Feasibility of the application of frequency modulated continuous wave radar trigger technique in abdominal magnetic resonance imaging
- 1Department of Radiology, The First Affiliated Hospital of Dalian Medical University, Dalian, China
- 2Shanghai United Imaging Healthcare, Shanghai, China
Objective: To evaluate and compare the image quality of T2-weighted abdominal scans using a respiratory belt trigger (RBT) and frequency-modulated continuous wave (FMCW)-trigger (FT) techniques and to explore the feasibility of FT in abdominal magnetic resonance imaging (MRI).
Methods: The study prospectively included 28 subjects, each undergoing abdominal scans with both RBT and FT. The analysis focused on 64 inconsistent trigger segments from the respiratory curves triggered by RBT and FMCW. Parameters such as inconsistent trigger type (ITT), number of inconsistent trigger points (ITPs), ratio of ITP (ITR), and single-segment ITR (SITR) were derived from these curves. Image quality was evaluated by two observers using subjective scoring, signal-to-noise ratio (SNR), and contrast-to-noise ratio (CNR). The assessments classified image quality as either “good” or “poor.” Consistency in image quality assessment between observers was determined using the kappa test and intraclass correlation coefficient (ICC). The chi-square test, Student’s t-test, and the Mann–Whitney U test were employed to compare the categorical and continuous variables between the RBT and FT groups.
Results: The observers showed a high level of agreement in image quality assessment. There were no significant differences in ITR, SITR, acquisition time, SNR, and CNR between the RBT and FT groups (all p > 0.05). Both subjective and objective evaluations indicated no notable difference in image quality between the two groups (p > 0.05).
Conclusion: The FMCW trigger technique is a viable alternative to the traditional respiratory belt trigger in scenarios of relatively stable breathing. It offers potential benefits, such as reducing operational demands on technicians and improving MRI workflow efficiency.
1 Introduction
Magnetic resonance imaging (MRI) is increasingly used in abdominal examinations and disease diagnosis due to its excellent soft tissue resolution and multi-planar imaging capabilities. The T2-weighted fast spin echo (T2-weighted-FSE) sequence is particularly valued in abdominal MRI for its effective fat suppression and superior contrast in non-adipose soft tissues [1].
However, respiratory motion remains a significant challenge in upper abdominal MRI, causing artifacts such as increased noise, decreased spatial resolution, and ghosting. These artifacts can hinder the accurate observation of organ structures and the diagnosis of lesions [2–4]. Techniques like breath-holding scans and respiratory gating are commonly used to mitigate these issues. Breath-holding is limited to patients with sufficient respiratory control and often results in lower signal-to-noise ratios (SNRs) [5]. Although multiple breath-holds can enhance SNRs, patients struggle with maintaining consistent diaphragm positioning, leading to potential misalignment of abdominal organs [5, 6]. Respiratory navigation gating identifies the diaphragm position using a fast sequence and allows continuous acquisition; however, while offering improved image quality, it extends acquisition times [7]. Free-breathing methods have shown some improvements in image quality but often extend the total scan duration. Despite advancements like deep learning applications for liver evaluation in free-breathing scans, satisfying results in addressing motion-related challenges remain elusive [8]. Other motion-robust techniques, such as the SENSE MultiVane, are under investigation but require further validation in the context of upper abdominal MRI [9].
The respiratory belt trigger (RBT) technique is a widely adopted clinical practice to collect continuous signals, employing a physiological sensor to monitor and control motion. This technique tracks respiratory movement through a belt that compresses and releases gas with each breath, triggering signal acquisition accordingly [10]. While RBT is advantageous in its non-interference with imaging and independence from field strength, it faces challenges regarding the accuracy of triggering, patient comfort, and operational burden, particularly in patients with abdominal trauma or surgery. To simplify the workflow, improve efficiency, and expand more clinical applications, the feasibility of noncontact physiological motion monitoring was explored.
Recent studies have explored electromagnetic methods for contactless physiological motion sensing. Techniques like the Pilot Tone in the RF receive chain of MRI systems, although effective in tracking phase errors, can introduce image artifacts via the RF coils [11–13]. Navigators and self-navigation during scans have been proposed to improve efficiency and image quality [14, 15]. Additionally, respiratory monitoring technologies, including ultrasonic and radar monitoring, have shown promise in detecting human respiratory rates [16, 17].
Radar technology has revolutionized the remote detection of vital signs, including heart rate and respiration, by capturing physiological signals through microwave emissions [18]. Radar systems are broadly classified into continuous wave radar and pulse wave radar, each employing distinct waveform types [19, 20]. The frequency-modulated continuous-wave (FMCW) radar, in particular, excels in measuring motion-related object information through phase unwrapping. Its widespread applications span transportation safety, communication, and healthcare [21–24]. FMCW radar is renowned for its high accuracy in distance measurement, attributed to its short wavelength. This feature renders it an invaluable tool for vital sign monitoring [25, 26]. Recently, FMCW radar has emerged as a promising technique for respiratory motion monitoring, showing immense potential in enhancing medical diagnosis and evaluation [27, 28]. The integration of FMCW radar technology into MRI enables a noncontact method for monitoring respiratory movement. This approach promises a simplified and precise respiratory triggering mechanism during MR scans, potentially revolutionizing the field [29, 30].
This study aims to evaluate and compare the image quality of T2-weighted abdominal scans obtained through the RBT technique and the novel frequency-modulated continuous wave-trigger (FT) technique. It seeks to explore the clinical utility of FT in abdominal magnetic resonance imaging, potentially offering a groundbreaking advancement in MRI technology.
2 Materials and methods
2.1 Clinical data
The study involved abdominal subjects who underwent MRI scans using both RBT and FT techniques from February to March 2022. The exclusion criteria were as follows: 1) Subjects whose respiratory curves during the scan displayed instability in both RBT and FT patterns, characterized by abnormal waveforms with obviously irregular widths and heights that deviated from typical respiratory patterns (refer to Supplementary Figure S1 for examples). 2) Subjects with completely consistent triggering in both RBT and FT, indicated by all points in the RBT and FT respiratory curves being triggered simultaneously and the positions of all triggered points in both curves being identical. Additionally, the study received approval from the institutional review board.
