Exploratory analysis on the association of dietary live microbe and non-dietary prebiotic/probiotic intake with serum cotinine levels in the general adult population
- 1Department of Ultrasound, The First Affiliated Hospital of Ningbo University, Ningbo, China
- 2Department of Respiratory and Critical Care Medicine, The First Affiliated Hospital of Ningbo University, Ningbo, China
- 3Department of Stomatology, The Affiliated Fuyang Hospital of Anhui Medical University, Fuyang, China
- 4Department of Ultrasound, Ninghai Third Hospital, Ningbo, China
Background: Previous research has indicated the potential involvement of the microbiota in smoking-related processes. The present study seeks to examine the relationship between dietary live microbes, as well as probiotic or prebiotic consumption, and serum cotinine levels.
Methods: This study used data from the National Health and Nutrition Examination Survey 1999–2018. Dietary intake information and probiotic/prebiotic intake data was collected through self-reported questionnaires. Participants were stratified into low, medium, and high intake groups according to their consumption of foods with varying microbial content. Multiple linear models were applied to explore the relationships of dietary live microbes, probiotic or prebiotic use with the serum cotinine level.
Results: A total of 42,000 eligible participants were included in the final analysis. The weighted median serum cotinine level was 0.05 (0.01, 10.90) ng/ml. Participants with low, medium, and high dietary microbe intake represented 35.4, 43.6, and 21.0% of the cohort, respectively. Furthermore, participants were stratified into three groups based on their overall consumption of foods with variable microbe contents. The association between dietary live microbe intake and serum cotinine levels remained robust across all models, with medium intake as the reference (Model 2: β = −0.14, 95% CI: −0.20, −0.07; High: β = −0.31, 95% CI: −0.39, −0.22). Moreover, both prebiotic and probiotic use exhibited an inverse relationship with serum cotinine levels (Prebiotic: β = −0.19, 95% CI: −0.37, −0.01; Probiotic: β = −0.47, 95% CI: −0.64, −0.30). Subgroup analyses revealed no discernible interactions between dietary live microbe, prebiotic, probiotic use, and serum cotinine levels.
Conclusion: Our findings suggest a negative correlation between dietary live microbe intake, as well as non-dietary prebiotic/probiotic consumption, and serum cotinine levels.
Introduction
Despite increased awareness of the adverse effects of cigarette smoking and ongoing tobacco control efforts, the global prevalence of regular smokers remains substantial, affecting 22.3% of the world’s population (1). Smoke exposure has been shown to increase susceptibility to infections caused by pathogens and exacerbate conditions such as asthma (2). The deleterious constituents of cigarette smoke, notably nicotine, are widely recognized as significant contributors to severe illnesses, prompting extensive investigation into their underlying pathological mechanisms (3). As a primary immediate metabolite of nicotine, cotinine serves as a reliable and sensitive indicator of exposure to cigarette smoke. Besides tobacco smoking, nicotine has been found in flora and some vegetables, such as tomatoes, potatoes, and peppers (4). While the nicotine content in these foods is typically low compared to tobacco products, its presence can still contribute to cotinine levels, albeit to a lesser extent (4).
Cigarette smoking and cessation can impact the gut environment, potentially inducing alterations in the commensal microbial community, including shifts in gut microbiota balance (5). Numerous studies have indicated that smoking may alter the composition of the periodontal, upper esophageal, and gastric, as well as respiratory microbiomes (6–8). An intriguing question arises regarding the potential influence of gut microbiota on smoking behaviors. Recent research suggests that disruptions in gut microbiota, termed dysbiosis, are implicated in various chronic human diseases (9). For instance, dysbiosis may contribute to the development of hypertension (10). Short-chain fatty acids (SCFAs), such as propionate, produced by gut bacteria from dietary fiber, have been found to mitigate organ damage associated with hypertension (10). Additionally, the antibiotic azithromycin has been observed to modulate gut microbiota, leading to reduced airway inflammation in individuals with allergic asthma (11). However, the involvement of microbiota in smoking-related chronic diseases, including chronic obstructive pulmonary disease, remains unclear.
Probiotics and prebiotics, commonly integrated into dietary supplements, which probiotics are living microorganisms characterized by their capacity to bestow health benefits upon the host and prebiotics are non-living substances that promote the growth and activity of beneficial bacteria in the gut. Numerous studies have underscored the therapeutic potential of probiotics in managing asthma and respiratory infections (12, 13). Probiotics, as living microorganisms, confer significant health advantages to the host when administered in sufficient quantities (14). Administration of probiotics has been shown to augment the development of immune and metabolic conditions such as obesity, diabetes, and inflammatory bowel disease (14, 15). Prebiotics, on the other hand, are non-digestible components in food that exert positive effects by selectively fostering the growth and activity of specific bacteria in the colon, ultimately enhancing individuals’ health (16). Moreover, prebiotics exhibit beneficial effects on conditions such as diarrhea, obesity, type II diabetes, and colorectal cancer (17).
Currently, substantial research attention has been devoted to the microbiota-gut-brain axis, which posits that alterations in gut microbiota may influence brain function (18). Notably, neurological functions of the brain have been found to be intricately linked to cigarette smoke. Specifically, two brain regions—the orbitofrontal cortex and the prefrontal cortex—have been identified as interacting to either activate or suppress nicotine cravings (19, 20). Furthermore, animal studies have directly illustrated that manipulation of the gut microbiome can modulate behaviors related to rewards and stress associated with substance abuse, including tobacco (21, 22). Hence, the microbiota may indeed play a role in the process of smoking, and consumption of live microbes could represent a potential avenue for addressing cigarette use. However, the precise relationships between dietary live microbes and smoking behaviors remain unclear. Our study seeks to investigate the association of dietary live microbes, probiotic or prebiotic usage, with serum cotinine levels, leveraging data from a large population obtained from the National Health and Nutrition Examination Survey (NHANES).
Materials and methods
Study population
The NHANES is an ongoing program conducted by the National Center for Health Statistics within the Centers for Disease Control and Prevention, administered through a stratified, multistage probability sampling design.1 All data and information from NHANES are publicly accessible. Trained interviewers collected participant data via administered standardized questionnaires in participants’ homes. Written informed consent was obtained from all individuals, and all research protocols were approved by the National Center for Health Statistics’ ethical review board. Further detailed information can be found on the NHANES website.
We collected data and characteristics of 101,316 participants from NHANES between 1999 and 2018, following NHANES sample weight guidelines.2 Participants with missing data assessing dietary live microbe intake (n = 11,876) were excluded. Additionally, individuals aged less than 20 (n = 40,689), those with missing serum cotinine data (n = 2,557), missing smoking status data (n = 35), pregnant individuals (n = 1,294), and those with extreme energy intake at baseline (n = 2,865) were excluded. In total, 42,000 eligible participants were included in the final analyses. Detailed information on the sample selection procedure is displayed in Figure 1.
Assessment of live microbe intake from foods and non-dietary prebiotic/probiotic intake
The estimation of dietary live microbe intake was established using a methodology developed by professional experts based on a total of 9,388 participant food codes in 48 subgroups within the NHANES program (23). Utilizing estimated levels of live microbial content, including bacteria and fungi, foods were classified into three groups based on the quantity of live microorganisms per gram of food: low (Lo, <104 CFU/g in foods), medium (Med, 104–107 CFU/g in foods), and high (Hi, >107 CFU/g in foods). The designations of Lo, Med, and Hi were selected to represent the expected quantities of viable microbes in foods. The Hi classification primarily consists of fermented items, such as yogurt and fermented kimchi (23). The Med classification comprises fresh fruits and vegetables typically consumed without peeling (23). The Lo levels could represent the numbers of microbes in pasteurized foods (23). Additionally, a fourth category, named MedHi, was established, encompassing individuals who consume foods from the Med, Hi, or both the Medium and High categories. When both Med and Hi foods were simultaneously analyzed as separate terms in the same model, their coefficients were found to be similar. This finding supports a simpler model where the exposure combines Med and Hi foods into a single variable termed MedHi (23). Non-dietary probiotic and prebiotic intake data were collected from the Food Frequency Questionnaire (FFQ) and Dietary Supplement Use 30-Day (DSQ) using detailed text-mining for key phrases.
