theta-gamma CFC, which increase in periods of fear, and is differentially modulated by task requirements (Stujenske et al., 2014). 4-Hz oscillation also seems to have a central role in emotional processing in the mPFC-amygdala circuit (Folschweiller and Sauer, 2021). 4-Hz synchronization between mPFC and BLA increase during freezing behavior, in which mPFC-4-Hz entrain BLA oscillations and neural spiking, suggesting a role in top-down control of fear expression (Dejean et al., 2016; Karalis et al., 2016). Importantly, both theta and 4-Hz oscillations synchronizes neuronal spiking between the mPFC and amygdala, contributing to the formation of NAs signaling specific parameters related to the task, as fear expression or extinction (Courtin et al., 2014; Likhtik et al., 2014; Dejean et al., 2016; Karalis et al., 2016). Altogether, this body of evidence strongly suggests that functional connectivity between the mPFC and anatomically connected structures supports several features of cognitive control of behavior.
3 Dysfunctional activity patterns in the mPFC in mental conditions
The evidence presented above links cognitive control with neural activity patterns in the mPFC and its functional connectivity with distributed networks (Figure 1A). Considering that some normal and pathological conditions display a strong alteration in the cognitive control of behavior, it is expected that neurophysiological processes supporting this operation would be also impaired. However, the limited access to the recording and modulation of neural activity patterns in human subjects restrains the knowledge relating neurophysiological phenomena to the decline of cognitive control. Therefore, studies in animal models have been proposed and used to address this issue. Given the development of reliable rodent models mimicking normal and pathological mental conditions in humans, we can assume that the decline in cognitive control in these rodent models can be similar to that in humans. Thus, the integration and comparison between finding in humans and rodent models could give relevant cues to the understanding of neurophysiological mechanisms involved in decline of cognitive control in normal and pathological conditions. In the following sections we discuss how prefrontal activity patterns and cognitive control are altered in patients and rodent models of normal cognitive aging, mood disorders and schizophrenia.
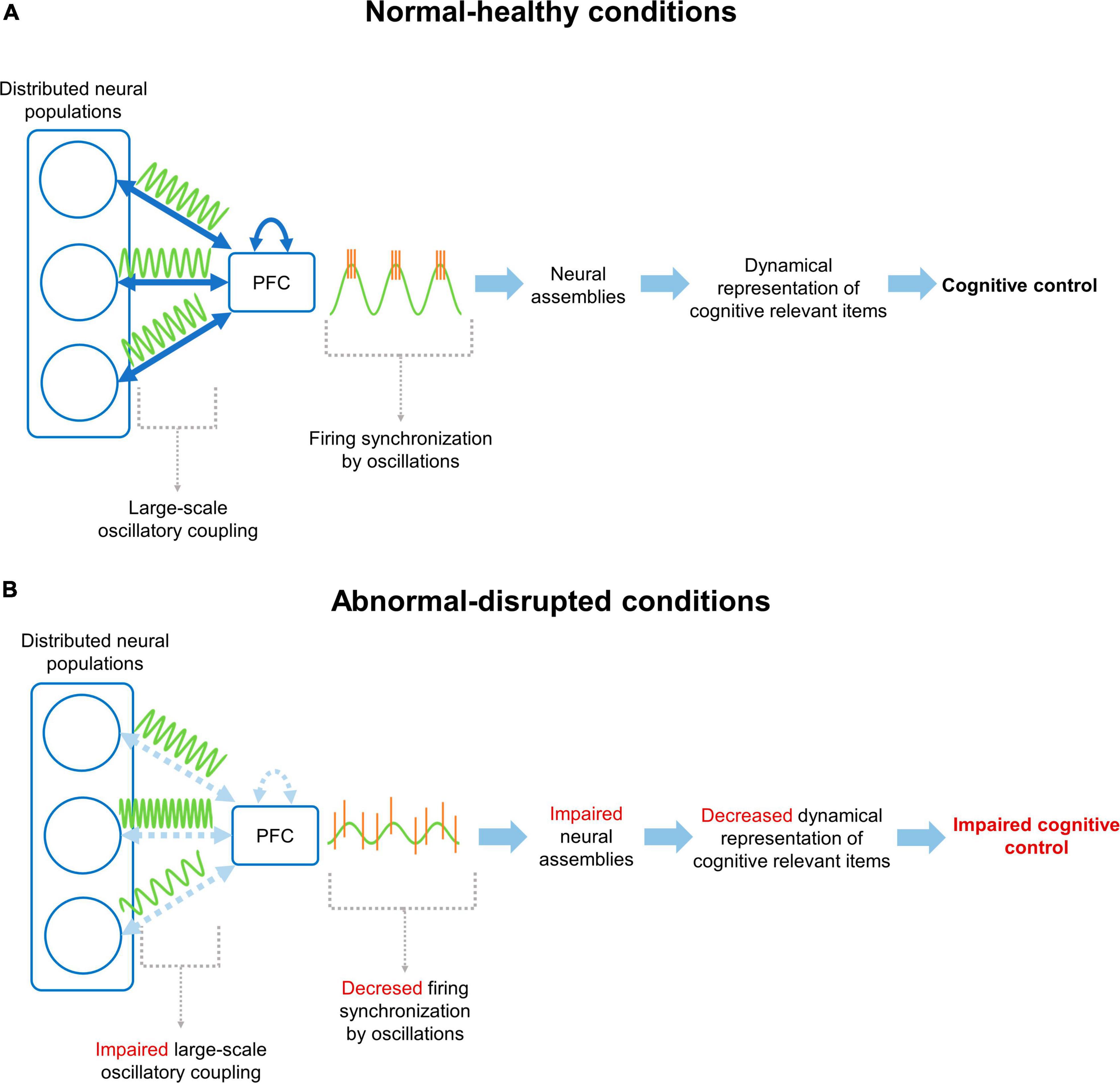
Figure 1. Neural mechanism for cognitive control through prefrontal cortex functional connectivity. (A) Under normal/healthy conditions, the PFC communicates with distributed neural populations through large-scale coupling of oscillatory activity patterns (i.e., functional connectivity). This coupling facilitates the synchronization of firing patterns in the PFC, promoting the formation, activation and updating of cognitively relevant NAs, supporting the cognitive control of behavior according to current demands. (B) On the other hand, under disrupted conditions, the connectivity between the PFC and distributed networks, or between prefrontal neurons, is altered. This prevents coupling between the mPFC and distributed networks, decreasing the synchronization of neuronal firing and hindering the organization of NAs. Finally, all this chain of events manifests as an impaired cognitive control of behavior.
3.1 Prefrontal oscillations and functional connectivity in normal cognitive aging
Aging has become a relevant topic in research because of the global increase in the proportion of older people. According to the World Health Organization (WHO), people aged 60 years or older currently reaches near 12% of the global population. Furthermore, it is projected that in the year 2050, the world population of people aged 60 years or older will have doubled (2.1 billion of people), reaching 22% of the total population (World Health Organization [WHO], 2022). Aging is a natural and irreversible process characterized by biological and social changes (Dziechciaż and Filip, 2014). However, elderly are also accompanied by a normal cognitive decline (NCA), a natural and gradual decline in cognitive functions that occurs normally over time, which differs from pathological syndromes, such as mild cognitive impairment and dementia (Harada et al., 2013; Dumas, 2015). NCA start at 40 years of age and progresses continuously (Harada et al., 2013). It has been estimated that between 3 and 8% of the population older than 65 years of age show a level of cognitive decline, whereas this proportion rise to 30% in the population older than 85 years (Blazer et al., 2015). This phenomenon strongly impact the autonomy and well-being of older people (Sánchez-García et al., 2019; Cylus and Al Tayara, 2021).
NCA is characterized by a decrease in “fluid” cognitive abilities, such as cognitive flexibility, problem-solving, and reasoning (Manard et al., 2014). In contrast, crystallized abilities, as accumulated knowledge of the world and over-learned familiar skills remain intact (McDonough et al., 2016; Salthouse, 2019). This differs from pathological conditions like dementia, in which more widespread brain functions impairment is observed, affecting both fluid and crystallized abilities, leading to a broader decline in cognitive capacities (Cadar, 2018). Deterioration of cognitive control is one of the main characteristics of NCA (Paxton et al., 2008), manifested as a diminished capacity to process new information and adapt to changing situations (Harada et al., 2013). Indeed, the cognitive decline is manifested as a deterioration of executive functions including working memory and cognitive flexibility (Paxton et al., 2008; Reinhart and Nguyen, 2019; Yagi et al., 2020). Notably relevant is the impairment of spatial memory (Head and Isom, 2010; Gazova et al., 2013; Bécu et al., 2023). Although these deficits are related to spatial components, as for example, spatial orientation (Moffat et al., 2006; Bécu et al., 2023), impairment on executive functions, such as strategy switching or attention, are significantly associated with deficits of spatial memory during aging (Rodgers et al., 2012; Wiener et al., 2013; Harris and Wolbers, 2014; Zhong and Moffat, 2018). This evidence suggests a relationship between cognitive decline and prefrontal function (West, 1996; Greenwood, 2000).
During aging, there is a general reduction in cortical thickness, volume, and weight of the brain (Dekaban and Sadowsky, 1978; Takao et al., 2012; Zheng et al., 2019; Cox et al., 2021). However, the reduction of cortical thickness is especially evident in the PFC (Dotson et al., 2015). Importantly, intrinsic and long-range anatomical connectivity of the PFC with distributed structures is decreased (Chadick et al., 2014; Pietrasik et al., 2023), which is associated with cognitive performance (Chadick et al., 2014). At neurophysiological level, EEG/MEG studies in humans have documented a decrease in the power of frontal low-frequency oscillations in aged subjects. For example, average power spectral density of theta frequencies is reduced in older subjects compared to young individuals (Vlahou et al., 2014; Meghdadi et al., 2021). Similarly, subjects with NCA display a decrease in spectral power at frequencies below 14 Hz in frontal superior and inferior areas during word-memory tasks (Healey and Kahana, 2020). In the same line, it has been shown that there is a decrease in theta (4–7 Hz), and alpha (9–14 Hz) power during memory tasks in the frontal areas of NCA patients compared to young adults (Rondina et al., 2016). During the resting state, the spectral power density of theta frequencies was significantly correlated with immediate and delayed verbal recall, attention, and executive function measures in older adults (Cummins and Finnigan, 2007; Finnigan and Robertson, 2011). Similarly, decrease of alpha rhythms in the frontal area correlates with performance during working memory task in older subjects (Clark et al., 2004). Interestingly, the evidence suggests that cognitive decline in working memory and behavioral flexibility in old age is associated with the difficulty of the PFC to synchronize at large-scale with distributed brain regions, such as temporal lobe and thalamus (Fama and Sullivan, 2015; Hakun et al., 2015; Reinhart and Nguyen, 2019). This evidence suggests that during the requirement of high cognitive demand, the cognitive decline in aging appears to be associated with the impairment of the slow-frequency synchronization of neural networks in the PFC, which could be related to the inability to recruit neural circuits during the task.
Naturally aged rodents (18–24 months) is the most common model for the study of aging (Yanai and Endo, 2021). Similar cognitive impairments to those observed in NCA in humans have been found in these rodent models (Brito et al., 2023). For instance, aged rodents display impairments in spatial memory (Rapp et al., 1987; Gallagher, 1997; Drapeau et al., 2003; Magnusson et al., 2003; Guidi et al., 2015; Lester et al., 2017), working memory, and strategy switching (Barnes et al., 1980; Breton et al., 2015; Yanai and Endo, 2021; Chong et al., 2023). Despite the existence of rodent models of aging, there are scarce studies concerning the neurophysiological phenomena in the mPFC related to the decline of cognitive control during aging. As in humans, aging in rodents is associated to a reduction in cortical thickness, volume, and weight of the rodent brain (Lessard-Beaudoin et al., 2015; Taylor et al., 2020). At neurophysiological level, and in agreement with human data, aged C57BL/6J mice show low power of theta and high-frequency oscillations during resting state in the mPFC (Rumschlag et al., 2021). Studies assessing the neuronal activity patterns in the mPFC related to impaired cognitive control during elderly is even scarcer. A recent study showed a decrease in the fraction of action-plan coding neurons in the mPFC of aged animals, which was related to slower learning in a working memory task (Chong et al., 2023). It has also been shown a reduced neural spike encoding of response latencies to stimuli in the mPFC during the delay period in an operant delayed-response task which was related with impaired performance (Caetano et al., 2012). These studies suggest a relationship between impaired neural encoding in the mPFC with the decline of cognitive abilities during aging. Altogether, these findings reflect that brain aging involves complex changes affecting prefrontal activity patterns, which may be key to understand the difficulties in the cognitive control observed during normal aging. Interestingly, brain stimulation mimicking theta-gamma CFC recovered working memory in older adults, supporting the role of prefrontal activity patterns in cognitive decline (Reinhart and Nguyen, 2019). Therefore, optogenetic stimulation, which offers high cell-identity, spatial and temporal precision, could be used in the mPFC of rodent models of aging, contributing to the development of evidence-based strategies to improve cognitive control in aged subjects.
3.2 Prefrontal oscillations and functional connectivity in mood disorders
Mood disorders includes a group of psychiatric diseases that affect the individual’s emotional processing, energy, and motivation. Some examples of these diseases are major depressive disorder (MDD) and anxiety disorders (AD). MDD has a lifetime prevalence of 16%, whereas anxiety disorders are even more prevalent than MDD, reaching up to 60% (Kessler et al., 2003). Genetic studies have shown a heritability of 37% for the generation of mood disorders. It also exists high comorbidity between both mental illnesses (Solomon et al., 2000). A large body of evidence indicates that subjects at higher risk of developing mood disorders are those who are genetically predisposed (Jaworska-Andryszewska and Rybakowski, 2019) and exposed to threatening and chronic life conditions, including people living in poverty, female victims of violence, the unemployed, neglected elderly persons and individuals exposed to bullying (McEwen and Akil, 2020). These threatening conditions generate a physiological response that allows adaptation to environmental threats, known as stress (McEwen, 2007). Stress-related mental diseases are characterized by a plethora of symptoms (American Psychiatric Association, 2013). However, the most important and invalidating features of mood disorders are related to cognitive control, which are strongly related to the impaired well-being of the patients. In this context, symptoms such as cognitive impairment, incapacity to control impulses and emotions are among the most relevant (Nezlek et al., 1994; Taylor Tavares et al., 2007; Hammar and Årdal, 2009; Millan et al., 2012). Current evidence shows that the PFC’s functioning is altered in mood disorders (Johnna and Swartz, 2013; Myers-schulz and Koenigs, 2014; Marrus et al., 2015; Mehta et al., 2018). One of the main components in mood disorders is the emotional dysregulation, i.e., the incapacity to regulate negative emotions. One study in humans showed that patients with MDD treated with antidepressants for 6 months presented an accelerated increase in the right dorso-lateral PFC activity during the regulation of negative affect in comparison to controls (Heller et al., 2013). Untreated MDD patients showed lower PFC activity in general, which altered the connectivity with the amygdala (Johnstone et al., 2007). This could be responsible for the characteristic emotional dysregulation symptom in this disease because this circuit is important for emotional processing. Changes in PFC engagement when regulating negative affect are inversely correlated with changes in depression severity (Johnstone et al., 2007). For social AD, it has been showed that patients presented lower amplitude fluctuations of low-frequency oscillations in the PFC in comparison to control patients (Zhang et al., 2015). Theta-gamma CFC alterations in the PFC is related to cognitive performance in MDD (Zheng and Zhang, 2013). Thus, this evidence supports the impairment of activity patterns in the PFC in mood disorders.
Several rodent models of mood disorders have been developed. Given the close relationship between mood disorders and chronic stress (McEwen and Akil, 2020), most of mood disorders models implicate the exposition of the animals to chronic-stressing conditions, such as chronic- and unpredictable stress, social-defeat stress, prenatal and early-life stress, or corticosterone manipulation, among others (Gururajan et al., 2019). These stressing conditions induce several behavioral manifestation of mood disorders, as increased anxiety, decreased locomotion and motivation, anhedonia, and social avoidance (Krishnan and Nestler, 2011; Wang et al., 2017; Gururajan et al., 2019). Also, these protocols induce cognitive impairment in spatial and recognition memory (Kleen et al., 2006; Conrad, 2010; Darcet et al., 2014), decision-making (Friedman et al., 2017), fear extinction (Negrón-Oyarzo et al., 2014) and behavioral flexibility (Hurtubise and Howland, 2017). Interestingly, during spatial learning, chronic stress induced a shift to more rigid stimulus-response strategies (Schwabe et al., 2008). This evidence suggests a stress-induced impairment in cognitive control.
It is widely documented that chronic stress induce dendritic pruning of PN in the mPFC (Cook and Wellman, 2004; Dias-Ferreira et al., 2009; Garrett and Wellman, 2009). At neurophysiological level, chronic stress reduced synaptic transmission in the mPFC (Yuen et al., 2012; Negrón-Oyarzo et al., 2014, 2015a) and reduce firing of prefrontal neurons (Mizoguchi et al., 2000; Goldwater et al., 2009; Wilber et al., 2011; Negrón-Oyarzo et al., 2015b). Similarly, social-defeat stress reduces spiking of prefrontal neurons (Abe et al., 2019). Thus, stress-related rodent models of mood disorders display profound alterations in prefrontal structure and function, which may be related to the impaired control of behavior. These neurophysiological impairments may impact in prefrontal activity patterns and FC during behavior implementation. Accordingly, it has been shown that chronic stress decreases mPFC-HPC coherence in the theta frequency band (Lee et al., 2011). Similarly, chronic stress reduced mPFC-HPC coherence at delta, theta and gamma bands during spatial memory task, which was related to the impairment of memory acquisition (Oliveira et al., 2013). Prenatal stress induced persistence of spatial memory during adulthood, suggesting a loss of behavioral flexibility, which was related to an increased synchronization between hippocampal-SWR and neuronal firing in the mPFC (Negrón-Oyarzo et al., 2015b). In a similar line, social-defeat stress induced a decrease in the 2–7 Hz oscillations in the mPFC, which correlated with stress-induced behavioral state (Kumar et al., 2014; Liu et al., 2022). Also, social-defeat stress reduced the incidence of 20–40 Hz events in susceptible animals during social interaction (Abe et al., 2019). Of relevance, social-defeat stress also reduced the prefrontal synchronization of neural spiking in the amygdala in susceptible animals (Kumar et al., 2014). If prefrontal neural representation and encoding of relevant cognitive evens is affected in rodent models of mood disorders is still unknown.