2.2 Theory
2.2.1 Principle of FMCW radar
FMCW radar operates by generating a transmitted signal, termed “chirp signals,” through frequency modulation. When this transmitted signal encounters an object, it reflects back and is received. The reflected signal is then mixed with the original signal to produce a beat signal, represented mathematically as follows:
where the
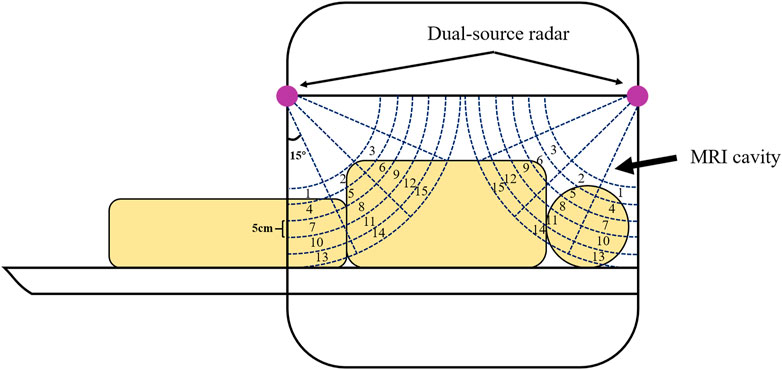
Figure 1. The dual-source FMCW radar is located at both ends of the MRI bore to detect almost all of the bore space. The detection region of each radar is divided into 15 bins with a range resolution of approximately 5 cm and an angle resolution of approximately 15° to cover from the thorax to the pelvis of the patient’s body.
2.2.2 Processing and analysis of FMCW radar
In the FMCW radar system, the abdomen field of view (FOV) is segmented into 15 distinct parts, extending from the thorax to the pelvis. The reflected signals from these regions are collected by the receivers, as illustrated in Figure 1. To minimize interference with MRI signals, the FMCW radar is strategically positioned at the end of the MRI scanner. Despite this positioning, there may be weak signal reception from the subject’s abdomen, necessitating enhanced signal processing.
Two key procedures are employed to enhance the quality of the reconstructed breathing signals. First, multichannel signal processing involves using range-fast Fourier transform (FFT) and angle-FFT techniques to mitigate mutual interference among different range signals. The respiratory movement is discerned by analyzing phase differences in the transmission and reception processes. The optimal channel for respiratory detection is identified as the one least affected by interference.
Second, abdomen breathing signals are refined by combining filtering with a first-order difference method. This approach is designed to minimize noise interference from the static environment. The final waveform is compiled by accumulating displacement increments in the detection areas, calculated between two consecutive measurements in the optimal channel. A triggering algorithm is developed to determine the respiratory motion’s trigger point during scanning. This trigger point is set when the respiratory amplitude decreases by 60% from the last peak, initiating the MR scan after a brief time delay. The extraction algorithm for respiration curves is efficient, taking approximately 9 ms, which is negligible compared to the duration of a respiration cycle.
The radar system divides the MRI bore into 15 bins based on range resolution (approximately 5 cm) and angle resolution (around 15°). Fourier transform analysis is performed on the signals from each bin to determine their frequency and amplitude. The bin with frequency and amplitude most closely resembling normal human respiratory patterns is identified as the “abdominal bin.” The respiratory curve from this bin is then output in real-time via an automatic algorithm, as depicted in Figure 2.
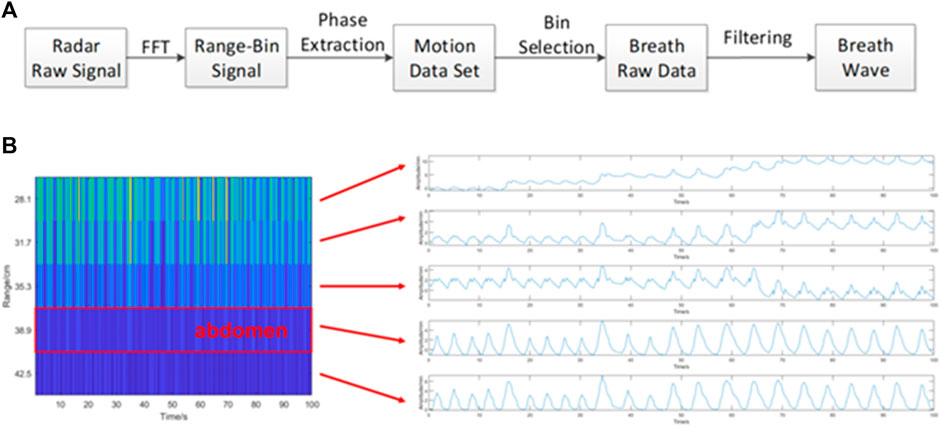
Figure 2. Flow chart of the FT technology detection algorithm. (A) Signal processing flow. (B) The waveforms of the signals extracted from several regions are displayed. The fourth waveform shows that the amplitudes and widths of the waveforms are the closest to each other, indicating that the signal in this area is the most stable and reflects the most normal breathing state, so the fourth bin is chosen as the best abdominal breath detection area because it has the least signal interference.
To evaluate the FMCW radar’s performance compared to the respiratory belt, patients were simultaneously equipped with a respiratory belt during scans. The belt’s pressure sensor converted the pressure variations caused by thoracic and abdominal movements during breathing into electrical signals. These signals were then used to form the RBT respiration curves, facilitating a comparative analysis between the FMCW and RBT methods.
2.3 MR imaging protocol
The MR imaging was conducted using a clinical 3.0-T MR scanner (uMR Omega; United Imaging Healthcare, Shanghai, China) equipped with a dedicated 24-channel body array coil. To facilitate comprehensive monitoring of the subjects’ respiratory movements, FMCW dual-source radars were integrated into the MRI scanner, covering the entire bore. Prior to the MR scan, subjects were required to fast for at least 6 h. They also received training to maintain steady breathing during the scan. The respiratory belt was strategically placed at the center of the abdomen, where it could capture the maximum breathing amplitude while avoiding the bilateral ribs.
