Savannah Phenological Dynamics Reveal Spatio-Temporal Landscape Heterogeneity in Karamoja Sub-region, Uganda
- 1Department of Environmental Management, Makerere University, Kampala, Uganda
- 2Regional Universities Forum for Capacity Building in Agriculture (RUFORUM), Kampala, Uganda
- 3Department of Geomatics and Land Management, College of Engineering, Design, Art and Technology, Makerere University, Kampala, Uganda
- 4Department of Biosecurity, Ecosystems and Veterinary Public Health, College of Veterinary Medicine, Animal Resources and Biosecurity, Makerere University, Kampala, Uganda
- 5Department of Geography and Social Studies, Kyambogo University, Kampala, Uganda
Phenological properties are critical in understanding global environmental change patterns. This study analyzed phenological dynamics in a savannah dominated semi-arid environment of Uganda. We used moderate-resolution imaging spectroradiometer normalized difference vegetation index (MODIS NDVI) imagery. TIMESAT program was used to analyse the imagery to determine key phenological metrics; onset of greenness (OGT), onset of greenness value, end of greenness time (EGT), end of greenness value, maximum NDVI, time of maximum NDVI, duration of greenup (DOG) and range of normalized difference vegetation index (RNDVI). Results showed that thicket and shrubs had the earliest OGT on day 85 ± 14, EGT on day 244 ± 32 and a DOG of 158 ± 25 days. Woodland had the highest NDVI value for maximum NDVI, OGT, EGT, and RNDVI. In the bushland, OGT occurs on average around day 90 ± 11, EGT on day 255 ± 33 with a DOG of 163 ± 36 days. The grassland showed that OGT occurs on day 96 ± 13, EGT on day 252 ± 36 with a total DOG of 156 ± 33 days. Early photosynthesis activity was observed in central to eastern Karamoja in the districts of Moroto and Kotido. There was a positive relationship between rainfall and NDVI across all vegetation cover types as well as between phenological parameters and season dynamics. Vegetation senescence in the sub-region occurs around August to mid-September (day 244–253). The varied phenophases observed in the sub-region reveal an inherent landscape heterogeneity that is beneficial to extensive pastoral livestock production. Continuous monitoring of savannah phenological patterns in the sub-region is required to decipher landscape ecosystem processes and functioning.
Introduction
Global environmental change, including climate change, is undoubtedly a pressing issue of global concern in recent times (Naeem et al., 2009; Bulkeley and Newell, 2010; Hussein, 2011). This in part is due to a rise in extreme events and associated threats to humanity, species and habitats (Birkmann et al., 2014; Allen and Allen, 2017; Ma et al., 2018). Climate variability and change in particular alters plant phenology because temperature tends to influence the timing of development, singularly and through interactions with other cues, such as photoperiods (Partanen et al., 1998; Fitchett et al., 2015; Suonan et al., 2019). In tropical regions and ecosystems, phenology dynamics are often less sensitive to temperature and photoperiods but more aligned to seasonal shifts in precipitation (Reich, 1995). These phenology patterns are attuned to environmental conditioning including the associated seasonality patterns (Cleland et al., 2007; Puppi, 2007). Shifts in plant phenology offers compelling evidence of ecosystem interactions with climatic patterns as well as with other components of global environmental change including the accumulation of atmospheric carbon dioxide (Cleland et al., 2007; Stocker et al., 2013). Phenology dynamics have important implications for biophysical and biogeochemical feedbacks to the climate system (Piao et al., 2019). Further, plant reproduction, population-level interactions, community dynamics and plant evolution and adaptations often influence ecosystem functions and services (Isbell et al., 2011; Maestre et al., 2012). These can, however, be altered by shifts in plant phenology (Peñuelas et al., 2013; Suonan et al., 2019).
Phenology examines organism-environment relationships using critical life cycle phenomena as the primary window (Liang and Schwartz, 2009). In situations where vegetation phenology varies at a scale relevant to the movements of individuals (e.g., dispersal or foraging ranges), such knowledge is essential to understanding processes and dynamics within such ecosystems (Cole and Sheldon, 2017). Analysis of phenology dynamics at landscape level is relevant because seasonal vegetation dynamics (including spatial and temporal patterns) within heterogeneous biophysical environments is critical for understanding the complex functioning of ecosystems. This includes the interactions of primary producers with seasonal and inter-annual environmental variability across landscapes (Liang and Schwartz, 2009). These heterogeneous phenological dynamics at regional and landscape-level are also important for animal populations (Haddad et al., 2011; Simms, 2013). This is because variation within a wide range of abiotic factors (e.g., soil moisture and nutrients, temperature, precipitation) often can cause plants at different locations to initiate growth at different times (Zheng et al., 2016). Evidence available indicates that plants and animals exhibit seasonal patterns in their activities. This is attributed to the fact that there is a seasonality in the suitability of their environment (Visser and Both, 2005).
In the Serengeti plains of Tanzania and Kenya, population viability of some grazers is directly influenced by access to patches of grassland that are varied in phenology as a result of spatial heterogeneity (Fryxell et al., 2005). Thus, plant phenology is an important parameter for showing long-term and seasonal variations in development patterns for both annual, biannual and perennial plants across different landscapes (Primack and Gallinat, 2017; He et al., 2018; Hegazy et al., 2018). Changes in phenological patterns of vegetation such as the rate and duration of photosynthetic activities, onset of greenness (OGT), end of greenness time (EGT) and duration of greenness (DOG) have the potential to serve as indicators of change in environmental quality including impacts on plant production (Vrieling et al., 2016; Ibrahim et al., 2018). Further, it is vital in detecting spatio-temporal variations in plant health and productivity (Tottrup and Rasmussen, 2004; Boke-Olén et al., 2016).
It is apparent that spatial heterogeneity in resources at landscape scale allows mobile consumers to compensate for temporal variability in resource availability at local scale (Fryxell et al., 2005). This is an important landscape characteristic of relevance to herbivores because it confers nutritional benefits by extending the foraging time as well as resource access at landscape level (Coogan et al., 2012). In water limited ecosystems especially semi-arid and arid areas, plant phenology is seldom synchronized across the landscape. However, it tends to vary asynchronously as a result of spatial and temporal variation in elevation, aspect, and weather (Chen and Pan, 2002; Hobbs et al., 2008). In these landscapes, wild herbivores, pastoralists and their livestock respond to gradients and pulses in forage quality and quantity by matching their distribution to spatially variable peaks and gradients in forage quality (Scoones, 1995; Hebblewhite et al., 2008). Responses occur in varying forms and at varying spatial and temporal scales. One adaptation strategy used by pastoral groups in response to this variability is to move across the landscape in search of abundant and nutritious forage (Ellis and Swift, 1988). These movements are key to sustaining the foraging and nutritional needs of livestock (Turner and Schlecht, 2019). They are critical for traditionally guided plant inflorescence-phenological dynamics (Dunning et al., 2016). They also form the basis for the ecological resilience of both pastoral livelihoods and rangeland ecosystems (Boles et al., 2019).
Accelerated environmental changes in pastoral landscapes in East Africa have been registered in the last two decades (Little, 1996; Boles et al., 2019). These changes include increased extreme weather events especially drought and flash floods (Ayal et al., 2018; Kimaro et al., 2018), land use land cover change and habitat fragmentation (Kimiti et al., 2018). These changes in part reflect policy shifts driven by outsiders that have historically viewed pastoralism as working against both environmental and development goals (Turner and Schlecht, 2019). In pursuit of “development,” restrictions on pastoral mobility were imposed and at the same time significant promotion of sedentarisation and crop farming in much of Karamoja sub-region (Krätli, 2010; Egeru, 2016). Restrictions were imposed following the need to bring “peace” by controlling livestock theft within Karamoja and with neighboring communities and across Kenya, Uganda and South Sudan. In essence, the traditional pastoral Karamojong was “quarantined” to graze within the sub-region without being able to explore the traditional grazing pathways. Such restrictions of mobility of people and animals prevents herbivores and pastoralists from matching their distribution to the resources they require to survive and reproduce (Hobbs et al., 2008). Such interruptions on migratory pathways lead to profound effects such as landscapes becoming unsuitable to support people and animals (Fryxell et al., 2005) and localized degradation (Egeru et al., 2019).
Amidst these environmental changes, political orientations and re-orientations to what constitutes “development” in Karamoja and climate variability and change remain unresolved (Gray, 2000; Jabs, 2005; Krätli, 2010; Egeru et al., 2019). The intensity, frequency and shortened return time of extreme events (especially drought) is more pronounced today (Mubiru et al., 2018; Nsubuga and Rautenbach, 2018). Neighboring pastoralists from Kenya (Turkana, Pokot) have recently had to spend longer grazing time on the Uganda side than in previous grazing cycles. By spending a longer grazing time in Karamoja, they impose an additional challenge to people whose mobility is restricted. Accordingly, a paucity of information exists on vegetation dynamics in the region and how it might be able to support the internal mobility of pastoral groups within Karamoja as well as the external grazing pressure from Kenya and South Sudan. This study assessed savannah vegetation phenology with the aim of identifying spatio-temporal dynamics of plant activity in Karamoja sub-region. In doing so, we hold the assumption that the pastoral groups from within and outside the sub-region have only been able to maintain their livestock herds because of an inherent landscape heterogeneity. Further, Turner and Schlecht (2019) have opined that pastoral transhumance responds more to seasonal variabilities and spatial heterogeneities that display some predictable regularities across the landscape.
