Design of Sustainable Renewable-Based Utility Plants in the Face of Uncertainty
- 1Departamento de Ingeniería Química, Tecnológico Nacional de México, Instituto Tecnológico de Celaya, Celaya, Mexico
- 2Department of Chemical Engineering, University of Salamanca, Salamanca, Spain
This work presents the formulation of a two-stage stochastic mixed-integer linear programming (MILP) model to include uncertainty in the design of renewable-based utility plants. The model is based on a superstructure that integrates technologies to process biomass, waste, solar radiation, and wind and considers uncertainty in availability of the renewable resources and on the utility demands. The uncertain parameter space is calculated based on a monthly probability density function for each uncertain parameter and discretized into different levels. It is shown that as uncertainty is considered in the model formulation, design flexibility improves with respect to the deterministic-based designs, although the flexibility is achieved at the expense of higher underused facilities and therefore unused investment cost.
Introduction
Renewable energy resources, such as solar and wind energy, are being considered with special interest due to their contribution toward the development of a sustainable energy industry. These types of energy sources are characterized for their intermittent behavior, which needs to be considered during the design process of renewable-based energy systems. Some authors have proposed the design of renewable-based systems that integrate different types of processing technologies and storage systems to mitigate the intermittency of renewables. For instance, Peng et al. (2020) proposed the use of fixed bed reactors to be integrated into concentrated solar plants (CSPs) as a thermochemical energy storage. Three 3 types of gas-solid reversible reactions, namely, redox, hydroxide, and carbonate reactions, were considered. In this configuration, a heat transfer fluid, heated by solar energy, is used during the daytime operation to drive an endothermic reaction, while during the night the working fluid, used in the power block, is sent to the reactor to absorb the heat released by the exothermic reaction. Wang et al. (2017) addressed the conceptual design of ammonia-based energy storage systems. Malheiro et al. (2015) formulated an MILP model to design hybrid electrical systems that integrate wind turbines, photovoltaics, diesel generators and batteries. The model provides the optimal sizing and scheduling of the system. Ranaweera and Midtgård (2016) studied the economic and operational implications of a system that couples PV technology with a battery system. They showed that such a scheme increases the daily economics benefits while reducing over-voltage problems caused by reverse power flow. Other authors have developed mathematical frameworks that include uncertainty as an effective tool to evaluate the effect of variability of parameters on the system performance. On this subject, one can distinguish two approaches to include uncertainty in an optimization model, a robust optimization approach (Ben-Tal et al., 2011; Zhang et al., 2016; Saeedi et al., 2019) and a two-stage stochastic approach (Grossmann et al., 2017). The first one consists of finding a feasible solution for every uncertain scenario, while the second approach optimizes the expected value over the possible realizations considered within a set of uncertain parameters. Both approaches have been used aiming at increasing the quality of the solutions of the mathematical models used to design renewable-based systems. For instance, Amusat et al. (2018) formulated a mathematical model to determine the optimal size and scheduling of a renewable-based integrated system under uncertainty. A stratified random sampling method was used to generate different scenarios to determine the best structure of the system. Allman et al. (2019) formulated a robust optimization approach to determine the optimal scheduling of a wind-powered ammonia plant. Baringo and Conejo (2013) used clustering methods to calculate representative structures to design wind-power production plants. Mallapragada et al. (2018) reported the impact of a model solution on the long-term expansions of renewable-based electrical systems; the variability of renewable resources was characterized by using two approaches, namely, representative days and time slices. Cao et al. (2018) used statistical extrapolation techniques to determine the optimal control scheme of a wind turbine. Zhang and Conejo (2017) formulated a model that considered long- and short-term uncertainty to determine the expansion planning of electrical systems. Long-term uncertainty was related to changes over time, including demand growth and capacity expansions, while short-term uncertainty considered renewable sources variability. The problem was solved using the primal Bender's decomposition algorithm. Martín (2016) formulated a two-stage stochastic approach to study the effect of uncertainty on the design and operation of renewable-based facilities that produce chemicals as a way to store wind and solar energy. Daneshvar et al. (2020) addressed the problem of the optimal scheduling of systems that integrate wind and thermal technologies with hydropower pumped storage (PHS). In that work, first-stage variables were defined in terms of production cost of electricity based on thermal and PHS units, while second-stage variables were related to dispatch costs of units.
In addition to the uncertain behavior of the supply of renewable resources, the design of utility plants must also consider the fluctuating demands of steam and electricity. On this subject, some authors have included uncertainty in the design of fossil fuel-based utility plants to improve their flexibility. For instance, Sun et al. (2017) developed a two-stage stochastic model to determine the best configuration of a utility plant that uses natural gas as fuel, and took steam demands and electricity prices as uncertain parameters. Zhao and You (2019) formulated a data-driven robust optimization model to determine the operating conditions that minimize the energy consumption of a fossil-based utility plant. The model considered uncertainty on utility demands (exogenous uncertainty) and on the efficiency of the pieces of equipment (endogenous uncertainty), while the uncertainty set was generated by fitting the Dirichlet process mixture model to historical industrial data. Bungener et al. (2015) evaluated the resilience of a steam network when unexpected boilers shutdowns take place, for which Monte-Carlo simulations were used to randomly generate failure scenarios. The study of resilience of utility plants under unexpected equipment failure has also been addressed in other works (Luo et al., 2013; Sun and Liu, 2015).
Pérez-Uresti et al. (2020) developed a multi-period MILP model to design renewable-based utility plants. The model considered the integration of wind, solar radiation, biomass, and waste to produce steam and electricity, and was used to study the effect of time discretization on the optimal design. The flexibility of the model has already been tested in several case studies including the design of utility plants located in Scotland (Pérez-Uresti et al., 2019a), and Spain (Perez Uresti, 2020). The model has also been used to estimate the production cost of renewable-based steam at different pressures levels (Pérez-Uresti et al., 2019b). One limitation of those works, however, is that uncertainty was not considered. The incentive is to design a system flexible enough to ensure continuous supply of steam and electricity, even when an increased flexibility will probably be achieved at the expense of bigger and more expensive designs.