The upper abdomen scanning protocol was as follows: Both groups underwent the same imaging sequence, specifically T2-FSE, spanning from the porta hepatis to the renal hilum. The scanning parameters were TR/TE = 3602/94.68 ms, number of average = 1, FOV = 380 mm2 × 380 mm2, matrix = 320 × 320, and slice thickness/gap = 6.0/1.2 mm. This standardized protocol ensured consistency in imaging conditions across all subjects, enabling a reliable comparison between the RBT and FMCW-triggered images.
2.4 Image analysis
2.4.1 Post-processing of the respiratory curve and corresponding images
Post-scanning, T2-FSE images were obtained using both RBT and FT methods. These images were acquired through multiple segmented acquisitions (refer to Supplementary Figure S2 for details). The imaging data included the number of segmented images and the acquisition time for each segment. The RBT1 respiratory curve was generated concurrently with the scanning process using the RBT technique, representing the actual respiratory pattern during RBT method imaging. In contrast, the FT2 curve, not involved in triggering image acquisition, served as a non-trigger control group. Similar conditions applied to the FT1 and RBT2 curves in comparison to the RBT1 and FT2.
The respiratory curves (RBT1+FT2 or RBT2+FT1) and trigger points for both RBT and FT were extracted and visualized using workstations. These curves displayed the total number of inconsistent trigger points (ITPs) and the total ratio of ITPs (ITRs) in percentage terms for both RBT and FT, as illustrated in Figures 3A–J. During the scan for each subject, respiratory curves for RBT and FT were produced, with all trigger points distributed along these curves. The positioning of these points was largely consistent between RBT and FT, resulting in many RBT and FT trigger points at the same places on the curve. However, some trigger positions featuring only one trigger point (either RBT or FT) were identified as “inconsistent trigger points.” For analysis purposes, the respiratory curve was evenly divided according to the number of image segments. The number of ITPs in each segment and their respective ratio to the total ITPs were calculated, thus determining the single-segment ITR (SITR).
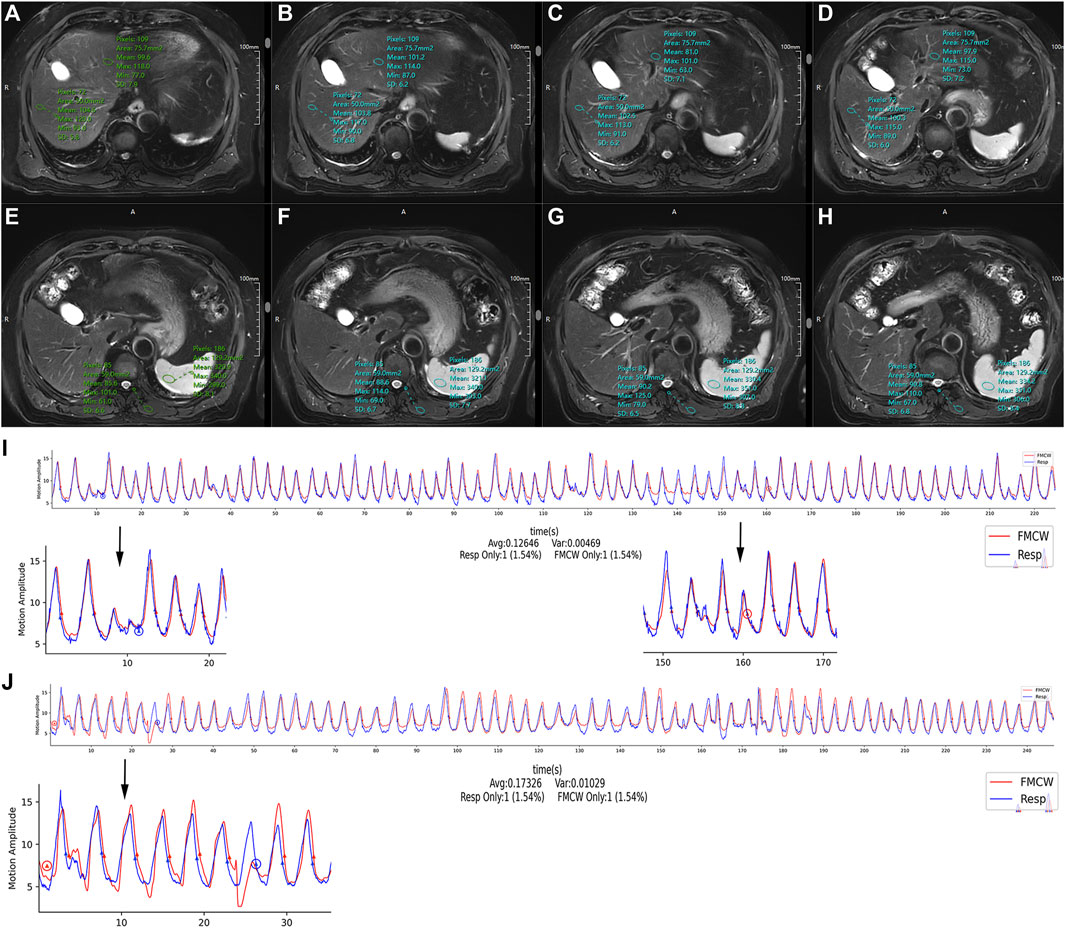
Figure 3. (A–I) A female subject, 59 years old, showing (A–H) axial T2-weighted image with FT, which was obtained by multiple segmented acquisitions (four segments), (A–D)/(E–H) corresponding to the first to fourth segments, respectively, the ROIs were manually placed on the left/right hepatic lobe and spleen. (I) The respiratory curve is simultaneously output when scanning (A–D); the x-axis represents the acquisition time, and the y-axis represents motion amplitude; the blue circles were only triggered with the belt trigger (B-type), and the red circles were only triggered by the FMCW trigger (F-type). “Resp Only” and “FMCW Only” are the total number and ratio of inconsistent trigger points for RBT and FT. The curve is divided into four segments according to the (A–D). There is a B-type point in the first segment and an F-type point in the third segment. (J) Respiratory curve of another female subject, 68 years old. The respiratory curve of the T2-weighted image-FT is divided into four segments, and there is both a B-type and an F-type point in the first segment.