Participants were stratified into three distinct groups based on their overall consumption of foods with varying contents of live microbes: low (participants exclusively consumed foods classified as Low in microbial content), moderate (participants consumed any foods classified as Medium but not High), and high (participants consumed any foods classified as High). Additionally, participants were categorized into three groups based on their consumption of MedHi foods to quantify the ingestion of live microbes: Group 1 (G1) comprised consumers with no intake of any MedHi food; Group 2 (G2) consisted of individuals with intake of MedHi food above zero but below the median level for consumers; and Group 3 (G3) encompassed participants with intake of MedHi food above the median level for consumers.
Assessment of serum cotinine
Serum cotinine measurements were obtained via venipuncture and analyzed utilizing an isotope-dilution high-performance liquid chromatography/atmospheric pressure chemical ionization tandem mass spectrometric (ID HPLC-APCI MS/MS) method. In brief, serum samples were fortified with methyl-D3-cotinine and methyl-D3-hydroxycotinine as internal standards. Following basification, the samples were applied to a supported liquid extraction (SLE) plate. Analytes were extracted using an isopropanol/methylene chloride mixture, and the resulting organic extract was concentrated before injection onto a C18 HPLC column. The mobile phase used for chromatographic separation consisted of solvent A (6 mM ammonium acetate in water/methanol) and solvent B (methanol). The chromatographic run was performed using gradient elution with a flow rate of 0.5 mL/min, column temperature of 30°C, and a gradient profile of 10–90% solvent B over 5 min. The total run time was 10 min, with an injection volume of 10 microliters. APCI-MS/MS monitored the eluent from these injections. Cotinine was quantified using the m/z 80 product ion from the m/z 177 quasi-molecular ion. Additional ions for the internal standards and confirmation were also monitored. Analyte concentrations were determined from the area ratios of native-to-labeled compounds in the sample, compared to a standard curve. Further details on specific laboratory procedures can be found on the website.3
Assessment of other covariates
We assessed the covariates as follows: age (20–39 years, 40–59 years, or ≥ 60 years), sex, race (non-Hispanic White, non-Hispanic Black, or other races), marital status (married/living with partner, or single/divorced/widowed), education level (below high school, high school, above high school), family poverty-to-income ratio (PIR), smoking status, drinking status, body mass index (BMI, <25.0 kg/m2, 25.0–29.9 kg/m2, or > 29.9 kg/m2), physical activity, healthy eating index (HEI), Charlson comorbidity index.
PIR served as an indicator of family income levels (24), with three categories established: ≤1.0, 1.1–3.0, >3.0. Smoking status was categorized into three groups: never smoker (individuals reporting having smoked fewer than 100 cigarettes throughout their lifetime), former smoker (those reporting having smoked more than 100 cigarettes but subsequently quit), current smoker (actively engaged in smoking) (25). Drinking status was divided into three groups: nondrinker, low-to-moderate drinker (consuming alcohol at a rate of less than 2 drinks per day for men and less than 1 drink per day for women), heavy drinker (exceeding 2 drinks per day for men and 1 or more drinks per day for women) (25). Physical activity was categorized based on metabolic equivalent (MET) levels, including three categories: inactive (no leisure-time physical activity), insufficiently active (moderate activity 1–5 times per week with MET ranging from 3 to 6, or vigorous activity 1–3 times per week with MET greater than 6), and active (engagement in physical activity beyond the levels described in the aforementioned categories) (25). The Healthy Eating Index (HEI) is a metric devised by the United States Department of Agriculture (USDA) to evaluate the overall nutritional quality of an individual’s or population’s diet (26). It furnishes a comprehensive score derived from multiple facets of dietary intake, indicative of adherence to fundamental dietary guidelines. HEI computation was predicated on the dietary intakes recorded on the initial day for each participant, adhering to HEI scoring standards outlined on the National Cancer Institute’s Epidemiology and Genomics Research Program website.4 The Charlson comorbidity index was utilized to quantify participants’ holistic health status, entailing the summation of scores attributed to various chronic conditions (27).
Statistical analyses
Adhering to the analytical guidelines delineated by the National Center for Health Statistics (NCHS), our analysis incorporated primary sampling units, sample weights, and strata to yield dependable national estimates. Data analyses were conducted employing the “survey” package in R to conduct weighted analyses. Participant characteristics were presented as means with standard errors (SEs) for continuous variables and as numbers with weighted percentages for categorical variables. Group-wise comparisons for continuous variables were executed utilizing weighted t-tests or one-way ANOVA, while categorical variables were compared among groups using weighted chi-square tests.
Multiple linear regression models were deployed to evaluate the relationship between dietary live microbe and non-dietary prebiotic/probiotic intake with serum cotinine levels among American adults. β coefficients and 95% confidence intervals were employed to assess these associations. In regression models utilizing dietary live microbe as a continuous exposure, the reported regression coefficients denote the adjusted mean difference in the outcome per 100-unit increase in the exposure. We formulated two models: Model 1, adjusted for age (20–39, 40–59, or ≥ 60), sex (male or female), race/ethnicity (non-Hispanic White, non-Hispanic Black, or other race), marital status (married/living with partner, or single/divorced/widowed), education level (below high school, high school, or above high school), family poverty-to-income ratio (≤1.0, 1.1–3.0, or > 3.0), drinking status (nondrinker, former drinker, or current drinker), BMI (<25.0, 25.0–29.9, or > 29.9), physical activity (inactive, insufficiently active, or active), Healthy Eating Index (HEI, in quartiles), and Charlson comorbidity index (continuous); Model 2, adjusted as Model 1 with the inclusion of smoking status (never smoker, former smoker, or current smoker).
Subgroup analyses, stratified by age, sex, race, marital status, educational level, family poverty-to-income ratio, smoking status, drinking status, physical activity, BMI, HEI, and Charlson comorbidity index, were conducted using stratified multivariate regression analysis. Additionally, we constructed forest plots depicting β coefficients and confidence intervals in different subgroups to facilitate visualization of the relationships between non-dietary prebiotic and probiotic intake and serum cotinine levels, respectively. All analyses were performed using R (version 4.3.2), and statistical significance was defined as p < 0.05.
Results
Baseline characteristics according to MedHi category
Table 1 presented baseline characteristics for 42,000 participants who consumed MedHi foods. Among them, 14,852 individuals were categorized in the low microbe consumption group, 18,302 individuals in the moderate microbe consumption group, and 8,846 individuals in the high microbe consumption group. Of the total participants, 13,252 (35.70%) were aged 20–39 years, 13,756 (38.29%) were aged 40–59 years, and 14,992 (26.01%) were aged ≥60 years. There were 21,629 (52.31%) females and 20,371 (47.69%) males. The weighted median of serum cotinine was 0.05 ng/mL. Participants in the high MedHi category were more likely to be middle-aged (40–59 years old), female, non-Hispanic White, single/divorced/widowed, never smokers, low-to-moderate drinkers, engaged in active physical activity, with higher family PIR, higher education level, lower Charlson comorbidity index, and lower serum cotinine level (all p < 0.001, except for Charlson comorbidity index where p = 0.02). Additionally, Supplementary Table S1 outlines the distribution of dietary live microbe intake among adults in the NHANES 1999–2018. Supplementary Table S2 provides a comprehensive overview of the sociodemographic and health status characteristics of adult participants from the NHANES 1999–2018, stratified by serum cotinine levels (<10 ng/mL, or ≥ 10 ng/mL).
