Agriculture by Irrigation Modifies Microbial Communities and Soil Functions Associated With Enhancing C Uptake of a Steppe Semi-Arid Soil in Northern Patagonia
- 1Laboratory of Soil Biochemistry and Microbiology, Center for Soil Biochemistry and Microbiology, National University of Quilmes, Buenos Aires, Argentina
- 2Instituto Nacional de Tecnología Agropecuaria (INTA) Marcos Juárez Agricultural Experiment Station, Córdoba, Argentina
- 3Gerente Técnico de Desarrollo (GTD) Proyecto Chacra Valle Irrigado Norte Patagónico (VINPA), Asociación Argentina de Productores en Siembra Directa, Santa Fe, Argentina
The transformation of the semiarid steppe soil after 5 years of intensive irrigated agriculture in Northern Patagonia was analyzed in an on-farm study. The private grower venture used conservative practices, including no-till to maintain soil structure, high crop rotation and cover crops. To characterize steppe soil changes by irrigated agriculture, we analyzed the enzymatic activities involved in the biogeochemical cycles (carbon, nitrogen, phosphorus and sulfur), the whole soil fatty acids profile, the state of soil aggregation, and the bacterial and fungal microbiota through DNA sequencing methods. After 5 years of management, irrigated agriculture soil increased organic matter (25–33%), enzymatic activities -Cellobiose-hydrolase (60–250%), Phosphatase (35–60%), Xylanase (101–185%), Aryl-sulphatase (32–100%), Chitinase (85%), β-Glucosidase (61–128%), Leucine-aminopeptidase (138%)—depending on soil series, and macro-aggregate formation at the expense of the abundance of micro-aggregates in the first 0–5 cm of soil. Whole soil fatty acids profiles changed, enhancing mono-unsaturated, branched, cyclic and methylated fatty acids. Microbial communities showed significant differences between irrigated agriculture sites and pristine valleys. The richness-based alpha-diversity established increased bacterial communities but decreased fungal communities in cultivated soil. Indicators selected using the LEfSe method revealed the bacterial taxa Acidothermus, Conexibacter and Thermoleophilum, associated with semiarid steppe soil while Asticcacaulis, Aquicella and Acromobacter with irrigated agriculture. Ascomycota Phylum changed its community composition, being both taxa Aspergillus and Alternaria reduced while Stagonospora and Metarhizium were enhanced in irrigated agriculture. Taxa belonging to Acidobacteria, Chloroflexi, and Betaproteobacteria, that were enriched in irrigated agriculture soils, were associated with higher capture of C but smaller values of aggregation, while taxa abundant on steppe soils belonging to Actinobacteria, Alphaproteobacteria, and Firmicutes were positively associated with soil aggregation but negatively with C uptake.
Introduction
Transforming non-productive areas of semiarid soil into productive agriculture fields by irrigation is an option that depends on its business balance and the challenge of improving food production without affecting soil biodiversity.
Developing a better understanding of soil biodiversity and its modification by agriculture is crucial for the rational exploitation of beneficial microbial communities to improve crop performance under water shortage conditions, which is anticipated to become even less consistent as global climate changes (1). Soil biodiversity is linked to soil organic matter (SOM), which composition, and origin are a matter of debate and ongoing studies (2, 3). It is accepted that both microbial necromass (4) and plant rhizo-deposition (5) are critical factors in SOM build-up and C sequestration. Both microbial necromass and microbial-derived compounds explain soil mineral-associated (MAOM) and particulate (POM) organic matter aggregation as a result of microbial activity (5). Soil organic matter (SOM) changes and losses have commonly been associated with soil cultivation, especially conventional agriculture practices (6). A substantial fraction of SOM is composed by extracellular polymeric substances (EPS), including polysaccharides, proteins, lipids and nucleic acids, resulting from substrate transformation by soil microbes (4, 7). EPS is claimed to be critical for initial soil particles aggregation (7) that finally determined soil structure as the result of the soil trophic interactions (8). Both bacteria and fungi showed a relevant function on soil macro-aggregate (2000-250 μm) formation, where Proteobacteria, Cyanobacteria, and Glomeromycota are key phylum for this activity (9). Fungal traits have been associated with this soil aggregation activity (10). Soil biodiversity is also associated with the maintenance of soil multifunctionality, which can be summarized by multiple ecosystem functions and services (11, 12), highlighting the importance of microbial biodiversity associated with C uptake and sequestration, both vital processes for the soil (13). Finally, within bacteria diversity, it remains the question of any bacteria, or particular taxa are most relevant for the soil ecological services (14).
In Argentina, a group of farmers succeeded to implement irrigated agriculture in the semiarid steppe in the northern part of Patagonia. They obtained promising results in terms of goods productivity in a relatively short period (7 years). We run an on-farm study analyzing the soil of the cultivated fields of this private initiative. This paper aimed to investigate the response of soil microbial communities and associated ecosystems functions to irrigated agriculture. For this, we evaluated three agricultural sites under drip irrigation for 7 years in the north Patagonia dryland region. Soil enzyme activities covering C, N, P, and S nutrient cycles and whole soil fatty acids (WSFA) were used to partially characterize soil biochemistry. Illumina high-throughput sequencing of 16S rRNA and ITS1 genes were used to estimate microbial community structures, and soil aggregation stability was used to estimate soil physical structure and biological integrated activity, since aggregation is considered the result of soil trophic interactions (7, 9). We hypothesize that (1) irrigated agriculture impacts the diversity and composition of soil bacterial and fungal communities; (2) the effects of irrigated agriculture on bacterial and fungal communities influence soil functions, changing soil enzymes profile; and (3) changes in microbial communities would modified extracellular polymeric substances production and soil trophic interactions affecting soil aggregation. The result of this study could improve our understanding of the effect of irrigated agriculture on soil microbes and ecosystems functions.
Materials and Methods
Site Description and Sampling
The on-farm study was conducted in three agricultural sites located north of the Argentinean Patagonian region, with similar histories of irrigated agriculture. The first two sites, called “Rio Negro” (RN) and “La Victoria” (LV), were located side by side, belonging to two different soil series within a circle of irrigation at the agricultural farm “Kaitaco” (39°53'3”S; 64°53'25”O) (Supplementary Figure 1). The third site, called “Chocorí” (CH), was located on the other margin of the Rio Negro river (39°59'08”S; 64°55'07”O), and both farms were located near to the city of General Conesa (Rio Negro province, Argentina) (Supplementary Figure 1). Both farms, “Kaitaco” and “Chocorí” belong to the project: “North Patagonian Irrigated Valley Farm” (VINPA) of the Argentine Association of No-Till Farmers (AAPRESID). The soils of the study area range from loamy sandy to loamy; therefore, the permeability of most soils is adequate for irrigation. The annual precipitation is about 260 mm, and the annual mean temperature is 15.6°C. The irrigation from sprinklers simulated growers' water application in the same area. The soil type, description, history of use and crop rotations can be found in Supplementary Table 1. The steppe soil reference plots were taken near the agricultural plots, 50–100 m outside the irrigation effect zone (Supplementary Figure 1). The plots were characterized as a Patagonian semi-arid steppe region, which is typically species-rich in forbs, grasses and lignified shrubs, with one grass (Festuca pallescens) and one shrub (Mulinum spinosum) contributing a large part of the total plant cover, with some species as Prosopis strombullifera characteristic for semi-arid and salted soils (15).
The soil samples were collected from two depths (0–5 and 0–20 cm) in August 2018. Ten plots were sampled in each site, five irrigated and five non-irrigated. Each plot has a 10 m2 area separated by 40 m buffer zones (Supplementary Figure 1). Twenty subsamples were randomly taken by soil sample auger in each plot, composed as a single sample and immediately sealed in plastic bags, placed in coolers, and transported to the laboratory. According to biological analysis, the soil samples were sieved (2 mm) and stored at 4°C for enzyme analyses, and at −20°C for WSFA and DNA analysis, respectively. The 0–20 cm samples were sent to the laboratory for chemical and physical analysis.
Chemical and Agronomic Parameters
The chemical analysis measured in the soil samples were soil organic matter (SOM), electrical conductivity (EC), sodium adsorption relationship (SAR), and pH. Briefly, SOM was determined by the potassium dichromate method based on Walkley and Black method (16). For EC: a filtrate was extracted from a 1:5 mixture of soil:water to measure the electrical conductivity (DSM−1). Soil pH was measured at a soil-water ratio of 1:2.5, using a compound electrode (Accumet, Westford, MA, USA). To measure SAR, we calculated the sodium (Na) concentration by flame spectrometry and the concentration of magnesium (Mg) and calcium (Ca) by colorimetry. SAR was calculated using the following equation:
Particle-Size Fractionation and Mean Weight Diameter
Soil was particle-size fractionated into five particle size classes (2000-63, 250-63, 63-20, 20-2, 2-0.1 μm) following Neumann et al. (17). Briefly, a total of 10 g dry weight was suspended in distilled water (soil/water ratio 1:5 w/v) and ultrasonicated with an energy input of 30 J mL−1. The ultrasonication was performed using an Ultrasonic Cleaner ultrasonicator (Testlab S.R.L., Argentina). The sand fraction (2000-250 and 250-63 μm) was separated from the other fractions by wet-sieving. The flow-through consisting of particles <63 μm was aliquoted to four glass tubes and centrifuged at 50 g for 15 min at 4°C. To separate clay size (<2 μm) from silt size (63-2 μm) particles, the supernatant containing the clay particles was decanted and collected in a 50 ml tube. The remaining pellets in the centrifugation tubes were resuspended in distilled water and centrifuged again. The centrifugation and suspension steps were repeated seven times with decreasing centrifugation times, 15, 13, 12, and 11 min, each twice, respectively. The last resuspended pellet was wet-sieved (by 20 μm), separating the coarse silt (63-20 μm) from the fine silt fractions (2–20 μm) in the flow-through. To enhance the flocculation of clay particles in the <2 μm supernatant, MgCl2 (final concentration 3.3 mM) was added to the 3-L beaker and kept at 4°C overnight. After decantation, the sedimented clay particles were further concentrated by centrifugation for 10 min at 2,400 g. All fractions were dried at 40°C and weighted.