In a given segment, the inconsistent trigger type (ITT) between RBT and FT groups could fall into three categories: ① B-type, where at least one trigger point appeared in RBT but not in FT; ② F-type, where at least one trigger point appeared in FT but not in RBT; and ③ B+F-type, encompassing both aforementioned situations (as shown in Figure 3J). This study focused only on analyzing B-type and F-type points. An observer identified segments with ITP on the respiratory curves in both the RBT and FT groups, along with their corresponding RBT and FT images. For each subject, the number of RBT and FT segments with ITPs, their SITRs, and the acquisition times of the MR images for these segments were recorded.
2.4.2 Image quality analysis
Two experienced observers, with 5 and 11 years in image diagnostics, independently assessed the quality of the selected images. These evaluations were blind with regard to the imaging method used (RBT or FT).
2.4.2.1 Subjective evaluation
The images were rated using a Likert scale. Observations were made of various organs—the liver, spleen, kidney, pancreas, and gastrointestinal tract, focusing on signal, internal structure/blood vessel visibility, and organ outline. The specific evaluation criteria and scoring system are detailed in Table 1. An image was deemed good quality if both observers rated the three indicators (overall quality, blurring, and hepatic vessel clarity) with scores of 3–4 (examples shown in Figures 4A, B). Conversely, an image was classified as poor quality if either observer assigned a score of 1–2 (refer to Figures 4C, D for examples). The numbers of images categorized as good or poor in both the RBT and FT groups were recorded.
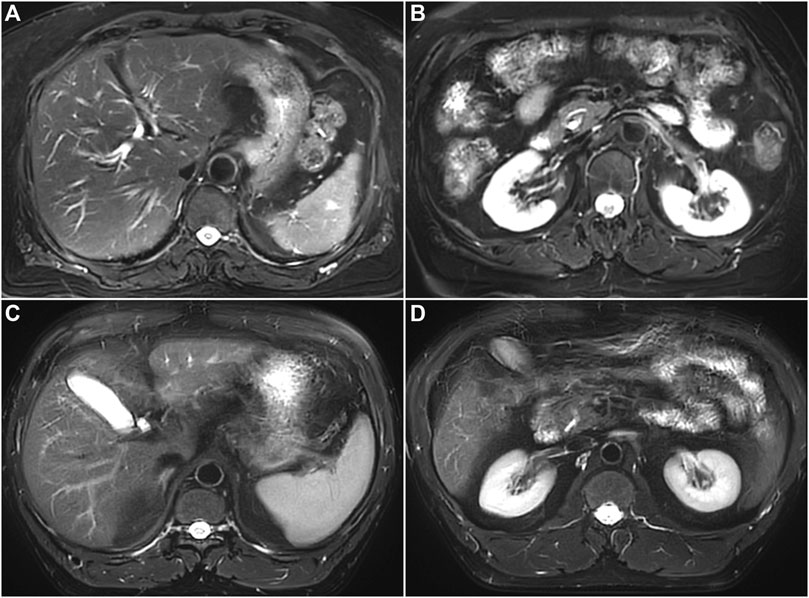
Figure 4. (A,B) A female subject, 61 years old, showing different layers of the same segment of the RBT scanned image. The two observers both assigned scores of 3 or higher for the image, and the image quality is classified as good. (C,D) A male subject, 49 years old, showing different layers of the same segment of the RBT scanned image. Because one of the observers assigned scores of 1 or 2 to the image, the image quality is classified as poor.
2.4.2.2 Objective evaluation
The two observers independently measured the signal intensity (SI) of the images. SNR and CNR were calculated from these measurements. For consistency, regions near the hepatic hilar area were chosen for region of interest (ROI) delineation, as illustrated in Figures 3A–H. The ROIs were uniformly placed and duplicated in the left hepatic lobe, the right hepatic lobe, the spleen, and the right erector spinae muscle, ensuring identical ROI sizes.
Elliptical ROIs were manually drawn in the margins of the left lateral lobe, the right posterior lobe of the liver, and the posterior medial part of the spleen, positioned at least 5 mm away from the hepatic and splenic capsules. The ROI areas ranged from approximately 50 mm2–150 mm2. For the erector spinae muscle, ROIs were drawn along the muscle space direction, selecting uniform signal areas and avoiding vessels in the liver, spleen, and back muscles. Figures 3A–H provide examples of ROI placement. The SIs for the liver, spleen, and erector spinae were recorded, using the SI of the erector spinae as the background SI. SNRs and CNRs for the left and right hepatic lobes and spleen were documented by the observers.
The formulas for SNR and CNR (taking the left hepatic lobe as an example) are as follows:
Here, SD refers to the standard deviation. The SD of the background SI in the images was used as the noise value [31].
2.5 Statistical analysis
The kappa test was used to evaluate the consistency of subjective image quality scores. The kappa values were interpreted as follows: 0–0.20 indicates extremely low consistency, 0.21–0.40 suggests average consistency, 0.41–0.60 reflects moderate consistency, 0.61–0.80 signifies high consistency, and 0.81–1.00 denotes almost complete consistency. The intraclass correlation coefficient (ICC) was used to assess the consistency of SNR and contrast-to-noise ratio (CNR) measurements. ICC values are classified as ≤0.40 for fair consistency, 0.41–0.60 for moderate consistency, 0.61–0.80 for good consistency, and 0.81–1.00 for excellent consistency. For SNR and CNR values demonstrating good to excellent consistency, the average measurements of the two observers were utilized for subsequent analysis. The chi-squared test was applied to analyze the differences in image quality (categorized as good or poor) and ITT between the RBT and FT groups. The Shapiro–Wilk test was used to assess the normal distribution of SNR, CNR, single-segment ITR (SITR), and acquisition time for RBT and FT images. Differences between the RBT and FT groups in these indices were compared using either Student’s t-test or the Mann–Whitney U test, depending on the normality of the data. A p-value of less than 0.05 was considered indicative of statistical significance. All statistical analyses were conducted using SPSS software (version 21, IBM, NY).