Finally, considering those antecedents, many prefrontal stimulation therapies have been developed to decrease symptoms of mood disorders (Cirillo et al., 2017). The search for new therapies is motivated by the limited effectiveness of pharmaceutical treatments (Nakagawa et al., 2017), as a great number of patients (between 12 and 55%, depending on the psychiatric illness) do not respond to this type of treatment (Nemeroff, 2007; Wiles et al., 2014). Stimulation therapies like deep brain stimulation (DBS) or transcranial magnetic stimulation (TMS) have been developed (Zrenner et al., 2020). Thus, precise, and evidence-oriented stimulation therapies may help patients to recover their abnormal oscillatory coupling characteristic of the pathophysiology of mood disorders. Therefore, rodent models of mood disorders are a reliable tool to test brain stimulation protocols (Okonogi and Sasaki, 2021). In rodent models, it has been shown that deep brain stimulation in the mPFC increased the synchronization between the HPC and the mPFC in the beta and gamma band, which may have an antidepressant-like effect, decreasing symptoms such as dysregulation in emotional processing (Jia et al., 2019). These amplitude fluctuations were related to the symptoms of the impairment. Interestingly, optogenetic stimulation of PN in the mPFC induced antidepressant-like effects in socially stressed mice (Covington et al., 2010; Kumar et al., 2013; Carlson et al., 2017). Similarly, optogenetic stimulation of prefrontal PN that project to DRN to amygdala recovered depressive-like behavior evaluated in the forced-swim test and social-interaction tests (Warden et al., 2012; Challis et al., 2014; Vialou et al., 2014). Of relevance, stimulation of prefrontal afferents to striatum recovered decision-making deficits induced by chronic stress (Friedman et al., 2017), suggesting that specific brain stimulation in the mPFC may recover cognitive control impaired in chronic-stress models of mood disorders (Biselli et al., 2021).
3.3 Prefrontal oscillations and functional connectivity in schizophrenia
Schizophrenia (SZ) is a severe mental disorder characterized by aberrant thoughts and behaviors. According to the WHO, it affects nearly 1% of the global population, reaching 21 million people worldwide (World Health Organization, 2012). SZ is most frequent in males, and the initial manifestation of the disease commonly appears in early adulthood (Häfner and an der Heiden, 1997). According to DSM-V (American Psychiatric Association, 2013) SZ is characterized by three main symptomatologic features: positive symptoms, which include delusion, paranoia and hallucinations; negative symptoms, such as abulia, alogia, anhedonia and avolition; and cognitive symptoms, manifested as an impairment on working memory, set-shifting, long-term memory recall, and selective attention (Goldman-Rakic, 1994; Hepp et al., 1996; Gold et al., 1997; Gallinat et al., 2004; Senkowski and Gallinat, 2015). These cognitive symptoms can be categorized as a detriment of executive control, in which the impairment in several forms of cognitive control have been considered as one of the most consistent, leading to the emergence of perseverative behaviors (Ridley, 1994; Crider, 1997; Lanser et al., 2002; Waltz, 2017). Thus, the deficit of cognitive control appears to be a hallmark of schizophrenia (Lesh et al., 2011).
Early neuroimaging investigations reported a decrease in the activation of the PFC in SZ patients (Ingvar and Franzén, 1974; Andreasen et al., 1992). Several of these findings have been largely replicated during the last decades (Shenton et al., 2001; Pomarol-Clotet et al., 2010; Penner et al., 2016). Importantly, reduced PFC activation has been correlated with impaired prefrontal-dependent cognitive operations in SZ (Perlstein et al., 2001, 2003). Thus, cognitive dysfunction observed in SZ is consistent with a deterioration of prefrontal function (Smucny et al., 2022). Structural connectivity analysis in SZ patients have revealed specific reduction of connectivity within the PFC and between the PFC with distributed structures (Kubicki et al., 2007). Prefrontal intrinsic connectivity is correlated with negative symptom (Hoptman et al., 2002; Wolkin et al., 2003) and decreased frontal-temporal connectivity is correlated with impairments in executive functions and memory (Kubicki et al., 2002, 2003). At a cellular level, postmortem studies revealed cytoarchitectonic alterations in the PFC of SZ, such as fewer dendritic spines in PN (Garey et al., 1998; Glantz and Lewis, 2000) reduction of neuropil (Selemon et al., 2004) and reduction of the mean clustering distance between cells (Casanova et al., 2008). Given its functional relevance, one of the most important post-mortem findings has been the reduction of the density of IN (Benes and Berretta, 2001) and GAD67 expressing cells in the PFC of SZ patients (Guidotti et al., 2000; Volk et al., 2000). Similar findings have been consistently found in several following postmortem studies (Beasley and Reynolds, 1997; Hashimoto et al., 2003, 2008; Bristow et al., 2015). Considering the role of INs in the emergence of brain oscillations, is not surprising that SZ patients displays aberrant oscillatory activity in the PFC (Uhlhaas and Singer, 2010; Senkowski and Gallinat, 2015; Hunt et al., 2017). Initial evidence reported an impairment in gamma oscillations. Specifically, it was found that the amplitude of gamma oscillations increased in the PFC of healthy controls when subjected to cognitive demands, effect not observed in SZ patients, which correlated with cognitive performance (Haig et al., 2000; Gallinat et al., 2004; Cho et al., 2006; Basar-Eroglu et al., 2007). This effect is observed in first episode SZ patients and is independent of medication status (Minzenberg et al., 2010). Importantly, the prefrontal cognitive-related impairment of gamma oscillations is the most consistent neurophysiological finding reported in SZ (Uhlhaas and Singer, 2010, 2013). This impairment of gamma is also observed at large-scale synchronization. For example, gamma synchronization between PFC and visual cortex is decreased in SZ patients, which correlated with the clinical state (Hirvonen et al., 2017). Interestingly, it has been found an increase of gamma oscillations during resting state in SZ patients (Boyden et al., 2005; Rutter et al., 2009; Kikuchi et al., 2011; Spencer, 2011; Andreou et al., 2015b; Grent-’t-Jong et al., 2018). This evidence suggests a difficulty to engage prefrontal gamma oscillations in SZ patients when it is required for cognitive control. Additionally, recent work has also found disturbances in theta oscillations in SZ patients. Similarly as for gamma oscillation, the impairment is evidenced as a decrease of prefrontal theta when the subject face cognitive challenge, such as working memory (Schmiedt et al., 2005; Griesmayr et al., 2014). Contrarily, it is observed an increased theta during resting state (Andreou et al., 2015a; Di Lorenzo et al., 2015). This impaired theta is also observed in long-range prefrontal coupling with distributed structures (Adams et al., 2020). In the same line, the increase of prefrontal theta-gamma- and delta-gamma CFC correlated with cognitive performance in healthy controls is not observed in SZ patients (Griesmayr et al., 2014; Missonnier et al., 2020). Therefore, the accumulated evidence suggests an impairment of gamma and theta oscillations, and in the interaction between these oscillation, in the PFC of SZ patients.
To date, several postulates have been developed to explain the neuropathology of SZ, as dopaminergic dysfunction (Knable and Weinberger, 1997), dysregulation of the excitatory/inhibitory balance (Lewis et al., 2005), and impaired cortical neurodevelopment (Garey, 2010). The combination of these hypotheses into an integrative vulnerability-stress model (known as a “two-hit model”), which combines genetic with pre- and postnatal environmental factors, has become one of the most accepted postulates for the development of SZ, which also reveals the complexity and interaction of several factors in its physiopathology (Bayer et al., 1999; Davis et al., 2016). Thus, considering these hypotheses, together with the most relevant factors for the development of SZ, several rodent models have been developed (Table 1), in which cognitive impairments are usually evident (Lipska and Weinberger, 2000; Marcotte et al., 2001; Jones et al., 2011; Winship et al., 2019; Speers and Bilkey, 2021). For example, neurodevelopmental models, such as prenatal administration of methylazomethanol (MAM) (Lodge and Grace, 2009) display impairments in several cognitive functions, including cognitive control (Kállai et al., 2020). In the same line, models of impaired E/I balance, as the adolescent-ketamine administration model (Becker et al., 2003) display impaired behavioral flexibility (Floresco et al., 2009; Zonneveld et al., 2019). Finally, genetic models that replicate genetic susceptibility, as the transgenic models Dlx5/6+/– and Df(16)A+/–, display impairment in working memory, behavioral flexibility and inhibitory control (Sigurdsson et al., 2010; Del Pino et al., 2013; Cho et al., 2015).
Rodent models of SZ replicate several neurophysiological features found in patients. For example, it have been found a reduced number of PV-INs in the mPFC in the MAM model (Lodge et al., 2009). Similarly, ketamine-treated rats during adolescence showed a reduced expression of GAD67 in PV-INs and a decreased GABAergic neurotransmission (Pérez et al., 2019). Similar impairment in inhibitory synaptic transmission has been observed in genetic transgenic mouse models (Fénelon et al., 2013). These results suggest impaired inhibitory neurotransmission in the mPFC of SZ rodent models (Lewis et al., 2005; Lewis, 2012; Del Pino et al., 2013; Cho et al., 2015; Dienel and Lewis, 2019; Sauer and Bartos, 2022). Cortical GABAergic INs are relevant for the emergence of several cortical oscillations (Buzsáki and Draguhn, 2004). Therefore, it is not surprising that animal models of SZ displays aberrant oscillatory activity in the PFC (Uhlhaas and Singer, 2010; Senkowski and Gallinat, 2015; Hunt et al., 2017). Several of these prefrontal oscillatory alterations observed in SZ patients have been also found in animal models of SZ (Speers and Bilkey, 2021). For example, it have been observed decreased prefrontal gamma oscillations in models of impaired E/I balance (Pinault, 2008; Ahnaou et al., 2017; Ma et al., 2018), in the MAM neurodevelopmental model (Lodge et al., 2009) and the genetic models as Dlx5/6+/–, DISC1-mutant mice and Arc/Arg3.1 deficient mice (Cho et al., 2015; Gao et al., 2019; Sauer and Bartos, 2022). Interestingly, baseline gamma oscillation was increased in the mPFC of MAM and Dlx5/6+/– models but was decreased in relationship to behavioral flexibility and inhibitory control (Lodge et al., 2009; Cho et al., 2015). Also theta oscillations in the mPFC are impaired in rodent models of SZ. For example, decreased task-evoked theta oscillations in the mPFC was found in the MAM neurodevelopmental model (Lodge et al., 2009). Similarly, it was found reduced theta power in the mPFC of Arc/Arg3.1 deficient mice (Gao et al., 2019). This impairment in theta has also observed in the long-range coordination of the mPFC with the HPC in several rodent models of SZ (Sigurdsson et al., 2010; Del Pino et al., 2013; Dickerson et al., 2014). Importantly, this impaired synchrony correlated with impairment in cognitive performance (Sigurdsson et al., 2010). Of relevance, synchronization of spiking of prefrontal neurons by prefrontal theta and gamma oscillations is impaired in these rodent models of SZ (Sigurdsson et al., 2010; Sauer and Bartos, 2022). If this impaired oscillatory coordination of prefrontal neurons impact in the emergence of NAs representing relevant cognitive features is still unknown. Interestingly, it has been found disorganization of NAs in the visual cortex of ketamine and Df(16)A+/– models of SZ (Hamm et al., 2017).
The recovery of altered prefrontal oscillation has been considered an opportunity for treatment in SZ patients. However, prefrontal stimulation is SZ patients at gamma frequency produced mixed results (Barr et al., 2011; Hoy et al., 2015; Haller et al., 2020). These differences may rely in the limited spatial, temporal, and cell-type specificity of brain stimulation tools used in patients (Cho and Sohal, 2014). Therefore, optogenetic stimulation in animals models seems a reliable tool to investigate this issue. It has been found that optogenetic stimulation of PV-INs at gamma-frequency in the mPFC recovered the behavioral flexibility observed in rodent models of SZ (Cho et al., 2015; Patrono et al., 2023). This evidence give supports the role of emerging prefrontal activity patterns in SZ.
Altogether the body of work reviewed here describe characteristics of rodent models that implicate alterations of PFC function in impaired cognitive control and compare well with human symptomatology in diseases and during the course of aging that affect cognitive control.
4 Concluding remarks and perspectives
In this review, we presented and discuss current bibliographic evidence suggesting that cognitive control is altered in normal cognitive decline and pathological mental conditions. We also presented bibliographic evidence showing that cognitive control of behavior is supported by the PFC, a structure that integrates relevant information for the generation of adaptive behavioral responses in a goal-directed manner. Importantly, from studies in rodent animal models, it is suggested that cognitive control is mechanistically supported by particular neural activity patterns in the mPFC, such as NA and brain oscillations, which originate from different levels of spatio-temporal interactions among neural components. Finally, both in patients and animal models, the evidence suggests a robust relationship between aberrant oscillatory activity and impaired cognitive control of behavior observed in normal cognitive decline and pathological mental conditions, such as those observed during aging, mood disorders and schizophrenia.
The collected evidence from human studies suggests that a common phenomenon across normal cognitive decline and pathological metal conditions is an abnormal oscillatory activity in the PFC associated with impaired cognitive control. However, given that the evaluation of micro- and mesoscale mechanisms underlying cognitive control is difficult to approach in human subjects, the utilization of animal models is crucial for understanding primary cause and, therefore, for the development of efficient therapeutic and preventive strategies. Importantly, research performed in animal models has offered relevant evidence about the neuronal and network mechanisms supporting oscillatory activity in the PFC required for cognitive control. Thus, from research in animal models, we suggest a mechanism that integrates research from human patients with data obtained from animal models (schematic diagram in Figure 1B). Particular etiological factors (congenital, environmental, etc.,) affect the organization of prefrontal neural network and its connectivity with distributed neural populations. This induce local and large-scale impairment in functional connectivity, which is macroscopically manifested as abnormal oscillatory activity in the PFC. Thus, the formation, updating, consolidation and activation of NAs representing relevant cognitive features in the PFC are impaired, hindering the adaptive accommodation of behavioral responses according to internal and environmental conditions. Ultimately, these phenomena manifest as perseverative behaviors, as observed in normal cognitive decline and pathological mental conditions.
Considering that the long-term effectivity of current pharmacological treatment for normal and pathological mental conditions is unclear (Barbui et al., 2009), we propose that upcoming therapeutic strategies should be focused on the re-establishment of prefrontal neural activity patterns, together with their coupling with large-scale structures. Therapeutic strategies based on non-invasive brain stimulation, such as repetitive transcranial magnetic stimulation (rTMS) and transcranial direct current stimulation (tDCS), seems to be a promising choice (Regenold et al., 2022). However, the outcome of these therapeutic strategies is mixed, in part due to a lack of understanding of how rhythmic stimulation interacts with ongoing brain dynamics (Sreekumar et al., 2017). Therefore, understanding of the neural processes involved in the implementation of cognitive control is central to the successful implementation of preventive and therapeutic strategies.
Author contributions
IN-O: Writing – review and editing, Writing – original draft, Supervision, Funding acquisition, Conceptualization. TD: Writing – review and editing, Writing – original draft, Conceptualization. LC-V: Writing – review and editing, Writing – original draft. NL-Q: Writing – review and editing, Writing – original draft. JU-P: Writing – review and editing, Writing – original draft.
Funding
The author(s) declare financial support was received for the research, authorship, and/or publication of this article. This work was funded by ANILLO Grant (ACT210053) from ANID, Chile (to IN-O); Fondecyt for Initiation into Research Grant (11160251) from ANID, Chile (to IN-O); INICI-UV, Universidad de Valparaíso, Chile (to IN-O); and Beca de Doctorado Nacional from ANID, Chile (to TD, LC-V, NL-Q, and JU-P).
Acknowledgments
We thank Dr. Nelson Espinosa and Dr. Ariel Lara-Vasquez for commenting on previous versions of the manuscript.
Conflict of interest
The authors declare that the research was conducted in the absence of any commercial or financial relationships that could be construed as a potential conflict of interest.
Publisher’s note
All claims expressed in this article are solely those of the authors and do not necessarily represent those of their affiliated organizations, or those of the publisher, the editors and the reviewers. Any product that may be evaluated in this article, or claim that may be made by its manufacturer, is not guaranteed or endorsed by the publisher.
References
Abe, R., Okada, S., Nakayama, R., Ikegaya, Y., and Sasaki, T. (2019). Social defeat stress causes selective attenuation of neuronal activity in the ventromedial prefrontal cortex. Sci. Rep. 9:9447. doi: 10.1038/s41598-019-45833-5
Adams, R. A., Bush, D., Zheng, F., Meyer, S. S., Kaplan, R., Orfanos, S., et al. (2020). Impaired theta phase coupling underlies frontotemporal dysconnectivity in schizophrenia. Brain 143, 1261–1277. doi: 10.1093/brain/awaa035
Adesnik, H., and Scanziani, M. (2010). Lateral competition for cortical space by layer-specific horizontal circuits. Nature 464, 1115–1160. doi: 10.1038/nature08935
Adhikari, A., Topiwala, M. A., and Gordon, J. A. (2010b). Synchronized activity between the ventral hippocampus and the medial prefrontal cortex during anxiety. Neuron 65, 257–269. doi: 10.1016/j.neuron.2009.12.002
Adhikari, A., Sigurdsson, T., Topiwala, M. A., and Gordon, J. A. (2010a). Cross-correlation of instantaneous amplitudes of field potential oscillations: A straightforward method to estimate the directionality and lag between brain areas. J. Neurosci. Methods 191, 191–200. doi: 10.1016/j.jneumeth.2010.06.019
Ahnaou, A., Huysmans, H., Van de Casteele, T., and Drinkenburg, W. H. I. M. (2017). Cortical high gamma network oscillations and connectivity: A translational index for antipsychotics to normalize aberrant neurophysiological activity. Transl. Psychiatry 7:1285. doi: 10.1038/s41398-017-0002-9
Alekseichuk, I., Turi, Z., Amador de Lara, G., Antal, A., and Paulus, W. (2016). Spatial working memory in humans depends on theta and high gamma synchronization in the prefrontal cortex. Curr. Biol. 26, 1513–1521. doi: 10.1016/j.cub.2016.04.035
Alexandra Kredlow, M., Fenster, R. J., Laurent, E. S., Ressler, K. J., and Phelps, E. A. (2022). Prefrontal cortex, amygdala, and threat processing: Implications for PTSD. Neuropsychopharmacology 47, 247–259. doi: 10.1038/s41386-021-01155-7
American Psychiatric Association (2013). DSM-V: American journal of psychiatry. Washington, DC: American Psychiatric Association.
