Effects of continuous versus intermittent theta-burst TMS on fMRI connectivity
- Department of Psychology, Florida State University, Tallahassee, FL, United States
Transcranial magnetic stimulation is a noninvasive technique that can be used to evoke distributed network-level effects. Previous work demonstrated that the Hippocampal-Cortical Network responds preferably (i.e., greater memory improvement and increases in hippocampal-network connectivity) to continuous theta-burst stimulation protocol relative to intermittent theta-burst and to 20-Hz rTMS. Here, these data were further analyzed to characterize effects of continuous versus intermittent theta-burst stimulation on network-level connectivity measures – as well as local connectedness – via resting-state fMRI. In contrast to theories that propose continuous and intermittent theta-burst cause local inhibitory versus excitatory effects, respectively, both protocols caused local decreases in fMRI connectivity around the stimulated parietal site. While iTBS caused decreases in connectivity across the hippocampal-cortical network, cTBS caused increases and decreases in connectivity across the network. cTBS had no effect on the parietal-cortical network, whereas iTBS caused decreases in the right parietal cortex (contralateral hemisphere to the stimulation target). These findings suggest that continuous theta-burst may have entrained the endogenous hippocampal-cortical network, whereas the intermittent train was unable to maintain entrainment that may have yielded the long-lasting effects measured in this study (i.e., within 20-min post-stimulation). Furthermore, these effects were specific to the hippocampal-cortical network, which has a putative endogenous functionally-relevant theta rhythm, and not to the parietal network. These results add to the growing body of evidence that suggests effects of theta-burst stimulation are not fully characterized by excitatory/inhibitory theories. Further work is required to understand local and network-level effects of noninvasive stimulation.
1 Introduction
Large-scale networks are often defined in human subjects as collections of discrete regions exhibiting temporally correlated activity, as measured by the blood-oxygen-level dependent signal in fMRI (i.e., “fMRI connectivity”). Functional properties of these networks can be probed noninvasively using transcranial magnetic stimulation (Fox et al., 2012), including frontal, occipital, and motor-cortical networks (Gratton et al., 2013; Rahnev et al., 2013; Mastropasqua et al., 2014; Valchev et al., 2015; Steel et al., 2016). The human hippocampal-cortical network (HCN) responds to repetitive stimulation delivered to parietal cortex (Eldaief et al., 2011; Halko et al., 2014), with long-lasting increases in fMRI connectivity throughout portions of the HCN following multi-day stimulation (Wang et al., 2014; Wang and Voss, 2015; Nilakantan et al., 2017; Kim et al., 2018; Warren et al., 2018; Freedberg et al., 2019; Warren et al., 2019; Hermiller et al., 2019b). These experiments have been important for causally testing the role of hippocampal-cortical networks in memory. However, mechanisms for network-level effects of rTMS are not fully understood. Some evidence suggests that certain rTMS protocols are capable of inducing trans-synaptic plasticity changes on functionally connected downstream regions (i.e., within a targeted brain network), modulating cognitive functions supported by these regions (Fox et al., 2012).
A previous study (Hermiller et al., 2019a, Hippocampus) evaluated the effectiveness of single sessions of different rTMS sequences at modulating HCN connectivity and memory retrieval. Specifically, this within-subjects design compared the effects of often used TMS protocols (e.g., continuous theta-burst, cTBS; intermittent theta-burst, iTBS; and 20-Hz rTMS) on memory-related hippocampal connectivity to other HCN regions, and found that the continuous theta-burst pattern caused the most robust increases in hippocampal connectivity and significantly enhanced memory retrieval (see Hermiller et al., 2019a, Hippocampus for details).
Notably, the theta-burst rhythm employed by the cTBS and iTBS protocols deliver bursts of gamma (50-Hz) triplets every 200-ms (5-Hz) in either continuous (cTBS) or intermittent (iTBS) trains (Huang et al., 2005; Rossi et al., 2009). TBS protocols were developed based on rodent and human studies indicating that theta rhythms are associated with long-term potentiation (LTP) (Berry and Thompson, 1978; Larson et al., 1986; Buzsaki, 2002). Lasting aftereffects on motor corticospinal output lasting between 30 and 60 min are generally reported for TBS protocols, with cTBS associated with inhibitory and iTBS associated with facilitatory cortico-motor effects (Pascual-Leone et al., 1994; Chen et al., 2003; Huang et al., 2005; Rossi et al., 2009; Di Lazzaro et al., 2011). However, effects of TBS in the motor cortex are not necessarily generalizable to other cortical areas. Notably, rTMS has been shown to evoke resonance-like in non-motor areas (Thut et al., 2011a,b; Chanes et al., 2013; Hanslmayr et al., 2014; Kim et al., 2016; Romei et al., 2016; Albouy et al., 2017; Lea-Carnall et al., 2017; Weinrich et al., 2017; Roberts et al., 2018; Riddle et al., 2019), including theta-patterned stimulation induced theta synchrony (Reinhart et al., 2015; Li et al., 2017). HCN regions exhibit neuronal synchrony and phase locking at theta-band frequencies (4-8-Hz) (Foster and Parvizi, 2012; Foster et al., 2013; Watrous et al., 2013; Foster et al., 2015), providing a possible mechanism for network communication and information processing (Buzsaki, 2002; Zhang and Jacobs, 2015). Theta-patterned stimulation of the rodent hippocampus preferentially induces long-term potentiation (Larson et al., 1986), which enhances connectivity of the hippocampal-cortical network (Canals et al., 2009). Therefore, theta-burst stimulation mimicking the “theta-nested gamma” activity pattern relevant to the HCN may optimally influence this network’s function via activity entrainment (i.e., resonance; see Thut et al. 2011a,b). Indeed, Hermiller et al. (2019a) found that the HCN responded preferentially to cTBS – but not iTBS or 20-Hz rTMS – leading to improved memory accuracy and increased memory-related hippocampal fMRI connectivity to other HCN regions. Here, we follow-up with this dataset to further evaluate the effects of continuous versus intermittent theta-burst stimulation on resting-state network connectedness, as well as local connectivity at the site of stimulation.
As previously reported (Hermiller et al., 2019a), stimulation was delivered to a parietal cortex location of the HCN, defined in each subject based on maximal fMRI connectivity with bilateral hippocampus (Figure 1). Notably, fMRI connectivity of the HCN is consistent with its known anatomical organization (Squire and Zola-Morgan, 1991; Suzuki and Amaral, 1994; Vincent et al., 2006; Buckner et al., 2008). This includes projections from parietal cortex to hippocampus via retrosplenial and parahippocampal cortex (Mesulam et al., 1977; Mufson and Pandya, 1984) that presumably allow parietal stimulation to affect downstream HCN locations (Fox et al., 2012; Wang et al., 2014). Subjects received cTBS, iTBS, 20-Hz, rTMS, and sham stimulation targeting the same individualized parietal target in separate sessions over different (nonconsecutive) days. After each stimulation session, a resting-state fMRI scan was acquired. The effects of stimulation was quantified using resting-state fMRI network connectedness analysis (Cole et al., 2010; Gotts et al., 2012; Steel et al., 2016; Warren et al., 2018), which identified areas where stimulation significantly affected mean fMRI connectivity with other network voxels relative to sham stimulation condition.
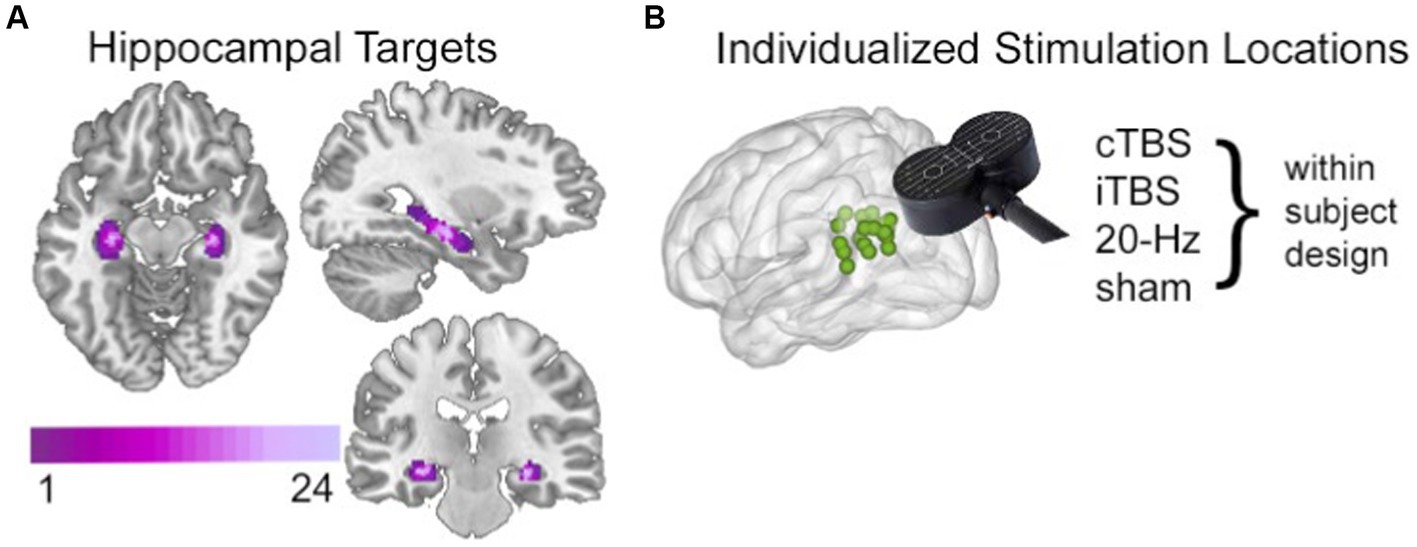
Figure 1. Targeting the HCN with noninvasive brain stimulation. (A) Hippocampal targets were defined as voxels with high fMRI interconnectivity within a bilateral anatomical mask of hippocampus. Targets are shown here as an overlap map across all subjects, with coloration indicating the number of subjects for which a given voxel was included as a hippocampal target. (B) The left parietal cortex stimulation locations were identified in each subject based on high fMRI connectivity with the subjectspecific hippocampal target, shown here as a green 2-mm sphere for each subject. A different stimulation condition was used in each experimental session in a within-subjects randomized counterbalanced order. The stimulation location was identical across the four conditions. Stimulation was delivered after the study phase and immediately before resting-state fMRI scanning and memory testing in each session. See Hermiller et al. (2019b), Hippocampus for full details.