Study Area
Karamoja sub-region is located in north-eastern Uganda and covers a total land area of 27,319 square kilometers. The sub-region is located between latitude 1°31′ to 4°N and longitude 33°30′ to 35°E. It is constituted by seven districts including: Kotido, Abim, Moroto, Amudat, Napak, Kaabong, and Nakapiripirit (Figure 1A). The sub-region consists of plains that rise toward the hilly terrain in the eastern parts of the region bordering the escarpment along the border with Turkana District of Kenya. The Kidepo Valley National park is part of the open grassland and woodland savannah ecosystems that predominate in the northern parts of sub-region. The landscape opens to the plains and low lands of central to western Karamoja, interrupted by Mt. Napak and isolated inselbergs and mountainous rises of the Iriri and Alekilek Mounts toward the border with the Teso sub-region to its west. In southern Karamoja in parts of Nakapiriti, occurs the Kadam Mountains that later open to the plains and flats in Namaalu with lush grassland ecosystem. This sub-region in Uganda is classified as semi-arid region and is known for its rainfall variability and intermittent droughts. Rainfall in the sub-region on average is about 800 mm with a non-uniform distribution ranging from 300 to 1200 mm in some parts of the sub-region (Egeru et al., 2014). Temperatures are considerably high and range from 28 to 32.5°C (maximum temperature) and from 15 to 18°C (minimum temperature) making evaporation rates in the sub-region similarly high.
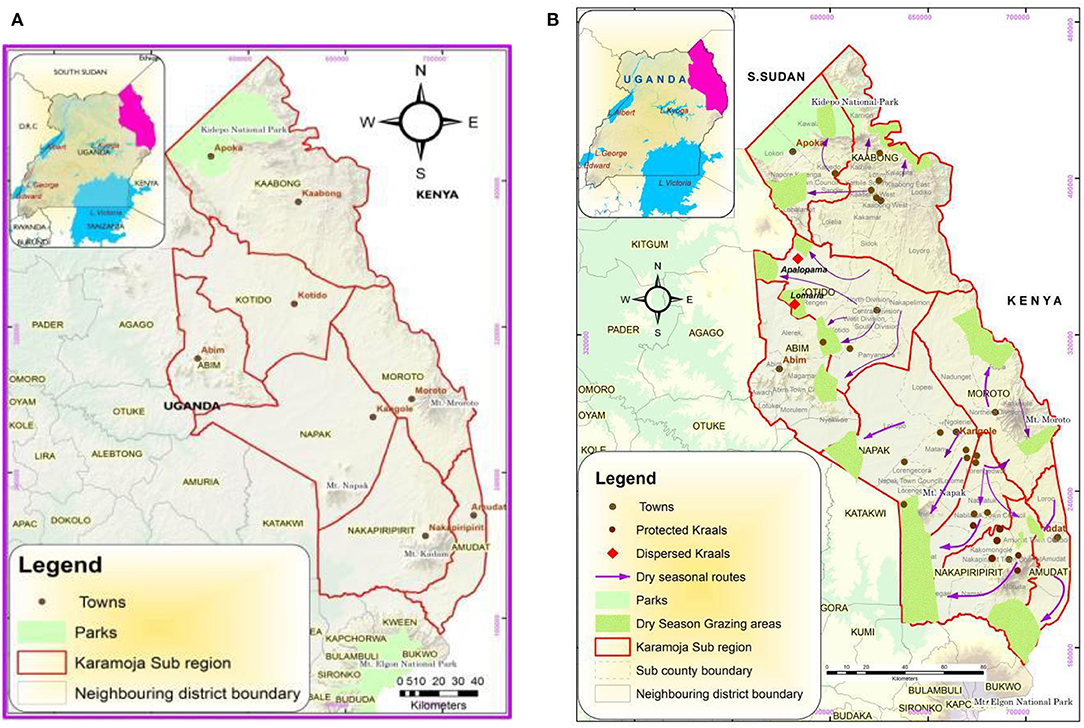
Figure 1. (A) Location of Karamoja sub-region. (B) Dry season grazing areas and transhumance routes in Karamoja sub-region.
The high evaporation rate in addition to the area sloping to the west leads to limited retention of water in the area as runoff rapidly accumulates in the lowland-wetlands of Teso, Lango, and Acholi sub-regions. The sub-region has had a marked irregularity in rainfall since the 1920s through 1940s and 1950s and intense droughts in the 1980s (Gulliver and Dyson-Hudson, 1967). This irregularity and unpredictability of rainfall patterns has exacerbated the fragility of local pastures and has required flexible and knowledgeable day-by-day herding decisions of pastoral households in the sub-region (Filipová and Johanisova, 2017). As a result, changes in the livelihood and lifestyle sources have occurred. For example, the International Organisation for Migration indicated that 40% of households in the sub-region now rely on natural resource extraction. Wood is used for charcoal production, topsoil is used to make bricks, and quarrying stone is used as a primary source of income and livestock management. Livestock, once dominant, is now a primary source of income for only 17% of the households in the sub-region. Despite this perceived income allocation sources, livestock remains a key livelihood asset and strategy as many households are striving to rebuild their livestock herds. In fact, Krätli (2010) indicated that the persistent food insecurity challenge in Karamoja is a livestock crisis indicator.
Traditionally, the Karamojong moved their livestock in a transhumant manner to manage grazing resources heterogeneity at landscape level. However, after the accumulation of guns in 1911 and pacification by the colonial administration from 1921, restrictions on movement in and out of Karamoja were imposed. These restrictions prevented the Karamojong from being able to freely graze their livestock within their traditional grazing lands (Knighton, 1990; Filipová and Johanisova, 2017). Most recently in the post disarmament exercise in 2007, a total ban on livestock from Karamoja leaving the sub-region was imposed. As a result, the pastoral households have had to manage their livestock numbers within the sub-region which has also had to accommodate the transhumant Turkana (herders from Kenya) who come to the sub-region as a dry season grazing ground (Egeru, 2016; Figure 1B). These dynamics have shaped resource use, governance and power relations. Where failure and competition for resources has been intense, violence and conflict over grazing lands and watering resources have been registered. This makes it critical to monitor vegetation dynamics in the sub-region.
Materials and Methods
Data, Data Sources, and Analysis
Landsat imagery and Moderate Resolution Imaging Spectro-radiometer sensor images (MODIS) were obtained from USGS (https://earthexplorer.usgs.gov/). The land cover and Normalized Difference Vegetation Index (NDVI) extractions across 2000-2017 time series were, respectively, used with a 16-day composite (MODIS NDVI) at 250 meters spatial resolution. In addition to the relatively finer spatial resolution, MODIS data products also have lower noise from clouds or atmospheric haze, aerosols and negligible water vapor impacts (Huete et al., 2002).
NDVI Pre-processing
The NDVI was computed following an established standard NDVI derivation process. The NDVI was used because the normalization in the equation partially compensates for illumination conditions and surface terrain effects such as minimizing soil and reducing background effects (Lillesand and Kiefer, 1979; Lillesand et al., 2004). The NDVI data at 250 m spatial resolution and 16-day composite temporal resolution interval acquired by MODIS on the Terra platform (MOD13Q1) were used to derive the NDVI time series relevant for phenological analysis. The frequent revisits allows a greater opportunity for obtaining cloud free coverage during important phenological stages of land covers (Reed et al., 1994). This resulted in 23 composite NDVI images per year, providing a total time series of 391 NDVI images. MODIS NDVI imagery obtained were mosaicked, re-projected from Sinusoidal Projection to WGS84 area projection suitable for analysis using MODIStsp-R GUI package. MODIStsp enables performance of several preprocessing steps (download, mosaicking, projection, and resize) on MODIS data available within a given time period (Busetto and Ranghetti, 2016). For each of the selected homogeneous sites (Figure 2), MODIS NDVI time series data was extracted through a time series of 2000–2017. The mean NDVI time series data were extracted for all sampling fields of interest excluding pixels that were affected by cloud cover. These data provided the basis for examining the NDVI trends and the associated phenology metrics for the different land cover types.