The objective of this work is to include uncertainty in the design of renewable-based utility plants to study the economic, operational, and technical implications of developing a system with increased flexibility. We formulate a two-stage multi-period MILP stochastic model that considers wind velocity, solar radiation, and utility demands as uncertain parameters. The model is based on a superstructure that includes biomass, biogas, and syngas boilers, a concentrated solar power plant (CSP), wind turbines, and a waste heat recovery system consisting of syngas and biogas turbines linked to a heat recovery steam generator (HRSG). To characterize uncertainty, monthly tree scenarios with probability density functions (PDF) are generated for each uncertain parameter based on historical data. Then, a different number of scenarios is considered to study the performance of the system under more detailed time discretization, along with its economic implications.
The rest of the paper is organized as follows. Section Overall Superstructure Description shows a general description of the superstructure. In section Problem Formulation the solution procedure is discussed, while in section Case Study the case study developed for the south-west region of Mexico is reported. Results and discussion are presented in section Results, and in section Conclusions, some conclusions of this work are given.
Overall Superstructure Description
Figure 1 shows the structure of the renewable-based utility plant. In the first section, biomass, solar radiation, waste, and wind energy are used as feedstocks to produce utilities. To process each renewable resource, different technologies are included, namely, biomass boilers, gasifiers, syngas, and biogas turbines, a concentrated solar power (CSP) plant, and wind turbines. The second section of the utility plant consists of a steam network that includes a steam turbine, steam headers, heat exchangers, and a cooling system. The optimal design of the plant is driven by the minimization of the total annual cost (TAC). Solar radiation, wind velocity, and utilities demand are considered under uncertainty.
Problem Formulation
The description of the problem formulation is presented in three sections. In the first one, the model and main assumptions are described. Section Definition of the First- and Second-Stage Variables shows the definition of the first- and second-stage variables involved in the two-stage stochastic approach. Finally, in section Procedure to Generate Scenarios and to Calculate Their Probabilities, the characterization of uncertainty including the generation of scenarios and the estimation of frequencies of occurrence is described.
Modeling Description
In this work, the model presented by Pérez-Uresti et al. (2019a) was extended to include the uncertainty related to renewable resources and utility demands. Uncertainty was included by formulating a two-stage multi-period stochastic program. In this section, only general aspects of the model are given; for a more detailed description, the reader is referred to Pérez-Uresti et al. (2019a).
Biomass Processing
The flowsheet of the biomass processing section is shown in Figure 2. It is assumed that the lignocellulosic biomass generated in a period of time can be stored or used in the same period to produce utilities. There are two processing options. In the first one, the biomass is burnt in a biomass boiler, which can produce as many as four types of steam. The model is based on mass and energy balances, and the production of any type of steam is conditioned by the existence of the boiler.
In the second option, biomass is converted into syngas through a gasification process. In this case, the model can select between direct and indirect gasification. The syngas is sent to a reforming stage, where the light hydrocarbons produced in the gasifier reactor are converted into H2 and CO. The reforming of hydrocarbons can be carried out by partial oxidation (POX) or by steam reforming (SR). Syngas can then be sent to a turbine or to a boiler. Within the syngas turbine, the syngas is compressed, burnt in a combustion chamber, and finally expanded to produce electricity. The flue gas leaving the turbine is sent to an HRSG system to produce at most two types of steam. The modeling of the gasification stage is based on a surrogate model that consists of four steps: (a) development of mass and energy balances to calculate the syngas composition exiting from gasifiers, (b) simulation of the reformer reactors in Aspen plus, (c) simulation of the syngas turbine in Aspen plus, and (d) development of an optimization subroutine to design the HRSG system. From the results, parameters needed for mass and energy balances for this section are calculated.
Concentrated Solar Power
The concentrated solar power plant (CSP) consists of two sections, the heliostat field and the steam generation section, see Figure 3. In the first section, solar radiation is captured by the heliostats and redirected to the top of a solar tower, where a heat exchanger, called receiver, is placed. The concentrated solar energy is used to heat a heat transfer fluid (in this case molten salts) from 290 to 565°C (Martín and Martín, 2013). Thereafter, the molten salts are sent to the thermal storage tanks, from which a fraction is sent to the second section of the plant. This section was modeled using energy balances to calculate the area of the heliostat field, with lower and upper bounds taken as 120 and 1,500,000 m2 (National Renewable Energy Laboratory, 2017). A value of 55% as the global efficiency for the heliostat field was considered (Martín and Martín, 2013).
The steam generation section consists of a set of heat exchangers that produce steam at different pressure levels (as many as four types) and reheat the steam coming from the steam turbine. The production of any type of steam is restricted by the existence of the plant. The modeling of the CSP plant is based on mass and energy balances in the splitting points shown in Figure 3, and on design equations to calculate the heat transfer area of each heat exchanger.
Biogas Processing
Biogas can be produced by using either municipal solid waste (MSW) or cattle manure (CM) as feedstock, processed in an anaerobic digestor (see Figure 4). In addition to methane, carbon dioxide, NH3, and H2S are also produced. These compounds are then removed in a purification stage. The biogas can be sent to a biogas boiler or to a waste heat recovery system that consists of a boiler linked to a heat recovery steam generator. This section of the plant is modeled based on a surrogate model that consists of three steps: (a) computation of mass and energy balances for the digestor using the yield data of each waste reported by León and Martín (2016); (b) simulation and sensitivity analysis (power produced vs. compression ratio) of the biogas turbine using Aspen plus; and (c) development of an optimization subroutine to design the HRSG.
Wind Farm
The wind farm was modeled based on power output curves of wind turbines fitted to the following correlation (De la Cruz and Martín, 2016),
where vsc,t is the wind velocity in period t and scenario sc. The selected design of wind turbine is characterized by Pnom = 1,500, a = 8.08 m/s, mp = 0.78 s/m. Finally, Nturbsc,t is the number of wind turbines used over time for each scenario. For wind farms, a lower bound of (where DWT is the diameter of the wind turbine) and an upper bound of 36 km2 (Aspen Environmental Group, 2018) were considered.