3 Results
3.1 Subject characteristics
Three subjects in the initial cohort were excluded due to unstable respiratory curves. This study primarily focused on the differences in image quality between the RBT and FT. Additionally, five subjects were excluded as their respiratory triggers were entirely consistent across both RBT and FT methods. Consequently, the study included 28 subjects, comprising 15 men and 13 women. The age range of the participants was broad, spanning from 24 to 80 years, with an average age of 49.9 ± 15.9 years.
3.2 Image information
The collected images and respiratory curves were categorized into two groups based on the triggering technique used during the scan: the RBT and FT groups. The total number of image segments scanned in both groups was 196, with 101 segments in the RBT group and 95 in the FT group. In total, 63 inconsistent trigger segments were identified for analysis, comprising 40 in the RBT group and 23 in the FT group.
A comparative analysis revealed no significant difference in the number of ITTs between the RBT and FT groups (p = 0.181). Similarly, the acquisition time for ITT segments did not significantly differ between the two groups (172.09 ± 31.70 s for RBT vs. 171.65 ± 28.05 s for FT; p = 0.956), as detailed in Table 2. Furthermore, there was no notable difference in the single-segment ITR (SITR) for both B-type and F-type triggers between the RBT and FT groups (both p > 0.05), as shown in Table 2.
3.3 Subjective evaluation of image quality
3.3.1 Consistency analysis of subjective image quality evaluation
The subjective scoring of images by the two observers demonstrated moderate consistency for both RBT and FT images, with kappa values between 0.6 and 0.8, as shown in Supplementary Table S1.
3.3.2 Difference of subjective evaluation of image quality between RBT and FT groups
The images were categorized based on the subjective evaluations by the two observers. The comparison revealed no significant difference in the quality of images between the RBT and FT groups (p > 0.05), as indicated in Table 3.
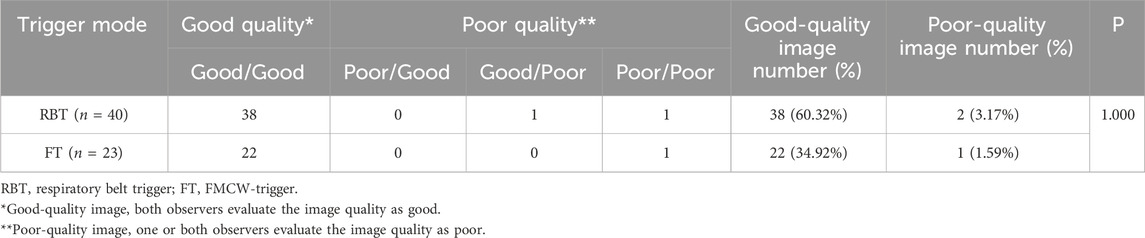
Table 3. Differences in subjective evaluation of image quality between RBT and FT groups by two observers.
3.4 Objective evaluation of image quality
3.4.1 Consistency analysis of image SNR and CNR
The SNR and CNR measurements for the left hepatic lobe, right hepatic lobe, and spleen in both the RBT and FT groups showed good agreement, with all ICCs exceeding 0.8. These results are detailed in Supplementary Table S2.
3.4.2 Difference in SNR and CNR between RBT and FT image
No significant differences were observed in the SNR and CNR values for the left hepatic lobe (p = 1.00 and 0.467), right hepatic lobe (p = 0.842 and 0.668), and spleen (p = 0.990, and 0.898) between the RBT and FT groups, as depicted in Figure 5.
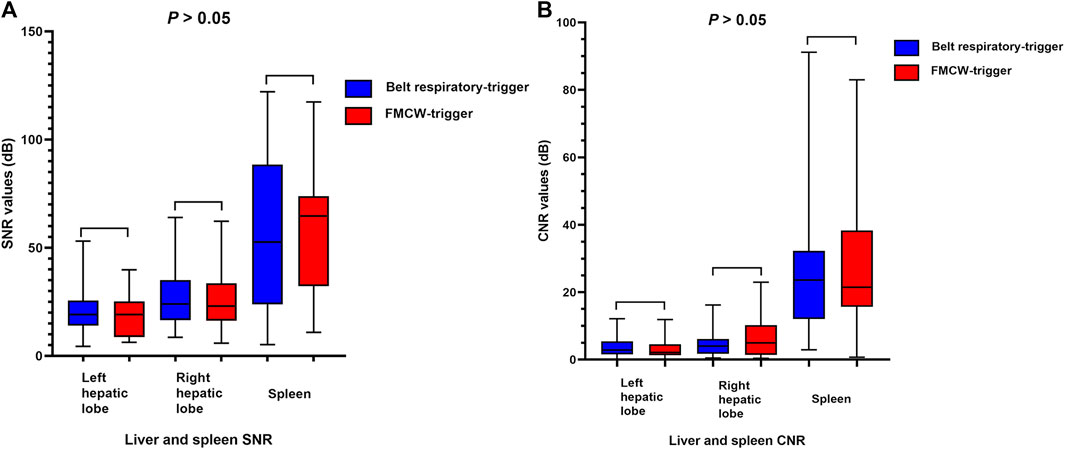
Figure 5. (A–B) Boxplot of image SNR and CNR between the RBT and FT groups. (A) There was no significant difference between the SNRs of the left hepatic lobe, the right hepatic lobe, and the spleen for the T2-weighted image-RBT and T2-weighted image-FT groups, and (B) there was no significant difference between the CNRs of the left hepatic lobe, the right hepatic lobe, and the spleen for the T2-weighted image-RBT and T2-weighted image-FT groups. RBT, respiratory belt trigger; FT, FMCW trigger.
3.5 Correlation between the image quality and inconsistent trigger type
The study also examined the relationship between image quality and the type of ITT, specifically B-type and F-type ITT segments. The analysis showed no significant difference in the number of B-type and F-type ITT segments between images classified as good or poor quality in the RBT and FT groups (all p > 0.05), as presented in Table 4.
4 Discussion
This study aimed to evaluate the effectiveness of FMCW trigger technology compared to the conventional RBT in abdominal MRI scans. Our findings indicate that there is no significant difference in image quality, acquisition time, or inconsistent trigger status, including ITT and SITR, between the FT and RBT groups. This suggests that FMCW trigger scans are approximately as effective as respiratory belt trigger scans.