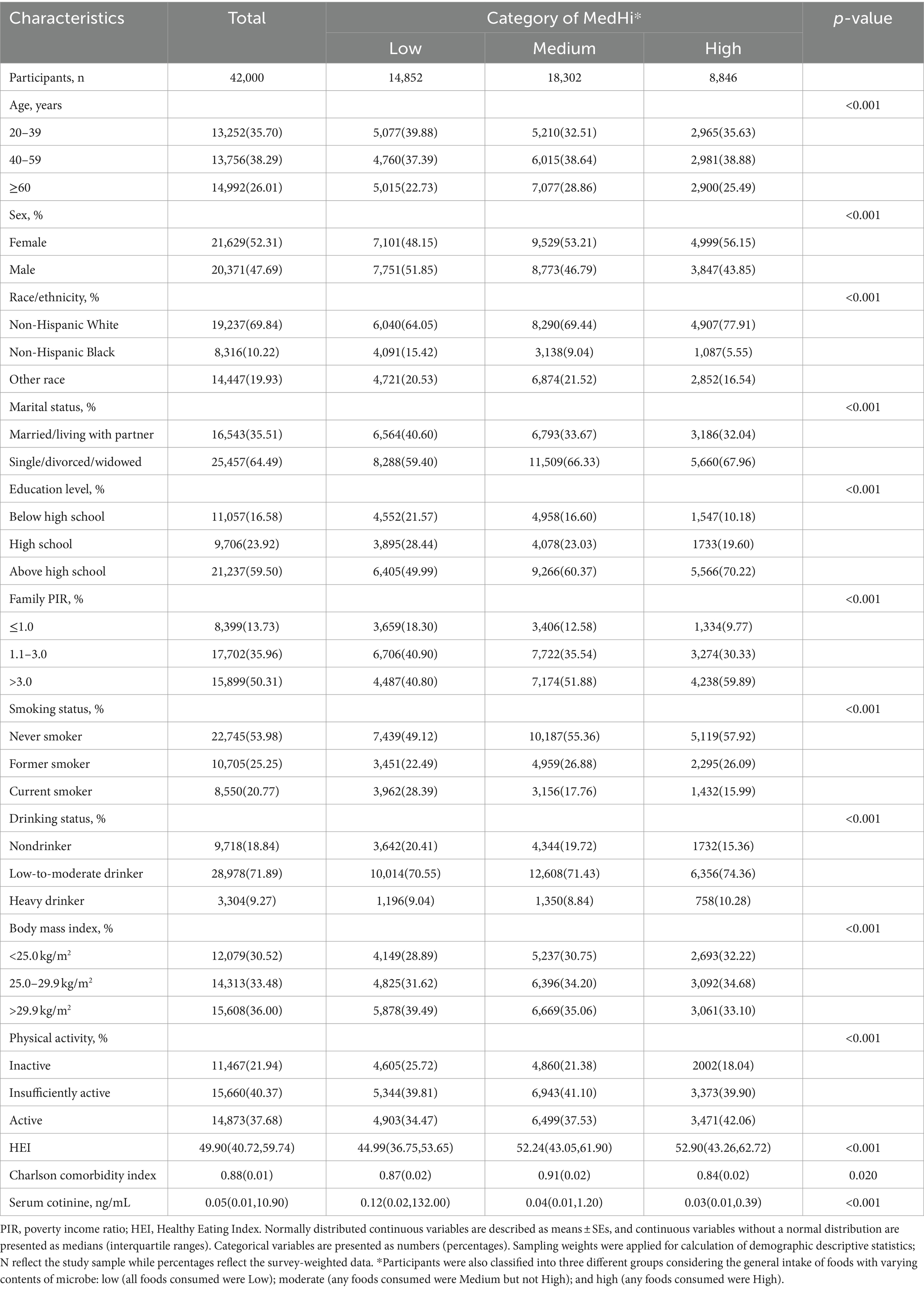
Table 1. Baseline characteristics of the adult participants by category of MedHi in NHANES 1999–2018.
Associations of dietary live microbe and non-dietary prebiotic/probiotic intake with serum cotinine levels
The results of linear regression analysis examining the associations of dietary live microbe and non-dietary prebiotic/probiotic intake with serum cotinine levels are presented in Table 2. Dietary live microbe intake exhibited a negative correlation with serum cotinine levels. Specifically, each 100-unit increase in MedHi foods was associated with average reductions in serum cotinine levels of −0.4 (95% CI: −0.43, −0.37; p < 0.001) ng/mL in crude analysis, −0.17 (95% CI: −0.19, −0.14; p < 0.001) ng/mL in model 1, and − 0.07 (95% CI: −0.09, −0.06; p < 0.001) ng/mL in model 2. Furthermore, when participants were stratified into three groups based on their general intake of foods with varying microbial contents, the association between dietary live microbe and serum cotinine levels remained significant across all models (with the Low category as reference; Model 2: Medium: β = −0.14, 95% CI: −0.20, −0.07; High: β = −0.31, 95% CI: −0.39, −0.22, p < 0.001). Similarly, categorization of participants into three groups based on MedHi consumption to quantify live microbe ingestion yielded significant results (with G1 as reference; Model 2: G2: β = −0.13, 95% CI: −0.20, −0.06; G3: β = −0.28, 95% CI: −0.36, −0.20, p < 0.001). Regarding prebiotic use outcomes, estimated effects demonstrated a negative association with serum cotinine levels across all models (Crude: β = −1.05, 95% CI: −1.36, −0.75; Model 1: β = −0.51, 95% CI: −0.80, −0.21; Model 2: β = −0.19, 95% CI: −0.37, −0.01). Similarly, probiotic use exhibited an inverse relationship with serum cotinine levels (Crude: β = −1.43, 95% CI: −1.69, −1.17; Model 1: β = −0.72, 95% CI: −0.99, −0.44; Model 2: β = −0.47, 95% CI: −0.64, −0.30).
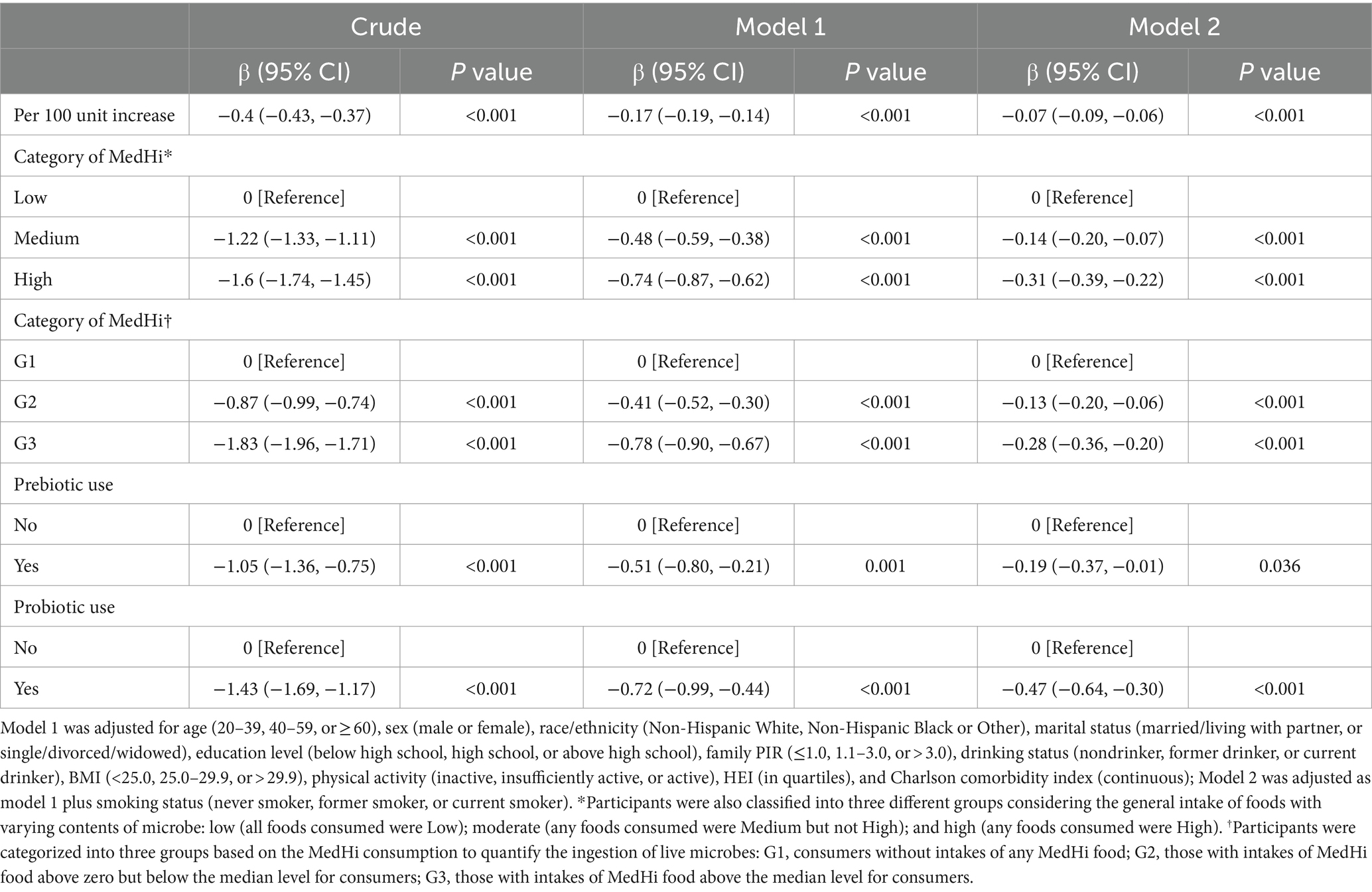
Table 2. Linear regression analysis of dietary live microbe and non-dietary prebiotic/probiotic intake with serum cotinine levels among adults in NHANES 1999–2018.