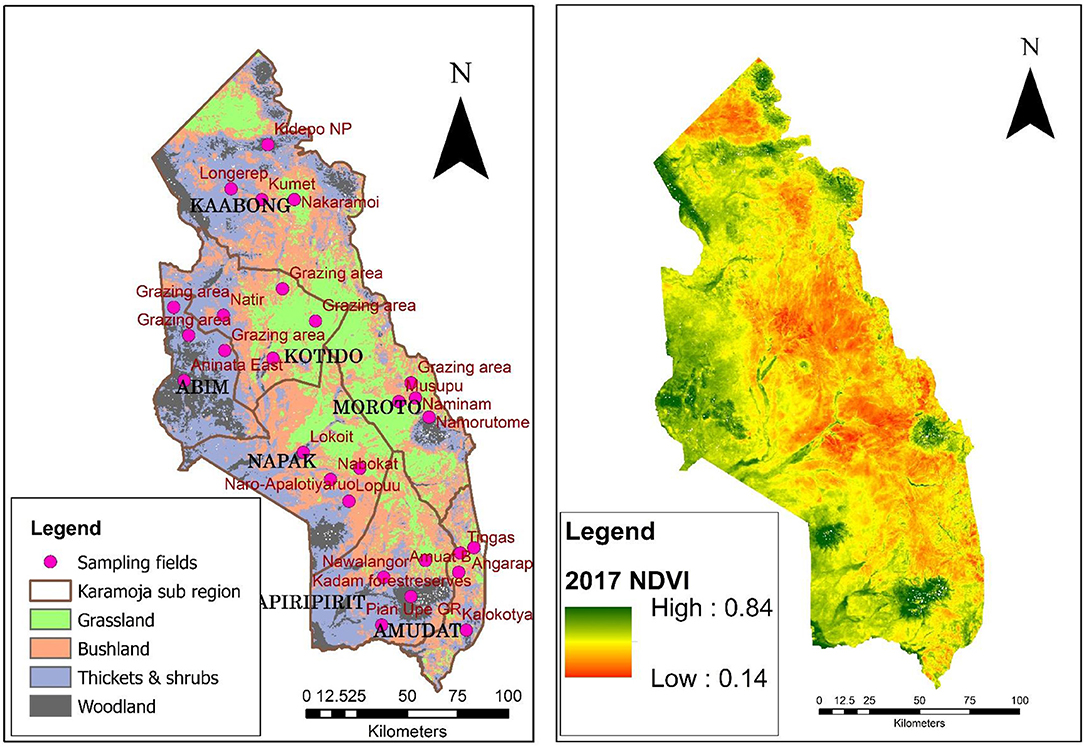
Figure 2. Left-Locations of homogeneous sample fields and major land cover map derived from NDVI and right- NDVI values for the study region in 2017.
Phenology Extraction
Both image-based and point-based NDVI time series were examined with respect to their growth and phenology patterns. However, only areas with relatively homogeneous land cover types (Figure 2) were selected to examine the variation in phenology and their response to climate variables. To sample the study area, several criteria were taken into account to ascertain representation of the major land cover types and avoid mixed or heterogeneous land cover patterns. Only areas that maintained their vegetation land cover types for the last 15 years were considered. Homogeneous land cover was identified by using stratified sampling and land cover strata were divided into homogeneous sub-groups for a better precision and accuracy. The community elders were involved to identify the different strata that have existed within a time limit of 15 years from each case study district. The NDVI time series data were used to derive land surface phenology-metrics measured in days.
The ArcMap cell statistics and zonal statistics were used to extract monthly mean NDVI values (Figure 3) that were used to extract savannah phenological characteristics within a time series of 2000–2017. A model builder was used to multi-task the processing of the numerous years (2000–2017). NDVI values were an input in the TIMESAT tool for extraction of the savannah phenological characteristics (Jönsson and Eklundh, 2004). Further detailed description of processes undertaken to execute a TIMESAT based analysis are described in Appendix 1.
Phenological Metrics
Phenological metrics of interest in this study are summarized in Table 1 (Reed et al., 1994; USGS, 2011). The Onset of Greenness Time (OGT) is the beginning of measurable photosynthesis in the vegetation type canopy. It is obtained as the day of year identified as having a consistent upward trend in time-series NDVI. The Onset of Greenness NDVI (OG NDVI) is the level of photosynthetic activity at the beginning of measurable photosynthesis. As such it is the NDVI value associated with OGT. End of Greenness Time (EGT) is the end of measurable photosynthesis in the vegetation type canopy, corresponding to the day of year identified at the end of a consistent downward trend in time series NDVI. Time of Maximum NDVI (TMaxNDVI) is the time of maximum photosynthesis in the vegetation type canopy, which is the day of year corresponding to the maximum NDVI in an annual time series. Maximum NDVI (MaxNDVI) is the maximum level of photosynthesis activity in the vegetation type canopy, corresponding to the MaxNDVI in an annual time series.
Duration of greenness (DOG) is the length of photosynthetic activity in the vegetation type canopy, corresponding to the number of days from the OGT and EGT. According to Reed et al. (1994) the range in NDVI (RNDVI) is computed by subtracting the NDVI value of either onset or end of greenness, whichever is lower, from the maximum NDVI value. Rate of Greenup (RtUP) and Senescence (RtDn) were computed as straight-line slopes from onset to maximum and from maximum to the end, respectively (Appendix 1). A dynamic threshold algorithm was used to extract the phenology-metrics (Eklundh and Jönsson, 2017) which included temporal NDVI metrics (based on the timing of an event), NDVI value metrics (the NDVI value at which events occur) and derived metrics as categorized by Reed et al. (1994) for each of the savannah vegetation types in Karamoja region. On this basis, the savannah vegetation multi-year mean per month was calculated for the period of 2000–2017 and smoothed to avoid outliers.
Threshold Value of Savannah Phenology
Considering the range of savannah vegetation phenological characteristics, the onset of greenness NDVI threshold value was set to 20% of the distance between minimum and maximum NDVI during the rising levels (Zhang et al., 2015). The end of greenness was also determined in a similar manner (Song et al., 2011). The 20% threshold accounts for noise in the NDVI data and prevents the identification of false starts and/or end of greenness time (Van Leeuwen et al., 2010).
The maximum NDVI (NDVImax) with in the year was obtained, thereafter, during the NDVI rising stage, minimum NDVI (NDVImin) was obtained and a 20% of the difference between NDVImax and NDVImin was set as the threshold (NDVIthreshold). Time of onset of greenness was defined as the date when NDVI reaches NDVIthreshold. The date when NDVI reaches to NDVI threshold for the first time was defined as time of onset of greenness. Time of end of greenness was defined in the same way during the descending stage (Appendix 1) for all vegetation types in the region as illustrated in 2001 for grassland vegetation (Appendix 1). Therefore, a threshold value of 0.1 NDVI as computed and obtained by several scholars (Lloyd, 1990; Reed et al., 1994; Jönsson and Eklundh, 2004; Song et al., 2011) was determined. This threshold was applied to all land cover types during the analysis.
Results
Phenological Temporal Dynamics in Karamoja
Temporal indices including onset of greenness (OGT), onset of greenness value, end of greenness time (EGT), end of greenness value, maximum NDVI, time of maximum NDVI, duration of greenup (DOG), and range of NDVI as phenological metrics of interest were computed for the four vegetation types: bushland, grassland, woodland, and thicket and shrub. Over the 18 years of analysis, there was high frequency of occurrence of an early EGT for bushland, thicket and shrub and woodland. Results of the respective vegetation cover showed that bushland on average has OGT occurring on day 90 ± 11, EGT around day 255 ± 33 with an average DOG of 163 ± 36 days. Meanwhile, maximum NDVI was 0.6 and the end value NDVI was 0.5 during the period of analysis (Table 2). A pronounced deviation in temporal phenology was observed in 2009 interrupting a relatively consistent trend in the measured metrics. There was a 67% probability of the OGT occurring in the fourth week of March and the first week of April (around the 90th day) with a 60% probability of maximum NDVI occurring in July. The green up ends around the 255th day, the second week of the month of September.
Grassland showed that on average OGT occurred on day 96 ± 13, EGT on day 252 ± 36 with a total DOG of 156 ± 33 days. It was observed that 2009 had the shortest photosynthesis activity of 74 days. Trend results showed a rapid increase in DOG in 2000, 2010, and 2017. Results also showed a relative consistency in phenological parameters from 2001–2007 with DOG ranging between 129–192 days. For all the years, the OGT ranged between day 79–105 and EGT ranged between day 222–291 except in the year 2000 where OGT was recorded on day 121 and EGT on day 325 of the year (Table 3). Onset of greenness were mainly observed in March and April (around day 96) with the highest occurrences in April (67%) in the first week of the month. Meanwhile, EGT exhibited strong variability across the months of July, August, October, November, and September with the highest (57%) chance of occurrence in the first week of September with a DOG of 156 days.
In the woodland the average OGT occurred on day 95 ± 16 with an onset of greenness value of 0.6 and EGT on day 253 ± 35 with a 0.7 end of greenness value. An overall DOG of 165 ± 47 days was observed. Compared to all other land cover types, woodland had a higher maximum NDVI value of 0.7 for the entire period of analysis (Table 4). The OGT, EGT and DOG held a similar trend for the period of analysis. Variation in the time of maximum measureable photosynthesis occurred between February and August in the woodland. The earliest onset of greenness time was revealed in 2006, 2001, 2011, occurring in the first 15 days of March. A relatively high (61%) probability of onset of greenness to occur was observed in March (61%) and in April (33%). End of greenness time varied across the period of analysis with occurrence in the months of July, August, September, October, and November.
