Urban Artificial Intelligence: From Automation to Autonomy in the Smart City
- Department of Geography, Trinity College Dublin, Dublin, Ireland
Technological innovation is constantly reshaping the materiality and mechanics of smart-city initiatives. Recently, innovation in artificial intelligence (AI) in the shape of self-driving cars, robots and city brains, has been pushing the so-called smart city to morph into an autonomous urban creature which is largely unknown. In this emerging strand of smart urbanism, artificially intelligent entities are taking the management of urban services as well as urban governance out of the hands of humans, operating the city in an autonomous manner. This paper explores, in theory and practice, how the development of AI intersects with the development of the city. The contribution of the paper is threefold. First, the paper advances a theoretical framework to understand AI specifically in urban contexts. It develops the concept of urban artificial intelligence, capturing the main manifestations of AI in cities. Second, the paper examines the case of Masdar City, an Emirati urban experiment, to show how the genesis of urban artificial intelligences is part of a long-standing process of technological development and a politico-economic agenda which together are enabling the transition from automation to autonomy. Third, it proposes a research agenda to investigate what the paper terms the autonomous city.
Introduction: AI Enters the City
Many cities are currently being developed under the smart city banner. As several studies show, a plethora of smart-city initiatives are being implemented across different geographical locations, forming a complex tapestry of urban visions (Fernandez-Anez et al., 2017; Pinna et al., 2017; Cowley et al., 2018; Datta, 2018; Karvonen et al., 2018a; Valdez et al., 2018; Wu et al., 2018; Dowling et al., 2019; Joss et al., 2019; Smigiel, 2019). A single definition and understanding of the smart-city ideal does not exist, and the very existence of a smart city is debatable. As Picon (2018, p. 270) argues, “the smart city belongs partly to the imagination”: a condition which makes the incarnations of this urban ideal hard to identify. As Caprotti (2019, p. 2466) points out, “there are few places and spaces in the contemporary city that can be visualized and made legible as clearly belonging to the smart city.” Moreover, although advocates and practitioners tend to draw upon a fairly homogeneous set of ideas inspired by an adamant faith in technology, actually existing smart-city projects manifest a number of contextual variegations (Angelidou, 2015; Shelton et al., 2015; Cugurullo, 2018a; Karvonen et al., 2018b; Bina et al., 2020).
Within this vast pool of alleged smart cities, information and communication technology (ICT) is a common denominator (Caragliu et al., 2011; Kitchin, 2014; Marvin et al., 2015; Coletta et al., 2018). The portfolio of a smart city normally includes smart grids, smart sensors and Internet of Things (IoT) technologies, deployed to produce large volumes of data on the metabolism of cities regarding, for instance, energy consumption and mobility. According to the narrative of smart urbanism, such big data can then be employed to penetrate and wipe out, metaphorically speaking, the “heart of darkness” of cities, in order to scientifically understand how they function and improve their sustainability. However, the presumed scientific mission of smart cities has frequently served the purpose of hiding economic and political agendas. Like in Conrad (2007)'s novel, science and progress have been used as rhetorical devices to legitimize the reproduction of existing power relations, ideologies and political economies. Smart technologies have not always been lights dispelling ignorance or darkness on the way the city functions, but rather profitable commodities for tech companies. As Trencher (2019, p. 117) remarks, in smart-city initiatives it is often the case that “neoliberal economic interests are prevailing at the expense of environmental and social concerns.” In essence, as many studies show, smart and sustainable are not necessarily synonymous with each other (Vanolo, 2016; Ahvenniemi et al., 2017; Bibri and Krogstie, 2017; Kaika, 2017; Cugurullo, 2018b; Machado Junior et al., 2018; Martin et al., 2018; Perng et al., 2018; Haarstad and Wathne, 2019; Parks and Rohracher, 2019; Colding et al., 2020).
The premise of this paper is that the challenge of critically exploring smart-city initiatives and extricating the rhetoric from what is actually happening on the ground, is exacerbated by the fact that the face of smart urbanism is constantly changing at a fast pace. Being smart-city projects fuelled by rapid processes of technological innovation, the moment a new typology of smart urban technology is released onto the market, the dynamics of smart urbanism change. This condition is of course not symptomatic of only smart-city agendas, inasmuch as the infrastructure and design of cities have historically been changing in sync with technological progress (Mumford, 1961). Emblematic is, for instance, the reconfiguration of cities in the early twentieth century due to the advent of the car (Hall, 2002). On these terms, what is new about smart urbanism is not that the evolution of the city follows the evolution of technology. The novelty springs largely from the smart technology that enters the city.
As a corollary of this premise, it can be argued that the more revolutionary and disruptive a smart technology is, the greater is likely to be the transformation of the city that integrates it. This tension has been observed by Batty (2018) in relation to artificial intelligence (AI). Today, innovation in AI in the shape of, for example, self-driving cars, robots and autonomous platforms of urban infrastructure management, is pushing the smart city to morph into an urban creature which is largely unknown (Batty, 2018; Allam and Dhunny, 2019). Arguably for the first time in history, artificially intelligent urban technologies are taking the management of urban services out of the hands of humans, operating the city in an autonomous way. There seems to be no limit to the agency of AI in cities. AI is now going beyond the provision of urban services and the maintenance of urban infrastructure. Artificial intelligences are entering the domains of urban governance and planning, thus becoming decision and policy makers (Zhang et al., 2019). This is an emerging strand of smart urbanism which the paper refers to as the autonomous city.
Building upon the above premise, the aim of this paper is to contribute to the understanding of the autonomous city, in empirical and theoretical terms. As noted by several scholars, to date there is little or no knowledge of the many ways through which AI is impacting on the development of smart-city initiatives (Del Casino V. J. Jr., 2016; Zakharenko, 2016; Ingrand and Ghallab, 2017; Milakis et al., 2017; Bissell, 2018; Salehi and Burgueño, 2018; Macrorie et al., 2020; Yigitcanlar et al., 2020). This study captures the evolution of smart urbanism in the era of artificial intelligence. It develops a theoretical framework to explain the manifestations and repercussions of AI in cities, and it explores empirically the emergence of artificial intelligence in an existing smart-city project. Structurally, the paper is divided into three parts. The first part discusses the theory of AI, particularly in relation to cities. It defines the concept of urban artificial intelligence and introduces three related categories (autonomous cars, robots and city brains), putting emphasis on both their revolutionary and conservative characters as opposite sides of the same coin. The second part analyses the case of Masdar City in Abu Dhabi, to show how the development of urban artificial intelligences is not an abrupt phenomenon, but rather part of a long-standing process of technological development and a politico-economic agenda which together are enabling the transition from automation to autonomy. The condition of being autonomous is examined from a technological point of view, and it is in the nascent capacity of AI to think and act in an unsupervised manner, that this study finds the key difference between traditional smart-city initiatives and emerging autonomous cities. The third and final part summarizes the main contributions of the paper, using them as foundations to build a research agenda for the study of the autonomous city.
Empirically, the paper is based on 10 months of field research in Abu Dhabi. In 2011, 20 semi-structured and 18 unstructured interviews were conducted with representatives from key state and non-state actors involved in the Masdar City project. In addition, the data that emerged from the interviews was triangulated through the analysis of key policy documents, such as masterplans and planning agendas. In 2019, five additional semi-structured interviews were carried out online, and more policy documents were collected by field assistants based in Abu Dhabi. Some of the material that is here discussed is not publicly available and the paper does not refer directly to those who disclosed it, in order to protect their anonymity. Taking into account Abu Dhabi's authoritarian regime and the safety of all research participants, sensitive data (including details about when and where exactly it was collected) has been anonymized.
Urban Artificial Intelligences
What is artificial intelligence? A single and universal definition of AI does not exist, nor a definitive blueprint to build one (Bostrom, 2017; Cave et al., 2020; Clifton et al., 2020). Yet, it is possible to identify the key characteristics and elements that are common among artificially intelligent entities. This section begins by discussing the core traits of artificial intelligence, to then use them as a stepping stone to clarify the meaning of urban artificial intelligences. In other words, the narrative starts from the notion of AI in general, subsequently narrowing down to AI specifically in urban contexts.
The concept of artificial intelligence is composed of two interrelated sub-concepts. The first one, artificial, is relatively easier to unpack. Something is commonly considered to be artificial when it does not arise from a natural process. As such, it is human-made or, as Bostrom (2017) observes, it can be made by machines. Whether it is created by humans or machines, the outcome of an artificial process of production is an artifact. Artifacts can have a multitude of shapes, ranging from a personal computer to a car. The shape of an artifact can be anthropomorphous or it might differ substantially from the physiognomy of the human body. Regardless of its shape, an AI normally resides in an artifact and this is a key characteristic to bear in mind as we continue the conceptual exploration of artificial intelligences. Many AIs, especially those that are the focus of this paper, are embodied. They possess and animate an otherwise inanimate artifact, thereby becoming an intrinsic part of it. An artifact such as a car, for example, when it is animated by an AI becomes an autonomous car endowed with intelligence.
