Estimating the Impact of Occupants' Behaviour on Energy Consumption by Pls-SEM: A Case Study of Pakdel Residential Complex in Isfahan, IRAN
- 1Department of Process, Energy and Transport Engineering, Munster Technological University, Cork, Ireland
- 2Department of Construction, Shahid Beheshti University, Tehran, Iran
The importance of saving energy in the current decade has drawn more attention to optimising energy consumption factors. One of the influential and well-known factors affecting energy consumption is the occupants' behaviours (OBs). Reviewing this factor is a requirement to assess buildings, particularly the residential sector, as the majority target of the building industry. In this study, occupants' energy behaviours in Shahid Pakdel residential complex in Isfahan, Iran, are studied based on Annex 53 questionnaire and building performance simulation (BPS). The main objective of this study is to identify the impact of apartment improvement and OBs, including windows opening, curtain controlling behaviour, and turning on heating equipment in the cold season on energy consumption. Due to the invisible effect of some behaviours, especially for gas consumption (GC) and electricity consumption (EC), structural equation modelling (SEM) is applied to evaluate the impacts of OBs. This case study showed that the most influential behaviour factor is related to the improvement sector with a 41.7% share of EC. Moreover, the most negligible influential factor is associated with windows opening with 21.6% of the EC. Regarding GC, the most and the least determining behaviour factor were attributed to apartment improvement and curtain controlling behaviour with an effective rate of 64.5 and 5%, respectively. This result showed the high impact of apartment improvement on reducing GC and EC. The overall effect of behaviour on GC and EC was 46 and 44%, respectively, measured by the partial least squares (PLS) regression and R2 score.
Introduction
It is estimated that by 2050, the energy demand will be increased to twice as much as the current level (EIA, 2020). Therefore, energy will become an essential part of economic, political, social, and environmental matters. The factors that can affect the energy consumption are climate, building shell, type of facilities, operation and maintenance of the building, activity and OBs, and indoor environmental quality (Al-mumin et al., 2003). According to the international energy agency Annex 53, one of the influential factors for building energy consumption is OBs (Yoshino et al., 2017). On the other hand, in the last two decades, the residential sector has been the largest consumer of energy with a share of more than 30% of energy consumption (EIA, 2015). At the same time, a household's fuel consumption leads to almost 30% of CO2 emissions and 6% of total emitted contamination (Delavar and Sahebi, 2020). According to published statistics from the Iran Fuel Conservation Company, 40% of energy resources are consumed in the building sector.
Meanwhile, energy consumption in Iran is 2.5 times higher than the global average. Iran is the ninth largest energy producer, the tenth-largest consumer of energy, and the eighth largest producer of greenhouse gases across the globe (EIA, 2019). As a result, focusing on the residential sector to improve energy efficiency and reduce energy consumption will gain remarkable environmental advantages (Ürge-Vorsatz et al., 2007; Belussi et al., 2019).
However, differences between predicted and actual building performance have been assumed as OBs. The connection between OBs and energy consumption was assigned to the occupants' environmental comfort. Thus, the three primary categories were recognised as environmentally-related, time-related, and random. While physical facets connected to building characteristics and location were considered environmentally-related variables, the routines of the occupants were described as time-related variables (Balvedi et al., 2018). The study is shown that neglecting the assumptions related to the behaviour such as occupant characteristic-related factors, including ‘age of householders’, ‘household size’, ‘income’, ‘education level’, ‘type of occupancy’, and ‘length of residency’ and simplification in energy consumption could explain 10.70% of the variance in electricity consumption. As a result, the ‘type of occupancy’ had the most significant impact, followed by ‘education level’, ‘length of residency’, ‘household size’, and ‘income’ (Xu et al., 2020). A review study highlighted that OB assumptions exist in building energy regulation and often cause unsuitable building envelopes, technologies, system design, and operation. A better understanding of actual occupancy patterns and behaviours would fill the gap between the predicted amount of consumption and the actual one (Hu et al., 2020). Therefore, recognising the factors that involve OBs can be considered essential and can reduce the measurement error between the consumption predicted by the simulation and actual consumption (Hoes et al., 2009; Hu et al., 2020). Despite numerous energy simulation efforts and the prioritising methods for reducing consumption, the practical impact of behaviour on energy consumption remains unknown. As a result, most current studies to date are inadequate in simulating and analysing data (Jami et al., 2021).
Numerous research and studies have been conducted on diverse aspects of energy modelling in the residential sector, and its significance in policy-making decisions (Barkhordar and Saboohi, 2014; Gabrielli and Ruggeri, 2019) can be noted. The modelling paths implemented in research can be classified into three primary types: 1-Optimisation modelling, 2-Simulation, and 3-Stochastic modelling (Ahmadi et al., 2020). The paper presented ten questions and answers related to occupant energy behaviour research, applications, and methodologies to increase energy efficiency and reduce energy use (Hong et al., 2017). According to a study by Xu et al. (2020), the energy consumption in residential buildings can be substantially affected by occupant characteristic-related factors, including age, size, income, education level, type of occupancy, and length of residency of householders. Besides, 10.70% of the variance in energy consumption was attributed to the type of occupancy (the most significant impact), education level, length of residency, household size, and income. The analysis method provides an effective tool for quantitatively assessing the impact of different occupant characteristics on the modelling of occupant behaviour and simulation of building energy (Xu et al., 2020). A study was conducted in 96 apartment blocks in Seoul, Korea, to represent differences in actual energy use in apartments. In that study, a model was developed considering the effect of occupant behaviour on energy consumption in the heating and electricity sectors, using the regression method. Gaussian Process Classification was applied to modify random occupant behaviours corresponding to the probability of energy consumption. The result showed that occupants' general heating controls (25% deviation) were between 3 and 8 h, and the temperatures setpoint was between 17 and 20°C. The operating hours of electric appliances and lighting were also approximated with the probabilities (Jang and Kang, 2016).