In the thicket and shrub, OGT occurred around day 85 ± 14, EGT at day 244 ± 32 while DOG was 158 ± 25 days (Table 5). Onset of greenness occurred in March (67%) and April (33%) throughout the period of analysis. Variability in the duration of greenness across the time frame of analysis was observed. Results also showed that thicket and shrub had a 0.7 as the maximum green up value. About 75% of the peak NDVI in the thicket and shrub occurred in the third week of July. Like in the other vegetation types, end of greenness time occurred variably in July, August, and September. A higher frequency (57%) of occurrence was observed in September with 43% of the EGT occurring in the third and fourth weeks of September. On average, thicket and shrub have a greenness duration of 158 days. In Appendix 2, annual spatial representation of OGT, EGT, and DOG for 2000–2017 are provided for the Karamoja sub-region.
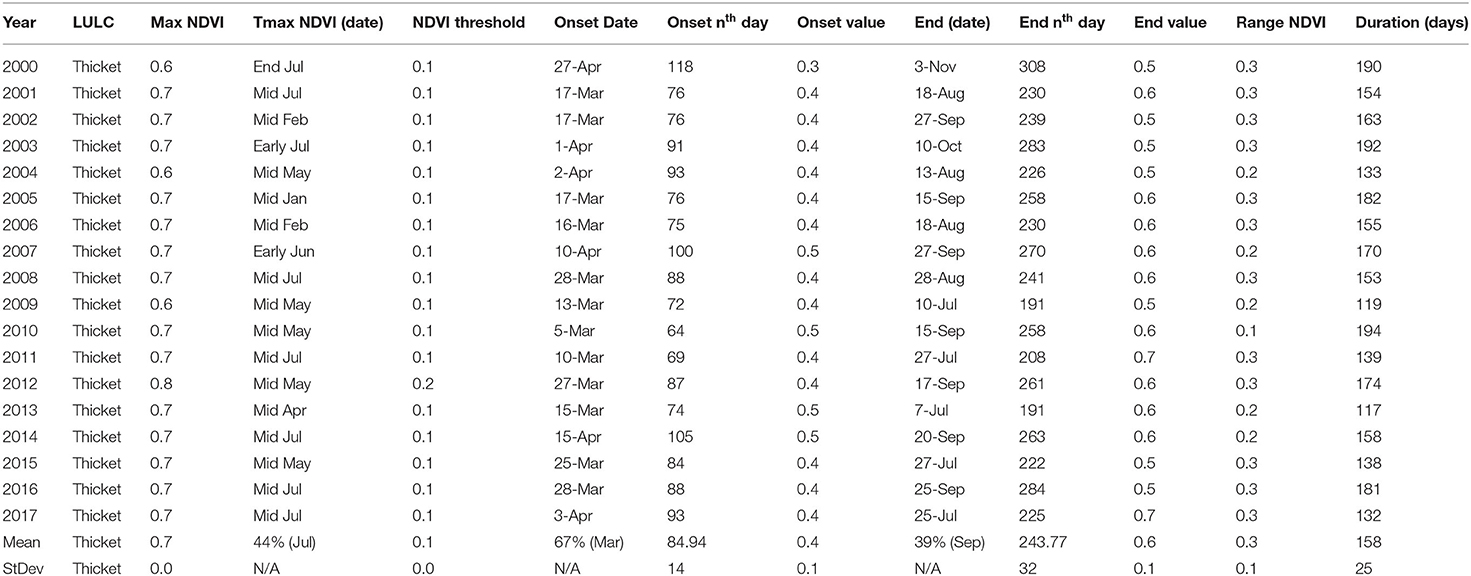
Table 5. Thicket and shrub phenological metrics for the Karamoja region in Uganda for the period 2000–2017.
Spatial Phenological Patterns in Karamoja
We spatially represented the general phenological attributes between 2000 and 2017. We considered time of onset of greenness (OGT), end of greenness time (EGT) and duration of greenness (DOG) from the four major vegetation types: bushland, grassland, woodland and thicket and shrub. Spatially, the OGT and EGT in the sub-region can be represented into three categories, namely early, normal and late OGT/EGT. We found a significant difference in the total land area occupied by each of the vegetation cover types as well as a significant difference in the spatial coverage of the areas under early, normal and late OGT across the land cover types (Table 6). On the other hand, there were non-significant differences in spatial coverage across vegetation types as well as in the early, normal and late (ENL) periods for the EGT. Spatially, grassland cover a larger part of the study area (44%), followed by bushland (28%), thicket and shrub (21%) and woodland (7%). With the exception of the woodland, all the vegetation cover types revealed that their OGT occurred within a similar range. Intra-vegetation cover OGT spatial patterns indicated that 84% woodland areas posted an early OGT occurrence compared to bushland (38%), grassland (33%) and thicket and shrub (42%).
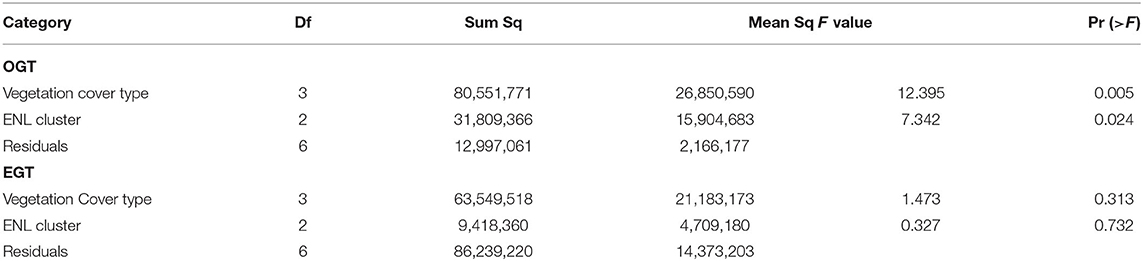
Table 6. Generalized linear models for spatial coverage and early, normal, and late (ENL) cluster for OGT and EGT.
In the grassland (Figure 4), early photosynthesis (early OGT) activity occurred predominantly in central-eastern parts of Moroto and Kotido districts as well as in the eastern bounds of Amudat district, south Karamoja. Some patchy observations of an early OGT were observed in parts of Kaabong district and further north in the Kidepo Valley National Park in Karenga district. Meanwhile, the late OGT was recorded predominantly in the western to southern parts of the study area mainly in the districts of Abim and Nakapiripirit and some patches of Napak district. These patterns are repeated in the EGT but with a significantly reduced presence of late EGT in the study area.
Bushland vegetation cover (Figure 5) occurs across most of the study area. Early OGT in Bushland vegetation closely follows the pattern set by grassland and dominates the central to eastern parts of the region with a late OGT mainly observed in the western parts of the region in the districts of Abim and Nakapiripirit. Spatially, intra-vegetation cover type EGT varied with bushland posting a 41% late EGT compared to grassland (49%), thicket and shrub (87%) and woodland (58%). However, inter-vegetation cover type spatial occurrence of the late EGT revealed that woodland (12%) had the largest area under late EGT followed by bushland (10%) and thicket and shrub (6%). Across all vegetation cover types, grassland experienced a larger spatial occurrence of early EGT (19%) compared to bushland (6%), woodland (2%) and thicket and shrub (1%).
The spatial patterns observed in the OGT and EGT have an influence on the observed patterns of the Duration of Greenness (DOG) time in the study region. Overall, the spatial distribution of DOG in the sub-region appeared to partition the sub-region into three key areas. This pattern is most pronounced in the grassland vegetation cover type (Figure 4) and the bushland that are widespread in the sub-region. In the grassland, areas with a shorter DOG (90–120 days; 44%) occurred mainly in the central to eastern parts of the sub-region (Kotido, Moroto and Amudat districts). The longer DOG (>150 days; 23%) was observed in the western frontier (Abim and Napak districts) and some patches in the north and northeastern (Kaabong-Karenga districts) as well as in the southwestern parts (Nakapiripirit and Nabilatuk districts). These spatial patterns observed in the grassland were repeated in the bushland with a DOG > 150 days (29%) and was most commonly observed in the western frontier of the sub-region. While the woodland (Figure 6) had areas with DOG > 180 days (11%), these appear to be confined to the highland areas in the sub-region, in areas such as Mt. Kadam (south), Mt. Moroto (east), Mt. Labwor (west) in Abim, and Mt. Morungole (north east apex). Thicket and shrub presented two cluster categories of DOG (Figure 7). There were those within the 100–130 days (21%) and others within 131–170 days (79%) which also had a larger spatial coverage. Across the four vegetation cover types, grassland (DOG 90–120 days) and thicket and shrub (DOG 131–170 days) had the most pronounced signals at 19 and 18%, respectively.