The second sub-concept, intelligence, has been the subject of fiery debates since the birth of philosophy. The aim here is not to provide a definition of the notion of intelligence, but rather to draw upon academic studies on AI to discuss what skills and capabilities intelligent entities are supposed to have, as a way to understand how intelligence manifests itself in AI. First, an intelligent entity is supposed to be capable of learning, by acquiring information on the surrounding environment (Russell and Norvig, 2016). AIs practice learning, intended as gaining knowledge, both directly by sensing the environment through, for instance, cameras and microphones, and indirectly by means of large data sets installed by the developers. A second interconnected skill is the capacity to make sense of the acquired data by extracting concepts from it (Bostrom, 2017). Examples vary from a straightforward concept like morning which an AI extracts from visual data capturing the sun rising, to a more complex concept such as danger which an AI might extract from visual data showing an unauthorized individual entering a private property. Third, an AI should be able to handle uncertainty (Kanal and Lemmer, 2014; Pearl, 2014). This is the ability to deal with complex situations in which some information is missing or the data is not completely clear. Fourth, an AI would use the collected and potentially incomplete information to make decisions and then act in a rational way. For AI scholars, acting rationally is about attempting to obtain the best possible results, according to pre-defined performance measures and goals which clarify what is right or wrong (Russell and Norvig, 2016). In this regard, a classic example is provided by Asimov (2018) and his Laws of Robotics. Assuming that a robot is meant not to injure humans, a rational action would be one seeking to avoid any kind of harm to human beings. Fifth and finally, an AI would show intelligence by exercising the above skills and capabilities in an autonomous way or, as Levesque (2017, p. 3) puts it, in an unsupervised manner, meaning that humans are out of the loop and do not control or steer the AI's decisions and actions.
By combining the two sub-concepts, artificial and intelligence, we can broadly understand an AI as an artifact able to acquire information on the surrounding environment and make sense of it, in order to act rationally and autonomously even in uncertain situations. It is beyond the scope of this paper to discuss whether or not the above capabilities imply that AIs can think or manifest consciousness, which is a highly debatable topic (Warwick and Shah, 2016; Carter et al., 2018). However, it is worth noticing that the capacities of extracting concepts and making decisions autonomously, in particular, resonate with what we commonly call thinking. Of course, as we will soon see empirically, AI theory is not perfect and should not be approached in a dogmatic way, given that even the theoretically simplest concept extracted by an artificial intelligence can empirically turn into a nightmare. This is because AI is a disruptive technology (Batty, 2018; Yigitcanlar et al., 2020). On these terms, it changes the system where it operates. It is also, as Greenfield (2018) notes, a radical technology since the changes that it triggers can be deep, significant and might invest every scale of the everyday, from the personal life of individuals to the governance of cities, and from the organization of states to global geopolitics. Therefore, it is safe to assume that when abstract theories of artificial intelligence meet the sheer complexity of the real world, discrepancies and tensions are bound to emerge.
Nonetheless, theory is useful to orientate any meaningful discussion on AI. Specifically for the purpose of this paper, we can use the above theoretical materials to understand urban artificial intelligences as artifacts operating in cities, which are capable of acquiring and making sense of information on the surrounding urban environment, eventually using the acquired knowledge to act rationally according to pre-defined goals, in complex urban situations when some information might be missing or incomplete. Moreover, urban AIs operate autonomously. They make decisions in an unsupervised manner, thus displaying a rudimentary form of thinking, and take actions which can potentially trigger radical changes in the city. In relation to this broad definition of urban artificial intelligence, we can observe three different, and yet similar, specific examples of urban AI, each one with its own characteristics, urban domain and potential set of urban repercussions.
The first category of urban artificial intelligence is represented by autonomous cars. Here the artifact where the artificial intelligence resides is a car. This is a technology which is being employed in an increasing number of cities, thereby gradually entering the everyday (Milakis et al., 2017; Talebian and Mishra, 2018; Acheampong and Cugurullo, 2019). The AI in question is capable of sensing the surrounding urban environment by means of cameras, radars and lidar systems. In addition, it can learn about the city through downloadable data sets capturing different urban aspects, such as roadmaps and weather forecasts. The artificial intelligence employs this information to drive the car to a given location and, at the highest level of autonomy (level 5), no human input or supervision is required, with the AI theoretically capable of handling uncertain situations autonomously. Practically, as the first pedestrian fatality caused by an autonomous car proves, this is an urban artificial intelligence whose skills, capabilities and, more in general, intelligence are still questionable. On the 18th of March 2018, in the city of Tempe (Arizona) an autonomous Uber car was not capable of handling an unexpected scenario, a woman crossing on foot a road outside the designated pedestrian crosswalk while carrying a bike, and it run over her (see also Stilgoe, 2019). In fact, although the technology of autonomous driving is rapidly progressing, severe technological limitations currently exist which are limiting both its employment in real-life environments and people's trust in it (Fridman et al., 2019). Yet, despite these limitations, several governments, car manufactures and ridesharing companies are keen to accelerate the deployment of autonomous vehicles in cities, and sociological studies show that a significant number of urban dwellers are ready to give up their current means of transport in favor of a car driven by an artificial intelligence (Hulse et al., 2018; Cugurullo et al., 2020). The transition to an autonomous urban transport would trigger a number of substantial urban changes. If enabled by sharing services, it could decrease the number of cars on the road and so the quantity of energy and, above all, urban space that they need to operate, thereby favoring a less car-centric redesign of the built environment (Duarte and Ratti, 2018; Cugurullo et al., 2020; Guériau et al., 2020). Conversely, private and highly comfortable autonomous cars which drive themselves while their users can work or sleep, might prompt “people to travel more frequently and across greater distances,” thus fostering commuting, suburbanization, an energy-intensive lifestyle and, in essence, the production of car-centric urban spaces (Hawkins and Nurul Habib, 2019, p. 69). In addition to reshaping the built environment and the geography of housing, the transition to autonomous cars is also likely to reshape urban mobility, particularly in relation to people with disabilities and minors currently not allowed to employ a car (Bennett et al., 2019). Last but not least, the emergence of artificial intelligences in urban transport poses concrete ethical challenges. Normally, the AI controlling a car is making decisions of a geographical nature: what route should be taken and where exactly in the city the car should go through, in order to reach the final destination. Occasionally, the same AI might have to make decisions of an ethical nature. Assuming a possible accident in which harm is unavoidable (perhaps because the brakes of the autonomous car are malfunctioning and the AI just does not have enough time to stop the car), how will the artificial intelligence choose to distribute inevitable harm? As several scholars have pointed out, this is a decision which could imply harming (and even killing) the passenger(s) or pedestrians, cyclists and other motorists (Goodall, 2014; Lin, 2016; Awad et al., 2018; Bonnefon et al., 2019). In the autonomous city, therefore, the AI's capacity of making decisions triggers moral dilemmas which require the machine to possess moral values.
The second category of urban artificial intelligence is represented by robots. This is a multiform category, since robots exist in many different shapes and populate diverse urban domains. We distinguish it from the first category, because autonomous cars operate in one specific domain (transport) and, despite heterogeneous brands, models and sizes, they all share the same fundamental design (they have an empty interior to accommodate passengers and need wheels to move on urban roads). On the contrary, urban robots escape rigid definitions and designs. Within this second category, the artifact animated by an AI can be an unmanned air vehicle (commonly called drone) as well as a humanoid machine mimicking the human body (Russell and Norvig, 2016). It can also be a nanobot almost invisible to the naked eye or an android almost indistinguishable from a person. Robots can be found in retail, customer service, hospitality, education, security and in the maintenance of urban infrastructure (Tiddi et al., 2019; Macrorie et al., 2020). Like autonomous cars, robots are equipped with sensors which make them capable of perceiving the built environment and acquiring information about what is around them. AI gives robots the ability to make sense of the acquired information, ultimately allowing them to interact with the prime inhabitants of the built environment: humans. Service robots, in particular, operate often in the frontline, communicating directing with customers (Wirtz et al., 2018; Mende et al., 2019). In this context, a robot operates in the presence of incomplete information, since every customer has an unknown personality and unknown requests which the artificial intelligence has to interpret and try to accommodate, without the help of a human operator. In so doing, the robot is de facto performing a job and it is precisely in the capacity of robots to generate labor their greatest source of disruption. As remarked by several scholars, robots constitute “a new class” of intelligent machines which is disrupting labor systems and “few employment fields are immune” (Bissell and Del Casino, 2017, p. 436; Del Casino V. J. Jr., 2016, p. 847). Pragmatically, unemployment is the most feared consequence of this type of urban AI, should robots in the long run outnumber and outsmart more expensive human workers (Korinek and Stiglitz, 2017).