Amzica, F., Massimini, M., and Manfridi, A. (2002). Spatial buffering during slow and paroxysmal sleep oscillations in cortical networks of glial cells in vivo. J. Neurosci. 22, 1042–1053. doi: 10.1523/JNEUROSCI.22-03-01042.2002
Anastasiades, P. G., and Carter, A. G. (2021). Circuit organization of the rodent medial prefrontal cortex. Trends Neurosci. 44, 550–563. doi: 10.1016/j.tins.2021.03.006
Anastasiades, P. G., Marlin, J. J., and Carter, A. G. (2018). Cell-type specificity of callosally evoked excitation and feedforward inhibition in the prefrontal cortex. Cell Rep. 22, 679–692. doi: 10.1016/j.celrep.2017.12.073
Anastassiou, C. A., Perin, R., Markram, H., and Koch, C. (2011). Ephaptic coupling of cortical neurons. Nat. Neurosci. 14, 217–223. doi: 10.1038/nn.2727
Andreasen, N. C., Flaum, M., and Arndt, S. (1992). The comprehensive assessment of symptoms and history (CASH). An instrument for assessing diagnosis and psychopathology. Arch. Gen. Psychiatry 49, 615–623. doi: 10.1001/archpsyc.1992.01820080023004
Andreou, C., Nolte, G., Leicht, G., Polomac, N., Hanganu-Opatz, I. L., Lambert, M., et al. (2015b). Increased resting-state gamma-band connectivity in first-episode schizophrenia. Schizophr. Bull. 41, 930–939. doi: 10.1093/schbul/sbu121
Andreou, C., Leicht, G., Nolte, G., Polomac, N., Moritz, S., Karow, A., et al. (2015a). Resting-state theta-band connectivity and verbal memory in schizophrenia and in the high-risk state. Schizophr. Res. 161, 299–307. doi: 10.1016/j.schres.2014.12.018
Aru, J., Aru, J., Priesemann, V., Wibral, M., Lana, L., Pipa, G., et al. (2015). Untangling cross-frequency coupling in neuroscience. Curr. Opin. Neurobiol. 31, 51–61. doi: 10.1016/j.conb.2014.08.002
Asinof, S. K., and Paine, T. A. (2014). The 5-choice serial reaction time task: A task of attention and impulse control for rodents. J. Visual. Exp. 90:e51574. doi: 10.3791/51574
Azevedo, E. P., Ivan, V. J., Friedman, J. M., and Stern, S. A. (2022). Higher-order inputs involved in appetite control. Biol. Psychiatry 91, 869–878. doi: 10.1016/j.biopsych.2021.07.015
Baeg, E. H., Kim, Y. B., Huh, K., Mook-Jung, I., Kim, H. T., and Jung, M. W. (2003). Dynamics of population code for working memory in the prefrontal cortex. Neuron 40, 177–188. doi: 10.1016/S0896-6273(03)00597-X
Baeg, E. H., Kim, Y. B., Kim, J., Ghim, J.-W., Kim, J. J., and Jung, M. W. (2007). Learning-induced enduring changes in functional connectivity among prefrontal cortical neurons. J. Neurosci. 27, 909–918. doi: 10.1523/JNEUROSCI.4759-06.2007
Bagur, S., Lefort, J. M., Lacroix, M. M., de Lavilléon, G., Herry, C., Chouvaeff, M., et al. (2021). Breathing-driven prefrontal oscillations regulate maintenance of conditioned-fear evoked freezing independently of initiation. Nat. Commun. 12:2605. doi: 10.1038/s41467-021-22798-6
Baker, B. I., and Bird, D. J. (2002). Analysis of projections from the medial prefrontal cortex to the thalamus in the rat, with emphasis on nucleus reuniens. J. Comp. Neurol. 442, 163–187. doi: 10.1002/CNE.10083
Barbui, C., Cipriani, A., and Saxena, S. (2009). Pharmacological treatment of mental disorders in primary health care. Geneva: World Health Organization, doi: 10.1017/CBO9781107415324.004
Barnes, C. A., Nadel, L., and Honig, W. K. (1980). Spatial memory deficit in senescent rats. Can. J. Psychol. 34, 29–39. doi: 10.1037/h0081022
Barr, M. S., Farzan, F., Arenovich, T., Chen, R., Fitzgerald, P. B., and Daskalakis, Z. J. (2011). The effect of repetitive transcranial magnetic stimulation on gamma oscillatory activity in schizophrenia. PLoS One 6:e22627. doi: 10.1371/journal.pone.0022627
Barthas, F., and Kwan, A. C. (2017). Secondary motor cortex: Where “sensory” meets “motor” in the rodent frontal cortex. Trends Neurosci. 40, 181–193. doi: 10.1016/j.tins.2016.11.006
Basar-Eroglu, C., Brand, A., Hildebrandt, H., Karolina Kedzior, K., Mathes, B., and Schmiedt, C. (2007). Working memory related gamma oscillations in schizophrenia patients. Int. J. Psychophysiol. 64, 39–45. doi: 10.1016/j.ijpsycho.2006.07.007
Bayer, T. A., Falkai, P., and Maier, W. (1999). Genetic and non-genetic vulnerability factors in schizophrenia: The basis of the “two hit hypothesis”. J. Psychiatr. Res. 33, 543–548. doi: 10.1016/s0022-3956(99)00039-4
Beasley, C. L., and Reynolds, G. P. (1997). Parvalbumin-immunoreactive neurons are reduced in the prefrontal cortex of schizophrenics. Schizophr. Res. 24, 349–355. doi: 10.1016/s0920-9964(96)00122-3
Bechara, A., Damasio, A. R., Damasio, H., and Anderson, S. W. (1994). Insensitivity to future consequences following damage to human prefrontal cortex. Cognition 50, 7–15. doi: 10.1016/0010-0277(94)90018-3
Bechara, A., Damasio, H., Tranel, D., and Anderson, S. W. (1998). Dissociation of working memory from decision making within the human prefrontal cortex. J. Neurosci. 18, 428–437. doi: 10.1523/JNEUROSCI.18-01-00428.1998
Becker, A., Peters, B., Schroeder, H., Mann, T., Huether, G., and Grecksch, G. (2003). Ketamine-induced changes in rat behaviour: A possible animal model of schizophrenia. Progr. Neuro Psychopharmacol. Biol. Psychiatry 27, 687–700. doi: 10.1016/S0278-5846(03)00080-0
Bécu, M., Sheynikhovich, D., Ramanoël, S., Tatur, G., Ozier-Lafontaine, A., Authié, C. N., et al. (2023). Landmark-based spatial navigation across the human lifespan. Elife 12:e81318. doi: 10.7554/eLife.81318
Bedwell, S. A., Billett, E. E., Crofts, J. J., and Tinsley, C. J. (2014). The topology of connections between rat prefrontal, motor and sensory cortices. Front. Syst. Neurosci. 8:177. doi: 10.3389/fnsys.2014.00177
Bellot-Saez, A., Cohen, G., van Schaik, A., Ooi, L., Morley, J., and Buskila, Y. (2018). Astrocytic modulation of cortical oscillations. Sci. Rep. 8, 1–13. doi: 10.1038/s41598-018-30003-w
Benchenane, K., Peyrache, A., Khamassi, M., Tierney, P. L., Gioanni, Y., Battaglia, F. P., et al. (2010). Coherent theta oscillations and reorganization of spike timing in the hippocampal- prefrontal network upon learning. Neuron 66, 921–936. doi: 10.1016/j.neuron.2010.05.013
Benchenane, K., Tiesinga, P. H., and Battaglia, F. P. (2011). Oscillations in the prefrontal cortex: A gateway to memory and attention. Curr. Opin. Neurobiol. 21, 475–485. doi: 10.1016/j.conb.2011.01.004
Benes, F. M., and Berretta, S. (2001). GABAergic interneurons: Implications for understanding schizophrenia and bipolar disorder. Neuropsychopharmacology 25, 1–27. doi: 10.1016/S0893-133X(01)00225-1
Bernard, A., Lubbers, L. S., Tanis, K. Q., Luo, R., Podtelezhnikov, A. A., Finney, E. M., et al. (2012). Transcriptional architecture of the primate neocortex. Neuron 73, 1083–1099. doi: 10.1016/j.neuron.2012.03.002
Berto, S., Fontenot, M. R., Seger, S., Ayhan, F., Caglayan, E., Kulkarni, A., et al. (2021). Gene-expression correlates of the oscillatory signatures supporting human episodic memory encoding. Nat. Neurosci. 24, 554–564. doi: 10.1038/s41593-021-00803-x
Berto, S., Wang, G.-Z., Germi, J., Lega, B. C., and Konopka, G. (2018). Human genomic signatures of brain oscillations during memory encoding. Cereb. Cortex 28, 1733–1748. doi: 10.1093/cercor/bhx083
Binder, S., Mölle, M., Lippert, M., Bruder, R., Aksamaz, S., Ohl, F., et al. (2019). Monosynaptic hippocampal-prefrontal projections contribute to spatial memory consolidation in mice. J. Neurosci. 39, 6978–6991. doi: 10.1523/JNEUROSCI.2158-18.2019
Biselli, T., Lange, S. S., Sablottny, L., Steffen, J., and Walther, A. (2021). Optogenetic and chemogenetic insights into the neurocircuitry of depression-like behaviour: A systematic review. Eur. J. Neurosci. 53, 9–38. doi: 10.1111/ejn.14603
Biskamp, J., Bartos, M., and Sauer, J. F. (2017). Organization of prefrontal network activity by respiration-related oscillations. Sci. Rep. 7, 1–11. doi: 10.1038/srep45508
Bitzenhofer, S. H., Ahlbeck, J., Wolff, A., Wiegert, J. S., Gee, C. E., Oertner, T. G., et al. (2017). Layer-specific optogenetic activation of pyramidal neurons causes beta-gamma entrainment of neonatal networks. Nat. Commun. 8:14563. doi: 10.1038/ncomms14563
Blazer, D. G., Yaffe, K., and Liverman, C. T. (2015). Cognitive aging. Washington, DC: National Academies Press, doi: 10.17226/21693
Blumenthal, A., Steiner, A., Seeland, K., and Redish, A. D. (2011). Effects of pharmacological manipulations of NMDA-receptors on deliberation in the multiple-T task. Neurobiol. Learn. Mem. 95, 376–384. doi: 10.1016/j.nlm.2011.01.011
Boehme, R., Lorenz, R. C., Gleich, T., Romund, L., Pelz, P., Golde, S., et al. (2017). Reversal learning strategy in adolescence is associated with prefrontal cortex activation. Eur. J. Neurosci. 45, 129–137. doi: 10.1111/ejn.13401
Bolkan, S. S., Stujenske, J. M., Parnaudeau, S., Spellman, T. J., Rauffenbart, C., Abbas, A. I., et al. (2017). Thalamic projections sustain prefrontal activity during working memory maintenance. Nat. Neurosci. 20, 987–996. doi: 10.1038/nn.4568
Boyden, E. S., Zhang, F., Bamberg, E., Nagel, G., and Deisseroth, K. (2005). Millisecond-timescale, genetically targeted optical control of neural activity. Nat. Neurosci. 8, 1263–1268. doi: 10.1038/nn1525
Breton, Y.-A., Seeland, K. D., and Redish, A. D. (2015). Aging impairs deliberation and behavioral flexibility in inter-temporal choice. Front. Aging Neurosci. 7:41. doi: 10.3389/fnagi.2015.00041
Bristow, G. C., Bostrom, J. A., Haroutunian, V., and Sodhi, M. S. (2015). Sex differences in GABAergic gene expression occur in the anterior cingulate cortex in schizophrenia. Schizophr. Res. 167, 57–63. doi: 10.1016/j.schres.2015.01.025
Brito, D. V. C., Esteves, F., Rajado, A. T., Silva, N., Araújo, I., Bragança, J., et al. (2023). Assessing cognitive decline in the aging brain: Lessons from rodent and human studies. NPJ Aging 9:23. doi: 10.1038/s41514-023-00120-6
Brockett, A. T., Tennyson, S. S., deBettencourt, C. A., Kallmyer, M., and Roesch, M. R. (2022). Medial prefrontal cortex lesions disrupt prepotent action selection signals in dorsomedial striatum. Curr. Biol. 32:3276–3287.e3. doi: 10.1016/j.cub.2022.06.025
Burgess, P. W. (2000). Strategy application disorder: The role of the frontal lobes in human multitasking. Psychol. Res. 63, 279–288. doi: 10.1007/s004269900006
Buschman, T. J., and Miller, E. K. (2007). Top-down versus bottom-up control of attention in the prefrontal and posterior parietal cortices. Science 315, 1860–1864. doi: 10.1126/science.1138071
Buskila, Y., Bellot-Saez, A., and Morley, J. W. (2019). Generating Brain Waves, the Power of Astrocytes. Front. Neurosci. 13:1125. doi: 10.3389/fnins.2019.01125
Buzsaki, G. (1989). Two-stage model of memory trace formation: A role for “noisy” brain states. Neuroscience 31, 551–570. doi: 10.1016/0306-4522(89)90423-5
Buzsáki, G. (2004). Large-scale recording of neuronal ensembles. Nat. Neurosci. 7, 446–451. doi: 10.1038/nn1233
Buzsáki, G. (2010). Neural syntax: Cell assemblies, synapsembles, and readers. Neuron 68, 362–385. doi: 10.1016/j.neuron.2010.09.023
Buzsáki, G., Anastassiou, C. A., and Koch, C. (2012). The origin of extracellular fields and currents – EEG, ECoG, LFP and spikes. Nat. Rev. Neurosci. 13, 407–420. doi: 10.1038/nrn3241
Buzsáki, G., and Draguhn, A. (2004). Neuronal oscillations in cortical networks. Science 304, 1926–1929. doi: 10.1126/science.1099745
Buzsáki, G., and Moser, E. I. (2013). Memory, navigation and theta rhythm in the hippocampal-entorhinal system. Nat. Neurosci. 16, 130–138. doi: 10.1038/nn.3304
Buzsáki, G., and Tingley, D. (2018). Space and time: The hippocampus as a sequence generator. Trends Cogn. Sci. 22, 853–869. doi: 10.1016/j.tics.2018.07.006
Buzsáki, G., and Watson, B. O. (2012). Brain rhythms and neural syntax: Implications for efficient coding of cognitive content and neuropsychiatric disease. Dialog. Clin. Neurosci. 14, 345–367.
Buzsáki, G., Logothetis, N., and Singer, W. (2013). Scaling brain size, keeping timing: Evolutionary preservation of brain rhythms. Neuron 80, 751–764. doi: 10.1016/j.neuron.2013.10.002
Buzsáki, G., Peyrache, A., and Kubie, J. (2014). Emergence of cognition from action. Cold Spring Harb. Symposia Quant. Biol. 79, 41–50. doi: 10.1101/sqb.2014.79.024679
Cadar, D. (2018). “Cognitive ageing,” in Geriatrics health, ed. H. Çakmur (London: IntechOpen), 49–65. doi: 10.5772/intechopen.79119
Caetano, M. S., Horst, N. K., Harenberg, L., Liu, B., Arnsten, A. F. T., and Laubach, M. (2012). Lost in transition: Aging-related changes in executive control by the medial prefrontal cortex. J. Neurosci. 32, 3765–3777. doi: 10.1523/JNEUROSCI.6011-11.2012
Canolty, R. T., and Knight, R. T. (2010). The functional role of cross-frequency coupling. Trends Cogn. Sci. 14, 506–515. doi: 10.1016/j.tics.2010.09.001
Cardenas, A., Papadogiannis, A., and Dimitrov, E. (2021). The role of medial prefrontal cortex projections to locus ceruleus in mediating the sex differences in behavior in mice with inflammatory pain. FASEB J. 35:e21747. doi: 10.1096/fj.202100319RR
Cardin, J. A. (2018). Inhibitory interneurons regulate temporal precision and correlations in cortical circuits. Trends Neurosci. 41, 689–700.
Cardin, J. A., Carlen, M., Meletis, K., Knoblich, U., Zhang, F., Deisseroth, K., et al. (2009). Driving fast-spiking cells induces gamma rhythm and controls sensory responses. Nature 459, 663–667. doi: 10.1038/nature08002
Carlén, M. (2017). What constitutes the prefrontal cortex? Science 358, 478–482. doi: 10.1126/science.aan8868
Carlson, D., David, L. K., Gallagher, N. M., Vu, M.-A. T., Shirley, M., Hultman, R., et al. (2017). Dynamically timed stimulation of corticolimbic circuitry activates a stress-compensatory pathway. Biol. Psychiatry 82, 904–913. doi: 10.1016/j.biopsych.2017.06.008
Carrillo-Reid, L., Yang, W., Kang Miller, J., Peterka, D. S., and Yuste, R. (2017). Imaging and optically manipulating neuronal ensembles. Annu. Rev. Biophys. 46, 271–293. doi: 10.1146/annurev-biophys-070816-033647
Casanova, M. F., Kreczmanski, P., Trippe, J., Switala, A., Heinsen, H., Steinbusch, H. W. M., et al. (2008). Neuronal distribution in the neocortex of schizophrenic patients. Psychiatry Res. 158, 267–277. doi: 10.1016/j.psychres.2006.12.009
Chadick, J. Z., Zanto, T. P., and Gazzaley, A. (2014). Structural and functional differences in medial prefrontal cortex underlie distractibility and suppression deficits in ageing. Nat. Commun. 5:4223. doi: 10.1038/ncomms5223
Challis, C., Beck, S. G., and Berton, O. (2014). Optogenetic modulation of descending prefrontocortical inputs to the dorsal raphe bidirectionally bias socioaffective choices after social defeat. Front. Behav. Neurosci. 8:43. doi: 10.3389/fnbeh.2014.00043
Chang, C., Knapska, E., Orsini, C. A., Rabinak, C. A., Zimmerman, J. M., and Maren, S. (2009). Fear extinction in rodents. Curr. Protoc. Neurosci. 8:23. doi: 10.1002/0471142301.ns0823s47
Chao, L. L., and Knight, R. T. (1995). Human prefrontal lesions increase distractibility to irrelevant sensory inputs. Neuroreport 6, 1605–1610. doi: 10.1097/00001756-199508000-00005
Chao, O. Y., de Souza Silva, M. A., Yang, Y.-M., and Huston, J. P. (2020). The medial prefrontal cortex – hippocampus circuit that integrates information of object, place and time to construct episodic memory in rodents: Behavioral, anatomical and neurochemical properties. Neurosci. Biobehav. Rev. 113, 373–407. doi: 10.1016/j.neubiorev.2020.04.007
Chao, O. Y., Nikolaus, S., Yang, Y.-M., and Huston, J. P. (2022). Neuronal circuitry for recognition memory of object and place in rodent models. Neurosci. Biobehav. Rev. 141:104855. doi: 10.1016/j.neubiorev.2022.104855
Chen, G., Zhang, Y., Li, X., Zhao, X., Ye, Q., Lin, Y., et al. (2017). Distinct inhibitory circuits orchestrate cortical beta and gamma band oscillations. Neuron 96, 1403–1418.