Relationship Between Rainfall and Vegetation Phenology
Results revealed that grassland, bushland, woodland and thicket and shrub had a strong positive correlation with rainfall throughout the years of analysis (Appendix 3). The strongest response signal was observed in bushland (r = 0.70), grassland (r = 0.72), and thicket and shrub (r = 0.70) while woodland posted a correlation of r = 0.6 for the period of analysis (2000–2018). Our results of the phenological patterns matched against rainfall-seasonal dynamics (Figure 8) revealed that there was a positive association between the rainfall-derived end of growing season (EGS) and vegetation-derived end of greenness (EGT) time as well as length of growing season (LGS) and duration of greenness time (DOG) in the bushland vegetation. For all the other three vegetation cover types (grassland, woodland, thicket and shrub), we also observed a positive relationship between the EGS and respective EGT for each vegetation cover type although the values were statistically non-significant. We also observed a positive association between the start of growing (SGS) and Onset of greenness time (OGT) in the thicket and shrub but grassland, bushland, and woodland posted non-significant negative associations (Figure 8).
Discussion
In this study, we assessed the phenology of savannah vegetation to identify the spatio-temporal dynamics of plant activity in the Karamoja sub-region. We observed variability in phenological metrics of the four savannah vegetation cover types, namely bushland, grassland, woodland and thicket and shrub. Both intra-annual and inter-annual dynamics in phenology were evident across the Karamoja landscape. The observed intra and inter-annual variations point to the existence of different physiological mechanisms of plant growth, nutrient cycling, and abiotic processes including those influenced by geomorphology, soil fertility, climate, and disturbances such as fire which is commonly associated with semi-arid landscapes (Myoung et al., 2013). Fire as a disturbance in semi-arid areas plays an intrinsic role in shaping biophysical attributes of savannah ecosystem by limiting biomass below its climatically determined potential (Singh et al., 2018). In addition to other biotic and abiotic factors, above-ground phytomass disturbance and variable plant responses to fire, leads to differences in the dynamics of plant phenology across semi-arid areas landscapes (Snyman, 2004; Tokura et al., 2018; Richter et al., 2019). These disturbances appear to have been responsible for a lower signal strength in the sub-region in 2009. This lower signal strength in 2009 is traceable to the 2009/2010 drought episode. Using satellite-based indices derived from NDVI, Nakalembe (2018) identified extreme drought in 2009 over the sub-region.
Whereas we anticipated to find intra and inter-annual variability in vegetation phenology primarily because of the inherent climate variability (Egeru et al., 2014, 2019) in the sub-region, the specific metrics such as OGT, EGT, and DOG posited unique patterns within each of the main vegetation types. However, all of these savannah vegetation types showed OGT signals within late March and early April with a probability ranging between 67 and 75%. This falls within the main rainfall period in the sub-region and in most of the semi-arid areas of Uganda. Variability in the onset for the March-May rainfall and green-up has been observed to be variable across most of Uganda from year to year and in several occasions has been observed with some delays of even up to 30 days, thus starting in mid-April instead of mid-March (Mubiru et al., 2012). Such variability appears to have been captured by the vegetation greenup signals in the current study. This study has further demonstrated that savannah vegetation responds to rainfall signals but at varying levels which is demonstrated by lag-time of nearly 3 weeks observed in this study.
The variability observed in OGT with respect to vegetation types could perhaps be explained by specific vegetation response to climate parameters especially rainfall (Kang et al., 2018). Thus, the lag-time seen in this study between the different vegetation types within a 3 week range could indicate a critical threshold required for the vegetation to grow leading to the commencement of the OGT between the thicket and shrubs, grassland and woodland. Previous studies have shown that vegetation grows when a critical threshold is met based in part on cumulative rainfall, the proportion of annual average rainfall and relative soil moisture index (Lyamchai et al., 1997; White et al., 1997; Tao et al., 2017). This is particularly important in semi-arid regions of Africa where cumulative rainfall stimulates vegetation green-up. The Onset observed in this study corresponds to earlier analysis of vegetation phenology over the Horn of Africa in which Zhang et al. (2005) observed a first set of onset occurring in April, with a second one in September. Further, Vrieling et al. (2013) have suggested that a delayed onset of green-up is logical because there is often a lag time between the onset of rainfall and green-up. It is however important to note that contrary to the expectations that grassland would experience an early OGT, it was the thicket and shrubs and woodland that had an early sprouting. We attribute this development to a pre-rain green-up that has previously been documented in African savannahs (Adole et al., 2018). It also strengthens the understanding that vegetation growth onset in semi-arid regions is more often than not biome-dependent. This was stressed earlier by Pau and Still (2014) who pointed out that the length of the growing season varied between different vegetation types.
Although we observed a nearly consistent pattern in onset in this study, the intra-vegetation cover type variability was a stronger signal for heterogeneity of livestock grazing resources in the sub-region. The strong varied spatial occurrence of green-up within and across vegetation cover types within the early, normal and late green-up delivers grazing resources at different periods of time and at different locations in the sub-region. This is vital for differentiated pastoral utilization of landscapes. For example, we have shown that woody vegetation cover (woodland, thicket and shrub, and bushland) generally have earlier green-up compared to grassland. In parts of the Horn of Africa that are semi-arid, it has already been shown that even within a dry-spell, vegetation and especially trees and shrubs, remains green and abundant (Vrieling et al., 2013). According to Ellis and Swift (1988) pastoralists have relied on these varying forms of vegetation response and at varying spatial and temporal scales to graze their livestock across landscapes and have thus formed a strong basis for the resilience of pastoral livelihoods and rangeland ecosystems (Boles et al., 2019).
Variability is inherent in the sub-region's phenology. We have observed varied commencements for EGT to occur between July-November. Taken across the vegetation cover types, we did not find this EGT significantly different from each other however the intra-vegetation cover type variability was pronounced. A late EGT for grassland (49%), thicket and shrub (87%) and woodland (58%) provides support for the idea that heterogeneity in livestock resources exists across the landscape. It has been observed that woody vegetation with leafy fodder is often more reliable for livestock nutrition than grasses (Opiyo et al., 2015). In this study, both thicket and shrub and woodland tended to have a late EGT thereby becoming important grazing points during dry conditions. Further, this variability often dictates seasonal movements between base locations observed by Turner and Schlecht (2019) among pastoral communities in sub-Saharan Africa.
We also observed varying duration of greenness time (DOG) which occurred as a direct result of the patterns of OGT and EGT. Accordingly, while thicket and shrubs green up much earlier, the woodland had a longer duration of greenness (DOG) compared to all other vegetation types. In their study in southern Africa, Dekker and Smit (1996) observed similar patterns with grassland having short life cycles. Grassland have also been shown to be less adaptive to periods of moisture deficits (Puppi, 2007; Rather et al., 2018). We have also seen that the DOG appears to partition the sub-region into three key areas namely eastern, central and western Karamoja corridors with the western areas generally having a longer DOG occurrence, followed by central and eastern having a shorter DOGs for most of the vegetation cover types. These observed patterns do align with the rainfall dynamics and gradients in the sub-region. For example, locations in the western to southern Karamoja that exhibit a longer DOG correspond to lowlands and some highland areas of Mt. Labwor, Mt. Napak and Mt. Kadam that receive relatively higher rainfall in the sub-region (Egeru et al., 2019). Similarly, patches observed in eastern Karamoja occur around Mt. Moroto area as well as in the Mt. Morungole highlands of northern Karamoja. These areas have also traditionally served as important dry season grazing grounds in the sub-region (OCHA, 2010; see Figure 1B). Owing to these patterns, these areas have through historical time served as dry season grazing landscapes for the Karamoja sub-region. Throughout historical times, these areas of supposed resource abundance have dictated relationships, alliances, power and conflict in the sub-region (Ocan and Ocan, 1994). Given recent developments in the sub-region which restrict the outward mobility from Karamoja to neigbouring sub-regions for grazing, the locations with extended duration of greenness have become increasingly important grazing locations.
Implications for Pastoral Transhumance in Karamoja Sub-region
Pastoral production strategies in East Africa have through historical time relied on opportunistic and extensive livestock transhumance in heterogeneous landscapes (BurnSilver et al., 2004). Transhumance responds to more seasonal variabilities and spatial heterogeneities across the landscape in response to the need for sustained livestock nutrition (Turner and Schlecht, 2019). This study has shown five important facts that point to the importance of landscape heterogeneity and therefore differentiated availability of livestock grazing resources in time and place. First, the onset of greenness varies across space and time and within vegetation cover types. Second, the end of greenness time, although non-significant across vegetation types, displays a strong intra-vegetation cover type variability in terms of early, normal and late end of greenness measures. Third, areas of the longer duration of greenness time are consistent with dry season grazing locations in the sub-region. Fourth, woody vegetation has a longer duration of greenness time but also generally has an earlier onset of greenness across the sub-region. Fifth, the sub-region's onset of greenness time and end of greenness time partitions the sub-region into three distinct zones which may be referred to as the eastern, central and western frontiers with the eastern frontier generally having a shorter duration of greenness and the western to southern frontiers having a longer duration of greenness.