Steam Network
The steam network includes a steam turbine with multiple extractions, a set of steam headers, a cooling tower, and a condenser. The steam produced by the renewable resources is sent to this section to be expanded through the steam turbine and, if needed, to be reheated. The model of this section is based on mass and energy balances. The steam network also provides different pathways to produce steam, which can be done through multiple extractions of the steam turbine with pressures corresponding to HP, MP, and LP steam.
Definition of the First- and Second-Stage Variables
The formulation of the two-stage stochastic program considers the following first- and second-stage decisions.
First-Stage Decisions
First-stage decisions are made before uncertainty is considered, and are related to the number and size of the units (i.e., investment or design variables). They remain fixed over all the scenarios. In this work, the first-stage variables are defined as follows.
Biomass Processing
Equations (2)–(6) are related to the biomass boiler, gasifiers, syngas turbine, syngas boiler, and the HRSG system, respectively.
CSP Plant
The design area for each heat exchanger in the steam generation section is restricted by Equation (7), while Equations (8) and (9) account for limits on the flowrate of molten salts and the design area of the heliostat field.
Biogas Processing
The design variables for biogas processing are constrained by Equations (10)–(13), which are related to the digestor, the biogas boiler, the biogas turbine and the HRSG system.
Wind Farm
A restriction for the wind farm is given by Equation (14).
Steam Network
Equation (15) applies to the heat exchangers involved in the steam network, while Equations (16), (17) are used for the steam turbine and the cooling tower.
After the structure of the system is obtained, the cost of each equipment unit is estimated from correlations of the form,
where A is a coefficient related to the scaling of the equipment unit, B is the fixed cost related to the unit, and y is a binary variable used to indicate the existence or selection of the unit. The total investment of the plant is calculated by adding the cost of the units involved in each processing section multiplied by a factor of 3.3 to consider costs of instrumentation, ancillary buildings and other components (El-Halwagi, 2012).
Second-Stage Decisions
Second-stage decisions are taken when uncertainty is considered and are associated to control variables. They depend on the scenario realization (i.e., operating variables such as flowrates of steam, power, and biomass). The definition of the second-stage variables as a function of each realization is shown below.
a) Production of steam under uncertainty. The production of any type of steam depends on each scenario realization as follows,
Mass balance for biomass-based steam
Mass balance for biogas-based steam
Global mass balance for the renewable-base steam
The procedure to generate the scenarios and their probabilities of occurrence is explained in section Procedure to Generate Scenarios and to Calculate Their Probabilities.
b) Operating cost. The expected operating cost of each section over all of the possible realizations is calculated as follows.
Biomass processing
where πsc,t is the probability associated with each scenario, Cbiom is the cost of biomass in $/t and Cstorage is the cost of storage of biomass, set equal to 6.5 $/t (Idaho National Laboratory, 2019).
Biogas Processing
CSP plant
Wind Farm
Procedure to Generate Scenarios and to Calculate Their Probabilities
Figure 5 shows a schematic representation for the calculation of the occurrence probability of each scenario. The procedure consists of five stages.
1. Generate monthly probability density functions for each uncertain parameter. As a first step, historical data, wθ,t, are used to estimate the monthly probability density function (PDF) of solar radiation, wind velocity, and electricity and steam demands, which are considered as uncertain parameters. The PDF helps to see how scattered the data of the uncertain parameters are over time. In addition, it is used to calculate the probability associated with the uncertain parameter, θ, taking a value of wθ,t, which is defined as .
2. Discretize the probability distribution of uncertain parameters. The probability function of each uncertain parameter can be approximated by discretization into a set of finite values ranging at different levels, represented by eθ. Each level is associated with the probability of occurrence of low, medium, and high values of each uncertain parameter. Once the number of levels is set, upper, and lower bounds, and are established.
3. Assign a nominal value to represent each level of the uncertain parameters. A nominal value, , that can represent each level is calculated as the weighted sum of the data included in each level
where wθ,t is the value that the uncertain parameter, θ, takes in the period of time t, represents the frequency of occurrence of the value wθ,t within that period of time, and and represent the upper and lower bounds that define the level eθ.
4. Calculate the probability of occurrence of the nominal value. The probability associated with the nominal value is calculated as the accumulated probability of the values included in each level,
where is the probability calculated from the PDF and associated with the uncertain parameter, θ, with a value of wθ,t, and represent the upper and lower bounds that define the level eθ.
5. Generate the possible scenarios and their probabilities of occurrence. The number of scenarios that are generated for each period of time, SCt, are calculated. They are given by the Cartesian product of the levels selected to discretize the probability function of each uncertain parameter.
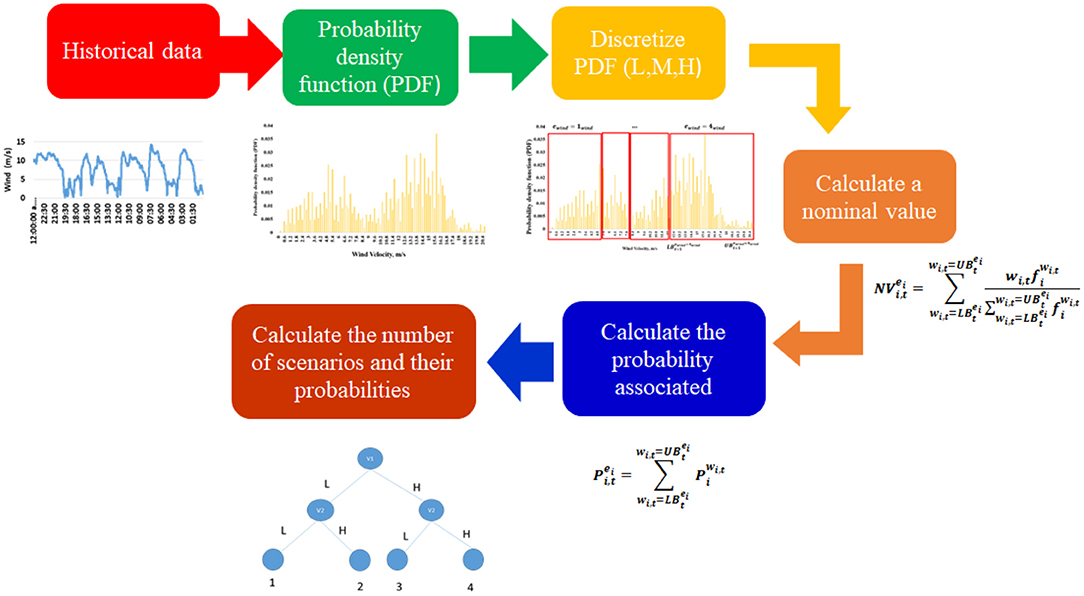
Figure 5. Schematic representation of procedure to generate scenarios and to calculate their probabilities.