2 Results
To evaluate the effects of theta-burst stimulation on network connectedness, we used a two-step voxel-wise measure of fMRI connectedness (Cole et al., 2010, Gotts et al., 2012, Steel et al., 2016, Warren et al., 2018) within two networks of interest: (1) the Hippocampal-Cortical Network (HCN), the brain regions with robust functional connectivity to the downstream hippocampus (i.e., our indirect target of our stimulation), and (2) the Parietal Network (PN), the brain regions with robust functional connectivity to the stimulated (i.e., local) parietal location. The HCN was defined at the group level as regions demonstrating high fMRI connectivity with the hippocampal targets identified for each subject using the sham condition fMRI data (Figure 2A, left). The PN was defined at the group level as regions demonstrating high fMRI connectivity with the parietal cortex stimulation location for each subject using fMRI data from the sham condition (Figure 2A, right).
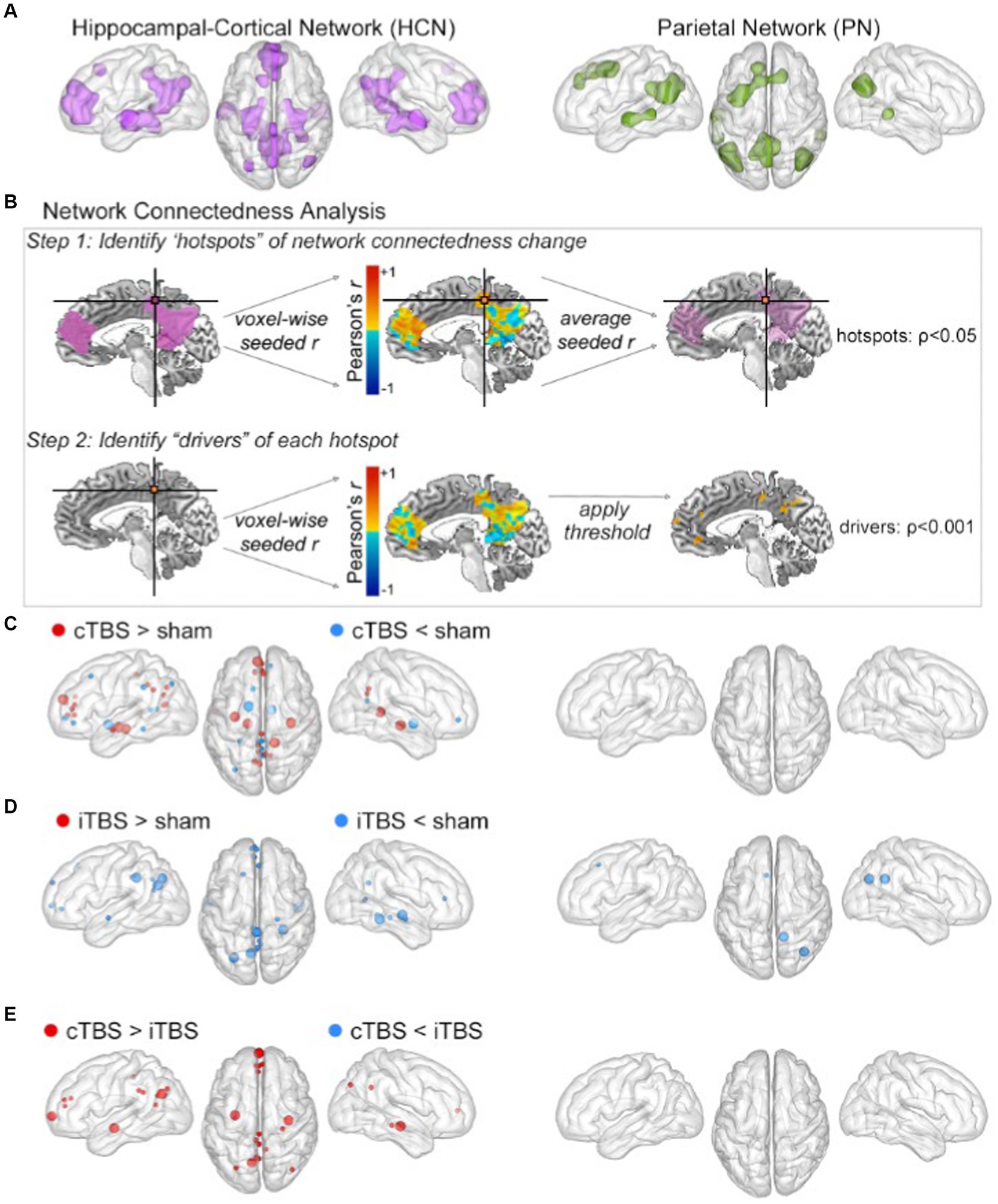
Figure 2. cTBS preferentially influenced HCN fMRI connectedness. (A) The HCN (left) and PN (right) masks were defined by resting-state fMRI connectivity with hippocampal and parietal targets, respectively. (B) Overview of the fMRI connectedness analyses performed in both networks. Step 1 identified hotspots of network connectedness changes (p < 0.05, cluster-size determined via Monte Carlo permutation). Step 2 used each hotspot in a network-constrained seed-based connectivity analysis to identify drivers of its connectivity change. (C) Clusters for cTBS versus sham shown as spheres at the center of mass in the HCN (left) and PN (right). Hotspots depicted as large spheres; drivers depicted as small spheres. Detailed cluster information is provided in Tables 1, 2. The same information for (D) iTBS versus sham and for (E) cTBS versus iTBS.
The two-step fMRI connectedness analyses were performed as pairwise contrasts of stimulation conditions (i.e., cTBS vs. sham, iTBS vs. sham, cTBS vs. iTBS) within each network (i.e., HCN and PN). This analysis first identified the connectedness “hotspots,” which were contiguous voxels for which mean fMRI connectivity with all other network voxels differed significantly between stimulation conditions. Then, each hotspot was used as a seed in a seed-based connectivity analysis to identify the “drivers” of these mean whole-network connectivity effects, which were regions in the networks that significantly differed in connectivity with the hotspots due to stimulation condition (Figure 2B; see methods). Each pairwise comparison of stimulation conditions thus yielded a set of connectedness hotspots and their corresponding drivers in the HCN (Table 1) and PN (Table 2). Monte Carlo permutation testing was performed to determine appropriate cluster sizes to control for type-1 errors and multiple comparisons (see Methods).