The index of aggregate stability, mean weight diameter (MWD), is based on a weighted average of the five aggregate size classes, which was calculated using the following equation (18):
Where Si is the average diameter (μm) for particles in their fraction and Pi is the weight percentage of the fraction in the bulk soil.
Soil Enzymes Activities
Enzymes activities were measured according to Marx et al. (19) method, based on the use of fluorogenic MUB-substrates and microplates (20). The bulk soil was analyzed for β-cellobiohydrolase (CEL), N-acetyl-β-glucosaminidase (NAG), β-glucosidase (BGLU), α-glucosidase (AGLU), phosphomonoesterase (PME), xylanase (XYL), leucine aminopeptidase (LAP), and arylsulfatase (SUL) using 4-MUB-β-d-cellobioside, 4- MUB -N-acetyl-β-glucosaminide, 4-MUB-β-d-glucoside, 4-MUB-α-d-glucoside, 4-MUB-phosphate, 4-MUB-β-1,4-xylosidase, l-Leucine-7-amino-4-methyl coumarin, and 4-MUB-sulfatase as substrates, respectively.
Briefly, 0.1 g of soil material was mixed with 10 ml of sterile 0.1 M MES buffer (2-(N-morpholino) ethanesulfonic acid) adjusted to pH 6.1 in a 20 ml tube with five steel balls homogenizing the soil material with a shaker for 5 min at 200 rpm. Soil slurry was immediately dispensed into 96-well microplates (Thermo Scientific Nunc) with buffer, sample, reference, and substrate following a strict order and position on the plate (ISO-TS-22939, 2010). The final substrate concentration in each well was 200 μM, that was a saturating concentration for all the enzymes (this was tested in preliminary assays in our lab). All chemicals supply by Sigma-Aldrich. Fluorescence intensity was read with an excitation of 355 nm and an emission of 460 nm on a POLARstar Omega automatic microplate fluorimeter (BMGLabtech, Ortenberg, Germany) for 20 cycles of 60 s at 30 °C. Enzyme activities were calculated based on three technical replicates by each soil sample and expressed as nmol h−1 g−1 (21).
Soil Whole Fatty Acids Lipidic Profile and Estimation of Microbial Structure Biomass
Whole soil fatty acids analysis (WSFA) was run according to Ferrari et al. (22). In brief, 1 g of freeze-dried and liquid N2 milled soil sample was saponified with a NaOH-methanol mixture and methylated with HCl-methanol. After extraction with hexane/methyl tert-butyl ether (MTBE) and amendment with 33.75 μg of nanodecanoic (19:0) methyl ester as internal standard, the extract was washed with NaOH, evaporated under N2 stream, resuspended in 100 μl of hexane and injected into an Agilent 6850 gas chromatography. The oven temperature was increased from 170 to 260°C with a five °C/min ramp, followed by another ramp (40°C/min) until a final temperature of 310°C. Hydrogen and nitrogen were used as carrier and make-up gases, respectively. A phenyl-siloxane (2.5%) column was used (25 m long, 200 μm ID, 0.33 μm film) with a flame ionization detector, fed by a hydrogen-air mixture. Fatty acids were analyzed through the MIDI microbial identification protocol (Sherlock® Microbial Identification System, version 6.2 and the RTSBA6 aerobe library). Nomenclature of fatty acids and grouping by chemical functions were done according to Ferrari et al. (22). The concentration of each fatty acid identified by the MIDI software was assessed concerning the 19:0 standard and expressed as nmol g−1. The following fatty acids were used as biological marker to estimate bacteria and fungi biomass, knowing the practical limitations of this estimation, according to Ferrari et al. (22, 23): Bacteria (15:0 iso, 15:0 anteiso, 17:0iso, 17:0 anteiso, 17:0 10-Methyl); Fungi (16:1 w5c, 18:1w9c, 18:3 w6c).
DNA Extraction and Sequencing
According to the manufacturer's instructions, soil microbial DNA was extracted and purified from soil subsamples (0.5 g) using the FastDNA TM SPIN Kit for Soil (MP Biomedicals, Solon, OH, USA). Quantification and quality of the extracted DNA were specified using the NanoDrop ND-1000 spectrophotometer (Thermo Fischer Scientific, USA) and electrophoresis gel, respectively.
Soil DNA was submitted to NOVOGEN SA for amplicon sequencing. A fragment of approximately 250 bp spanning the V3-V4 region of 16S rRNA was amplified by PCR using primers 341F-806R. Sequencing of fungi was conducted using the ITS1-5F region of the ITS rRNA gene with primers ITS5-1737F and ITS2-2043R. Libraries were sequenced using an Illumina MiSeq system, generating 250 bp paired-end amplicon reads. The amplicon data were multiplexed using dual barcode combinations for each sample. Amplicons were mixed at roughly equivalent ratios based on electrophoretic band intensity and purified using Agencourt Ampure XP magnetic bead purification kit (Beckman Coulter, CA, USA).
Bioinformatics Analysis
The 16S raw reads were processed with the mothur v.1.35.0 software (24). Reads were trimmed with the following criteria: minimum length: 425 PB; minimum quality score: 25; degree of mismatching allowed: 1 mismatch to the primer and no mismatch to the barcode; homopolymers no longer than 10. Reads with ambiguous bases and singletons were removed. Chimera was checked with Uchime implemented in mothur (25) and removed from the dataset. 16S rDNA sequences were aligned and classified against SILVA bacterial SSU reference database v119. Denoised sequences were clustered into operational taxonomic units (OTUs) employing the average neighbor-clustering algorithm implemented in mothur at 97% sequence identity. The richness and diversity indexes were normalized, considering the number of sequences obtained from the smallest sample. Fungal reads were truncated to 200 bp and trimmed following the criteria described for bacterial reads using Usearch v.11 (26). Remaining sequences were collapsed into unique sequence types on a per-sample basis while preserving read counts and excluding singletons. These sequences served as the input for OTU clustering at a 97% similarity, while simultaneously removing putatively chimeras using Usearch v.11. Representative sequences of the OTUs were subjected to a similarity search against the UNITE database (27). Raw reads were deposited in the NCBI Short-Read Archive under accession number for bacteria SUB10673264 and fungi SUB10791814, BioProject PRJNA784308: Norpatagonia Agriculture project, Locus Tag Prefixes: LQ767 (SAMN23482046).
Statistical Analysis
To evaluate soil enzymatic activities and soil lipids (WSFA) analyses, permutational multivariate analysis of variance (PERMANOVA) was employed to quantitatively evaluate the effects of Site on Treatment and for calculated relative abundance data using “Adonis” function in the vegan package based on 999 permutations and method Bray-Curtis (28). Statistical analyses were performed by using R. Two-way analysis of variance (ANOVA) with Fisher's Least Significant Difference (LDS) pairwise comparisons at p < 0.05 were used to assess differences between soil uses and sites. Two multivariate methods of ordination were used to analyze enzymatic profiles and WSFA profile; LDA (Linear discriminant analysis) and PCoA (Principal component analysis) were used to assess which parameters contribute mostly to the separation of treatments. Two different data set of fatty acids were used: one built with those fatty acids present in at least 20% of all samples, in order to eliminate very rare fatty acids from the data set; the second data set comprised only those fatty acids that did not show interaction between use and site effects (Supplementary Table 2).
To evaluate the DNA data, statistical analyses were performed by using R. Two-way analysis of variance (ANOVA) with Fisher's Least Significant Difference (LDS) pairwise comparisons at p < 0.05 were used to assess differences between soil use and sites. Interactions between the main effects were evaluated using different packages (vegan, agricolae, gplots, ggplots2, RColorBrewer, edger, phyloseq). PERMANOVA analysis was based on Bray-Curtis dissimilarity using 10.000 permutations calculated from OTUs abundances. Alpha-diversity indexes (Observed richness and Shannon) were calculated per sample based on 100 times randomly subsampled read count data. The non-metric multidimensional scaling (NMDS) was also performed to visualize the most relevant patterns in microbial communities. Additionally, we tested for differential OTU abundance between agriculture and steppe communities (same thresholder OTU tables) of both kingdoms using likelihood ratio tests (LRT) with the R package edgeR (29). To graphically display the abundance distribution of the 50 most abundant genera across the different treatments, a heatmap based on centered and scaled log10-transformed relative abundances from taxon was drawn. LEfSe (Linear discriminant analysis Effect Size) analysis was conducted using the online analyzer at http://huttenhower.sph.harvard.edu/galaxy (30), to find the representative biomarker (specific abundance taxa) of different group treatment. The factorial Kruskal-Wallis test among different treatments was conducted with an α value of 0.05. The threshold on the logarithmic LDA (Linear discriminant analysis) score for discriminative features was 3.0 for bacterial and fungal communities. The strategy of “all-against-all” was used for multi-class analysis. Mantel test and Spearman correlation were used to correlating the bacterial/archaeal and fungal communities with chemical, physical, and biochemical parameters.
Results
Chemical and Physical Analysis
The physicochemical properties of the soil samples are shown in Table 1. In general, sites were significantly different according to soil properties (p < 0.001). The irrigated agriculture modified physicochemical soil properties compared to the steppe. SOM was increased in CH (25%) and LV (33%), but not statistically significant (p > 0.05). The soil pH significant decreased in CH (p = 0.0106) while the other sites showed a non-significant decrease (p > 0.05). The EC showed three times significant increase in CH (p < 0.001), while the other sites showed a non-significant decrease (p > 0.05). SAR showed a decrease in three sites (18% in CH, 17% in LV and 30% in RN) but was not statically significant in any site (Table 1).
The soil aggregation showed significant changes between the semiarid steppe and agricultural one. The MWD showed a significant increase in the three irrigated agriculture sites (p < 0.001) (Table 2). This parameter was reflected in the significant increase of the macroaggregate fraction (2000-250 μm), which also significantly increased 36%, 168%, and 188% in CH, LV, and RN, respectively (P < 0.001). The aggregate fractions 63-20, 20-2, and 2-0.1 μm presented a significant decrease in cultivated soils in CH and LV (Table 2).