Subjective evaluations revealed that both the FT and RBT groups produced predominantly good-quality images, with no notable difference in the number of high-quality images between the two groups. Similar SNR and CNR values were observed, as well as comparable acquisition times for images acquired by both techniques. Pioneering work by Wang H et al. on applying the FMCW trigger technique to MRI scans found similar results, with no significant difference in image quality between this technique and the respiratory belt trigger. The synchronous output respiratory curve analysis showed that the respiratory waveform of FT was generally consistent with that triggered by the traditional respiratory belt [30]. Liang X et al., in their comparison of a respiratory belt trigger, diaphragm navigation, and an FMCW trigger in T2-weighted and DWI scans, also found no significant difference in image quality among these respiratory trigger techniques [29]. This study supports these findings, suggesting that both the RBT and FT methods can effectively control the impact of respiratory movement on image quality, yielding clinically satisfactory images in subjects with steady breathing.
However, limitations exist in previous research. Wang’s study, which investigated the relationship between image quality and trigger mode, was limited to only one subject. Similarly, Liang X et al. compared the overall image quality between the FT, RBT, and diaphragm navigation techniques but did not delve into the relationship between the quality of different image segments and the corresponding trigger status of the respiratory curve. Our study, in contrast, conducted a more nuanced analysis by comparing the respiratory curves of FT1+RBT2 and RBT1+FT2, thereby evaluating the performance of both the RBT and FT in relation to image quality. This approach enabled a preliminary investigation into how inconsistencies in triggering between an FT and RBT affect image quality, considering the characteristics of segmented and interval image acquisition. This detailed analysis offers a more comprehensive understanding of the potential implications and effectiveness of these triggering methods in clinical MRI applications.
The study discovered the presence of both B-type and F-type ITT within the FMCW FT and RBT groups. Interestingly, good-quality images were predominantly observed in both groups, irrespective of the trigger type. The B-type ITT was typically linked to alterations in the respiratory pattern. The FMCW, being sensitive to abnormal breathing and changes in respiratory regions, occasionally missed triggers if the respiratory amplitude did not reach its threshold. Conversely, the F-type ITT likely occurred due to shallow respiratory amplitudes or patient positional changes during the scan that the respiratory belt sensor failed to detect. Nevertheless, the FT was still capable of detecting such breathing patterns and continued to trigger.
In the RBT group, some segments demonstrated good image quality despite the presence of B-type ITT. This could be attributed to the FT’s high sensitivity to breathing cycle variations that did not immediately impact image quality. For segments with good image quality that showed an F-type ITT, the cause might have been the respiratory belt sensor’s failure to trigger due to subtle respiratory cycle changes that did not compromise image acquisition quality. In these cases, the RBT did not activate, whereas the FT did so accurately.
In the FT group, segments with good image quality and F-type ITT indicated that the respiratory belt sensor missed some regular trigger points, while the FT assessed more accurately. Good-quality segments with B-type ITT suggested that the FT could detect changes in the respiratory cycle that potentially affected image quality.
The scan time did not significantly differ between the two groups, implying that missed triggers in either technique did not substantially prolong image acquisition time. A few segments in the RBT group with poor image quality and B-type ITT might be due to partial respiratory cycle changes impacting image quality, where the FT detected abnormal breathing and refrained from triggering, but the RBT continued passively. Similarly, in the FT group, some segments exhibited poor image quality with B-type ITT, possibly due to abrupt changes in respiratory cycles during the scan. Managing the effects of respiratory movements proved challenging with both trigger techniques.
No significant difference was observed in the number of B-type and F-type segments and their corresponding SITRs between the RBT and FT groups. This finding suggests that the FMCW trigger’s judgment was consistent with that of the respiratory belt. Moreover, there was no significant disparity in image quality between the two ITT groups when patients maintained normal breathing patterns.
The “pre-scan” interval, defined as the duration from a patient’s entry into the scanning room to the commencement of the scan [32], significantly influences the overall workflow. Prior research indicates that non-imaging acquisition activities, predominantly involving pre-scanning, constitute about 30% of total workflow time [32]. Therefore, optimizing “pre-scan” activities, such as respiratory gating, is crucial for streamlining MRI procedures. In our study, although RBT demonstrated inconsistencies with FMCW FT in terms of trigger omissions, these discrepancies did not markedly affect image quality. The ITRs for both B-type and F-type triggers were comparable, and the overall acquisition time remained unaffected by any omissions in FMCW triggering. Additionally, the capability of FMCW to correct abnormal breathing patterns in real-time via the respiratory curve further enhances its utility. As a result, the FMCW radar, being a noncontact respiratory trigger technique, shows significant promise in reducing non-scan time, enhancing MRI scan efficiency, and simplifying the workflow process. This aspect of FMCW radar technology could play a pivotal role in improving patient throughput and operational efficiency in clinical MRI settings.
The study, while providing valuable insights, is not without its limitations. First, it was conducted at a single center and involved a relatively small number of subjects. To mitigate the impact of this small cohort size, we employed a strategy of segmenting the images and respiratory curves, thereby generating a larger pool of data for analysis. Despite this approach, including more cases in a larger cohort is essential to thoroughly assess the feasibility of these techniques. Second, the study was restricted to subjects exhibiting relatively steady breathing. Future research should broaden the scope to include subjects with respiratory instability, allowing for a more comprehensive evaluation of the clinical applicability of these techniques in varied patient populations. Finally, this investigation was limited to evaluating the FMCW trigger technique in T2-FSE sequences. Consequently, there is a need for additional studies to explore and confirm the effectiveness of this technology across different MRI sequences, which would provide a more complete understanding of its utility in diverse clinical scenarios.
5 Conclusion
In conclusion, our study demonstrates that the image quality of T2-FSE sequences obtained using the FMCW trigger technique is comparable to those acquired through the conventional respiratory belt trigger. Moreover, when subjects maintained relatively steady breathing, the acquisition times between these two triggering methods were similar. The FMCW trigger technique proved effective in reducing respiratory motion artifacts, thereby maintaining image quality. Consequently, the FMCW trigger can serve as a viable supplementary method to the traditional respiratory belt trigger, offering significant benefits in reducing the operational burden on scan technicians and streamlining the MRI workflow. However, the utility of the FMCW trigger technique in patients with unsteady respiration remains an area for further investigation. Future studies are needed to explore the effectiveness and applicability of this technique in a broader clinical context, including its potential benefits in managing more challenging respiratory patterns.