Subgroup analysis and interaction analysis
Table 3 presents the associations of dietary live microbe intake within different subgroups stratified by various covariates with serum cotinine levels. An interaction test, stratified by age, sex, race, marital status, education level, PIR, smoking status, drinking status, physical activity, BMI, HEI, and Charlson comorbidity index, was conducted. A significant interaction between race (P for interaction = 0.007), PIR (P for interaction = 0.037), smoking status (P for interaction = 0.007) and serum cotinine levels. However, no statistically significant interaction between dietary live microbe intake and serum cotinine levels was observed in other subgroups. This indicates that the association remained consistent across different demographic and health-related variables, including age, sex, marital status, education level, alcohol consumption, physical activity, BMI, HEI, and Charlson Comorbidity Index (all P for interaction >0.05). Figure 2 illustrates the stratified analyses of the associations between prebiotic and probiotic use and serum cotinine levels through a forest plot. No discernible interaction was found between prebiotic and probiotic use and serum cotinine levels in these subgroup results.
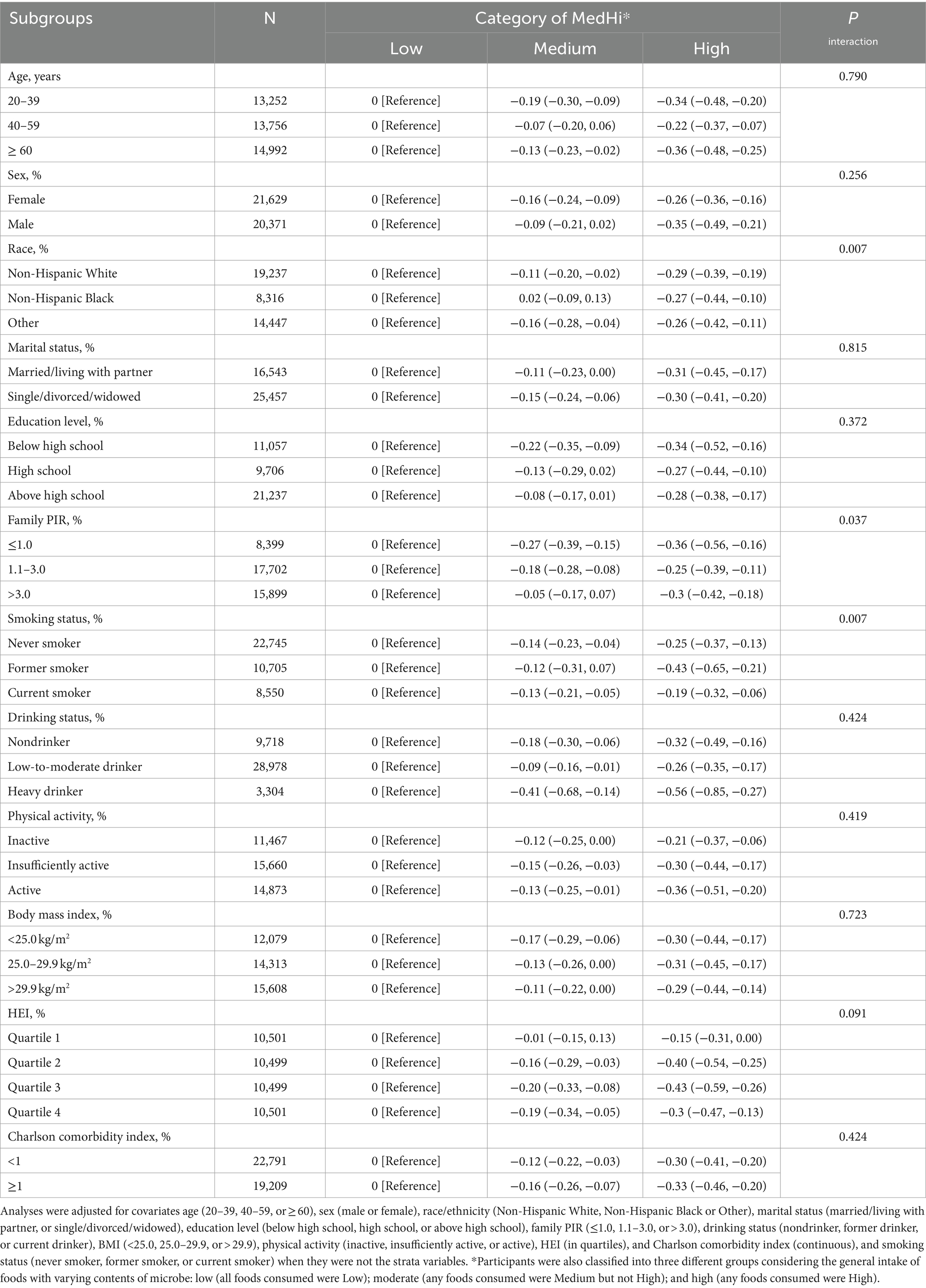
Table 3. Stratified analyses of the associations between dietary live microbe intake and serum cotinine levels among adults in NHANES 1999–2018.