Soil Enzyme Activities
Soil enzymatic profiles were different between agricultural and steppe soil, according to a PCA multivariate analysis (Figure 1 and Supplementary Table 2), being enzymes activities higher in agricultural soil than in the semiarid steppe. The increments of activities varied with sites. Average increments were observed as: SUL (32-100%); NAG (85%); XYL (101-185%); CEL (60-250%); BGLU; (61-128%); PME (35-60%) and LAP (138%) (Supplementary Table 2). Besides soil management, enzymatic profiles are also grouped according to soils series or sites under study (Figure 1). Despite similar agricultural management on two different soil series, LV and RN being part of the same agriculture irrigation circle (Supplementary Figure 1), the most relevant enzymatic activities characterizing each soil series differed. NAG and SUL activities were associated with RN soil. In contrast, all the other activities were associated with LV (Figure 1). The site CH, geographically apart from the other two and with different agricultural strategies as an alfalfa irrigated pasture instead of crop rotation, generally showed lower enzymatic activity (Figure 1 and Supplementary Table 2).
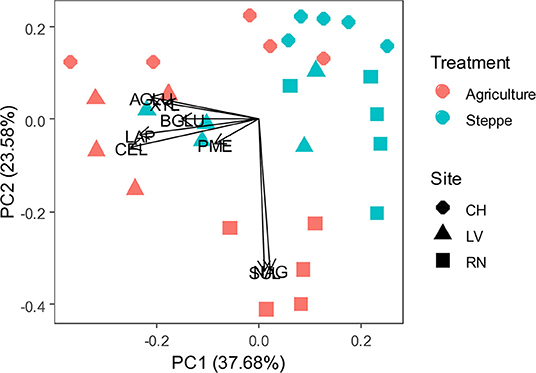
Figure 1. Principal component analysis (PCA) of soil enzymatic pattern response to irrigated agriculture and steppe soils. CEL, β-cellobiohydrolase; NAG, N-acetyl-β-glucosaminidase; BGLU, β-glucosidase; AGLU, α-glucosidase; PME, phosphomonoesterase; XYL, xylanase; LAP, leucine aminopeptidase; SUL, arylsulfatase.
Soil Lipidic Structure Measured by WSFA
The irrigated agriculture significantly increased the abundance of total WSFA, which summarize the total microbial biomass in the soil, total bacteria, and total fungi by 206.42, 145.28, and 135.50%, respectively (p < 0.01) (Figure 2). In contrast, the fungal-bacterial ratio decreased from steppe to irrigated agriculture, but the decrease was non-significant. Most relevant is the change in the whole soil lipidic composition. Out of a hundred detected fatty acids, the list was reduced to 49, deleting the rarest ones (Supplementary Table 3). A PERMANOVA analysis of this list showed significant effect by treatment (P 0.001) and by the site (P 0.002) and showed significant site-treatment interaction (Table 3A). An LDA analysis showed clear discrimination among soil use and management and sites (Figure 3A). The fatty acid with more significant loading on LDA showed a different pattern between soil use. MUFAs (14:1ω5c, 16:1ω7c alcohol, 17:1ω5c, 17:1ANTEISOω9c, 17:1ANTEISO A, 17:1ω7c, 18:1ω7c, 18:1ω9c characterized cultivated soil, while linear fatty acids (14:0, 16:0, 17:0), PUFA 20:4ω6,9,12,15c -usually considered marker for mesofauna- and 16:1ω5c -marker for arbuscular mycorrhiza- characterized steppe native soil. Analyzing the site-treatment interaction for each fatty acid in the WSFA profile, it was possible to reduce the WSFA list to a group of 31 fatty acids that did not show a significant site-treatment interaction (Supplementary Table 3). The PERMANOVA run on this new data set showed significant treatment effect (P 0.001) and site effect (P 0.003) without site/treatment interaction (Table 3B). LDA or PCA multivariate analyses showed clear separation of samples between irrigated agriculture and steppe soils (Figure 3B). This separation, suggesting a different lipidic structure between soils, was sustained by PCA when the fatty acids were grouped by their chemical function [see M&M and (22)]. The PC1 and PC2 of this new analysis explained 36.5 and 16.5% of the total variation, respectively. The samples were separated by treatment according to axis PC1, while the sites were mainly separated according to PC2 (Figure 3B).
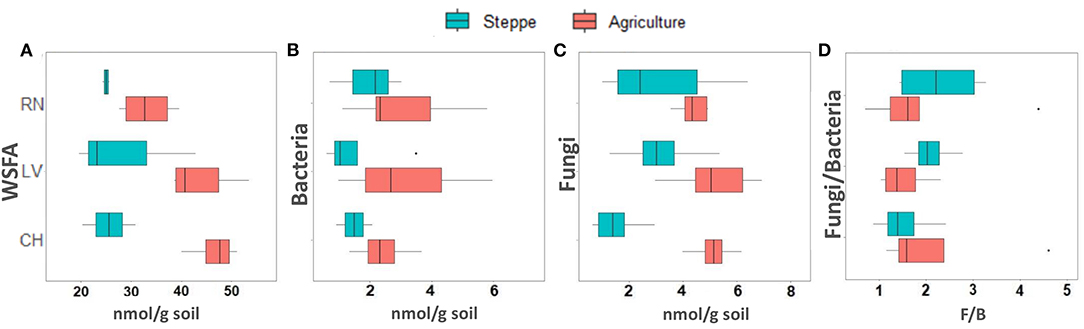
Figure 2. Boxplots showing (A) total WSFA, (B) total bacteria, (C) total fungi, and (D) fungi-bacteria ratio estimated from WSFA.
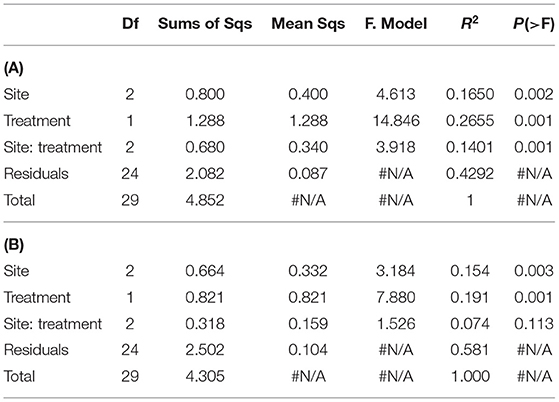
Table 3. PERMANOVA analysis on (A), trimmed list of detected WSFA eliminating the rare ones -present in less than 20% of the analyzed samples- (see M&M) (n = 49); and on (B), trimmed list of detected WSFA that did not show site-treatment interaction.
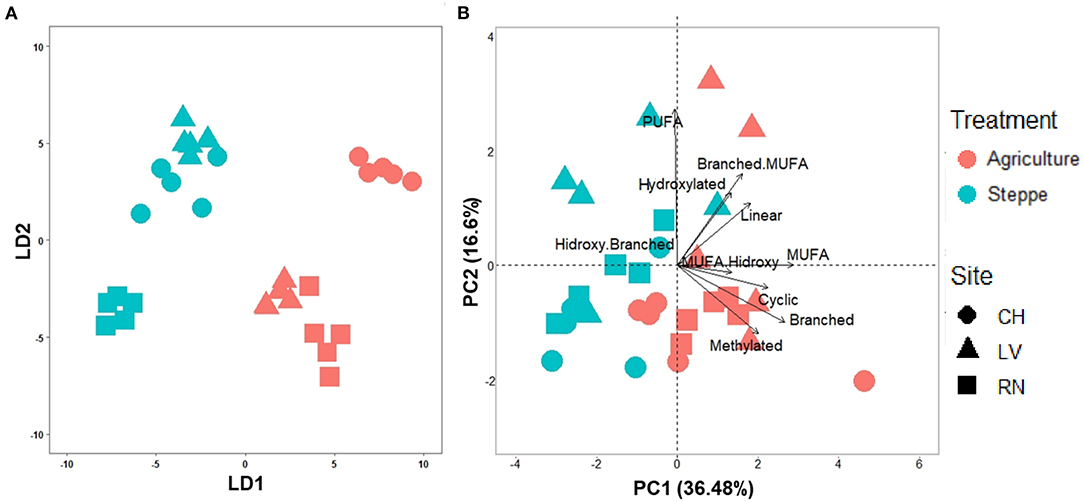
Figure 3. (A) Lineal discriminant analysis (LDA) plots for the soil samples considering the trimmed fatty acids profile (49 fatty acids), and (B) Principal component analysis (PCA) plot of soil samples based on fatty acids grouped by their chemical functions for irrigated agriculture and steppe soils.
Soil Microbial Structure Measured by Soil DNA Sequencing
Bacterial and Fungal Community Diversity
Bacterial/archaeal diversity showed significant differences between irrigated agriculture and steppe treatments (Figures 4A,B). The agricultural treatment increased the diversity in both indices, richness, measured by Observed index (p = 0.016) and Shannon (p = 0.001) indexes. The Shannon index showed significant differences for the site (p < 0.001) and the interaction among both factors (p = 0.03), too. Only RN presented significant differences between treatments when the Shannon index was analyzed in each site (p < 0.001). For the fungal community, the diversity showed significant differences between treatments (p = 0.0152) and interactions among treatment and site (p = 0.0125) for richness, with a tendency to reduce diversity by irrigated agriculture. Only CH showed significantly higher diversity in the steppe (p < 0.001) (Figure 4C). In contrast, the Shannon index did not show significant differences between treatments or sites (p > 0.05) (Figure 4D).