Data availability statement
The raw data supporting the conclusion of this article will be made available by the authors, without undue reservation.
Ethics statement
The studies involving humans were approved by the Ethics Committee of the First Affiliated Hospital of Dalian Medical University. The studies were conducted in accordance with the local legislation and institutional requirements. The ethics committee/institutional review board waived the requirement of written informed consent for participation from the participants or the participants’ legal guardians/next of kin.
Author contributions
QlS: formal analysis, investigation, methodology, visualization, and writing–original draft. YH: investigation, methodology, software, and writing–original draft. LC: investigation, methodology, and writing–original draft. XX: methodology and writing–original draft. NW: investigation, methodology, and writing–original draft. QwS: data curation and writing–original draft. AL: conceptualization, supervision, and writing–review and editing.
Funding
The authors declare that no financial support was received for the research, authorship, and/or publication of this article.
Conflict of interest
Authors YH and XX were employed by the Shanghai United Imaging Healthcare company.
The remaining authors declare that the research was conducted in the absence of any commercial or financial relationships that could be construed as a potential conflict of interest.
Publisher’s note
All claims expressed in this article are solely those of the authors and do not necessarily represent those of their affiliated organizations, or those of the publisher, the editors, and the reviewers. Any product that may be evaluated in this article, or claim that may be made by its manufacturer, is not guaranteed or endorsed by the publisher.
Supplementary material
The Supplementary Material for this article can be found online at: https://www.frontiersin.org/articles/10.3389/fphy.2024.1319678/full#supplementary-material
Abbreviations
RBT, Respiratory belt trigger; FMCW, frequency-modulated continuous wave; FT, frequency-modulated continuous wave-trigger; ITT, inconsistent trigger type; ITR, ratio of the inconsistent trigger type; ITP, inconsistent trigger point; SITR, single-segment inconsistent trigger type; SNR, signal-to-noise ratio; CNR, contrast-to-noise ratio; ICC, intraclass correlation coefficient; RF, radio frequency.
References
1. Kargar S, Borisch EA, Froemming AT, Grimm RC, Kawashima A, King BF, et al. Modified acquisition strategy for reduced motion artifact in super resolution T2 FSE multislice MRI: application to prostate. Magn eason Med (2020) 84(5):2537–50. doi:10.1002/mrm.28315
2. Kang KA, Kim YK, Kim E, Jeong WK, Choi D, Lee WJ, et al. T2-Weighted liver MRI using the MultiVane technique at 3T: comparison with conventional T2-weighted MRI. Korean J Radiol (2015) 16(5):1038–46. doi:10.3348/kjr.2015.16.5.1038
3. Li H, Hu C, Yang Y, Zhao Y, Lin C, Li Z, et al. Single-breath-hold T2WI MRI with artificial intelligence-assisted technique in liver imaging: as compared with conventional respiratory-triggered T2WI. Magn eason Imaging (2022) 93:175–80. doi:10.1016/j.mri.2022.08.012
4. Lewis CE, Prato FS, Drost DJ, Nicholson RL. Comparison of respiratory triggering and gating techniques for the removal of respiratory artifacts in MR imaging. Radiology (1986) 160(3):803–10. doi:10.1148/radiology.160.3.3737921
5. Feinberg DA, Rofsky NM, Johnson G. Multiple breath-hold averaging (MBA) method for increased SNR in abdominal MRI. Magn eason Med (1995) 34(6):905–9. doi:10.1002/mrm.1910340617
6. Murphy IG, Graves MJ, Reid S, Patterson AJ, Patterson I, Priest AN, et al. Comparison of breath-hold, respiratory navigated and free-breathing MR elastography of the liver. Magn eason Imaging (2017) 37:46–50. doi:10.1016/j.mri.2016.10.011
7. Morimoto D, Hyodo T, Kamata K, Kadoba T, Itoh M, Fukushima H, et al. Navigator-triggered and breath-hold 3D MRCP using compressed sensing: image quality and method selection factor assessment. Abdom Radiol (New York) (2020) 45(10):3081–91. doi:10.1007/s00261-020-02403-y
8. Kim DH, Kim B, Lee HS, Benkert T, Kim H, Choi JI, et al. Deep learning-accelerated liver diffusion-weighted imaging: intraindividual comparison and additional phantom study of free-breathing and respiratory-triggering acquisitions. Invest Radiol (2023) 58(11):782–90. doi:10.1097/rli.0000000000000988
9. Niitsu M, Saruya S, Sakaguchi K, Watarai K, Yoneyama M, Katsumata Y, et al. Motion-robust MR imaging of the shoulder using compressed SENSE MultiVane. Eur J Radiol open (2022) 9:100450. doi:10.1016/j.ejro.2022.100450
10. Ehman RL, McNamara MT, Pallack M, Hricak H, Higgins CB. Magnetic resonance imaging with respiratory gating: techniques and advantages. AJR Am J roentgenology (1984) 143(6):1175–82. doi:10.2214/ajr.143.6.1175
11. Vahle T, Bacher M, Rigie D, Fenchel M, Speier P, Bollenbeck J, et al. Respiratory motion detection and correction for MR using the pilot tone: applications for MR and simultaneous PET/MR examinations. Invest Radiol (2020) 55(3):153–9. doi:10.1097/rli.0000000000000619
12. Solomon E, Rigie DS, Vahle T, Paška J, Bollenbeck J, Sodickson DK, et al. Free-breathing radial imaging using a pilot-tone radiofrequency transmitter for detection of respiratory motion. Magn eason Med (2021) 85(5):2672–85. doi:10.1002/mrm.28616