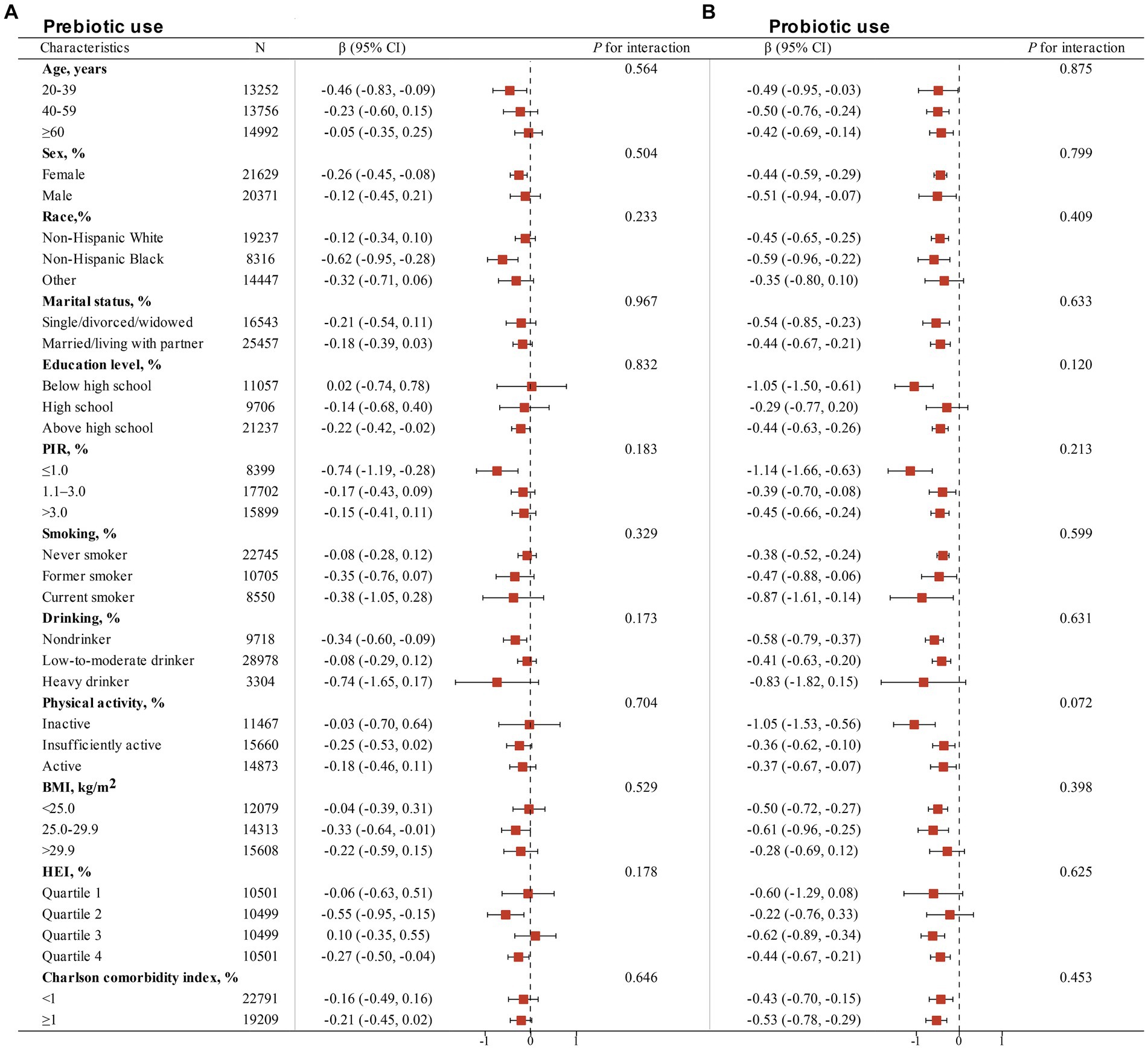
Figure 2. Stratified analyses of the associations of non-dietary prebiotic (A) and probiotic (B) intake with serum cotinine levels among adults in NHANES 1999–2018. Analyses were adjusted for covariates age (20–39, 40–59, or ≥ 60), sex (male or female), race/ethnicity (Non-Hispanic White, Non-Hispanic Black or Other), marital status (married/living with partner, or single/divorced/widowed), education level (below high school, high school, or above high school), family PIR (≤1.0, 1.1–3.0, or > 3.0), drinking status (nondrinker, former drinker, or current drinker), BMI (<25.0, 25.0–29.9, or > 29.9), physical activity (inactive, insufficiently active, or active), HEI (in quartiles), and Charlson comorbidity index (continuous), and smoking status (never smoker, former smoker, or current smoker) when they were not the strata variables.
Sensitivity analysis
To ensure the robustness of the findings, a series of sensitivity analyses were conducted. Supplementary Table S3 presents the results of a sensitivity analysis conducted to examine the association between dietary live microbe intake and serum cotinine levels categorized as <10 ng/mL or ≥ 10 ng/mL among adults participating in NHANES 1999–2018. Consistent with the linear regression analysis, the results of logistic regression indicated that increased intake of dietary live microbe and non-dietary prebiotic/probiotic intake were associated with reduced odds of having serum cotinine levels ≥10 ng/mL (Supplementary Table S3). This association remained statistically significant across all models. Supplementary Table S4 presents the results of stratified analyses investigating the associations between dietary live microbe intake and serum cotinine levels (<10 ng/mL or ≥ 10 ng/mL), stratified by various demographic and health-related factors. The findings suggest that the association between dietary live microbe intake and serum cotinine levels was robust and not significantly modified by demographic or health-related factors examined in this analysis.
Supplementary Tables S5, S6 present the results of linear regression analyses investigating the relationship between serum cotinine levels and dietary live microbe intake, as well as non-dietary prebiotic/probiotic intake among adults participating in NHANES 1999–2018. Despite this difference in categorization of dietary live microbe intakes in Supplementary Tables S5, S6, the results consistently demonstrated an inverse association between dietary live microbe intake and serum cotinine levels. Similarly, individuals who reported prebiotic or probiotic use exhibited lower serum cotinine levels compared to those who did not use prebiotics or probiotics.
Discussion
This study investigated the association between dietary intake of live microorganisms and non-dietary consumption of prebiotics/probiotics with serum cotinine levels among adults. Our findings revealed that the consumption of foods containing live microbes was associated with lower serum cotinine levels. This negative association persisted across different levels of MedHi categorization (G1–G3) in Table 2. Similarly, the use of probiotic and prebiotic supplements demonstrated similar results. Subgroup analyses and interaction assessments consistently indicated the persistence of this negative association across various demographic and health-related factors (Table 3). To the best of our knowledge, this study represents the first comprehensive investigation into the association between dietary intake of live microorganisms and non-dietary consumption of prebiotics/probiotics with serum cotinine levels.
The consumption of live and safe microbes has been advocated as a recommendation due to their association with health benefits (28). Dietary intake of live microbes has been demonstrated to reduce markers of inflammation, enhance immunity, modulate stress responses, and mitigate the risk of several chronic diseases (28, 29). One plausible explanatory mechanism for these findings revolves around the interconnectedness of food, the gut, and overall health (30). An expanding body of evidence, albeit partly indirect, suggests potential biological rationales for the impact of commensal gut microbiota, particularly probiotics such as Bifidobacterium, on smoking behavior. The vagus nerve is believed to serve as a prominent modulatory and constitutive communication pathway linking intestinal bacteria to the brain (31). Through the vagus nerve, Bifidobacterium longum has been observed to transmit signals to the brain, leading to increased dopamine secretion (31). Additionally, the influence of the intestinal microbiome on smoking behavior may be mediated through neurotransmitters. Some reports indicate that Bifidobacterium contributes to enhanced serotonin (5-HT) biosynthesis in colonic enterochromaffin cells (32). This process is achieved by promoting the activity of the CGA/ADRα2A pathway and modulating the TRP/TPH-OR pathways (33). Given that 5-HT has been identified as a therapeutic target for addiction to various substances, including alcohol, cocaine, and drugs, it is conceivable that targeting serotonin may also offer therapeutic potential for smoking addiction (34). The observed impact of Bifidobacterium on smoking behavior may be attributed to the close association of its metabolites, such as short-chain fatty acids like acetate, or components like peptidoglycan, with the central nervous system (CNS) (35, 36). Short-chain fatty acids may play a significant role in microglial function and brain physiology (37). While these studies provide some evidence for the association between gut microbiota and smoking behavior, the underlying mechanisms remain incompletely understood. The gap between serum cotinine levels and comprehensive smoking activity has not been investigated at the population level. Future studies should focus on elucidating specialized mechanisms and strengthening observational evidence in this domain.