These five facts raise three key implications for pastoral transhumance when these are taken together with other macro-scale political and socio-economic factors that are shaping and re-shaping pastoral dynamics in the sub-region. First, intra-sub-regional pastoral mobility will continue in response to the heterogeneity of livestock resources brought about by the inter and intra-variability in vegetation cover and biomass; second, the pronounced early onset of greenness and late duration of greenness by woody vegetation (woodland, bushland and thicket and shrub) in the sub-region could drive the pastoral communities to shift to adopting browser based livestock species such as camels and goats. Already, increased proliferation of camels from Kenya to Karamoja in southern Karamoja in parts of Amudat brought by their Pokot kinsmen (Nampala, 2013) and among the Matheniko communities of Moroto district brought by their Turkana kinsmen has been on the increase. Owning camels in the sub-region for example has been linked to a 20% increase in household resilience (Asiimwe et al., 2020). Third, the areas with extended duration of greenness will remain important dry season grazing grounds in the sub-region. These will shape local alliances among tribal groups, maintenance of peace and/or potential conflict hotspots as well as land use competition between grazing and crop cultivation in the sub-region. As the pastoralists in Karamoja are incrementally sedentarising, areas posting greater duration of greenness, and thus a longer growing season duration, are also targeted for crop production development. It is predicted that this will only intensify land use change conflicts in the future.
Conclusion
We analyzed the phenological dynamics of the Karamoja sub-region using a suit of phenological metrics across four vegetation types (bushland, grassland, woodland, and thicket and shrub). We observed intra and inter-annual variability in key metrics including OGT, EGT, and DOG during the period of analysis. There was an apparent consistency in the onset of greenness time around late March and first week of April across the study area. However, woodland and the thicket and shrubs showed a relatively earlier OGT in the sub-region which was most pronounced around the districts of Napak and Nakapiripirit. This could point to a pre-rain green-up that has also been previously documented in other studies associated with savannah ecosystems across Africa. Within the variable DOG, we found that grassland vegetation was associated with a shorter DOG while woodland had a longer DOG across the sub-region. The highland areas in Karamoja region experienced longer periods of greenness compared to low-lying areas. Longer and shorter DOG was associated with longer and shorter length of growing season, respectively. Owing to the inherent variability in space and time observed in the sub-region, we confirm that the sub-region has an inherent heterogeneity. This heterogeneity could be important to livestock herders in the sub-region particularly because the EGT is spread variably across different landscapes and vegetation types. This could be aiding pastoral mobility and supporting their ability to meet their livestock nutrition across the year. Based on apparent variability and consistencies-regularities observed in this study, a practical direction should be to understand how these patterns are shaping pastoral mobility patterns in the sub-region. Further, an examination of how local institutions (both state and non-state) are helping to support mobility within a complex system of variability and heterogeneity, statehood, competing land uses, alliances and a rapidly evolving yet dangerous notion of modernization by eliminating mobility in the sub-region, are requisite conversations.
Data Availability Statement
The datasets generated for this study are available on request to the corresponding author.
Author Contributions
AE and JM conceptualizing. AG and BB providing technical back stopping on data analysis and imagery. AE, JM, DK, and AS imagery processing and drafting manuscript. JN overall project coordination. AE funds sourcing and field activity arrangements. All authors contributed to the article and approved the submitted version.
Funding
This work was originally funded by the Carnegie Corporation of New York through Makerere University under the Post-Doc 2017 grant to AE.
Conflict of Interest
The authors declare that the research was conducted in the absence of any commercial or financial relationships that could be construed as a potential conflict of interest.
Supplementary Material
The Supplementary Material for this article can be found online at: https://www.frontiersin.org/articles/10.3389/fsufs.2020.541170/full#supplementary-material
References
Adole, T., Dash, J., and Atkinson, P. M. (2018). Large-scale prerain vegetation green-up across Africa. Glob. Change Biol. 24, 4054–4068. doi: 10.1111/gcb.14310
Allen, M. F., and Allen, E. B. (2017). “Mycorrhizal mediation of soil fertility amidst nitrogen eutrophication and climate change,” in Mycorrhizal Mediation of Soil, eds. Johnson, N. C., Gehring, C., and Jansa, J (Elsevier), 213–231.
Asiimwe, R., Ainembabazi, J. H., Egeru, A., Isoto, R., Aleper, D. K., Namaalwa, J., et al. (2020). The role of camel production on household resilience to droughts in pastoral and agro-pastoral households in Uganda. Pastoralism 10:5. doi: 10.1186/s13570-020-0160-x
Ayal, D. Y., Radeny, M., Desta, S., and Gebru, G. (2018). Climate variability, perceptions of pastoralists and their adaptation strategies: implications for livestock system and diseases in Borana zone. Int. J. Clim. Change Strat. Manage. 10, 596–615. doi: 10.1108/IJCCSM-06-2017-0143
Birkmann, J., Kienberger, S., and Alexander, D. E. (2014). Introduction Vulnerability: A Key Determinant of Risk and Its Importance for Risk Management and Sustainability, (Elsevier). ix-xiii. doi: 10.1016/B978-0-12-410528-7.02001-4
Boke-Olén, N., Lehsten, V., Ardö, J., Beringer, J., Eklundh, L., Holst, T., et al. (2016). Estimating and analyzing savannah phenology with a lagged time series model. PLoS ONE 11:e0154615. doi: 10.1371/journal.pone.0154615
Boles, O. J., Shoemaker, A., Mustaphi, C. J. C., Petek, N., Ekblom, A., and Lane, P. J. (2019). Historical ecologies of pastoralist overgrazing in kenya: long-term perspectives on cause and effect. Hum. Ecol. 47, 1–16. doi: 10.1007/s10745-019-0072-9
BurnSilver, S. B., Boone, R. B., and Galvin, K. A. (2004). “Linking pastoralists to a heterogeneous landscape,” in: People and the Environment, eds J. Fox, R. R. Rindfuss, S. J. Walsh, and V. Mishra (Boston, MA: Springer).
Busetto, L., and Ranghetti, L. (2016). MODIStsp: an R package for automatic preprocessing of MODIS land products time series. Comput. Geosci. 97, 40–48. doi: 10.1016/j.cageo.2016.08.020
Chen, X., and Pan, W. (2002). Relationships among phenological growing season, time-integrated normalized difference vegetation index and climate forcing in the temperate region of eastern. Int. J. Clomatol. 22, 1781–1792. doi: 10.1002/joc.823
Cleland, E. E., Chuine, I., Menzel, A., Mooney, H. A., and Schwartz, M. D. (2007). Shifting plant phenology in response to global change. Trends Ecol. Evol. 22, 357–365. doi: 10.1016/j.tree.2007.04.003
Cole, E. F., and Sheldon, B. C. (2017). The shifting phenological landscape: within-and between-species variation in leaf emergence in a mixed-deciduous woodland. Ecol. Evol. 7, 1135–1147. doi: 10.1002/ece3.2718
Coogan, S. C., Nielsen, S. E., and Stenhouse, G. B. (2012). Spatial and temporal heterogeneity creates a “brown tide” in root phenology and nutrition. ISRN Ecol. 2012:8. doi: 10.5402/2012/618257
Dekker, B., and Smit, G. N. (1996). Browse production and leaf phenology of some trees and shrubs in different Colophospermum mopane savanna communities. Afr. J. Range Forage Sci. 13, 15–23. doi: 10.1080/10220119.1996.9647888
Dunning, C. M., Black, E. C., and Allan, R. P. (2016). The onset and cessation of seasonal rainfall over Africa. J. Geophys. Res. Atmos. 121, 11–405. doi: 10.1002/2016JD025428
Egeru, A. (2016). Climate risk management information, sources and responses in a pastoral region in East Africa. Clim. Risk Manage. 11, 1–14. doi: 10.1016/j.crm.2015.12.001
Egeru, A., Barasa, B., Nampijja, J., Siya, A., Makooma, M. T., and Majaliwa, M. G. J. (2019). Past, present and future climate trends under varied representative concentration pathways for a sub-humid region in Uganda. Climate 7:35. doi: 10.3390/cli7030035
Egeru, A., Osaliya, R., MacOpiyo, L., Mburu, J., Wasonga, O., Barasa, B., et al. (2014). Assessing the spatio-temporal climate variability in semi-arid Karamoja sub-region in north-eastern Uganda. Int. J. Environ. Stud. 71, 490–509. doi: 10.1080/00207233.2014.919729
Eklundh, L., and Jönsson, P. (2017). TIMESAT 3.3 With Seasonal Trend Decomposition and Parallel Processing Software Manual. Sweden: Lund and Malmo University.
Ellis, J. E., and Swift, D. M. (1988). Stability of african pastoral ecosystems: alternate paradigms and implications for development. Rangeland Ecology & Management/Journal of Range Management Archives, 41, 450–459.