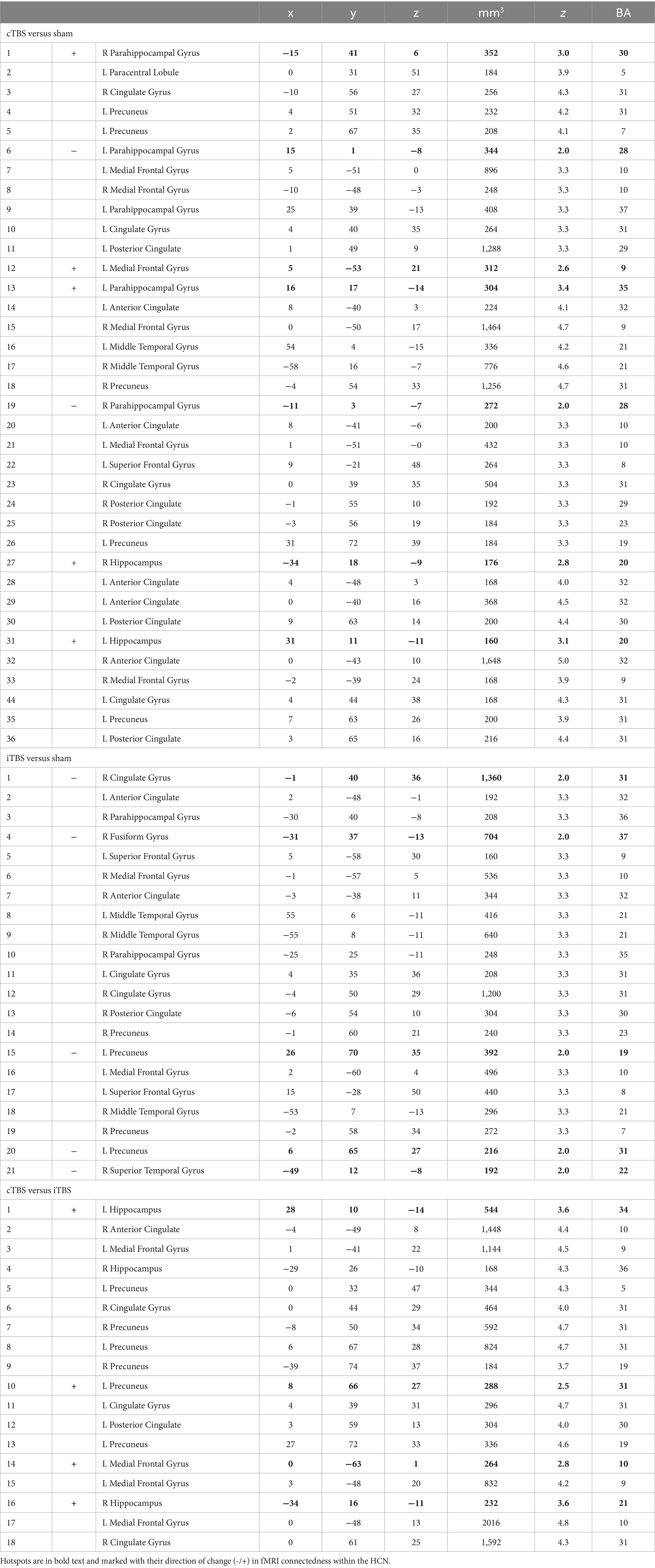
Table 1. Numbered list of HCN connectedness clusters with their center Talairach coordinates, volume (mm3), peak standardized t-statistic (z), and approximate Brodmann Area (BA).
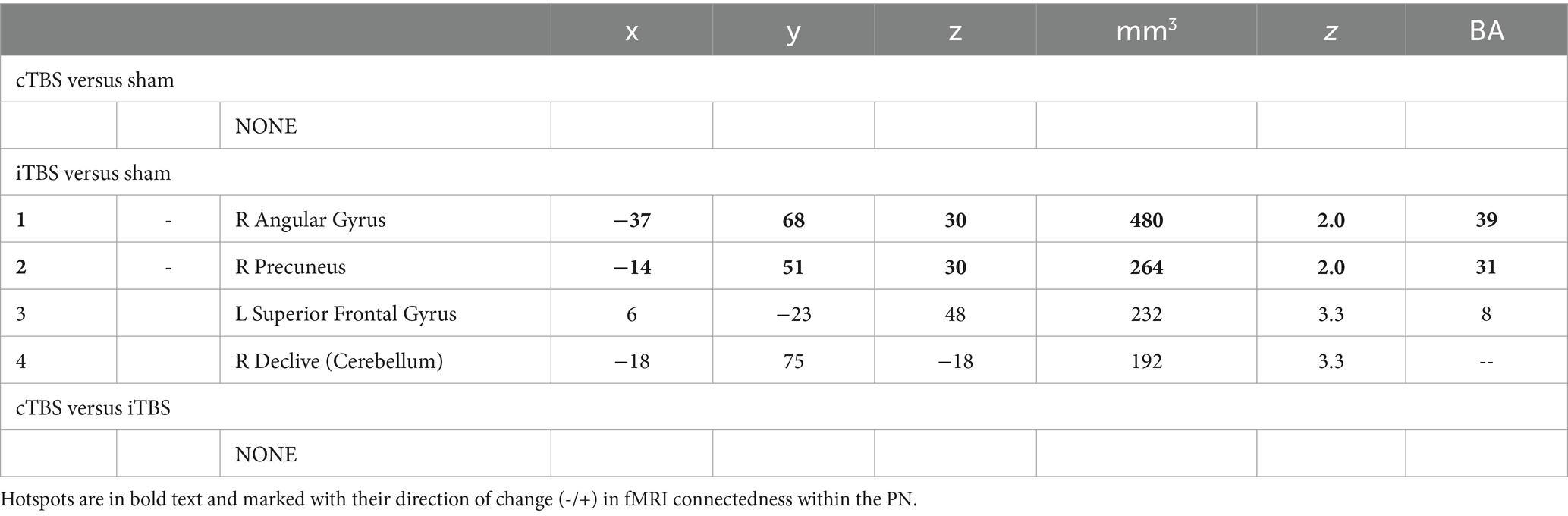
Table 2. Numbered list of PN connectedness clusters with their center Talairach coordinates, volume (mm3), peak standardized t-statistic (z), and approximate Brodmann Area (BA).
2.1 Hippocampal-cortical network connectedness results
Results from the analysis of HCN connectedness for cTBS and iTBS (Figure 2) are presented in detail in Table 1. F or cTBS versus sham, seven hotspots with 29 corresponding driver regions were identified (36 total connectedness clusters; Table 1; Figure 2C, left), with a mix of increases and decreases in fMRI connectedness. These hotspots and drivers were distributed across the HCN, suggested widespread network-level effects in response to cTBS. Five hotspots were identified in the HCN fMRI connectedness analyses for iTBS relative to sham, producing a total of 21 connectedness clusters, all with decreases in HCN fMRI connectedness (Table 1; Figure 2D, left). In a direct comparison of cTBS to iTBS, four hotspots and 14 drivers showed significantly greater fMRI connectivity for cTBS (18 connectedness clusters; Table 1; Figure 2E, left). These clusters were located in core HCN regions, including bilateral hippocampus and medial and lateral prefrontal and parietal locations. This indicated substantially greater HCN engagement by cTBS than iTBS, particularly for core regions of the HCN such as the hippocampus and anterior and posterior midline areas.
2.2 Parietal network connectedness results
Results from the analysis of PN connectedness for cTBS and iTBS (Figure 2) are presented in detail in Table 2. cTBS did not differ from sham, failing to yield any hotspots or drivers (Figure 2C, right). Two hotspots and a total of four connectedness clusters were identified in the PN fMRI connectedness analyses for iTBS relative to sham (Table 2; Figure 2D, right). The hotspot in the right precuneus yielded drivers in the superior frontal gyrus and cerebellum, whereas the hotspot in the right angular gyrus did not yield drivers. Thus, the effects of iTBS on PN fMRI connectedness were mostly constrained within the lateral posterior PN regions. No hotspots or drivers were identified for cTBS relative to iTBS (Table 2; Figure 2E, right).
2.3 Local effects of stimulation on fMRI connectivity of parietal cortex
In order to evaluate local effects of stimulation, we calculated fMRI connectedness within a mask of left parietal cortex that encompassed the stimulation locations and adjacent cortex (Figure 3A). Both theta-burst conditions caused clusters of significant connectedness decrease relative to sham (Figures 3B,C), with direct comparisons among theta-burst conditions indicating greater decreases for cTBS relative to iTBS (Figure 3D). It is notable that both cTBS and iTBS caused local decreases in connectedness but varied in their effects on network connectedness as described above. It is therefore unlikely that these difference network-level effects are due to differences in local effects of these stimulation conditions, as measured by fMRI connectedness.