Moreover, a previous meta-analysis has indicated a significant enhancement in host oxidative stress homeostasis through the substantial improvement of glutathione (GSH) levels following probiotic or prebiotic consumption (38). Additionally, lactic acid bacteria have been shown to ameliorate oxidative imbalance among antioxidant factors by regulating AhR and Nrf2 gene expression in bronchial epithelial cells exposed to cigarette smoke (39). A recent animal study demonstrated that the probiotic Bifidobacterium longum subsp. longum alleviates lung inflammation induced by cigarette smoke in mice, as evidenced by a reduction in the count of immune cells in bronchoalveolar lavage fluid (BALF) and lung parenchyma (40). The administration of Bifidobacterium probiotics has been associated with a decreased occurrence of respiratory infections in humans (41, 42). The reduced expression of adhesion molecules Icam1 and Vcam1 is linked to the mitigation of immune cell adhesion and migration from the bloodstream to lung tissue, highlighting the anti-inflammatory effects of B. longum subsp. longum. The aforementioned evidence suggests that probiotics may have the potential to alleviate the burden of smoking-related chronic diseases by addressing their pathogenic mechanisms. Prebiotics are non-digestible fibers that selectively stimulate the growth and activity of beneficial bacteria in the gut (43). Prebiotics can alter the composition of the gut microbiota, favoring the growth of certain beneficial bacteria over others (44). In turn, these beneficial bacteria can influence various metabolic processes in the body, including those related to xenobiotic metabolism, such as nicotine (45, 46). Prebiotics have been shown to have anti-inflammatory and antioxidant effects in some studies. A study explored the influence of prebiotics in bread on antioxidative status and antioxidative ability in smokers and non-smokers (47). They found that consuming prebiotic bread could decrease the levels of stress biomarkers (47). The potential relationship between prebiotics and cotinine levels has not been extensively studied, more mechanisms need to be explored.
The decrease of cotinine in plasma typically correlates with a reduction in tobacco consumption. Cotinine is a metabolite of nicotine, serving as a reliable and objective measure of nicotine exposure (48). Cotinine has a longer half-life than nicotine and remains detectable in bodily fluids such as blood, urine, and saliva for a longer duration, making it an excellent biomarker for assessing recent tobacco exposure (48). Cigarette smoking has been demonstrated to induce alterations in the composition of the gut microbiota (49). Compared to non-smokers, current smokers exhibit a decreased Firmicutes/Bacteroidetes (F/B) ratio and a lower relative abundance of the phyla Firmicutes and Proteobacteria (49). Several studies have suggested that the gut microbiota may play a role in both the development and potential treatment of smoking-induced diseases. The gut microbiota may have the potential to metabolize cotinine (50). Some research indicated that certain bacterial species can metabolize nicotine and its major metabolites into other compounds (50). Prebiotics and probiotics represent promising targets for treatment in chronic diseases caused by cigarette smoking, such as chronic obstructive pulmonary disease (COPD). Previous studies have indicated that the administration of probiotic B. longum subsp. longum mitigates lung inflammation induced by cigarette smoke in mice (40). Administration of probiotics, such as L. rhamnosus and P. goldsteinii, may attenuate symptoms in tobacco smoking-induced COPD by reducing the inflammatory response (51, 52). Prebiotics, as non-digestible food components that promote the growth and activity of beneficial bacteria in the colon, have also shown promise in promoting overall health (16). Feeding mice a high-fiber diet rich in cellulose or pectin for 3 weeks has demonstrated a protective effect against the progression of emphysema induced by cigarette smoking (53). This evidence suggests that utilizing probiotics tailored to the composition of the gut microbiota could serve as a promising therapeutic approach for ameliorating symptoms associated with chronic diseases caused by tobacco smoking.
Our study has some advantages. Our research represents the inaugural investigation into the correlation between dietary live microbes and serum cotinine levels on a large scale within a nationally representative program. This pioneering effort lays the groundwork for future studies in this area. Moreover, by utilizing data from a nationally representative program, our study offers insights that are likely to be generalizable to the broader population. This enhances the external validity of our findings and contributes to a better understanding of the relationship between dietary live microbes and serum cotinine levels at a national level. Furthermore, we comprehensively assessed dietary live microbe intake and serum cotinine levels, providing a detailed examination of these variables within the context of smoking behavior. This thorough approach strengthens the robustness of our analysis and allows for a more nuanced interpretation of the results.
However, several limitations of our research should be mentioned. First of all, the cross-sectional nature of our study limits our ability to establish causal relationships between dietary live microbe intake, prebiotic/probiotic consumption, and serum cotinine levels. Future longitudinal studies are warranted to elucidate the directionality of these associations over time. Moreover, the reliance on self-reported dietary live microbe and prebiotic/probiotic intake data introduces the potential for memory bias and reporting inaccuracies. Participants may not accurately recall their dietary habits, leading to measurement errors and potential misclassification of exposure levels. Thirdly, while we made efforts to estimate dietary live microbe intake, the precision of these measurements may be compromised due to inherent limitations in quantifying microbial content in food. Variations in food processing, storage conditions, and microbial composition introduce uncertainty into these estimates. Further, our findings may not be directly applicable to populations outside of the study’s geographic region or cultural context. The classification of food and the prevalence of live microbe intake can vary significantly across different regions and populations, limiting the generalizability of our results.
Conclusion
Our study, the first of its kind to investigate the relationship between dietary live microbes and serum cotinine levels on a large, nationally representative scale, yields significant insights into the interplay between microbiota and exposure to cigarette smoke. We found a consistent negative association between the consumption of live microbes, probiotics, or prebiotics and serum cotinine levels, suggesting a potential protective effect against the harmful effects of smoking. Future studies should employ longitudinal designs to better elucidate causality and explore the potential therapeutic implications of modulating the gut microbiota in individuals exposed to cigarette smoke.
Data availability statement
The datasets presented in this study can be found in online repositories. The names of the repository/repositories and accession number(s) can be found in the article/Supplementary material.
Ethics statement
The studies involving humans were approved by the CDC/NCHS Research Ethics Review Board from NAHENS. The studies were conducted in accordance with the local legislation and institutional requirements. The participants provided their written informed consent to participate in this study. The animal study was approved by the CDC/NCHS Research Ethics Review Board from NHANES. The study was conducted in accordance with the local legislation and institutional requirements.
Author contributions
SL: Conceptualization, Data curation, Formal analysis, Investigation, Software, Writing – original draft, Writing – review & editing. NZ: Conceptualization, Data curation, Formal analysis, Investigation, Methodology, Writing – original draft, Writing – review & editing. YZ: Data curation, Methodology, Project administration, Resources, Writing – review & editing. HM: Data curation, Formal analysis, Methodology, Software, Writing – review & editing. SZ: Conceptualization, Data curation, Project administration, Resources, Supervision, Validation, Visualization, Writing – review & editing.
Funding
The author(s) declare financial support was received for the research, authorship, and/or publication of this article. This study was supported by Science and Technology Project of Medicine and Health in Zhejiang Province (2024KY1546).
Acknowledgments
The data from NHANES collection were sponsored by the CDC.
Conflict of interest
The authors declare that the research was conducted in the absence of any commercial or financial relationships that could be construed as a potential conflict of interest.
Publisher’s note
All claims expressed in this article are solely those of the authors and do not necessarily represent those of their affiliated organizations, or those of the publisher, the editors and the reviewers. Any product that may be evaluated in this article, or claim that may be made by its manufacturer, is not guaranteed or endorsed by the publisher.
Supplementary material
The Supplementary material for this article can be found online at: https://www.frontiersin.org/articles/10.3389/fnut.2024.1405539/full#supplementary-material