Finally, the probability associated with each scenario, πsc,t, is calculated as follows,
A condition to meet is that the summation of the probabilities of each scenario generated in the period of time t has to be equal to one.
Objective Function
The objective function consists of minimizing the total annual cost (TAC) of the utility plant, defined as the summation of the annualized first-stage cost (investment cost) plus the expected second-stage cost (operating cost).
s.t.
where d are the design variables, x are flows, y are integer variables, and θsc is the uncertain parameter for scenario sc.
Case Study
The model was applied to design a renewable-based utility plant located in the south-west region of Mexico. The utility plant is assumed to supply LP steam to a bioethanol plant while delivering electricity to a city. Wind velocity, solar radiation, LP steam demand and electricity demand were taken as uncertain parameters, for which historical data for the years 2015–2018 (Centro Nacional de Control de Energía, 2019; National Renewable Energy Laboratory, 2019) were used to calculate the probability of each scenario. LP steam demand was calculated by correlating the monthly gasoline consumption (Secretaria de Energía, 2018) with the LP steam consumption of a typical bioethanol plant (Karuppiah et al., 2008) and assuming a 5.8% blend of bioethanol in gasoline (Secretaria de Energía, 2016). For a more detailed description of the case study, the reader is referred to Pérez-Uresti et al. (2019a). To solve the problem, four levels of wind velocity, and three levels for solar radiation and demands for electricity and LP steam were considered, which resulted in a total number of scenarios per month of 108. The problem consists of 963,960 continuous variables, 1,634,964 equations and 200 binary variables. It was coded in the GAMS software environment, and solved using CPLEX in 149,827 s of CPU time, with a gap below 1%.
Results
Section Design of a Renewable-Based Utility Plant Under Uncertainty shows the optimal design of the utility plant that includes uncertainty, along with an analysis of the effect of reducing the discretization level of the uncertainty on the optimal solution. In section A Comparison Between Deterministic Solutions and Solutions Including Uncertainty, a comparative analysis between a design based on a deterministic formulation and the one that includes uncertainty is reported.
Design of a Renewable-Based Utility Plant Under Uncertainty
When 108 scenarios were considered, the utility plant integrated a CSP plant and a biomass boiler, see Figure 6. The CSP plant, consisting of 7,017 heliostats, is used to produce VHP steam and to reheat the steam coming from the steam turbine. Figure 7A shows the average monthly use of heliostats, out of which, on average, 30% remain idle over the year. The CSP plant supplies 86% of the total electricity requirements, and the rest is supplied by the biomass boiler (see Figures 7B,C). One can also observe that the biomass is used as a backup for the CSP plant. Figure 7D shows that biomass is consumed over the year (and a fraction is stored) reaching its highest level in June, when the electricity demand is also the highest. Results also showed that the LP steam required by the bioethanol plant is produced by expanding some of the VHP steam in the steam turbine.
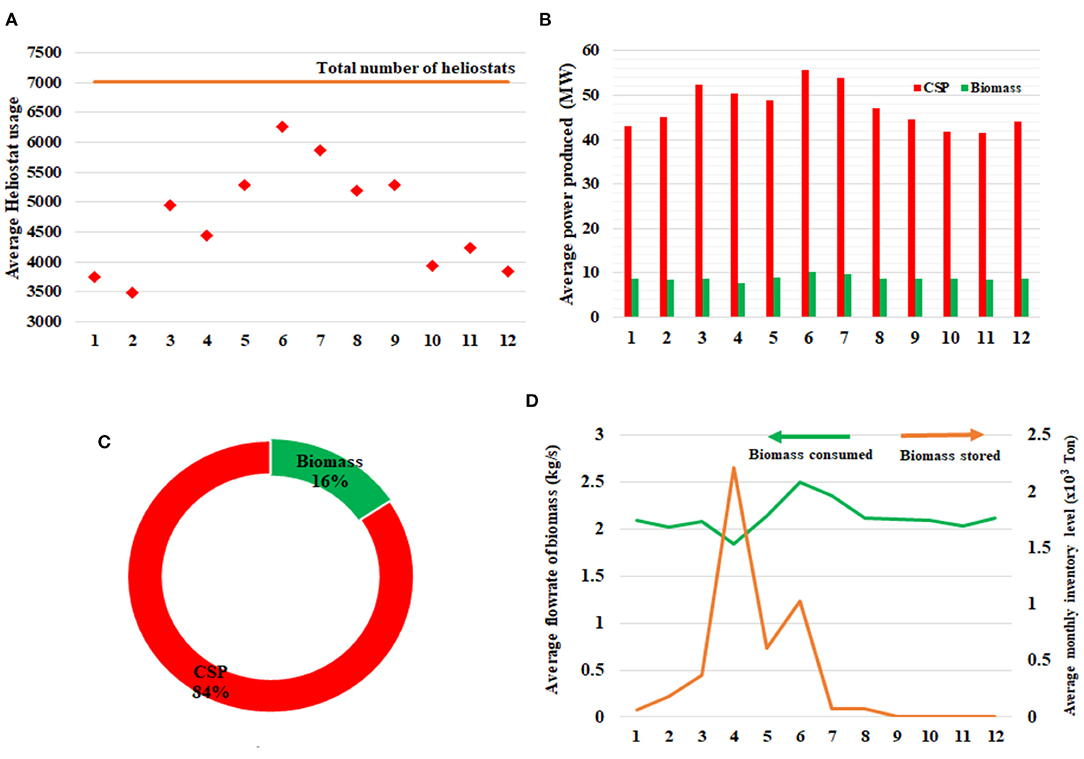
Figure 7. Results for the 108sc-based design: (A) Average monthly heliostats usage over year, (B) Average monthly production of power, (C) contribution percentage of each renewable resource (D) profile of biomass over the year.