The third category of urban artificial intelligence is the city brain. This is the most elusive manifestation of AI in the built environment. In this case, the artifact where the artificial intelligence is located is a digital platform and, for this reason, city brains can be also understood as an instance of platform urbanism (see van der Graaf and Ballon, 2019; Barns, 2020; Caprotti and Liu, 2020; Leszczynski, 2020). As exemplified by Alibaba's City Brain, this is an AI originally created for autonomous traffic management (Alibaba, 2020). A city brain acquires information about urban traffic directly by means of the so-called Internet of Things (more specifically, hundreds of interconnected cameras distributed all around the city) and indirectly by being fed with large data sets installed by Alibaba's computer scientists. It then uses the acquired knowledge to control traffic lights and direct the flows of vehicles and people in the city. Transport is only the initial domain influenced by the intelligence of the city brain. According to Alibaba (2020), this AI will go beyond the management of traffic and it will soon be employed in the realms of urban planning, health, safety and governance. Should this transition take place, its repercussions would be considerable and of a scale substantially larger compared to the case of autonomous cars and robots. In practice, a city brain treats the city like a giant artifact which can be controlled and optimized. However, there is a stark difference between an AI controlling a car and deciding autonomously about what the best route is, and an AI controlling an entire city and deciding autonomously about what the best strategy of urban development is. From a purely logistical point of view, formulating the best route for a car to follow implies dealing with information regarding a limited time frame while, as Batty (2018, p. 5) notes, urban planning is about the long term and deciding on not just what is best in the present, but also what will be beneficial to the city in the foreseeable future. From a technical perspective, this leads to the question of whether AI will be capable of dealing with high levels of uncertainty connected to the broad time windows that are typical in urban planning. From a philosophical perspective instead, given that urban planning and so urban governance are also about deciding on what is right or wrong, good or bad, sustainable or unsustainable, there is the thorny ethical question of how a non-human intelligence comes to determine what is ideal for a human environment. Here the fields of AI and urban studies overlap again with the field of ethics, showing that the autonomous city's nascent capacity of making decisions in an unsupervised manner presupposed a set of moral values which might be underdeveloped or, worse, missing.
Considered together, urban artificial intelligences like autonomous cars, robots and city brains, raise important questions regarding the future of the city. Because of the technological innovation that they embody and the novel urban scenarios that they are shaping, theirs is a narrative which easily tends to focus on the future and the extraordinary, thus risking to neglect the past and the ordinary. Indeed, some of the prospects presented by contemporary urban artificial intelligences are unprecedented. For example, the possibility of an entire city governed by a single artificial intelligence, as it is now presented by Alibaba's City Brain, escapes even the realm of mainstream science fiction. However, AI as an idea, as a field of research and as a technology is not new, nor is its application in cities. The field of artificial intelligence begun to be cultivated in the middle of the twentieth century and, since then, many applications of AI (albeit in an embryonic form compared to what we see today) have touched the built environment. Particularly in relation to cars, for instance, in the early 2000s scholars were already noticing how “it is not just the driver who possesses intelligence” and “has the capacity to act,” the car itself is “able to sense its environment, make judgments, and act accordingly” (Featherstone, 2004, p. 10; Thrift, 2004; Dodge and Kitchin, 2007). Meanwhile, in the domestic space, Dodge and Kitchin (2009, p. 1349) were finding several objects possessing “awareness of their environment” by means of sensors, and the ability to share information through the then emerging Internet of Things. “It is a world where we not only think of cities, but cities think of us” Crang and Graham (2007, p. 789) reflected, starting to feel the formation of an intelligence within urban infrastructure.
AI has a genealogy and it is therefore important to consider urban artificial intelligences as part of a broader process of technological and philosophical development in which new pieces are constantly added to a long-standing mosaic. On these terms then, it is equally important to identify what new technologies and ideas are being added, how they relate with the older pieces of the mosaic, and how the big picture that the whole mosaic is showing has been changing over the years. Moreover, although AI is a disruptive and radical technology, this does not necessarily mean that everything that AI touches is drastically altered. On the contrary, as Greenfield (2018, p. 8) stresses, “allegedly disruptive technologies” like artificial intelligence often “leave existing modes of domination mostly intact.” The technology per se might change and so its design and packaging, but the broader politico-economic dynamics underpinning its production, as well as the elites that benefit the most from the fruits of technological innovation, might remain unchallenged. In the case of autonomous cars, for instance, recent studies suggest that while the presence of a non-biological intelligence behind the wheel is out of the ordinary, the politics of autonomous urban transport follows ordinary neoliberal trajectories leading to all-too-familiar episodes of social injustice and undemocratic governance (Henderson, 2018; Cugurullo et al., 2020).
In light of the above, it is essential then to empirically examine recent urban artificial intelligences, bearing in mind that they are part of a broader and older process of technological innovation which might radically change some aspects of the city while leaving others intact. It is empirical research which can determine, on a case-by-case basis, what novel manifestations of intelligence are permeating the built environment and how cities are responding to them. This is the purpose of the next section which shifts the focus from the theory to a single case study, in the attempt to capture an example of the evolution of urban artificial intelligence from a condition of automation to one of autonomy while also picturing the surrounding politico-economic context. The paper now turns to the case of Masdar City and operationalizes the theoretical framework illustrated above, by identifying an instance of urban artificial intelligence and unpacking its core skills and capabilities. In so doing, the paper sheds light on how the Masdarian urban artificial intelligence learns, makes decisions and acts autonomously in a real-life environment, simultaneously triggering and preventing radical changes.
From Automation to Autonomy in Masdar City
Masdar City is a new master-planned settlement under construction in Abu Dhabi (United Arab Emirates). The project was launched in 2007 by the ruler of Abu Dhabi, Sheikh Khalifa, and is expected to be completed in 2030. Masdar City is a state-led and funded urban project managed by a public company called the Masdar Initiative. This ambitious US$ 20 billion-project is now in its second decade of development. Built with the aim of serving as a testbed for experimental urban technology, Masdar City has seen for over 10 years the development of a plethora of smart technologies ranging from smart grids to driverless vehicles (Cugurullo, 2016; Griffiths and Sovacool, 2020). During this time frame, the Masdarian technology has been exposed to the influence of innovation and modernization and, like most technologies, it has evolved, thereby changing its shape, mechanics and function. More recently, AI has become a prominent feature of the technological portfolio of the Emirati city which, due to its experimental nature and prolonged experience in smart urbanism, is a useful case study to observe the gradual emergence of urban artificial intelligence and trace back empirically its origin.
This section focuses on urban transport and its technological evolution in Masdar City, as arguably the most emblematic dimension of the Emirati project where AI manifests itself not as an abrupt phenomenon, but rather as part of a broader and multifaceted process of development. The story of Masdar City's system of urban transport begins in 2009, shortly after the launch of the Emirati project, with the construction of the Personal Rapid Transit (PRT). The PRT is a system of driverless cars operating in an underground level of the city, called the undercroft (see Figure 1). The original vision of the planners of Masdar City, the international architecture firm Foster+Partners, was that of a city divided into two levels: above a compact car-free city characterized by narrow pedestrian streets, below the undercroft with shared PRT cars functioning as a public transport system. The image of the PRT (cars not driven by a human being) might resonate with the notion of artificial intelligence, but in reality this is not the case. The PRT is an automated technology, not an autonomous technology and to understand this crucial difference, the first step is to examine in more detail how and where PRT cars operate.
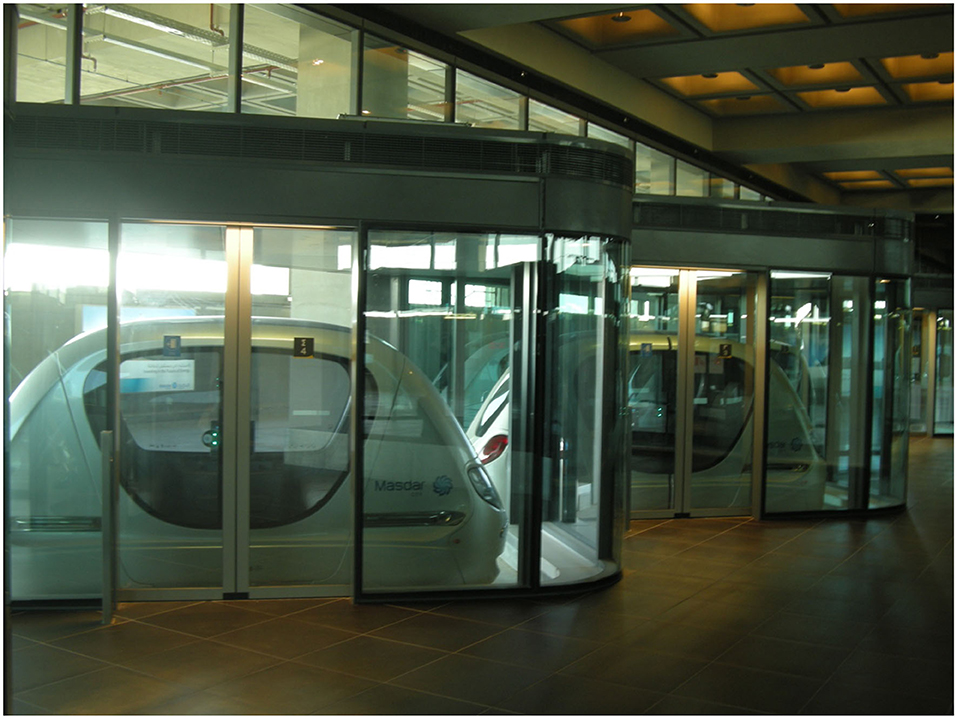
Figure 1. PRT driverless cars in a station located in Masdar City's undercroft. Source: author's original.
A journey on the PRT starts always in a PRT station which looks and is experienced like a common metro station. As a passenger you find yourself in an underground environment designed and built exclusively for PRT cars to stop and take on passengers. Before entering a PRT car, it is necessary to press a button which opens first the locked sliding door of the glass box where the vehicle is parked, and then the door of the vehicle itself. The first door only opens if there is an available PRT car behind it, meaning that this is a confined urban space which can be entered only by entering a PRT car. Once inside, it is time to choose the destination. To do so, next to the seats is a touchscreen where your options are visualized. PRT cars can move only from a PRT station to another PRT station, according to routes pre-determined by the planners of Masdar City. After one of the pre-defined destinations has been selected, the PRT begins to move, leaving its glass box and traversing the undercroft at a speed of maximum 40 km/h.