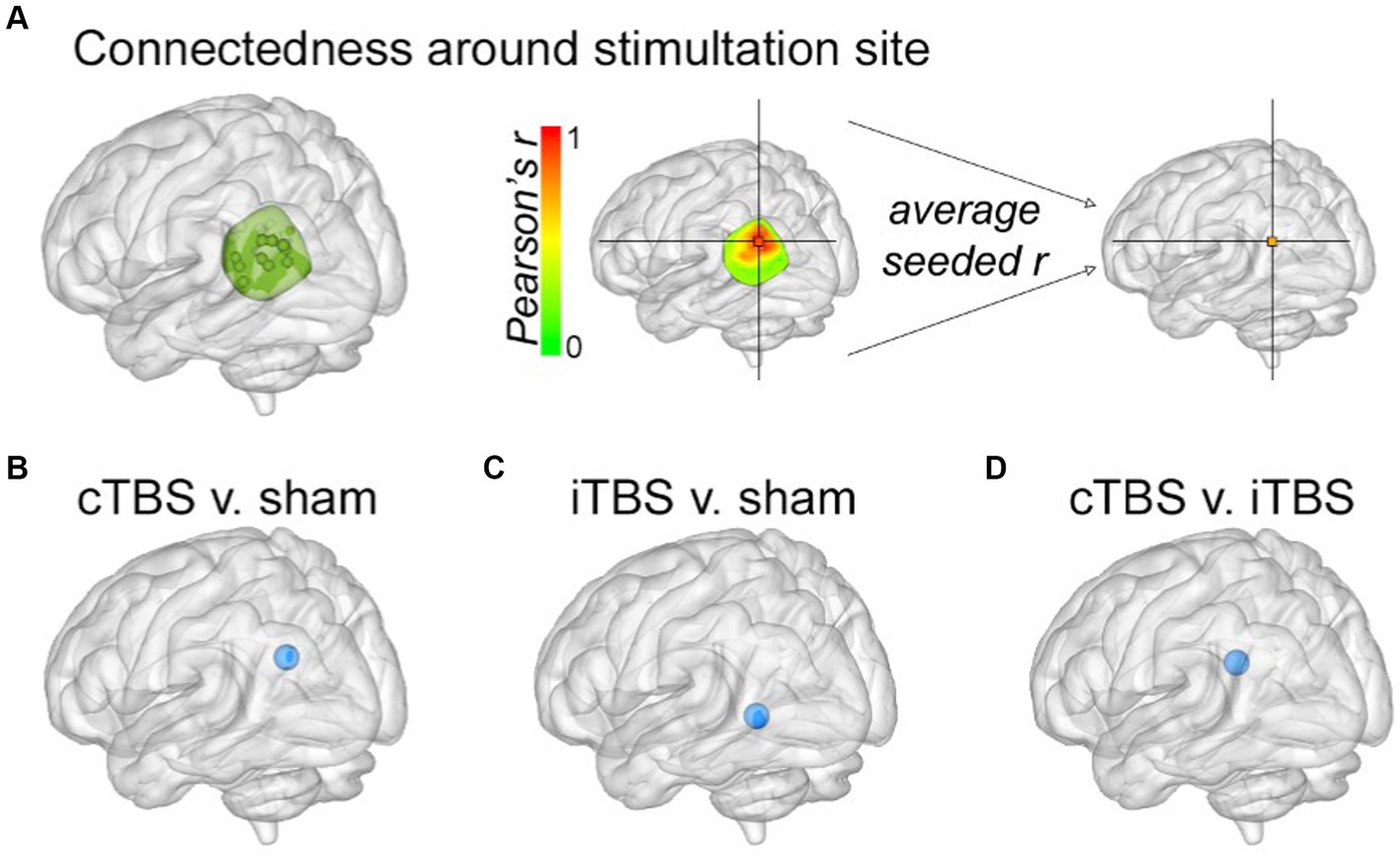
Figure 3. Local changes in parietal fMRI connectedness due to stimulation. (A) The parietal mask used when determining stimulation locations (see Methods in Hermiller et al., 2019a, Hippocampus) was used to measure changes in local fMRI connectedness. Results from the fMRI connectedness analyses within the parietal mask for (B) cTBS versus sham, (C) iTBS versus sham, and (D) cTBS versus iTBS. Blue spheres indicate decreased fMRI connectedness relative to sham for each of the indicated stimulation conditions, as in Figure 2.
3 Discussion
cTBS modulated HCN fMRI connectedness to a greater extent than did iTBS stimulation patterns. This continuous train of theta-patterned stimulation delivered to the parietal cortex influenced “core” HCN regions including hippocampus and parahippocampal cortex, changing their fMRI connectedness with other regions distributed throughout the HCN. In contrast, cTBS had minimal effects on the PN, which was defined as the fMRI network of the parietal cortex location that was directly stimulated. cTBS effects were thus observed downstream of the stimulation location, affecting fMRI connectivity of distributed HCN regions.
Mechanisms for network-level effects of TMS are not fully understood. Compensation-oriented explanations propose that networks “compensate” against changes in local activity due to stimulation, such that local excitation of regions with positive connections to a network will result in network connectivity reductions, and vice versa for local inhibition (Eldaief et al., 2011; Fox et al., 2012; Cocchi et al., 2015; Steel et al., 2016; Davis et al., 2017). Often, cTBS is considered an inhibitory sequence and iTBS is considered excitatory, based primarily on cortico-motor effects (Pascual-Leone et al., 1994; Chen et al., 2003; Huang et al., 2005). The parietal area stimulated in this study has known positive connectivity with the HCN. Consistent with compensation explanations, we found decreases in downstream connectivity in the HCN due to iTBS relative to sham. However, the effects of cTBS were more complicated, with both positive and negative downstream effects relative to sham. Furthermore, the effects of cTBS were specific to the HCN, with no significant changes identified in the PN. iTBS caused decreases in the right parietal region of the PN (contralateral side of the stimulation target). Thus, cTBS and iTBS did not produce opposite effects on HCN or PN fMRI connectivity, as would have been expected based on compensation-oriented explanations of network-level TMS effects due to putative inhibitory/excitatory local effects. Rather, these effects may instead be indicative of a network-level response to the resonance of the stimulation pattern. That is, intermittent trains of theta-burst did not entrain the putative endogenous HCN theta rhythm as effectively as the continuous train, and thus, did not induce as robust transient aftereffects on hippocampal-cortical network connectivity.
Resonance-oriented explanations propose that stimulation applied at frequencies matching the targeted network’s endogenous activity rhythms entrains oscillatory neural activity throughout network regions and promotes network synchrony and related functions (Thut et al., 2011a,b; Chanes et al., 2013; Kim et al., 2016; Romei et al., 2016; Salinas et al., 2016; Lea-Carnall et al., 2017; Weinrich et al., 2017). Indeed, resonance-like effects have been demonstrated by findings that noninvasive theta-patterned stimulation synchronizes theta oscillations (Reinhart et al., 2015; Li et al., 2017). Human hippocampal theta-frequency activity and theta phase locking with other HCN regions have been associated with working and long-term memory functions (Goutagny et al., 2009; Axmacher et al., 2010; Steinvorth et al., 2010; Foster and Parvizi, 2012; Lega et al., 2012; Foster et al., 2013; Watrous et al., 2013; Kaplan et al., 2014; Foster et al., 2015; Lin et al., 2017). Theta synchrony among HCN regions may support information processing and interregional communication (Buzsaki, 2002; Buzsaki and Draguhn, 2004; Burke et al., 2013; Zhang and Jacobs, 2015). The frequency-specificity of these effects support our hypothesis that the HCN would be preferentially engaged by stimulation continuously engaging its endogenous theta rhythm (Buzsaki, 2002; Anderson et al., 2010; Foster and Parvizi, 2012; Lega et al., 2012; Foster et al., 2013; Burke et al., 2014; Zhang and Jacobs, 2015; Lega et al., 2017; Lin et al., 2017). This may also explain in part why the PN (i.e., a distributed fronto-parietal network) displays beta-frequency activity as its hallmark (Rosanova et al., 2009; Samaha et al., 2017; Riddle et al., 2019). A limitation of the current study is that interregional communication was measured only indirectly as fMRI connectivity, which is a metric of low-frequency coupling of the fMRI signal at frequencies below the theta band (Foster et al., 2015; Hacker et al., 2017), although it is possible that theta-frequency neural activities contribute to fMRI connectivity measures via vasomotor entrainment (Mateo et al., 2017).
In line with the theory that theta-burst stimulation may cause downstream HCN entrainment, we conducted a simultaneous TMS/MRI study and delivered 2-s volleys of theta-burst stimulation to individualized parietal locations of subject’s HCN. We found site-specific (i.e., HCN vs. out-of-network location) and frequency-specific (i.e., theta-burst vs. 12.5-Hz) effects of stimulation, such that theta-burst delivered to the HCN improved memory performance and increased hippocampal BOLD signal during encoding (Hermiller et al., 2020). Notably, the 2-s volleys of theta-burst employed here was not an intermittent train of TBS (2-s on, 8-s off). Rather, the 2-s volleys were randomly interleaved with a range of ~11–39 s between volleys. Thus, the volleys were unlikely to have cumulative effects throughout the session as would a train of iTBS (Huang et al., 2005; Demeter et al., 2016). Instead, the 2-s volleys served to immediately affect activity in the hippocampus at an immediate trial-level, putatively via entrainment with the endogenous HCN theta rhythms. Indeed even single stimulation pulses have been reported to evoke activity at the natural frequencies of brain networks, with distinct evoked activity frequencies for various cortical regions (Rosanova et al., 2009). The immediate effects of stimulation reported by Hermiller et al. (2020) suggest that it selectively influenced hippocampal neural activity, as opposed to neuroplasticity and/or neuromodulatory mechanisms that can support persistent/long-lasting effects of stimulation on network function (Cirillo et al., 2017). It is possible that brief TBS volleys affect activity in only those areas most sensitive to this stimulation pattern (e.g., hippocampus) whereas more extensive network-wide effects are recruited with longer theta-burst trains (see Hermiller et al., 2019a, 2022). Further study, including directly measuring hippocampal theta activity as a result of theta-burst stimulation, will be required in order to directly test this hypothesis.
It is noteworthy that intermittent versus continuous theta-burst varied in their effects on the distributed networks (HCN vs. PN), but caused similar local effects at the site of stimulation. That is, both theta-burst conditions decreased fMRI connectedness around the stimulated location (Figure 3). These results indicate that local effects of theta-burst stimulation may not be fully characterized as either inhibitory or excitatory, and that local disruption may fundamentally differ how distributed network-level effects are achieved. Furthermore, although stimulation was delivered to a parietal cortical location, the most robust effects were on fMRI connectedness of the downstream hippocampus and its network locations, rather than within the network of regions with robust connectivity to the stimulated parietal site.