Filipová, Z., and Johanisova, N. (2017). Changes in pastoralist commons management and their implications in Karamoja (Uganda). J. Polit. Ecol. 24, 881–900. doi: 10.2458/v24i1.20972
Fitchett, J. M., Grab, S. W., and Thompson, D. I. (2015). Plant phenology and climate change: progress in methodological approaches and application. Prog. Phys. Geogr. 39, 460–482. doi: 10.1177/0309133315578940
Fryxell, J. M., Wilmshurst, J. F., Sinclair, A. R. E., Haydon, D. T., Holt, R. D., and Abrams, P. A. (2005). Landscape scale, heterogeneity, and the viability of Serengeti grazers. Ecol. Lett. 8, 328–335. doi: 10.1111/j.1461-0248.2005.00727.x
Gray, S. J. (2000). A memory of loss: ecological politics, local history, and the evolution of Karimojong violence. Hum. Org. 59, 401–418. doi: 10.17730/humo.59.4.jv233363240n8656
Gulliver, P. H., and Dyson-Hudson, N. (1967). Karimojong politics. Man 2, 226–248. doi: 10.2307/2799523
Haddad, N. M., Crutsinger, G. M., Gross, K., Haarstad, J., and Tilman, D. (2011). Plant diversity and the stability of foodwebs. Ecol. Lett. 14:42–6. doi: 10.1111/j.1461-0248.2010.01548.x
He, M., Yang, B., Shishov, V., Rossi, S., Bräuning, A., Ljungqvist, F. C., et al. (2018). Projections for the changes in growing season length of tree-ring formation on the Tibetan Plateau based on CMIP5 model simulations. Int. J. Biometeorol. 62, 631–641. doi: 10.1007/s00484-017-1472-4
Hebblewhite, M., Merrill, E., and McDermid, G. (2008). A multi-scale test of the forage maturation hypothesis in a partially migratory ungulate population. Ecol. Monogr. 78, 141–166. doi: 10.1890/06-1708.1
Hegazy, A. K., Kabiel, H. F., Hosni, H. A., Badawi, E. S. M., and Lovett-Doust, L. (2018). Spatial and temporal variation in plant community phenology in the Jebel Marra region of Darfur. Folia Geobotan. 53, 389–403. doi: 10.1007/s12224-018-9333-4
Hobbs, N. T., Galvin, K. A., Stokes, C. J., Lackett, J. M., Ash, A. J., Boone, R. B., et al. (2008). Fragmentation of rangelands: implications for humans, animals, and landscapes. Glob. Environ. Change 18, 776–785. doi: 10.1016/j.gloenvcha.2008.07.011
Huete, A., Didan, K., Miura, T., Rodriguez, E. P., Gao, X., and Ferreira, L. G. (2002). Overview of the radiometric and biophysical performance of the MODIS vegetation indices. Remote Sens. Environ. 83, 195–213. doi: 10.1016/S0034-4257(02)00096-2
Hussein, M. A. (2011). “Climate change impacts on East Africa,” in Climate Change Management, ed. Filho L. W. (Berlin, Heidelberg: Springer), 589–601.
Ibrahim, S., Balzter, H., Tansey, K., and Tsutsumida, N. (2018). Estimating fractional cover of plant functional types in African savannah from harmonic analysis of MODIS time-series data. Int. J. Remote Sens. 39, 2718–2745. doi: 10.1080/01431161.2018.1430914
Isbell, F., Calcagno, V., Hector, A., Connolly, J., Harpole, W. S., Reich, P. B., et al. (2011). High plant diversity is needed to maintain ecosystem services. Nature 477, 199–202. doi: 10.1038/nature10282
Jabs, L. B. (2005). Collectivism and conflict: conflict response styles in Karamoja, Uganda. Int. J. Conflict Manage. 16, 354–378.
Jönsson, P., and Eklundh, L. (2004). TIMESAT - A program for analyzing time-series of satellite sensor data. Comput. Geosci. 30, 833–845. doi: 10.1016/j.cageo.2004.05.006
Kang, W., Wang, T., and Liu, S. (2018). The response of vegetation phenology and productivity to drought in semi-arid regions of Northern China. Remote Sens. 10:727. doi: 10.3390/rs10050727
Kimaro, E. G., Mor, S. M., and Toribio, J. A. L. (2018). Climate change perception and impacts on cattle production in pastoral communities of northern Tanzania. Pastoralism 8:19. doi: 10.1186/s13570-018-0125-5
Kimiti, K. S., Western, D., Mbau, J. S., and Wasonga, O. V. (2018). Impacts of long-term land-use changes on herd size and mobility among pastoral households in Amboseli ecosystem, Kenya. Ecol. Process. 7:4. doi: 10.1186/s13717-018-0115-y
Knighton, B. (1990). Christian Enculturation in Karamoja, Nirobi. Unpublished Ph.D. thesis. Durham: Durham University.
Krätli, S. (2010). Karamoja with the rest of “the rest of Uganda.” Nomadic Peoples 14, 3–23. doi: 10.3167/np.2010.140202
Liang, L., and Schwartz, M. D. (2009). Landscape phenology: an integrative approach to seasonal vegetation dynamics. Landsc. Ecol. 24, 465–472. doi: 10.1007/s10980-009-9328-x
Lillesand, T. M., and Kiefer, R. W. (1979). Remote sensing and image interpretation. Remote Sens. Image Interpret. 146, 448–449. doi: 10.2307/634969
Lillesand, T. M., Kiefer, R. W., and Chipman, J. W. (2004). Remote Sensing and Image Interpretation. New York Chichester Brisbane Toronto 6IS s, New York, NY: John Wiley and Sons.
Little, P. D. (1996). Pastoralism, biodiversity, and the shaping of savanna landscapes in East Africa. Africa 66, 37–51. doi: 10.2307/1161510
Lloyd, D. (1990). A phenological classification of terrestrial vegetation cover using shortwave vegetation index imagery. Int. J. Remote Sens. 11, 2269–2279. doi: 10.1080/01431169008955174
Lyamchai, C. J., Gillespie, T. J., and Brown, D. M. (1997). Estimating maize yield in northern Tanzania by adapting SIMCOY, a temperate-zone simulation model. Agricul. Forest Meteorol. 85, 75–86. doi: 10.1016/S0168-1923(96)02367-2
Ma, A., Bohan, D. A., Canard, E., Derocles, S. A., Gray, C., Lu, X., et al. (2018). “A replicated network approach to ‘big data'in ecology,” in Advances in Ecological Research, eds. Bohan, D. A., Dumbrell, A. J., Woodward, G., and Jackson, M. Vol. 59, 225–264. doi: 10.1016/bs.aecr.2018.04.001
Maestre, F. T., Quero, J. L., Gotelli, N. J., Escudero, A., Ochoa, V., Delgado-Baquerizo, M., et al. (2012). Plant species richness and ecosystem multifunctionality in global drylands. Science. 335, 214–8. doi: 10.1126/science.1215442
Mubiru, D. N., Komutunga, E., Agona, A., Apok, A., and Ngara, T. (2012). Characterising agrometeorological climate risks and uncertainties: crop production in Uganda. South African J. Sci. 108, 108–18. doi: 10.4102/sajs.v108i3/4.470
Mubiru, D. N., Radeny, M., Kyazze, F. B., Zziwa, A., Lwasa, J., Kinyangi, J., et al. (2018). Climate trends, risks and coping strategies in smallholder farming systems in Uganda. Clim. Risk Manage. 22, 4–21. doi: 10.1016/j.crm.2018.08.004
Myoung, B., Choi, Y. S., Hong, S., and Park, S. K. (2013). Inter-and intra-annual variability of vegetation in the northern hemisphere and its association with precursory meteorological factors. Glob. Biogeochem. Cycles 27, 31–42. doi: 10.1002/gbc.20017
Naeem, S., Bunker, D. E., Hector, A., Loreau, M., and Perrings, C. (2009). Biodiversity, Ecosystem Functioning, and Human Wellbeing: An Ecological and Economic Perspective, Oxford: Oxford University Press.
Nakalembe, C. (2018). Characterizing agricultural drought in the Karamoja subregion of Uganda with meteorological and satellite-based indices. Nat. Hazards 91, 837–862. doi: 10.1007/s11069-017-3106-x
Nampala, M. (2013). Camels, Pokot's gift to Uganda. The New Vision. Available online at: https://www.newvision.co.ug/news/1336123/camels-pokot-gift-uganda (accessed October 18, 2020).
Nsubuga, F. W., and Rautenbach, H. (2018). Climate change and variability: a review of what is known and ought to be known for Uganda. Int. J. Clim. Change Strat. Manage. 10, 752–771. doi: 10.1108/IJCCSM-04-2017-0090
Ocan, C. E., and Ocan, C. (1994). Pastoral resources and conflicts in north-eastern Uganda: the Karimojong case. Nomadic peoples 34/35, 123–135.