The journey has begun and there are three important aspects to note. First, there is no driver nor any form of intelligence which is sensing the surrounding environment and then deciding on what the best route is. Second, the PRT is just following a pre-defined track which is clearly visible on the surface of the road (see Figure 2). What is happening has little to do with AI as it was understood in the previous theoretical section. The roads of the undercroft are simply filled with sensors drilled into the ground, and placed in a sequential manner. Every sequence of sensors forms a PRT track. These sensors send a signal to the PRT car which is capable of detecting them. In practice, they act like magnets and this is how an engineer from the Masdar Initiative described them in an interview. The magnets irresistibly attract the machine which is uncapable of sensing anything else. During an interview with one of the managers of Masdar City, the following exchange occurred:
Interviewer: What would happen if, hypothetically speaking, I walked in front of a PRT car in motion?
Interviewee: It's very simple. The PRT would run over you.
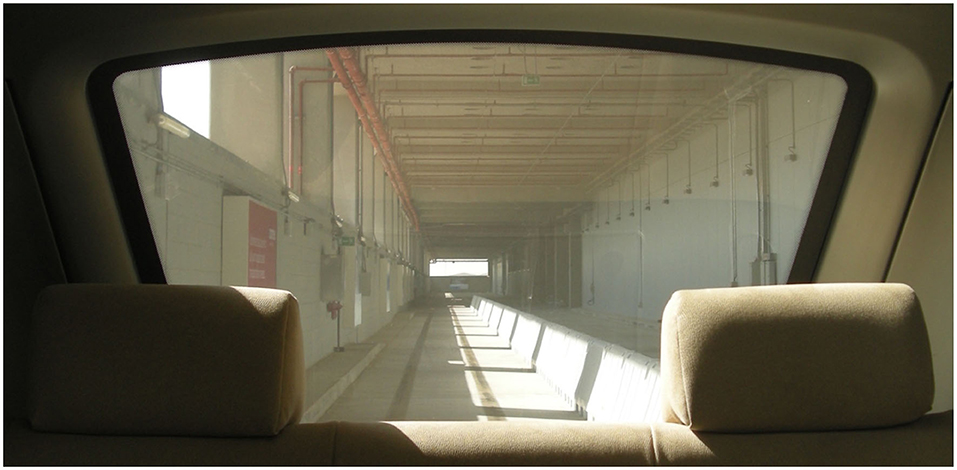
Figure 2. View from inside a PRT vehicle in motion. The machine is traversing Masdar City's undercroft. The two parallel lines visible on the road ahead form the track that the PRT car is following. Source: author's original.
This second aspect is connected to the third one: the design of the built environment. The undercroft was designed as a confined space where pedestrians and non-PRT vehicles are not allowed. This type of urban design serves the purpose of eliminating uncertainty. More specifically, given that the machine is not capable of sensing the passage of humans and other vehicles, the surrounding environment is designed precisely to prevent the passage of humans and other vehicles, thereby ultimately preventing the risk of pedestrian fatalities and car accidents. The boundaries of the undercroft are walled and the few entrances close to the PRT tracks are blocked by means of barriers (see Figure 3). Essentially, the undercroft is a space of repetition where the same exact action is supposed to take place under the same exact conditions. Any variation on the Masdarian theme could be deadly, and the built environment is literally a barrier blocking the out of the ordinary.
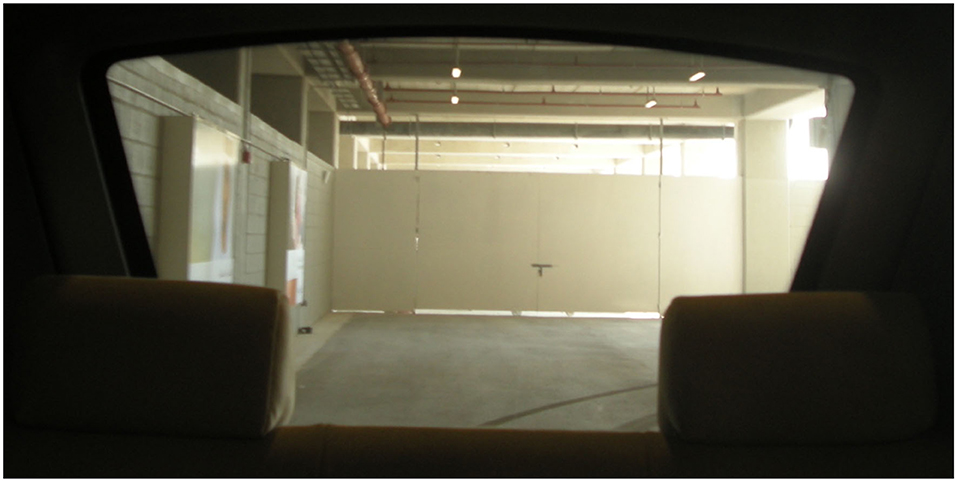
Figure 3. On its way to the next station, the PRT car turns passing close to a blocked entrance leading outside Masdar City. The track that the vehicle is following is still visible on the ground. Source: author's original.
Ten years after the beginning of the PRT experiment, the Masdar Initiative started to experiment with another form of urban transport: the autonomous car. The model introduced and currently operational in the Emirati City is a Navya Autonom, a technology which is considerably different from the PRT (Navya, 2020a). The three key aspects observed earlier along a trip on a PRT car are absent. First, although like in the case of the PRT there is no apparent driver, the Autonom is an artifact animated by an artificial intelligence capable of perceiving the surrounding environment. The AI itself is the driver. The Autonom is equipped with two lidar systems and two cameras which acquire information on the space where the car operates and detect obstacles (Navya, 2020b). Second, the Autonom does not follow a track nor any pre-determined route. On the basis of the data collected, it makes a decision on the run, determining the route in an unsupervised manner (Navya, 2020c). The roads that will be taken to get to the final destination are chosen by the AI. Third, the Autonom's environment is not a confined space. This is a machine employed in open urban spaces designed to accommodate the flow of people and other vehicles, instead of preventing them like the undercroft does.
When compared, the PRT and the Autonom present remarkable differences. The former is an example of automation since its actions are pre-determined by decisions made beforehand by engineers and computer scientists working for the Masdar Initiative. The PRT operates without a human driver, but it possesses no intelligence. It is automated in the sense that it endlessly repeats what was instructed to do, in a confined space designed by Masdar City's planners to allow no variation. The latter instead represents autonomy because its actions are based on decisions which the machine itself has reached in an unsupervised manner. The Autonom is artificially intelligent due to the fact that it is an artifact able to learn about the surrounding environment and then act in the face of uncertainty. The built environment is here a key differential. The Autonom functions in urban spaces where repeating the same exact action under the same exact conditions is not possible. A city (contrary to the undercroft) is an open and ever-changing system. Specifically in relation to transport and mobility, every day a single ordinary road can be experienced by different vehicles and people moving according to geometries which will not be exactly the same the day after. Weather conditions might change too, and so the road itself as a result of repair works. Road closures can temporarily remove some spaces from the map, but such uncertain factors do not stop an intelligent machine like the Autonom from determining the best route. In automation, there is one pre-defined best and it is static. In autonomy, the understanding of best is constantly changing with the changing scenarios where urban artificial intelligences operate.
On the one hand, the advent of autonomous cars in Masdar City has considerably altered urban transport and mobility in the new Emirati city. Just the simple fact that now it is not necessary anymore to walk to an underground station to get access to a car, is a significant difference. While PRT vehicles continue to be operative exclusively in the undercroft, the rest of Masdar City is served by the Autonom which is accessible overground in unconfined spaces. Above all, the crucial distinction between the Autonom and Masdar City's conventional road transport lies in the AI's capacity of making decisions autonomously. As an urban artificial intelligence, the Autonom is capable of extracting concepts and choosing what to do. These are skills that, as noted in the previous section, are not simply logistical and geographical. An autonomous car does not just choose a route, it also executes the chosen route thereby placing itself in a scenario in which accidents and, thus, unavoidable harm are possible. What is new and different then is that, in Masdar City, there are machines which sooner or later will have to make ethical choices.
On the other hand however, despite the novelty brought by the Autonom, there is a considerable tripartite continuity between this recent urban artificial intelligence and Masdar City's older automated transport system. First, both the PRT and the Autonom are part of the same process of urban experimentation. As an experimental city, Masdar City has been trialing innovative technologies of urban transport for more than 10 years. This endeavor started in 2009 with the PRT and recently ended (at least for now) with cars driven by an AI. Members of the Masdar Initiative have tested both the PRT and the Autonom in the real-life environment provided by the new city, and it is the autonomous car that the Emirati company has judged the most successful transport technology. The implementation of the PRT has been halted and its service limited to ~10% of Masdar City. Newly paved roads for autonomous cars are the current priority of the Masdar Initiative.