To summarize, these findings demonstrate that distinct large-scale brain networks (i.e., HCN vs. PN) are differentially engaged by network-specific noninvasive stimulation delivered to the same location (i.e., individualized parietal locations). The current findings add to this evolving understanding of network-level neuromodulation by suggesting that correspondence between stimulation and endogenous network rhythms could be an important factor in the network’s response to stimulation. Indeed, it was particularly noteworthy that the network-level effects that varied based on stimulation continuity in the current study, as local effects showed little variation. Furthermore, the network-level effects were specific to the network (i.e., HCN rather than PN) that reportedly has intrinsic rhythms that may have been entrained to the theta-burst stimulation pattern. This is a striking demonstration that networks stimulated location may respond preferentially to specific rhythms, and is at odds with existing compensation-oriented explanations of local stimulation effects on networks, which suggest that network-level changes are due to a “balancing” against purely local excitation or inhibition of activity by stimulation (Eldaief et al., 2011; Fox et al., 2012; Cocchi et al., 2015; Steel et al., 2016).
4 Methods
4.1 Participants
A final sample of 24 participants with complete datasets was included in all analyses (14 females, ages 19–28 years, average age = 23.5, SD = 2.6). The target sample size of 24 was based solely on previous studies using similar methods (Eldaief et al., 2011; Nee and D’Esposito, 2016). All participants gave written informed consent approved by the Northwestern University Institutional Review Board and were paid for participation. See Hermiller et al. (2019a), Hippocampus for further details about recruitment, eligibility, and exclusion criteria.
4.2 Experimental procedure
All experiment procedures are reported in detail in Hermiller et al. (2019a), Hippocampus. In sum, participants completed a baseline session and four experimental sessions on separate days using a within-subjects, single-blinded, counterbalanced design. The experimental sessions were separated by at least 48 h, with an average interval of 3.6 days between each session (range 2.0–5.8 days). Each experimental session consisted of a short practice session, during which participants ran through an example of the different phases of the day’s experiment and verbally confirmed they understood task instructions. After this, the experimental session commenced, starting with a memory task study phase, followed by TMS, resting-state fMRI scanning, and a memory task test phase. The memory task and results are not reported here; please see Hermiller et al. (2019a), Hippocampus for details about the memory task and the effects of stimulation on memory performance and memory-related connectivity.
4.3 Transcranial magnetic stimulation
A MagPro X100 stimulator and a MagPro Cool-B65 liquid-cooled butterfly coil (MagVenture A/S, Farum, Denmark) were used to deliver stimulation, along with an MRI-guided navigation system using individual MRIs (Localite GmbH, St. Augustin, Germany) to ensure accurate and reproducible targeting. Participants sat in an ergonomic chair with a stabilizing headrest and a vacuum-conforming pillow. Resting motor threshold (RMT), the minimum percentage of stimulator output (%SO) needed to produce visible contractions of the right abductor pollicis brevis (i.e., thumb muscle) for five out of 10 consecutive pulses, was determined during each subject’s baseline session. As previously reported, RMT values ranged between 34 and 68 %SO (mean = 49.2 %SO, SD = 7.5).
A different stimulation condition (cTBS, iTBS, 20-Hz rTMS, or sham) was delivered in each of the four different sessions/days, in a counterbalanced order across subjects (see Hermiller et al., 2019a, Hippocampus for details). Theta-burst stimulation utilized in the reported datasets here followed standard guidelines (Huang et al., 2005; Rossi et al., 2009, 2021), with a total of 600 pulses arranged in 50-Hz triplet bursts delivered every 200-ms (5-Hz). In cTBS, bursts were delivered in one continuous train (40-s duration), whereas in iTBS, bursts were delivered in intermittent trains (2-s on, 8-s off; 190-s duration). Theta-bust stimulation was delivered at an intensity of 80% RMT. The sham condition used a standard 20-Hz rTMS protocol (2-s on, 28-s off; 20 min duration), but with intensity lowered to 10% RMT as to be unlikely to affect neuronal activity. A 20-Hz active condition was also utilized, but not reported here.
TMS was delivered in a 20 min protocol while participants played “2048,” a single-player sliding-block puzzle on a computer tablet.1 This game served to engage the participants during the 20-min stimulation protocol, to prevent them from rehearsing the learned stimuli from the encoding phase, and to distract them from the variances in stimulation patterns across sessions. We chose this game as it includes numbers (i.e., no overlapping stimuli or semantics with the memory task stimuli), requires low motor effort (i.e., finger swipe) and is not timed (i.e., so participants would not be. jumpy’ or on edge to make fast responses, which could potentially cause head movement during the TMS delivery). TMS was delivered in a room adjacent to the MRI scanner room. Stimulation effects have been reported to last approximately 60 min post-stimulation (Huang et al., 2005; Rossi et al., 2009; Thut and Pascual-Leone, 2010; Wischnewski and Schutter, 2015). All subsequent testing procedures occurred within this time window.
4.4 MRI acquisition and preprocessing
See Hermiller et al. (2019a), Hippocampus for full details on MRI data collection and preprocessing. In summary, a Siemens 3 T Prisma whole-body scanner with a 64-channel head coil was used to collect resting-state functional images (TE 22-ms; TR 555-ms; flip angle 47°; voxel resolution 2.0×2.0×2.0-mm, 550 frames, 64 slices, multi-band factor of 8). Participants were instructed to lie as still as possible, to keep their eyes open and focused on a fixation cross presented in the center of the screen, and to let their minds wander without thinking of anything specific. Structural images were acquired using a T1-weighted MPRAGE sequence (TE 1.69-ms; TR 2170-ms; TI 1100-ms; flip angle 7°; voxel resolution 1.0×1.0×1.0-mm, 176 frames). MRI data were preprocessed using AFNI software (Cox, 1996), and included the outlier suppression, spatial normalization to the Talairach et al. (1988) Colin27 template, spatial smoothing (4-mm Gaussian smoothing kernel), and signal intensity normalization. Bandpass filtering (0.01–0.1-Hz), motion censoring (values replaced with zero), and nuisance time series (estimates of motion parameters and derivatives) were detrended from each voxel simultaneously as a linear regression model to yield a residual time series for subsequent analysis reported here.
4.5 Individualized stimulation target identification
Individualized hippocampal targets and parietal cortex stimulation locations were defined for each subject via fMRI connectivity measures using the data collected during each subject’s baseline session (Figure 1). Additional details are provided in Hermiller et al. (2019a), Hippocampus.
4.6 Network definition
The HCN and the PN were defined based on the individualized hippocampal targets and stimulation locations, respectively. These served as seeds in a group-level voxel-wise whole-brain connectivity analysis using the sham fMRI datasets. Contiguous voxels (3dClustSim cluster size threshold α < 0.01) with significant (p < 1.0×10−8) functional connectivity with the target were saved as network regions, and dilated (3dmask_tool). All surviving voxels were saved as the network mask. The HCN mask consisted of 15,325 voxels and the PN mask consisted of 9,047 voxels, with 2,833 voxels overlapping between networks (Figure 2A).
4.7 Statistical analyses methods
Statistical significance between each stimulation condition relative to sham was evaluated at the group-level using paired two-tailed t-tests. The sham condition was used in this comparison (i.e., rather than the baseline resting-state scan), as the sham condition underwent the exact same experimental features (e.g., encoding phase of the memory task, 2048 gam during stimulation session). The baseline resting state scan was not preceded with these features. Separate results were obtained for each stimulation contrast and were not used in analyses of other stimulation contrasts, thus eliminating circular analysis. Imaging analysis was done using AFNI (Cox, 1996) and Matlab (The MathWorks, Inc. Natick, MA, United States).2 MRI results were visualized using the BrainNet Viewer Matlab toolbox (Xia et al., 2013).
4.8 fMRI network connectedness analyses
A two-step voxel-wise within-network analysis was performed to identify voxels within networks of interest that changed in fMRI connectedness with other voxels within the network due to stimulation (Cole et al., 2010; Gotts et al., 2012; Steel et al., 2016; Warren et al., 2018). This yielded a set of connectedness clusters (“hotspots” of network connectedness change) (see Figure 2B, top). These hotspots were then used as seeds in seed-based connectivity analyses (constrained to the network the hotspot was identify in) to identify the “drivers” of each hotspot’s connectivity change (see Figure 2B, bottom). This was performed separately for each stimulation condition contrast in each network (Tables 1, 2; Figures 2C–E).