13. Kwok WE. Basic principles of and practical guide to clinical MRI radiofrequency coils. Radiographics: a Rev Publ Radiological Soc North America, Inc (2022) 42(3):898–918. doi:10.1148/rg.210110
14. Rutz T, Piccini D, Coppo S, Chaptinel J, Ginami G, Vincenti G, et al. Improved border sharpness of post-infarct scar by a novel self-navigated free-breathing high-resolution 3D whole-heart inversion recovery magnetic resonance approach. Int J Cardiovasc Imaging (2016) 32(12):1735–44. doi:10.1007/s10554-016-0963-4
15. Dong Y, Riedel M, Koolstra K, van Osch MJP, Börnert P. Water/fat separation for self-navigated diffusion-weighted multishot echo-planar imaging. NMR Biomed (2023) 36(1):e4822. doi:10.1002/nbm.4822
16. Arlotto P, Grimaldi M, Naeck R, Ginoux JM. An ultrasonic contactless sensor for breathing monitoring. Sensors (Basel, Switzerland) (2014) 14(8):15371–86. doi:10.3390/s140815371
17. Yang F, He Z, Fu Y, Li L, Jiang K, Xie F. Noncontact detection of respiration rate based on forward scatter radar. Sensors (Basel, Switzerland) (2019) 19(21):4778. doi:10.3390/s19214778
18. Li C, Lubecke VM, Boric-Lubecke O, Lin J. JIToMT, techniques: a review on recent advances in Doppler radar sensors for noncontact healthcare monitoring. IEEE Trans Microwave Theor Tech (2013) 61(5):2046–60. doi:10.1109/TMTT.2013.2256924
19. Zhengyu P, José MM-F, Yao T, Chenhui L. Theory RJIToM et al: A Portable FMCW Interferometry Radar With Programmable Low-IF Architecture for Localization. ISAR Imaging, and Vital Sign Tracking (2017). doi:10.1109/TMTT.2016.2633352
20. Maaref N, Millot P, Pichot C, Picon OJITG. Sensing R: a study of UWB FM-CW radar for the detection of human beings in motion inside a building. IEEE Trans Geosci Remote Sensing (2009) 47(5):1297–300. doi:10.1109/tgrs.2008.2010709
21. Tarafder P, Choi W. Deep reinforcement learning-based coordinated beamforming for mmWave massive MIMO vehicular networks. Sensors (Basel, Switzerland) (2023) 23(5):2772. doi:10.3390/s23052772
22. Sun R, Suzuki K, Owada Y, Takeda S, Umehira M, Wang X, et al. A millimeter-wave automotive radar with high angular resolution for identification of closely spaced on-road obstacles. Scientific Rep (2023) 13(1):3233. doi:10.1038/s41598-023-30406-4
23. Mercuri M, Torfs T, Rykunov M, Laureti S, Ricci M, Crupi F. Analysis of signal processing methods to reject the DC offset contribution of static reflectors in FMCW radar-based vital signs monitoring. Sensors (Basel, Switzerland) (2022) 22(24):9697. doi:10.3390/s22249697
24. Lin JJ, Guo JI, Shivanna VM, Chang SY. Deep learning derived object detection and tracking technology based on sensor fusion of millimeter-wave radar/video and its application on embedded systems. Sensors (Basel, Switzerland) (2023) 23(5):2746. doi:10.3390/s23052746
25. Turppa E, Kortelainen JM, Antropov O, Kiuru T. Vital sign monitoring using FMCW radar in various sleeping scenarios. Sensors (Basel, Switzerland) (2020) 20(22):6505. doi:10.3390/s20226505
26. Chuang HR, Kuo HC, Lin FL, Huang TH, Kuo CS, Ou YWJISJ. 60-GHz millimeter-wave life detection system (MLDS) for noncontact human. Vital-Signal Monit (2012) 12(3):602–9. doi:10.1109/JSEN.2011.2118198
27. Shafiq G, Veluvolu KC. Surface chest motion decomposition for cardiovascular monitoring. Scientific Rep (2014) 4:5093. doi:10.1038/srep05093
28. Lei P, Liang J, Guan Z, Wang J, Zheng TJIA. Acceleration of FPGA based convolutional neural network for human activity classification using millimeter-wave radar. IEEE Access (2019) 7(99):88917–26. doi:10.1109/access.2019.2926381
29. Liang X, Bi Z, Yang C, Sheng R, Xia X, Zhang Z, et al. Free-breathing liver magnetic resonance imaging with respiratory frequency-modulated continuous-wave radar-trigger technique: a preliminary study. Front Oncol (2022) 12:918173. doi:10.3389/fonc.2022.918173
30. Wang H, Li Y, Xia X, Hu L, Zhao J, Chen Q. Non-contact respiratory triggering for clinical MRI using frequency modulated continuous wave radar. In: Conference on Medical Imaging: Physics of Medical Imaging: 2021; 14 – 17 February 2021 (2021).
31. Ren J, Li Y, Liu FS, Liu C, Zhu JX, Nickel MD, et al. Comparison of a deep learning-accelerated T2-weighted turbo spin echo sequence and its conventional counterpart for female pelvic MRI: reduced acquisition times and improved image quality. Insights into imaging (2022) 13(1):193. doi:10.1186/s13244-022-01321-5
Keywords: magnetic resonance imaging, respiratory gating, frequency-modulated continuous wave, respiratory belt trigger, respiratory motion artifacts
Citation: Song Q, He Y, Chen L, Xia X, Wang N, Song Q and Liu A (2024) Feasibility of the application of frequency modulated continuous wave radar trigger technique in abdominal magnetic resonance imaging. Front. Phys. 12:1319678. doi: 10.3389/fphy.2024.1319678
Received: 14 October 2023; Accepted: 20 February 2024;
Published: 08 April 2024.
Edited by:
Florian Wiesinger, GE Global Research, GermanyCopyright © 2024 Song, He, Chen, Xia, Wang, Song and Liu. This is an open-access article distributed under the terms of the Creative Commons Attribution License (CC BY). The use, distribution or reproduction in other forums is permitted, provided the original author(s) and the copyright owner(s) are credited and that the original publication in this journal is cited, in accordance with accepted academic practice. No use, distribution or reproduction is permitted which does not comply with these terms.
*Correspondence: Ailian Liu, liuailian@dmu.edu.cn