Effect of the Discretization Level of the Uncertainty
As mentioned above, the previous results were based on 108 scenarios generated by discretizing the PDF of uncertain parameters into different levels. Under a two-stage stochastic approach, care must be taken not to use a number of scenarios so high that it could lead to intractable problems, with very expensive CPU burdens. To handle this problem, Peng et al. (2019) characterized the solar radiance variability by classifying it at two scales, low- and high-frequency. The former was related to seasonal variability represented through representative periods, while the latter was related to daily variations represented through different modes (i.e., day and night modes) using time periods within a day. In this work, we use a coarse time discretization considering different values of demand and renewable resources. In this way, we ensure the inclusion of wider ranges of values for the uncertain parameters, while avoiding intractable problems. Then, we implement a reduction of the discretization level of the uncertainty to analyze its effect on the first-stage decisions and objective function values, as well as on the associated CPU burden. The levels of PDF discretization for each uncertainty parameter are shown in Table 1. Overall, one can observe that the reduction of scenarios did not have a significant effect on the selection of the renewable resources (see Table 2). The topology of the plant showed minor changes, the most important of which was related to the size of CSP plants (i.e., first-stage variables). It was observed that as a lower level of discretization for the uncertainty was considered, the solution included a smaller CSP plant, for which the number of heliostats were reduced from 7,017 (when considering 108 sc) to 6,271 (when considering 16 sc). However, it is worthy of mention that the effect on the TAC was not significant, as it was only reduced by 2.6%. On the other hand, the number of scenarios affected CPU times significantly. In the extreme case, when 16 scenarios were considered, the CPU time was reduced by 99% with respect to the case with 108 scenarios (see Table 3).
A Comparison Between Deterministic Solutions and Solutions Including Uncertainty
In this section, a comparative analysis is conducted between the solutions considering uncertainty and the deterministic formulations based on monthly and weekly time discretization. The selection of renewable resources and their flexibility are compared, along with the economic implications.
Renewable Resources Selection and Economic Implications
Results show that including uncertainty provides more flexibility since demands are met under a wider range of operating conditions, aided by a larger CSP plant (see Table 2). Similarly to the weekly-based design, when wider ranges of wind velocity and utility demands are considered wind energy becomes less attractive than CSP plants to produce utilities. The absence of wind turbines lowers the investment requirements from $150.8 MMUSD for the monthly-based-deterministic solution to $137.3 MMUSD for the stochastic-based solutions. However, the integration of a larger CSP plant increases the operating costs and, consequently, the total annual cost of the plant by 19.8%. Biomass is used in both types of solutions as a backup unit for the CSP plant. However, when comparing biomass usage profiles, stochastic solutions show a lower amount of biomass storage than the ones from the deterministic-based designs (see Table 2). This result is due to the recourse actions involved in the two-stage stochastic approach to minimize the expected cost of the second-stage variables, i.e., the operating costs.
Design Flexibility
A flexibility test similar to the one by García-Herreros et al. (2014) was conducted for each design to analyze the solutions obtained from each approach (i.e., deterministic and two-stage stochastic). First, the values of the first-stage variables, such as the number of heliostats, wind turbines and equipment size, were fixed. Then, simulations of the monthly-based designs with weekly variations of the renewable resources and utility demands were conducted and compared to the deterministic monthly-based design reported by Pérez-Uresti et al. (2020). The results are shown in Figure 8, and it can be observed that under weekly variations the deterministic solution does not offer the flexibility needed to meet the demand, which makes electricity imports necessary in the amount of 68,716 MWh. On the other hand, when the formulation considers uncertainty the flexibility of the design improves, with sufficient slack capacity to meet the weekly demands without imports.
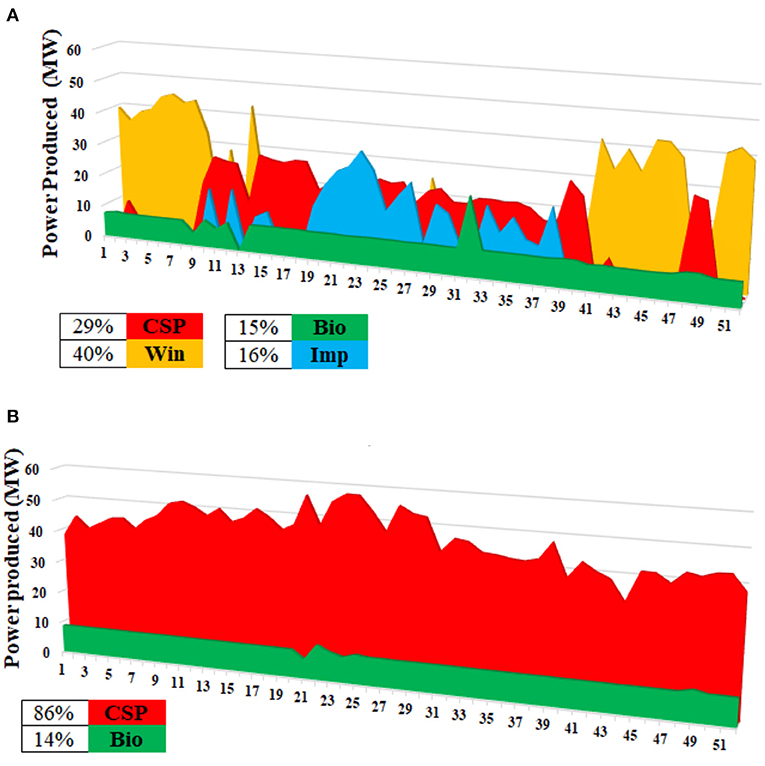
Figure 8. Results for the designs under weekly variations: (A) deterministic monthly-based design, (B) stochastic 108sc-based design.