Step 1 (determine “hotspots”): Network fMRI connectedness maps were created by correlating (Pearson’s r) the time series in each voxel with every other voxel in the network-of-interest mask, averaging the correlations, and assigning the mean value to that voxel in the subject’s network connectedness map (3dTcorrMap). Fisher’s z transformation was applied to yield normally distributed network connectedness maps for each subject in each stimulation condition. Differences in network connectedness between stimulation conditions were evaluated at the group-level to identify hotspots of network connectedness change using post-hoc paired t-tests (two-tailed) in each voxel (3dttest++) to identify clusters of voxels with differences in network connectedness (p < 0.05 uncorrected, z(t)-threshold = 1.96). A statistical threshold of p < 0.05 was used to identify these hotspots (Gotts et al., 2012; Steel et al., 2016), considering the dilution of correlation strength that occurs when averaging connectedness across the entire network. The rationale for using a lenient threshold in this first step, was to be as inclusive as possible when determining hotspots of change. Monte Carlo permutation testing was performed to determine the appropriate hotspot cluster-size threshold in each network and to reduce the number of type 1 errors. The t-tests were repeated 100 times for each stimulation condition relative to sham in each network, with random flipping of condition labels for half the subjects to generate a probability distribution of hotspot sizes. A α < 0.05 cutoff yielded a hotspot cluster-size threshold of 20 voxels on the HCN distribution and 28 voxels on the PN distribution. Using these permutation-defined cluster-size thresholds, a set of hotspots was determined for each stimulation condition contrast in each network (Tables 1, 2). Subsequent analyses with a set of hotspots were performed using datasets only from the two stimulation conditions that were contrasted to determine that set of hotspots.
Step 2: Identify “driver” regions of the hotspots. The spatially averaged time series of each hotspot was used as a seed in seed-based, voxel-wise analyses (3dTcorr1D). A Fisher’s z transformation was applied to yield a normally distributed network correlation map for the hotspot for each subject in the relevant stimulation conditions. Between-condition differences in a hotspot’s connectivity were evaluated at the group level to identify drivers of that hotspot’s connectivity change. Monte Carlo permutation testing was performed to determine the appropriate driver cluster-size threshold for each set of hotspots. The difference in connectivity for each hotspot was evaluated using post-hoc paired t-tests (two-tailed) in each voxel (3dttest++) to identify clusters of voxels with differences in hotspot connectivity (p < 0.001 uncorrected, z(t)-threshold = 3.291). This was repeated 100 times for each hotspot, with random flipping of condition labels for half the subjects to generate a probability distribution of driver sizes. A α < 0.05 cutoff was used to determine the driver cluster-size threshold for each set of hotspots. Across both networks and all sets of hotspots, the number of voxels required to meet a α < 0.05 cutoff ranged from 7 to 15. A more conservative 20+ voxel cluster-size threshold was imposed to determine the drivers across both networks.
Each set of stimulation condition contrasts generated separate lists of connectedness clusters (hotspots and drivers) in each network. The maximum standardized t-statistic (z-score) was extracted (3dROIstats) for each connectedness cluster (Tables 1, 2).
Data availability statement
Publicly available datasets were analyzed in this study. This data can be found at: https://nda.nih.gov/.
Ethics statement
The studies involving humans were approved by Northwestern University Institutional Review Board. The studies were conducted in accordance with the local legislation and institutional requirements. The participants provided their written informed consent to participate in this study.
Author contributions
MH: Data curation, Formal analysis, Visualization, Writing – original draft, Writing – review & editing.
Funding
The author(s) declare that financial support was received for the research, authorship, and/or publication of this article. Neuroimaging was performed at the Northwestern University Center for Translational Imaging, supported by Northwestern University Department of Radiology, funded by National Institute of Mental Health, Grant/Award Number: R01-MH106512R01-MH111790; National Institute of Neurological Disorders and Stroke, Grant/Award Number: T32-NS047987. This research was supported in part through the computational resources and staff contributions provided for Quest, the high-performance computing facility at Northwestern University, which is jointly supported by the Office of the Provost, the Office for Research and Northwestern University Information Technology. Support was also provided by High Performance Computing (HPC) Cluster at the Florida State University Research Computing Center, supported by the Florida State University Information Technology Services. The content is solely the responsibility of the author and does necessarily represent the official view of the National Institutes of Health.
Acknowledgments
Thank you to Joel L. Voss for his continuous support.
Conflict of interest
The author declares that the research was conducted in the absence of any commercial or financial relationships that could be construed as a potential conflict of interest.
Publisher’s note
All claims expressed in this article are solely those of the authors and do not necessarily represent those of their affiliated organizations, or those of the publisher, the editors and the reviewers. Any product that may be evaluated in this article, or claim that may be made by its manufacturer, is not guaranteed or endorsed by the publisher.
Footnotes
References
Albouy, P., Weiss, A., Baillet, S., and Zatorre, R. J. (2017). Selective entrainment of Theta oscillations in the dorsal stream causally enhances auditory working memory performance. Neuron 94, 193–206.e5. doi: 10.1016/j.neuron.2017.03.015
Anderson, K. L., Rajagovindan, R., Ghacibeh, G. A., Meador, K. J., and Ding, M. (2010). Theta oscillations mediate interaction between prefrontal cortex and medial temporal lobe in human memory. Cereb. Cortex 20, 1604–1612. doi: 10.1093/cercor/bhp223
Axmacher, N., Henseler, M. M., Jensen, O., Weinreich, I., Elger, C. E., and Fell, J. (2010). Cross-frequency coupling supports multi-item working memory in the human hippocampus. Proc. Natl. Acad. Sci. USA 107, 3228–3233. doi: 10.1073/pnas.0911531107
Berry, S. D., and Thompson, R. F. (1978). Prediction of learning rate from the hippocampal electroencephalogram. Science 200, 1298–1300. doi: 10.1126/science.663612
Buckner, R. L., Andrews-Hanna, J. R., and Schacter, D. L. (2008). The brain’s default network: anatomy, function, and relevance to disease. Ann. N. Y. Acad. Sci. 1124, 1–38. doi: 10.1196/annals.1440.011
Burke, J. F., Sharan, A. D., Sperling, M. R., Ramayya, A. G., Evans, J. J., Healey, M. K., et al. (2014). Theta and high-frequency activity mark spontaneous recall of episodic memories. J. Neurosci. 34, 11355–11365. doi: 10.1523/JNEUROSCI.2654-13.2014
Burke, J. F., Zaghloul, K. A., Jacobs, J., Williams, R. B., Sperling, M. R., Sharan, A. D., et al. (2013). Synchronous and asynchronous theta and gamma activity during episodic memory formation. J. Neurosci. 33, 292–304. doi: 10.1523/JNEUROSCI.2057-12.2013
Buzsaki, G. (2002). Theta oscillations in the hippocampus. Neuron 33, 325–340. doi: 10.1016/S0896-6273(02)00586-X
Buzsaki, G., and Draguhn, A. (2004). Neuronal oscillations in cortical networks. Science 304, 1926–1929. doi: 10.1126/science.1099745
Canals, S., Beyerlein, M., Merkle, H., and Logothetis, N. K. (2009). Functional MRI evidence for LTP-induced neural network reorganization. Curr. Biol. 19, 398–403. doi: 10.1016/j.cub.2009.01.037
Chanes, L., Quentin, R., Tallon-Baudry, C., and Valero-Cabré, A. (2013). Causal frequency-specific contributions of frontal spatiotemporal patterns induced by non-invasive neurostimulation to human visual performance. J. Neurosci. 33, 5000–5005. doi: 10.1523/JNEUROSCI.4401-12.2013
Chen, R., Yung, D., and Li, J. Y. (2003). Organization of ipsilateral excitatory and inhibitory pathways in the human motor cortex. J. Neurophysiol. 89, 1256–1264. doi: 10.1152/jn.00950.2002
Cirillo, G., di Pino, G., Capone, F., Ranieri, F., Florio, L., Todisco, V., et al. (2017). Neurobiological after-effects of non-invasive brain stimulation. Brain Stimul. 10, 1–18. doi: 10.1016/j.brs.2016.11.009
Cocchi, L., Sale, M. V., Lord, A., Zalesky, A., Breakspear, M., and Mattingley, J. B. (2015). Dissociable effects of local inhibitory and excitatory theta-burst stimulation on large-scale brain dynamics. J. Neurophysiol. 113, 3375–3385. doi: 10.1152/jn.00850.2014
Cole, M. W., Pathak, S., and Schneider, W. (2010). Identifying the brain’s most globally connected regions. NeuroImage 49, 3132–3148. doi: 10.1016/j.neuroimage.2009.11.001
Cox, R. W. (1996). AFNI: software for analysis and visualization of functional magnetic resonance neuroimages. Comput. Biomed. Res. 29, 162–173. doi: 10.1006/cbmr.1996.0014
Davis, S. W., Luber, B., Murphy, D. L. K., Lisanby, S. H., and Cabeza, R. (2017). Frequency-specific neuromodulation of local and distant connectivity in aging and episodic memory function. Hum. Brain Mapp. 38, 5987–6004. doi: 10.1002/hbm.23803
Demeter, E., Mirdamadi, J. L., Meehan, S. K., and Taylor, S. F. (2016). Short theta burst stimulation to left frontal cortex prior to encoding enhances subsequent recognition memory. Cogn. Affect. Behav. Neurosci. 16, 724–735. doi: 10.3758/s13415-016-0426-3