Research is carried out in which the effect of behaviour was measured by a monitoring system that compared the actual and the expected energy consumption of the residential buildings in Germany. For each refurbishment strategy (with values up to 287% based on measured savings), the energy performance gap was evaluated: on average, the energy performance gap of the entire field test changed to 117% in 2011, 107% in 2012, 41% in 2013, and 60% in 2014 (Cal, 2016). A detailed post-occupancy evaluation of a UK EcoHomes with the highest rating of the predecessor to the Code for Sustainable Homes investigated the energy performance, comfort, and occupant satisfaction. The study was implemented to distinguish consumption patterns using surveys and interviews. Results demonstrated that energy-related occupant behaviours among dwellings account for 51, 37, and 11% of the variance in heat, electricity, and water consumption, respectively (Gill et al., 2015).
Another study (Schweiker and Shukuya, 2010) compared three cases of building envelope improvements using energy analysis to modify the occupant's behavioural pattern in a dormitory building. The assumptions of occupant behaviour were set up based on the field measurements in steady-state conditions. It was found that the potential of occupant behavioural changes in reduced energy consumption was more affected by the outdoor temperature compared to building envelope improvements. The influence of occupant behaviour on energy consumption was more than 90% in regions with a moderate climate, and a slight difference was found between indoor and outdoor temperatures. Furthermore, depending on the outside conditions, the combination of both measures results in a reduction ranging from 76 to 95%.
D'Oca et al. (2014) examined the energy management system in 31 homes in Italy. The results showed that the incentive communication strategy (competition between similar households) effectively reduced energy consumption. The average energy savings in these households were affected by incentive schemes by up to 18% (D'Oca et al., 2014). The four types of occupant control measures, including opening and closing windows, canopies, the use of light and heat, and measuring the factors influencing OBs, were analysed. The outside temperature often influenced the use of windows and heating systems. Artificial lighting was also strongly related to the available sunlight, the intensity of the lighting, and the outdoor temperature (Andersen, 2009).
While most studies, as shown in Table 1, evaluated the impact of occupant behaviour on energy performance utilising different integrated methods. The current study aimed to present a method to evaluate the influence of OBs on building energy consumption in the midrise residential sector. Therefore, this study is implemented by surveys and energy performance simulations of similar apartments to investigate the energy performance gap, the differences between actual behaviour models with pre-assumed ASHRAE, and the effectiveness of improvement strategies on the energy performance and OBs. The current study aims to identify the number of different aspects of occupant behaviour and building improvement on energy performance in the residential sector using SEM. The novelty of this method is the application of SEM to mitigate the uncertainty related to predictions of occupant energy behaviours. Considering the share of residential buildings for most energy consumption and the complexity of comfort conditions expectation in the house, attention to energy saving is more critical in this section. Therefore, the importance of conducting this study concerns narrowing down the difference between the actual and predicted consumption by accurate estimation of OBs. To this end, based on previous studies, the research methodology was first defined. Following that, the results of the Pls-SEM model analysis were shown, and the discussions, limitations, and future research directions are finally presented in detail.
Materials and Methods
The methodology, as shown in Figure 1, includes two main stages. The OBs are extracted using questionnaire data from the selected households in the first stage. These extracted OBs are integrated via a building simulation model in DesignBuilder v5.5.2.007. In order to validate the model, the EC and GC predictions in the simulation are compared with the yearly and monthly bills of selected households. In the second stage, a building statistical model is developed. Due to latent variables in OBs, measured by the observable factors in the questionnaire, a structural equation model (SEM) based on the partial least squares method is developed (Kroonenberg and Lohmoller, 1990). This SEM measured the significant path between invisible factors in OBs. In the following sections, a detailed explanation of each step is provided.
Investigated Buildings
The building database referred to 42 typical apartments with the same built age, constructed in 1960 in Esfahan, Iran (see Figure 2). The campus has 13 building blocks with a slight south-east orientation of 10° (see Figures 2, 3). In terms of weather conditions, Esfahan has mild spring and autumn, hot and dry summer with average temperatures between 30 and 40°C, and cold and dry winter. The location of the studied buildings based on Köppen climate classification is in the (BWh) category.
The blocks have typical plans. Each floor plan has two apartments, circulation spaces, and a shared staircase located at the centre part. The bedrooms are located on the south, and the living rooms and kitchens are located on the north side (see Figure 4). The buildings were characterised by high-energy demand with low insulation of the building envelope, a single glass window, and low-efficiency equipment. The heating/cooling system includes gas heaters to meet the space heating and domestic hot water demand and evaporative coolers (electrical) to meet the space cooling need. The electricity and gas utilities were imported from the national grid. It should be noted that 20 apartments of this model have been improved in the 2000s by replacing the gas heaters with radiators, changing flooring material, renovating evaporative coolers, and replacing regular windows with double-glazed ones (see Table 2).