Economic Implications of Design Flexibility
The design of the renewable-based utility plant that included uncertainty was able to meet a wider range of demands because of its larger slack capacity at the cost of larger fractions of underused facilities, and therefore larger unused investment costs. The economic implications of improving the design flexibility can be estimated as the summation of units that remain idle during each period of time multiplied by their unit investment costs. For comparison purposes, two scenarios were considered for the stochastic solutions, one with the highest and the other one with the lowest facility usage. From the results shown in Figure 9, it can be observed that the monthly percentage of unused investment for the monthly-based design is, on average, 16%, while that for the 108sc-based design is 35% (Figure 9A), which causes an increase of the unused investment from $168.7 MMUSD for the monthly-based design to $569.6 MMUSD for the 108sc-based design (Figure 9B).
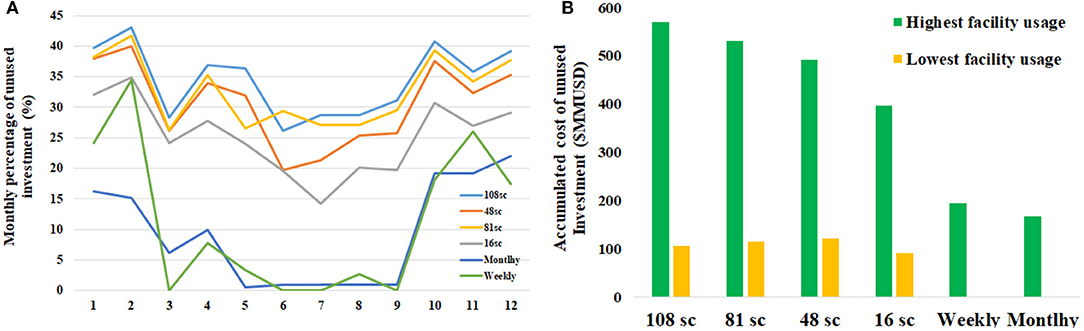
Figure 9. Economic implications of design flexibility. (A) Monthly percentage of unused investment, (B) accumulated cost of unused investment cost.
Conclusions
The design of sustainable utility plants based on renewable resources is hampered by the inherent uncertainty of the availability of resources such as wind and solar sources. An approach to the design of utility plants that includes uncertainty in renewable energy resources has been presented, for which a two-stage stochastic programming has been formulated. The model is based on a superstructure that considers the integration of different technologies to process wind, solar radiation, biomass, and waste to produce steam and electricity. Monthly probability distribution functions, PDF, based on historical data of each uncertain parameter were developed to generate scenarios and to calculate their probability of occurrence. In particular, the effect of uncertainty on the optimal topology of the plant and on its flexibility was studied. For this purpose, a case study for the south-west region of Mexico was taken. Results showed that when uncertainty was included in the model formulation, the optimal solution for the plant integrated a larger CSP plant with respect to deterministic-based designs, which allowed the plant to have enough slack capacity to meet a wider range of utility demands. This item was tested by studying the performance of design under a more detailed time discretization. Results showed that, unlike the monthly-based deterministic design, the stochastic-based design was able to meet the demands predicted under a weekly time discretization. However, increased flexibility also implied an increase in underused facilities. It was observed that, on average, the unused investment for the stochastic-based design was more than twice larger than the one for the monthly-based deterministic design. Moreover, environmental advantages of the proposed approach were shown to be remarkable, as the CO2 emissions were 2,580% lower than the ones generated by a fossil fuel-based system. This type of analysis provides policy-makers with valuable information to make well-informed decisions.
Data Availability Statement
The original contributions presented in the study are included in the article/supplementary material, further inquiries can be directed to the corresponding author.
Author Contributions
MM, AJ-G, and SP-U conducted the modeling and optimization of utility plants and prepared the manuscript. All authors contributed to the article and approved the submitted version.
Funding
Support from the National Council for Science and Technology, CONACYT, Mexico from its Mixed-Scholarships Program, for a twelve-month stay of SP-U at the University of Salamanca, Spain, is gratefully acknowledged.
Conflict of Interest
The authors declare that the research was conducted in the absence of any commercial or financial relationships that could be construed as a potential conflict of interest.
Publisher's Note
All claims expressed in this article are solely those of the authors and do not necessarily represent those of their affiliated organizations, or those of the publisher, the editors and the reviewers. Any product that may be evaluated in this article, or claim that may be made by its manufacturer, is not guaranteed or endorsed by the publisher.
Acknowledgments
The assistance of the PSEM3 research group is gratefully acknowledged.
References
Allman, A., Palys, M. J., and Daoutidis, P. (2019). Scheduling-informed optimal design of systems with time-varying operation: A wind-powered ammonia case study. AIChE J. 65, e16434. doi: 10.1002/aic.16434
Amusat, O. O., Shearing, P. R., and Fraga, E. S. (2018). Optimal design of hybrid energy systems incorporating stochastic renewable resources fluctuations. J. Energy Storage. 15, 379–399. doi: 10.1016/j.est.2017.12.003
Aspen Environmental Group (2018). Alta-Oak Creek Mojave Wind Project. Available online at: http://www.aspeneg.com/projects/alta-oak-creek-mojave-project/ (accessed July, 2018).
Baringo, L., and Conejo, A. J. (2013). Correlated wind-power production and electric load scenarios for investment decisions. Appl. Energy. 101, 475–482. doi: 10.1016/j.apenergy.2012.06.002
Ben-Tal, A., Do Chung, B., Mandala, S. R., and Yao, T. (2011). Robust optimization for emergency logistics planning: risk mitigation in humanitarian relief supply chains. Transp. Res. B-Meth 45, 1177–1189. doi: 10.1016/j.trb.2010.09.002
Bungener, S. L., Van Eetvelde, G. M., Descales, B., and Maréchal, F. (2015). Resilient Decision Making in Steam Network Investments (No. CONF). Milan: The Italian Association of Chemical Engineering, 73–78.
Cao, Y., Zavala, V. M., and D'Amato, F. (2018). Using stochastic programming and statistical extrapolation to mitigate long-term extreme loads in wind turbines. Appl. Energy 230, 1230–1241. doi: 10.1016/j.apenergy.2018.09.062
Centro Nacional de Control de Energía (2019). Estimación de la Demanda Real del Sistema. Available online at: https://www.cenace.gob.mx/SIM/VISTA/REPORTES/DemandaRealSist.aspx (accessed July, 2019).