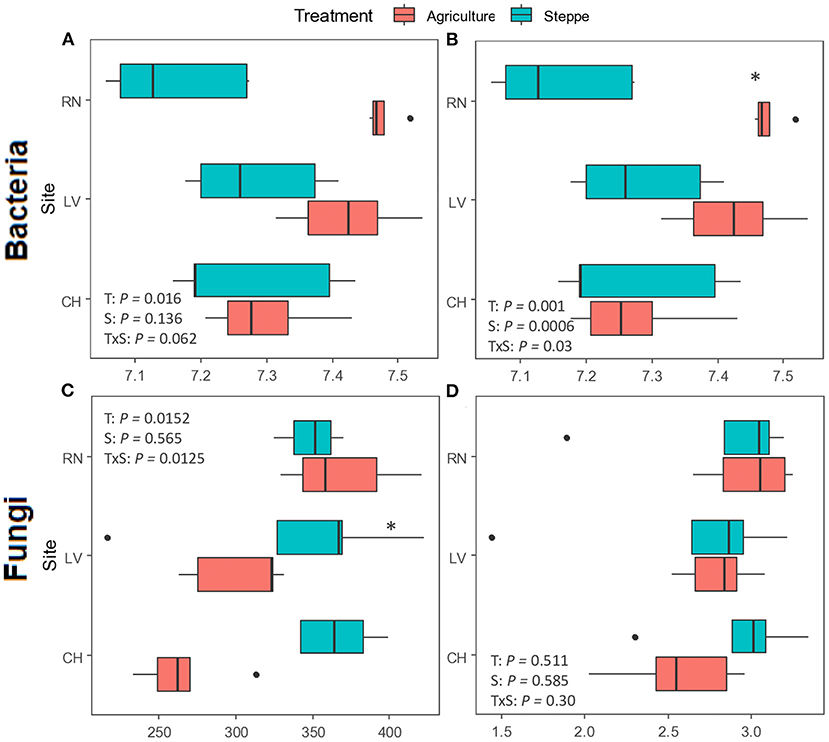
Figure 4. Alpha diversity measures of the bacteria (A,B) and fungi (C,D) communities in irrigated agriculture and steppe. Richness (observed OTUs) and diversity (Shannon-Wiener) indices were evaluated in both treatments (n = 5). Boxes represent 25–75% of the data, solid lines the median, dots in the box mean, the tips represent the minimum and maximum values excluding the outliers (1.5 times lesser or greater than the lower or upper quantiles) represented by dots outside of the boxes. Data were tested for significance by two-way ANOVA followed by Fisher‘s LSD test. *Denote significant differences between irrigation agriculture and steppe (p < 0.05).
We visualized and quantified the differences between microbial communities (β-diversity) using unconstrained non-metric multidimensional scaling (NMDS) and permutational multivariate analysis of variance (PERMANOVA) on Bray-Curtis dissimilarities. The PERMANOVA showed similar differences between treatments (p < 0.001) and sites (p < 0.001). The PERMANOVA results showed that sites explained 23.2% for bacteria and 10.9% for fungi's variation while the treatment explained 35.6 and 28.4% of the variation between samples for bacteria and fungi, respectively (Supplementary Table 4). The NMDS analysis showed that the communities were spatially structured along with the soil treatment, followed by the effects of sites (Figures 5A,B). Similarly, the Edger analysis showed that irrigated agriculture and steppe presented different microbial habitats with specific sets of microbes (Figures 5C,D). Irrigated agriculture and steppe soils shared 90.74 and 52.84% of the OTUs for bacteria/archaea and fungi, respectively. The steppe presented more unique fungi sequences than agriculture, while the opposite trend appeared for bacteria/archaea (Supplementary Figures 2A,B).
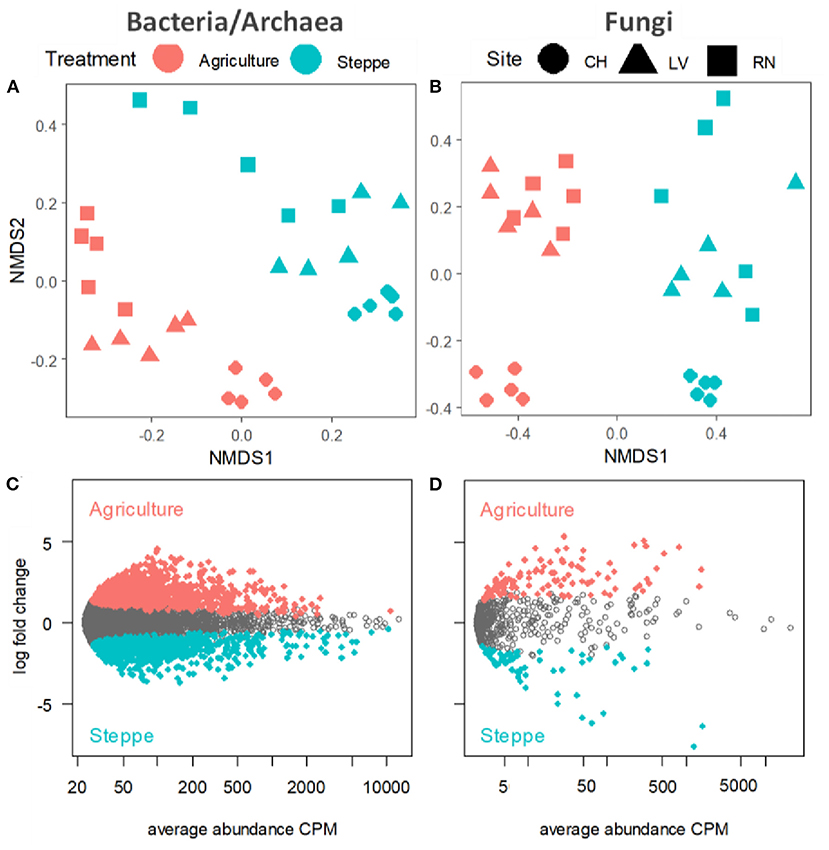
Figure 5. Non-metric multi-dimensional scaling (NMDS) plots of community composition in irrigated agriculture and steppe plots in each site for (A) bacteria/archaea and (B) fungi. Plots display the abundance patterns of (C) bacteria and (D) fungi in irrigated agriculture and steppe soil. X-axis reports average OTU abundance (as counts per million, CPM), and Y-axis log2-fold change. Agriculture and steppe-specific OTUs were colored in redish and greenish, respectively, and non-differentially abundant OTUs are in gray (likelihood ratio test, p < 0.05, FDR corrected).
Bacterial and Fungal Community Structure
The analysis of the taxonomic composition on bacterial phylum level revealed that Proteobacteria (34.67%), Actinobacteria (32.02%), Bacteroidetes (9.33%), Acidobacteria (9.13%), Gemmatimonadetes (4.25%), and Firmicutes (4.20%) were dominating in all soil samples. These taxa accounted for >93% of the bacterial sequence in all treatments (Supplementary Figure 3A). Proteobacteria, Bacteroidetes, TM7, and Nitrospira showed a significantly higher abundance in agricultural treatment (p < 0.05), while Actinobacteria, Gemmatimonadetes, Verrucomicrobia, Planctomycetes, and Armatimonadetes were significantly higher in the steppe (p < 0.05). Acidobacteria only showed a significant difference in CH, being higher in agriculture. Finally, Firmicutes did not present any significant differences. All the bacterial phylum presented significant differences between sites (P < 0.05), except for Verrucomicrobia, Planctomycetes, and Armatimonadetes.
The fungal community showed that the most abundant phyla were Ascomycota (53.48%), Basidiomycota (21.17%), fungi unclassified (21.07%), and Mortierellomycota (2.41%). These taxa accounted for >96% of the fungal sequences in all treatments. Considering the average of the relative phyla abundances, Mortierellomycota and Aphelidiomycota quantities significantly increased (226 and 100%, respectively), while the Basidiomycota and Chytridiomycota decreased (−55 and −60%, respectively), in irrigated agricultural soils compared to steppe values (Supplementary Figure 3B). Fungal phyla did not present statistical differences in abundance between sites, except for the phylum Kickxellomycota (p = 0.02517).
For bacterial/archaeal and fungal analysis, the analysis based on genus cluster heatmap (Figure 6) showed a similar pattern as NMDS (Figures 5A,B), where the samples clustered according to treatment and then by the site. The genus analysis showed significant differences between soil treatments for Actinobacteria, Rubrobacter and Rhizobiales unclassified, and Acidobacteria (Solibacterales, GP3, GP4, Gp6, Gp7, and Gp10) (Figure 6A). Most of the detected genera were unclassified. Those enhanced in steppe were related to functional traits that support desiccation and can sporulate; meanwhile, those enhanced in irrigated agriculture belong to Proteobacteria genera known to be rhizospheric or plant-associated bacteria, probably an interaction enhanced in the irrigated agricultural plots. Some fungal genera identified with steppe treatment were Fusariella, Exophiala, Gelasinospora, Rhizophlyctis, Lectera, Blumeria, Cyphellophora, Chaetonium, Penicillium, while agriculture treatment enhanced Byssochlamys, Aspergillus, Alternaria, Trichoderma, Nosetophorma, Ascobolum, and Coriochaeta (Figure 6B).