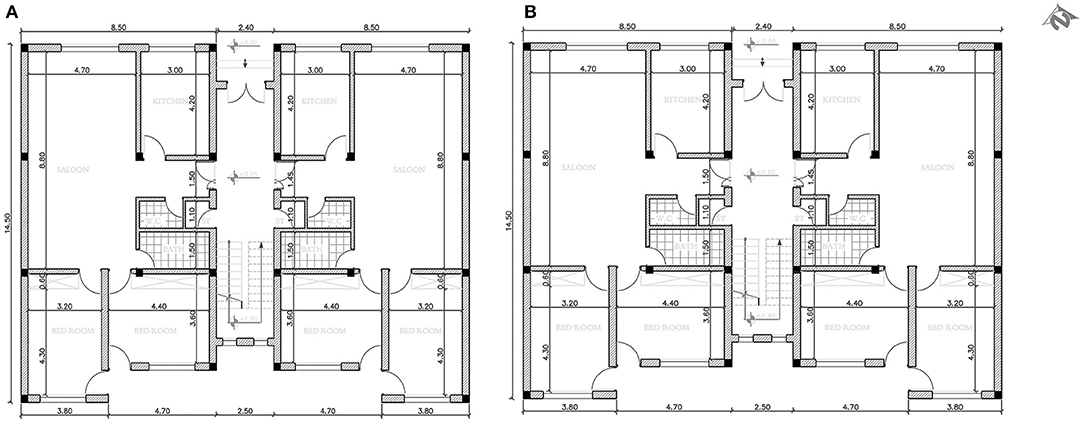
Figure 4. Typical floor plans of the blocks; left to right: (A) Ground floor plan. (B) First and second-floor plan.
Field Study
The current study uses the questionnaire method to evaluate the OBs from different aspects that affect the residential building's energy consumption. The research questionnaire was compiled based on Annex 53 (methods of evaluation and comprehensive analysis of building energy consumption) concerning ethical and cultural principles and facilities of residential buildings in Iran. Several items were presented on a reversed scale to minimise the potential effects of respondent inertia. Furthermore, the order of all statements in the questionnaire was randomised. A pilot questionnaire was then distributed to ensure validity and show the ambiguity and accuracy of the respondents' perceptions. After minor changes, it was distributed to the occupants. The final questionnaire included three sections: 1) Specifications of building occupants, 2) Behaviour of using heating/cooling system, 3) opening the windows (OPWIN) and curtain controlling behaviour (CURB). The study participants perform university employee jobs. In total, 40 valid responses were received from the selected 42 apartments.
As shown in Table 3, statistical parameters, i.e., min, max, median, mean and standard deviation, are calculated for questions with a ratio scale. In some questions, the mean was not calculated because of their ordinal scale, and instead of the standard deviation, entropy was considered.
Simulation and Validation
The case studies were simulated (see Figure 5) by DesignBuilder v5.5.2.007 as an EnergyPlus based software tool. Then the model is calibrated utilising a validated numerical model by actual energy data and user surveys. Occupancy patterns of using lighting, heating, cooling systems, and window opening were implemented based on the surveys to increase the accuracy of energy in the simulation model. In order to validate the simulation model, the simulated and actual performances were compared based on the utility data of GC and EC.
Building Statistical Models
The GC and EC are analysed separately by simulation. Then, the actual consumption was added to the previous data by accessing the gas and electricity bills of each house. Statistical tests performed in SPSS v.23.0 software measured the difference between actual and simulated consumption data and concluded that the data for all seasons except for summer was significantly different.
Each of the behaviours was examined with several different questions. The impossibility of asking about each behaviour directly from the occupants made it necessary to use confirmatory factor analysis and SEM. SEM is one of the main methods of analysing complex and multivariate data structures whose main feature is the simultaneous analysis of several independent and dependent factors (Geladi and Kowalski, 1986). The approach used in this study to obtain relationships between factors is the PLS, the second generation of SEM and aims to predict (Hair et al., 2011). The first generation is the Covariance-Based Structural Equation Modelling (CBSEM) method, which reduces the difference between the theoretical model covariance matrix and the fulfilled values' covariance matrix placement (Leguina, 2015). The selected approach, called PLS, was chosen due to the small sample size and the lack of dependence of this method on the data normality. The analyses were implemented using smart PLS v. 2.0 and bootstrapping algorithms to obtain reliability and validity indices.
Sample Size and Hypothesis
There is a method to estimate the minimum sample size in Pls-SEM. The minimum sample size of this research model was measured by the most common form, i.e., the 10-times rule. Due to this study having a small sample size, this rule is assumed to obtain the smallest number of samples required for a research model. It is calculated by multiplying the number of internal or external links of an invisible variable (whichever was larger) by 10 (Peng and Lai, 2012; Leguina, 2015). In this research, the most frequent connections for a latent variable were 4, which has led to a sample number of 40. The hypotheses of the study are shown in Table 4. Considering that the total number of studied apartments was 42, to build a model with validity and generalizability to the whole residential complex and obtain reliable results, all 42 apartments were examined in this study. Totally 40 valid responses were received.
Structural Equation Modelling
In the SEM, two models are considered: 1) Measurement model, in which links between observed variable and latent factor is measured, 2) Structural model, in which links among latent factors are measured.