The reason why the PRT experiment was ended, while the deployment of autonomous cars continues, relates to the second common denominator between the two transport technologies. They are both part of the same business project. In Masdar City, urban experimentation goes hand in hand with commodification. Every smart technology tested in Masdar City eventually becomes a product which is commercialized and sold internationally by the company that develops it. The Masdar Initiative offers the real-life environment where experimental technologies are tested, together with a team of engineers and computer scientists providing assistance during the experiment. For these services, once a product which was tested in Masdar City is sold, the Emirati company gets a percentage of the total revenues (Cugurullo, 2013a). Given these overarching economic dynamics, it is not surprising that the Masdar Initiative tends to prioritize the experimentation of those technologies for which there is more demand and that are more likely to generate revenues. On these terms, the shift from the PRT to the autonomous car follows a predictable business rationale as, with many countries around the world now keen to deploy autonomous cars, the Emirati company is simply betting on the most trending technology.
The third interconnected continuity is about what the Masdarian experimental technology and business project are supposed to sustain which, put simply, is the status quo. The Masdar Initiative (a public company and instrument in the hands of the government of Abu Dhabi) has been testing and commercializing smart technologies to diversify the national economy, so to preserve the political position of the royal family in the inevitable post-oil era (Luomi, 2009; Cugurullo, 2016). The political stability of the Emirati ruling class depends on the stability of the Emirati economy which is currently based on oil and meant to collapse, unless alternative sources of capital are soon found. Seen from this perspective, in the context of the Emirati political economy, AI is technologically new but politically it serves the purpose of keeping the long-standing power of the sheikh intact, thus conserving a regime which as critical scholars have repeatedly remarked is authoritarian and undemocratic in nature (Ponzini, 2011; Crot, 2013; Cugurullo, 2013b; Caprotti, 2014). In the desert of Abu Dhabi, technological progress has been fast, with a newly built city showing in the space of 10 years the passage from automation to autonomy and, yet, the broader politico-economic vision remains unchallenged showing little or no sign of progress.
Conclusions: Approaching the Autonomous City
The emergence of artificial intelligence in cities is a complex phenomenon, both theoretically and empirically, in which question marks outnumber the theories and empirics currently present in urban scholarship. This paper has taken some of the initial theoretical and empirical steps toward an understanding of the autonomous city. While the term autonomous city was previously used by scholars like Vasudevan (2017) and Norman (2018) to describe cities which are politically and economically independent, the word autonomous has been here employed to stress the presence of urban artificial intelligences capable of thinking and acting in an unsupervised manner. On these terms, an autonomous city is a city where autonomous cars, robots and city brains are increasingly performing tasks and taking on roles which have traditionally been the domain of humans. With humans left out of the loop, a city becomes autonomous in the sense that it is capable of operating without human inputs.
By developing the concept of urban artificial intelligence, this study has illuminated a fundamental aspect of the autonomous city. Theoretically, the paper has unpacked the core skills and capabilities of artificial intelligences operating in cities, explaining the meaning of being intelligent in relation to cars, robots and digital platforms, and exposing the principal urban challenges of non-biological intelligence. Empirically, it has tracked the emergence of AI in Masdar City, showing how smart-city solutions are evolving into autonomous technologies which manage parts of the city without human supervision. However, substantial gaps in knowledge remain unexplored and, rather than a portrait of the autonomous city, this contribution should be intended as a door to enter the autonomous city and begin its empirical and theoretical investigation. This final section highlights the key findings of the paper, using them as stepping stones to advance a research agenda. Technological innovation in the field of AI is progressing rapidly, and the social sciences and humanities should not lag behind. The following six points seek to orientate urban studies and cognate disciplines toward a critical understanding of AI in cities.
First, AI is being incarnated in an number of urban artifacts. Urban artificial intelligences are artifacts located and operative in cities, which have the capacity to learn, think and act autonomously. Specifically, in relation to the categories presented in this paper, examples can be found in urban transport services managed by autonomous vehicles, in restaurants and shops managed by service robots, and in the governance of cities managed by city brains. However, the three categories of urban artificial intelligence proposed here remain empirically underexplored and are open to be theoretically refined and expanded on the basis of empirical data. For example, while there is a burgeoning literature on autonomous cars, little is known about urban robots and city brains. This paper has remarked that robots represent an extremely diverse category of urban artificial intelligence. A taxonomy of urban robots is therefore needed to identify what specific types of robot are populating cities, and to locate their sphere of influence. Similarly, there is a lack of understanding of the range of action of city brains, from a geographical and operational perspective. What outdoor and indoor urban spaces are exactly being sensed by a city brain, in what urban domains this large-scale AI is operative and what kind of decisions it has the power to make, are aspects in need of research. Furthermore, besides the embodied AIs discussed in this paper, many artificial intelligences exist without a physical incarnation. In AI literature, they are commonly called software agents because of their capacity to act despite missing a body (Russell and Norvig, 2016). These AIs abound in cities and, although their actions are immaterial, their consequences are tangible. A computer program deciding autonomously on who is worthy of an insurance or a mortgage (O'Neil, 2016). Contact-tracing apps monitoring the mobility and health of people, and determining who has to be in quarantine during a pandemic (Kitchin, 2020; Morley et al., 2020). The autonomous city has an immaterial and invisible dimension which has to be urgently examined.
Second, this paper has emphasized the presence of intelligence in urban artifacts and discussed the notion of artificial intelligence by drawing upon AI literature. However, the concept of intelligence goes well-beyond the field of AI and encompasses a plethora of other disciplines such as philosophy, neuroscience, psychology, linguistics and behavioral economics (Sternberg, 2020). It is therefore crucial to interrogate from an interdisciplinary perspective the intelligence of autonomous cars, robots, city brains and other urban artificial intelligences, by clarifying what exactly makes them intelligent and in relation to what urban domain. Intelligence, and so artificial intelligence, should not be approached nor depicted in black and white. Being intelligent is a hyper complex quality full of gray areas. A person might manifest intelligence in one domain, while showing stupidity in others. Likewise, urban artificial intelligences might intelligently perform a specific task and utterly fail to comprehend another activity. Moreover, intelligence is not a box to tick, but rather a quality expressed at different levels and degrees. Research questions like Are city brains, robots and autonomous cars intelligent? should then be rephrased by adding To what extent in the beginning.
Third, this paper has shown that the manifestation of AI in cities is neither a sudden phenomenon, nor an extensive urban revolution. From a technological point of view, urban artificial intelligences are part of a broader process of development which is now culminating in the passage from automation to autonomy. While this passage presents some innovative elements, such as the formation of novel technologies capable of thinking and acting autonomously, there are also components which have been around for several decades. Autonomous cars, for example, employ sensors to perceive the surrounding environment. The cameras of the city brain capture information and then pass it to a central digital platform by means of the Internet of Things. City brains, autonomous cars and robots can all be fed with large data sets, the so-called big data, to indirectly learn about the city where they operate. Smart sensors, the Internet of Things and big data are traditional features of smart-city initiatives, far from being novel, and can be seen as points of intersection between smart urbanism and an emerging autonomous urbanism. From a politico-economic perspective instead, artificial intelligence is part of broader programmes of economic growth and diversification, as well as it is embedded in political agendas and ideologies that long predate the genesis of AI. Urban artificial intelligences can thus be technologically revolutionary while, at the same time, preventing the outbreak of political, economic and ideological revolutions. Careful empirical research is therefore much needed to identify the technological lineage of autonomous urbanism and, above all, what doors this urbanism is opening or keeping closed in politico-economic and ideological terms. There is a tension in particular which should be investigated and exposed. If smart-city operations and technologies have been about producing data and gaining knowledge, then the passage to autonomy via AI suggests a further step: the capacity to use data in order to extract concepts and make decisions. In this sense, the key innovation differentiating the smart city from the autonomous city, is in the latter's ability of thinking. Assuming that, as originally theorized by Turing (1950), machines can indeed think, it is vital to understand and evaluate what they are thinking. This line of research would shed light on what concepts and decisions are being developed by urban artificial intelligences, and assess the extent to which their thinking echoes existing ideologies and regimes. The machine's act of thinking might be innovative, but the thinking per se could be conservative.
Fourth, the case study examined in this book has demonstrated that the emergence of urban artificial intelligences is context dependent. The specific experience of Masdar City is that of an experimental city built from scratch which has been trialing and commercializing new technologies for over a decade, in order to preserve the political structure and power of a sheikhdom. Similar experiences are certainly possible. For example, Saudi Arabia (a Middle Eastern authoritarian state like Abu Dhabi) is investing $500 billion in the construction of a new master-planned city, Neom, in which according to the Saudi Crown Prince “everything will have a link with artificial intelligence” and robots will outnumber humans (Bloomberg, 2017; Neom, 2020). In a different geographical and political context, experimental urbanism meets again autonomous urbanism in China where the Government of Shanghai has released official plans to build the first town in the world to be completely operated by an artificial intelligence: Beiyang AI Town. However, these ambitious urban experiments à la Masdar are likely to remain exceptional, due to the sky-high costs and planning challenges typical of ex novo experimental urban mega projects (see Cugurullo and Ponzini, 2018). It is therefore important to study urban artificial intelligences in other strands of experimental urbanism, particularly in small-scale experimental urban projects affecting existing cities (Evans et al., 2016; Bulkeley et al., 2019). Besides avant-garde experimental cities, geographical studies are essential to understand how AI penetrates inside ordinary cities, coexisting or clashing with older automated and manual technologies, across a broad spectrum of political systems and ideologies ranging from neoliberalism to socialism. While, as shown in this paper, experimental urbanism tends to promote the formation of confined spaces of repetition, outside urban experiments uncertainties are everywhere (Scoones and Stirling, 2020). It is in spaces of uncertainty that AI will reveal its greatest limitations and that is the terrain where it should be observed the most.