Chen, S., Tan, Z., Xia, W., Gomes, C. A., Zhang, X., Zhou, W., et al. (2021). Theta oscillations synchronize human medial prefrontal cortex and amygdala during fear learning. Sci. Adv. 7:eabf4198. doi: 10.1126/sciadv.abf4198
Chen, W., Xia, X., Song, N., Wang, Y., Zhu, H., Deng, W., et al. (2016). Cross-species analysis of gene expression and function in prefrontal cortex, hippocampus and striatum. PLoS One 11:e0164295. doi: 10.1371/journal.pone.0164295
Cho, K. K. A., and Sohal, V. S. (2014). Optogenetic approaches for investigating neural pathways implicated in schizophrenia and related disorders. Hum. Mol. Genet. 23, R64–R68. doi: 10.1093/hmg/ddu225
Cho, K. K. A., Hoch, R., Lee, A. T., Patel, T., Rubenstein, J. L. R., and Sohal, V. S. (2015). Gamma rhythms link prefrontal interneuron dysfunction with cognitive inflexibility in Dlx5/6(+/-) mice. Neuron 85, 1332–1343. doi: 10.1016/j.neuron.2015.02.019
Cho, K. K. A., Shi, J., Phensy, A. J., Turner, M. L., and Sohal, V. S. (2023). Long-range inhibition synchronizes and updates prefrontal task activity. Nature 617, 548–554. doi: 10.1038/s41586-023-06012-9
Cho, R. Y., Konecky, R. O., and Carter, C. S. (2006). Impairments in frontal cortical gamma synchrony and cognitive control in schizophrenia. Proc. Natl. Acad. Sci. U.S.A. 103, 19878–19883. doi: 10.1073/pnas.0609440103
Chong, H. R., Ranjbar-Slamloo, Y., Ho, M. Z. H., Ouyang, X., and Kamigaki, T. (2023). Functional alterations of the prefrontal circuit underlying cognitive aging in mice. Nat. Commun. 14:7254. doi: 10.1038/s41467-023-43142-0
Cirillo, G., Di Pino, G., Capone, F., Ranieri, F., Florio, L., Todisco, V., et al. (2017). Neurobiological after-effects of non-invasive brain stimulation. Brain Stimul. 10, 1–18. doi: 10.1016/j.brs.2016.11.009
Clark, C. R., Veltmeyer, M. D., Hamilton, R. J., Simms, E., Paul, R., Hermens, D., et al. (2004). Spontaneous alpha peak frequency predicts working memory performance across the age span. Int. J. Psychophysiol. 53, 1–9. doi: 10.1016/j.ijpsycho.2003.12.011
Cohen, A. O., and Meyer, H. C. (2020). Ventral hippocampus projections to prelimbic cortex support contextual fear memory. J. Neurosci. 40, 8410–8412. doi: 10.1523/JNEUROSCI.1285-20.2020
Colgin, L. L. (2011). Oscillations and hippocampal-prefrontal synchrony. Curr. Opin. Neurobiol. 21, 467–474. doi: 10.1016/j.conb.2011.04.006
Collett, T. S., and Graham, P. (2004). Animal navigation: Path integration, visual landmarks and cognitive maps. Curr. Biol. 14, R475–R477. doi: 10.1016/j.cub.2004.06.013
Conrad, C. D. (2010). A critical review of chronic stress effects on spatial learning and memory. Progr. Neuro Psychopharmacol. Biol. Psychiatry 34, 742–755. doi: 10.1016/j.pnpbp.2009.11.003
Cook, S. C., and Wellman, C. L. (2004). Chronic stress alters dendritic morphology in rat medial prefrontal cortex. J. Neurobiol. 60, 236–248. doi: 10.1002/neu.20025
Cools, R., and Arnsten, A. F. T. (2022). Neuromodulation of prefrontal cortex cognitive function in primates: The powerful roles of monoamines and acetylcholine. Neuropsychopharmacology 47, 309–328. doi: 10.1038/s41386-021-01100-8
Courtin, J., Chaudun, F., Rozeske, R. R., Karalis, N., Gonzalez-Campo, C., Wurtz, H., et al. (2014). Prefrontal parvalbumin interneurons shape neuronal activity to drive fear expression. Nature 505, 92–96. doi: 10.1038/nature12755
Covington, H. E., Lobo, M. K., Maze, I., Vialou, V., Hyman, J. M., Zaman, S., et al. (2010). Antidepressant effect of optogenetic stimulation of the medial prefrontal cortex. J. Neurosci. 30, 16082–16090. doi: 10.1523/JNEUROSCI.1731-10.2010
Cox, S. R., Harris, M. A., Ritchie, S. J., Buchanan, C. R., Valdés Hernández, M. C., Corley, J., et al. (2021). Three major dimensions of human brain cortical ageing in relation to cognitive decline across the eighth decade of life. Mol. Psychiatry 26, 2651–2662. doi: 10.1038/s41380-020-00975-1
Crider, A. (1997). Perseveration in schizophrenia. Schizophr. Bull. 23, 63–74. doi: 10.1093/schbul/23.1.63
Cross, L., Brown, M. W., Aggleton, J. P., and Warburton, E. C. (2012). The medial dorsal thalamic nucleus and the medial prefrontal cortex of the rat function together to support associative recognition and recency but not item recognition. Learn. Mem. 20, 41–50. doi: 10.1101/lm.028266.112
Croxson, P. L., Walton, M. E., Boorman, E. D., Rushworth, M. F. S., and Bannerman, D. M. (2014). Unilateral medial frontal cortex lesions cause a cognitive decision-making deficit in rats. Eur. J. Neurosci. 40, 3757–3765. doi: 10.1111/ejn.12751
Cummins, T. D. R., and Finnigan, S. (2007). Theta power is reduced in healthy cognitive aging. Int. J. Psychophysiol. 66, 10–17. doi: 10.1016/j.ijpsycho.2007.05.008
Cylus, J., and Al Tayara, L. (2021). Health, an ageing labour force, and the economy: Does health moderate the relationship between population age-structure and economic growth? Soc. Sci. Med. 287:114353. doi: 10.1016/j.socscimed.2021.114353
d’Isa, R., and Gerlai, R. (2022). Designing animal-friendly behavioral tests for neuroscience research: The importance of an ethological approach. Front. Behav. Neurosci. 16:1090248. doi: 10.3389/fnbeh.2022.1090248
Darcet, F., Mendez-David, I., Tritschler, L., Gardier, A. M., Guilloux, J.-P., and David, D. J. (2014). Learning and memory impairments in a neuroendocrine mouse model of anxiety/depression. Front. Behav. Neurosci. 8:136. doi: 10.3389/fnbeh.2014.00136
Daume, J., Gruber, T., Engel, A. K., and Friese, U. (2017). Phase-amplitude coupling and long-range phase synchronization reveal frontotemporal interactions during visual working memory. J. Neurosci. 37, 313–322. doi: 10.1523/JNEUROSCI.2130-16.2016
Davis, J., Eyre, H., Jacka, F. N., Dodd, S., Dean, O., McEwen, S., et al. (2016). A review of vulnerability and risks for schizophrenia: Beyond the two hit hypothesis. Neurosci. Biobehav. Rev. 65, 185–194. doi: 10.1016/j.neubiorev.2016.03.017
de Bruin, J. P., Moita, M. P., de Brabander, H. M., Joosten, R. N., Debruin, J., Moita, M. P., et al. (2001). Place and response learning of rats in a Morris water maze: Differential effects of fimbria fornix and medial prefrontal cortex lesions. Neurobiol. Learn. Mem. 75, 164–178. doi: 10.1006/nlme.2000.3962
de Bruin, J. P., Swinkels, W. A., and de Brabander, J. M. (1997). Response learning of rats in a Morris water maze: Involvement of the medical prefrontal cortex. Behav. Brain Res. 85, 47–55.
Dejean, C., Courtin, J., Karalis, N., Chaudun, F., Wurtz, H., Bienvenu, T. C. M., et al. (2016). Prefrontal neuronal assemblies temporally control fear behaviour. Nature 535, 420–424. doi: 10.1038/nature18630
Dekaban, A. S., and Sadowsky, D. (1978). Changes in brain weights during the span of human life: Relation of brain weights to body heights and body weights. Ann. Neurol. 4, 345–356. doi: 10.1002/ana.410040410
Del Pino, I., García-Frigola, C., Dehorter, N., Brotons-Mas, J. R., Alvarez-Salvado, E., Martínez de Lagrán, M., et al. (2013). Erbb4 deletion from fast-spiking interneurons causes schizophrenia-like phenotypes. Neuron 79, 1152–1168. doi: 10.1016/j.neuron.2013.07.010
Delevich, K., Tucciarone, J., Huang, Z. J., and Li, B. (2015). The mediodorsal thalamus drives feedforward inhibition in the anterior cingulate cortex via parvalbumin interneurons. J. Neurosci. 35, 5743–5753. doi: 10.1523/JNEUROSCI.4565-14.2015
Di Lorenzo, G., Daverio, A., Ferrentino, F., Santarnecchi, E., Ciabattini, F., Monaco, L., et al. (2015). Altered resting-state EEG source functional connectivity in schizophrenia: The effect of illness duration. Front. Hum. Neurosci. 9:234. doi: 10.3389/fnhum.2015.00234
Diamond, A. (2013). Exectuve functions. Annu. Rev. Psychol. 64, 135–168. doi: 10.1146/annurev-psych-113011-143750.Executive
Dias-Ferreira, E., Sousa, J. C., Melo, I., Morgado, P., Mesquita, A. R., Cerqueira, J. J., et al. (2009). Chronic stress causes frontostriatal reorganization and affects decision-making. Science 325, 621–625. doi: 10.1126/science.1171203
Dickerson, D. D., Overeem, K. A., Wolff, A. R., Williams, J. M., Abraham, W. C., and Bilkey, D. K. (2014). Association of aberrant neural synchrony and altered GAD67 expression following exposure to maternal immune activation, a risk factor for schizophrenia. Transl. Psychiatry 4:e418. doi: 10.1038/tp.2014.64
Dienel, S. J., and Lewis, D. A. (2019). Alterations in cortical interneurons and cognitive function in schizophrenia. Neurobiol. Dis. 131:104208. doi: 10.1016/j.nbd.2018.06.020
Do-Monte, F. H., Manzano-Nieves, G., Quiñones-Laracuente, K., Ramos-Medina, L., and Quirk, G. J. (2015). Revisiting the role of infralimbic cortex in fear extinction with optogenetics. J. Neurosci. 35, 3607–3615. doi: 10.1523/JNEUROSCI.3137-14.2015
Donoghue, J. P., and Wise, S. P. (1982). The motor cortex of the rat: Cytoarchitecture and microstimulation mapping. J. Comp. Neurol. 212, 76–88. doi: 10.1002/cne.902120106
Dotson, V. M., Szymkowicz, S. M., Sozda, C. N., Kirton, J. W., Green, M. L., O’Shea, A., et al. (2015). Age differences in prefrontal surface area and thickness in middle aged to older adults. Front. Aging Neurosci. 7:250. doi: 10.3389/fnagi.2015.00250
Dragoi, G., and Tonegawa, S. (2011). Preplay of future place cell sequences by hippocampal cellular assemblies. Nature 469, 397–401. doi: 10.1038/nature09633
Drapeau, E., Mayo, W., Aurousseau, C., Le Moal, M., Piazza, P.-V., and Abrous, D. N. (2003). Spatial memory performances of aged rats in the water maze predict levels of hippocampal neurogenesis. Proc. Natl. Acad. Sci. U.S.A. 100, 14385–14390. doi: 10.1073/pnas.2334169100
Dudchenko, P. A. (2004). An overview of the tasks used to test working memory in rodents. Neurosci. Biobehav. Rev. 28, 699–709. doi: 10.1016/j.neubiorev.2004.09.002
Dumas, J. A. (2015). What is normal cognitive aging? Evidence from task-based functional neuroimaging. Curr. Behav. Neurosci. Rep. 2, 256–261. doi: 10.1007/s40473-015-0058-x
Dziechciaż, M., and Filip, R. (2014). Biological psychological and social determinants of old age: Bio-psycho-social aspects of human aging. Ann. Agric. Environ. Med. 21, 835–838. doi: 10.5604/12321966.1129943
Eichenbaum, H. (2017). Prefrontal-hippocampal interactions in episodic memory. Nat. Rev. Neurosci. 18, 547–558. doi: 10.1038/nrn.2017.74
Eickhoff, S. B., and Müller, V. I. (2015). “Functional connectivity,” in Brain mapping: An encyclopedic reference, ed. A. W. Toga (Waltham: Academic Press), 187–201. doi: 10.1016/B978-0-12-397025-1.00212-8
Elston, G. N. (2003). Cortex, cognition and the cell: New insights into the pyramidal neuron and prefrontal function. Cereb. Cortex 13, 1124–1138. doi: 10.1093/cercor/bhg093
Engel, A. K., and Fries, P. (2010). Beta-band oscillations–signalling the status quo? Curr. Opin. Neurobiol. 20, 156–165. doi: 10.1016/j.conb.2010.02.015
Engel, A. K., Fries, P., and Singer, W. (2001). Dynamic predictions: Oscillations and synchrony in top-down processing. Nat. Rev. Neurosci. 2, 704–716. doi: 10.1038/35094565
Eslinger, P. J., and Damasio, A. R. (1985). Severe disturbance of higher cognition after bilateral frontal lobe ablation: Patient EVR. Neurology 35, 1731–1741. doi: 10.1212/wnl.35.12.1731
Euston, D. R., Gruber, A. J., and McNaughton, B. L. (2012). The role of medial prefrontal cortex in memory and decision making. Neuron 76, 1057–1070. doi: 10.1016/j.neuron.2012.12.002
Fama, R., and Sullivan, E. V. (2015). Thalamic structures and associated cognitive functions: Relations with age and aging. Neurosci. Biobehav. Rev. 54, 29–37. doi: 10.1016/j.neubiorev.2015.03.008
Fell, J., and Axmacher, N. (2011). The role of phase synchronization in memory processes. Nat. Rev. Neurosci. 12, 105–118. doi: 10.1038/nrn2979
Fénelon, K., Xu, B., Lai, C. S., Mukai, J., Markx, S., Stark, K. L., et al. (2013). The pattern of cortical dysfunction in a mouse model of a schizophrenia-related microdeletion. J. Neurosci. 33, 14825–14839. doi: 10.1523/JNEUROSCI.1611-13.2013
Fernandez-Ruiz, A., Sirota, A., Lopes-dos-Santos, V., and Dupret, D. (2023). Over and above frequency: Gamma oscillations as units of neural circuit operations. Neuron 111, 936–953. doi: 10.1016/j.neuron.2023.02.026
Finnigan, S., and Robertson, I. H. (2011). Resting EEG theta power correlates with cognitive performance in healthy older adults. Psychophysiology 48, 1083–1087. doi: 10.1111/j.1469-8986.2010.01173.x
Floresco, S. B. (2015). The nucleus accumbens: An interface between cognition, emotion, and action. Annu. Rev. Psychol. 66, 25–52. doi: 10.1146/annurev-psych-010213-115159
Floresco, S. B., Zhang, Y., and Enomoto, T. (2009). Neural circuits subserving behavioral flexibility and their relevance to schizophrenia. Behav. Brain Res. 204, 396–409. doi: 10.1016/j.bbr.2008.12.001
Foley, J., Blutstein, T., Lee, S., Erneux, C., Halassa, M. M., and Haydon, P. (2017). Astrocytic IP(3)/Ca(2+) signaling modulates theta rhythm and REM sleep. Front. Neural Circ. 11:3. doi: 10.3389/fncir.2017.00003
Folschweiller, S., and Sauer, J. F. (2021). Respiration-driven brain oscillations in emotional cognition. Front. Neural Circ. 15:761812. doi: 10.3389/fncir.2021.761812
Franken, P., Malafosse, A., and Tafti, M. (1998). Genetic variation in EEG activity during sleep in inbred mice. Am. J. Physiol. 275, R1127–R1137. doi: 10.1152/ajpregu.1998.275.4.R1127
Frankland, P. W., and Bontempi, B. (2005). The organization of recent and remote memories. Nat. Rev. Neurosci. 6, 119–130. doi: 10.1101/lm.97905
Friedman, A., Homma, D., Bloem, B., Gibb, L. G., Amemori, K., Hu, D., et al. (2017). Chronic stress alters striosome-circuit dynamics, leading to aberrant decision-making. Cell 171:1191-1205.e28. doi: 10.1016/j.cell.2017.10.017
Fries, P. (2005). A mechanism for cognitive dynamics: Neuronal communication through neuronal coherence. Trends Cogn. Sci. 9, 474–480. doi: 10.1016/j.tics.2005.08.011
Fries, P. (2009). Neuronal gamma-band synchronization as a fundamental process in cortical computation. Annu. Rev. Neurosci. 32, 209–224. doi: 10.1146/annurev.neuro.051508.135603
Friston, K. J. (2011). Functional and effective connectivity: A review. Brain Connect. 1, 13–36. doi: 10.1089/brain.2011.0008
Fujisawa, S., Amarasingham, A., Harrison, M. T., and Buzsáki, G. (2008). Behavior-dependent short-term assembly dynamics in the medial prefrontal cortex. Nat. Neurosci. 11, 823–833. doi: 10.1038/nn.2134
Fujisawa, S., and Buzsáki, G. (2011). A 4 Hz Oscillation adaptively synchronizes prefrontal, VTA, and hippocampal activities. Neuron 72, 153–165. doi: 10.1016/j.neuron.2011.08.018
Fulcher, B. D., Murray, J. D., Zerbi, V., and Wang, X. J. (2019). Multimodal gradients across mouse cortex. Proc. Natl. Acad. Sci. U.S.A. 116, 4689–4695. doi: 10.1073/pnas.1814144116
Fuster, J. M. (2001). The prefrontal cortex–an update. Neuron 30, 319–333. doi: 10.1016/S0896-6273(01)00285-9
Fuster, J. M. (2008). The prefrontal cortex. Creativity 1, 15–17. doi: 10.1016/S0896-6273(00)80673-X
Gabbott, P. L. A., Warner, T. A., Jays, P. R. L., Salway, P., and Busby, S. J. (2005). Prefrontal cortex in the rat: Projections to subcortical autonomic, motor, and limbic centers. J. Comp. Neurol. 492, 145–177. doi: 10.1002/cne.20738
Gallagher, M. (1997). Animal models of memory impairment. Philos. Trans. R. Soc. Lond. Ser. B Biol. Sci. 352, 1711–1717. doi: 10.1098/rstb.1997.0153
Gallinat, J., Winterer, G., Herrmann, C. S., and Senkowski, D. (2004). Reduced oscillatory gamma-band responses in unmedicated schizophrenic patients indicate impaired frontal network processing. Clin. Neurophysiol. 115, 1863–1874. doi: 10.1016/j.clinph.2004.03.013
Gao, X., Grendel, J., Muhia, M., Castro-Gomez, S., Süsens, U., Isbrandt, D., et al. (2019). Disturbed prefrontal cortex activity in the absence of schizophrenia-like behavioral dysfunction in Arc/Arg3.1 deficient mice. J. Neurosci. 39, 8149–8163. doi: 10.1523/JNEUROSCI.0623-19.2019
Garey, L. (2010). When cortical development goes wrong: Schizophrenia as a neurodevelopmental disease of microcircuits. J. Anat. 217, 324–333. doi: 10.1111/j.1469-7580.2010.01231.x
Garey, L. J., Ong, W. Y., Patel, T. S., Kanani, M., Davis, A., Mortimer, A. M., et al. (1998). Reduced dendritic spine density on cerebral cortical pyramidal neurons in schizophrenia. J. Neurol. Neurosurg. Psychiatry 65, 446–453. doi: 10.1136/jnnp.65.4.446
Garrett, J. E., and Wellman, C. L. (2009). Chronic stress effects on dendritic morphology in medial prefrontal cortex: Sex differences and estrogen dependence. Neuroscience 162, 195–207. doi: 10.1016/j.neuroscience.2009.04.057
Gaykema, R. P., Gaál, G., Traber, J., Hersh, L. B., and Luiten, P. G. (1991). The basal forebrain cholinergic system: Efferent and afferent connectivity and long-term effects of lesions. Acta Psychiatr. Scand. Suppl. 366, 14–26. doi: 10.1111/j.1600-0447.1991.tb03105.x
Gazova, I., Laczó, J., Rubinova, E., Mokrisova, I., Hyncicova, E., Andel, R., et al. (2013). Spatial navigation in young versus older adults. Front. Aging Neurosci. 5:94. doi: 10.3389/fnagi.2013.00094
Girardeau, G., and Zugaro, M. (2011). Hippocampal ripples and memory consolidation. Curr. Opin. Neurobiol. 21, 452–459. doi: 10.1016/j.conb.2011.02.005
Girardeau, G., Benchenane, K., Wiener, S. I., Buzsáki, G., and Zugaro, M. B. (2009). Selective suppression of hippocampal ripples impairs spatial memory. Nat. Neurosci. 12, 1222–1223. doi: 10.1038/nn.2384
Glantz, L. A., and Lewis, D. A. (2000). Decreased dendritic spine density on prefrontal cortical pyramidal neurons in schizophrenia. Arch. Gen. Psychiatry 57, 65–73. doi: 10.1001/archpsyc.57.1.65
Gold, J. M., Carpenter, C., Randolph, C., Goldberg, T. E., and Weinberger, D. R. (1997). Auditory working memory and wisconsin card sorting test performance in schizophrenia. Arch. Gen. Psychiatry 54, 159–165. doi: 10.1001/archpsyc.1997.01830140071013
Goldman-Rakic, P. S. (1994). Working memory dysfunction in schizophrenia. J. Neuropsychiatry Clin. Neurosci. 6, 348–357. doi: 10.1176/jnp.6.4.348
Goldwater, D. S., Pavlides, C., Hunter, R. G., Bloss, E. B., Hof, P. R., McEwen, B. S., et al. (2009). Structural and functional alterations to rat medial prefrontal cortex following chronic restraint stress and recovery. Neuroscience 164, 798–808. doi: 10.1016/j.neuroscience.2009.08.053
Gordon, J. A. (2011). Oscillations and hippocampal-prefrontal synchrony. Curr. Opin. Neurobiol. 21, 486–491. doi: 10.1016/j.conb.2011.02.012
Graybiel, A. M. (2008). Habits, rituals, and the evaluative brain. Annu. Rev. Neurosci. 31, 359–387. doi: 10.1146/annurev.neuro.29.051605.112851
Greenwood, P. M. (2000). The frontal aging hypothesis evaluated. J. Int. Neuropsychol. Soc. 6, 705–726. doi: 10.1017/S1355617700666092
Grent-’t-Jong, T., Gross, J., Goense, J., Wibral, M., Gajwani, R., Gumley, A. I., et al. (2018). Resting-state gamma-band power alterations in schizophrenia reveal E/I-balance abnormalities across illness-stages. Elife 7:e37799. doi: 10.7554/eLife.37799
Griesmayr, B., Berger, B., Stelzig-Schoeler, R., Aichhorn, W., Bergmann, J., and Sauseng, P. (2014). EEG theta phase coupling during executive control of visual working memory investigated in individuals with schizophrenia and in healthy controls. Cogn. Affect. Behav. Neurosci. 14, 1340–1355. doi: 10.3758/s13415-014-0272-0
Guidi, M., Rani, A., Karic, S., Severance, B., Kumar, A., and Foster, T. C. (2015). Contribution of N-methyl-D-aspartate receptors to attention and episodic spatial memory during senescence. Neurobiol. Learn. Mem. 125, 36–46. doi: 10.1016/j.nlm.2015.07.015
Guidotti, A., Auta, J., Davis, J. M., Di-Giorgi-Gerevini, V., Dwivedi, Y., Grayson, D. R., et al. (2000). Decrease in reelin and glutamic acid decarboxylase67 (GAD67) expression in schizophrenia and bipolar disorder: A postmortem brain study. Arch. Gen. Psychiatry 57, 1061–1069.