Before constructing the model, attention has been put into validating the simulated EC and GC with in-field data. Since the data does not follow a normal distribution, simulated samples and actual consumption is compared based on the Wilcoxon test. Both in GC and EC during spring, autumn, and winter, there was a significant difference between simulated and actual consumption at a significant level above 99%: sig 0.000. There was no difference between simulated and actual consumption in summer (p > 0.05), so this factor was eliminated from the research model. Also, GC in summer is deleted since there was no GC for cooling in summer, and it was just for cooking.
Structural equations with the PLS approach measured research structures (OBs) and their impact on energy consumption. The software SMART PLS V.3.3.2 is used, and the indicators of validity, reliability, and Path coefficients were obtained from the outputs of this software. Each behaviour was considered a factor measured by some questions. The descriptive and statistical inference to obtain a specific pattern of behaviour and its effects on energy consumption was studied by observable and measurable factors within the questionnaire (see Table 5). Six latent factors on behaviours are as follows:
• Heating Behaviour in the cold weather (HB).
• Curtain controlling Behaviour (CURB).
• Apartment Improvement (AI).
• Gas Consumption (GC).
• Electricity Consumption (EC).
• Opening the Windows (OPWIN).
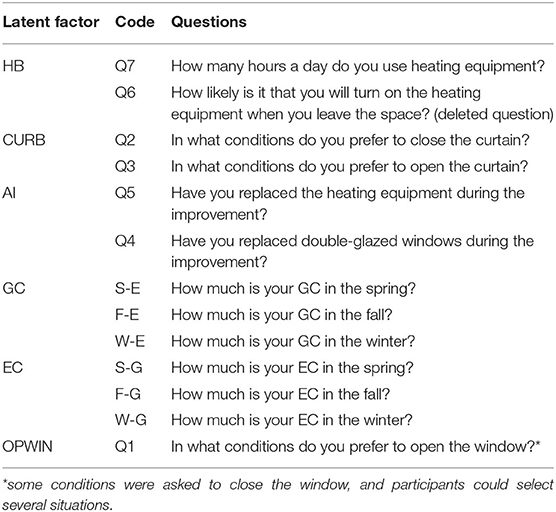
Table 5. Questions table with their latent factor and factor loading measured by running PLS algorithm.
Furthermore, based on past research, brainstorming has been carried out to examine the correlation and determine whether factors were constructive or reflective. It was found that the questions related to each factor were highly intertwined. The factors were identified as reflective (see Figure 6). The factor loading of one question (Q6) was deleted because it was less than 0.4 in the initial model (Hulland, 1999).
Results
Measurement Model
Measurement model or confirmatory factor analysis (CFA) means to look into the association between the variables (latent and observed). For example, the measurement model specifies relationships between latent constructs (e.g., AI) and their observed variable (e.g., Q4 and Q5). The measurement model must hold before testing the hypothesised relationships among the constructs of the model (Anderson and Gerbing, 1988; Cheng, 2001).
As shown in Table 6, factor loadings were obtained with the PLS algorithm and path weighting setting (Var = l and Mean = 0, Maximum iteration 300). By running the Bootstrap, algorithm t-statistics were calculated. According to the t-distribution table for p < 0.05, t-statistics must be greater than 2.022 for significance.
Composite Reliability, Convergent Validity (AVE), Discriminant Validity, and Quality of the Reflective Measurement Model (CV com)
Due to the insensitivity of Pls-SEM to the sample size and the possibility of using non-normal data, this method has been used to obtain high validity results. Composite reliability, convergent validity, discriminant validity, and the quality of the reflective measurement model have been used to measure the validity and reliability of measurement models in this study. If the validity and reliability index of the measurement model is accepted, it can be declared that the results obtained from this model can be reliable and accurate.
Cronbach's alpha reliability is assumed to have the same weights in the measurement model. However, in the composite reliability index, factor loadings are used when calculating the same priority over the Cronbach's alpha method. As proposed by Fornell and Larkers, this criterion was calculated, and it should be above 0.7 (Fornell and Larcker, 1981).
Convergent validity (CV) and discriminant validity were used to assess validity, which is part of construct validity. Convergent validity describes the degree to which the scale of observable factors sincerely represents the latent factors (Kurian, 2014). The Average Variance (AVE) was implemented to establish convergent validity. This index was introduced to estimate inner model validity. The minimum size of the index was considered 0.5 (Fornell and Larcker, 1981).
Discriminant validity means the factor loadings of visible variables in each reflective measurement model should be more than visible variables in other measurement models (Gefen and Straub, 2005). This difference between factor loadings was more than 0.1, shown in Table 6 (Refer to Supplementary Material). The BF command should be positive to test the quality of the Reflective Measurement Model (see Table 7).
Structural Equation Modelling
After evaluating the outer model, the SEM is operated. The SEM examines the relationships between latent variables and their significance. First, the PLS algorithm was implemented, and the path coefficients were determined. Then, the bootstrap algorithm was run to check the significance (p.values <0.05). The results of path coefficients and the significance of each behaviour on EC or GC are shown in Table 8.
The R2 coefficient is used to evaluate endogenous latent factors. The index indicates the percentage of endogenous variance factors exerted by the exogenous factors 0.75, 0.50, 0.25 (Henseler et al., 2009; Hair et al., 2011; Strong, medium, and weak). As demonstrated in Table 9, the R2 value for GC and EC was 46 and 44%, respectively. This value implies that 46% of the variance of the GC and 44% of EC can be predicted by OBs, including HB, OPWIN, AI aspects, and CURB.