Footnotes
1. ^https://www.cdc.gov/nchs/nhanes/index.htm
2. ^https://wwwn.cdc.gov/nchs/nhanes/analyticguidelines.aspx
3. ^https://wwwn.cdc.gov/Nchs/Nhanes/2013-2014/COT_H.htm
4. ^https://epi.grants.cancer.gov/hei/hei-scoring-method.html
References
1. World Health Organization. Tobacco. (2022). Available at: https://www.who.int/tobacco. (Accessed July 28, 2022).
2. Oberg, M, Jaakkola, MS, Woodward, A, Peruga, A, and Prüss-Ustün, A. Worldwide burden of disease from exposure to second-hand smoke: a retrospective analysis of data from 192 countries. Lancet. (2011) 377:139–46. doi: 10.1016/S0140-6736(10)61388-8
3. Lee, PN, and Fariss, MW. A systematic review of possible serious adverse health effects of nicotine replacement therapy. Arch Toxicol. (2017) 91:1565–1594. doi: 10.1007/s00204-016-1856-y
4. Ma, C, Liu, Y, Neumann, S, and Gao, X. Nicotine from cigarette smoking and diet and Parkinson disease: a review. Transl Neurodegener. (2017) 6:18. doi: 10.1186/s40035-017-0090-8
5. Gui, X, Yang, Z, and Li, MD. Effect of cigarette smoke on gut microbiota: state of knowledge. Front Physiol. (2021) 12:673341. doi: 10.3389/fphys.2021.673341
6. Allais, L, Kerckhof, FM, Verschuere, S, Bracke, KR, De Smet, R, Laukens, D, et al. Chronic cigarette smoke exposure induces microbial and inflammatory shifts and mucin changes in the murine gut. Environ Microbiol. (2016) 18:1352–63. doi: 10.1111/1462-2920.12934
7. Morris, A, Beck, JM, Schloss, PD, Campbell, TB, Crothers, K, Curtis, JL, et al. Comparison of the respiratory microbiome in healthy nonsmokers and smokers. Am J Respir Crit Care Med. (2013) 187:1067–75. doi: 10.1164/rccm.201210-1913OC
8. Vogtmann, E, Flores, R, Yu, G, Freedman, ND, Shi, J, Gail, MH, et al. Association between tobacco use and the upper gastrointestinal microbiome among Chinese men. Cancer Causes Control. (2015) 26:581–8. doi: 10.1007/s10552-015-0535-2
9. Durack, J, and Lynch, SV. The gut microbiome: Relationships with disease and opportunities for therapy. J Exp Med. (2019) 216:20–40. doi: 10.1084/jem.20180448
10. Li, J, Zhao, F, Wang, Y, Chen, J, Tao, J, Tian, G, et al. Gut microbiota dysbiosis contributes to the development of hypertension. Microbiome. (2017) 5:14. doi: 10.1186/s40168-016-0222-x
11. Park, HK, Choi, Y, Lee, DH, Kim, S, Lee, JM, Choi, SW, et al. Altered gut microbiota by azithromycin attenuates airway inflammation in allergic asthma. J Allergy Clin Immunol. (2020) 145:1466–1469.e8. doi: 10.1016/j.jaci.2020.01.044
12. Sagar, S, Morgan, ME, Chen, S, Vos, AP, Garssen, J, van Bergenhenegouwen, J, et al. Bifidobacterium breve and Lactobacillus rhamnosus treatment is as effective as budesonide at reducing inflammation in a murine model for chronic asthma. Respir Res. (2014) 15:46. doi: 10.1186/1465-9921-15-46
13. Hougee, S, Vriesema, AJM, Wijering, SC, Knippels, LMJ, Folkerts, G, Nijkamp, FP, et al. Oral treatment with probiotics reduces allergic symptoms in ovalbumin-sensitized mice: a bacterial strain comparative study. Int Arch Allergy Immunol. (2010) 151:107–17. doi: 10.1159/000236000
14. Hill, C, Guarner, F, Reid, G, Gibson, GR, Merenstein, DJ, Pot, B, et al. Expert consensus document. The International Scientific Association for Probiotics and Prebiotics consensus statement on the scope and appropriate use of the term probiotic. Nat Rev Gastroenterol Hepatol. (2014) 11:506–14. doi: 10.1038/nrgastro.2014.66
15. Ballini, A, Santacroce, L, Cantore, S, Bottalico, L, Dipalma, G, Topi, S, et al. Probiotics efficacy on oxidative stress values in inflammatory bowel disease: a randomized double-blinded placebo-controlled pilot study. Endocr Metab Immune Disord Drug Targets. (2019) 19:373–81. doi: 10.2174/1871530319666181221150352
16. Gibson, GR, and Roberfroid, MB. Dietary modulation of the human colonic microbiota: introducing the concept of prebiotics. J Nutr. (1995) 125:1401–12. doi: 10.1093/jn/125.6.1401
17. Olveira, G, and González-Molero, I. An update on probiotics, prebiotics and symbiotics in clinical nutrition. Endocrinol Nutr. (2016) 63:482–94. doi: 10.1016/j.endonu.2016.07.006
18. Cryan, JF, O'Riordan, KJ, Cowan, CSM, Sandhu, KV, Bastiaanssen, TFS, Boehme, M, et al. The microbiota-gut-brain axis. Physiol Rev. (2019) 99:1877–2013. doi: 10.1152/physrev.00018.2018
19. Spinella, M. Correlations between orbitofrontal dysfunction and tobacco smoking. Addict Biol. (2002) 7:381–4. doi: 10.1080/1355621021000005964
20. Hayashi, T, Ko, JH, Strafella, AP, and Dagher, A. Dorsolateral prefrontal and orbitofrontal cortex interactions during self-control of cigarette craving. Proc Natl Acad Sci USA. (2013) 110:4422–7. doi: 10.1073/pnas.1212185110
21. Meckel, KR, and Kiraly, DD. A potential role for the gut microbiome in substance use disorders. Psychopharmacology. (2019) 236:1513–30. doi: 10.1007/s00213-019-05232-0
22. Lee, K, Vuong, HE, Nusbaum, DJ, Hsiao, EY, Evans, CJ, and Taylor, AMW. The gut microbiota mediates reward and sensory responses associated with regimen-selective morphine dependence. Neuropsychopharmacology. (2018) 43:2606–14. doi: 10.1038/s41386-018-0211-9
23. Marco, ML, Hutkins, R, Hill, C, Fulgoni, VL, Cifelli, CJ, Gahche, J, et al. A classification system for defining and estimating dietary intake of live microbes in US adults and children. J Nutr. (2022) 152:1729–36. doi: 10.1093/jn/nxac074
24. Kailembo, A, Quiñonez, C, Lopez Mitnik, GV, Weintraub, JA, Stewart Williams, J, Preet, R, et al. Income and wealth as correlates of socioeconomic disparity in dentist visits among adults aged 20 years and over in the United States, 2011-2014. BMC Oral Health. (2018) 18:147. doi: 10.1186/s12903-018-0613-4
25. Qiu, Z, Chen, X, Geng, T, Wan, Z, Lu, Q, Li, L, et al. Associations of serum carotenoids with risk of cardiovascular mortality among individuals with type 2 diabetes: results from NHANES. Diabetes Care. (2022) 45:1453–61. doi: 10.2337/dc21-2371
26. Araki, T, and Zhao, Y. Diet quality and its associated factors among adults with overweight and obesity: findings from the 2015–2018 National Health and Nutrition Examination Survey. Br J Nutr. (2024) 131:134–42. doi: 10.1017/S0007114523001587
27. Kim, CY, Sivasundaram, L, LaBelle, MW, Trivedi, NN, Liu, RW, and Gillespie, RJ. Predicting adverse events, length of stay, and discharge disposition following shoulder arthroplasty: a comparison of the Elixhauser Comorbidity Measure and Charlson Comorbidity Index. J Shoulder Elb Surg. (2018) 27:1748–55. doi: 10.1016/j.jse.2018.03.001
28. Marco, ML, Sanders, ME, Gänzle, M, Arrieta, MC, Cotter, PD, De Vuyst, L, et al. The International Scientific Association for Probiotics and Prebiotics (ISAPP) consensus statement on fermented foods. Nat Rev Gastroenterol Hepatol. (2021) 18:196–208. doi: 10.1038/s41575-020-00390-5
29. Berding, K, Bastiaanssen, TFS, Moloney, GM, Boscaini, S, Strain, CR, Anesi, A, et al. Feed your microbes to deal with stress: a psychobiotic diet impacts microbial stability and perceived stress in a healthy adult population. Mol Psychiatry. (2023) 28:601–10. doi: 10.1038/s41380-022-01817-y