Gururajan, A., Reif, A., Cryan, J. F., and Slattery, D. A. (2019). The future of rodent models in depression research. Nat. Rev. Neurosci. 20, 686–701. doi: 10.1038/s41583-019-0221-6
Haber, S. N., Liu, H., Seidlitz, J., and Bullmore, E. (2022). Prefrontal connectomics: From anatomy to human imaging. Neuropsychopharmacology 47, 20–40. doi: 10.1038/s41386-021-01156-6
Häfner, H., and an der Heiden, W. (1997). Epidemiology of schizophrenia. Rev. Can. Psychiatrie 42, 139–151. doi: 10.1177/070674379704200204
Haig, A. R., Gordon, E., De Pascalis, V., Meares, R. A., Bahramali, H., and Harris, A. (2000). Gamma activity in schizophrenia: Evidence of impaired network binding? Clin. Neurophysiol. 111, 1461–1468. doi: 10.1016/s1388-2457(00)00347-3
Hakun, J. G., Zhu, Z., Johnson, N. F., and Gold, B. T. (2015). Evidence for reduced efficiency and successful compensation in older adults during task switching. Cortex 64, 352–362. doi: 10.1016/j.cortex.2014.12.006
Haller, N., Hasan, A., Padberg, F., Brunelin, J., da Costa Lane Valiengo, L., and Palm, U. (2020). Gamma transcranial alternating current stimulation in patients with negative symptoms in schizophrenia: A case series. Neurophysiol. Clin. 50, 301–304. doi: 10.1016/j.neucli.2020.06.004
Hamilton, D. A., and Brigman, J. L. (2015). Behavioral flexibility in rats and mice: Contributions of distinct frontocortical regions. Genes Brain Behav. 14, 4–21. doi: 10.1111/gbb.12191
Hamm, J. P., Peterka, D. S., Gogos, J. A., and Yuste, R. (2017). Altered cortical ensembles in mouse models of schizophrenia. Neuron 94:153–167.e8. doi: 10.1016/j.neuron.2017.03.019
Hammar, Å, and Årdal, G. (2009). Cognitive functioning in major depression – A summary. Front. Hum. Neurosci. 3:26. doi: 10.3389/neuro.09.026.2009
Hanganu-Opatz, I. L., Klausberger, T., Sigurdsson, T., Nieder, A., Jacob, S. N., Bartos, M., et al. (2023). Resolving the prefrontal mechanisms of adaptive cognitive behaviors: A cross-species perspective. Neuron 111, 1020–1036. doi: 10.1016/j.neuron.2023.03.017
Harada, C. N., Natelson Love, M. C., and Triebel, K. L. (2013). Normal cognitive aging. Clin. Geriatr. Med. 29, 737–752. doi: 10.1016/j.cger.2013.07.002
Harris, J. A., Mihalas, S., Hirokawa, K. E., Whitesell, J. D., Choi, H., Bernard, A., et al. (2019). Hierarchical organization of cortical and thalamic connectivity. Nature 575, 195–202. doi: 10.1038/s41586-019-1716-z
Harris, M. A., and Wolbers, T. (2014). How age-related strategy switching deficits affect wayfinding in complex environments. Neurobiol. Aging 35, 1095–1102. doi: 10.1016/j.neurobiolaging.2013.10.086
Hashimoto, T., Arion, D., Unger, T., Maldonado-Avilés, J. G., Morris, H. M., Volk, D. W., et al. (2008). Alterations in GABA-related transcriptome in the dorsolateral prefrontal cortex of subjects with schizophrenia. Mol. Psychiatry 13, 147–161. doi: 10.1038/sj.mp.4002011
Hashimoto, T., Volk, D. W., Eggan, S. M., Mirnics, K., Pierri, J. N., Sun, Z., et al. (2003). Gene expression deficits in a subclass of GABA neurons in the prefrontal cortex of subjects with schizophrenia. J. Neurosci. 23, 6315–6326. doi: 10.2967/jnumed.108.060368
Head, D., and Isom, M. (2010). Age effects on wayfinding and route learning skills. Behav. Brain Res. 209, 49–58. doi: 10.1016/j.bbr.2010.01.012
Headley, D. B., and Paré, D. (2017). Common oscillatory mechanisms across multiple memory systems. NPJ Sci. Learn. 2:1. doi: 10.1038/s41539-016-0001-2
Healey, M. K., and Kahana, M. J. (2020). Age-related differences in the temporal dynamics of spectral power during memory encoding. PLoS One 15:e0227274. doi: 10.1371/journal.pone.0227274
Hebb, D. O. (1949). The organization of behavior: A neuropsychological theory. Sci. Educ. 44:335. doi: 10.2307/1418888
Helfrich, R. F., and Knight, R. T. (2016). Oscillatory dynamics of prefrontal cognitive control. Trends Cogn. Sci. 20, 916–930. doi: 10.1016/j.tics.2016.09.007
Heller, A. S., Johnstone, T., Peterson, M. J., Kolden, G. G., Kalin, N. H., and Davidson, R. J. (2013). Increases in prefrontal cortex activity when regulating negative emotion predicts symptom severity trajectory over six months in depression. JAMA Psychiatry 70, 1181–1189. doi: 10.1001/jamapsychiatry.2013.2430.Increases
Henny, P., and Jones, B. E. (2008). Projections from basal forebrain to prefrontal cortex comprise cholinergic, GABAergic and glutamatergic inputs to pyramidal cells or interneurons. Eur. J. Neurosci. 27, 654–670. doi: 10.1111/j.1460-9568.2008.06029.x
Hepp, H. H., Maier, S., Hermle, L., and Spitzer, M. (1996). The stroop effect in schizophrenic patients. Schizophr. Res. 22, 187–195. doi: 10.1016/S0920-9964(96)00080-1
Hirvonen, J., Wibral, M., Palva, J. M., Singer, W., Uhlhaas, P., and Palva, S. (2017). Whole-brain source-reconstructed MEG-data reveal reduced long-range synchronization in chronic schizophrenia. eNeuro 4, doi: 10.1523/ENEURO.0338-17.2017
Hok, V., Save, E., Lenck-Santini, P. P., and Poucet, B. (2005). Coding for spatial goals in the prelimbic/infralimbic area of the rat frontal cortex. Proc. Natl. Acad. Sci. U.S.A. 102, 4602–4607. doi: 10.1073/pnas.0407332102
Hoover, W., and Vertes, R. (2007). Anatomical analysis of afferent projections to the medial prefrontal cortex in the rat. Brain Struct. Funct. 212, 149–179. doi: 10.1007/s00429-007-0150-4
Hoptman, M. J., Volavka, J., Johnson, G., Weiss, E., Bilder, R. M., and Lim, K. O. (2002). Frontal white matter microstructure, aggression, and impulsivity in men with schizophrenia: A preliminary study. Biol. Psychiatry 52, 9–14. doi: 10.1016/s0006-3223(02)01311-2
Hoy, K. E., Bailey, N. W., Arnold, S. L., and Fitzgerald, P. B. (2015). The effect of transcranial direct current stimulation on gamma activity and working memory in schizophrenia. Psychiatry Res. 228, 191–196. doi: 10.1016/j.psychres.2015.04.032
Hunt, M. J., Kopell, N. J., Traub, R. D., and Whittington, M. A. (2017). Aberrant network activity in schizophrenia. Trends Neurosci. 40, 371–382. doi: 10.1016/j.tins.2017.04.003
Hurtubise, J. L., and Howland, J. G. (2017). Effects of stress on behavioral flexibility in rodents. Neuroscience 345, 176–192. doi: 10.1016/j.neuroscience.2016.04.007
Hyafil, A., Giraud, A. L., Fontolan, L., and Gutkin, B. (2015). Neural cross-frequency coupling: Connecting architectures, mechanisms, and functions. Trends Neurosci. 38, 725–740. doi: 10.1016/j.tins.2015.09.001
Hyman, J. M., Zilli, E. A., Paley, A. M., and Hasselmo, M. E. (2005). Medial prefrontal cortex cells show dynamic modulation with the hippocampal theta rhythm dependent on behavior. Hippocampus 15, 739–749. doi: 10.1002/hipo.20106
Ingvar, D. H., and Franzén, G. (1974). Distribution of cerebral activity in chronic schizophrenia. Lancet 2, 1484–1486. doi: 10.1016/s0140-6736(74)90221-9
Insausti, R., Herrero, M. T., and Witter, M. P. (1997). Entorhinal cortex of the rat: Cytoarchitectonic subdivisions and the origin and distribution of cortical efferents. Hippocampus 7, 146–183. doi: 10.1002/(SICI)1098-1063(1997)7:2<146::AID-HIPO4>3.0.CO;2-L
Ito, H. T. (2018). Prefrontal–hippocampal interactions for spatial navigation. Neurosci. Res. 129, 2–7. doi: 10.1016/j.neures.2017.04.016
Jadhav, S. P. P., Rothschild, G., Roumis, D. K. K., and Frank, L. M. M. (2016). Coordinated excitation and inhibition of prefrontal ensembles during awake hippocampal sharp-wave ripple events. Neuron 90, 113–127. doi: 10.1016/j.neuron.2016.02.010
Jadhav, S. P., Kemere, C., German, P. W., and Frank, L. M. (2012). Awake hippocampal sharp-wave ripples support spatial memory. Science 336, 1454–1458. doi: 10.1126/science.1217230
Jaworska-Andryszewska, P., and Rybakowski, J. K. (2019). Childhood trauma in mood disorders: Neurobiological mechanisms and implications for treatment. Pharmacol. Rep. 71, 112–120. doi: 10.1016/j.pharep.2018.10.004
Jay, T. M., and Witter, M. P. (1991). Distribution of hippocampal CA1 and subicular efferents in the prefrontal cortex of the rat studied by means of anterograde transport of Phaseolus vulgaris-leucoagglutinin. J. Comp. Neurol. 313, 574–586. doi: 10.1002/cne.903130404
Jeffery, K., Donnett, J., and O’Keefe, J. (1995). Medial septal control of theta-correlated unit firing in the entorhinal cortex of awake rats. Neurophysiol. Basic Clin. 6, 2166–2170.
Jia, L., Sun, Z., Shi, D., Wang, M., Jia, J., He, Y., et al. (2019). Effects of different patterns of electric stimulation of the ventromedial prefrontal cortex on hippocampal–prefrontal coherence in a rat model of depression. Behav. Brain Res. 356, 179–188. doi: 10.1016/j.bbr.2018.08.032
Johnna, R., and Swartz, C. S. M. (2013). The role of corticolimbic circuitry in the development of anxiety disorders in children and adolescents. Behav. Neurosci. 16, 133–148.