To evaluate the quality of the structural model, Q2, the Stone Cal Sealer index, was used. It is the most well-known criterion for measuring the quality, and it was measured by running blindfolding in SMART PLS (V 3.3.2) and CV red table. Q2 values, above zero, indicating that the observed values were well reconstructed, and the model had the predictive capability (see Table 9).
Model Fit Test
Unlike the covariance-based method, the variance-based method does not have a global index for measuring GOF. Henseler and Sarstedt (Henseler and Sarstedt, 2013) reported that GOF was suggested by Tenenhaus et al., does not estimate an accurate fit measure and should not be used. However, it may be estimated good result for PLS multi- group when the Pl-SEM results of different groups were compared. In this study, this index was calculated and shown in Table 10 (Tenenhaus et al., 2004).
As shown in Table 10, the model fit index in this study was evaluated by three indexes. The other two indices calculated by the software include NFI and SRMR. NFI (Normed Fit Index) was introduced by Bentler and Bonett (1980) and for which a value between 0 and 1 has been set. Recent research shows that an NFI above 0.95 (Hu and Bentler, 1999) can indicate a good model fit. The main drawback of this index is its sensitivity to the sample size, and for samples, less than 200 cannot be a useful model fit index (Mulaik et al., 1989; Bentler, 1990) SRMR (Standardised Root Mean Square Residual) is another index used (Henseler et al., 2014). The SRMR is a model fit index for Pls-SEM that can avoid model misspecification. The SRMR lower than 0.10 or 0.08 were considered a good fit.
Finally, Cohen, defined as the criterion for the size of the effect, was used to determine the intensity of the relationship between latent variables. Through this criterion, the value of the effect of the exogenous variable to endogenous variable was estimated. Each behaviour's effect size on energy consumption is presented in Table 11 (p < 0.05).
Discussion
This paper highlights the role of OBs in electricity and gas consumption utilising a Pls-SEM-based approach. A combined method is used; collecting information based on Annex 53 questionnaire, energy simulation, and statistical approach to identify the effect of each behaviour. The authors selected four OBs factors, AI, CURB, OPWIN, and HB. The main findings show a significant impact of OBs in explaining the EC and GC up to 44 and 46%, respectively. There were some limitations in this study. Firstly, the questionnaire survey was conducted in a short period and was not repeated. Long-term monitoring of occupancy instead of one-time off surveys is necessary to obtain more precise OBs analysis. Another limitation of the current study is that OBs are based on occupant declaration rather than observations, which may affect the accuracy of the data. Finally, the sample of studied buildings is small, with the same building construction inhabited occupants with similar cultures and jobs.
Among all selected OBs in this study, AI and OPWIN had the most (41.7%) and the least (21.6%) significant effect on EC, respectively. Therefore, the result showed that the occupants in the improved building were much more satisfied with indoor environment conditions and used heating, cooling, lighting systems less than other buildings; this result is consistent with previous studies (Jones et al., 2017). The current study highlights the importance of considering the accurate OBs in building simulation studies and particularly retrofitting buildings strategy as the most influential factor on electricity consumption by retrofit planners, policymakers, and standards.
Moreover, descriptive analysis of survey and simulation results showed HB had a significant positive effect on GC and had negligible EC usage. This effect appeared due to the high EC arising from OBs in excessive heating systems used for 16–24 h per day. Besides, the occupants prefer to wear fewer clothes and not turn off the heating system when they leave home. Regarding energy subsidiary and relatively low-cost energy in Iran, this study showed that occupants are unconcerned about using energy so educating occupants to change their behaviours of using HB seems more practical to reduce energy consumption which is consistent with previous studies (Jami et al., 2021).
On the other hand, CURB had a significant and inverse effect on EC considering the effect of different CURB, whereas it did not impact GC. Therefore, the more the curtains were controlled, the less EC was used. More considerate about CURB, occupants had sufficient daylighting and used less artificial lighting during the day. Regarding GC, AI, and CURB had the most 64.5% and the least 5% significant effect. AI had a positive and negative impact on EC and GC, respectively, based on the path coefficients. This impact is caused by significant improvement strategies for replacing gas heating equipment with a radiator (electric one). Also, double-glazed windows were another improvement strategy that reduced energy waste and, consequently, GC.
In the improved building, OPWIN significantly increased EC affected by the misuse of electric heating equipment. Due to the replaced windows having lower U-values and infiltration rates, occupants open windows more frequently while heating systems are operating, causing an increase in energy consumption. In contrast, this behaviour did not significantly affect GC. More research needs to be carried out to realise the main reasons behind the insignificant effect on GC. The results are consistent with previous studies indicating that leaving windows open for extended periods reduces the effectiveness of increasing window layers (Wei et al., 2017).
Due to different OBs patterns, simulation results in the same buildings and locations may differ. Decision-makers can reduce energy consumption by choosing the most effective OBs that benefit from current OB patterns. Energy simulations are used during the design of new buildings to inform decision-makers about the variation and uncertainty of OBs. Furthermore, this study recommends that although AI significantly reduces energy consumption, it is preferable to emphasise occupant awareness and education about energy-saving behaviours and the critical roles of occupants in the success of an energy retrofit project. Raising occupants' responsibility and energy awareness through educational methods could be effective and practical (Bull et al., 2017). Furthermore, motivating programs such as displaying real-time consumption data to occupants could be critical in changing wasteful energy behaviours. Obtaining immediate energy feedback from smart devices could be useful (Emeakaroha et al., 2012).