OCHA (2010). Uganda: Karamoja Sub-Region - Dry Season Migration Routes & Kraal Locations. Available online at: https://reliefweb.int/map/uganda/uganda-karamoja-sub-region-dry-season-migration-routes-kraal-locations-23-mar-2010 (accessed October 18, 2020).
Opiyo, F., Wasonga, O., Nyangito, M., Schilling, J., and Munang, R. (2015). Drought adaptation and coping strategies among the Turkana pastoralists of northern Kenya. Int. J. Disas. Risk Sci. 6, 295–309. doi: 10.1007/s13753-015-0063-4
Partanen, J., Koski, V., and Hänninen, H. (1998). Effects of photoperiod and temperature on the timing of bud burst in Norway spruce (Picea abies). Tree Physiol. 18, 811–816. doi: 10.1093/treephys/18.12.811
Pau, S., and Still, C. J. (2014). Phenology and productivity of C3 and C4 Grassland in Hawaii. PLoS ONE 9:e107396. doi: 10.1371/journal.pone.0107396
Peñuelas, J., Sardans, J., Estiarte, M., Ogaya, R., Carnicer, J., Coll, M., et al. (2013). Evidence of current impact of climate change on life: a walk from genes to the biosphere. Glob. Change Biol. 19, 2303–2338. doi: 10.1111/gcb.12143
Piao, S., Liu, Q., Chen, A., Janssens, I. A., Fu, Y., Dai, J., et al. (2019). Plant phenology and global climate change: current progresses and challenges. Glob. Change Biol. 25, 1922–1940. doi: 10.1111/gcb.14619
Primack, R. B., and Gallinat, A. S. (2017). Insights into grass phenology from herbarium specimens. New Phytol. 213, 1567–1568. doi: 10.1111/nph.14439
Puppi, G. (2007). Origin and development of phenology as a science. Ital. J. Agrometeorol. 3, 24–29.
Rather, R. N., Wani, A. A., Kashtwari, M., and Beigh, Z. A. (2018). Climate change phenological shifts due to climate change and. Clim. Change 4, 80–86.
Reed, B. C., Brown, J. F., VanderZee, D., Loveland, T. R., Merchant, J. W., and Ohlen, D. O. (1994). Measuring phenological variability from satellite imagery. J. Veg. Sci. 5, 703–714. doi: 10.2307/3235884
Reich, P. B. (1995). Phenology of tropical forests: patterns, causes, and consequences. Can. J. Bot. 73, 164–174. doi: 10.1139/b95-020
Richter, C., Rejmánek, M., Miller, J. E., Welch, K. R., Weeks, J., and Safford, H. (2019). The species diversity × fire severity relationship is hump-shaped in semiarid yellow pine and mixed conifer forests. Ecosphere 10, 1–16. doi: 10.1002/ecs2.2882
Scoones, I. (1995). Exploiting heterogeneity: habitat use by cattle in dryland Zimbabwe. J. Arid Environ. 29, 221–237. doi: 10.1016/S0140-1963(05)80092-8
Simms, E. L. (2013). “Plant-animal interactions,” in Encyclopedia of Biodiversity, 2nd Edn. (Elsevier). p.39–55. doi: 10.1016/B978-0-12-384719-5.00109-X
Singh, J., Levick, S. R., Guderle, M., Schmullius, C., and Trumbore, S. E. (2018). Variability in fire-induced change to vegetation physiognomy and biomass in semi-arid savanna. Ecosphere 9:e02514. doi: 10.1002/ecs2.2514
Snyman, H. A. (2004). Short-term response in productivity following an unplanned fire in a semi-arid rangeland of South Africa. J. Arid Environ. 56, 465–485. doi: 10.1016/S0140-1963(03)00069-7
Song, C., You, S., Ke, L., Liu, G., and Zhong, X. (2011). Spatio-temporal variation of vegetation phenology in the Northern Tibetan Plateau as detected by MODIS remote sensing. Chin. J. Plant Ecol. 35, 853–863. doi: 10.3724/SP.J.1258.2011.00853
Stocker, T. F. D., Qin, G.-K., Plattner, M. T., Allen, S. K., Boschung, J., et al. (2013). “IPCC, 2013: summary for policymakers,” in Climate Change 2013: The Physical Science Basis. Contribution of Working Group I to the Fifth Assessment Report of the IPCC. Cambridge University Press.
Suonan, J., Classen, A. T., Sanders, N. J., and He, J. S. (2019). Plant phenological sensitivity to climate change on the Tibetan Plateau and relative to other areas of the world. Ecosphere 10, e02543. doi: 10.1002/ecs2.2543
Tao, Z., Wang, H., Liu, Y., Xu, Y., and Dai, J. (2017). Phenological response of different vegetation types to temperature and precipitation variations in northern China during 1982–2012. Int. J. Remote Sens. 38, 3236–3252. doi: 10.1080/01431161.2017.1292070
Tokura, W., Jack, S. L., Anderson, T., and Hoffman, M. T. (2018). Long-term variability in vegetation productivity in relation to rainfall, herbivory and fire in Tswalu Kalahari Reserve. Koedoe 60, 1–18. doi: 10.4102/koedoe.v60i1.1473
Tottrup, C., and Rasmussen, M. S. (2004). Mapping long-term changes in savannah crop productivity in Senegal through trend analysis of time series of remote sensing data. Agricul. Ecosyst. Environ. 103, 545–560. doi: 10.1016/j.agee.2003.11.009
Turner, M. D., and Schlecht, E. (2019). Livestock mobility in sub-Saharan Africa: a critical review. Pastoralism 9:13. doi: 10.1186/s13570-019-0150-z
USGS (2011). USGS Science for a Changing World, Conterminous U.S. 1 km AVHRR Remote Sensing Phenology Data. Available online at: https://www.usgs.gov/centers/eros/science/remote-sensing-phenology?qt-science_center_objects=0#qt-science_center_objects (accessed October 18, 2020).
Van Leeuwen, W. J., Davison, J. E., Casady, G. M., and Marsh, S. E. (2010). Phenological characterization of desert sky island vegetation communities with remotely sensed and climate time series data. Remote Sens. 2, 388–415. doi: 10.3390/rs2020388
Visser, M. E., and Both, C. (2005). Shifts in phenology due to global climate change: the need for a yardstick. Proc. R. Soc. B Biol. Sci. 272, 2561–2569. doi: 10.1098/rspb.2005.3356
Vrieling, A., De Leeuw, J., and Said, M. Y. (2013). Length of growing period over Africa: variability and trends from 30 years of NDVI time series. Remote Sens. 5, 982–1000. doi: 10.3390/rs5020982
Vrieling, A., Meroni, M., Mude, A. G., Chantarat, S., Ummenhofer, C. C., and de Bie, K. C. (2016). Early assessment of seasonal forage availability for mitigating the impact of drought on East African pastoralists. Remote Sens. Environ. 174, 44–55. doi: 10.1016/j.rse.2015.12.003
White, M. A., Thornton, P. E., and Running, S. W. (1997). A continental phenology model for monitoring vegetation responses to interannual climatic variability. Glob. Biogeochem. Cycles 11, 217–234. doi: 10.1029/97GB00330
Zhang, J., Chang, Q., and Yao, F. (2015). Grassland phenology in different eco-geographic regions over the Tibetan Plateau. Int. J. Environmen. Ecol. Eng, 9, 1301–1306. doi: 10.5281/zenodo.1108364
Zhang, X., Friedl, M. A., Schaaf, C. B., Strahler, A. H., and Liu, Z. (2005). Monitoring the response of vegetation phenology to precipitation in Africa by coupling MODIS and TRMM instruments. J. Geophys. Res. Atmos. 110, 1–14. doi: 10.1029/2004JD005263
Keywords: conflict, drylands, grazing, mobility, pastoral
Citation: Egeru A, Magaya JP, Kuule DA, Siya A, Gidudu A, Barasa B and Namaalwa JJ (2020) Savannah Phenological Dynamics Reveal Spatio-Temporal Landscape Heterogeneity in Karamoja Sub-region, Uganda. Front. Sustain. Food Syst. 4:541170. doi: 10.3389/fsufs.2020.541170
Received: 07 March 2020; Accepted: 16 November 2020;
Published: 08 December 2020.
Edited by:
Carol Kerven, University College London, United KingdomReviewed by:
Joseph Sang, Jomo Kenyatta University of Agriculture and Technology, KenyaTimm Hoffman, University of Cape Town, South Africa
Copyright © 2020 Egeru, Magaya, Kuule, Siya, Gidudu, Barasa and Namaalwa. This is an open-access article distributed under the terms of the Creative Commons Attribution License (CC BY). The use, distribution or reproduction in other forums is permitted, provided the original author(s) and the copyright owner(s) are credited and that the original publication in this journal is cited, in accordance with accepted academic practice. No use, distribution or reproduction is permitted which does not comply with these terms.
*Correspondence: Anthony Egeru, egeru81@gmail.com