Fifth, this paper has argued that, from an AI perspective, the city itself can be seen as an artifact animated by a non-human intelligence. This view does not necessarily negate that of the fluid city made of flows, as it is commonly pictured in urban political ecology (Heynen et al., 2005; Kaika, 2005; Gandy, 2014). On the contrary, the image of the artifact implies changes and flows, because cities are constantly (re)built, modified, expanded and traversed by flows of energy, data, people and capital. What matters for the study of the autonomous city is that like an AI can possess an inanimate object such as a car, so it can possess the built environment and the infrastructure of a city. This is the idea at the foundation of large-scale urban artificial intelligences, like Alibaba's City Brain, which are developed to animate and control entire cities. Although the principle is the same, the paper has noted how there is a substantial difference between managing the route of a car and the development of a city. The bottom line is that every urban artificial intelligence is different and will impact differently on the city. Researchers should avoid treating AI as a homogeneous category of intelligence, because too much divergence exists among and within urban artificial intelligences. What is needed is empirical research examining how specific (and diverse) models of autonomous cars, robots and city brains are reshaping cities. As in science we do not generalize biological intelligence, instead appreciating the variety of intelligent life forms on Earth, the same logic applies to the study of artificial intelligence.
Sixth, the emergence of city brains and large-scale artificial intelligences raises important questions about the governance of cities since, arguably for the first time in history, non-biological intelligences are beginning to determine urban development in an unsupervised manner. In principle, as recent findings in computer science point out, “autonomous machines can learn to establish cooperative relationships with people and other machines, even in the midst of conflicting interests and threats of being exploited” (Crandall et al., 2018, p. 8). The evident similarity between the complexity of the abstract scenarios used by computer scientists in their simulations and the complexity of real-life urban governance, suggests the hypothesis that AI might be used in the management of cities to address complex urban problems, including issues of sustainability (Vinuesa et al., 2020). Such hypothesis remains untested and should be approached with care, because as smart and sustainable are not synonymous which each other, it is unlikely that autonomous will suddenly become synonym for sustainable. This gap in knowledge calls for further empirical research on artificial intelligence in urban governance, with a focus on sustainability intended not in a one-dimensional way so to capture the full spectrum of social, environmental and economic variables that can be affected by AI (Floridi et al., 2018, 2020). This analytical task, however, requires an important preliminary conceptual step and, more specifically, “the scientific study of intelligent machines, not as engineering artifacts, but as a class of actors with particular behavioral patterns and ecology” (Rahwan et al., 2019, p. 477). In essence, humans need to learn how to cooperate with machines and vice versa, in the challenging game of governance. On these terms, an AI is seen as an actor which, similarly to a human being, has an intelligence, manifests a behavior and acts autonomously in the governance of the city.
On the one hand, the challenge is technical in nature. Codifying principles of urban governance to inform artificial intelligences, for instance, requires interdisciplinary studies conducted by AI scholars and governance scholars, under the premise that as a single universal model of governance does not exist, so a single blueprint for artificial intelligence cannot be found. On the other hand, the challenge is a deeply philosophical one. For what reason and for whose purpose should urban artificial intelligences be employed? What is right and what is wrong in urban development when cities are populated by humans as well as by intelligent machines? These are crucial questions of ethics whose answers should not be postponed until the technology is already functional. Nor should these answers be left to the technology itself. As Bostrom (2017) remarks, the values, ideals and goals of an AI might be considerably different from those of its creators, simply because we cannot expect a non-human intelligence to think exactly like humans do. By the same token, the agenda of a city brain might diverge substantially from traditional urban agendas, and thus have unexpected negative consequences for the dwellers of the autonomous city. Philosophical inquiry should therefore be proactive and inform the development of AI, as it now intersects with the development of the city.
Data Availability Statement
The datasets generated for this study will not be made publicly available to protect the anonymity of the interviewees.
Author Contributions
The author confirms being the sole contributor of this work and has approved it for publication.
Funding
The fieldwork in Abu Dhabi was funded by the Royal Geographical Society via the Dudley Stamp Memorial Award.
Conflict of Interest
The author declares that the research was conducted in the absence of any commercial or financial relationships that could be construed as a potential conflict of interest.
Acknowledgments
The author is grateful to Gregory Trencher, James Evans, Andrew Karvonen, and the two reviewers for their invaluable intellectual support. This research is part of WISE-ACT (COST Action 16222).
References
Acheampong, R. A., and Cugurullo, F. (2019). Capturing the behavioural determinants behind the adoption of autonomous vehicles: conceptual frameworks and measurement models to predict public transport, sharing and ownership trends of self-driving cars. Transp. Res. Part F Traffic Psychol. Behav. 62, 349–375. doi: 10.1016/j.trf.2019.01.009
Ahvenniemi, H., Huovila, A., Pinto-Seppä, I., and Airaksinen, M. (2017). What are the differences between sustainable and smart cities? Cities 60, 234–245. doi: 10.1016/j.cities.2016.09.009
Alibaba (2020). City Brain Overview. Available online at: https://www.alibabacloud.com/et/city (accessed June 11, 2020).
Allam, Z., and Dhunny, Z. A. (2019). On big data, artificial intelligence and smart cities. Cities 89, 80–91. doi: 10.1016/j.cities.2019.01.032
Angelidou, M. (2015). Smart cities: a conjuncture of four forces. Cities 47, 95–106. doi: 10.1016/j.cities.2015.05.004
Awad, E., Dsouza, S., Kim, R., Schulz, J., Henrich, J., Shariff, A., et al. (2018). The moral machine experiment. Nature 563, 59–64. doi: 10.1038/s41586-018-0637-6
Barns, S. (2020). Platform Urbanism: Negotiating Platform Ecosystems in Connected Cities. Berlin: Springer.
Batty, M. (2018). Artificial intelligence and smart cities. Environ. Plann. B 15, 3–6. doi: 10.1177/2399808317751169
Bennett, R., Vijaygopal, R., and Kottasz, R. (2019). Attitudes towards autonomous vehicles among people with physical disabilities. Transp. Res. A Policy Pract. 127, 1–17. doi: 10.1016/j.tra.2019.07.002
Bibri, S. E., and Krogstie, J. (2017). Smart sustainable cities of the future: An extensive interdisciplinary literature review. Sust. Cities Soc. 31, 183–212. doi: 10.1016/j.scs.2017.02.016
Bina, O., Inch, A., and Pereira, L. (2020). Beyond techno-utopia and its discontents: on the role of utopianism and speculative fiction in shaping alternatives to the smart city imaginary. Futures 115:102475. doi: 10.1016/j.futures.2019.102475
Bissell, D. (2018). Automation interrupted: How autonomous vehicle accidents transform the material politics of automation. Political Geogr. 65, 57–66. doi: 10.1016/j.polgeo.2018.05.003
Bissell, D., and Del Casino, V. J. Jr. (2017). Whither labor geography and the rise of the robots? Soc. Cultur. Geogr. 18, 435–442. doi: 10.1080/14649365.2016.1273380
Bloomberg (2017). Sun, Sea and Robots: Saudi Arabia's Sci-Fi City in the Desert. Available online at: https://www.bloomberg.com/graphics/2017-neom-saudi-mega-city/ (accessed June 19, 2020).
Bonnefon, J. F., Shariff, A., and Rahwan, I. (2019). The trolley, the bull bar, and why engineers should care about the ethics of autonomous cars [point of view]. Proc. IEEE 107, 502–504. doi: 10.1109/JPROC.2019.2897447
Bulkeley, H., Marvin, S., Palgan, Y. V., McCormick, K., Breitfuss-Loidl, M., Mai, L., et al. (2019). Urban living laboratories: conducting the experimental city? Eur. Urban Reg. Stud. 26, 317–335. doi: 10.1177/0969776418787222
Caprotti, F. (2019). Spaces of visibility in the smart city: flagship urban spaces and the smart urban imaginary. Urban Stud. 56, 2465–2479. doi: 10.1177/0042098018798597
Caprotti, F., and Liu, D. (2020). Emerging platform urbanism in China: reconfigurations of data, citizenship and materialities. Techn. Forecast. Soc. Chang. 151:119690. doi: 10.1016/j.techfore.2019.06.016
Caragliu, A., Del Bo, C., and Nijkamp, P. (2011). Smart cities in Europe. J. Urban Techn. 18, 65–82. doi: 10.1080/10630732.2011.601117
Carter, O., Hohwy, J., Van Boxtel, J., Lamme, V., Block, N., Koch, C., et al. (2018). Conscious machines: defining questions. Science 359, 400–400. doi: 10.1126/science.aar4163
Cave, S., Dihal, K., and Dillon, S., (eds.). (2020). AI Narratives: A History of Imaginative Thinking About Intelligent Machines. Oxford: Oxford University Press.