This study highlighted the significant role of OBs in residential buildings energy consumption in Iran. In addition, the potential of reducing energy consumption by AI actions (e.g., double-glazing and using high-performance systems) and raising awareness of occupants about the impact of OBs patterns on optimising energy consumption. Therefore, this study recommends that the policymakers omit energy subsidisation to make AI actions logical financially and provide an incentive plan to encourage occupants to choose energy savings OBs patterns.
Conclusion
The necessity of reducing energy consumption in the residential sector has prompted researchers to look into the impact of buildings' physical characteristics and OBs on energy consumption. Therefore, increasing the accuracy of understanding and predicting OB is significant regarding the arising impacts on the building energy performance.
This study aimed to answer the question, ‘To what extent can OBs patterns and AI strategies affect energy consumption?’ in a residential complex using an integrated method. The study used field measurements, questionnaire surveys, and simulation to assess the effect of observable OBs factors and Pls-SEM model analysis to assess latent OBs factors. In addition, this study aimed to reduce and optimise the gaps between simulated energy performance and actual energy consumption of buildings. The main findings, as well as future research, are summarised as follows:
• The estimations demonstrated that 46% of the variance of the GC and 44% of EC are affected by OBs.
• Among all the factors influencing GC and EC, AI and OPWIN had the most 41.7% and the least 21.6% significant effect on EC, respectively.
• The Pls-SEM method was developed to accurately predict the effect of OBs on energy consumption by taking into account invisible factors with a statistical model.
• To narrow down the gap between the actual and predicted consumption and optimise the energy performance of buildings, it is highly required to estimate the influence of OBs on building energy performance.
• Encouraging occupants through education and policies could play an essential role in changing OBs patterns to optimise energy consumption.
• Further research should assess the effect of OBs on energy consumption in other climates, different cultures, large scale samples, and other building types (i.e., offices, educational).
Data Availability Statement
The raw data supporting the conclusions of this article will be made available by the authors, without undue reservation.
Ethics Statement
Ethical review and approval was not required for the study on human participants in accordance with the local legislation and institutional requirements. Written informed consent for participation was not required for this study in accordance with the national legislation and the institutional requirements.
Author Contributions
ET: conceptualisation, methodology, software, investigation, and writing–original draft. AN: software, investigation, and formal analysis. ZZ and MT: conceptualisation and supervision. MH: editing and visualisation. All authors contributed to the article and approved the submitted version.
Conflict of Interest
The authors declare that the research was conducted in the absence of any commercial or financial relationships that could be construed as a potential conflict of interest.
Publisher's Note
All claims expressed in this article are solely those of the authors and do not necessarily represent those of their affiliated organizations, or those of the publisher, the editors and the reviewers. Any product that may be evaluated in this article, or claim that may be made by its manufacturer, is not guaranteed or endorsed by the publisher.
Acknowledgments
This article is based on the first author's master thesis, entitled Presenting the energy consumption model of middle-rise residential buildings in Isfahan. The authors thank the Professors and staff dormitory of Isfahan University and Gas Company of Isfahan-Region 5 for their assistance and practical support in gathering surveys and energy consumptions of buildings.
Supplementary Material
The Supplementary Material for this article can be found online at: https://www.frontiersin.org/articles/10.3389/frsc.2022.700090/full#supplementary-material
Abbreviations
sHB, Heating Behaviour in the cold weather; CURB, Curtain controlling Behaviour; AI, Apartment Improvement; OPWIN, Opening the Windows.
References
Ahmadi, S., Fakehi, A. H., Vakili, A., and Moeini-Aghtaie, M. (2020). An optimization model for the long-term energy planning based on useful energy, economic and environmental pollution reduction in residential sector: a case of Iran. J. Build. Eng 30, 101–247. doi: 10.1016/j.jobe.2020.101247
Al-mumin, A., Khattab, O., and Sridhar, G. (2003). Occupants' behavior and activity patterns influencing the energy consumption in the Kuwaiti residences. Energy Build. 35, 549–559. doi: 10.1016/S0378-7788(02)00167-6
Andersen, R. V., et al. (2009). Survey of occupant behaviour and control of indoor environment in Danish dwellings. Energy Build. 41, 11–16. doi: 10.1016/j.enbuild.2008.07.004
Anderson, J. C., and Gerbing, D. W. (1988). Structural equation modeling in practice: a review and recommended two-step approach. Psychol. Bull. 103, 411–423. doi: 10.1037/0033-2909.103.3.411
Balvedi, B. F., Ghisi, E., and Lamberts, R. (2018). A review of occupant behaviour in residential buildings. Energy Build. 174, 495–505. doi: 10.1016/j.enbuild.2018.06.049
Barkhordar, Z. A., and Saboohi, Y. (2014). Modelling useful energy demand system as derived from basic needs in the household sector. Energy Efficiency 7, 903–921. doi: 10.1007/s12053-014-9257-7
Belussi, L., Barozzi, B., Bellazzi, A., Danza, L., Devitofrancesco, A., Fanciulli, C., et al. (2019). A review of performance of zero energy buildings and energy efficiency solutions. J. Build. Eng. 25, 100772. doi: 10.1016/j.jobe.2019.100772
Bentler, P. M. (1990). Comparative fit indexes in structural models. Psychol. Bull. 107, 238–246. doi: 10.1037/0033-2909.107.2.238
Bentler, P. M., and Bonett, D. G. (1980). Significance tests and goodness of fit in the analysis of covariance structures. Psychol. Bull. 88, 588–606. doi: 10.1037/0033-2909.88.3.588
Bull, R., Laskari, M., Jennings, N., and Romanowicz, J. (2017). “Switching off? challenges in engaging students in energy efficiency. Findings from an eu wide energy saving project,” in Proceedings of eceee 2017 Summer Study: Consumption, Efficiency & Limits, Belambra Les Criques, Toulon/Hyères, France, 29 May-3 June 2017 (Stockholm: European Council for an Energy Efficient Economy), 1997–2002.