Johnstone, T., van Reekum, C. M., Urry, H. L., Kalin, N. H., and Davidson, R. J. (2007). Failure to regulate: Counterproductive recruitment of top-down prefrontal-subcortical circuitry in major depression. J. Neurosci. 27, 8877–8884. doi: 10.1523/jneurosci.2063-07.2007
Jones, B. F., Groenewegen, H. J., and Witter, M. P. (2005). Intrinsic connections of the cingulate cortex in the rat suggest the existence of multiple functionally segregated networks. Neuroscience 133, 193–207. doi: 10.1016/j.neuroscience.2005.01.063
Jones, C. A., Watson, D. J. G., and Fone, K. C. F. (2011). Animal models of schizophrenia. Br. J. Pharmacol. 164, 1162–1194. doi: 10.1111/j.1476-5381.2011.01386.x
Jones, K. T., Johnson, E. L., and Berryhill, M. E. (2020). Frontoparietal theta-gamma interactions track working memory enhancement with training and tDCS. Neuroimage 211:116615. doi: 10.1016/j.neuroimage.2020.116615
Jones, M. W., and Wilson, M. A. (2005). Theta rhythms coordinate hippocampal-prefrontal interactions in a spatial memory task. PLoS Biol. 3:e402. doi: 10.1371/journal.pbio.0030402
Joyce, M. K. P., Marshall, L. G., Banik, S. L., Wang, J., Xiao, D., Bunce, J. G., et al. (2022). Pathways for memory, cognition and emotional context: Hippocampal, subgenual area 25, and amygdalar axons show unique interactions in the primate thalamic reuniens nucleus. J. Neurosci. 42, 1068–1089. doi: 10.1523/JNEUROSCI.1724-21.2021
Jung, F., Witte, V., Yanovsky, Y., Klumpp, M., Brankačk, J., Tort, A. B. L., et al. (2022). Differential modulation of parietal cortex activity by respiration and h oscillations. J. Neurophysiol. 127, 801–817. doi: 10.1152/jn.00376.2021
Jung, M. W., Qin, Y., McNaughton, B. L., and Barnes, C. A. (1998). Firing characteristics of deep layer neurons in prefrontal cortex in rats performing spatial working memory tasks. Cereb. Cortex 8, 437–450. doi: 10.1093/cercor/8.5.437
Kahn, J. B., Ward, R. D., Kahn, L. W., Rudy, N. M., Kandel, E. R., Balsam, P. D., et al. (2012). Medial prefrontal lesions in mice impair sustained attention but spare maintenance of information in working memory. Learn. Mem. 19, 513–517. doi: 10.1101/lm.026302.112
Kállai, V., Lénárd, L., Péczely, L., Gálosi, R., Dusa, D., Tóth, A., et al. (2020). Cognitive performance of the MAM-E17 schizophrenia model rats in different age-periods. Behav. Brain Res. 379:112345. doi: 10.1016/j.bbr.2019.112345
Karalis, N., and Sirota, A. (2022). Breathing coordinates cortico-hippocampal dynamics in mice during offline states. Nat. Commun. 13, 1–20. doi: 10.1038/s41467-022-28090-5
Karalis, N., Dejean, C., Chaudun, F., Khoder, S., Rozeske, R., Wurtz, H., et al. (2016). 4-Hz oscillations synchronize prefrontal-amygdala circuits during fear behavior. Nat. Neurosci. 19, 605–612. doi: 10.1038/nn.4251
Kashdan, T. B., and Rottenberg, J. (2010). Psychological flexibility as a fundamental aspect of health. Clin. Psychol. Rev. 30, 865–878. doi: 10.1016/j.cpr.2010.03.001
Kawaguchi, Y., and Kubota, Y. (1997). GABAergic cell subtypes and their synaptic connections in rat frontal cortex. Cereb. Cortex 7, 476–486. doi: 10.1093/cercor/7.6.476
Kessler, R. C., Berglund, P., Demler, O., Jin, R., Koretz, D., Merikangas, K. R., et al. (2003). The epidemiology of major depressive disorder. Evid. Based Eye Care 4, 186–187. doi: 10.1097/00132578-200310000-00002
Ketz, N. A., Jensen, O., and O’Reilly, R. C. (2015). Thalamic pathways underlying prefrontal cortex-medial temporal lobe oscillatory interactions. Trends Neurosci. 38, 3–12. doi: 10.1016/j.tins.2014.09.007
Khanna, A. R., and Williams, Z. M. (2021). Genes that give our brains their rhythms. Nat. Neurosci. 24, 455–456. doi: 10.1038/s41593-021-00805-9
Kikuchi, M., Hashimoto, T., Nagasawa, T., Hirosawa, T., Minabe, Y., Yoshimura, M., et al. (2011). Frontal areas contribute to reduced global coordination of resting-state gamma activities in drug-naïve patients with schizophrenia. Schizophr. Res. 130, 187–194. doi: 10.1016/j.schres.2011.06.003
Kim, T., Thankachan, S., McKenna, J. T., McNally, J. M., Yang, C., Choi, J. H., et al. (2015). Correction: Cortically projecting basal forebrain parvalbumin neurons regulate cortical gamma band oscillations. Proc. Natl. Acad. Sci. U.S.A. 112:35350. doi: 10.1073/pnas.1507465112
Kitamura, T., Macdonald, C. J., and Tonegawa, S. (2015). Entorhinal-hippocampal neuronal circuits bridge temporally discontiguous events. Learn. Mem. 22, 438–443. doi: 10.1101/lm.038687.115
Kleen, J. K., Sitomer, M. T., Killeen, P. R., and Conrad, C. D. (2006). Chronic stress impairs spatial memory and motivation for reward without disrupting motor ability and motivation to explore. Behav. Neurosci. 120, 842–851. doi: 10.1037/0735-7044.120.4.842
Knable, M. B., and Weinberger, D. R. (1997). Dopamine, the prefrontal cortex and schizophrenia. J. Psychopharmacol. 11, 123–131. doi: 10.1177/026988119701100205
Kolb, B., and Walkey, J. (1987). Behavioural and anatomical studies of the posterior parietal cortex in the rat. Behav. Brain Res. 23, 127–145. doi: 10.1016/0166-4328(87)90050-7
Krishnan, V., and Nestler, E. J. (2011). Animal models of depression: Molecular perspectives. Curr. Top. Behav. Neurosci. 7, 121–147. doi: 10.1007/7854_2010_108
Kubicki, M., McCarley, R., Westin, C.-F., Park, H.-J., Maier, S., Kikinis, R., et al. (2007). A review of diffusion tensor imaging studies in schizophrenia. J. Psychiatr. Res. 41, 15–30. doi: 10.1016/j.jpsychires.2005.05.005
Kubicki, M., Westin, C.-F., Maier, S. E., Frumin, M., Nestor, P. G., Salisbury, D. F., et al. (2002). Uncinate fasciculus findings in schizophrenia: A magnetic resonance diffusion tensor imaging study. Am. J. Psychiatry 159, 813–820. doi: 10.1176/appi.ajp.159.5.813
Kubicki, M., Westin, C.-F., Nestor, P. G., Wible, C. G., Frumin, M., Maier, S. E., et al. (2003). Cingulate fasciculus integrity disruption in schizophrenia: A magnetic resonance diffusion tensor imaging study. Biol. Psychiatry 54, 1171–1180. doi: 10.1016/s0006-3223(03)00419-0
Kumar, S., Black, S. J., Hultman, R., Szabo, S. T., DeMaio, K. D., Du, J., et al. (2013). Cortical control of affective networks. J. Neurosci. 33, 1116–1129. doi: 10.1523/jneurosci.0092-12.2013
Kumar, S., Hultman, R., Hughes, D., Michel, N., Katz, B. M., and Dzirasa, K. (2014). Prefrontal cortex reactivity underlies trait vulnerability to chronic social defeat stress. Nat. Commun. 5:4537. doi: 10.1038/ncomms5537
Lai, C., Tanaka, S., Harris, T. D., and Lee, A. K. (2023). Volitional activation of remote place representations with a hippocampal brain-machine interface. Science 382, 566–573. doi: 10.1126/science.adh5206
Landolt, H.-P. (2011). Genetic determination of sleep EEG profiles in healthy humans. Progr. Brain Res. 193, 51–61. doi: 10.1016/B978-0-444-53839-0.00004-1
Lanser, M. G., Berger, H. J. C., Ellenbroek, B. A., Cools, A. R., and Zitman, F. G. (2002). Perseveration in schizophrenia: Failure to generate a plan and relationship with the psychomotor poverty subsyndrome. Psychiatry Res. 112, 13–26. doi: 10.1016/s0165-1781(02)00178-6
Laubach, M., Amarante, L. M., Swanson, K., and White, S. R. (2018). What, if anything, is rodent prefrontal cortex? eNeuro 5, doi: 10.1523/ENEURO.0315-18.2018
Le Merre, P., Ährlund-Richter, S., and Carlén, M. (2021). The mouse prefrontal cortex: Unity in diversity. Neuron 109, 1925–1944. doi: 10.1016/j.neuron.2021.03.035
LeDoux, J. E. (2000). Emotion circuits in the brain. Annu. Rev. Neurosci. 23, 155–184. doi: 10.1146/annurev.neuro.23.1.155
Lee, A. R., Kim, J. H., Cho, E., Kim, M., and Park, M. (2017). Dorsal and ventral hippocampus differentiate in functional pathways and differentially associate with neurological disease-related genes during postnatal development. Front. Mol. Neurosci. 10:331. doi: 10.3389/fnmol.2017.00331
Lee, H. S., Ghetti, A., Pinto-Duarte, A., Wang, X., Dziewczapolski, G., Galimi, F., et al. (2014). Astrocytes contribute to gamma oscillations and recognition memory. Proc. Natl. Acad. Sci. U.S.A. 111, E3343–E3352. doi: 10.1073/pnas.1410893111
Lee, Y. A., Poirier, P., Otani, S., and Goto, Y. (2011). Dorsal-ventral distinction of chronic stress-induced electrophysiological alterations in the rat medial prefrontal cortex. Neuroscience 183, 108–120. doi: 10.1016/j.neuroscience.2011.03.039
Lesh, T. A., Niendam, T. A., Minzenberg, M. J., and Carter, C. S. (2011). Cognitive control deficits in schizophrenia: Mechanisms and meaning. Neuropsychopharmacology 36, 316–338. doi: 10.1038/npp.2010.156
Lessard-Beaudoin, M., Laroche, M., Demers, M. J., Grenier, G., and Graham, R. K. (2015). Characterization of age-associated changes in peripheral organ and brain region weights in C57BL/6 mice. Exp. Gerontol. 63, 27–34. doi: 10.1016/j.exger.2015.01.003
Lester, A. W., Moffat, S. D., Wiener, J. M., Barnes, C. A., and Wolbers, T. (2017). The aging navigational system. Neuron 95, 1019–1035. doi: 10.1016/j.neuron.2017.06.037
Lesting, J., Daldrup, T., Narayanan, V., Himpe, C., Seidenbecher, T., and Pape, H. C. (2013). Directional theta coherence in prefrontal cortical to Amygdalo-hippocampal pathways signals fear extinction. PLoS One 8:e77707. doi: 10.1371/journal.pone.0077707
Lewis, D. A. (2012). Cortical circuit dysfunction and cognitive deficits in schizophrenia - implications for preemptive interventions. Eur. J. Neurosci. 35, 1871–1878. doi: 10.1111/j.1460-9568.2012.08156.x
Lewis, D. A., Hashimoto, T., and Volk, D. W. (2005). Cortical inhibitory neurons and schizophrenia. Nat. Rev. Neurosci. 6, 312–324. doi: 10.1038/nrn1648
Li, S., Bai, W., Liu, T., Yi, H., and Tian, X. (2012). Increases of theta-low gamma coupling in rat medial prefrontal cortex during working memory task. Brain Res. Bull. 89, 115–123. doi: 10.1016/j.brainresbull.2012.07.012
Likhtik, E., Stujenske, J. M., Topiwala, M., Harris, A. Z., and Gordon, J. (2014). Prefrontal entrainment of amygdala activity signals safety in learned fear and innate anxiety. Nat. Neurosci. 17, 106–113. doi: 10.1038/nn.3582
Lipska, B. K., and Weinberger, D. R. (2000). To model a psychiatric disorder in animals: Schizophrenia as a reality test. Neuropsychopharmacology 23, 223–239. doi: 10.1016/S0893-133X(00)00137-8
Lisman, J. E., and Jensen, O. (2013). The θ-γ neural code. Neuron 77, 1002–1016. doi: 10.1016/j.neuron.2013.03.007
Liu, T., Qi, C., Bai, W., Tian, X., and Zheng, X. (2022). Behavioral state-dependent oscillatory activity in prefrontal cortex induced by chronic social defeat stress. Front. Neurosci. 16:885432. doi: 10.3389/fnins.2022.885432
Lodge, D. J., and Grace, A. A. (2009). Gestational methylazoxymethanol acetate administration: A developmental disruption model of schizophrenia. Behav. Brain Res. 204, 306–312. doi: 10.1016/j.bbr.2009.01.031
Lodge, D. J., Behrens, M. M., and Grace, A. A. (2009). A loss of parvalbumin-containing interneurons is associated with diminished oscillatory activity in an animal model of schizophrenia. J. Neurosci. 29, 2344–2354. doi: 10.1523/JNEUROSCI.5419-08.2009
Logothetis, N. K., Eschenko, O., Murayama, Y., Augath, M., Steudel, T., Evrard, H. C., et al. (2012). Hippocampal-cortical interaction during periods of subcortical silence. Nature 491, 547–553. doi: 10.1038/nature11618
Lowet, E., Roberts, M. J., Bonizzi, P., Karel, J., and De Weerd, P. (2016). Quantifying neural oscillatory synchronization: A comparison between spectral coherence and phase-locking value approaches. PLoS One 11:e0146443. doi: 10.1371/journal.pone.0146443
Ma, L., Skoblenick, K., Johnston, K., and Everling, S. (2018). Ketamine alters lateral prefrontal oscillations in a rule-based working memory task. J. Neurosci. 38, 2482–2494. doi: 10.1523/JNEUROSCI.2659-17.2018
Magnusson, K. R., Scruggs, B., Aniya, J., Wright, K. C., Ontl, T., Xing, Y., et al. (2003). Age-related deficits in mice performing working memory tasks in a water maze. Behav. Neurosci. 117, 485–495. doi: 10.1037/0735-7044.117.3.485
Malagon-Vina, H., Ciocchi, S., Passecker, J., Dorffner, G., and Klausberger, T. (2018). Fluid network dynamics in the prefrontal cortex during multiple strategy switching. Nat. Commun. 9:309. doi: 10.1038/s41467-017-02764-x
Maling, N., and McIntyre, C. (2016). Local field potential analysis for closed-loop neuromodulation. Closed Loop Neurosci. 1, 67–80. doi: 10.1016/B978-0-12-802452-2.00005-6
Manard, M., Carabin, D., Jaspar, M., and Collette, F. (2014). Age-related decline in cognitive control: The role of fluid intelligence and processing speed. BMC Neurosci. 15:7. doi: 10.1186/1471-2202-15-7
Marcotte, E. R., Pearson, D. M., and Srivastava, L. K. (2001). Animal models of schizophrenia: A critical review. J. Psychiatry Neurosci. 26, 395–410.
Marek, R., Strobel, C., Bredy, T. W., and Sah, P. (2013). The amygdala and medial prefrontal cortex: Partners in the fear circuit. J. Physiol. 591, 2381–2391. doi: 10.1113/jphysiol.2012.248575
Marek, R., Xu, L., Sullivan, R. K. P., and Sah, P. (2018). Excitatory connections between the prelimbic and infralimbic medial prefrontal cortex show a role for the prelimbic cortex in fear extinction. Nat. Neurosci. 21, 654–658. doi: 10.1038/s41593-018-0137-x
Marrus, N., Belden, A., Nishino, T., Handler, T., Ratnanather, T., Miller, M., et al. (2015). Ventromedial prefrontal cortex thinning in preschool-onset depression. J. Affect. Disord. 180, 79–86.
Mashour, G. A., Pal, D., and Brown, E. N. (2022). Prefrontal cortex as a key node in arousal circuitry. Trends Neurosci. 45, 722–732. doi: 10.1016/j.tins.2022.07.002
Mecklinger, A. (2010). The control of long-term memory: Brain systems and cognitive processes. Neurosci. Biobehav. Rev. 34, 1055–1065. doi: 10.1016/j.neubiorev.2009.11.020
McDonough, I. M., Bischof, G. N., Kennedy, K. M., Rodrigue, K. M., Farrell, M. E., and Park, D. C. (2016). Discrepancies between fluid and crystallized ability in healthy adults: A behavioral marker of preclinical Alzheimer’s disease. Neurobiol. Aging 46, 68–75. doi: 10.1016/j.neurobiolaging.2016.06.011
McEwen, B. S. (2007). Physiology and neurobiology of stress and adaptation: Central role of the brain. Physiol. Rev. 87, 873–904. doi: 10.1152/physrev.00041.2006
McEwen, B. S., and Akil, H. (2020). Revisiting the stress concept: Implications for affective disorders. J. Neurosci. 40, 12–21. doi: 10.1523/JNEUROSCI.0733-19.2019
Meghdadi, A. H., Karic, M. S., McConnell, M., Rupp, G., Richard, C., Hamilton, J., et al. (2021). Resting state EEG biomarkers of cognitive decline associated with Alzheimer’s disease and mild cognitive impairment. PLoS One 16:e0244180. doi: 10.1371/journal.pone.0244180
Mehta, N. D., Haroon, E., Xu, X., Woolwine, B. J., and Li, Z. (2018). Inflammation negatively correlates with amygdala-ventromedial prefrontal functional connectivity in association with anxiety in patients with depression: Preliminary results. Brain Behav. Immun. 73, 725–730.
Mikhalevich, I., Powell, R., and Logan, C. (2017). Is behavioural flexibility evidence of cognitive complexity? How evolution can inform comparative cognition. Interface Focus 7:20160121. doi: 10.1098/rsfs.2016.0121
Millan, M. J., Agid, Y., Brüne, M., Bullmore, E. T., Carter, C. S., Clayton, N. S., et al. (2012). Cognitive dysfunction in psychiatric disorders: Characteristics, causes and the quest for improved therapy. Nat. Rev. Drug Discov. 11, 141–168. doi: 10.1038/nrd3628
Miller, E. K. (2000). The prefrontal cortex and cognitive control. Nat. Rev. Neurosci. 1, 59–65. doi: 10.1038/35036228
Milner, B. (1963). Effects of different brain lesions on card sorting: The role of the frontal lobes. Arch. Neurol. 9, 90–100. doi: 10.1001/archneur.1963.00460070100010
Milner, B. (1965). Visually-guided maze learning in man: Effects of bilateral hippocampal, bilateral frontal, and unilateral cerebral lesions. Neuropsychologia 3, 317–338. doi: 10.1016/0028-3932(65)90005-9
Minzenberg, M. J., Firl, A. J., Yoon, J. H., Gomes, G. C., Reinking, C., and Carter, C. S. (2010). Gamma oscillatory power is impaired during cognitive control independent of medication status in first-episode schizophrenia. Neuropsychopharmacology 35, 2590–2599. doi: 10.1038/npp.2010.150
Missonnier, P., Prévot, A., Herrmann, F. R., Ventura, J., Padée, A., and Merlo, M. C. G. (2020). Disruption of gamma-delta relationship related to working memory deficits in first-episode psychosis. J. Neural Trans. 127, 103–115. doi: 10.1007/s00702-019-02126-5
Mitchell, A. S., and Chakraborty, S. (2013). What does the mediodorsal thalamus do? Front. Syst. Neurosci. 7:37. doi: 10.3389/fnsys.2013.00037
Mitchell, A. S., and Gaffan, D. (2008). The magnocellular mediodorsal thalamus is necessary for memory acquisition, but not retrieval. J. Neurosci. 28, 258–263. doi: 10.1523/JNEUROSCI.4922-07.2008
Mitchell, A. S., Czajkowski, R., Zhang, N., Jeffery, K., and Nelson, A. J. D. (2018). Retrosplenial cortex and its role in spatial cognition. Brain Neurosci. Adv. 2:2398212818757098. doi: 10.1177/2398212818757098
Mitchell, S. J., Rawlins, J. N. P., Steward, O., and Olton, D. S. (1982). Medial septal area lesions disrupt θ rhythm and cholinergic staining in medial entorhinal cortex and produce impaired radial arm maze behavior in rats. J. Neurosci. 2, 292–302. doi: 10.1523/jneurosci.02-03-00292.1982
Mizoguchi, K., Yuzurihara, M., Ishige, A., Sasaki, H., Chui, D. H., and Tabira, T. (2000). Chronic stress induces impairment of spatial working memory because of prefrontal dopaminergic dysfunction. J. Neurosci. 20, 1568–1574.
Moffat, S. D., Elkins, W., and Resnick, S. M. (2006). Age differences in the neural systems supporting human allocentric spatial navigation. Neurobiol. Aging 27, 965–972. doi: 10.1016/j.neurobiolaging.2005.05.011
Moser, E. I., Kropff, E., and Moser, M.-B. (2008). Place cells, grid cells, and the brain’s spatial representation system. Annu. Rev. Neurosci. 31, 69–89. doi: 10.1146/annurev.neuro.31.061307.090723
Müller, V., Anokhin, A. P., and Lindenberger, U. (2017). Genetic influences on phase synchrony of brain oscillations supporting response inhibition. Int. J. Psychophysiol. 115, 125–132. doi: 10.1016/j.ijpsycho.2016.06.001
Myers-schulz, B., and Koenigs, M. (2014). Functional anatomy of ventromedial prefrontal cortex: Implications for mood and anxiety disorders. Mol. Psychiatry 17, 132–141. doi: 10.1038/mp.2011.88.Functional
Nakagawa, A., Mitsuda, D., Sado, M., Abe, T., Fujisawa, D., Kikuchi, T., et al. (2017). Effectiveness of supplementary cognitive-behavioral therapy for pharmacotherapy-resistant depression: A randomized controlled trial. J. Clin. Psychiatry 78, 1126–1135. doi: 10.4088/JCP.15m10511
Negrón-Oyarzo, I., Dagnino-Subiabre, A., and Muñoz Carvajal, P. (2015a). Synaptic impairment in layer 1 of the prefrontal cortex induced by repeated stress during adolescence is reversed in adulthood. Front. Cell. Neurosci. 9:442. doi: 10.3389/fncel.2015.00442
Negrón-Oyarzo, I., Neira, D., Espinosa, N., Fuentealba, P., and Aboitiz, F. (2015b). Prenatal stress produces persistence of remote memory and disrupts functional connectivity in the hippocampal-prefrontal cortex axis. Cereb. Cortex 25, 3132–3143. doi: 10.1093/cercor/bhu108
Negrón-Oyarzo, I., Espinosa, N., Aguilar-Rivera, M., Fuenzalida, M., Aboitiz, F., and Fuentealba, P. (2018). Coordinated prefrontal–hippocampal activity and navigation strategy-related prefrontal firing during spatial memory formation. Proc. Natl. Acad. Sci. U.S.A. 115, 7123–7128. doi: 10.1073/pnas.1720117115
Negrón-Oyarzo, I., Pérez, M. Á, Terreros, G., Muñoz, P., and Dagnino-Subiabre, A. (2014). Effects of chronic stress in adolescence on learned fear, anxiety, and synaptic transmission in the rat prelimbic cortex. Behav. Brain Res. 259, 342–353.