Clifton, J., Glasmeier, A., and Gray, M. (2020). When machines think for us: the consequences for work and place. Cambridge J. Reg. Econ. Soc. 13, 3–23. doi: 10.1093/cjres/rsaa004
Colding, J., Colding, M., and Barthel, S. (2020). The smart city model: a new panacea for urban sustainability or unmanageable complexity? Environ. Plan. B Urban Anal. City Sci. 47, 179–187. doi: 10.1177/2399808318763164
Coletta, C., Evans, L., Heaphy, L., and Kitchin, R., (eds.). (2018). Creating Smart Cities. London: Routledge.
Cowley, R., Joss, S., and Dayot, Y. (2018). The smart city and its publics: insights from across six UK cities. Urban Res. Pract. 11, 53–77. doi: 10.1080/17535069.2017.1293150
Crandall, J. W., Oudah, M., Ishowo-Oloko, F., Abdallah, S., Bonnefon, J. F., Cebrian, M., et al. (2018). Cooperating with machines. Nat. Commun. 9:233. doi: 10.1038/s41467-017-02597-8
Crang, M., and Graham, S. (2007). Sentient cities ambient intelligence and the politics of urban space. Inf. Commun. Soc. 10, 789–817. doi: 10.1080/13691180701750991
Crot, L. (2013). Planning for sustainability in non-democratic polities: the case of Masdar City. Urban Stud. 50, 2809–2825. doi: 10.1177/0042098012474697
Cugurullo, F. (2013a). How to build a sandcastle: an analysis of the genesis and development of masdar city. J. Urban Techn. 20, 23–37. doi: 10.1080/10630732.2012.735105
Cugurullo, F. (2013b). The business of utopia: estidama and the road to the sustainable city. Utopian Stud. 24, 66–88. doi: 10.5325/utopianstudies.24.1.0066
Cugurullo, F. (2016). Urban eco-modernisation and the policy context of new eco-city projects: where Masdar City fails and why. Urban Stud. 53, 2417–2433. doi: 10.1177/0042098015588727
Cugurullo, F. (2018a). “The origin of the Smart City imaginary: from the dawn of modernity to the eclipse of reason,” in The Routledge Companion to Urban Imaginaries, eds C. Lindner and M. Meissner (London: Routledge), 113–124.
Cugurullo, F. (2018b). Exposing smart cities and eco-cities: Frankenstein urbanism and the sustainability challenges of the experimental city. Environ. Plan A 50, 73–92. doi: 10.1177/0308518X17738535
Cugurullo, F., Acheampong, R. A., Gueriau, M., and Dusparic, I. (2020). The transition to autonomous cars, the redesign of cities and the future of urban sustainability. Urban Geogr. 1–27. doi: 10.1080/02723638.2020.1746096
Cugurullo, F., and Ponzini, D. (2018). “The transnational smart city as urban eco-modernisation.” in Inside Smart Cities: Place, Politics and Urban Innovation, eds A. Karvonen. F. Cugurullo, and F. Caprotti (London: Routledge), 149–162.
Datta, A. (2018). The digital turn in postcolonial urbanism: Smart citizenship in the making of India's 100 smart cities. Trans. Inst. Br. Geogr. 43, 405–419. doi: 10.1111/tran.12225
Del Casino, V. J. Jr. (2016). Social geographies ii: robots. Prog. Hum. Geogr. 40, 846–855. doi: 10.1177/0309132515618807
Dodge, M., and Kitchin, R. (2007). The automatic management of drivers and driving spaces. Geoforum 38, 264–275. doi: 10.1016/j.geoforum.2006.08.004
Dodge, M., and Kitchin, R. (2009). Software, objects, and home space. Environ. Plann. A 41, 1344–1365. doi: 10.1068/a4138
Dowling, R., McGuirk, P., and Gillon, C. (2019). Strategic or piecemeal? Smart city initiatives in Sydney and Melbourne. Urban Policy Res. 37, 429–441. doi: 10.1080/08111146.2019.1674647
Duarte, F., and Ratti, C. (2018). The impact of autonomous vehicles on cities: a review. J. Urban Tech. 25, 3–18. doi: 10.1080/10630732.2018.1493883
Featherstone, M. (2004). Automobilities: an introduction. Theory Cult. Soc. 21, 1–24. doi: 10.1177/0263276404046058
Fernandez-Anez, V., Fernández-Güell, J. M., and Giffinger, R. (2017). Smart City implementation and discourses: an integrated conceptual model. the case of Vienna. Cities 78, 4–16. doi: 10.1016/j.cities.2017.12.004
Floridi, L., Cowls, J., Beltrametti, M., Chatila, R., Chazerand, P., Dignum, V., et al. (2018). AI4People—an ethical framework for a good AI society: opportunities, risks, principles, and recommendations. Minds Mach. 28, 689–707. doi: 10.1007/s11023-018-9482-5
Floridi, L., Cowls, J., King, T. C., and Taddeo, M. (2020). How to design AI for social good: seven essential factors. Sci. Eng. Ethics 26, 1771–1796. doi: 10.1007/s11948-020-00213-5
Fridman, L., Brown, D. E., Glazer, M., Angell, W., Dodd, S., Jenik, B., et al. (2019). MIT advanced vehicle technology study: large-scale naturalistic driving study of driver behavior and interaction with automation. IEEE Access 7, 102021–102038. doi: 10.1109/ACCESS.2019.2926040
Gandy, M. (2014). The Fabric of Space: Water, Modernity, and The Urban Imagination. Cambridge: MIT Press.
Goodall, N. J. (2014). Ethical decision making during automated vehicle crashes. Transp. Res. Rec. 2424, 58–65. doi: 10.3141/2424-07
Griffiths, S., and Sovacool, B. K. (2020). Rethinking the future low-carbon city: carbon neutrality, green design, and sustainability tensions in the making of Masdar City. Energy Res. Soc. Sci. 62:101368. doi: 10.1016/j.erss.2019.101368
Guériau, M., Cugurullo, F., Acheampong, R., and Dusparic, I. (2020). Shared autonomous mobility-on-demand: learning-based approach and its performance in the presence of traffic congestion. IEEE Intell. Transp. Syst. Mag.
Haarstad, H., and Wathne, M. W. (2019). Are smart city projects catalyzing urban energy sustainability? Energy Policy 129, 918–925. doi: 10.1016/j.enpol.2019.03.001
Hawkins, J., and Nurul Habib, K. (2019). Integrated models of land use and transportation for the autonomous vehicle revolution. Transp. Rev. 39, 66–83. doi: 10.1080/01441647.2018.1449033
Henderson, J. (2018). “Google buses and uber cars: the politics of tech mobility and the future of urban liveability,” in The Routledge Handbook on Spaces of Urban Politics, eds K. Ward, A. E. G. Jonas, B. Miller, and D. Wilson (London: Routledge), 439–450.
Heynen, N., Kaika, M., and Swyngedouw, E., (eds.). (2005). In The Nature of Cities. Urban Political Ecology and The Politics of Urban Metabolism. London: Routledge.
Hulse, L. M., Xie, H., and Galea, E. R. (2018). Perceptions of autonomous vehicles: relationships with road users, risk, gender and age. Safe. Sci. 102, 1–13. doi: 10.1016/j.ssci.2017.10.001
Ingrand, F., and Ghallab, M. (2017). Deliberation for autonomous robots: a survey. Artif. Intell. 247, 10–44. doi: 10.1016/j.artint.2014.11.003
Joss, S., Sengers, F., Schraven, D., Caprotti, F., and Dayot, Y. (2019). The smart city as global discourse: Storylines and critical junctures across 27 cities. J. Urban Technol. 26, 3–34. doi: 10.1080/10630732.2018.1558387
Kaika, M. (2017). “Don't call me Resilient Again!” The new urban agenda as immunology or what happens when communities refuse to be vaccinated with ‘smart cities' and indicators. Environ. Urban. 29, 89–102. doi: 10.1177/0956247816684763
Kanal, L. N., and Lemmer, J. F., (eds.). (2014). Uncertainty in Artificial Intelligence. Amsterdam: Elsevier.
Karvonen, A., Cugurullo, F., and Caprotti, F. (2018a). “Introduction: situating smart cities,” in Inside Smart Cities: Place, Politics and Urban Innovation, eds A. Karvonen, F. Cugurullo, and F. Caprotti (London: Routledge), 1–12.
Karvonen, A., Cugurullo, F., and Caprotti, F., (eds.). (2018b). Inside Smart Cities: Place, Politics and Urban Innovation. London: Routledge.
Kitchin, R. (2014). The real-time city? Big data and smart urbanism. GeoJ. 79, 1–14. doi: 10.1007/s10708-013-9516-8
Kitchin, R. (2020). Civil liberties or public health, or civil liberties and public health? Using surveillance technologies to tackle the spread of COVID-19. Space Polity 1–20. doi: 10.1080/13562576.2020.1770587
Korinek, A., and Stiglitz, J. E. (2017). Artificial intelligence and its implications for income distribution and unemployment (No. w24174). National Bureau of Economic Research. Available online at: https://www8.gsb.columbia.edu/faculty/jstiglitz/sites/jstiglitz/files/AI_labor.pdf
Leszczynski, A. (2020). Glitchy vignettes of platform urbanism. Environ. Plan. D 38, 189–208. doi: 10.1177/0263775819878721
Levesque, H. J. (2017). Common Sense, the Turing Test, and the Quest for Real AI: Reflections on Natural and Artificial Intelligence. Cambridge: MIT Press.