Cal, A. D. (2016). Energy performance gap in refurbished German dwellings: lesson learned from a field test. Energy Build. 127, 1146–1158. doi: 10.1016/j.enbuild.2016.05.020
Cheng, E. W. (2001). SEM being more effective than multiple regression in parsimonious model testing for management development research. J. Manag. Dev. 20, 650–667. doi: 10.1108/02621710110400564
Delavar, H., and Sahebi, H. (2020). A sustainable mathematical model for design of net zero energy buildings. Heliyon 6, e03190. doi: 10.1016/j.heliyon.2020.e03190
D'Oca, S., Corgnati, S. P., and Buso, T. (2014). Smart meters and energy savings in Italy: determining the effectiveness of persuasive communication in dwellings. Energy Res. Soc. Sci. 3, 131–142. doi: 10.1016/j.erss.2014.07.015
EIA (2020). Annual Energy Outlook 2020 With Projections to 2050. International Energy Agency. Available online at: https://www.eia.gov/aeo.
EIA. (2019). Country Analysis Executive Summary: Iran. New York, NY: US Energy Information Administration.
Emeakaroha, A., Ang, C., and Yan, Y. (2012). Challenges in improving energy efficiency in a university campus through the application of persuasive technology and smart sensors. Challenges 3, 290–318. doi: 10.3390/challe3020290
Fornell, C., and Larcker, D. F. (1981). Evaluating structural equation models with unobservable variables and measurement error. J. Market. Res. 18, 39–50. doi: 10.1177/002224378101800313
Gabrielli, L., and Ruggeri, A. (2019). “Developing a model for energy retrofit in large building portfolios: energy assessment, optimization and uncertainty,” in 26th Annual European Real Estate Society Conference. ERES: Conference. Cergy-Pontoise: Europena Real Estate Soceity.
Gefen, D., and Straub, D. (2005). “A practical guide to factorial validity using PLSGraph: tutorial and annotated example,” in Communications of the Association for Information Systems, Vol 16, 91–109.
Geladi, P., and Kowalski, B. R. (1986). Partial least-squares regression: a tutorial. Anal. Chim. Acta 185, 1–17. doi: 10.1016/0003-2670(86)80028-9
Gill, Z. M., Tierney, M. J., Pegg, I. M., Allan, N., Gill, Z. M., Tierney, M. J., et al. (2015). to actual performance Low-energy dwellings : the contribution of behaviours to actual performance. Build. Res. Inf. 38, 37–41. doi: 10.1080/09613218.2010.505371
Hair, J. F., Ringle, C. M., and Sarstedt, M. (2011). PLS-SEM: indeed a silver bullet. J. Market. Theory Pract. 19, 139–152. doi: 10.2753/MTP1069-6679190202
Henseler, J., Ringle, C. M., and Sarstedt, M. (2014). A new criterion for assessing discriminant validity in variance-based structural equation modeling. J. Acad. Market. Sci. 43, 115–135. doi: 10.1007/s11747-014-0403-8
Henseler, J., Ringle, C. M., and Sinkovics, R. R. (2009). The use of partial least squares path modeling in international marketing. Adv. Int. Market. 20, 277–319. doi: 10.1108/S1474-7979(2009)0000020014
Henseler, J., and Sarstedt, M. (2013). Goodness-of-fit indices for partial least squares path modeling. Comput. Stat. 28, 565–580. doi: 10.1007/s00180-012-0317-1
Hoes, P., Hensen, J. L. M., Loomans, M. G. L. C., de Vries, B., and Bourgeois, D. (2009). User behavior in whole building simulation. Energy Build. 41, 295–302. doi: 10.1016/j.enbuild.2008.09.008
Hong, T., Yan, D., D'Oca, S., and fei Chen, C. (2017). Ten questions concerning occupant behavior in buildings: the big picture. Build. Environ. 114, 518–530. doi: 10.1016/j.buildenv.2016.12.006
Hu, L. T., and Bentler, P. M. (1999). Cutoff criteria for fit indexes in covariance structure analysis: conventional criteria versus new alternatives. Struct. Equ. Model. 6, 1–55. doi: 10.1080/10705519909540118
Hu, S., Yan, D., Azar, E., and Guo, F. (2020). A systematic review of occupant behavior in building energy policy. Build. Environ. 175, 106807. doi: 10.1016/j.buildenv.2020.106807
Hulland, J. (1999). Use of partial least squares (PLS) in strategic management research: A review of four recent studies. Strategic Manag. J. 20, 195–204. doi: 10.1002/(SICI)1097-0266(199902)20:2<195::AID-SMJ13>3.0.CO;2-7
Jami, S., forouzandeh, N., Zomorodian, Z. S., Tahsildoost, M., and Khoshbakht, M. (2021). The effect of occupant behaviors on energy retrofit: a case study of student dormitories in Tehran. J. Clean. Prod. 278, 123556. doi: 10.1016/j.jclepro.2020.123556
Jang, H., and Kang, J. (2016). A stochastic model of integrating occupant behaviour into energy simulation with respect to actual energy consumption in high-rise apartment buildings. Energy Build. 121, 205–216. doi: 10.1016/j.enbuild.2016.03.037
Jones, P., Li, X. J., Perisoglou, E., and Patterson, J. (2017). Five energy retrofit houses in South Wales. Energy Build. 154, 335–342. doi: 10.1016/j.enbuild.2017.08.032
Kroonenberg, P. M., and Lohmoller, J.-B. (1990). Latent variable path modeling with partial least squares. J. Am. Stat. Assoc. 85, 909–910. doi: 10.2307/2290049
Kurian, G. (2014). Reliability and Validity Assessment: The Encyclopedia of Political Science. Washington, DC: CQ Press. p. 1449–1450.