Nemeroff, C. B. (2007). Prevalence and management of treatment-resistant depression. J. Clin. Psychiatry 68, 17–25. doi: 10.4088/JCP.0707e17
Nezlek, J. B., Imbrie, M., and Shean, G. D. (1994). Depression and everyday social interaction. J. Pers. Soc. Psychol. 67, 1101–1111. doi: 10.1037/0022-3514.67.6.1101
Nieuwenhuis, I. L. C., and Takashima, A. (2010). The role of the ventromedial prefrontal cortex in memory consolidation. Behav. Brain Res. 218, 325–334. doi: 10.1016/j.bbr.2010.12.009
O’Neill, P.-K., Gordon, J., and Sigurdsson, T. (2013). Theta oscillations in the medial prefrontal cortex are modulated by spatial working memory and synchronize with the hippocampus through its ventral subregion. J. Neurosci. 33, 14211–14224. doi: 10.1523/JNEUROSCI.2378-13.2013
Oberto, V. J., Boucly, C. J., Gao, H. Y., Todorova, R., Zugaro, M. B., and Wiener, S. I. (2022). Distributed cell assemblies spanning prefrontal cortex and striatum. Curr. Biol. 32:1-13.e6. doi: 10.1016/j.cub.2021.10.007
Okonogi, T., and Sasaki, T. (2021). Theta-range oscillations in stress-induced mental disorders as an oscillotherapeutic target. Front. Behav. Neurosci. 15:698753. doi: 10.3389/fnbeh.2021.698753
Oliveira, J. F., Dias, N. S., Correia, M., Gama-Pereira, F., Sardinha, V. M., Lima, A., et al. (2013). Chronic stress disrupts neural coherence between cortico-limbic structures. Front. Neural Circ. 7:10. doi: 10.3389/fncir.2013.00010
Olsen, G. M., Hovde, K., Kondo, H., Sakshaug, T., Sømme, H. H., Whitlock, J. R., et al. (2019). Organization of posterior parietal-frontal connections in the rat. Front. Syst. Neurosci. 13:38. doi: 10.3389/fnsys.2019.00038
Olson, J. M., Li, J. K., Montgomery, S. E., and Nitz, D. A. (2020). Secondary motor cortex transforms spatial information into planned action during navigation. Curr. Biol. 30:1845-1854.e4. doi: 10.1016/j.cub.2020.03.016
Orozco-Cabal, L., Pollandt, S., Liu, J., Vergara, L., Shinnick-Gallagher, P., and Gallagher, J. P. (2006). A novel rat medial prefrontal cortical slice preparation to investigate synaptic transmission from amygdala to layer V prelimbic pyramidal neurons. J. Neurosci. Methods 151, 148–158. doi: 10.1016/j.jneumeth.2005.07.002
Ortiz, C., Navarro, J. F., Jurek, A., Märtin, A., Lundeberg, J., and Meletis, K. (2020). Molecular atlas of the adult mouse brain. Sci. Adv. 6:eabb3446. doi: 10.1126/sciadv.abb3446
Oyanedel, C. N., Durán, E., Niethard, N., Inostroza, M., and Born, J. (2020). Temporal associations between sleep slow oscillations, spindles and ripples. Eur. J. Neurosci. 52, 4762–4778. doi: 10.1111/ejn.14906
Papale, A. E., Zielinski, M. C., Frank, L. M., Jadhav, S. P., and Redish, A. D. (2016). Interplay between hippocampal sharp-wave-ripple events and vicarious trial and error behaviors in decision making. Neuron 92, 975–982. doi: 10.1016/j.neuron.2016.10.028
Pape, H. C., Narayanan, R. T., Smid, J., Stork, O., and Seidenbecher, T. (2005). Theta activity in neurons and networks of the amygdala related to long-term fear memory. Hippocampus 15, 874–880. doi: 10.1002/hipo.20120
Parnaudeau, S., O’Neill, P. K., Bolkan, S. S., Ward, R. D., Abbas, A. I., Roth, B. L., et al. (2013). Inhibition of mediodorsal thalamus disrupts thalamofrontal connectivity and cognition. Neuron 77, 1151–1162. doi: 10.1016/j.neuron.2013.01.038
Patrono, E., Hrůzova, K., Svoboda, J., and Stuchlík, A. (2023). The role of optogenetic stimulations of parvalbumin-positive interneurons in the prefrontal cortex and the ventral hippocampus on an acute MK-801 model of schizophrenia-like cognitive inflexibility. Schizophr. Res. 252, 198–205. doi: 10.1016/j.schres.2022.12.047
Paxton, J. L., Barch, D. M., Racine, C. A., and Braver, T. S. (2008). Cognitive control, goal maintenance, and prefrontal function in healthy aging. Cereb. Cortex 18, 1010–1028. doi: 10.1093/cercor/bhm135
Peeters, B. W., Kerbusch, J. M., Coenen, A. M., Vossen, J. M., and van Luijtelaar, E. L. (1992). Genetics of spike-wave discharges in the electroencephalogram (EEG) of the WAG/Rij inbred rat strain: A classical Mendelian crossbreeding study. Behav. Genet. 22, 361–368. doi: 10.1007/BF01066667
Penner, J., Ford, K. A., Taylor, R., Schaefer, B., Théberge, J., Neufeld, R. W. J., et al. (2016). Medial prefrontal and anterior insular connectivity in early schizophrenia and major depressive disorder: A resting functional MRI evaluation of large-scale brain network models. Front. Hum. Neurosci. 10:132. doi: 10.3389/fnhum.2016.00132
Pérez, M. Á, Morales, C., Santander, O., García, F., Gómez, I., Peñaloza-Sancho, V., et al. (2019). Ketamine-treatment during late adolescence impairs inhibitory synaptic transmission in the prefrontal cortex and working memory in adult rats. Front. Cell. Neurosci. 13:372. doi: 10.3389/fncel.2019.00372
Perlstein, W. M., Carter, C. S., Noll, D. C., and Cohen, J. D. (2001). Relation of prefrontal cortex dysfunction to working memory and symptoms in schizophrenia. Am. J. Psychiatry 158, 1105–1113. doi: 10.1176/appi.ajp.158.7.1105
Perlstein, W. M., Dixit, N. K., Carter, C. S., Noll, D. C., and Cohen, J. D. (2003). Prefrontal cortex dysfunction mediates deficits in working memory and prepotent responding in schizophrenia. Biol. Psychiatry 53, 25–38. doi: 10.1016/s0006-3223(02)01675-x
Pesaran, B., Vinck, M., Einevoll, G. T., Sirota, A., Fries, P., Siegel, M., et al. (2018). Investigating large-scale brain dynamics using field potential recordings: Analysis and interpretation. Nat. Neurosci. 21, 903–919. doi: 10.1038/s41593-018-0171-8
Peters, J., Kalivas, P. W., and Quirk, G. J. (2009). Extinction circuits for fear and addiction overlap in prefrontal cortex. Learn. Mem. 16, 279–288. doi: 10.1101/lm.1041309
Peyrache, A., Khamassi, M., Benchenane, K., Wiener, S. I., and Battaglia, F. P. (2009). Replay of rule-learning related neural patterns in the prefrontal cortex during sleep. Nat. Neurosci. 12, 919–926. doi: 10.1038/nn.2337
Pezzulo, G., van der Meer, M. A. A., Lansink, C. S., and Pennartz, C. M. A. (2014). Internally generated sequences in learning and executing goal-directed behavior. Trends Cogn. Sci. 18, 647–657. doi: 10.1016/j.tics.2014.06.011
Pietrasik, W., Cribben, I., Olsen, F., and Malykhin, N. (2023). Diffusion tensor imaging of superficial prefrontal white matter in healthy aging. Brain Res. 1799:148152. doi: 10.1016/j.brainres.2022.148152
Pinault, D. (2008). N-methyl d-aspartate receptor antagonists ketamine and MK-801 induce wake-related aberrant gamma oscillations in the rat neocortex. Biol. Psychiatry 63, 730–735. doi: 10.1016/j.biopsych.2007.10.006
Place, R., Farovik, A., Brockmann, M., and Eichenbaum, H. (2016). Bidirectional prefrontal-hippocampal interactions support context-guided memory. Nat. Neurosci. 19, 992–994. doi: 10.1038/nn.4327
Pomarol-Clotet, E., Canales-Rodríguez, E. J., Salvador, R., Sarró, S., Gomar, J. J., Vila, F., et al. (2010). Medial prefrontal cortex pathology in schizophrenia as revealed by convergent findings from multimodal imaging. Mol. Psychiatry 15, 823–830. doi: 10.1038/mp.2009.146
Popa, D., Duvarci, S., Popescu, A. T., Léna, C., and Paré, D. (2010). Coherent amygdalocortical theta promotes fear memory consolidation during paradoxical sleep. Proc. Natl. Acad. Sci. U.S.A. 107, 6516–6519. doi: 10.1073/pnas.0913016107
Powell, N. J., and Redish, A. D. (2016). Representational changes of latent strategies in rat medial prefrontal cortex precede changes in behaviour. Nat. Commun. 7, 1–11. doi: 10.1038/ncomms12830
Preston, A. R., and Eichenbaum, H. (2013). Interplay of hippocampus and prefrontal cortex in memory. Curr. Biol. 23, R764–R773. doi: 10.1016/j.cub.2013.05.041
Proskovec, A. L., Wiesman, A. I., and Wilson, T. W. (2019). The strength of alpha and gamma oscillations predicts behavioral switch costs. Neuroimage 188, 274–281. doi: 10.1016/j.neuroimage.2018.12.016
Ragozzino, M. E., and Kesner, R. P. (2001). The role of rat dorsomedial prefrontal cortex in working memory for egocentric responses. Neurosci. Lett. 308, 145–148. doi: 10.1016/s0304-3940(01)02020-1
Rapp, P. R., Rosenberg, R. A., and Gallagher, M. (1987). An evaluation of spatial information processing in aged rats. Behav. Neurosci. 101, 3–12. doi: 10.1037//0735-7044.101.1.3
Redish, A. D. (2016). Vicarious trial and error. Nat. Rev. Neurosci. 17, 147–159. doi: 10.1038/nrn.2015.30
Regenold, W. T., Deng, Z.-D., and Lisanby, S. H. (2022). Noninvasive neuromodulation of the prefrontal cortex in mental health disorders. Neuropsychopharmacology 47, 361–372. doi: 10.1038/s41386-021-01094-3
Reinhart, R. M. G., and Nguyen, J. A. (2019). Working memory revived in older adults by synchronizing rhythmic brain circuits. Nat. Neurosci. 22, 820–827. doi: 10.1038/s41593-019-0371-x
Remijnse, P. L., Nielen, M. M. A., Uylings, H. B. M., and Veltman, D. J. (2005). Neural correlates of a reversal learning task with an affectively neutral baseline: An event-related fMRI study. Neuroimage 26, 609–618. doi: 10.1016/j.neuroimage.2005.02.009
Riaz, S., Puveendrakumaran, P., Khan, D., Yoon, S., Hamel, L., and Ito, R. (2019). Prelimbic and infralimbic cortical inactivations attenuate contextually driven discriminative responding for reward. Sci. Rep. 9:3982. doi: 10.1038/s41598-019-40532-7
Rich, E. L., and Shapiro, M. (2009). Rat prefrontal cortical neurons selectively code strategy switches. J. Neurosci. 29, 7208–7219. doi: 10.1523/JNEUROSCI.6068-08.2009
Richard’s, M. M., Krzemien, D., Valentina, V., Vernucci, S., Zamora, E. V., Comesaña, A., et al. (2021). Cognitive flexibility in adulthood and advanced age: Evidence of internal and external validity. Appl. Neuropsychol. 28, 464–478. doi: 10.1080/23279095.2019.1652176
Richards, B. A., Xia, F., Santoro, A., Husse, J., Woodin, M. A., Josselyn, S. A., et al. (2014). Patterns across multiple memories are identified over time. Nat. Neurosci. 17, 981–986. doi: 10.1038/nn.3736
Ridley, R. M. (1994). The psychology of perserverative and stereotyped behaviour. Progr. Neurobiol. 44, 221–231. doi: 10.1016/0301-0082(94)90039-6
Riga, D., Matos, M. R., Glas, A., Smit, A. B., Spijker, S., and Van den Oever, M. C. (2014). Optogenetic dissection of medial prefrontal cortex circuitry. Front. Syst. Neurosci. 8:230. doi: 10.3389/fnsys.2014.00230
Rodgers, M. K., Sindone, J. A. III, and Moffat, S. D. (2012). Effects of age on navigation strategy. Neurobiol. Aging 33:202.e15-22. doi: 10.1016/j.neurobiolaging.2010.07.021
Rondina, R., Olsen, R. K., McQuiggan, D. A., Fatima, Z., Li, L., Oziel, E., et al. (2016). Age-related changes to oscillatory dynamics in hippocampal and neocortical networks. Neurobiol. Learn. Mem. 134, 15–30. doi: 10.1016/j.nlm.2015.11.017
Rosanova, M., Casali, A., Bellina, V., Resta, F., Mariotti, M., and Massimini, M. (2009). Natural frequencies of human corticothalamic circuits. J. Neurosci. 29, 7679–7685. doi: 10.1523/JNEUROSCI.0445-09.2009
Rose, J. E., and Woolsey, C. N. (1948). The orbitofrontal cortex and its connections with the mediodorsal nucleus in rabbit, sheep and cat. Res. Public. Assoc. Res. Nervous Ment. Dis. 27, 210–232.