30. Ağagündüz, D, Yılmaz, B, Şahin, T, Güneşliol, BE, Ayten, Ş, Russo, P, et al. Dairy lactic acid bacteria and their potential function in dietetics: the food-gut-health axis. Foods. (2021) 10:3099. doi: 10.3390/foods10123099
31. Bercik, P, Park, AJ, Sinclair, D, Khoshdel, A, Lu, J, Huang, X, et al. The anxiolytic effect of Bifidobacterium longum NCC3001 involves vagal pathways for gut-brain communication. Neurogastroenterol Motil. (2011) 23:1132–9. doi: 10.1111/j.1365-2982.2011.01796.x
32. Tian, P, Wang, G, Zhao, J, Zhang, H, and Chen, W. Bifidobacterium with the role of 5-hydroxytryptophan synthesis regulation alleviates the symptom of depression and related microbiota dysbiosis. J Nutr Biochem. (2019) 66:43–51. doi: 10.1016/j.jnutbio.2019.01.007
33. Lu, Y, Zhang, Z, Tong, L, Zhou, X, Liang, X, Yi, H, et al. Mechanisms underlying the promotion of 5-hydroxytryptamine secretion in enterochromaffin cells of constipation mice by Bifidobacterium and Lactobacillus. Neurogastroenterol Motil. (2021) 33:e14082. doi: 10.1111/nmo.14082
34. Kirby, LG, Zeeb, FD, and Winstanley, CA. Contributions of serotonin in addiction vulnerability. Neuropharmacology. (2011) 61:421–32. doi: 10.1016/j.neuropharm.2011.03.022
35. Cheng, Y, Liu, J, and Ling, Z. Short-chain fatty acids-producing probiotics: A novel source of psychobiotics. Crit Rev Food Sci Nutr. (2022) 62:7929–59. doi: 10.1080/10408398.2021.1920884
36. Kobayashi, Y, Sugahara, H, Shimada, K, Mitsuyama, E, Kuhara, T, Yasuoka, A, et al. Therapeutic potential of Bifidobacterium breve strain A1 for preventing cognitive impairment in Alzheimer's disease. Sci Rep. (2017) 7:13510. doi: 10.1038/s41598-017-13368-2
37. Silva, YP, Bernardi, A, and Frozza, RL. The role of short-chain fatty acids from gut microbiota in gut-brain communication. Front Endocrinol. (2020) 11:25. doi: 10.3389/fendo.2020.00025
38. Zheng, HJ, Guo, J, Wang, Q, Wang, L, Wang, Y, Zhang, F, et al. Probiotics, prebiotics, and synbiotics for the improvement of metabolic profiles in patients with chronic kidney disease: a systematic review and meta-analysis of randomized controlled trials. Crit Rev Food Sci Nutr. (2021) 61:577–98. doi: 10.1080/10408398.2020.1740645
39. Babic, M, Veljovic, K, Popović, N, Golic, N, Radojkovic, D, and Stankovic, M. Antioxidant effect of lactic acid bacteria in human bronchial epithelial cells exposed to cigarette smoke. J Appl Microbiol. (2023) 134:lxad257. doi: 10.1093/jambio/lxad257
40. Budden, KF, Gellatly, SL, Vaughan, A, Amorim, N, Horvat, JC, Hansbro, NG, et al. Probiotic Bifidobacterium longum subsp. longum protects against cigarette smoke-induced inflammation in mice. Int J Mol Sci. (2022) 24:252. doi: 10.3390/ijms24010252
41. Luoto, R, Ruuskanen, O, Waris, M, Kalliomäki, M, Salminen, S, and Isolauri, E. Prebiotic and probiotic supplementation prevents rhinovirus infections in preterm infants: a randomized, placebo-controlled trial. J Allergy Clin Immunol. (2014) 133:405–13. doi: 10.1016/j.jaci.2013.08.020
42. King, S, Glanville, J, Sanders, ME, Fitzgerald, A, and Varley, D. Effectiveness of probiotics on the duration of illness in healthy children and adults who develop common acute respiratory infectious conditions: a systematic review and meta-analysis. Br J Nutr. (2014) 112:41–54. doi: 10.1017/S0007114514000075
43. Gibson, GR, Probert, HM, Loo, JV, Rastall, RA, and Roberfroid, MB. Dietary modulation of the human colonic microbiota: updating the concept of prebiotics. Nutr Res Rev. (2004) 17:259–75. doi: 10.1079/NRR200479
44. Li, HY, Zhou, DD, Gan, RY, Huang, SY, Zhao, CN, Shang, A, et al. Effects and mechanisms of probiotics, prebiotics, synbiotics, and postbiotics on metabolic diseases targeting gut microbiota: a narrative review. Nutrients. (2021) 13:3211. doi: 10.3390/nu13093211
45. Al-Zyoud, W, Hajjo, R, Abu-Siniyeh, A, and Hajjaj, S. Salivary microbiome and cigarette smoking: a first of its kind investigation in Jordan. Int J Environ Res Public Health. (2019) 17:256. doi: 10.3390/ijerph17010256
46. Das, A, Srinivasan, M, Ghosh, TS, and Mande, SS. Xenobiotic Metabolism and Gut Microbiomes. PLoS One. (2016) 11:e0163099. doi: 10.1371/journal.pone.0163099
47. Seidel, C, Boehm, V, Vogelsang, H, Wagner, A, Persin, C, Glei, M, et al. Influence of prebiotics and antioxidants in bread on the immune system, antioxidative status and antioxidative capacity in male smokers and non-smokers. Br J Nutr. (2007) 97:349–56. doi: 10.1017/S0007114507328626
48. Benowitz, NL, Hukkanen, J, and Jacob, P 3rd. Nicotine chemistry, metabolism, kinetics and biomarkers. Handb Exp Pharmacol. (2009) 192:29–60. doi: 10.1007/978-3-540-69248-5_2
49. Lee, SH, Yun, Y, Kim, SJ, Lee, EJ, Chang, Y, Ryu, S, et al. Association between cigarette smoking status and composition of gut microbiota: population-based cross-sectional study. J Clin Med. (2018) 7:282. doi: 10.3390/jcm7090282
50. Sun, J, Jin, J, Beger, RD, Cerniglia, CE, Yang, M, and Chen, H. Metabolomics evaluation of the impact of smokeless tobacco exposure on the oral bacterium Capnocytophaga sputigena. Toxicol In Vitro. (2016) 36:133–41. doi: 10.1016/j.tiv.2016.07.020
51. Carvalho, JL, Miranda, M, Fialho, AK, Castro-Faria-Neto, H, Anatriello, E, Keller, AC, et al. Oral feeding with probiotic Lactobacillus rhamnosus attenuates cigarette smoke-induced COPD in C57Bl/6 mice: Relevance to inflammatory markers in human bronchial epithelial cells. PLoS One. (2020) 15:e0225560. doi: 10.1371/journal.pone.0225560
52. Lai, HC, Lin, TL, Chen, TW, Kuo, YL, Chang, CJ, Wu, TR, et al. Gut microbiota modulates COPD pathogenesis: role of anti-inflammatory Parabacteroides goldsteinii lipopolysaccharide. Gut. (2022) 71:309–21. doi: 10.1136/gutjnl-2020-322599
Keywords: dietary live microbes, probiotic/prebiotic intake, serum cotinine, NHANES, smoking
Citation: Lin S, Zhu N, Zhu Y, Mao H and Zhang S (2024) Exploratory analysis on the association of dietary live microbe and non-dietary prebiotic/probiotic intake with serum cotinine levels in the general adult population. Front. Nutr. 11:1405539. doi: 10.3389/fnut.2024.1405539
Edited by:
George Grant, Independent Researcher, Aberdeen, United KingdomReviewed by:
Valentina Echeverria Moran, United States Department of Veterans Affairs, United StatesJourdan Lakes, Oak Ridge Institute for Science and Education (ORISE), United States
Copyright © 2024 Lin, Zhu, Zhu, Mao and Zhang. This is an open-access article distributed under the terms of the Creative Commons Attribution License (CC BY). The use, distribution or reproduction in other forums is permitted, provided the original author(s) and the copyright owner(s) are credited and that the original publication in this journal is cited, in accordance with accepted academic practice. No use, distribution or reproduction is permitted which does not comply with these terms.
*Correspondence: Shengmin Zhang, nbsmzhang@163.com
†These authors have contributed equally to this work and share first authorship