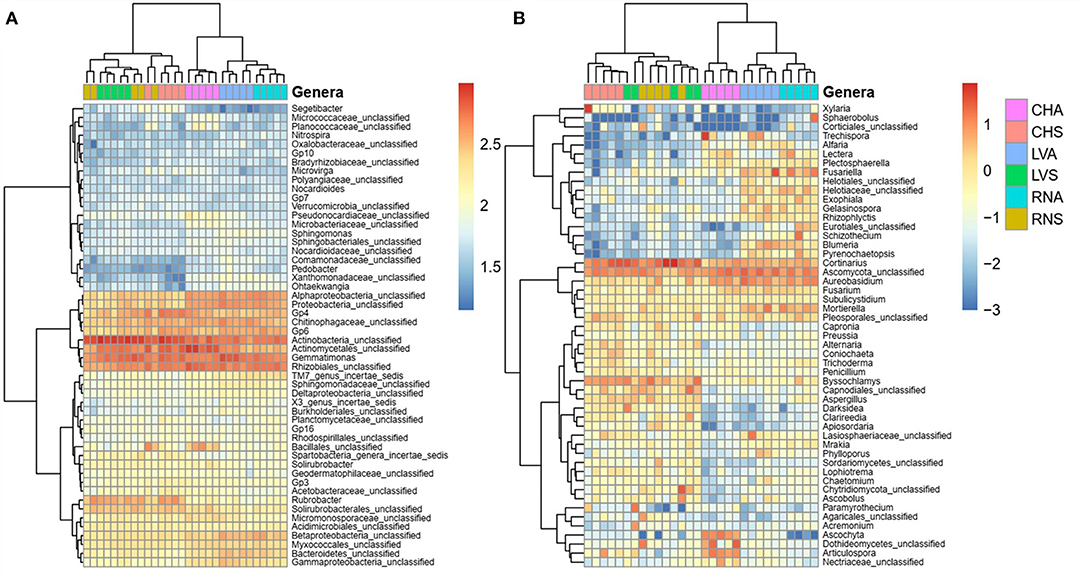
Figure 6. Heatmap showing the abundances of the 50 most abundant (A) bacteria/archaea and (B) fungi genera in irrigated agriculture and steppe soils under. Data were centered and scaled to the mean of each taxon's log-transformed relative abundance. Top dendrogram cluster samples according to the community composition. Side dendrogram cluster taxa according to their relative abundance distribution across samples.
Bacterial and Fungal Community Indicators
After LefSe analysis, a total of 4 and 8 bacterial taxa with LDA scores of >3 were selected as biomarkers of steppe and agriculture treatments, respectively (Figure 7A). The bacterial taxa associated with steppe were Acidothermus, Conexibacter, Thermoleophilum (all Actinobacteria), Terrimonas (Bacteroidetes), and Chelatococcus (Alpha-proteobacteria). In contrast, agriculture biomarkers were associated with Ammoniphilus (Firmicutes), Sphingomonas and Asticcacaulis (both Alpha-proteobacteria), Xanthomonas and Aquicella (both Gamma-proteobacteria), and Achromobacter (Beta-proteobacteria) (Figure 7A). Regarding the 28 fungal biomarkers detected, the predominant fungal phylum was Ascomycota, with 25/28 representatives. The steppe soil was associated with 15 fungal biomarkers like Darksidea, Alternaria, Lophiostoma, Lapidomyces, Neostagonospora, Bipolaris, Neodidymelliopsis (Dothideomycetes, Ascomycota), Byssochlamys, Aspergillus, Penicillium (Eurotiomycetes, Ascomycota), Xylaria, Coniochaeta, Apiosordaria (Sordariomycetes, Ascomycota), Clarireedia (Leotiomycetes, Ascomycota), and Cortinarius (Agaromycetes, Basidiomycota). For agricultural irrigated soil, the biomarkers identified (13) were Macroconia, Alfaria, Plectosphaerella, Fusarium, Lectera, Fusariella (Sordariomycetes, Ascomycota), Cladosporium, Neosetophoma, AureobasidIum (Dothideomycetes, Ascomycota), Cyphellophora (Eurotiomycetes, Ascomycota), Entoloma (Basidiomycota), and Rhizophlyctis (Chytridiomycota) (Figure 7B).
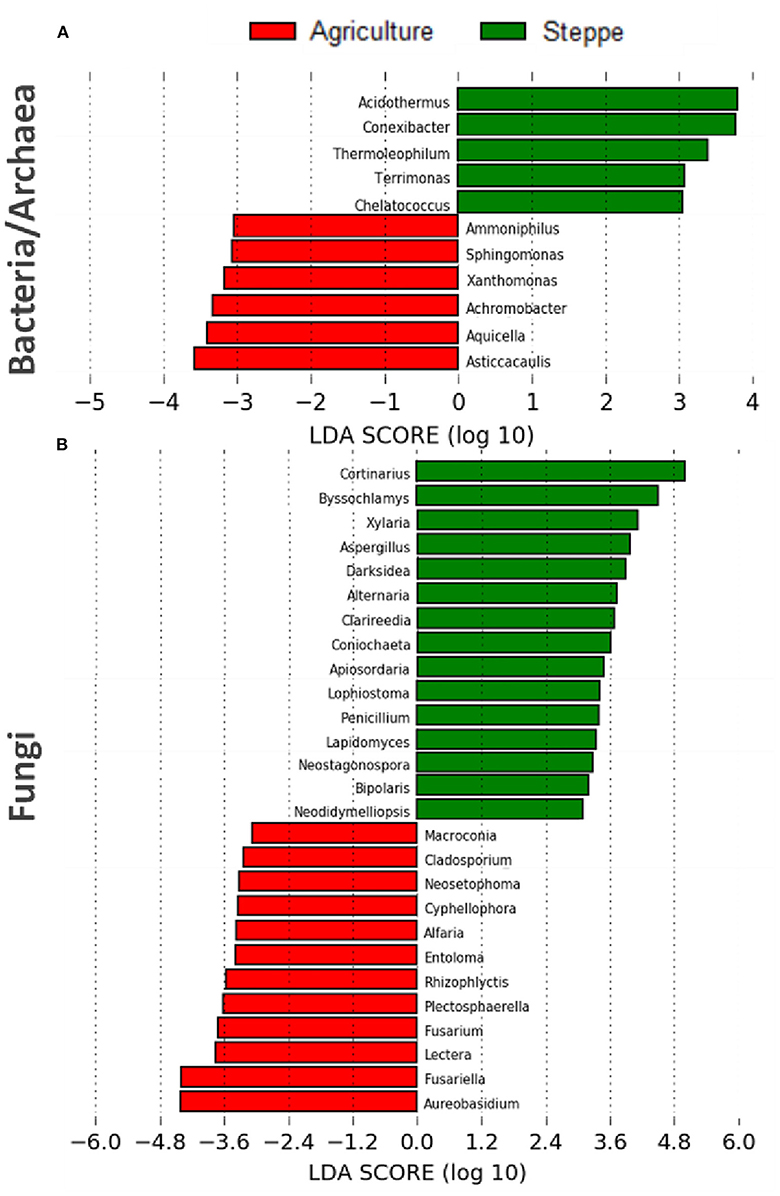
Figure 7. Linear discriminant analysis effect size (LEfSe) analysis showing (A) bacterial/archaea and (B) fungal microbiota changes between the irrigated agriculture and steppe (n = 15).
Correlation Analysis
Mantel test showed that soil variables significantly influenced the soil microbial community structures. MWD (F = 0.294, p = 0.001), SOM (F = 0.199, p = 0.004), and SAR (F = 0.197, p = 0. 009) jointly shaped the bacterial community structure, while MWD (F = 0.192, p = 0.003) has significantly affected the fungal community structure (Table 4).
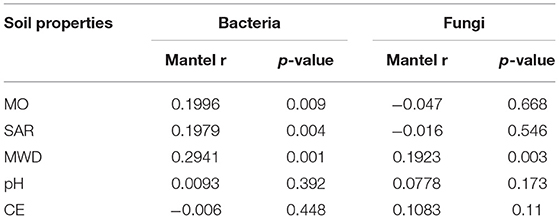
Table 4. Mantel correlation between bacteria/archaea and fungi with soil physicochemical properties.
The SOM significantly positively correlated with Proteobacteria, Gemmatimonadetes, Nitrospira, OP1, and OD1 while negatively correlated with Chloroflexi (Figure 8A). The SAR significantly correlated with Proteobacteria, Acidobacteria, Gemmatimonadetes, Nitrospira, OP1, and BCR1 while negatively correlated with Chloroflexi (Figure 8A). MWD showed a positive correlation with Actinobacteria, Chloroflexi, WS3, and TM7. MWD negatively impacted on Acidobacteria, Chlorobi, Deinococcus-Thermus, Planctomycetes, and Spirochaetes. Some Bacteria phyla correlated positively with different enzymes (p < 0.05): Proteobacteria with LAP and NAG, Actinobacteria with BGLU; Acidobacteria with NA; Verrucomicrobia with BGLU; Firmicutes with XYL and CEL; OP11 with LAP, NAG and SUL; TM7 with CEL, SUL and PME; and WS3 with BGLU and SUL. Other phyla show negative correlations with enzymes (p < 0.05): Acidobacteria with XYL and BGLU, Deinococcus-Thermus with BGLU and SUL, Chloroflexi with LAP and NAG, Chlorobi with SUL, Chlamydiae with CEL; and Spirochaetes with BGLU and PME (Figure 8A). Within the fungal phylum, SOM positively correlated with Mortierollomycota, Chytridiomycota, and Kickxellomycota (Figure 8B). Mortierollomycota and Kickxellomycota negatively correlated with SAR. MWD positively correlated with Rozellomycota and negatively correlated with Basidiomycota. Different phyla positively correlated with different soil enzyme activities: Ascomycota with CEL; Chytridiomycota with LAP, Mortierollomycota with LAP, NAG and CEL; Rozellomycota with XYL, BGLU and SUL; and Zoopagomycota with BGLU. Basidiomycota was the only fungal phylum that negatively correlated with CEL and BGLU (Figure 8B).