Rothschild, G., Eban, E., and Frank, L. M. (2017). A cortical-hippocampal-cortical loop of information processing during memory consolidation. Nat. Neurosci. 20, 251–259. doi: 10.1038/nn.4457
Ruediger, S., Spirig, D., Donato, F., and Caroni, P. (2012). Goal-oriented searching mediated by ventral hippocampus early in trial-and-error learning. Nat. Neurosci. 15, 1563–1571. doi: 10.1038/nn.3224
Rumschlag, J. A., Lovelace, J. W., and Razak, K. A. (2021). Age- and movement-related modulation of cortical oscillations in a mouse model of presbycusis. Hear. Res. 402:108095. doi: 10.1016/j.heares.2020.108095
Rutter, L., Carver, F. W., Holroyd, T., Nadar, S. R., Mitchell-Francis, J., Apud, J., et al. (2009). Magnetoencephalographic gamma power reduction in patients with schizophrenia during resting condition. Hum. Brain Mapp. 30, 3254–3264. doi: 10.1002/hbm.20746
Sakurai, Y. (1999). How do cell assemblies encode information in the brain? Neurosci. Biobehav. Rev. 23:785. doi: 10.1016/S0149-7634(99)00017-2
Sakurai, Y., Nakazono, T., Ishino, S., Terada, S., Yamaguchi, K., and Takahashi, S. (2013). Diverse synchrony of firing reflects diverse cell-assembly coding in the prefrontal cortex. J. Physiol. Paris 107, 459–447. doi: 10.1016/j.jphysparis.2013.05.004
Salthouse, T. A. (2019). Trajectories of normal cognitive aging. Psychol. Aging 34, 17–24. doi: 10.1037/pag0000288
Sanchez-Carpintero, R., Urrestarazu, E., Cieza, S., Alegre, M., Artieda, J., Crespo-Eguilaz, N., et al. (2020). Abnormal brain gamma oscillations in response to auditory stimulation in Dravet syndrome. Eur. J. Paediatr. Neurol. 24, 134–141. doi: 10.1016/j.ejpn.2019.12.004
Sánchez-García, S., García-Peña, C., Ramírez-García, E., Moreno-Tamayo, K., and Cantú-Quintanilla, G. R. (2019). Decreased autonomy in community-dwelling older adults. Clin. Interv. Aging 14, 2041–2053. doi: 10.2147/CIA.S225479
Sardinha, V. M., Guerra-Gomes, S., Caetano, I., Tavares, G., Martins, M., Reis, J. S., et al. (2017). Astrocytic signaling supports hippocampal-prefrontal theta synchronization and cognitive function. Glia 65, 1944–1960. doi: 10.1002/glia.23205
Sauer, J.-F., and Bartos, M. (2022). Disrupted-in-schizophrenia-1 is required for normal pyramidal cell-interneuron communication and assembly dynamics in the prefrontal cortex. Elife 11:e79471. doi: 10.7554/eLife.79471
Schlesiger, M. I., Cannova, C. C., Boublil, B. L., Hales, J. B., Mankin, E. A., Brandon, M. P., et al. (2015). The medial entorhinal cortex is necessary for temporal organization of hippocampal neuronal activity. Nat. Neurosci. 18, 1123–1132. doi: 10.1038/nn.4056
Schlichting, M. L., and Preston, A. R. (2015). Memory integration: Neural mechanisms and implications for behavior. Curr. Opin. Behav. Sci. 1, 1–8. doi: 10.1016/j.cobeha.2014.07.005
Schmiedt, C., Brand, A., Hildebrandt, H., and Basar-Eroglu, C. (2005). Event-related theta oscillations during working memory tasks in patients with schizophrenia and healthy controls. Cogn. Brain Res. 25, 936–947. doi: 10.1016/j.cogbrainres.2005.09.015
Schwabe, L., Dalm, S., Schächinger, H., and Oitzl, M. S. (2008). Chronic stress modulates the use of spatial and stimulus-response learning strategies in mice and man. Neurobiol. Learn. Mem. 90, 495–503. doi: 10.1016/j.nlm.2008.07.015
Selemon, L. D., Rajkowska, G., and Goldman-Rakic, P. S. (2004). Evidence for progression in frontal cortical pathology in late-stage Huntington’s disease. J. Comp. Neurol. 468, 190–204. doi: 10.1002/cne.10938
Senkowski, D., and Gallinat, J. (2015). Dysfunctional prefrontal gamma-band oscillations reflect working memory and other cognitive deficits in schizophrenia. Biol. Psychiatry 77, 1010–1101. doi: 10.1016/j.biopsych.2015.02.034
Seo, D.-O., Funderburk, S. C., Bhatti, D. L., Motard, L. E., Newbold, D., Girven, K. S., et al. (2016). A GABAergic projection from the centromedial nuclei of the amygdala to ventromedial prefrontal cortex modulates reward behavior. J. Neurosci. 36, 10831–10842. doi: 10.1523/JNEUROSCI.1164-16.2016
Shemesh, Y., and Chen, A. (2023). A paradigm shift in translational psychiatry through rodent neuroethology. Mol. Psychiatry 28, 993–1003. doi: 10.1038/s41380-022-01913-z
Shenton, M. E., Dickey, C. C., Frumin, M., and McCarley, R. W. (2001). A review of MRI findings in schizophrenia. Schizophr. Res. 49, 1–52. doi: 10.1016/s0920-9964(01)00163-3
Shepherd, G. M. G., and Yamawaki, N. (2021). Untangling the cortico-thalamo-cortical loop: Cellular pieces of a knotty circuit puzzle. Nat. Rev. Neurosci. 22, 389–406. doi: 10.1038/S41583-021-00459-3
Shin, J. D., Tang, W., and Jadhav, S. P. (2019). Dynamics of awake hippocampal-prefrontal replay for spatial learning and memory-guided decision making. Neuron 104:1110-1125.e7. doi: 10.1016/j.neuron.2019.09.012
Siapas, A. G., Lubenov, E. V., and Wilson, M. A. (2005). Prefrontal phase locking to hippocampal theta oscillations. Neuron 46, 141–151. doi: 10.1016/j.neuron.2005.02.028
Sierra-Mercado, D., Padilla-Coreano, N., and Quirk, G. J. (2011). Dissociable roles of prelimbic and infralimbic cortices, ventral hippocampus, and basolateral amygdala in the expression and extinction of conditioned fear. Neuropsychopharmacology 36, 529–538. doi: 10.1038/npp.2010.184
Sigurdsson, T., Stark, K. L., Karayiorgou, M., Gogos, J. A., and Gordon, J. A. (2010). Impaired hippocampal-prefrontal synchrony in a genetic mouse model of schizophrenia. Nature 464, 763–767. doi: 10.1038/nature08855
Sirota, A., Montgomery, S., Fujisawa, S., Isomura, Y., Zugaro, M., and Buzsáki, G. (2008). Entrainment of neocortical neurons and gamma oscillations by the hippocampal theta rhythm. Neuron 60, 683–697. doi: 10.1016/j.neuron.2008.09.014
Smucny, J., Dienel, S. J., Lewis, D. A., and Carter, C. S. (2022). Mechanisms underlying dorsolateral prefrontal cortex contributions to cognitive dysfunction in schizophrenia. Neuropsychopharmacology 47, 292–308. doi: 10.1038/s41386-021-01089-0
Sohal, V. S., Zhang, F., Yizhar, O., and Deisseroth, K. (2009). Parvalbumin neurons and gamma rhythms enhance cortical circuit performance. Nature 459, 698–702. doi: 10.1038/nature07991
Solomon, D. A., Keller, M. B., Leon, A. C., Mueller, T. I., Lavori, P. W., Shea, M. T., et al. (2000). Multiple recurrences of major depressive disorder. Am. J. Psychiatry 157, 229–233. doi: 10.1176/appi.ajp.157.2.229
Speers, L. J., and Bilkey, D. K. (2021). Disorganization of oscillatory activity in animal models of schizophrenia. Front. Neural Circ. 15:741767. doi: 10.3389/fncir.2021.741767
Spencer, K. M. (2011). Baseline gamma power during auditory steady-state stimulation in schizophrenia. Front. Hum. Neurosci. 5:190. doi: 10.3389/fnhum.2011.00190
Sreekumar, V., Wittig, J. H. J., Sheehan, T. C., and Zaghloul, K. A. (2017). Principled approaches to direct brain stimulation for cognitive enhancement. Front. Neurosci. 11:650. doi: 10.3389/fnins.2017.00650
Stuber, G. D., and Wise, R. A. (2016). Lateral hypothalamic circuits for feeding and reward. Nat. Neurosci. 19, 198–205. doi: 10.1038/nn.4220
Stujenske, J. M., Likhtik, E., Topiwala, M. A., and Gordon, J. A. (2014). Fear and safety engage competing patterns of theta-gamma coupling in the basolateral amygdala. Neuron 83, 919–933. doi: 10.1016/j.neuron.2014.07.026
Takao, H., Hayashi, N., and Ohtomo, K. (2012). A longitudinal study of brain volume changes in normal aging. Eur. J. Radiol. 81, 2801–2804. doi: 10.1016/j.ejrad.2011.10.011
Tamura, M., Spellman, T. J., Rosen, A. M., Gogos, J. A., and Gordon, J. A. (2017). Hippocampal-prefrontal theta-gamma coupling during performance of a spatial working memory task. Nat. Commun. 8:2182. doi: 10.1038/s41467-017-02108-9
Tang, W., Shin, J. D., Frank, L. M., and Jadhav, S. P. (2017). Hippocampal-prefrontal reactivation during learning is stronger in awake compared with sleep states. J. Neurosci. 37, 11789–11805. doi: 10.1523/JNEUROSCI.2291-17.2017
Taub, A. H., Perets, R., Kahana, E., and Paz, R. (2018). Oscillations synchronize amygdala-to-prefrontal primate circuits during aversive learning. Neuron 97:291-298.e3. doi: 10.1016/j.neuron.2017.11.042
Taylor Tavares, J. V., Clark, L., Cannon, D. M., Erickson, K., Drevets, W. C., and Sahakian, B. J. (2007). Distinct profiles of neurocognitive function in unmedicated unipolar depression and bipolar II depression. Biol. Psychiatry 62, 917–924. doi: 10.1016/j.biopsych.2007.05.034
Taylor, E. N., Huang, N., Wisco, J., Wang, Y., Morgan, K. G., and Hamilton, J. A. (2020). The brains of aged mice are characterized by altered tissue diffusion properties and cerebral microbleeds. J. Transl. Med. 18, 1–11. doi: 10.1186/s12967-020-02441-6
Uddin, L. Q. (2021). Cognitive and behavioural flexibility: Neural mechanisms and clinical considerations. Nat. Rev. Neurosci. 22, 167–179. doi: 10.1038/s41583-021-00428-w
Uhlhaas, P. J., and Singer, W. (2010). Abnormal neural oscillations and synchrony in schizophrenia. Nat. Rev. Neurosci. 11, 100–113. doi: 10.1038/nrn2774
Uhlhaas, P. J., and Singer, W. (2013). High-frequency oscillations and the neurobiology of schizophrenia. Dialog. Clin. Neurosci. 15, 301–313. doi: 10.31887/DCNS.2013.15.3/puhlhaas
Uylings, H. B. M., Groenewegen, H. J., and Kolb, B. (2003). Do rats have a prefrontal cortex? Behav. Brain Res. 146, 3–17. doi: 10.1016/j.bbr.2003.09.028
van Aerde, K. I., Heistek, T. S., and Mansvelder, H. D. (2008). Prelimbic and infralimbic prefrontal cortex interact during fast network oscillations. PLoS One 3:e2725. doi: 10.1371/journal.pone.0002725
van Beijsterveldt, C. E., Molenaar, P. C., de Geus, E. J., and Boomsma, D. I. (1996). Heritability of human brain functioning as assessed by electroencephalography. Am. J. Hum. Genet. 58, 562–573.
Vann, S. D., Aggleton, J. P., and Maguire, E. A. (2009). What does the retrosplenial cortex do? Nat. Rev. Neurosci. 10, 792–802. doi: 10.1038/nrn2733
Veit, J., Hakim, R., Jadi, M. P., Sejnowski, T. J., and Adesnik, H. (2017). Cortical gamma band synchronization through somatostatin interneurons. Nat. Neurosci. 20, 951–959. doi: 10.1038/nn.4562
Vertes, R. P. (2004). Differential projections of the infralimbic and prelimbic cortex in the rat. Synapse 51, 32–58. doi: 10.1002/syn.10279
Vertes, R. P., Hoover, W. B., Szigeti-Buck, K., and Leranth, C. (2007). Nucleus reuniens of the midline thalamus: Link between the medial prefrontal cortex and the hippocampus. Brain Res. Bull. 71, 601–609. doi: 10.1016/j.brainresbull.2006.12.002
Vialou, V., Bagot, R. C., Cahill, M. E., Ferguson, D., Robison, A. J., Dietz, D. M., et al. (2014). Prefrontal cortical circuit for depression- and anxiety-related behaviors mediated by cholecystokinin: Role of ΔFosB. J. Neurosci. 34, 3878–3887. doi: 10.1523/JNEUROSCI.1787-13.2014
Vlahou, E. L., Thurm, F., Kolassa, I. T., and Schlee, W. (2014). Resting-state slow wave power, healthy aging and cognitive performance. Sci. Rep. 4, 33–36. doi: 10.1038/srep05101
Volk, D. W., Austin, M. C., Pierri, J. N., Sampson, A. R., and Lewis, D. A. (2000). Decreased glutamic acid decarboxylase67 messenger RNA expression in a subset of prefrontal cortical gamma-aminobutyric acid neurons in subjects with schizophrenia. Arch. Gen. Psychiatry 57, 237–245.
Voloh, B., Valiante, T. A., Everling, S., and Womelsdorf, T. (2015). Theta-gamma coordination between anterior cingulate and prefrontal cortex indexes correct attention shifts. Proc. Natl. Acad. Sci. U.S.A. 112, 8457–8462. doi: 10.1073/pnas.1500438112
Wagner, I. C., van Buuren, M., and Fernández, G. (2019). Thalamo-cortical coupling during encoding and consolidation is linked to durable memory formation. Neuroimage 197, 80–92. doi: 10.1016/j.neuroimage.2019.04.055
Waltz, J. A. (2017). The neural underpinnings of cognitive flexibility and their disruption in psychotic illness. Neuroscience 345, 203–217. doi: 10.1016/j.neuroscience.2016.06.005
Wamsley, E. J. (2019). Memory consolidation during waking rest. Trends Cogn. Sci. 23, 171–173. doi: 10.1016/j.tics.2018.12.007
Wang, C., Chen, X., and Knierim, J. J. (2020). Egocentric and allocentric representations of space in the rodent brain. Curr. Opin. Neurobiol. 60, 12–20. doi: 10.1016/j.conb.2019.11.005
Wang, Q., Timberlake, M. A. II, Prall, K., and Dwivedi, Y. (2017). The recent progress in animal models of depression. Progr. Neuro Psychopharmacol. Biol. Psychiatry 77, 99–109. doi: 10.1016/j.pnpbp.2017.04.008
Warden, M. R., Selimbeyoglu, A., Mirzabekov, J. J., Lo, M., Thompson, K. R., Kim, S.-Y., et al. (2012). A prefrontal cortex-brainstem neuronal projection that controls response to behavioural challenge. Nature 492, 428–432. doi: 10.1038/nature11617
Wegener, J. G., and Stamm, J. S. (1966). Behavior flexibility and the frontal lobes. Cortex 2, 188–201. doi: 10.1016/S0010-9452(66)80002-3
West, R. L. (1996). An application of prefrontal cortex function theory to cognitive aging. Psychol. Bull. 120, 272–292. doi: 10.1037/0033-2909.120.2.272
Whitlock, J. R., Sutherland, R. J., Witter, M. P., Moser, M. B., and Moser, E. I. (2008). Navigating from hippocampus to parietal cortex. Proc. Natl. Acad. Sci. U.S.A. 105, 14755–14762. doi: 10.1073/pnas.0804216105
Wiener, J. M., de Condappa, O., Harris, M. A., and Wolbers, T. (2013). Maladaptive bias for extrahippocampal navigation strategies in aging humans. J. Neurosci. 33, 6012–6017. doi: 10.1523/JNEUROSCI.0717-12.2013
Wilber, A. A., Walker, A. G., Southwood, C. J., Farrell, M. R., Lin, G. L., Rebec, G. V., et al. (2011). Chronic stress alters neural activity in medial prefrontal cortex during retrieval of extinction. Neuroscience 174, 115–131. doi: 10.1016/j.neuroscience.2010.10.070
Wiles, N., Thomas, L., Abel, A., Barnes, M., Carroll, F., Ridgway, N., et al. (2014). Clinical effectiveness and cost-effectiveness of cognitive behavioural therapy as an adjunct to pharmacotherapy for treatment-resistant depression in primary care: The CoBalT randomised controlled trial. Health Technol. Assess. 18, 1–167. doi: 10.3310/hta18310
Winship, I. R., Dursun, S. M., Baker, G. B., Balista, P. A., Kandratavicius, L., Maia-de-Oliveira, J. P., et al. (2019). An overview of animal models related to schizophrenia. Can. J. Psychiatry 64, 5–17. doi: 10.1177/0706743718773728
Wolkin, A., Choi, S. J., Szilagyi, S., Sanfilipo, M., Rotrosen, J. P., and Lim, K. O. (2003). Inferior frontal white matter anisotropy and negative symptoms of schizophrenia: A diffusion tensor imaging study. Am. J. Psychiatry 160, 572–574. doi: 10.1176/appi.ajp.160.3.572
World Health Organization (2012). World health statistics 2012, Vol. 27. Geneva: World Health Organization, doi: 10.2307/3348165
Yagi, A., Nouchi, R., Murayama, K., Sakaki, M., and Kawashima, R. (2020). The role of cognitive control in age-related changes in well-being. Front. Aging Neurosci. 12:198. doi: 10.3389/fnagi.2020.00198
Yanai, S., and Endo, S. (2021). Functional aging in male C57BL/6J mice across the life-span: A systematic behavioral analysis of motor, emotional, and memory function to define an aging phenotype. Front. Aging Neurosci. 13:697621. doi: 10.3389/fnagi.2021.697621
Yang, M., Logothetis, N. K., and Eschenko, O. (2019). Occurrence of hippocampal ripples is associated with activity suppression in the mediodorsal thalamic nucleus. J. Neurosci. 39, 434–444. doi: 10.1523/JNEUROSCI.2107-18.2018
Yanovsky, Y., Ciatipis, M., Draguhn, A., Tort, A. B. L., and Brankačk, J. (2014). Slow oscillations in the mouse hippocampus entrained by nasal respiration. J. Neurosci. 34, 5949–5964. doi: 10.1523/JNEUROSCI.5287-13.2014
Ye, L., Allen, W. E., Thompson, K. R., Tian, Q., Hsueh, B., Ramakrishnan, C., et al. (2016). Wiring and molecular features of prefrontal ensembles representing distinct experiences. Cell 165, 1776–1788. doi: 10.1016/j.cell.2016.05.010
Yener, G. G., and Başar, E. (2013). Brain oscillations as biomarkers in neuropsychiatric disorders: Following an interactive panel discussion and synopsis. Suppl. Clin. Neurophysiol. 62, 343–363. doi: 10.1016/B978-0-7020-5307-8.00016-8
Yuen, E. Y., Wei, J., Liu, W., Zhong, P., Li, X., and Yan, Z. (2012). Repeated stress causes cognitive impairment by suppressing glutamate receptor expression and function in prefrontal cortex. Neuron 73, 962–977. doi: 10.1016/j.neuron.2011.12.033
Zeng, H., Shen, E. H., Hohmann, J. G., Oh, S. W., Bernard, A., Royall, J. J., et al. (2012). Large-scale cellular-resolution gene profiling in human neocortex reveals species-specific molecular signatures. Cell 149, 483–496. doi: 10.1016/j.cell.2012.02.052
Zhang, Y., Zhu, C., Chen, H., Duan, X., Lu, F., Li, M., et al. (2015). Frequency-dependent alterations in the amplitude of low-frequency fluctuations in social anxiety disorder. J. Affect. Disord. 174, 329–335. doi: 10.1016/j.jad.2014.12.001
Zheng, C., and Zhang, T. (2013). Alteration of phase-phase coupling between theta and gamma rhythms in a depression-model of rats. Cogn. Neurodyn. 7, 167–172. doi: 10.1007/s11571-012-9225-x
Zheng, F., Liu, Y., Yuan, Z., Gao, X., He, Y., Liu, X., et al. (2019). Age-related changes in cortical and subcortical structures of healthy adult brains: A surface-based morphometry study. J. Magnet. Reson. Imaging 49, 152–163. doi: 10.1002/jmri.26037
Zhong, J. Y., and Moffat, S. D. (2018). Extrahippocampal contributions to age-related changes in spatial navigation ability. Front. Hum. Neurosci. 12:272. doi: 10.3389/fnhum.2018.00272
Zhong, W., Ciatipis, M., Wolfenstetter, T., Jessberger, J., Müller, C., Ponsel, S., et al. (2017). Selective entrainment of gamma subbands by different slow network oscillations. Proc. Natl. Acad. Sci. U.S.A. 114, 4519–4524. doi: 10.1073/pnas.1617249114
Zonneveld, H. I., Pruim, R. H., Bos, D., Vrooman, H. A., Muetzel, R. L., Hofman, A., et al. (2019). Patterns of functional connectivity in an aging population: The Rotterdam study. Neuroimage 189, 432–444. doi: 10.1016/j.neuroimage.2019.01.041
Zrenner, B., Zrenner, C., Gordon, P. C., Belardinelli, P., McDermott, E. J., Soekadar, S. R., et al. (2020). Brain oscillation-synchronized stimulation of the left dorsolateral prefrontal cortex in depression using real-time EEG-triggered TMS. Brain Stimul. 13, 197–205. doi: 10.1016/j.brs.2019.10.007
Keywords: cognitive control, prefrontal cortex, brain oscillations, functional connectivity, rodent models
Citation: Negrón-Oyarzo I, Dib T, Chacana-Véliz L, López-Quilodrán N and Urrutia-Piñones J (2024) Large-scale coupling of prefrontal activity patterns as a mechanism for cognitive control in health and disease: evidence from rodent models. Front. Neural Circuits 18:1286111. doi: 10.3389/fncir.2024.1286111
Received: 30 August 2023; Accepted: 11 March 2024;
Published: 04 April 2024.
Edited by:
Cristina Martins E. Silva, Universidade Federal do Espírito Santo, BrazilReviewed by:
Owen Chao, University of Minnesota, United StatesSusheel Vijayraghavan, Western University, Canada
Copyright © 2024 Negrón-Oyarzo, Dib, Chacana-Véliz, López-Quilodrán, and Urrutia-Piñones. This is an open-access article distributed under the terms of the Creative Commons Attribution License (CC BY). The use, distribution or reproduction in other forums is permitted, provided the original author(s) and the copyright owner(s) are credited and that the original publication in this journal is cited, in accordance with accepted academic practice. No use, distribution or reproduction is permitted which does not comply with these terms.
*Correspondence: Ignacio Negrón-Oyarzo, ignacio.negron@uv.cl