Di Lazzaro, V., Dileone, M., Pilato, F., Capone, F., Musumeci, G., Ranieri, F., et al. (2011). Modulation of motor cortex neuronal networks by rTMS: comparison of local and remote effects of six different protocols of stimulation. J. Neurophysiol. 105, 2150–2156. doi: 10.1152/jn.00781.2010
Eldaief, M. C., Halko, M. A., Buckner, R. L., and Pascual-Leone, A. (2011). Transcranial magnetic stimulation modulates the brain’s intrinsic activity in a frequency-dependent manner. Proc. Natl. Acad. Sci. USA 108, 21229–21234. doi: 10.1073/pnas.1113103109
Foster, B. L., Kaveh, A., Dastjerdi, M., Miller, K. J., and Parvizi, J. (2013). Human retrosplenial cortex displays transient theta phase locking with medial temporal cortex prior to activation during autobiographical memory retrieval. J. Neurosci. 33, 10439–10446. doi: 10.1523/JNEUROSCI.0513-13.2013
Foster, B. L., and Parvizi, J. (2012). Resting oscillations and cross-frequency coupling in the human posteromedial cortex. NeuroImage 60, 384–391. doi: 10.1016/j.neuroimage.2011.12.019
Foster, B. L., Rangarajan, V., Shirer, W. R., and Parvizi, J. (2015). Intrinsic and task-dependent coupling of neuronal population activity in human parietal cortex. Neuron 86, 578–590. doi: 10.1016/j.neuron.2015.03.018
Fox, M. D., Halko, M. A., Eldaief, M. C., and Pascual-Leone, A. (2012). Measuring and manipulating brain connectivity with resting state functional connectivity magnetic resonance imaging (fcMRI) and transcranial magnetic stimulation (TMS). NeuroImage 62, 2232–2243. doi: 10.1016/j.neuroimage.2012.03.035
Freedberg, M., Reeves, J. A., Toader, A. C., Hermiller, M. S., Voss, J. L., and Wasserman, E. M. (2019). Persistent enhancement of hippocampal network connectivity by parietal rTMS is reproducible. eNeuro. 6:ENEURO.0129. doi: 10.1523/ENEURO.0129-19.2019
Gotts, S. J., Simmons, W. K., Milbury, L. A., Wallace, G. L., Cox, R. W., and Martin, A. (2012). Fractionation of social brain circuits in autism spectrum disorders. Brain 135, 2711–2725. doi: 10.1093/brain/aws160
Goutagny, R., Jackson, J., and Williams, S. (2009). Self-generated theta oscillations in the hippocampus. Nat. Neurosci. 12, 1491–1493. doi: 10.1038/nn.2440
Gratton, C., Lee, T. G., Nomura, E. M., and D’Esposito, M. (2013). The effect of theta-burst TMS on cognitive control networks measured with resting state fMRI. Front. Syst. Neurosci. 7:124
Hacker, C. D., Snyder, A. Z., Pahwa, M., Corbetta, M., and Leuthardt, E. C. (2017). Frequency-specific electrophysiologic correlates of resting state fMRI networks. NeuroImage 149, 446–457. doi: 10.1016/j.neuroimage.2017.01.054
Halko, M. A., Farzan, F., Eldaief, M. C., Schmahmann, J. D., and Pascual-Leone, A. (2014). Intermittent theta-burst stimulation of the lateral cerebellum increases functional connectivity of the default network. J. Neurosci. 34, 12049–12056. doi: 10.1523/JNEUROSCI.1776-14.2014
Hanslmayr, S., Matuschek, J., and Fellner, M. C. (2014). Entrainment of prefrontal beta oscillations induces an endogenous echo and impairs memory formation. Curr. Biol. 24, 904–909. doi: 10.1016/j.cub.2014.03.007
Hermiller, M. S., Chen, Y. F., Parrish, T. B., and Voss, J. L. (2020). Evidence for immediate enhancement of hippocampal memory encoding by network-targeted theta-burst stimulation during concurrent fMRI. J. Neurosci. 40, 7155–7168. doi: 10.1523/JNEUROSCI.0486-20.2020
Hermiller, M. S., Dave, S., Wert, S. L., VanHaerents, S., Riley, M., Weintraub, S., et al. (2022). Evidence from theta-burst stimulation that age-related de-differentiation of the hippocampal network is functional for episodic mempory. Neuobiol. Aging 109, 145–157. doi: 10.1016/j.neurobiolaging.2021.09.018
Hermiller, M. S., Karp, E., Nilakantan, A. S., and Voss, J. L. (2019b). Episodic memory improvements due to noninvasive stimulation targeting the cortical-hippocampal network: a replication and extension experiment. Brain Behav. 9:e01393. doi: 10.1002/brb3.1393
Hermiller, M. S., VanHaerents, S., Raij, T., and Voss, J. L. (2019a). Frequency-specific noninvasive modulation of memory retreival and its relationship with hippocampal network connectivity. Hippocampus 29, 595–609. doi: 10.1002/hipo.23054
Huang, Y. Z., Edwards, M. J., Rounis, E., Bhatia, K. P., and Rothwell, J. C. (2005). Theta burst stimulation of the human motor cortex. Neuron 45, 201–206. doi: 10.1016/j.neuron.2004.12.033
Kaplan, R., Bush, D., Bonnefond, M., Bandettini, P. A., Barnes, G. R., Doeller, C. F., et al. (2014). Medial prefrontal theta phase coupling during spatial memory retrieval. Hippocampus 24, 656–665. doi: 10.1002/hipo.22255
Kim, K., Ekstrom, A. D., and Tandon, N. (2016). A network approach for modulating memory processes via direct and indirect brain stimulation: toward a causal approach for the neural basis of memory. Neurobiol. Learn. Mem. 134 Pt A, 162–177. doi: 10.1016/j.nlm.2016.04.001
Kim, S., Nilakantan, A. S., Hermiller, M. S., Palumbo, R. T., VanHaerents, S., and Voss, J. L. (2018). Selective and coherent activity increases due to stimulation indicate functional distinctions between episodic memory networks. Science. Advances 4:2768. doi: 10.1126/sciadv.aar2768
Larson, J., Wong, D., and Lynch, G. (1986). Patterned stimulation at the theta frequency is optimal for the induction of hippocampal long-term potentiation. Brain Res. 368, 347–350. doi: 10.1016/0006-8993(86)90579-2
Lea-Carnall, C. A., Trujillo-Barreto, N. J., Montemurro, M. A., El-Deredy, W., and Parkes, L. M. (2017). Evidence for frequency-dependent cortical plasticity in the human brain. Proc. Natl. Acad. Sci. USA 114, 8871–8876. doi: 10.1073/pnas.1620988114
Lega, B., Germi, J., and Rugg, M. (2017). Modulation of oscillatory power and connectivity in the human posterior cingulate cortex supports the encoding and retrieval of episodic memories. J. Cogn. Neurosci. 29, 1415–1432. doi: 10.1162/jocn_a_01133
Lega, B. C., Jacobs, J., and Kahana, M. (2012). Human hippocampal theta oscillations and the formation of episodic memories. Hippocampus 22, 748–761. doi: 10.1002/hipo.20937
Li, S., Jin, J. N., Wang, X., Qi, H. Z., Liu, Z. P., and Yin, T. (2017). Theta and alpha oscillations during the retention period of working memory by rTMS stimulating the parietal lobe. Front. Behav. Neurosci. 11:170. doi: 10.3389/fnbeh.2017.00170
Lin, J. J., Rugg, M. D., Das, S., Stein, J., Rizzuto, D. S., Kahana, M. J., et al. (2017). Theta band power increases in the posterior hippocampus predict successful episodic memory encoding in humans. Hippocampus 27, 1040–1053. doi: 10.1002/hipo.22751
Mastropasqua, C., Bozzali, M., Ponzo, V., Giulietti, G., Caltagirone, C., Cercignani, M., et al. (2014). Network based statistical analysis detects changes induced by continuous theta-burst stimulation on brain activity at rest. Front. Psych. 5:97. doi: 10.3389/fpsyt.2014.00097
Mateo, C., Knutsen, P. M., Tsai, P. S., Shih, A. Y., and Kleinfeld, D. (2017). Entrainment of arteriole vasomotor fluctuations by neural activity is a basis of blood-oxygenation-level-dependent “resting-state” connectivity. Neuron 96, 936–948.e3. doi: 10.1016/j.neuron.2017.10.012
Mesulam, M. M., Van Hoesen, G. W., Pandya, D. N., and Geschwind, N. (1977). Limbic and sensory connections of the inferior parietal lobule (area PG) in the rhesus monkey: a study with a new method for horseradish peroxidase histochemistry. Brain Res. 136, 393–414. doi: 10.1016/0006-8993(77)90066-X
Mufson, E. J., and Pandya, D. N. (1984). Some observations on the course and composition of the cingulum bundle in the rhesus monkey. J. Comp. Neurol. 225, 31–43. doi: 10.1002/cne.902250105
Nee, D. E., and D’Esposito, M. (2016). The hierarchical organization of the lateral prefrontal cortex. eLife 5:12112. doi: 10.7554/eLife.12112
Nilakantan, A. S., Bridge, D. J., Gagnon, E. P., VanHaerents, S. A., and Voss, J. L. (2017). Stimulation of the posterior cortical-hippocampal network enhances precision of memory recollection. Curr. Biol. 27, 465–470. doi: 10.1016/j.cub.2016.12.042
Pascual-Leone, A., Valls-Solé, J., Wassermann, E. M., and Hallett, M. (1994). Responses to rapid-rate transcranial magnetic stimulation of the human motor cortex. Brain 117, 847–858. doi: 10.1093/brain/117.4.847
Rahnev, D., Kok, P., Munneke, M., Bahdo, L., de Lange, F. P., and Lau, H. (2013). Continuous theta burst transcranial magnetic stimulation reduces resting state connectivity between visual areas. J. Neurophysiol. 110, 1811–1821. doi: 10.1152/jn.00209.2013
Reinhart, R. M., Zhu, J., Park, S., and Woodman, G. F. (2015). Synchronizing theta oscillations with direct-current stimulation strengthens adaptive control in the human brain. Proc. Natl. Acad. Sci. USA 112, 9448–9453. doi: 10.1073/pnas.1504196112
Riddle, J., Hwang, K., Cellier, D., Dhanani, S., and D’Esposito, M. (2019). Causal evidence for the role of neuronal oscillations in top-down and bottom- up attention. J. Cogn. Neurosci. 31, 768–779. doi: 10.1162/jocn_a_01376
Roberts, B. M., Clarke, A., Addante, R. J., and Ranganath, C. (2018). Entrainment enhances theta oscillations and improves episodic memory. Cogn Neurosci 9, 181–193. doi: 10.1080/17588928.2018.1521386
Romei, V., Thut, G., and Silvanto, J. (2016). Information-based approaches of noninvasive transcranial brain stimulation. Trends Neurosci. 39, 782–795. doi: 10.1016/j.tins.2016.09.001
Rosanova, M., Casali, A., Bellina, V., Resta, F., Mariotti, M., and Massimini, M. (2009). Natural frequencies of human corticothalmaic circuits. J. Neurophysiol. 29, 7679–7685. doi: 10.1523/JNEUROSCI.0445-09.2009
Rossi, S., Antal, A., Bestmann, S., Bikson, M., Brewer, C., Brockmoller, J., et al. (2021). Basis of this article began with a Consensus Statement from the Ifcn Workshop on Present, F. o. T. M. S. S. E. G. S. O. u. t. A. (2021) Safety and recommendations for TMS use in healthy subjects and patient populations, with updates on training, ethical and regulatory issues: Expert Guidelines. Clin Neurophysiol. 132, 269–306.