Daneshvar, M., Mohammadi-Ivatloo, B., Zare, K., and Asadi, S. (2020). Two-stage stochastic programming model for optimal scheduling of the wind-thermal-hydropower-pumped storage system considering the flexibility assessment. Energy 193:116657. doi: 10.1016/j.energy.2019.116657
De la Cruz, V., and Martín, M. (2016). Characterization and optimal site matching of wind turbines: effects on the economics of synthetic methane production. J. Clean. Prod. 133, 1302–1311. doi: 10.1016/j.jclepro.2016.06.019
El-Halwagi, M. M. (2012). Sustainable Design through Process Integration: Fundamentals Applications to Industrial Pollution Prevention, Resource Conservation, and Profitability Enhancement. Oxford: Butterworth-Heinemann. doi: 10.1016/B978-1-85617-744-3.00009-6
García-Herreros, P., Wassick, J. M., and Grossmann, I. E. (2014). Design of resilient supply chains with risk of facility disruptions. Ind. Eng. Chem. Res. 53, 17240–17251. doi: 10.1021/ie5004174
Grossmann, I. E., Apap, R. M., Calfa, B. A., Garcia-Herreros, P., and Zhang, Q. (2017). Mathematical programming techniques for optimization under uncertainty and their application in process systems engineering. Theor. Found. Chem. Eng. 51, 893–909. doi: 10.1134/S0040579517060057
Idaho National Laboratory (2019). Feedstock Supply System Design and Economics for Conversion of Lignocellulosic Biomass to Hydrocarbon Fuels Conversion Pathway : Biological Conversion of Sugars to Hydrocarbons. The 2017 Design Case. Available online at: https://bioenergy.inl.gov/Reports/Design%20Case%202017.pdf (accessed June, 2019).
Karuppiah, R., Peschel, A., Grossmann, I. E., Martín, M., Martinson, W., and Zullo, L. (2008). Energy optimization for the design of corn-based ethanol plants. AIChE J. 54, 1499–1525. doi: 10.1002/aic.11480
León, E., and Martín, M. (2016). Optimal production of power in a combined cycle from manure based biogas. Energ. Convers. Manage. 114, 89–99. doi: 10.1016/j.enconman.2016.02.002
Luo, X., Zhang, B., Chen, Y., and Mo, S. (2013). Operational planning optimization of steam power plants considering equipment failure in petrochemical complex. Appl. Energy 112, 1247–1264. doi: 10.1016/j.apenergy.2012.12.039
Malheiro, A., Castro, P. M., Lima, R. M., and Estanqueiro, A. (2015). Integrated sizing and scheduling of wind/PV/diesel/battery isolated systems. Renew. Energ. 83, 646–657. doi: 10.1016/j.renene.2015.04.066
Mallapragada, D., Papageorgiou, D., Venkatesh, A., Lara, C. L., and Grossmann, I. E. (2018). Impact of model resolution on scenario outcomes for electricity sector system expansion. Energy 163, 1231–1244. doi: 10.1016/j.energy.2018.08.015
Martín, L., and Martín, M. (2013). Optimal year-round operation of a concentrated solar energy plant in the south of Europe. Appl. Therm. Eng. 59, 627–633. doi: 10.1016/j.applthermaleng.2013.06.031
Martín, M. (2016). Methodology for solar and wind energy chemical storage facilities design under uncertainty: methanol production from CO2 and hydrogen. Comp. Chem. Eng. 92, 43–54. doi: 10.1016/j.compchemeng.2016.05.001
National Renewable Energy Laboratory (2017). Concentrating Solar Power Projects. Available online at: https://www.nrel.gov/csp/solarpaces/ (accessed July, 2019).
National Renewable Energy Laboratory (2019). National Solar Radiation Database 1998-2018. Available online at: https://rredc.nrel.gov/solar/old_data/nsrdb/ (accessed August, 2019).
Peng, X., Root, T. W., and Maravelias, C. T. (2019). Optimization-based process synthesis under seasonal and daily variability: Application to concentrating solar power. AIChE J. 65:e16458. doi: 10.1002/aic.16458
Peng, X., Yao, M., Root, T. W., and Maravelias, C. T. (2020). Design and analysis of concentrating solar power plants with fixed-bed reactors for thermochemical energy storage. Appl. Energy 262:114543. doi: 10.1016/j.apenergy.2020.114543
Perez Uresti, S. I. (2020). Diseño de Plantas Renovables de Servicios Auxiliares. Available online at: https://rinacional.tecnm.mx/handle/TecNM/461 (accessed November, 2020).
Pérez-Uresti, S. I., Martín, M., and Jiménez-Gutiérrez, A. (2019a). Superstructure approach for the design of renewable-based utility plants. Comp. Chem. Eng. 123, 371–388. doi: 10.1016/j.compchemeng.2019.01.019
Pérez-Uresti, S. I., Martín, M., and Jiménez-Gutiérrez, A. (2019b). Estimation of renewable-based steam costs. Appl. Energy 250, 1120–1131. doi: 10.1016/j.apenergy.2019.04.189
Pérez-Uresti, S. I., Martín, M., and Jiménez-Gutiérrez, A. (2020). A methodology for the design of flexible renewable-based utility plants. ACS Sust. Chem. Eng. 8, 4580–4597. doi: 10.1021/acssuschemeng.0c00362
Ranaweera, I., and Midtgård, O. M. (2016). Optimization of operational cost for a grid-supporting PV system with battery storage. Renew. Energy 88, 262–272. doi: 10.1016/j.renene.2015.11.044
Saeedi, M., Moradi, M., Hosseini, M., Emamifar, A., and Ghadimi, N. (2019). Robust optimization based optimal chiller loading under cooling demand uncertainty. Appl. Therm. Eng. 148, 1081–1091. doi: 10.1016/j.applthermaleng.2018.11.122
Secretaria de Energía (2016). Prospectivas de Energí as Renovables 2016-2030. Available online at: https://www.gob.mx/cms/uploads/attachment/file/284342/Prospectiva_de_Energ_as_Renovables_2017.pdf (accessed August, 2019).
Secretaria de Energía (2018). Estadí sticas de los hidrocarburos. Available online at: https://estadisticashidrocarburos.energia.gob.mx/gas.aspx (accessed July, 2019).