Leguina, A. (2015). A primer on partial least squares structural equation modeling (PLS-SEM). Int. J. Res. Method Educ. 38, 220–221. doi: 10.1080/1743727X.2015.1005806
Maier, T., Krzaczek, M., and Tejchman, J. (2009). Comparison of physical performances of the ventilation systems in low-energy residential houses. Energy Build. 41, 337–353. doi: 10.1016/j.enbuild.2008.10.007
Martinaitis, V., Edmundas, K. Z., Violeta, M., and Tatjana, V. (2015). Importance of occupancy information when simulating energy demand of energy efficient house: a case study. Energy Build. 101, 64–75. doi: 10.1016/j.enbuild.2015.04.031
Mulaik, S. A., James, L. R., Van Alstine, J., Bennet, N., Lind, S., and Stilwell, C. D. (1989). Evaluation of goodness-of-fit indices for structural equation models. Psychol. Bull. 105, 430–445. doi: 10.1037/0033-2909.105.3.430
Naji, S., Shahaboddin, S., Hamed, B., Johnson Alengaram, U., Mohd, Z. J., and Mohsen, A. (2016). Soft computing methodologies for estimation of energy consumption in buildings with different envelope parameters. Energy Efficiency 435–453. doi: 10.1007/s12053-015-9373-z
Peng, D. X., and Lai, F. (2012). Using partial least squares in operations management research: a practical guideline and summary of past research. J. Operat. Manage. 30, 467–480. doi: 10.1016/j.jom.2012.06.002
Schakib-Ekbatan, K., Çakici, F. Z., Schweiker, M., and Wagner, A. (2015). Does the occupant behavior match the energy concept of the building?-Analysis of a German naturally ventilated office building. Build. Environ. 84, 142–150. doi: 10.1016/j.buildenv.2014.10.018
Schweiker, M., and Shukuya, M. (2010). Comparative effects of building envelope improvements and occupant behavioural changes on the exergy consumption for heating and cooling. Energy Policy 38, 2976–2986. doi: 10.1016/j.enpol.2010.01.035
Shipworth, M. (2011). Thermostat settings in English houses: No evidence of change between 1984 and 2007. Build. Environ. 46, 635–642. doi: 10.1016/j.buildenv.2010.09.009
Tenenhaus, M., Amato, S., and Vinzi, E. V. (2004). “A global goodness-of fit index for PLS structural equation modelling,” in The XLII SIS Scientific Meeting (Padova: CLEUP), 739–742.
Ürge-Vorsatz, D., Danny Harvey, L. D., Mirasgedis, S., and Levine, M. D. (2007). Mitigating CO2 emissions from energy use in the world's buildings. Build. Res. Inf. 35, 379–398. doi: 10.1080/09613210701325883
Wei, S., Hassan, T. M., Firth, S. K., and Fouchal, F. (2017). Impact of occupant behaviour on the energy-saving potential of retrofit measures for a public building in the UK. Intell. Build. Int 9, 97–106. doi: 10.1080/17508975.2016.1139538
Xu, X., Xiao, B., and Li, C. Z. (2020). Critical factors of electricity consumption in residential buildings: an analysis from the point of occupant characteristics view. J. Clean. Prod. 250, 120423. doi: 10.1016/j.jclepro.2020.120423
Keywords: occupants' behaviours (OBs), structural equation modelling (SEM), energy consumption (EC), residential buildings, retrofit
Citation: Tavakoli E, Nikkhah A, Zomorodian ZS, Tahsildoost M and Hoonejani MR (2022) Estimating the Impact of Occupants' Behaviour on Energy Consumption by Pls-SEM: A Case Study of Pakdel Residential Complex in Isfahan, IRAN. Front. Sustain. Cities 4:700090. doi: 10.3389/frsc.2022.700090
Received: 25 April 2021; Accepted: 02 February 2022;
Published: 09 March 2022.
Edited by:
Selin Yilmaz, Université de Genève, SwitzerlandReviewed by:
Edward A. Awafo, University of Energy and Natural Resources, GhanaSoufiane Boukarta, University of Blida, Algeria
Copyright © 2022 Tavakoli, Nikkhah, Zomorodian, Tahsildoost and Hoonejani. This is an open-access article distributed under the terms of the Creative Commons Attribution License (CC BY). The use, distribution or reproduction in other forums is permitted, provided the original author(s) and the copyright owner(s) are credited and that the original publication in this journal is cited, in accordance with accepted academic practice. No use, distribution or reproduction is permitted which does not comply with these terms.
*Correspondence: Zahra Sadat Zomorodian, z_zomorodian@sbu.ac.ir