Rossi, S. H. M., Rossini, P. M., and Pascual-Leone, A. Safety of TMS Consensus Group (2009). Safety, ethical considerations, and application guidelines for the use of transcranial magnetic stimulation in clinical practice and research. Clin. Neurophysiol. 120, 2008–2039. doi: 10.1016/j.clinph.2009.08.016
Salinas, F. S., Franklin, C., Narayana, S., Szabo, C. A., and Fox, P. T. (2016). Repetitive transcranial magnetic stimulation educes frequency-specific causal relationships in the motor network. Brain Stimul. 9, 406–414. doi: 10.1016/j.brs.2016.02.006
Samaha, J., Gosseries, O., and Postle, B. R. (2017). Distinct oscillatory frequencies underlie excitability of human occipital and parietal cortex. J. Neurosci. 37, 2824–2833. doi: 10.1523/JNEUROSCI.3413-16.2017
Squire, L. R., and Zola-Morgan, S. (1991). The medial temporal lobe memory system. Science 253, 1380–1386. doi: 10.1126/science.1896849
Stanislaw, H., and Todorov, N. (1999). Calculation of signal detection theory measures. Behav. Res. Methods Instrum. Comput. 31, 137–149. doi: 10.3758/BF03207704
Steel, A., Song, S., Bageac, D., Knutson, K. M., Keisler, A., Saad, Z. S., et al. (2016). Shifts in connectivity during procedural learning after motor cortex stimulation: a combined transcranial magnetic stimulation/functional magnetic resonance imaging study. Cortex 74, 134–148. doi: 10.1016/j.cortex.2015.10.004
Steinvorth, S., Wang, C., Ulbert, I., Schomer, D., and Halgren, E. (2010). Human entorhinal gamma and theta oscillations selective for remote autobiographical memory. Hippocampus 20, 166–173. doi: 10.1002/hipo.20597
Suzuki, W. A., and Amaral, D. G. (1994). Perirhinal and parahippocampal cortices of the macaque monkey: cortical afferents. J. Comp. Neurol. 350, 497–533. doi: 10.1002/cne.903500402
Thut, G., and Pascual-Leone, A. (2010). A review of combined TMS-EEG studies to characterize lasting effects of repetive TMS and assess their usefullness in cognitive and clinincal neuroscience. Brain Topogr. 22, 219–232. doi: 10.1007/s10548-009-0115-4
Thut, G., Schyns, P. G., and Gross, J. (2011b). Entrainment of perceptually relevant brain oscillations by non-invasive rhythmic stimulation of the human brain. Front. Psychol. 2:170
Thut, G., Veniero, D., Romei, V., Miniussi, C., Schyns, P., and Gross, J. (2011a). Rhythmic TMS causes local entrainment of natural oscillatory signatures. Curr. Biol. 21, 1176–1185. doi: 10.1016/j.cub.2011.05.049
Valchev, N., Curcic-Blake, B., Renken, R. J., Avenanti, A., Keysers, C., Gazzola, V., et al. (2015). cTBS delivered to the left somatosensory cortex changes its functional connectivity during rest. NeuroImage 114, 386–397. doi: 10.1016/j.neuroimage.2015.04.017
Vincent, J. L., Snyder, A. Z., Fox, M. D., Shannon, B. J., Andrews, J. R., Raichle, M. E., et al. (2006). Coherent spontaneous activity identifies a hippocampal-parietal memory network. J. Neurophysiol. 96, 3517–3531. doi: 10.1152/jn.00048.2006
Wang, J. X., Rogers, L. M., Gross, E. Z., Ryals, A. J., Dokucu, M. E., Brandstatt, K. L., et al. (2014). Targeted enhancement of cortical-hippocampal brain networks and associative memory. Science 345, 1054–1057. doi: 10.1126/science.1252900
Wang, J. X., and Voss, J. L. (2015). Long-lasting enhancements of memory and hippocampal-cortical functional connectivity following multiple-day targeted noninvasive stimulation. Hippocampus 25, 877–883. doi: 10.1002/hipo.22416
Warren, K. N., Hermiller, M. S., Nilakanatan, A. S., O’Neil, J., Palumbo, R. T., Voss, J., et al. (2018). Increased fMRI activity correlations in autobiographical memory versus resting states. Hum. Brain Mapp. 39, 4312–4321. doi: 10.1002/hbm.24248
Warren, K. N., Hermiller, M. S., Nilakantan, A., and Voss, J. L. (2019). Stimulating the hippocampal posterior-medial network enhances task-dependent connectivity and memory. eLife 8:458. doi: 10.7554/eLife.49458
Watrous, A. J., Tandon, N., Conner, C. R., Pieters, T., and Ekstrom, A. D. (2013). Frequency-specific network connectivity increases underlie accurate spatiotemporal memory retrieval. Nat. Neurosci. 16, 349–356. doi: 10.1038/nn.3315
Weinrich, C. A., Brittain, J. S., Nowak, M., Salimi-Khorshidi, R., Brown, P., and Stagg, C. J. (2017). Modulation of long-range connectivity patterns via frequency-specific stimulation of human cortex. Curr. Biol. 27, 3061–3068.e3. doi: 10.1016/j.cub.2017.08.075
Wischnewski, M., and Schutter, D. J. (2015). Efficacy and time course of theta burst stimulation in healhty humans. Brain Stimul. 8, 685–692. doi: 10.1016/j.brs.2015.03.004
Xia, M., Wang, J., and He, Y. (2013). BrainNet viewer: a network visualization tool for human brain connectomics. PLoS One 8:e68910. doi: 10.1371/journal.pone.0068910
Keywords: hippocampus, neuroplasticity, TMS, functional connectivity, theta-burst stimulation
Citation: Hermiller MS (2024) Effects of continuous versus intermittent theta-burst TMS on fMRI connectivity. Front. Hum. Neurosci. 18:1380583. doi: 10.3389/fnhum.2024.1380583
Edited by:
Changming Wang, Capital Medical University, ChinaReviewed by:
Zhipeng Liu, Chinese Academy of Medical Sciences & Peking Union Medical College, ChinaJessica N. Kraft, The University of Texas at Dallas, United States
Laure Spieser, UMR7077 Centre de Recherche en Psychologie et Neurosciences, France
Zhengcao Cao, Beijing Normal University, China
Copyright © 2024 Hermiller. This is an open-access article distributed under the terms of the Creative Commons Attribution License (CC BY). The use, distribution or reproduction in other forums is permitted, provided the original author(s) and the copyright owner(s) are credited and that the original publication in this journal is cited, in accordance with accepted academic practice. No use, distribution or reproduction is permitted which does not comply with these terms.
*Correspondence: Molly S. Hermiller, mhermiller@fsu.edu