Sun, L., Gai, L., and Smith, R. (2017). Site utility system optimization with operation adjustment under uncertainty. Appl. Energy 186, 450–456. doi: 10.1016/j.apenergy.2016.05.036
Sun, L., and Liu, C. (2015). Reliable and flexible steam and power system design. Appl. Therm. Eng. 79, 184–191. doi: 10.1016/j.applthermaleng.2014.11.076
Wang, G., Mitsos, A., and Marquardt, W. (2017). Conceptual design of ammonia-based energy storage system: system design and time-invariant performance. AIChE J. 63, 1620–1637. doi: 10.1002/aic.15660
Zhang, Q., Morari, M. F., Grossmann, I. E., Sundaramoorthy, A., and Pinto, J. M. (2016). An adjustable robust optimization approach to scheduling of continuous industrial processes providing interruptible load. Comp. Chem. Eng. 86, 106–119. doi: 10.1016/j.compchemeng.2015.12.018
Zhang, X., and Conejo, A. J. (2017). Robust transmission expansion planning representing long-and short-term uncertainty. IEEE T. Power Syst. 33, 1329–1338. doi: 10.1109/TPWRS.2017.2717944
Zhao, L., and You, F. (2019). A data-driven approach for industrial utility systems optimization under uncertainty. Energy 182, 559–569. doi: 10.1016/j.energy.2019.06.086
Nomenclature
Parameters
πsc,t: probability associated with scenario sc in period t
Variables
: total of steam, s, flowrate produced by biomass processing in period t and scenario sc (kg/s)
: flowrate of steam, s generated in biomass boiler in period t and scenario sc (kg/s)
: flowrate of steam, s, produced in HRSG, n, by using exhaust gas, in period t and scenario sc (kg/s)
: flowrate of steam, s, produced by a syngas boiler which uses syngas, SYq,j in period t and scenario sc (kg/s)
: flowrate of steam, s, generated in HRSG, n by recovery heat from flue gas, Dq in period t and scenario sc (kg/s)
: total flowrate of biogas-based steam, s, flowrate produced in period t and scenario sc (kg/s)
: flowrate of steam, s generated in biogas boiler in period t and scenario sc (kg/s)
: flowrate of biogas-based steam, s, produced in HRSG, nb, by using exhaust gas, in period t and scenario sc (kg/s)
: biomass that is sent to the storage plant in period t and scenario sc (kg/s)
: biomass that is sent directly to the utility plant in period t and scenario sc (kg/s)
: inventory of biomass in period t and scenario sc (kg/s)
: heat required to produce steam in period t(kJ/s)
: heat required to reheat steam in period t (kJ/s)
(Qc)D: design variable for the biomass boiler (kJ/s)
SYq,j,t: flowrate of syngas produced in the gasifier, q and reformer, j, in period t (kg/s)
(SYq,j)D: design variable for the gasification process (kg/s)
(Pq,j,k)D: design variable for the syngas turbine, k
: heat required to produce steam in syngas boiler in period t (kJ/s)
: heat required to reheat steam in the syngas boiler in period t (kJ/s)
(QSBq,j)D: design variable for the syngas boiler (kJ/s)
: design variable for the HRSG, n (kg/s)
: design variable for the heat exchanger, l, of the CSP plant (m2)
(Asf)D: design variable for the heliostat field (m2)
(Wastez)D: design variable for the digestor (kg/s)
(QBGB)D: design variable for the biogas boiler (kJ/s)
: design variable for the syngas turbine, kb
: design variable for the HRSG, n (kg/s)
(Nturb)D: design variable for the wind farm
(ACUn)D: design variable for heat exchanger, CUn, of the steam network (m2)
(PowerST)D: design variable for the steam turbine (kW)
(Qcooling)D: design variable for the cooling tower (kJ/s)
: flowrate of steam, s, produced in HRSG, n, by using exhaust gas, in period t (kg/s)
: flowrate of steam, s, generated in HRSG, n by recovery heat from flue gas, Dq in period t (kg/s)
: flowrate of steam, s, produced by a syngas boiler which uses syngas, SYq,j in period t (kg/s)
Sets
SC: set for scenarios ({sc|sc = 1, …, SC} )
T: set for time period ({t|t = 1, …, T} )
S: set for types of steam produced ({s|s = LP, MP, HP, VHP} )
N: set for possible HRSG configuration in the syngas processing section to produce steam ({n|n = 1, …, N} )
J: set for reforming stage ({j|j = Pox, SR} )
Q: set for gasification stage ({q|q = IG, DG} )
K: set for syngas turbine ({k|k = GT − 10, GT − 20, GT − 50} )
Z: set for waste used to produce biogas ({z|z = MW, CM} )
L: set for heat exchanger units in the CSP plant ({l|l = EC1 EV1, …, L })
KB: set for biogas turbine ({kb|kb = GTB − 10, GTB − 20, GTB − 50} )
NB: set for possible HRSG configuration in the biogas processing section to produce steam ({nb|nb = 1, …, NB})
Keywords: renewable resources, utility plants, uncertainty, stochastic optimization, flexible designs
Citation: Pérez-Uresti SI, Martín M and Jiménez-Gutiérrez A (2021) Design of Sustainable Renewable-Based Utility Plants in the Face of Uncertainty. Front. Sustain. 2:779174. doi: 10.3389/frsus.2021.779174
Received: 18 September 2021; Accepted: 22 October 2021;
Published: 23 November 2021.
Edited by:
Mauro Antonio Da Silva Sá Ravagnani, State University of Maringá, BrazilReviewed by:
Michael Short, University of Surrey, United KingdomLinlin Liu, Dalian University of Technology, China
Copyright © 2021 Pérez-Uresti, Martín and Jiménez-Gutiérrez. This is an open-access article distributed under the terms of the Creative Commons Attribution License (CC BY). The use, distribution or reproduction in other forums is permitted, provided the original author(s) and the copyright owner(s) are credited and that the original publication in this journal is cited, in accordance with accepted academic practice. No use, distribution or reproduction is permitted which does not comply with these terms.
*Correspondence: Arturo Jiménez-Gutiérrez, arturo@iqcelaya,itc.mx