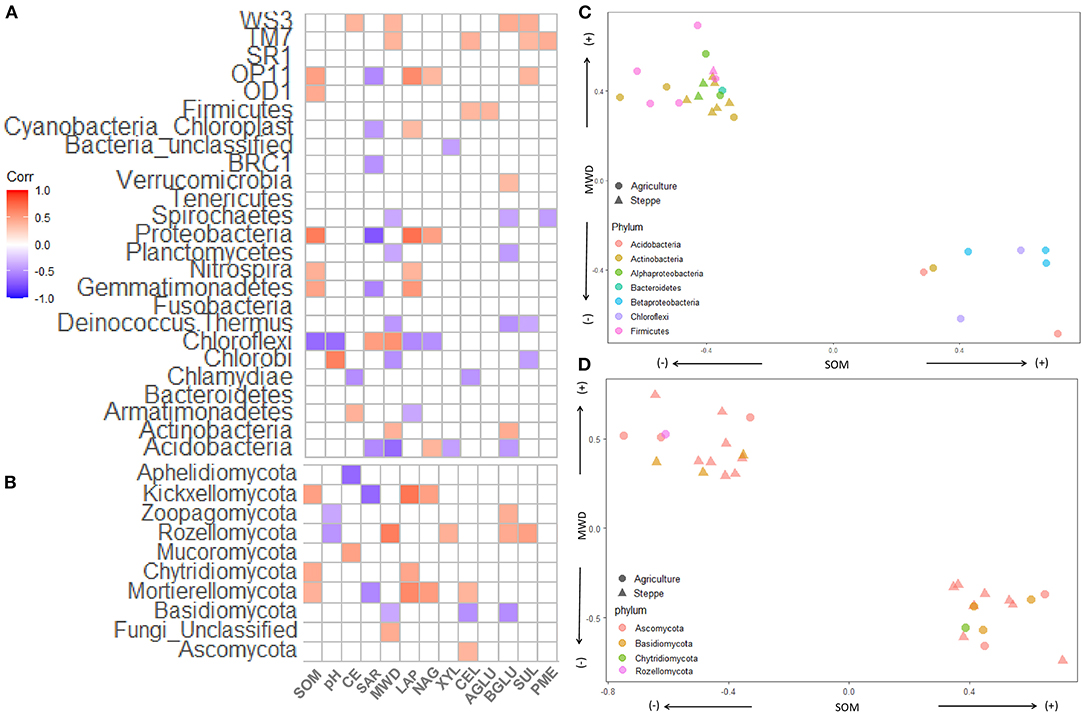
Figure 8. Correlation heatmap of (A) bacterial/archaea and (B) fungal and soil properties. The shade of color indicates the correlation between relative abundance of each microbiota and soil physical and chemical parameters. (C,D) Showing spearman correlation between bacteria/archaea or fungi, respectively, with MWD in the y axis and SOM in the x-axis. SOM, soil organic matter; CE, cation exchange; SAR, sodium absorption ratio; CEL, β-cellobiohydrolase; NAG, N-acetyl-β-glucosaminidase; BGLU, β-glucosidase; AGLU, α-glucosidase; PME, phosphomonoesterase; XYL, XYLanase; LAP, leucine aminopeptidase; SUL, arylsulfatase.
Finally, we plot the bacteria/archaeal (Figure 8C) and fungal (Figure 8D) genus, which significantly correlated with SOM and MWD. The phylum and classes Beta-Proteobacteria (3), Chloroflexi (2), Acidobacteria (2), and Actinobacteria (1), which were more abundant in agriculture, presented a positive correlation with SOM and negatively correlated with MWD. While Actinobacteria (9), Alpha-proteobacteria (6), Firmicutes (4), and Bacteroidetes (1) negatively correlated with SOM and positively with MWD (Figure 8C). At the fungal level, Ascomycota, Basidiomycota, and Rozellomycota genus correlated positively and negatively with SOM and MWD (Figure 8D). Additionally, one Chytridiomycota genus positively correlated with SOM and negatively with MWD.
Discussion
This study examined the soil biochemistry and biological diversity of bacterial/archaeal and fungi after converting semiarid steppe in the north of Patagonia into cropland by no-till irrigated agricultural systems. A general soil microbial structure estimated from WSFA showed a shift toward bacterial dominance in irrigated agriculture soils. According to irrigated agriculture, the modification observed in the microbial community structure strongly shifted enzymatic profiles. An apparent increase of biological activities was observed for hydrolases as SUL, XYL, CEL, BGLU, PME, most probably related to water availability and crop rhizodeposition.
Monounsaturated, branched cyclic and methylated fatty acids were enhanced by irrigated agriculture in SOM. This modification of fatty acids profile seems to indicate soil health improvement (Wall et al., unpublished). Additionally, the changes observed in the WSFA profile also suggested changes in soil organic matter processed by the soil microbiota, as suggested by Liang et al. (3, 4). The enhancement of soil aggregation found at the macroaggregate fraction (2000-250 mm) could also be related to the change in the WSFA profile since WSFA would be part of soil EPS involved in soil aggregation (7).
The introduction mentioned that biodiversity is crucial in sustainable agroecosystems to maintain soil ecosystems services (12). The microbiome structure obtained from soil DNA sequencing confirmed that semiarid steppe and irrigated agriculture soil significantly differed on bacterial and fungal community abundance, showing two different niches probably due to new physical and chemical conditions. Irrigated agriculture had a different impact according to the microbial kingdom at alpha-diversity indexes (richness and Shannon), increasing bacterial diversity while the fungal diversity tent not to change or decrease (31). Irrigated agriculture significantly altered soil-borne fungi resources and environment, affecting diversity patterns (32–34). Indeed, decrement in fungal diversity due to fertilization has been broadly reported (32, 35), and such response is associated with the alteration of soil nutrient and carbon conditions (32, 36–38). Our study is consistent with other studies that showed a change in bacterial diversity associated with the amount of soil water (39).
Proteobacteria and Bacteroidetes are two significant phyla that positively respond to cover vegetation (40–42), while Mortierellomycota can be saprophytic on the residual limbs of plants and animals and decompose their remains (43). Proteobacteria and Bacteroidetes from bacteria and Mortierellomycota from fungi significantly increased in irrigated agricultural plots. Furthermore, Proteobacteria showed a significant correlation with SOM in LV and RN sites. On the opposite, Actinobacteria, Gemmatimonadetes, Verrucomicrobia, Planctomycetes, and Armatimonadetes for bacteria and Basidiomycota for fungi were higher in the semiarid steppe and decreased in irrigated agriculture. These phyla have been recognized as oligotrophy with a slow growth rate (23, 44) and had been displaced by copiotroph bacteria like Proteobacteria and Bacteroidetes (45) in the cultivated plots.
Agricultural practices can substantially impact symbiotic species more than saprotrophic fungi (46). According to a study conducted by Chen et al. (47) along a chronosequence after converting a desert area to irrigated agriculture, the shift in the fungal community composition can be extended for decades. While species belonging to Ascomycota are mainly ubiquitous, Basidiomycota and Zygomycota are less abundant in cropping ecosystems (36, 47, 48). The LefSe analyses in the fungal community showed that Cortinarius (Basidiomycota), the largest and most species-rich genus of ectomycorrhizal fungi in Patagonia (49), appeared as an indicator of the semiarid steppe soil but not of the agricultural sites. Before agriculture adoption, the pristine steppe was characterized by lignified shrubs, putative hosts for Cortinarius ectomycorrhizae. Therefore, we infer that the reduction of Basidiomycota abundances in irrigated agricultural sites can be related to substituting of autochthonous vegetation, crucial hosts for symbiotic Basidiomycota species.
The bacterial biomarkers identified by LEfSe in agricultural soils belong mainly to Proteobacteria, which are associated with the soil degradation and decomposition of plant residues (50). These results agree with Bastida et al. (39) and Frenk et al. (51). The bacterial biomarkers associated with the steppe, such as Terrimonas, Acidothermus, and Conexibacter, have been associated with well-developed (52) and enriched soil (50), probably related in our case to the development and evolution of these alluvial soils along the Valley of Río Negro. Actinobacteria played a vital role in the desert (12) related to this semiarid steppe environment. These results were confirmed by the heatmaps analysis, showing the most remarkable differences at Actinobacteria phylum. On the other side, LefSe analyses in the fungal community showed Cortinarius as an indicator of the semiarid steppe soil, which is considered the largest and most species-rich genus of the ectomycorrhizal fungi in Patagonia (49). The steppe was characterized by lignified shrubs, putative hosts for those ectomycorrhizae. Likewise, Apiosordaria, Lophiostoma and Penicillium have been identified as putative drought tolerance endophytes (53–55) were also indicators of the steppe soil. On the other hand, the differential abundance of fungi in the irrigated agriculture situation was represented by taxa belonging to generalist saprotrophic genera such as Fusarium, commonly found in croplands (48, 56). The improvement in soil water conditions under irrigated fields was also indicated by the significant increase of Rhizophlyctis abundance, which augmented sporulation in aqueous soil extracts (57).
Bacteria and Fungi have been described as the critical factor in soil aggregation, particularly at the macroaggregate (2000-250 mm) level (9). Soil aggregation is an important property involved in C sequestration and stabilization (2), where macro-aggregates enhanced these processes, either protecting C stock from degradation (58) or creating the niche for C transformation and final uptake (3). Soil aggregation, measured by Mean Weight Diameter (MWD), was more important than other properties in the shaping of bacterial and fungal communities. Regarding bacteria, Chloroflexi, Actinobacteria, WS3 and TM7 taxa were positively associated with soil aggregation, in agreement with a previous works that showed a significant role of Actinobacteria and Proteobacteria in soil aggregate formation (9). Edaphic properties are among the main drivers of fungal diversity and community composition (59). For example, moderately acidic soils have been identified as more favorable for saprotrofic (59) and pathogenic fungi (60). Fungal hyphae adhere to and physically entangle soil particles and stabilize micro-and macro-aggregates by releasing extracellular compounds (61). Basidiomycota displayed an insufficient capacity to form aggregates compared to other main phyla due to their low, dense mycelia (10). Hence, this trait can be associated with the negative correlation between MWD and Basidiomycota observed in this study.
In our study, we found negative correlations between soil pH and Rozellomycota and Zoopagomycota abundances. Considering that both phyla are mainly obligate mycoparasites and predators of nematode, amebae and protists (62), they might have responded indirectly to habitat preferences of host organisms. Probably, the shift within the whole fungal community driven by specific mycoparasitism by Rozellomycota may have reduced the secretion of fungal compounds that detrimentally affect soil aggregation (10, 63). However, in the absence of direct experimental evidence, we cannot explain the positive associations between SOM and the saprotrophic decomposers phyla Kickxellomycota, Chitridiomycota, and Morteriellomycota. Despite our observations being in line with previous studies in agricultural soils (64, 65), the causal mechanism behind the correlations remains uncertain, and further evidence is necessary to disentangle the role of minor phyla in soil C cycling, EPS synthesis and aggregate formation.