Lin, P. (2016). “Why ethics matters for autonomous cars,” in Autonomous Driving: Technical, Legal and Social Aspects, eds M. Maurer, J. C. Gerdes, B. Lenz, and H. Winner (Berlin: Springer), 69–85.
Luomi, M. (2009). Abu Dhabi's alternative-energy initiatives: seizing climate-change opportunities. Middle East Policy 16:102. doi: 10.1111/j.1475-4967.2009.00418.x
Machado Junior, C., Ribeiro, D. M. N. M., da Silva Pereira, R., and Bazanini, R. (2018). Do Brazilian cities want to become smart or sustainable? J. Clean. Produc. 199, 214–221. doi: 10.1016/j.jclepro.2018.07.072
Macrorie, R., Marvin, S., and While, A. (2020). Robotics and automation in the city: a research agenda. Urban Geogr. 1–21. doi: 10.1080/02723638.2019.1698868
Martin, C. J., Evans, J., and Karvonen, A. (2018). Smart and sustainable? Five tensions in the visions and practices of the smart-sustainable city in Europe and North America. Technol. Forecast. Soc. Change 133, 269–278. doi: 10.1016/j.techfore.2018.01.005
Marvin, S., Luque-Ayala, A., and McFarlane, C., (eds.). (2015). Smart Urbanism: Utopian Vision Or False Dawn? London: Routledge.
Mende, M., Scott, M. L., van Doorn, J., Grewal, D., and Shanks, I. (2019). Service robots rising: how humanoid robots influence service experiences and elicit compensatory consumer responses. J. Market. Res. 56, 535–556. doi: 10.1177/0022243718822827
Milakis, D., Van Arem, B., and Van Wee, B. (2017). Policy and society related implications of automated driving: a review of literature and directions for future research. J. Intell. Transp. Syst. 21, 324–348. doi: 10.1080/15472450.2017.1291351
Morley, J., Cowls, J., Taddeo, M., and Floridi, L. (2020). Ethical guidelines for COVID-19 tracing apps. Nature 582, 29–31. doi: 10.1038/d41586-020-01578-0
Mumford, L. (1961). The City in History: Its Origins, Its Transformations, and Its Prospects. New York, NY: Brace & World.
Navya (2020a). The Brain of the Autonoms. Available online at: https://navya.tech/en/intelligence-en/system/ (accessed June 19, 2020).
Navya (2020b). The Autonom Shuttle. Available online at: https://navya.tech/en/autonom-shuttle/ (accessed June 19, 2020).
Navya (2020c). Autonom Shuttle Brochure. Available onlin at: https://navya.tech/wp-content/uploads/documents/Brochure_Shuttle_EN.pdf (accessed June 19, 2020).
Neom (2020). Vision. Available online at: https://www.neom.com (accessed June 19, 2020).
Norman, B. (2018). Are autonomous cities our urban future? Nat Commun. 9:2111. doi: 10.1038/s41467-018-04505-0
O'Neil, C. (2016). Weapons of Math Destruction: How Big Data Increases Inequality and Threatens Democracy. London: Penguin.
Parks, D., and Rohracher, H. (2019). From sustainable to smart: re-branding or re-assembling urban energy infrastructure? Geoforum 100, 51–59. doi: 10.1016/j.geoforum.2019.02.012
Pearl, J. (2014). Probabilistic Reasoning in Intelligent Systems: Networks of Plausible Inference. Amsterdam: Elsevier.
Perng, S. Y., Kitchin, R., and Mac Donncha, D. (2018). Hackathons, entrepreneurial life and the making of smart cities. Geoforum 97, 189–197. doi: 10.1016/j.geoforum.2018.08.024
Picon, A. (2018). Urban infrastructure, imagination and politics: from the networked metropolis to the smart city. Int. J. Urban Region. Res. 42, 263–275. doi: 10.1111/1468-2427.12527
Pinna, F., Masala, F., and Garau, C. (2017). Urban policies and mobility trends in italian smart cities. Sustainability 9:494. doi: 10.3390/su9040494
Ponzini, D. (2011). Large scale development projects and star architecture in the absence of democratic politics: the case of Abu Dhabi, UAE. Cities 28, 251–259. doi: 10.1016/j.cities.2011.02.002
Rahwan, I., Cebrian, M., Obradovich, N., Bongard, J., Bonnefon, J. F., Breazeal, C., et al. (2019). Machine behaviour. Nature 568, 477–486. doi: 10.1038/s41586-019-1138-y
Russell, S. J., and Norvig, P. (2016). Artificial Intelligence: A Modern Approach. Harlow: Pearson Education Limited.
Salehi, H., and Burgueño, R. (2018). Emerging artificial intelligence methods in structural engineering. Eng. Struct. 171, 170–189. doi: 10.1016/j.engstruct.2018.05.084
Scoones, I., and Stirling, A. (2020). The Politics of Uncertainty: Challenges of Transformation. London: Routledge.
Shelton, T., Zook, M., and Wiig, A. (2015). The ‘actually existing smart city'. Cambridge J. Reg. Econ. Soc. 8, 13–25. doi: 10.1093/cjres/rsu026
Smigiel, C. (2019). Urban political strategies in times of crisis: a multiscalar perspective on smart cities in Italy. Eur. Urban Region. Stud. 26, 336–348. doi: 10.1177/0969776418792049
Sternberg, R. J. (2020). The Cambridge Handbook of Intelligence. Cambridge: Cambridge University Press.
Stilgoe, J. (2019). Who's Driving Innovation?: New Technologies and the Collaborative State. Berlin: Springer Nature.
Talebian, A., and Mishra, S. (2018). Predicting the adoption of connected autonomous vehicles: a new approach based on the theory of diffusion of innovations. Transp. Res. Part C Emerg. Technol. 95, 363–380. doi: 10.1016/j.trc.2018.06.005
Tiddi, I., Bastianelli, E., Daga, E., d'Aquin, M., and Motta, E. (2019). Robot–city interaction: mapping the research landscape—a survey of the interactions between robots and modern cities. Int. J. Soc. Robot. 12, 299–324.
Trencher, G. (2019). Towards the smart city 2.0: Empirical evidence of using smartness as a tool for tackling social challenges. Techn. Forecast. Soc. Chang. 142, 117–128. doi: 10.1016/j.techfore.2018.07.033
Turing, A. (1950). Computing machinery and intelligence. Mind 236, 433–460. doi: 10.1093/mind/LIX.236.433
Valdez, A. M., Cook, M., and Potter, S. (2018). Roadmaps to utopia: tales of the smart city. Urban Stud. 55, 3385–3403. doi: 10.1177/0042098017747857
van der Graaf, S., and Ballon, P. (2019). Navigating platform urbanism. Techn. Forecast. Soc. Chang. 142, 364–372. doi: 10.1016/j.techfore.2018.07.027
Vanolo, A. (2016). Is there anybody out there? The place and role of citizens in tomorrow's smart cities. Futures 82, 26–36. doi: 10.1016/j.futures.2016.05.010
Vinuesa, R., Azizpour, H., Leite, I., Balaam, M., Dignum, V., Domisch, S., et al. (2020). The role of artificial intelligence in achieving the sustainable development goals. Nat. Commun. 11:233. doi: 10.1038/s41467-019-14108-y
Warwick, K., and Shah, H. (2016). Can machines think? A report on turing test experiments at the royal society. J Exp. Theor. Artif. Intell. 28, 989–1007. doi: 10.1080/0952813X.2015.1055826
Wirtz, J., Patterson, P. G., Kunz, W. H., Gruber, T., Lu, V. N., Paluch, S., et al. (2018). Brave new world: service robots in the frontline. J. Serv. Manage. 29, 907–931. doi: 10.1108/JOSM-04-2018-0119
Wu, Y., Zhang, W., Shen, J., Mo, Z., and Peng, Y. (2018). Smart city with Chinese characteristics against the background of big data: Idea, action and risk. J. Clean. Produc. 173, 60–66. doi: 10.1016/j.jclepro.2017.01.047
Yigitcanlar, T., Desouza, K. C., Butler, L., and Roozkhosh, F. (2020). Contributions and risks of artificial intelligence (AI) in building smarter cities: Insights from a systematic review of the literature. Energies 13:1473. doi: 10.3390/en13061473
Zakharenko, R. (2016). Self-driving cars will change cities. Reg. Sci. Urban Econ. 61, 26–37. doi: 10.1016/j.regsciurbeco.2016.09.003
Keywords: smart city, artificial intelligence, autonomous city, governance, sustainability, autonomy
Citation: Cugurullo F (2020) Urban Artificial Intelligence: From Automation to Autonomy in the Smart City. Front. Sustain. Cities 2:38. doi: 10.3389/frsc.2020.00038
Received: 28 August 2019; Accepted: 24 June 2020;
Published: 30 July 2020.
Edited by:
Gregory Patrick Trencher, Tohoku University, JapanReviewed by:
Rob Kitchin, Maynooth University, IrelandSimon Marvin, University of Sheffield, United Kingdom
Copyright © 2020 Cugurullo. This is an open-access article distributed under the terms of the Creative Commons Attribution License (CC BY). The use, distribution or reproduction in other forums is permitted, provided the original author(s) and the copyright owner(s) are credited and that the original publication in this journal is cited, in accordance with accepted academic practice. No use, distribution or reproduction is permitted which does not comply with these terms.
*Correspondence: Federico Cugurullo, cugurulf@tcd.ie