Conclusions
Agricultural systems adopting no-tillage, cover crops, and crop rotations, and in this particular case, sustained by irrigation, improved ecosystem biodiversity and soil organic matter content. Consequently, enhanced natural soil fertility and the biodiversity of microbial communities. Our detection of particular taxa related to those soil improvements suggests that similar analyses worldwide would help finding new soil health indicators based on biological data obtained from the study of the soil microbiome in agricultural systems. Understanding the microbial and biochemical soil changes associated with agriculture managements would help find keystone microbial markers and soil biological indicators that would allow farmers to make decisions on the sustainability of the productive processes while keeping soil healthy.
Data Availability Statement
The datasets presented in this study can be found in online repositories. The names of the repository/repositories and accession number(s) can be found at: https://www.ncbi.nlm.nih.gov/, SAMN23482046.
Author Contributions
JF: bacteria/archaea community analysis and correlations between soil variables—manuscript writing. VF: fungi community analyses—contribution to manuscript writing. JC and DR: soil lipids determination and data analysis. LG: soil sampling, soil enzymes activities determination and data analysis, and manuscript revision. MG: field assay supervision, chemical analyses, and guide for soil sampling. LW: general idea and study design, laboratory analyses and data supervision, discussion of results, and Manuscript writing and edition. All authors contributed to the article and approved the submitted version.
Funding
This work was supported by grants PUNQ EXPTE 1306/19 and project PICT 2803/17 of the Argentinean National Agency for Scientific and Technological Promotion (ANPCyT). LW and LG are members of the Argentinean National Council for Scientific and Technical Research (CONICET).
Conflict of Interest
The authors declare that the research was conducted in the absence of any commercial or financial relationships that could be construed as a potential conflict of interest.
Publisher's Note
All claims expressed in this article are solely those of the authors and do not necessarily represent those of their affiliated organizations, or those of the publisher, the editors and the reviewers. Any product that may be evaluated in this article, or claim that may be made by its manufacturer, is not guaranteed or endorsed by the publisher.
Acknowledgments
The authors are especially grateful to Chacra VINPA-AAPRESID in Argentina, that manage the agricultural production by irrigation and allowed this on farm study, contributing with the chemical soil data. Farms Kaitaco, La Julia and Chocorí also contributed with this work.
Supplementary Material
The Supplementary Material for this article can be found online at: https://www.frontiersin.org/articles/10.3389/fsoil.2022.835849/full#supplementary-material
References
1. de Vries FT, Liiri ME, Bjørnlund L, Bowker MA, Christensen S, Setälä HM, et al. Land use alters the resistance and resilience of soil food webs to drought. Nat Clim Chang. (2012) 2:276–80. doi: 10.1038/nclimate1368
2. Cotrufo MF, Soong JL, Horton AJ, Campbell EE, Haddix ML, Wall DH, et al. Formation of soil organic matter via biochemical and physical pathways of litter mass loss. Nat Geosci. (2015) 8:776–9. doi: 10.1038/ngeo2520
3. Liang C, Kästner M, Joergensen RG. Microbial necromass on the rise: the growing focus on its role in soil organic matter development. Soil Biol Biochem. (2020) 2020:108000. doi: 10.1016/j.soilbio.2020.108000
4. Liang BC, VandenBygaart AJ, MacDonald JD, Cerkowniak D, McConkey BG, Desjardins RL, et al. Revisiting no-till's impact on soil organic carbon storage in Canada. Soil Tillage Res. (2020) 198:104529. doi: 10.1016/j.still.2019.104529
5. Villarino SH, Pinto P, Jackson RB, Piñeiro G. Plant rhizodeposition: A key factor for soil organic matter formation in stable fractions. Sci Adv. (2021) 7:eabd3176. doi: 10.1126/sciadv.abd3176
6. Bot A, Benitez J. The Importance of Soil Organic Matter. Key to Drought-Resistant Soil and Sustained Food Production. Rome: FAO Soils Bulletin (2005).
7. Costa OYA, Raaijmakers JM, Kuramae EE. Microbial extracellular polymeric substances: ecological function and impact on soil aggregation. Front Microbiol. (2018) 9:1636. doi: 10.3389/fmicb.2018.01636
8. Erktan A, Or D, Scheu S. The physical structure of soil: Determinant and consequence of trophic interactions. Soil Biol Biochem. (2020) 2020:107876. doi: 10.1016/j.soilbio.2020.107876
9. Lehmann A, Zheng W, Rillig MC. Soil biota contributions to soil aggregation. Nat Ecol Evol. (2017) 1:1828–35. doi: 10.1038/s41559-017-0344-y
10. Lehmann A, Zheng W, Ryo M, Soutschek K, Roy J, Rongstock R, et al. Fungal traits important for soil aggregation. Front Microbiol. (2020) 10:2904. doi: 10.3389/fmicb.2019.02904
11. Hector A, Bagchi R. Biodiversity and ecosystem multifunctionality. Nature. (2007) 448:188–90. doi: 10.1038/nature05947
12. Wagg C, Dudenhöffer J, Widmer F, van der Heijden MGA. Linking diversity, synchrony and stability in soil microbial communities. Func Ecol. (2019) 32:1280–92. doi: 10.1111/1365-2435.13056
13. Domeignoz-Horta LA, Pold G, Liu XJA, Frey SD, Melillo JM, et al. Microbial diversity drives carbon use efficiency in a model soil. Nat Comm. (2020) 11:1–10. doi: 10.1038/s41467-020-17502-z
14. Hartman K, van der Heijden MGA, Wittwer RA, et al. Cropping practices manipulate abundance patterns of root and soil microbiome members paving the way to smart farming. Microbiome. (2018) 6:14. doi: 10.1186/s40168-017-0389-9
15. Aguiar MR, Paruelo JM, Sala OE, Lauenroth WK. Ecosystem responses to changes in plant functional type composition: An example from the Patagonian steppe. J. Veget. Sci. (1996) 7:381–90. doi: 10.2307/3236281
17. Neumann D, Heuer A, Hemkemeyer M, Martens R, Tebbe CC. Response of microbial communities to long-term fertilization depends on their microhabitat. FEMS ME. (2013) 86:71–84. doi: 10.1111/1574-6941.12092
18. van Bavel CHM. Mean Weight-Diameter of soil aggregates as a statistical index of aggregation. Soil Sci Soc Am J. (1950) 14:20–3. doi: 10.2136/sssaj1950.036159950014000C0005x
19. Marx MC, Wood M, Jarvis SC. A microplate fluorometric assay for the study of enzyme diversity in soils. Soil Biol Biochem. (2001) 33:1633–40. doi: 10.1016/S0038-0717(01)00079-7
20. Truong C, Gabbarini LA, Corrales A, Mujic AB, Escobar JM, Moretto A, et al. Ectomycorrhizal fungi and soil enzymes exhibit contrasting patterns along elevation gradients in southern Patagonia. New Phytol. (2019) 222:1936–50. doi: 10.1111/nph.15714
21. DeForest JL. The influence of time, storage temperature, and substrate age on potential soil enzyme activity in acidic forest soils using MUB-linked substrates and L-DOPA. Soil Biol Biochem. (2009) 41:1180–6. doi: 10.1016/j.soilbio.2009.02.029
22. Ferrari AE, Ravnskov S, Wall LG. Crop rotation in no-till soils modifies the soil fatty acids signature. Soil Use Manag. (2018) 34:427–36. doi: 10.1111/sum.12440
23. Fierer N, Bradford MA, Jackson RB. Toward an ecological classification of soil bacteria. Ecology. (2007) 88:1354–64. doi: 10.1890/05-1839
24. Schloss PD, Westcott SL, Ryabin T, Hall JR, Hartmann M, et al. Introducing mothur: open-source, platform-independent, community-supported software for describing and comparing microbial communities. Appl Environ Microbiol. (2009) 75:7537–741. doi: 10.1128/AEM.01541-09
25. Edgar RC, Haas BJ, Clemente JC, Quince C, Knight R. UCHIME improves sensitivity and speed of chimera detection. Bioinformatics. (2011) 27:2194–200. doi: 10.1093/bioinformatics/btr381
26. Edgar RC. Search and clustering orders of magnitude faster than BLAST. Bioinformatics. (2010) 26:2460–1. doi: 10.1093/bioinformatics/btq461
27. Nilsson RH, Larsson K.-H., Taylor AFS, Bengtsson-Palme J, Jeppesen TS, et al. The UNITE database for molecular identification of fungi: handling dark taxa and parallel taxonomic classifications. Nucleic Acids Res. (2018) 47: D259–64. doi: 10.1093/nar/gky1022
28. Oksanen J, Guillaume Blanchet F, Friendly M, Kindt R, Legendre P, McGlinn D, et al. Vegan: Community Ecology Package. R package Version 2.4-3 (2017). Available online at: https://CRAN.R-project.org/package=vegan
29. Robinson MD, McCarthy DJ, Smyth GK. edgeR: a bioconductor package for differential expression analysis of digital gene expression data. Bioinformatics. (2010) 26:139–40. doi: 10.1093/bioinformatics/btp616
30. Segata N, Izard J, Waldron L, Gevers D, Miropolsky L, Garrett WS, et al. Metagenomic biomarker discovery and explanation. Genome Biol. (2011) 12:R60. doi: 10.1186/gb-2011-12-6-r60
31. Chen Y, Xu Z, Feng K, Yang G, Fu W, Chen B. Nitrogen and water addition regulate soil fungal diversity and co-occurrence networks. J Soil Sediments. (2020) 20:3192–203. doi: 10.1007/s11368-020-02629-9
32. Sun R, Dsouza M, Gilbert JA. Fungal community composition in soils subjected to long-term chemical fertilization is most influenced by the type of organic matter. Environ Microbiol. (2016) 18:5137–50. doi: 10.1111/1462-2920.13512
33. García-Delgado C, Barba-Vicente V, Marín-Benito JM, Igual J, Sánchez-Martín MJ, Rodríguez-Cruz SM. Influence of different agricultural management practices on soil microbial community over dissipation time of two herbicides. Sci Total Environ. (2019) 646:1478–88. doi: 10.1016/j.scitotenv.2018.07.395
34. Vukicevich E, Lowery T, Bennett JA, Hart M. Influence of groundcover vegetation, soil physicochemical properties, and irrigation practices on soil fungi in semi-arid vineyards. Front Ecol Evol. (2019) 7:1–10. doi: 10.3389/fevo.2019.00118
35. Nie S, Lei X, Zhao L. Fungal communities and functions response to long-term fertilization in paddy soils. Appl Soil Ecol. (2018) 130:251–8. doi: 10.1016/j.apsoil.2018.06.008
36. Yang X, Ma L, Ji L. Long-term nitrogen fertilization indirectly affects soil fungi community structure by changing soil and pruned litter in a subtropical tea (Camellia sinensis L.) plantation in China. Plant Soil. (2019) 444:409–26. doi: 10.1007/s11104-019-04291-8
37. Zhu L, Wang X, Chen F, Li C, Wu L. Effects of the successive planting of Eucalyptus urophylla on soil bacterial and fungal community structure, diversity, microbial biomass, enzyme activity. Degrad Dev. (2019) 30:636–46. doi: 10.1002/ldr.3249
38. Ning Q, Chen L, Zhang C. Saprotrophic fungal communities in arable soils are strongly associated with soil fertility and stoichiometry. Appl Soil Ecol. (2021) 159:103843. doi: 10.1016/j.apsoil.2020.103843
39. Bastida F, Torres IF, Romero-Trigueros C, Baldrian P, Větrovský T, et al. Combined effects of reduced irrigation and water quality on the soil microbial community of a citrus orchard under semi-arid conditions. Soil Biol Biochem. (2017) 104:226–37. doi: 10.1016/j.soilbio.2016.10.024
40. Mavrodi DV, Mavrodi OV, Elbourne LDH, Tetu S, Bonsall RF, Parejko J, et al. Long-term irrigation affects the dynamics and activity of the wheat rhizosphere microbiome. Front Plant Sci. (2018) 9:345. doi: 10.3389/fpls.2018.00345
41. Gao Y, Xu X, Ding J, Bao F, De Costa YG, Zhuang W, et al. The responses to long-term water addition of soil bacterial, archaeal, and fungal communities in a desert ecosystem. Microorganisms. (2021) 9:981. doi: 10.3390/microorganisms9050981
42. Zhang H, Wu C, Wang F, Wang H, Chen G. Wheat yellow mosaic enhances bacterial deterministic processes in a plant-soil system. Sci. Total Environ. (2021) 2021:151430. doi: 10.1016/j.scitotenv.2021.151430
43. Zhang L, Xie Z, Zhao R, Zhang Y. Plant, microbial community and soil property responses to an experimental precipitation gradient in a desert grassland. Appl Soil Ecol. (2018) 127:87–95. doi: 10.1016/j.apsoil.2018.02.005
44. Dang Q, Tan W, Zhao X, Li D, Li Y, Yang T, et al. Linking the response of soil microbial community structure in soils to long-term wastewater irrigation and soil depth. Sci Total Environ. (2019) 688:26–36. doi: 10.1016/j.scitotenv.2019.06.138
45. Hartmann M, Brunner I, Hagedorn F, Bardgett RD, Stierli B, Herzog C, et al. A decade of irrigation transforms the soil microbiome of a semi-arid pine forest. Mol Ecol. (2017) 26:1190–206. doi: 10.1111/mec.13995
46. Liao L, Wang X, Wang J, Liu G, Zhang C. Nitrogen fertilization increases fungal diversity and abundance of saprotrophs while reducing nitrogen fixation potential in a semiarid grassland. Plant Soil. (2021) 465:515–32. doi: 10.1007/s11104-021-05012-w
47. Chen J, Wu Q, Li S, Ge J, Liang C, Qin H, et al. Diversity and function of soil bacterial communities in response to long-term intensive management in a subtropical bamboo forest. Geoderma. (2019) 354:113894. doi: 10.1016/j.geoderma.2019.113894
48. Wang JT, Shen JP, Zhang LM. Generalist taxa shape fungal community structure in cropping ecosystems. Front Microbiol. (2021) 12:e678290. doi: 10.3389/fmicb.2021.678290
49. Truong C, Mujic AB, Healy R. How to know the fungi: combining field inventories and DNA-barcoding to document fungal diversity. New Phytol. (2017) 214:913–9. doi: 10.1111/nph.14509
50. Fu H, Li H, Yin P, Mei H, Li J, Zhou P. Integrated application of rapeseed cake and green manure enhances soil nutrients and microbial communities in tea garden soil. Sustainability. (2021) 13:2967. doi: 10.3390/su13052967
51. Frenk S, Hadar Y, Minz D. Resilience of soil bacterial community to irrigation with water of different qualities under Mediterranean climate. Environ Microbiol. (2014) 16:559–69 doi: 10.1111/1462-2920.12183
52. Sánchez-Marañón M, Miralles I, Aguirre-Garrido JF, Anguita-Maeso M, Millán V, Ortega R, et al. Changes in the soil bacterial community along a pedogenic gradient. Sci Rep. (2017) 7:14593. doi: 10.1038/s41598-017-15133-x
53. Murphy BR, Martin Nieto L, Doohan FM, Hodkinson TR. Fungal endophytes enhance agronomically important traits in severely drought-stressed barley. J Agron Crop Sci. (2015) 201:419–27. doi: 10.1111/jac.12139
54. Sadeghi F, Samsampour D, Askari Seyahooei M, Bagheri A, Soltani J. Fungal endophytes alleviate drought-induced oxidative stress in mandarin (Citrus reticulata L.): Toward regulating the ascorbate–glutathione cycle. Sci Hortic. (2020) 261:108991. doi: 10.1016/j.scienta.2019.108991
55. Soares Fortes NG, dos Santos MAL, Vitoria NS. Apiosordaria nigeriensis (Ascomycota): a new record for the Americas. Rodriguesia. (2020) 71:1092. doi: 10.1590/2175-7860202071092
56. Panelli S, Capelli E, Comandatore F, Landinez-Torres A, Granata MU, Tosi S, et al. A metagenomic-based, cross-seasonal picture of fungal consortia associated with Italian soils subjected to different agricultural managements. Fungal Ecol. (2017) 30:1–9. doi: 10.1016/j.funeco.2017.07.005
57. Willoughby LG. The activity of Rhizophlyctis rosea in soil: Some deductions from laboratory observations. Mycologist. (2001) 15:113–7. doi: 10.1016/S0269-915X(01)80032-X
58. Six J, Bossuyt H, Degryze S, Denef K. A history of research on the link between (micro) aggregates, soil biota, and soil organic matter dynamics. Soil Till Res. (2004) 79:7–31. doi: 10.1016/j.still.2004.03.008
59. Tedersoo L, Bahram M, Põlme S, Kõljalg U, Yorou NS, Wijesundera R, et al. Global diversity and geography of soil fungi. Science. (2014) 346:e1256688. doi: 10.1126/science.1256688
60. Lekberg Y, Arnillas CA, Borer ET, Bullington LS, Fierer N, Kennedy PG, et al. Nitrogen and phosphorus fertilization consistently favor pathogenic over mutualistic fungi in grassland soils. Nat Comm. (2021) 12:1–8. doi: 10.1038/s41467-021-23605-y
61. Lehmann A, Rillig MC. Understanding mechanisms of soil biota involvement in soil aggregation: A way forward with saprobic fungi? Soil Biol Biochem. (2015) 88:298–302. doi: 10.1016/j.soilbio.2015.06.006
62. Tedersoo L, Sánchez-Ramírez S, Kõljalg U, Bahram M, Döring M, Schigel D, et al. High-level classification of the Fungi and a tool for evolutionary ecological analyses. Fungal Divers. (2018) 90:135–59. doi: 10.1007/s13225-018-0401-0
63. Walker JK, Cohen H, Higgins LM, Kennedy PG. Testing the link between community structure and function for ectomycorrhizal fungi involved in a global tripartite symbiosis. New Phytol. (2014) 202:287–96. doi: 10.1111/nph.12638
64. Jiao S, Lu Y. Abundant fungi adapt to broader environmental gradients than rare fungi in agricultural fields. Glob Chang Biol. (2020) 26:4506–20. doi: 10.1111/gcb.15130
Keywords: soil enzymes, soil lipids, soils use change, soil microbiota, soil aggregates, irrigated agriculture
Citation: Frene JP, Faggioli V, Covelli J, Reyna D, Gabbarini LA, Sobrero P, Ferrari A, Gutierrez M and Wall LG (2022) Agriculture by Irrigation Modifies Microbial Communities and Soil Functions Associated With Enhancing C Uptake of a Steppe Semi-Arid Soil in Northern Patagonia. Front. Soil Sci. 2:835849. doi: 10.3389/fsoil.2022.835849
Received: 15 December 2021; Accepted: 07 February 2022;
Published: 11 March 2022.
Edited by:
Patricia Dorr De Quadros, University of Waterloo, CanadaReviewed by:
Sebastian Loeppmann, Christian-Albrechts-Universität zu Kiel, GermanyMalak M. Tfaily, University of Arizona, United States
Copyright © 2022 Frene, Faggioli, Covelli, Reyna, Gabbarini, Sobrero, Ferrari, Gutierrez and Wall. This is an open-access article distributed under the terms of the Creative Commons Attribution License (CC BY). The use, distribution or reproduction in other forums is permitted, provided the original author(s) and the copyright owner(s) are credited and that the original publication in this journal is cited, in accordance with accepted academic practice. No use, distribution or reproduction is permitted which does not comply with these terms.
*Correspondence: Luis G. Wall, wall.luisgabriel@gmail.com
†These authors have contributed equally to this work